The Role of Non-Ambiguous Words in Natural Language Disambiguation
On+the+Translato...
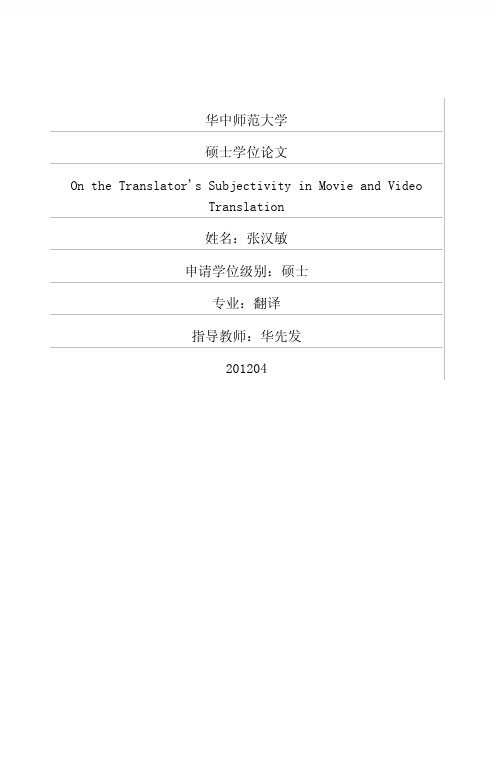
华中师范大学硕士学位论文On the Translator's Subjectivity in Movie and VideoTranslation姓名:***申请学位级别:硕士专业:翻译指导教师:***201204摘要译者是翻译的主体,在翻译过程中无论译者怎样追求客观也难逃自身的“主体性”经验。
译者的主体性包含三个方面:能动性,受动性和为我性。
哲学阐释学、权利话语和目的论分别为这三个方面提供了理论基础。
从哲学阐释学的角度说,主体经验是人类认知世界的必由之路。
人类的各种认知,包括对数理、科学和文学等等的理解都建立在由个体经验汇成的主体性经验之上。
这就为译者发挥主观能动性提供了基础。
从话语理论的角度来说,译者在翻译时要受到原文语言、读者要求和译者所处的历史文化背景的制约,这就是译者受动性的主要内容。
从目的论的角度说,译者的能动性和受动性是矛盾的两个方面,偏重任何一方都不妥。
平抑两者矛盾的标准就是译者的为我性。
影视翻译有其鲜明的特征,这是因为影视欣赏首先是一个读图的过程而非语言输入的过程。
镜头语言作为影视语言重要的组成部分以其直观性而成为了某种意义上的“世界语”,这就是人类认知具有共通性的例证,也是影视翻译中译者发挥主观能动性的先决条件和重要保障。
可译性是翻译中另一个重要的哲学问题:从哲学的角度来看,loo%的翻译,即绝对的翻译根本就不存在,所谓可译性就是无限接近绝对翻译即实现相对翻译的可能性。
在影视翻译中,有了图像作保障,合理发挥译者的主观能动性有可能使译文所表达的内容和承载的文化信息更贴近原文的内容和信息。
在影视翻译中,创造性叛逆是发挥主观能动性的重要表现形式,也是一种有效的翻译策略。
探讨影视翻译中译者的主体性也不能局限于分析其主观能动性,还必须考虑译者的受动性。
影视翻译究其实质仍是文本的翻译,因此译者必须尊重原文的话语权;与此同时,译者也要考虑观众的期待和要求;此外,译者同样还会受到其所处历史文化背景的影响。
高二英语英语学术论文写作单选题30题答案解析版

高二英语英语学术论文写作单选题30题答案解析版1.In academic writing, it is important to be _______ in presenting your arguments.A.preciseB.vagueC.casualD.hasty答案:A。
在学术写作中,精确地呈现你的论点很重要。
选项B“vague”( 模糊的)不符合学术写作要求;选项C“casual”( 随意的)和选项D“hasty” 匆忙的)也不适合学术写作的严谨性。
2.When writing an academic paper, you should avoid using _______ language.A.colloquialB.formalC.technicalD.sophisticated答案:A。
写学术论文时,应避免使用口语化的语言。
选项B“formal”正式的)、选项C“technical”专业的)和选项D“sophisticated”(复杂的)在学术写作中有其特定用途,而口语化语言不适合学术写作。
3.A good academic paper is characterized by its _______ analysis.A.superficialB.thoroughC.hastyD.cursory答案:B。
一篇好的学术论文以其全面的分析为特点。
选项A“superficial”( 肤浅的)、选项C“hasty”( 匆忙的)和选项D“cursory” 粗略的)都不能体现学术论文的高质量分析。
4.In academic writing, you should use _______ sources to support your arguments.A.reliableB.dubiousC.unreliableD.questionable答案:A。
在学术写作中,你应该使用可靠的来源来支持你的论点。
通过模仿学习英语的作文的提纲
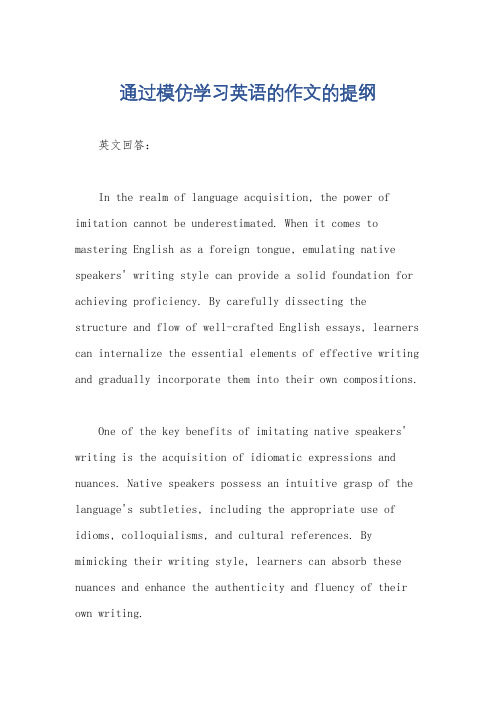
通过模仿学习英语的作文的提纲英文回答:In the realm of language acquisition, the power of imitation cannot be underestimated. When it comes to mastering English as a foreign tongue, emulating native speakers' writing style can provide a solid foundation for achieving proficiency. By carefully dissecting thestructure and flow of well-crafted English essays, learners can internalize the essential elements of effective writing and gradually incorporate them into their own compositions.One of the key benefits of imitating native speakers' writing is the acquisition of idiomatic expressions and nuances. Native speakers possess an intuitive grasp of the language's subtleties, including the appropriate use of idioms, colloquialisms, and cultural references. By mimicking their writing style, learners can absorb these nuances and enhance the authenticity and fluency of their own writing.Furthermore, imitation helps learners develop a strong sense of grammar and syntax. Native speakers' writing typically adheres to the conventions of standard English grammar, providing a reliable model for learners to follow. By studying how native speakers construct grammatically correct sentences and paragraphs, learners can internalize the rules of the language and avoid common errors.Additionally, imitating native speakers' writingfosters a keen awareness of sentence structure and organization. English essays often follow a logical progression of ideas, with each paragraph building upon the previous one to create a cohesive narrative. By examining how native speakers structure their essays, learners can develop a deep understanding of the principles of organization and coherence.Beyond the technical aspects of writing, imitation also contributes to the development of critical thinking skills. By analyzing native speakers' essays, learners can gain insights into their thought processes and approaches toargumentation. This exposure to diverse perspectives and styles of thinking can broaden learners' own intellectual horizons and enhance their ability to express complex ideas effectively.To effectively imitate native speakers' writing,learners should engage in a systematic and focused approach. This involves reading a wide range of English literature, paying close attention to the language used and the techniques employed by skilled writers. Learners shouldalso practice writing regularly, imitating the styles and structures they encounter in their readings. With regular practice and consistent feedback, learners can gradually refine their writing skills and develop a writing stylethat is both authentic and effective.In summary, imitating native speakers' writing offers numerous benefits for learners of English as a foreign language. By internalizing the idiomatic expressions, grammar, sentence structure, and organization used bynative speakers, learners can significantly enhance their writing proficiency. Through a combination of focusedreading, practice, and feedback, learners can master theart of writing in English and effectively communicate their ideas with clarity and confidence.中文回答:在语言习得领域,模仿的力量不容小觑。
07 阅读理解之态度与看法类 2023高考英语二轮复习
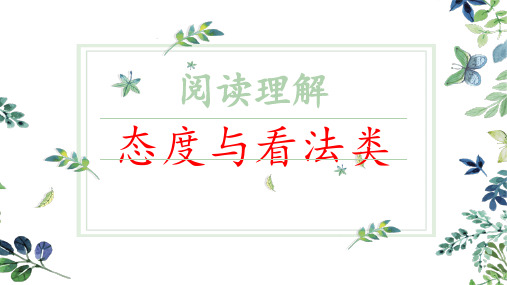
态度与看法类
观点态度题是什么?
作者观点态度题就是指针对作者的写作意图、 观点态度和对事件的评价设问的阅读理解题目。 作者的观点和态度除了直接表达外,还经常在 文章中间接表达出来。考生可以通过全文的叙 述,从文章的主要内容去理解作者的观点;有 时作者也会在文章中用特殊的词汇表达自己的 思想感情。同学们要从文章中的用词、语气或 对某个细节的陈述来推断作者的态度、观点等。
Technology, said he wasn’t surprised by the promotion about
the launch of the fast lane, and thought the concept would appeal
to shoppers all over the world. “Crowded parking lots and busy
shopping centers tend to be two of the biggest complaints of
shoppers over the festive season,” he said. “I think the fast
lanes are a new approach. However, I suspect it w根的il据评l b本论e 段aI tb第hiitn四lkik行the处e fGasatrylaMneosrtaimreear
A. Supportive C. Critical
B. Indifferent 势和劣势,因此他的态度是客观 D. Objective 的。故选D。
My uniform might not be what I would wear in my
own time, but it gives me a sense of belonging, takes
关于桥牌的英文术语介绍
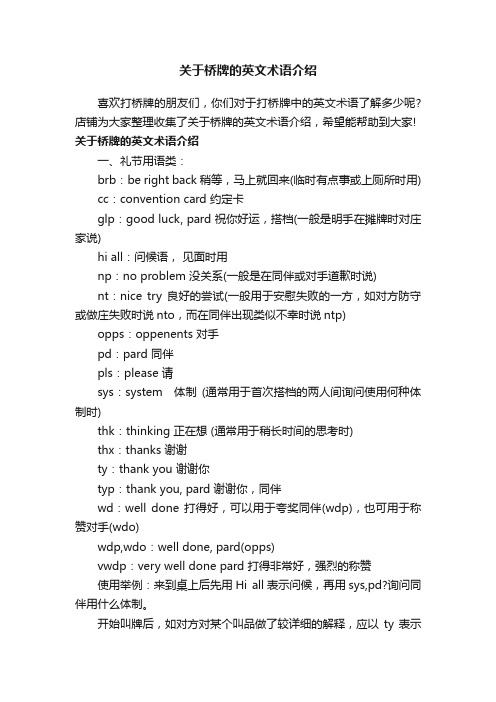
