Structured
structuredchatagent用法
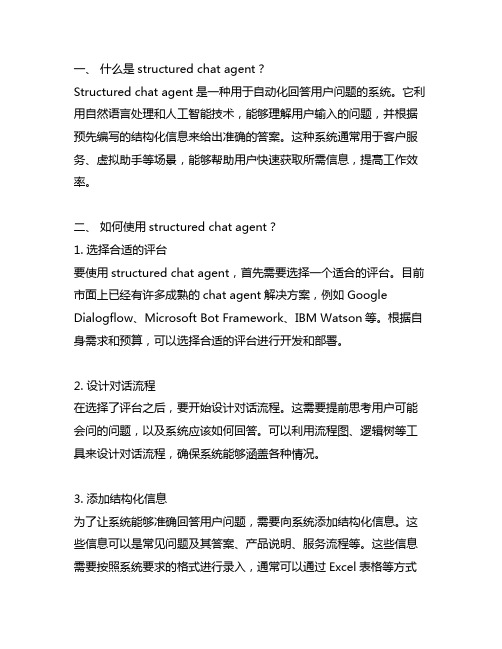
一、什么是structured chat agent?Structured chat agent是一种用于自动化回答用户问题的系统。
它利用自然语言处理和人工智能技术,能够理解用户输入的问题,并根据预先编写的结构化信息来给出准确的答案。
这种系统通常用于客户服务、虚拟助手等场景,能够帮助用户快速获取所需信息,提高工作效率。
二、如何使用structured chat agent?1. 选择合适的评台要使用structured chat agent,首先需要选择一个适合的评台。
目前市面上已经有许多成熟的chat agent解决方案,例如Google Dialogflow、Microsoft Bot Framework、IBM Watson等。
根据自身需求和预算,可以选择合适的评台进行开发和部署。
2. 设计对话流程在选择了评台之后,要开始设计对话流程。
这需要提前思考用户可能会问的问题,以及系统应该如何回答。
可以利用流程图、逻辑树等工具来设计对话流程,确保系统能够涵盖各种情况。
3. 添加结构化信息为了让系统能够准确回答用户问题,需要向系统添加结构化信息。
这些信息可以是常见问题及其答案、产品说明、服务流程等。
这些信息需要按照系统要求的格式进行录入,通常可以通过Excel表格等方式进行批量导入。
4. 集成到现有系统一旦设计好对话流程并添加了结构化信息,就可以将structured chat agent集成到现有系统中。
这通常需要开发人员进行编码工作,以便在全球信息站、App等评台上嵌入chat agent,使用户能够方便地访问和使用。
5. 测试和优化集成完成后,需要对structured chat agent进行测试和优化。
这包括测试系统对各种问题的回答准确性、用户体验等。
根据反馈和数据分析,不断优化系统,提高其智能化水平。
三、 structured chat agent的优势1. 提高效率由于structured chat agent能够快速准确地回答用户问题,能够大大提高工作效率。
structured abstract举例
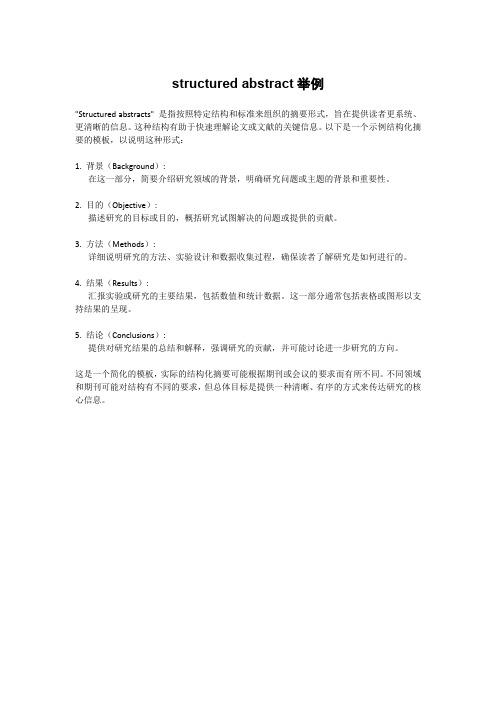
structured abstract举例
"Structured abstracts" 是指按照特定结构和标准来组织的摘要形式,旨在提供读者更系统、更清晰的信息。
这种结构有助于快速理解论文或文献的关键信息。
以下是一个示例结构化摘要的模板,以说明这种形式:
1. 背景(Background):
在这一部分,简要介绍研究领域的背景,明确研究问题或主题的背景和重要性。
2. 目的(Objective):
描述研究的目标或目的,概括研究试图解决的问题或提供的贡献。
3. 方法(Methods):
详细说明研究的方法、实验设计和数据收集过程,确保读者了解研究是如何进行的。
4. 结果(Results):
汇报实验或研究的主要结果,包括数值和统计数据。
这一部分通常包括表格或图形以支持结果的呈现。
5. 结论(Conclusions):
提供对研究结果的总结和解释,强调研究的贡献,并可能讨论进一步研究的方向。
这是一个简化的模板,实际的结构化摘要可能根据期刊或会议的要求而有所不同。
不同领域和期刊可能对结构有不同的要求,但总体目标是提供一种清晰、有序的方式来传达研究的核心信息。
结构化状态空间序列模型

结构化状态空间序列模型
结构化状态空间序列模型(Structured State Space Sequential Model)是一种用于建模时序数据的统计模型。
该模型假设观
测数据的生成过程是由一个隐含的、非观测的状态序列驱动的,其中每个状态都对应着一个观测数据。
在结构化状态空间序列模型中,状态之间的转换是通过一个转移矩阵来建模的。
该转移矩阵描述了从一个状态转移到另一个状态的概率分布。
此外,模型还包括一个观测矩阵,用于描述从状态生成观测数据的概率分布。
根据具体需求,可以选择不同的结构化状态空间序列模型来建模不同类型的时序数据。
一些常见的结构化状态空间序列模型包括隐马尔可夫模型(Hidden Markov Model, HMM)、条件
随机场(Conditional Random Field, CRF)等。
结构化状态空间序列模型被广泛应用于自然语言处理、机器翻译、语音识别、图像处理等领域,用于解决一些与时序数据相关的问题,如序列标注、音频识别、模式识别等。
凡事预则立,不预则废---structure表示计划、安排
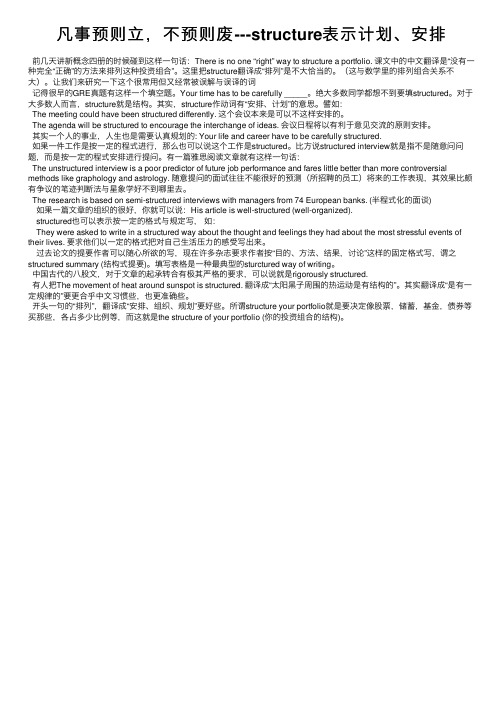
凡事预则⽴,不预则废---structure表⽰计划、安排前⼏天讲新概念四册的时候碰到这样⼀句话:There is no one “right” way to structure a portfolio. 课⽂中的中⽂翻译是“没有⼀种完全“正确”的⽅法来排列这种投资组合”。
这⾥把structure翻译成“排列”是不⼤恰当的。
(这与数学⾥的排列组合关系不⼤)。
让我们来研究⼀下这个很常⽤但⼜经常被误解与误译的词记得很早的GRE真题有这样⼀个填空题。
Your time has to be carefully _____。
