User Identification Based on the Analysis of the Forces Applied by a User to a
基于大数据的用户行为分析与预测研究(英文中文双语版优质文档)

基于大数据的用户行为分析与预测研究(英文中文双语版优质文档)I. IntroductionWith the development of Internet technology, people are increasingly inseparable from the Internet, and more and more information is recorded, which is called big data. The mining and analysis of big data is crucial to the decision-making and development of enterprises, and user behavior data is a very important part of it. User behavior data refers to various data generated by users when using products or services, including user access records, click records, purchase records, etc. These data reflect users' needs, interests, and behavioral habits for products or services. Said that these data can be used for user behavior analysis and prediction.2. User Behavior AnalysisUser behavior analysis is to obtain the rules and trends of user behavior through statistics, analysis and mining of user behavior data, so as to provide a basis for enterprise decision-making. User behavior analysis mainly includes the following aspects:1. User interest analysisUser interest analysis refers to the analysis of user behavior data to understand the user's points of interest, so as to recommend personalized products or services for enterprises. Specifically, by analyzing the user's search records, purchase records, browsing records, etc., the user's points of interest and preferences can be obtained, and products or services that better meet the user's needs can be recommended for enterprises.2. User behavior path analysisUser behavior path analysis refers to the analysis of user behavior data to understand the behavior path of users when using products or services, so as to provide better products or services for enterprises. Specifically, by analyzing the user's click records, browsing records, and purchase records in products or services, we can understand the user's behavior path and provide a basis for companies to improve products or services.3. User churn analysisUser churn analysis refers to the analysis of user behavior data to understand the reasons for user churn when using products or services, so as to provide enterprises with directions for improvement. Specifically, through the analysis of users' usage records, access records, evaluation records, etc. in products or services, we can understand the reasons for user loss and provide companies with improvement plans.3. User Behavior PredictionUser behavior prediction refers to the analysis and mining of user behavior data to obtain the future behavior trend of users, so as to provide decision-making basis for enterprises. User behavior prediction mainly includes the following aspects:1. User purchase predictionUser purchase prediction refers to the analysis and mining of behavior data such as user purchase records and browsing records to obtain the user's future purchase trends, so as to formulate better marketing strategies for enterprises. Specifically, by analyzing users' browsing records, click records, purchase records, etc. in products or services, we can understand users' purchasing preferences, purchasing power, purchasing cycle, etc., and provide personalized recommendations and marketing solutions for enterprises.2. User Churn PredictionUser churn prediction refers to the analysis and mining of user behavior data to obtain the trend and reasons for possible user loss in the future, so as to provide preventive measures for enterprises. Specifically, by analyzing the user's usage records, access records, evaluation records, etc. in products or services, we can understand the user's usage habits, satisfaction, etc., and provide personalized services and improvement plans for enterprises.3. User Conversion PredictionUser conversion prediction refers to the analysis and mining of user behavior data to obtain possible conversion trends of users in the future, so as to provide better conversion strategies for enterprises. Specifically, by analyzing users' usage records, browsing records, and click records in products or services, we can understand users' points of interest, conversion intentions, etc., and provide personalized conversion recommendations and services for enterprises.4. Application of Big Data Technology in User Behavior Analysis and PredictionBig data technology is the key to user behavior analysis and prediction. Big data technology can obtain the rules and trends of user behavior through the rapid processing and analysis of massive data, and improve the accuracy and efficiency of user behavior analysis and prediction. Big data technology mainly includes the following aspects:1. Data collectionData acquisition is the first step in big data analysis. Data collection needs to collect various behavioral data generated by users when using products or services, including user access records, click records, purchase records, etc. Data collection can be achieved through various channels, including websites, apps, social media, etc.2. Data storageData storage is an important part of big data analysis. Big data analysis needs to process massive amounts of data, so it needs to store massive amounts of data. Data storage can be implemented in various ways, including relational databases, NoSQL databases, distributed file systems, etc.3. Data cleaningData cleaning is a necessary step in big