关于桥牌的英文术语介绍喜欢打桥牌的朋友们,你们对于打桥牌中的英文术语了解多少呢?店铺为大家整理收集了关于桥牌的英文术语介绍,希望能帮助到大家! 关于桥牌的英文术语介绍一、礼节用语类:brb:be right back 稍等,马上就回来(临时有点事或上厕所时用) cc:convention card 约定卡glp:good luck, pard 祝你好运,搭档(一般是明手在摊牌时对庄家说)hi all:问候语,见面时用np:no problem 没关系(一般是在同伴或对手道歉时说)nt:nice try 良好的尝试(一般用于安慰失败的一方,如对方防守或做庄失败时说nto,而在同伴出现类似不幸时说ntp)opps:oppenents 对手pd:pard 同伴pls:please 请sys:system 体制(通常用于首次搭档的两人间询问使用何种体制时)thk:thinking 正在想 (通常用于稍长时间的思考时)thx:thanks 谢谢ty:thank you 谢谢你typ:thank you, pard 谢谢你,同伴wd:well done 打得好,可以用于夸奖同伴(wdp),也可用于称赞对手(wdo)wdp,wdo:well done, pard(opps)vwdp:very well done pard 打得非常好,强烈的称赞使用举例:来到桌上后先用Hi all表示问候,再用sys,pd?询问同伴用什么体制。
开始叫牌后,如对方对某个叫品做了较详细的解释,应以ty表示感谢。
如本方主打,则明手摊牌时说glp以祝同伴好运,而庄家以typ 表示感谢。
如同伴做成定约或打出了正确的防守,则应说wdp及nto,以称赞同伴,安慰对手;反之,则可说wdo及ntp以体现风度。
如果同伴或对方打出了佳着,则可说vwdp或vwdo。
如有事暂离片刻,则可说brb。
二、桥牌术语类ASK Asking bid 问叫BAL Balanced 平均牌型BW Blackwood 黑木问ACUE Cue-bid 扣叫DBL or X Double 加倍F Forcing 逼叫F1 Forcing one round 逼叫一轮FG Forcing to game 逼叫到局Fit-showing Show bidded suit and support partner’s suit.显示所叫的花色,并且对同伴的花色也有支持4SF Fourth suit forcing. 第四花色逼叫GSF Grand slam forcing 大满贯逼叫G/T Game try 进局试探H Honour (Ace, King or Queen) 大牌(指A,K 或Q)HCP High Card Points 大牌点INV Invitational 邀请,邀叫JTB Jacoby transfer bid 杰可贝转移叫KCB Keycard blackwood 关键张黑木问叫LHO The opponent on your left 左手敌方L/S Long suit 长套花色M Major 高级花色(指S或H)m Minor 低级花色(指D或C)M’s Majors 双高花m’s Minors 双低花MAX Maximum, Maximal, Maximal Overcall Double 高限,高限竞叫性加倍MIN Minimum 低限MULTI 多用途NF Nonforcing 不逼叫NT Notrump 无将RDBL Redouble 再加倍RESP Responder; Response; Responsive 应叫者;应叫;应叫性REV Reverse 反的;逆叫RHO The opponent on your right 右手敌方RKCB Roman keycard blackwood 罗马关键张问叫SPL Splinter, or short suit 爆裂叫,斯泼令特,或短套花色S/T Slam try 满贯试探STAY Stayman 斯台曼SUPP Support 支持UNT Unusual Notrump 不寻常无将WK Weak 弱;弱牌x Any suit; Any small card 任何一门花色;任何一张小牌4th Fourth best leads 长四首攻三、其他术语:ART Artificial 人为,人为的ATT Attitude 姿态B Black suit(s) 黑花色(指S和C)CAB Contral asking bid 控制问叫CB Checkback 重询斯台曼COMP Competitive 竞争,竞叫CONC Concentrated (all values in the bid suits) 牌力集中(所有的牌力集中在叫过的花色上)CONST Constructive 建设性CTRL Control 控制DISCG Discourage(ing) 不欢迎DURRY 德鲁利约定叫E Even 偶数张ENCRG Encourage(ing) 鼓励,欢迎FRAG Fragment 碎片叫(如5431牌型先叫5张,再叫4张,第3次再叫3张套。
内蒙古师范大学复试英语笔试作文
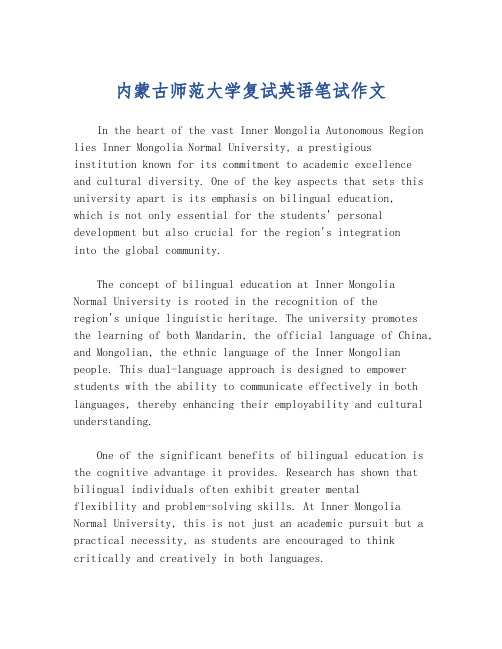
内蒙古师范大学复试英语笔试作文In the heart of the vast Inner Mongolia Autonomous Region lies Inner Mongolia Normal University, a prestigiousinstitution known for its commitment to academic excellence and cultural diversity. One of the key aspects that sets this university apart is its emphasis on bilingual education,which is not only essential for the students' personal development but also crucial for the region's integrationinto the global community.The concept of bilingual education at Inner Mongolia Normal University is rooted in the recognition of theregion's unique linguistic heritage. The university promotes the learning of both Mandarin, the official language of China, and Mongolian, the ethnic language of the Inner Mongolian people. This dual-language approach is designed to empower students with the ability to communicate effectively in both languages, thereby enhancing their employability and cultural understanding.One of the significant benefits of bilingual education is the cognitive advantage it provides. Research has shown that bilingual individuals often exhibit greater mentalflexibility and problem-solving skills. At Inner Mongolia Normal University, this is not just an academic pursuit but a practical necessity, as students are encouraged to think critically and creatively in both languages.Moreover, the bilingual education system at theuniversity fosters a deep appreciation for cultural diversity. Students are not only taught the languages but also the rich histories and traditions associated with them. This holistic approach to education helps to preserve and promote theunique cultural identity of the Inner Mongolian people while also preparing them to contribute to a multicultural society.In conclusion, the bilingual education policy at Inner Mongolia Normal University is a testament to theinstitution's forward-thinking approach to education. It not only prepares students for the challenges of the modern world but also instills in them a sense of pride in their cultural heritage. As the world becomes increasingly interconnected,the importance of bilingualism and cultural literacy canhardly be overstated, and Inner Mongolia Normal University is leading the way in this regard.。
美国文学选读_河海大学中国大学mooc课后章节答案期末考试题库2023年
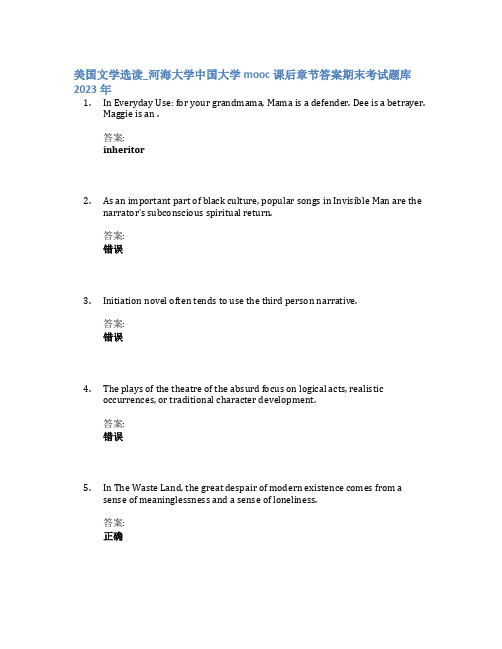
美国文学选读_河海大学中国大学mooc课后章节答案期末考试题库2023年1.In Everyday Use: for your grandmama, Mama is a defender. Dee is a betrayer.Maggie is an .答案:inheritor2.As an important part of black culture, popular songs in Invisible Man are thenarrator’s subconscious spiritual return.答案:错误3.Initiation novel often tends to use the third person narrative.答案:错误4.The plays of the theatre of the absurd focus on logical acts, realisticoccurrences, or traditional character development.答案:错误5.In The Waste Land, the great despair of modern existence comes from asense of meaninglessness and a sense of loneliness.答案:正确6.When Rip begins to wonder about his ______ in the story, this feeling ofstrangeness and confusion climbs up to a climax .答案:identity7.Washington Irving attached a note by Knickerbocker in Rip Van Winkle,because _______.答案:B. he attempted to improve the authenticity of story.8.Scholars have long pointed out the link between Puritanism and capitalism:Both rest on ambition , _______, and an intense striving for success.答案:B. hard work9.The Birth-Mark indicates the _______of husband and wife.答案:D. power struggle10.Freud’s theory of per sonality is structured into three parts, the _______ , ego,and superego .答案:A. id11.Upward movement of Gothic architecture suggests ______ .答案:B. heavenward aspiration12.We can learn from Invisible Man that is a devastating force, possessing thepower to render black Americans virtually invisible.