绝⼤多数同学都想不到要填structured。
对于⼤多数⼈⽽⾔,structure就是结构。
其实,structure作动词有“安排、计划”的意思。
譬如:The meeting could have been structured differently. 这个会议本来是可以不这样安排的。
The agenda will be structured to encourage the interchange of ideas. 会议⽇程将以有利于意见交流的原则安排。
其实⼀个⼈的事业,⼈⽣也是需要认真规划的: Your life and career have to be carefully structured.如果⼀件⼯作是按⼀定的程式进⾏,那么也可以说这个⼯作是structured。
⽐⽅说structured interview就是指不是随意问问题,⽽是按⼀定的程式安排进⾏提问。
有⼀篇雅思阅读⽂章就有这样⼀句话:The unstructured interview is a poor predictor of future job performance and fares little better than more controversial methods like graphology and astrology. 随意提问的⾯试往往不能很好的预测(所招聘的员⼯)将来的⼯作表现,其效果⽐颇有争议的笔迹判断法与星象学好不到哪⾥去。
结构化面试是什么意思

结构化面试是什么意思结构化面试什么意思结构化面试(Structured Interviewing)为根据特定职位的胜任特征要求,遵循固定的程序,采用专门的题库、评价标准和评价方法,通过考官小组与应考者面对面的言语交流等方式,评价应考者是否符合招聘岗位要求的人才测评方法。
结构化面试是在工作分析的基础上精心设计与工作有关的问题和各种可能的答案,并根据被试者回答的速度和内容对其作出等级评价的面试。
一种比较规范的面试形式。
有效性和可靠性较高,但不能进行设定问题外的提问,局限了面试的深度,而且问题均为事先安排好的,整个过程显得不自然,且提问可能显得唐突。
结构化面试流程步骤有哪些第一,抽签确定顺序。
具体地说,就是考生在规定的时间进入候考室后,一般是先按要求将手机等通讯工具,统一交到工作人员处集中保存,然后抽签,确定每个人的面试考场、考号顺序。
第二,进门鞠躬敬礼。
在工作人员喊考号请你进入考场后,一般由引导员送你到考场门口,进门时无须敲门,轻轻推开进入即可。
进门后,应当向考官鞠躬致意,说一声“各位考官好!”,在考官回应后在考生桌位前就座。
第三,看题思考回答。
这是面试中,最关键的环节,它最终决定您的面试成绩。
这个环节包含阅读放在桌面上的试题,有的地方是主考官宣读;略作思考,必要时列个大纲目,思考时间有限制;逐题按顺序回答等内容。
第四,完毕起身告辞。
全部题目回答结束后,应当说一声“回答完毕”,主考官示意或者说“你可以离开了”后,即再次鞠躬,道一句“谢谢!”转身离开考场。
第五,再次进场听分。
上午或者下午的一场面试全部结束后,考生往往会按组或几名一起进入考场,听主考官宣布每一名考生的面试成绩。
至此,面试的过程就彻底结束了。
结构化面试与半结构化面试的区别一、结构化面试。
所谓的结构化面试,是一种标准化、流程化都十分严格的面试组织形式,所有考生依次入场答题,考试过程只能在同样的题目框架下发挥,考官不能进行追问,全程没有互动。
st语言ldp用法
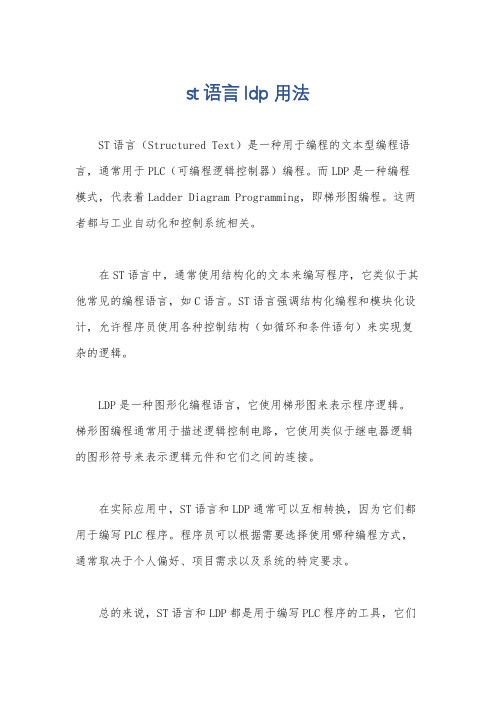
st语言ldp用法
ST语言(Structured Text)是一种用于编程的文本型编程语言,通常用于PLC(可编程逻辑控制器)编程。
而LDP是一种编程模式,代表着Ladder Diagram Programming,即梯形图编程。
这两者都与工业自动化和控制系统相关。
在ST语言中,通常使用结构化的文本来编写程序,它类似于其他常见的编程语言,如C语言。
ST语言强调结构化编程和模块化设计,允许程序员使用各种控制结构(如循环和条件语句)来实现复杂的逻辑。
LDP是一种图形化编程语言,它使用梯形图来表示程序逻辑。
梯形图编程通常用于描述逻辑控制电路,它使用类似于继电器逻辑的图形符号来表示逻辑元件和它们之间的连接。
在实际应用中,ST语言和LDP通常可以互相转换,因为它们都用于编写PLC程序。
程序员可以根据需要选择使用哪种编程方式,通常取决于个人偏好、项目需求以及系统的特定要求。
总的来说,ST语言和LDP都是用于编写PLC程序的工具,它们
各自有着自己的特点和适用场景,程序员可以根据实际情况进行选择和应用。
structured text 语言中的指令
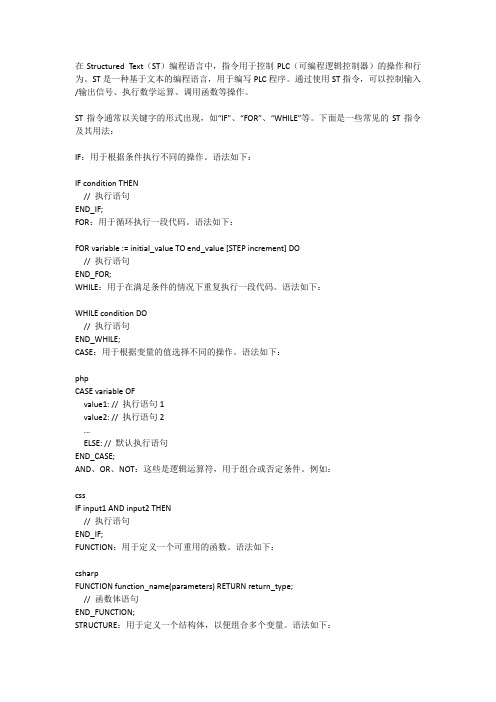
在Structured Text(ST)编程语言中,指令用于控制PLC(可编程逻辑控制器)的操作和行为。
ST是一种基于文本的编程语言,用于编写PLC程序。
通过使用ST指令,可以控制输入/输出信号、执行数学运算、调用函数等操作。
ST指令通常以关键字的形式出现,如“IF”、“FOR”、“WHILE”等。
下面是一些常见的ST指令及其用法:IF:用于根据条件执行不同的操作。