data analysis. Data cleaning requires deduplication, screening, conversion and other processing of the collected data to ensure the quality and accuracy of the data. Data cleaning can be achieved using various techniques, including ETL tools, data mining tools, etc.4. Data analysisData analysis is the core of big data technology. Data analysis requires statistics, analysis, mining and other processing of the collected data, so as to obtain the rules and trends of user behavior. Data analysis can be achieved using various techniques, including data mining, machine learning, deep learning, etc.5. Visual AnalysisVisual analysis is an important means of big data analysis. Visual analysis can present the analysis results in the form of charts, graphs, etc., and intuitively display the results of data analysis. Visual analysis can be implemented using various tools, including Tableau, Power BI, etc.6. Model buildingModel building is an important part of big data analysis. Model building needs to establish a model based on the collected data and analysis results, verify and optimize it, so as to obtain the prediction results of user behavior. Model building can be achieved using various techniques, including regression analysis, decision trees, neural networks, etc.In short, big data technology has played an important role in user behavior analysis and prediction, providing enterprises with more accurate and finer data analysis and prediction capabilities, thereby helping enterprises better understand user needs, optimize services, improve user experience and Market Competitiveness.一、引言随着互联网技术的发展,人们越来越离不开网络,越来越多的信息被记录下来,这些数据被称为大数据。
互联网时代下信息真伪辨别的重要性英语作文范文

互联网时代下信息真伪辨别的重要性英语作文范文Title: The Importance of Discerning Information Authenticity in the Internet AgeIntroduction:The rapid development of the internet has brought about unprecedented convenience in accessing information. However, it has also introduced significant challenges in distinguishing between authentic and deceptive information. This essay explores the crucial role of discerning information authenticity in the digital era and provides insights into effective strategies for individuals to navigate the vast online landscape.Body:1. The proliferation of misinformation:a. The ease of publishing and sharing information online.b. The lack of stringent fact-checking procedures.c. The spread of misinformation through social media platforms.2. The consequences of misinformation:a. Influencing public opinion and perception.b. Undermining credibility and trust in institutions.c. Potentially leading to adverse actions and behaviors.3. The importance of information verification:a. Preserving the accuracy and reliability of knowledge.b. Making informed decisions based on verified information.c. Safeguarding personal and collective well-being.4. Strategies for discerning information authenticity:a. Assessing the credibility of sources:i. Verifying author credentials and expertise.ii. Evaluating the reputation and reliability of the publishing platform.iii. Cross-referencing information with multiple credible sources.b. Analyzing the content:i. Scrutinizing the use of logical fallacies or emotional manipulation.ii. Identifying bias and agendas.iii. Fact-checking claims and statistical data through reputable fact-checking organizations.c. Utilizing critical thinking:i. Questioning the veracity and motives behind the information.ii. Considering alternative viewpoints and counterarguments.iii. Relying on evidence-based reasoning.5. The role of technology and media literacy:a. Promoting digital literacy education:i. Educating individuals about information verification techniques.ii. Teaching critical thinking skills in the digital landscape.iii. Encouraging media literacy to recognize misinformation patterns.b. Leveraging technology for fact-checking:i. Utilizing automated tools for identifying false information.ii. Supporting initiatives for AI-driven detection offake news.iii. Encouraging social media platforms to emphasize accurate information sharing.Conclusion:In the internet era, the ability to discern information authenticity is crucial to maintaining an informed societyand avoiding the detrimental consequences of misinformation. By implementing strategies like source credibility assessment, content analysis, and critical thinking, individuals can navigate the vast online landscape more effectively. Furthermore, technology and media literacy play a vital rolein promoting information verification and combating thespread of fake news. By collectively prioritizing the importance of information authenticity, we can safeguard the integrity of knowledge and make well-informed decisions inthe digital age.。
信息安全身份认证策略.ppt
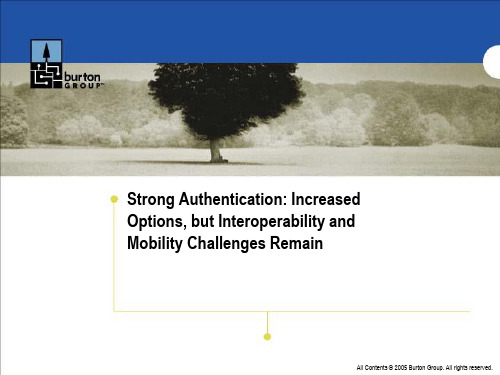
• Knowledge-based authentication (KBA) alone is not sufficient identity proofing
• Lot of buzz in recent years, but not many deployments • Requires network access • Not as secure • Typically requires two phase authentication (with a wait in between)
• Organizations are increasingly deploying smart cards, due to their security and application coverage, including physical access and OTP
• Biometrics have their rightful place in the strong authentication landscape
Strong Authentication: Increased Options, but Interoperability and Mobility Challenges Remain
All Contents © 2005 Burton Group. All rights reserved.