答案:racism13.Gary Snyder proposes three categories of nature: nature, the wild, and .答案:C. wildness14.InA Day’s Wait, the son took the centigrade as Fahrenheit wrongly.答案:错误15.The theme of The Road Not Taken is about _____ and encourages people tolive their individualism to the fullest.答案:non-conformism16.Psychologist Carl Jung firstly proposed the word ____ in his idea of “CollectiveUnconscious”.答案:archetype##%_YZPRLFH_%##"archetype"17. A Day’s Wait was written by _______ who won the Nobel Prize for literature in1954.答案:Ernest Hemingway##%_YZPRLFH_%##Hemingway18.Rip Van Winkle story reflects the psychological truth of the American peoplebefore and after the________.答案:American War of Independence##%_YZPRLFH_%##AmericanRevolution##%_YZPRLFH_%##War of Independence19.The differences between imagist poem and ancient Chinese poem lie in .答案:B. personal feelings20.The Transcendentalist movement was a reaction against .答案:B. rationalism of 18th century21.T.S. Eliot’s poem is hard to read because the poet .答案:A. uses a lot of obscure allusions and makes the work in fragment.22.Initiation novel usually has a similar plot pattern, it is .答案:C. departure—ordeal—transformation—maturity.23.In The Birth-Mark, Aylmer finally gave up removing Georgiana’s birth markon the face out of a husband’s sense of duty.答案:错误24.Washington Irving is regarded as the first internationally recognizedAmerican author.答案:正确25.In order to escape his nagging wife, Rip Van Winkle took his gun and his dogwolf with him to enter the forests in the Catskills.答案:正确26.Goodman Brown left home to attend a witch’s Sabbath in the forest, whichwill be performed between sunset and sunrise.答案:正确27.To T.S. Eliot, the second world war not only destroyed people’s home, butalso their mentality.答案:正确28.In Death of A Salesman, Willy Loman’s tragedy reveals the disillusionment of .答案:American dream29.is the god of wine and dance, of irrationality and chaos, and appeals toemotions and instincts.答案:Dionysus30.“Bildungsroman”, a literary genre that focuses on the psychological andmoral growth of a protagonist from to adulthood.答案:youth。
语言学相关英语作文高中

语言学相关英语作文高中Title: The Fascinating World of Linguistics。
Introduction:Language, the quintessential tool of human communication, embodies a rich tapestry of history, culture, and cognitive mechanisms. Exploring the realm oflinguistics unravels the mysteries behind the construction, evolution, and diversity of languages worldwide. In this essay, we delve into the fascinating world of linguistics, examining its fundamental concepts, theoretical frameworks, and real-world applications.The Nature of Language:Language is a complex system of symbols and rules usedto convey meaning. Linguists study its structure, sounds, meanings, and contexts to understand how it functions in communication. One of the central pillars of linguistics isthe study of phonetics and phonology, which deals with the sounds of language and their organization. From the articulation of sounds to the patterns of stress and intonation, phonetics and phonology provide insights into the acoustic and perceptual aspects of speech.Grammar, another cornerstone of linguistics, encompasses syntax, morphology, and semantics. Syntax examines the arrangement of words in sentences, while morphology investigates the internal structure of words and their formation. Semantics delves into the meanings of words and sentences, exploring how language conveys information and expresses concepts.Language Diversity and Universality:Languages vary widely across cultures and regions, reflecting the unique histories and identities of communities. Linguists classify languages into different families based on their structural and historical similarities. The Indo-European, Sino-Tibetan, and Afro-Asiatic language families, among others, illustrate theintricate web of linguistic connections spanning continents.Despite this diversity, linguists have identified universal principles that underlie all languages. Noam Chomsky's theory of Universal Grammar posits that humansare innately predisposed to acquire language and share certain grammatical principles. This universality suggests that while languages may differ in their surface forms,they adhere to common underlying structures shaped by cognitive processes.Language Change and Evolution:Languages are not static entities but dynamic systems that evolve over time. Historical linguistics traces the development of languages through processes such as sound change, lexical borrowing, and grammaticalization. By analyzing language data from different time periods,linguists reconstruct ancestral languages and track the trajectories of language families.Sociolinguistics investigates how language interactswith society, exploring issues such as dialect variation, language contact, and language policy. From regional accents to social dialects, language reflects social identity and cultural norms. Sociolinguistic research sheds light on language attitudes, language maintenance, and language shift in multilingual communities.Applied Linguistics:Beyond theoretical inquiry, linguistics has practical applications in various fields. Applied linguistics encompasses areas such as language teaching, translation, and language planning. Language acquisition research informs pedagogical approaches to second language learning, while computational linguistics develops algorithms for natural language processing.Translation and interpreting bridge linguistic and cultural divides, facilitating communication in diverse contexts. Language planning involves the deliberate efforts to regulate, standardize, or promote languages within a community or nation. These applied domains showcase therelevance of linguistics in addressing real-world challenges related to communication and intercultural understanding.Conclusion:Linguistics offers a window into the intricate workings of human language, from its structural complexities to its cultural significance. By investigating the nature, diversity, and evolution of languages, linguists unravel the mysteries of human communication and contribute to interdisciplinary knowledge. As we navigate the ever-changing landscape of language and society, the insights gleaned from linguistics continue to enrich our understanding of the world around us.。