语法如下:IF condition THEN// 执行语句END_IF;FOR:用于循环执行一段代码。
语法如下:FOR variable := initial_value TO end_value [STEP increment] DO// 执行语句END_FOR;WHILE:用于在满足条件的情况下重复执行一段代码。
语法如下:WHILE condition DO// 执行语句END_WHILE;CASE:用于根据变量的值选择不同的操作。
语法如下:phpCASE variable OFvalue1: // 执行语句1value2: // 执行语句2...ELSE: // 默认执行语句END_CASE;AND、OR、NOT:这些是逻辑运算符,用于组合或否定条件。
例如:cssIF input1 AND input2 THEN// 执行语句END_IF;FUNCTION:用于定义一个可重用的函数。
语法如下:csharpFUNCTION function_name(parameters) RETURN return_type;// 函数体语句END_FUNCTION;STRUCTURE:用于定义一个结构体,以便组合多个变量。
语法如下:arduinoSTRUCTURE structure_name STRUCT;variable1: type1;variable2: type2;...END_STRUCT;这些指令只是ST编程语言中的一部分,还有其他指令可用于实现更复杂的控制逻辑和数据处理操作。
structuredoutputparser 使用-概述说明以及解释

structuredoutputparser 使用-概述说明以及解释1.引言1.1 概述概述结构化输出解析器(Structured Output Parser)是一种用于从非结构化数据中提取结构化信息的工具。
随着数据量的不断增加,很多数据存在于文本、图像、视频等非结构化格式中,这给数据分析和处理带来了挑战。
结构化输出解析器的出现为我们提供了一种解决这一问题的方法。
通过结构化输出解析器,我们可以将不规则、混乱的数据转化为规范化、有序的结构化数据。
这不仅有助于提高数据的利用率和可操作性,还能帮助我们更加高效地进行数据分析、挖掘和应用。
在信息检索、自然语言处理、计算机视觉等领域,结构化输出解析器都发挥着重要的作用。
本文将详细介绍什么是structured output parser、其工作原理、应用领域、优势以及未来发展趋势,希望可以为读者提供全面的了解和实际指导。
1.2 文章结构本文分为三个主要部分:引言,正文和结论。
在引言部分,我们将介绍文章的背景和重要性,并简要概述structured output parser的基本概念。
我们将讨论文章的结构和目的,以便读者对后续内容有一个清晰的了解。
正文部分将深入探讨structured output parser的定义、工作原理和应用领域。
我们将解释什么是structured output parser以及它是如何解析结构化输出的。
我们还将介绍structured output parser在不同领域的应用,并探讨其在现实生活中的重要性。
在结论部分,我们将总结本文的主要观点和结论。
我们将强调structured output parser的优势和未来发展前景,以及对该技术的总体评价。
最后,我们将提出一些展望和建议,以帮助读者更好地理解和应用structured output parser。
1.3 目的结构化输出解析器(structured output parser)的目的是为了帮助用户从结构化的输出数据中提取有用信息。
- 1、下载文档前请自行甄别文档内容的完整性,平台不提供额外的编辑、内容补充、找答案等附加服务。
- 2、"仅部分预览"的文档,不可在线预览部分如存在完整性等问题,可反馈申请退款(可完整预览的文档不适用该条件!)。
- 3、如文档侵犯您的权益,请联系客服反馈,我们会尽快为您处理(人工客服工作时间:9:00-18:30)。
1Corpora for Information Retrieval ResearchWilliam Hersh, MD Professor and ChairDepartment of Medical Informatics & Clinical EpidemiologyOregon Health & Science Universityhersh@ 2Overview•Information retrieval (IR) use case •Corpora developed for IR research–Clinical document retrieval –Genomics document retrieval –Image retrieval3The information retrieval (IR) process (Hersh, 2003)MetadataMetadata Queries Queries ContentContent SearchengineSearch engine Retrieval-Boolean-Natural languageIndexing-Words-Terms -Attributes4IR in science: from literatureto knowledgeAll literature