Strong Authentication
• RSA SecurID is not a participant (70% market share)
CV Samples
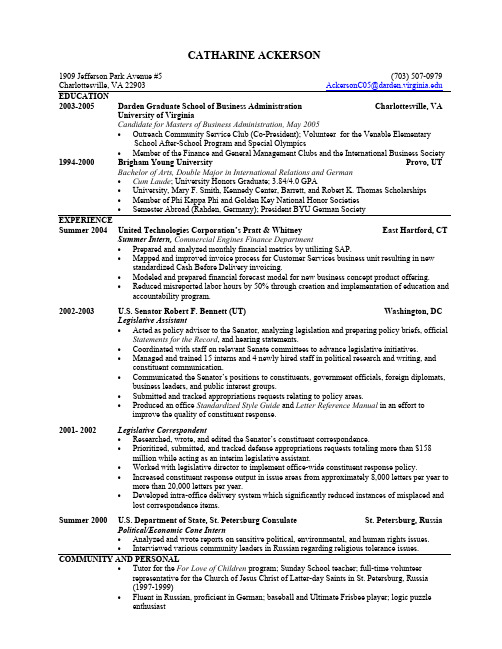
ANDREW M. ADAMS
153 Spring Mtn. Rd. • Charlottesville, VA • 22902 • (732) 267.3408 • AdamsA05@ EDUCATION Darden Graduate School of Business Administration – University of Virginia Charlottesville, VA Candidate for Masters of Business Administration, May 2005 • Elected to Student Admissions Committee; Selected to co-lead the Alumni Showcase Board • Member of the Consulting Club, Darden Private Equity Network, and Latin American Student Association • GMAT – 720 University of Virginia Charlottesville, VA Bachelor of Arts, Economics, Minor in Astronomy, May 1999 • Semester Abroad (U.K.): University of Lancaster, Management School, Spring 1998 • Financed educational costs by starting and managing a commercial landscaping firm (1994 – 1999) EXPERIENCE Monitor Group (2004) Cambridge, MA Monitor Group is a leading global strategy consulting firm with 29 offices worldwide. Summer Associate Serving as an integral member of the case team, helped a leading international beverage company develop a global marketing platform based on market research analysis and market segmentation techniques • Designed market research instrument and managed selection of market research vendor; presented both to client • Analyzed market research results to help define actionable market segments and understand these segments’ behaviors • Presented case summary and key analytical findings to summer classmates and senior partners Dayton, NJ Sunshine Bouquet Co. (1999 – 2003) An international grower and distributor of fresh- cut flowers, Sunshine Bouquet Co. primarily serves grocery stores throughout the eastern United States and generated revenues of $65 million in 2004. International Operations Management (2002 – 2003) Bogotá, Colombia Promoted to Operations Manager for firm’s most critical production facility; led a five person management team and production workforce of over 200 people • Markedly improved product design, inventory management, production processes and management capability, transforming the Bogotá plant into the firm’s principal production facility • Doubled production capacity within one year by adding over 100 line positions, numerous supervisors and several managers. Expansion reduced U.S. operating costs by over $1.2 million Venture Development (2001) Princeton, NJ Assumed turn-around responsibility for a failing subsidiary (start-up retail venture) • Grew revenues 40% within six months by improving sales development, supply operations, product quality, merchandising and staff (replaced numerous employees and hired two salaried managers) • Enhanced the venture’s brand strength through new marketing campaign (e.g. direct mail, events, in-store activities) • Designed and managed a just-in-time procurement and production process to distribute internationally sourced products to multiple retail locations Marketing & Sales Development (2000) Worked with Chief Operating Officer to implement key marketing initiatives • Created numerous sales proposals that helped reverse declining sales, resulting in a $6 million gain • Authored and designed firm’s new marketing materials and online presence () Dayton, NJ
个人信息安全评估指南英语
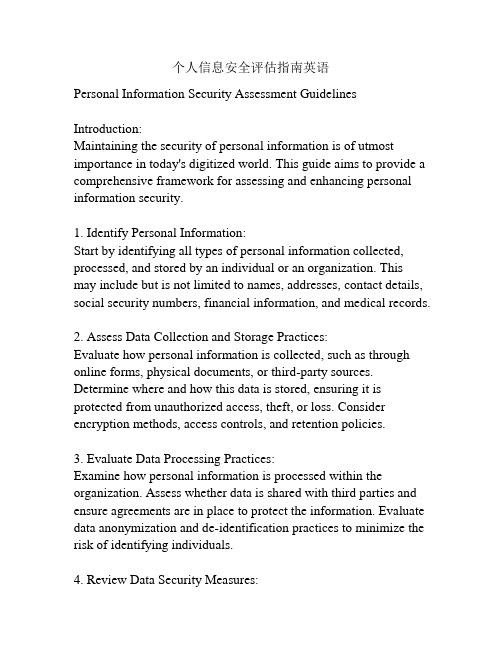
个人信息安全评估指南英语Personal Information Security Assessment Guidelines Introduction:Maintaining the security of personal information is of utmost importance in today's digitized world. This guide aims to provide a comprehensive framework for assessing and enhancing personal information security.1. Identify Personal Information:Start by identifying all types of personal information collected, processed, and stored by an individual or an organization. This may include but is not limited to names, addresses, contact details, social security numbers, financial information, and medical records.2. Assess Data Collection and Storage Practices:Evaluate how personal information is collected, such as through online forms, physical documents, or third-party sources. Determine where and how this data is stored, ensuring it is protected from unauthorized access, theft, or loss. Consider encryption methods, access controls, and retention policies.3. Evaluate Data Processing Practices:Examine how personal information is processed within the organization. Assess