Extracting and evaluating general world knowledge from the Brown corpus
天然植物中有效成分的提取、分离技术研究进展

第33卷第1期内蒙古民族大学学报(自然科学版)Vol.33No.1 2018年1月Journal of Inner Mongolia University for Nationalities Jan.2018DOI:10.14045/ki.15-1220.2018.01.004天然植物中有效成分的提取、分离技术研究进展周佳儒1,李久明1,2,3,徐宁1,春英1,敖亮亮1(1.内蒙古民族大学化学化工学院国家级实验教学示范中心,内蒙古通辽028043;2.内蒙古自治区高校蓖麻产业工程技术研究中心,内蒙古通辽028000;3.内蒙古自治区天然产物化学及功能分子合成重点实验室,内蒙古通辽028000)〔摘要〕归纳了目前天然植物有效成分的提取分离和纯化技术中广泛应用的技术,主要分析了以下八种提取分离技术:超临界流体萃取技术(SFE),微波萃取技术(MAE),超声波提取技术(UE),生物酶提取技术,膜分离技术,大孔树脂吸附分离技术,高速逆流色谱分离技术(HSCCC)以及分子印迹分离技术(MIT),重点分析这八种现代分离技术在天然植物中的应用,系统地介绍了现代提取技术的基本原理、特点、适用范围并举例说明了现代提取技术在天然产物的提取分离中的研究进展和发展趋势.〔关键词〕天然植物;有效成分;提取;分离;应用〔中图分类号〕TQ28〔文献标识码〕A〔文章编号〕1671-0185(2018)01-0014-05Progress in Extraction and Separationof Effective Components from Natural PlantsZHOU Jia-ru1,LI Jiu-ming1,2,3,XU Ning1,3,Chunying1,AO Liang-liang1(1.College of Chemistry and Chemical Engineering,Inner Mongolia University for Nationalities,Tongliao028043,China;2.Inner Mongolia Industrial Engineering Research Center of Universities forCastor,Tongliao028000,China;3.Inner Mongolia Key Laboratory for the Natural Products Chemistry andFunctional Molecular Synthesis,Tongliao028000,China)Abstract:New methods of separation and purification technology of bioactive components in natural products arepresented.These include eight kinds of extraction and separation technologies:supercritical fluid extraction(SFE),microwave extraction(MAE),ultrasonic extraction(UE),enzyme extraction,membrane separation,centrifugalseparation and macroporous resin adsorption separation,high-speed counter-current chromatography separation(HSCCC)and separation of molecular imprinting(MIT).The application of these eight modern separation tech-niques is analyzed,systematically introducing the basic principle of modern extraction technology,characteristics,scope of application and illustrating the research progress and development trend in the extraction and separation ofnatural products.Key words:Natural plant;Active ingredients;Extraction;Separation;Application自然界中天然植物的种类很多,都含有多种有效而又复杂的化学成分.