- 1、下载文档前请自行甄别文档内容的完整性,平台不提供额外的编辑、内容补充、找答案等附加服务。
- 2、"仅部分预览"的文档,不可在线预览部分如存在完整性等问题,可反馈申请退款(可完整预览的文档不适用该条件!)。
- 3、如文档侵犯您的权益,请联系客服反馈,我们会尽快为您处理(人工客服工作时间:9:00-18:30)。
The Role of Non-Ambiguous Words in Natural Language DisambiguationRada MihalceaDepartment of Computer Science and EngineeringUniversity of North Texasrada@AbstractThis paper describes an unsupervised approach fornatural language disambiguation,applicable to am-biguity problems where classes of equivalence canbe defined over the set of words in a lexicon.Lexi-cal knowledge is induced from non-ambiguous wordsvia classes of equivalence,and enables the automaticgeneration of annotated corpora.The only require-ments are a lexicon and a raw textual corpus.Themethod was tested on two natural language ambigu-ity tasks in several languages:part of speech tagging(English,Swedish,Chinese),and word sense disam-biguation(English,Romanian).Classifiers trained onautomatically constructed corpora were found to havea performance comparable with classifiers that learnfrom expensive manually annotated data.1IntroductionAmbiguity is inherent to human language.Success-ful solutions for automatic resolution of ambiguity in natural language often require large amounts of annotated data to achieve good levels of accuracy. While recent advances in Natural Language Process-ing(NLP)have brought significant improvements in the performance of NLP methods and algorithms, there has been relatively little progress on address-ing the problem of obtaining annotated data required by some of the highest-performing algorithms.As a consequence,many of today’s NLP applications ex-perience severe data bottlenecks.According to recent studies(e.g.Banko and Brill2001),the NLP research community should“direct efforts towards increasing the size of annotated data collections”,since large amounts of annotated data are likely to significantly impact the performance of current algorithms.For instance,supervised part of speech tagging on English requires about3million words,each of them annotated with their corresponding part of speech,to achieve a performance in the range of94-96%.State-of-the-art in syntactic parsing in English is close to 88-89%(Collins96),obtained by training parser mod-els on a corpus of about600,000words,manually parsed within the Penn Treebank project,an annota-tion effort that required2man-years of work(Mar-cus et al.93).Increased level of problem complexity results in increasingly severe data bottlenecks.The data created so far for supervised English sense dis-ambiguation consist of tagged examples for about200 ambiguous words.At a throughput of one tagged ex-ample per minute(Edmonds00),with a requirement of about500tagged examples per word(Ng&Lee 96),and with20,000ambiguous words in the common English vocabulary,this leads to about160,000hours of tagging–nothing less but80man-years of human annotation rmation extraction,anaphora resolution,and other tasks also strongly require large annotated corpora,which often are not available,or can be found only in limited quantities. Moreover,problems related to lack of annotated data multiply by an order of magnitude when lan-guages other than English are considered.The study of a new language(according to a recent article in the Scientific American(Gibbs02),there are7,200dif-ferent languages spoken worldwide)implies a simi-lar amount of work in creating annotated corpora re-quired by the supervised applications in the new lan-guage.In this paper,we describe a framework for unsu-pervised corpus annotation,applicable to ambiguity problems where classes of equivalence can be de-fined over the set of words in a lexicon.Part of speech tagging,word sense disambiguation,named entity disambiguation,are examples of such applica-tions,where the same tag can be assigned to a set of words.In part of speech tagging,for instance, an equivalence class can be represented by the set of words that have the same functionality(e.g.noun).In word sense disambiguation,equivalence classes are formed by words with similar meaning(synonyms). The only requirements for this algorithm are a lexicon that defines the possible tags that a word might have, which is often readily available or can be build with minimal human effort,and a large raw corpus.The underlying idea is based on the distinction be-tween ambiguous and non-ambiguous words,and the knowledge that can be induced from the latter to the former via classes of equivalence.When building lex-ically annotated corpora,the main problem is repre-sented by the words that,according to a given lexi-con,have more than one possible tag.These words are ambiguous for the specific NLP problem.For in-stance,“work”is morphologically ambiguous,since it can be either a noun or a verb,depending on the context where it occurs.Similarly,“plant”carries on a semantic ambiguity,having both meanings of“fac-tory”or“living organism”.Nonetheless,there are also words that carry only one possible tag,which are non-ambiguous for the given NLP problem.Since there is only one possible tag that can be assigned, the annotation of non-ambiguous words can be accu-rately performed in an automatic fashion.Our method for unsupervised natural language disambiguation re-lies precisely on this latter type of words,and on the equivalence classes that can be defined among words with similar tags.Shortly,for an ambiguous word W,an attempt is made