whether data is shared with third parties and ensure agreements are in place to protect the information. Evaluate data anonymization and de-identification practices to minimize the risk of identifying individuals.4. Review Data Security Measures:Assess the effectiveness of security measures in place to protect personal information. This includes analyzing firewalls, antivirus software, intrusion detection systems, and regular security audits. Review physical security measures for data centers, servers, and backup storage.5. Implement Access Controls:Ensure appropriate access controls are in place to limit access to personal information. This involves assigning user roles and permissions based on job responsibilities, implementing strong password requirements, and utilizing multi-factor authentication where possible.6. Train Employees:Educate employees on personal information security awareness and best practices. Regularly provide training sessions to raise awareness about phishing attacks, social engineering tactics, and the importance of protecting personal information.7. Develop Incident Response Plan:Create a detailed incident response plan that outlines steps to be taken in the event of a data breach or security incident. Assign responsibilities, establish communication channels, and create a framework for notifying affected individuals, regulatory bodies, and law enforcement if necessary.8. Conduct Regular Audits:Perform regular audits to ensure ongoing compliance with personal information security guidelines. Check for any vulnerabilities, review system logs, and assess the effectiveness of securitycontrols. This helps identify and address any potential weaknesses in data protection practices.9. Stay Up-to-Date with Legal and Regulatory Requirements: Regularly monitor and update personal information security practices to stay in compliance with relevant laws and regulations. Stay informed about new data protection policies and guidelines issued by industry-specific regulatory bodies.Conclusion:By following these guidelines, individuals and organizations can evaluate their personal information security measures, identify areas for improvement, and ensure the protection of sensitive data. Regular assessments and updates are necessary to stay proactive in the face of evolving security threats.。
User Authentication.pdf
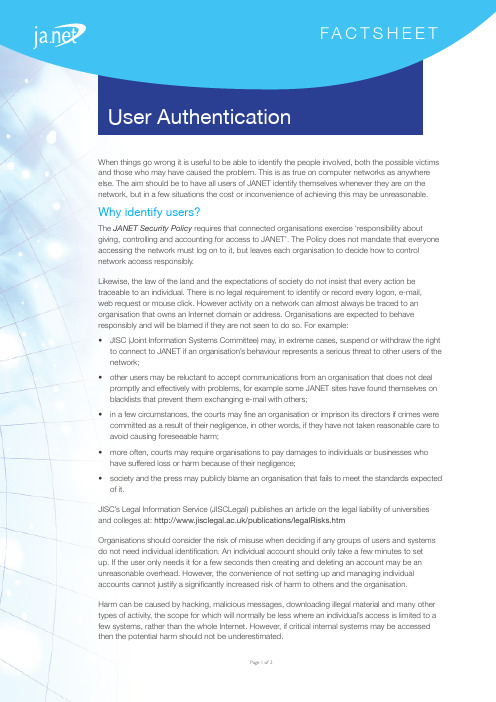
User Authentication
When things go wrong it is useful to be able to identify the people involved, both the possible victims and those who may have caused the problem. This is as true on computer networks as anywhere else. The aim should be to have all users of JANET identify themselves whenever they are on the network, but in a few situations the cost or inconvenience of achieving this may be unreasonable.
publications/technical-guides/logfiles.pdf
If usernames and passwords are used there are a number of ways to issue them. Staff and students of the organisation should have their own local accounts. Visitors may also have lbe enabled to set up daily accounts for their guests. Visitors from other organisations may be authenticated by their home organisation if both organisations are members of JANET Roaming or another partner in the TERENA (Trans-European Research and Education Network Association) eduroam federation. Even if individual identities are not checked, access to the JANET network must still be limited to those who are known to the organisation. Knowingly providing network access to strangers is likely to be a breach of JANET policies and to be considered irresponsible by other users of the network. Access may be limited by physical barriers, although this does not work for wireless networks, or by providing temporary access codes to guests such as conference delegates. Organisations may wish to arrange their networks so that these visitors do not accidentally obtain access to internal resources controlled or licensed by IP address. Organisations that provide access to networks, and users who benefit from that access, should regard it as normal to require an individual identity. Systems for establishing electronic identity are becoming easier to use and manage. In a few situations there may be a justification for not checking and recording identity but this should only be done after a rational assessment of the risks and benefits.