Possibly relevantliterature Definitely relevantliteratureStructured knowledgeInformation retrievalInformationextraction,text mining5Evaluation of IR systems•System-oriented –how well system performs–Historically focused on relevance-based measures•Recall –# relevant retrieved / # relevant in collection •Precision –# relevant retrieved / # retrieved by search–When documents ranked, can aggregate both using mean average precision (MAP)•User-oriented –how well user performs with system–e.g., performing task, user satisfaction, etc.6System-oriented IR evaluation•Historically assessed with test collections, which consist of–Content –fixed yet realistic collections of documents, images, etc.–Topics –statements of information need that can be fashioned into queries entered into retrieval systems –Relevance judgments –by expert humans for which content items should be retrieved for which topics•Evaluation consists of “runs”with output for each topic measured and averaged7Some well-known system-orientedevaluation forums•Text Retrieval Conference (TREC, ; Voorhees, 2005)–Many “tracks”of interest, such as Web searching, question-answering, etc.–Non-medical, with exception of Genomics Track (Hersh, 2006)•Cross-Language Evaluation Forum (CLEF, )–Focus on retrieval across languages, European-based–Additional focus on image retrieval, which includes medical image retrieval tasks (Hersh, 2006)•Both operate on annual cycle of test collection release, experiments, and analysis of results8Corpora for IR evaluation•Clinical document retrieval –OHSUMED •Genomics document retrieval and categorization –The TREC Genomics Track•Image retrieval –ImageCLEFmed9General information•Needs and tasks–Information retrieval, i.e., search•Availability–Via registration and signing usage agreement (required by publishers)•Annotation–Use annotation from source–Topics and relevance judgments added10OHSUMEDftp:///pub/OHSUMED•Good early test collection with substantial use–My highest ranking citation in Google Scholar is ACM SIGIR conference proceedings paper (Hersh, 1994) about collection(!)