to identify one or more non-ambiguous words W’in the same class of equivalence,so that W’can be annotated in an automatic fashion.Next,lexical knowledge is induced from the non-ambiguous words W’to the ambiguous words W using classes of equiv-alence.The knowledge induction step is performed using a learning mechanism,where the automatically partially tagged corpus is used for training to annotate new raw texts including instances of the ambiguous word W.The paper is organized as follows.Wefirst describe the main algorithms explored so far in semi-automatic construction of annotated corpora.Next,we present our unsupervised approach for building lexically an-notated corpora,and show how knowledge can be induced from non-ambiguous words via classes of equivalence.The method is evaluated on two natural language disambiguation tasks in several languages: part of speech tagging for English,Swedish,and Chi-nese,and word sense disambiguation for English and Romanian.2Related WorkSemi-automatic methods for corpus annotation as-sume the availability of some labeled examples,which can be used to generate models for reliable annotation of new raw data.2.1Active LearningTo minimize the amount of human annotation effort required to construct a tagged corpus,the active learn-ing methodology has the role of selecting for annota-tion only those examples that are the most informa-tive.While active learning does not eliminate the need of human annotation effort,it reduces significantly the amount of annotated training examples required to achieve a certain level of performance. According to(Dagan et al.95),there are two main types of active learning.Thefirst one uses member-ships queries,in which the learner constructs exam-ples and asks a user to label them.In natural language processing tasks,this approach is not always appli-cable,since it is hard and not always possible to con-struct meaningful unlabeled examples for training.In-stead,a second type of active learning can be applied to these tasks,which is selective sampling.In this case,several classifiers examine the unlabeled data and identify only those examples that are the most in-formative,that is the examples where a certain level of disagreement is measured among the classifiers.In natural language processing,active learning was successfully applied to part of speech tagging(Dagan et al.95),text categorization(Liere&Tadepelli97), semantic parsing and information extraction(Thomp-son et al.99).2.2Co-trainingStarting with a set of labeled data,co-training al-gorithms,introduced by(Blum&Mitchell98),at-tempt to increase the amount of annotated data using some(large)amounts of unlabeled data.Shortly,co-training algorithms work by generating several classi-fiers trained on the input labeled data,which are then used to tag new unlabeled data.From this newly anno-tated data,the most confident predictions are sought, which are subsequently added to the set of labeled data.The process may continue for several iterations. Co-training was applied to statistical parsing (Sarkar01),reference resolution(Mueller et al.02), part of speech tagging(Clark et al.03),statisti-cal machine translation(Callison-Burch02),and oth-ers,and was generally found to bring improvement over the case when no additional unlabeled data are used.However,as noted in(Pierce&Cardie01),co-training has some limitations:too little labeled data yield classifiers that are not accurate enough to sus-tain co-training,while too many labeled examples re-sult in classifiers that are“too accurate”,in the sense that only little improvement is achieved by using ad-ditional unlabeled data.2.3Self-trainingWhile co-training(Blum&Mitchell98)and itera-tive classifier construction(Yarowsky95)have beenlong considered to be variations of the same algo-rithm,they are however fundamentally different(Ab-ney02).The algorithm proposed in(Yarowsky95) starts with a set of labeled data(seeds),and builds a classifier,which is then applied on the set of unlabeled data.Only those instances that can be classified with a precision exceeding a certain minimum threshold are added to the labeled set.The classifier is then trained on the new set of labeled examples,and the process continues for several iterations.As pointed out in(Abney02),the main difference between co-training and iterative classifier construc-tion consists in the independence assumptions under-lying each of these algorithms:while the algorithm from(Yarowsky95)relies on precision independence, the assumption made in co-training consists in view independence.Our own experiments in semi-supervised genera-tion of sense tagged data(Mihalcea02)have shown that self-training can be successfully used to bootstrap relatively small sets of labeled examples into large sets of sense tagged data.2.4Counter-trainingCounter-training was recently proposed as a form of bootstrapping for classification problems where learn-ing is performed simultaneously for multiple cate-gories,with the effect of steering the bootstrapping process from ambiguous instances.The approach was applied successfully in learning semantic lexi-cons(Thelen&Riloff02),(Yangarber03).3Equivalence Classes for BuildingAnnotated CorporaThe method introduced in this paper relies on classes of equivalence defined among ambiguous and non-ambiguous words.The method assumes the availabil-ity of:(1)a lexicon that lists the possible tags a word might have,and(2)a large raw corpus.The algorithm consists of the following three main steps:1.Given a set of possible tags,and a lexiconwith words,i=1,,each word admit-ting the tags,j=1,,determine equivalence classes,j=1,containing all words that ad-mit the tag.2.Identify in the raw corpus all instances of wordsthat belong to only one equivalence class.These are non-ambiguous words that represent the starting point for the annotation process.Eachsuch non-ambiguous word is annotated with the corresponding tag from.3.The partially annotated corpus from step2isused to learn the knowledge required to annotate ambiguous words.Equivalence relations defined by the classes of equivalence are used to de-termine ambiguous words that are equivalent to the already annotated words.A label is as-signed to each such ambiguous word by applying the following steps:(a)Detect all classes of equivalence that in-clude the word.