半导体术语
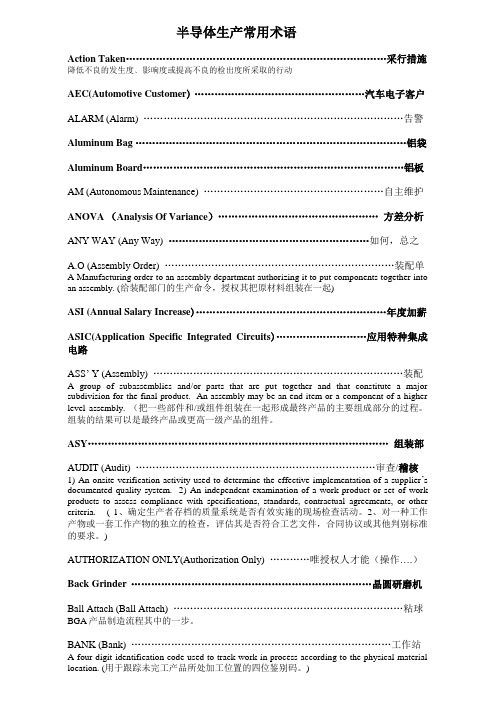
半导体生产常用术语Action Taken……………………………………………………………………采行措施降低不良的发生度﹑影响度或提高不良的检出度所采取的行动AEC(Automotive Customer)……………………………………………汽车电子客户ALARM (Alarm)……………………………………………………………………告警Aluminum Bag ………………………………………………………………………铝袋Aluminum Board……………………………………………………………………铝板AM (Autonomous Maintenance)………………………………………………自主维护ANOVA (Analysis Of Variance)…………………………………………方差分析ANY WAY (Any Way)……………………………………………………如何,总之A.O (Assembly Order)……………………………………………………………装配单A Manufacturing order to an assembly department authorizing it to put components together into an assembly. (给装配部门的生产命令,授权其把原材料组装在一起)ASI (Annual Salary Increase)…………………………………………………年度加薪ASIC(Application Specific Integrated Circuits)………………………应用特种集成电路ASS’ Y (Assembly)…………………………………………………………………装配A group of subassemblies and/or parts that are put together and that constitute a major subdivision for the final product. An assembly may be an end item or a component of a higher level assembly. (把一些部件和/或组件组装在一起形成最终产品的主要组成部分的过程。
简明分析方案英文
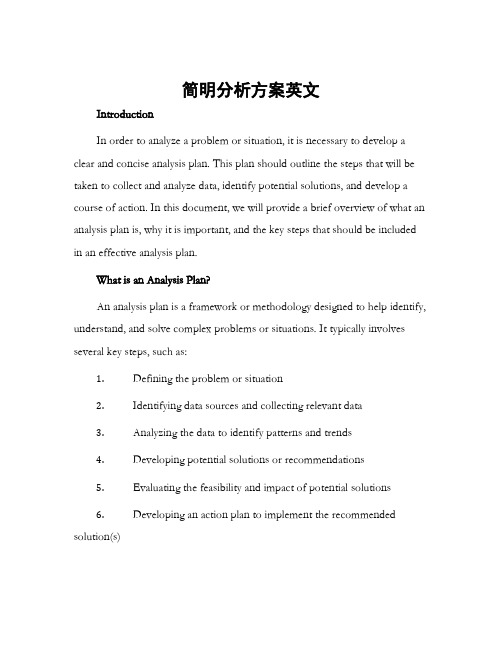
简明分析方案英文IntroductionIn order to analyze a problem or situation, it is necessary to develop a clear and concise analysis plan. This plan should outline the steps that will be taken to collect and analyze data, identify potential solutions, and develop a course of action. In this document, we will provide a brief overview of what an analysis plan is, why it is important, and the key steps that should be included in an effective analysis plan.What is an Analysis Plan?An analysis plan is a framework or methodology designed to help identify, understand, and solve complex problems or situations. It typically involves several key steps, such as:1.Defining the problem or situation2.Identifying data sources and collecting relevant data3.Analyzing the data to identify patterns and trends4.Developing potential solutions or recommendations5.Evaluating the feasibility and impact of potential solutions6.Developing an action plan to implement the recommended solution(s)Why is an Analysis Plan Important?An analysis plan is important for several reasons:1.It helps ensure that the problem or situation is clearly identified and defined2.It provides a structured approach to collecting and analyzing data, which helps ensure that data is accurate and complete3.It helps identify potential solutions or recommendations that are feasible and effective4.It provides a roadmap for implementing solutions and monitoring progressWithout an analysis plan, it can be difficult to identify and solve complex problems or situations. For example, if a company is experiencing declining sales, it may be tempting to simply try different marketing strategies without first understanding the underlying causes of the problem.Key Steps in Developing an Analysis PlanThe key steps in developing an effective analysis plan include:1.Defining the problem or situation: This involves clearly defining the problem or situation that needs to be addressed. It may be helpful to use a problem-solving framework, such as a fishbone diagram or SWOT analysis, to help identify potential causes and factors.2.Identifying data sources and collecting relevant data: This step involves identifying the data that will be needed to analyze the problem or situation. This may include internal data, such as sales data or customer feedback, or external data, such as industry reports or customer surveys.3.Analyzing the data to identify patterns and trends: This step involves analyzing the data to identify patterns or trends that may be contributing to the problem or situation. Various statistical tools and software applications may be used to help with this step.4.Developing potential solutions or recommendations: Based on the data analysis, potential solutions or recommendations can be developed. It is important to develop solutions that are feasible and effective, and that address the underlying causes of the problem or situation.5.Evaluating the feasibility and impact of potential solutions: This step involves evaluating the potential solutions or recommendations to determine their feasibility and impact. Factors such as cost, resources, and time frame should be considered when evaluating solutions.6.Developing an action plan to implement the recommended solution(s): Finally, an action plan should be developed to implement the recommended solution(s). This may include a timeline, specific tasks and responsibilities, and a plan for monitoring progress and evaluating results.ConclusionDeveloping an analysis plan is a critical step in solving complex problems or situations. By following a structured analysis plan, it is possible to identify potential solutions that are feasible and effective, and to develop a roadmap for implementing those solutions. With a clear and concise analysis plan in place, organizations are better able to address the challenges they face and achieve their goals and objectives.。