•Stats–348,566 MEDLINE records–106 topics from general medicine–Relevance judgments at two levels, definitely and possibly relevant•Caveats–Old –documents from 1987-1991–“Clustered”collection –from general medical journals only, not all of MEDLINE11TREC Genomics Track•New biotechnologies have revolutionized data collection, management, and analysis •Prototype use case: gene microarray experiment generating dozens of genes previously unknown to be involved in a physiological or disease process•Scientists must find information about these genes efficiently12TREC Genomics Track /genomics/•Overall focus on retrieval of documents about genomics by biomedical researchers •2004-2005 –focus on two user tasks–Ad hoc retrieval –finding documents based on information needs (Hersh, 2006)–Article “triage”–does a paper have data that would warrant inclusion in the Mouse Genome Informatics database? (Cohen, 2006)•2006 –passage retrieval (Hersh, 2006)–Users approach the literature with questions and want•Passages of text that indicate answer in document •Aspects that passages on same topic•Linkage to documents that contain these passages13TREC 2006 Genomics –from passages to aspects to documentsPassagesAspectsDocuments14Image retrieval•Biomedical professionals increasingly use images for research, clinical care, andeducation, yet we know very little about how they find them•Image retrieval is a “poor stepchild”to textretrieval, with less understanding of how people use systems and how well they work•Most image retrieval work has focused on either text annotation retrieval or image processing, but–Not both in combination –Not from an IR perspective15Image retrieval approaches(Müller, 2004)•Semantic or textual –by text annotation, e.g.,–Narrative description–Controlled terminology assignment –Other types of textual metadata, e.g., modality, location•Visual–Identification of features, e.g., colors, texture, shape, segmentation16Example of visual image retrievalinterfacehttp://www.sim.hcuge.ch/medgift/w01_Presentation_EN.htm17ImageCLEFmed/image/•Aims to simulate general searching over wide variety of medical images•Uses standard IR approach with test collection consisting of–Content –Topics–Relevance judgments•Has operated through two cycles of CLEF (2005-2006)–Both used same image collection–Developed new topics and performed relevance judgments for each cycle18ImageCLEFmed 2005-2006test collectionGerman –7805English –780578057805PathologyPathoPICEnglish –323193231932319Pathology Pathology Education Instructional Resource (PEIR)English –4071177407Nuclear medicineMallinckrodt Institute of Radiology (MIR)English –177French –189987252076Mixed CasimageAnnotations Images Cases Predominant images Collection19Example case from CasimageID : 4272Description : A large hypoechoic mass is seen in the spleen. CDFI reveals it to be hypovascular and distorts the intrasplenic blood vessels. This lesion is consistent with a metastatic lesion. Urinary obstruction is present on the right with pelvo-caliceal and uretreal dilatation secondary to a soft tissue lesion at the junction of the ureter and baldder. This is another secondary lesion of the malignant melanoma. Surprisingly, these lesions are not hypervascular on doppler nor on CT. Metastasis are also visible in the liver.Diagnosis : Metastasis of spleen and ureter, malignant melanomaClinical Presentation : Workup in a patient with malignant melanoma. Intravenous pyelography showed no excretion of contrast on the right.ImagesCaseannotation20For more information•/•ftp:///pub/OHSUMED/•/genomics •/image•Questions?21References•Hersh WR,Information Retrieval: A Health and Biomedical Perspective , New York: Springer-Verlag, 2003.•Hersh WR, Buckley C, Leone TJ, Hickam DH, OHSUMED: an interactive retrieval evaluation and new large test collection for research, Proceedings of the 17th Annual ACM SIGIR Conference on Research and Development in Information Retrieval , 1994, 192-201.•Hersh WR, Bhupatiraju RT, Ross L, Johnson P, Cohen AM, Kraemer DF, Enhancing access to the bibliome: the TREC 2004 Genomics Track, Journal of Biomedical Discovery and Collaboration , 2006, 1:3, //content/1/1/3.•Cohen AM, Hersh WR, The TREC 2004 Genomics Track categorization task: classifying full text biomedical documents, Journal of Biomedical Discovery and Collaboration , 2006, 1:4, /content/1/1/4.•Hersh WR, Müller H, Jensen J, Yang J, Gorman P, Ruch P, Advancing biomedical image retrieval: development and analysis of a test collection, Journal of the American Medical Informatics Association , 2006, 13: 488-496.•Hersh W, Cohen AM, Roberts P, Rekapalli HP, TREC 2006 Genomics Track overview, The Fifteenth Text Retrieval Conference -TREC 2006, /~hersh/trec-06-genomics.pdf.。