(b)In the corpus obtained at step2,find all ex-amples that are annotated with one of thetags.(c)Use the examples from the previous step toform a training set,and use it to classify thecurrent ambiguous instance.For illustration,consider the process of assigning a part of speech label to the word“work”,which may assume one of the labels NN(noun)or VB(verb). We identify in the corpus all instances of words that were already annotated with one of these two labels. These instances constitute training examples,anno-tated with one of the classes NN or VB.A classifier is then trained on these examples,and used to automat-ically assign a label to the current ambiguous word “work”.The following sections detail on the type of features extracted from the context of a word to create training/test examples.3.1Examples of Equivalence Classes in NaturalLanguage DisambiguationWords can be grouped into various classes of equiva-lence,depending on the type of language ambiguity. Part of Speech TaggingA class of equivalence is constituted by words that have the same morphological functionality.The gran-ularity of such classes may vary,depending on spe-cific application requirements.Corpora can be anno-tated using coarse tag assignments,where an equiv-alence class is constructed for each coarse part of speech tag(verb,noun,adjective,adverb,and the other main close-class tags).Finer tag distinctions are also possible,where for instance the class of plural nouns is separated from the class of singular nouns. Examples of suchfine grained classes of morphologi-cal equivalence are listed below:=cat,paper,work=men,papers=work,be,create=lists,works,is,causesWord Sense DisambiguationWords with similar meaning are grouped in classes of semantic equivalence.Such classes can be de-rived from readily available semantic networks like WordNet(Miller95)or EuroWordNet(V ossen98). For languages that lack such resources,the synonymy relations can be induced using bilingual dictionaries (Nikolov&Petrova00).The granularity of the equiv-alence classes may vary from near-synonymy,to large abstract classes(e.g.artifact,natural phenomenon, etc.)For instance,the followingfine grained classes of semantic equivalence can be extracted from Word-Net:=car,auto,automobile,machine,motorcar =mother,female parent=begin,get,start out,start,set about,set out,commenceNamed entity taggingEquivalence classes group together words that rep-resent similar entities(anization,person,lo-cation,and others).A distinction is made between named entity recognition,which consists in labeling new unseen entities,and named entity disambigua-tion,where entities that allow for more than one pos-sible tag(s that can represent a person or an organization)are annotated with the corresponding tag,depending on the context where they occur. Starting with a lexicon that lists the possible tags for several entities,the algorithm introduced in this paper is able to annotate raw text,by doing a form of named entity disambiguation.A named entity recog-nizer can be then trained on this annotated corpus,and subsequently used to label new unseen instances.4EvaluationThe method was evaluated on two natural language ambiguity problems.Thefirst one is a part of speech tagging task,where a corpus annotated with part of speech tags is automatically constructed.The annota-tion accuracy of a classifier trained on automatically labeled data is compared against a baseline that as-signs by default the most frequent tag,and against the accuracy of the same classifier trained on manually labeled data.The second task is a semantic ambiguity problem, where the corpus construction method is used to gen-erate a sense tagged corpus,which is then used to train a word sense disambiguation algorithm.The performance is again compared against the baseline, which assumes by default the most frequent sense, and against the performance achieved by the same dis-ambiguation algorithm,trained on manually labeled data.The precisions obtained during both evaluations are comparable with their alternatives relying on manu-ally annotated data,and exceed by a large margin the simple baseline that assigns to each word the most fre-quent tag.Note that this baseline represents in fact a supervised classification algorithm,since it relies on the assumption that frequency estimates are available for tagged words.Experiments were performed on several languages. The part of speech corpus annotation task was tested on English,Swedish,and Chinese,the sense annota-tion task was tested on English and Romanian.4.1Part of Speech TaggingThe automatic annotation of a raw corpus with part of speech tags proceeds as follows.Given a lexicon that defines the possible morphological tags for each word,classes of equivalence are derived for each part of speech.Next,in the raw corpus,we identify and tag accordingly all the words that appear only in one equivalence class(i.e.non-ambiguous words).On av-erage(as computed over several runs with various cor-pus sizes),about75%of the words can be tagged at this ing the equivalence classes,we identify ambiguous words in the corpus,which have one or more equivalent non-ambiguous words that were al-ready tagged in the previous stage.Each occurrence of such non-ambiguous equivalents results in a train-ing example.The training set derived in this way is used to classify the ambiguous instances.For this task,a training example is formed using the following