- 1、下载文档前请自行甄别文档内容的完整性,平台不提供额外的编辑、内容补充、找答案等附加服务。
- 2、"仅部分预览"的文档,不可在线预览部分如存在完整性等问题,可反馈申请退款(可完整预览的文档不适用该条件!)。
- 3、如文档侵犯您的权益,请联系客服反馈,我们会尽快为您处理(人工客服工作时间:9:00-18:30)。
User Identification Based on the Analysis of the Forces Applied by a User to a Computer MouseCurtis S. Ikehara and Martha E. CrosbyUniversity of Hawai'i at ManoaDepartment of Information and Computer Sciencescikehara@, crosby@AbstractThis paper describes the framework for a branch of augmented cognition research performed at the Adaptive Multimodal Laboratory at the University of Hawai’i and a specific application involving the identification of a computer user based on the forces applied to a computer mouse (i.e., click signature) during a task. Data was collected from six people during a pilot study. Two methods used to identify users were a back propagation neural network and discriminant analysis. Results indicate that the discriminant analysis was slightly better at identifying users than the neural network, but it’s primary advantage was that it required less data preparation. Continuous identification of the user is possible with either method. Successful, identification of the user is a useful first step to proceed to the next stage of the research framework, which is to identify the user’s cognitive state for implementation in an augmented cognition system.1. IntroductionThis paper is divided into two parts. The first section describes the framework of the augmented cognition research performed at the Adaptive Multimodal Laboratory at the University of Hawai’i. The second section involves an initial step toward implementation of augmented cognition using the forces applied to a computer mouse. This involves a pilot study where data collected during a task from a force sensitive computer mouse is evaluated using two different methods.1.1 FrameworkThe objective of augmented cognition research is to identify user attributes that automatically prompt modifications of information presented to the user to enhance task performance. One of many implementations of augmented cognition would be to automatically identify the level of user attention to a task and to modify the rate of information being presented to optimize task completion. For example, when we read text, we naturally speed through those sections that are not difficult and less relevant to the task at hand, while slowing down at sections where comprehension of the information is critical to the task.Unless all the information needed for a task is presented on a visual display at once, this natural visual scanning ability must be combined with a method to present the missing information. Presentation of information at a fixed rate is the least desirable since information necessary to a task may be missed because the rate of presentation is too rapid to be understood. Also, presenting information too slow for the user creates an excessive load on the user’s memory when a large number of items must be remembered for a long period of time [1]. A better method of information presentation would be at the control of the user, but this method would require constant rate adjustments which would interfere with optimum performance. The best method would be for the information to be presented at a rate optimal for the task at hand without the user constantly being taken away from the task to make information presentation rate adjustments. To make the best option possible, the choice of user attribute which would control the rate of information presentation must be passively acquired so as to not impact on taskFigure 2. Attributes of several people Figure 1. Attributes Figure 3. Measuring attributesFigure 4. Individual attribute statesperformance. The assessment method must continuouslyidentify those attributes of the user that can direct the automatic change of the rate of information presentation.This best method would be an example of augmented cognition.Figure 1 shows a Venn diagram of attributes common to all people and attributes specific to the individual.The attributes of interest are those which can be obtained passively without interfering with the task performance of the individual. As the diagram shows, there is an expected overlap between all people and the individual.Figure 2 shows the attributes of three different people.Note that although each person has some common attributes, each person also has a slightly different set of distinguishing attributes. When multiple measurements are taken, either from a single sensor, multiple sensors or multiple sensors measuring different attributes, as shown in Figure 3, it becomes possible to distinguishthese people from each other. Figure 4 shows that anindividual can also have attributes that vary depending on their cognitive state (e.g., alert, stressed, etc.). The individual cognitive state can then be used to augment cognition. In the example of the previous paragraph regarding the pace of information presentation, detection of stress or reduced alertness could be used to direct the computer to reduce the speed of information presentation.The problem of distinguishing between people (i.e.,Figure 3) and an individual’s cognitive states (i.e.,Figure 4) appear to be very similar, but there are significant differences. The set of attributes that easy differentiate people can be completely different from the set of attributes that will indicate a person’s different cognitive state. Also, given any specific passively observable attribute, differences between people are likely to be easier to detect than the different cognitive states of an individual. Yet, differentiating between people and a person’s different cognitive states follow the same methodology.1.2 Pilot StudyThe characteristics of the desired attribute to measure would be that it be passive, continuous, allow the identification of user’s cognitive state and identify the unique characteristics of the user. The passive types ofFigure 5. Mean click signatures of subjects.measure that have been used are: eye position tracking (i.e., gaze) [2], pupil dilation [3], galvanic skin conductivity, heart rate and peripheral body temperature [4]. The attribute measured in this paper is force applied to computer mouse button while clicking responses to a task (See Figure 5). When a user performs a task requiring a computer mouse, the force applied to the mouse button is constantly measured. The authors noticed that each person appeared to have a unique pattern. Also, there is precedence for using pressure or force measurements on a tool as an indication of a person’s cognitive state since increased pressure while writing has been associated with increased stress [5].The objective of a pilot study was to evaluate two potential methods of using the forces applied to the computer mouse button (i.e., click signature) during a task to identify a user. Two methods of identification are compared: back propagation neural network and discriminant analysis.2. Method2.1 SubjectsSix university graduate student and faculty participated in this pilot study.Figure 7. Image viewed by the subject when square #0 is the target to be clicked on.2.2 Apparatus A computer mouse modified to measure the force applied to the buttons was used in conjunction with a custom designed data acquisition board which transmitted the data to the computer. This mouse from all outwards appearance looked exactly like a standard computer mouse. All data was recorded by the computer the user was performing the task on.2.3 Task Description Seven sets of tasks were presented. The task was to click each square as it appears. There are seven sets of tasks with 16 squares to each set. The position of the squares displayed are shown in Figure 6.1.Set 1 presents the square targets in sequentially order.This task was that fastest and easiest for the subject.2.Set 2 presents the square targets in a psuedo-random sequence. The psuedo-random sequence was constructed to eliminate sequences of two or three adjacent squared being presented and required the subject to move the computer mouse approximately the same total distance as the subsequent psuedo-random sequences of subsequent sets. This sequence increased the amount of attention required to perform the task which increases the task completion time. 3.Set 3 presents small square targets in a psuedo-random sequence. Psuedo-randomization and smallersquare targets increase the required attention and difficulty.4.Set 4 presents low contrast square targets in a psuedo-random sequence. This method is a different way to increases the attention and difficulty of the task.5.Set 5 presents small low contrast square targets in a psuedo-random sequence. This method compoundsall previous methods of increasing the attentionrequired and the difficulty.6.Set 6 presents the square targets in a regular sequenceof three square targets (i.e., second square left 2, third square right 1). This set is used for as part of another experiment looking for implicit learning. 7.Set 7 presents the square targets as in set 2, butpresented in a different psuedo-random order. Theseventh set is used as a comparison standard to Set 2to determine the change in performance over theexperiment.Figure 7 shows the image presented to the subject.There is a single square which the subject must clickbefore proceeding to click the next square.2.4 ProcedureSubjects were instructed to click the start button, thenboxes as they appeared on the screen. The subjects werewarned that boxes may become more difficult, but to dotheir best.2.5 Data AnalysisData from the experiment was analyzed using twodifferent methods to identify the subjects from their mouse click signatures. The first method ofidentification used a neural network with back-propagation training (see Figure 8) and the second method used a discriminant analysis of the mouse clicksignatures. For the neural network, the over 