features:(1)two words to the left and one word to the right of the target word,and their corre-sponding parts of speech(if available,or“?”other-wise);(2)aflag indicating whether the current word starts with an uppercase letter;(3)aflag indicating whether the current word contains any digits;(4)the last three letters of the current word.For learning,we use a memory based classifier(Timbl(Daelemans et al.01)).For each ambiguous word defined in the lexi-con,we determine all the classes of equivalenceto which it belongs,and identify in the training set all the examples that are labeled with one of the tags.The classifier is then trained on these examples, and used to assign one of the labels to the current instance of the ambiguous word.The unknown words(not defined in the lexicon)are labeled using a similar procedure,but this time assum-ing that the word may belong to any class of equiva-lence defined in the lexicon.Hence,the set of train-ing examples is formed with all the examples derived from the partially annotated corpus.The unsupervised part of speech annotation is eval-uated in two ways.First,we compare the annotation accuracy with a simple baseline,that assigns by de-fault the most frequent tag to each ambiguity class. Second,we compare the accuracy of the unsuper-vised method with the performance of the same tag-ging method,but trained on manually labeled data.In all cases,we assume the availability of the same lex-icon.Experiments and comparative evaluations are performed on English,Swedish,and Chinese.4.1.1Part of Speech Tagging for EnglishFor the experiments on English,we use the Penn Treebank Wall Street Journal part of speech tagged texts.Section60,consisting of about22,000tokens, is set aside as a test corpus;the rest is used as a source of text data for training.The training corpus is cleaned of all part of speech tags,resulting in a raw corpus of about3million words.To identify classes of equivalence,we use a fairly large lexicon consist-ing of about100,000words with their corresponding parts of speech.Several runs are performed,where the size of the lexically annotated corpus varies from as few as 10,000tokens,up to3million tokens.In all runs,for both unsupervised or supervised algorithms,we use the same lexicon of about100,000words.Training Evaluation on test setsize manually0(baseline)88.37%92.17%92.78%93.31%93.31%93.52%Table1:Corpus size,and precision on test set using automatically or manually tagged training data(En-glish)Table1lists results obtained for different training sizes.The table lists:the size of the training cor-pus,the part of speech tagging precision on the test data obtained with a classifier trained on(a)automat-ically labeled corpora,or(b)manually labeled cor-pora.For a3million words corpus,the classifier rely-ing on manually annotated data outperforms the tag-ger trained on automatically constructed examples by 2.3%.There is practically no cost associated with the latter tagger,other than the requirement of obtaining a lexicon and a raw corpus,which eventually pays off for the slightly smaller performance.4.1.2Part of Speech Tagging for SwedishFor the Swedish part of speech tagging experiment, we use text collections ranging from10,000words up to to1million words.We use the SUC corpus (SUC02),and again a lexicon of about100,000words. The tagset is the one defined in SUC,and consists of 25different tags.As with the previous English based experiments, the corpus is cleaned of part of speech tags,and run through the automatic labeling procedure.Table 2lists the results obtained using corpora of various sizes.The accuracy continues to grow as the size of the training corpus increases,suggesting that larger corpora are expected to lead to higher precisions.Training Evaluation on test setsize manually0(baseline)83.07%87.28%88.43%89.20%90.02%Table2:Corpus size,and precision on test set us-ing automatically or manually tagged training data (Swedish)4.1.3Part of Speech Tagging for ChineseFor Chinese,we were able to identify only a fairly small lexicon of about10,000entries.Similarly,the only part of speech tagged corpus that we are aware of does not exceed100,000tokens(the Chinese Tree-bank(Xue et al.02)).All the comparative evalua-tions of tagging accuracy are therefore performed on limited size corpora.Similar with the previous ex-periments,about10%of the corpus was set aside for testing.The remaining corpus was cleaned of part of speech tags and automatically labeled.Training on 90,000manually labeled tokens results in an accuracy of87.5%on the test ing the same training corpus,but automatically labeled,leads to a perfor-mance on the same test corpus of82.05%.In an-other experiment,we increase the corpus size to about 2million words,using the segmented Chinese cor-pus made publicly available by(Hockenmaier&Brew 98).The corpus is then automatically labeled with part of speech tags,and used as additional training data,resulting in a precision of87.05%on the same test set.The conclusion drawn from these three experiments is that non-ambiguous words represent a useful source of knowledge for the task of part of speech tagging. The results are comparable with previously explored methods in unsupervised part of speech tagging:(Cut-ting et al.92)and(Brill95)report a precision of95-96%for part of speech tagging for English,using un-supervised annotation,under the assumption that all words in the test set are known.Under a similar as-sumption(i.e.all words in the test set are included in the lexicon),the performance of our unsupervised approach raises to95.2%.4.2Word Sense DisambiguationThe annotation method was also evaluated on a word sense disambiguation problem.Here,the equivalence classes consist of words that are semantically related. Such semantic relations are often readily encoded in semantic networks,e.g.WordNet or EuroWordNet, can be induced using bilingual