100 mouse clicks from each subject was broken into two mutually exclusive sets. The first set, called the training set, used the first 90 mouse clicks signatures of each subject to create a mean click signature for each of the six subjects (see Figure 2). Mean click signature of subjects were used since previous experiments using the actual data produced a neural network with poor results. The second set, called the testing set, consisted of the last ten mouse clicks of each subject.012345678910111213141516Figure 6. Position of squares displayed.Figure 8. Neural network used to identifysubjects from mouse click signatures.Figure 9. The neural network and discriminant analysis mean of the percent correct subject identification from the mouse click signature.The Neuroshell program, by Ward Systems Group was used for the analysis. The neural network parameters were adjusted and the network was trained using the mean click signatures of the subjects, then identification of the test set mouse clicks was performed. The data from the test set was used to determine the percent of correct identifications to subjects. Several runs were performed to determine the best way to present the data to the network and the optimum number of hidden layer nodes (i.e., five nodes).A discriminant analysis, using SPSS software by SPSS Inc., was performed using the first 100 mouse click signatures of the subjects. Using discriminant analysis, a cross validation identification of the subjects from mouse click signatures were performed. Cross validation uses discriminant analysis functions which excluded the data of the mouse click signature being identified.3. ResultsThe neural network was able identify the subject from a single mouse click signature an average of 68% of the time. Using the discriminant analysis method,identification of the subject from a single mouse click signature was an average of 79% of the time (see Figure 9).For the neural network method of identification, on average, three mouse clicks would be required to have an accuracy greater than 95%. For the discriminant analysis method of identification, on average, two mouse clicks would be required to have an accuracy greater than 95%.4. DiscussionThe neural network required several experiments to determine the optimum number of hidden nodes that would produce the optimal identification output. The discriminant analysis produces five functions used to identify the subjects and was slightly better at identifying subjects. The discriminant analysis method was less complex to implement. The discriminant analysis two-dimensional plot indicates that some subjects are very similar to each other while others are very different (see Figure 10), but when multiple dimensions are incorporated, discrimination is possible. A study of a large population of subjects is necessary, but the discriminant analysis method seems to be the simpler method of analysis.There are several methods that can be implemented to improve the reliability of user identification. First, aFigure 10. The two dimensional spatial relationship of the six subjects based on two of the five functions used to discriminate subjects.higher sampling rate of the force on the mouse will provide more information to differentiate subjects. Second, the use of multiple mouse clicks. Third, a combination of the force applied to a computer mouse with individual characteristic response latencies. Finally, a combination of the mouse click signature with other biometric information.In this pilot study, the mouse click signature is shown be able to indicate the identity of the subject. There is precedence for using pressure or force on a tool as an indication of a person’s cognitive state. Increased pressure while writing has been associated with increased stress [5], so forces applied to a computer mouse could be used as an indicator of stress. A procedure similar that used to identify subjects can be used to identify an individual’s different cognitive states. The methodology to determine user identification, demonstrated in this pilot study, is the initial step toward using forces on a computer mouse to identify the cognitive states of the user and subsequently implementing different modes of augmented cognition.5. References[1] C. D. Wickens, Engineering Psychology and Human Performance, 1984, pp. 138.[2] L. King, “The Relationship between Scene and Eye Movements”, Hawaii International Conference on System Science, Jan. 2002.[3] M. E. Crosby, C. Ikehara, D. N. Chin, “Measures of Real Time Assessment to use in Adaptive Augmentation”, Cognitive Science, in press 2002.[4] W. Ark, D. C. Dryer, Davia J. Lu, “The Emotion Mouse”, IBM Research Division, Almaden CA, 1999.[5] C. Lange-Küüttner, Perceptual and motor skills, 1998 vol. 86(3 Pt 2), pp. 1299-310.6. AcknowledgmentsThis research was supported in part by the Office of Naval Research grant no. N00014970578 and DARPA grant no. NBCH1020004.。