dictionaries(Nikolov &Petrova00).First,one or more non-ambiguous equivalents are identified for each possible meaning of the ambiguous word considered.For instance,the noun“plant”,with the two meanings of“living organism”and“man-ufacturing plant”,has the monosemous equivalents “flora”and“industrial plant”.Next,the monosemous equivalents are used to ex-tract several examples from a raw textual corpus, which constitute training examples for the semantic annotation task.The feature set used for this task con-sists of a surrounding window of two words to the left and right of the target word,the verbs before and after the target word,the nouns before and after the tar-get word,and sense specific keywords.Similar with the experiments on part of speech tagging,we use the Timbl memory based learner.The performance obtained with the automatically tagged corpus is evaluated against:(1)a simple base-line,which assigns by default the most frequent sense (as determined from the training corpus);and(2)a su-pervised method that learns from manually annotated corpora(the performance of the supervised method is estimated through ten-fold cross validations)Most Disambig.precisionfreq.Training corpuscorpus size auto.10710792.52%2009571.57%20020075.62%20020080.59%20018869.14%10020063.69%18417176.60%1The manually annotated corpus for these words is available from /˜rada/downloads.html4.2.2Word Sense Disambiguation for Romanian Since a Romanian WordNet is not yet available, monosemous equivalents forfive ambiguous words were hand-picked by a native speaker using a paper-based dictionary.The raw corpus consists of a collec-tion of Romanian newspapers collected on the Web over a three years period(1999-2002).The monose-mous equivalents are used to extract several examples, again with a surrounding window of4sentences.An interesting problem that occurred in this task is the presence of gender,which may influence the classifi-cation decision.To avoid possible miss-classifications due to gender mismatch,the native speaker was in-structed to pick the monosemous equivalents such that they all have the same gender(which is not necessar-ily the gender of their equivalent ambiguous word). Table4lists thefive ambiguous words,their monosemous equivalents,the size of the training cor-pus automatically generated,and the precision ob-tained on the test set using the simple most fre-quent sense heuristic and the instance based classi-fier.Again,the classifier trained on the automatically labeled data exceeds by a large margin the simple heuristic that assigns the most frequent sense by de-fault.Since the size of the test set created for these words is fairly small(50examples or less for each word),the performance of a supervised method could not be estimated.Most freq.size precision volum(book/quantity)52.85%20080.00% canal(channel/tube)69.62%6783.3% vas(container/ship)60.9%A VERAGE61.63%Table4:Corpus size,disambiguation precision using most frequent sense,and using automatically sense tagged data(Romanian)5ConclusionThis paper introduced a framework for unsupervised natural language disambiguation,applicable to ambi-guity problems where classes of equivalence can be defined over the set of words in a lexicon.Lexical knowledge is induced from non-ambiguous words via classes of equivalence,and enables the automatic gen-eration of annotated corpora.The only requirements are a dictionary and a raw textual corpus.The method was tested on two natural language ambiguity tasks,on several languages.In part of speech tagging,clas-sifiers trained on automatically constructed training corpora performed at accuracies in the range of88-94%,depending on training size,comparable with the performance of the same tagger when trained on man-ually labeled data.Similarly,in word sense disam-biguation experiments,the algorithm succeeds in cre-ating semantically annotated corpora,which enable good disambiguation accuracies.In future work,we plan to investigate the application of this algorithm to very,very large corpora(Banko&Brill01),and eval-uate the impact on disambiguation performance.AcknowledgmentsThanks to Sofia Gustafson-Capkov´a for making avail-able the SUC corpus,and to Li Yang for his help with the manual sense annotations.References(Abney02)S.Abney.Bootstrapping.In Proceedings of the40st Annual Meeting of the Association for Compu-tational Linguistics ACL2002,pages360–367,Philadel-phia,PA,July2002.(Banko&Brill01)M.Banko and E.Brill.Scaling to very very large corpora for natural language disam-biguation.In Proceedings of the39th Annual Meeting of the Association for Computational Lingusitics(ACL-2001),Toulouse,France,July2001.(Blum&Mitchell98)A.Blum and -bining labeled and unlabeled data with co-training.In COLT:Proceedings of the Workshop on Computational Learning Theory,Morgan Kaufmann Publishers,1998.(Brill95)E.Brill.Unsupervised learning of disambigua-tion rules for part of speech tagging.In Proceedings of the ACL Third Workshop on Very Large Corpora,pages 1–13,Somerset,New Jersey,1995.(Callison-Burch02)C.Callison-Burch.Co-training for statistical machine translation.Unpublished M.Sc.the-sis,University of Edinburgh,2002.(Clark et al.03)S.Clark,J.R.Curran,and M.Osborne.Bootstrapping pos taggers using unlabelled data.In Walter Daelemans and Miles Osborne,editors,Proceed-ings of CoNLL-2003,pages49–55.Edmonton,Canada, 2003.(Collins96)M.Collins.A new statistical parser based on bigram lexical dependencies.In Proceedings of the34th Annual Meeting of the ACL,Santa Cruz,1996.(Cutting et al.92)D.Cutting,J.Kupiec,J.Pedersen,and P.Sibun.A practical part-of-speech tagger.In Proceed-ings of the Third Conference on Applied Natural Lan-guage Processing ANLP-92,1992.(Daelemans et al.01)W.Daelemans,J.Zavrel,K.van der Sloot,and A.van den Bosch.Timbl:Tilburg memory based learner,version4.0,reference guide.Technical report,University of Antwerp,2001.。