这些成分可分为有机酸、挥发油、香豆素、甾体类、苷类、生物碱、糖类、植物色素等.植物中有效成分的提取分离技术是根据植物中有效成分在不同条件下的存在状态、形状、溶解性等物理和化学性质来确定的,目前被广泛使用的分离技术可分为膜分离法和传统分离法两种,传统分离法又分为水蒸气蒸馏法、升华法、冷浸法、沉淀法、渗漉法、煎基金项目:内蒙古自治区高校蓖麻产业工程技术研究中心开放基金(MDK2016001);通辽市与内蒙古民族大学科技合作项目(SXYB2012049);内蒙古民族大学国家基金培育项目(NMDGP1503);内蒙古民族大学研究生科研立项项目(NMDSS1752)作者简介:周佳儒,内蒙古民族大学化学化工学院硕士研究生.李久明为通讯作者.第1期周佳儒等:天然植物中有效成分的提取、分离技术研究进展15煮法、索氏提取法等.这些分离方法都具有一定的局限性,如效率低、溶剂用量大、操作复杂、提取时间长等.随着现代科学技术的飞速发展,一些新型提取分离技术应时而生,如超声波萃取技术(SE)、超临界萃取技术(SFE)、微波萃取技术(MAE)、大孔树脂吸附分离技术、生物酶解技术、分子印迹分离技术(MIT)、高速逆流色谱分离技术(HSCCC)等.本论文对目前从天然植物中提取分离有效成分的一些新技术和方法进行了评价和综述,并分析了今后天然植物有效成分提取分离技术的研究动态和发展趋势.1提取、分离纯化新技术1.1超临界流体萃取技术(SFE)超临界流体萃取技术是20世纪60年代兴起的一种新型分离技术,该技术所用的萃取剂是超临界流体(SF).某一特定的物质存在一个临界点,在临界点以上物质处于介于气液之间的一种非气态又非液态的物态,这个范围之内的物质称为超临界流体(SF).通常以二氧化碳作为超临界流体,因为二氧化碳具有无色、无毒、无腐蚀性、化学惰性、使用安全、廉价等优点.超临界流体萃取技术基本特点有以下几个方面:①萃取时间快、生产周期短,一般萃取20min便有成分分离出来,2.0~4.0h便可完全萃取.②超临界流体二氧化碳萃取操作的参数易控制,因此,所萃取的产物质量比较稳定.③萃取能力强,萃取率高.④超临界流体二氧化碳萃取操作温度低(30~70℃)能较好的保留中药有效成分.⑤在萃取过程中不使用任何有机溶剂,这样生产的产品无有害溶剂残留.利用超临界CO2流体萃取技术可从药用植物中大量提取有效成分,尤其对脂肪酸、植物碱、醚类、酮类、甘油酯等具有特殊溶解作用.20世纪70年代末,日本的研究小组采用此法从药用植物苍术、黄连、蛇床子和菌陈蒿等植物中提取多种有效成分.相关研究资料显示用超临界流体CO2萃取技术从青蒿中提取分离出来蒿素、十八醇等有效成分,提取率比传统的溶剂法提高了10%~60%,提取时间明显缩短,同时降低了成本〔1-2〕.Manuel A.Falcão等〔3〕在压力为300bar的条件下,以乙醇作为辅助剂从长春花中提取具有抗肿瘤作用的长春碱,这种方法的提取率高达92%,提取效率远高于传统的固-液萃取法.超临界流体萃取技术除了在中草药有效成分的提取方面有着明显的优势外,也可应用于其它领域.虽然SFE设备有压力高、投资大等缺点,但随着工业技术的发展,超临界流体萃取技术在实际生产中的应用也在逐步扩大.1.2微波萃取技术(MAE)所谓微波萃取技术是利用微波能来提高提取率的一种新型技术,目前微波提取大多应用于水提、醇提等的项目.微波萃取主要有以下基本特点:①微波提取物纯度高,可采用水、醇、酯等常用溶剂进行提取,适用范围广.②溶剂量少(比常规法少50%~90%).③由于微波采取穿透式加热,大大缩短了提取时间.微波提取设备可在几十分钟内完成常规的多功能萃取罐8.0h的提取工作,节省时间达到90%.④微波能有超强的提取能力,同样的原料在微波场下仅一次就可提净,而常规法则需多次才可提净,简化了工艺流程.⑤微波萃取更易于控制,能够实现即时停止和加热.微波萃取技术在天然植物提取方面有重要的作用.此方法在提高生产率和萃取物纯度的同时又能降低萃取时间、能源及溶剂的消耗,又可降低废物的产生,是一种具有良好发展前途的新工艺.由于微波加热是一种“体加热”方式,是内外同时加热,因此在萃取时加热均匀,且升温迅速,在提高萃取效率同时显著缩短了萃取时间〔4〕.采用微波技术从羽扇豆种子中提取金雀花碱,提取率比传统的提取法提高了20%,在缩短了提取时间同时减少了溶剂用量〔5〕.用微波提取甘草黄酮,提取时间为1min,提取量为24.6g/L,而水提法的提取时间为5h,提取量为11.4g/L,大大缩短了提取时间,提高了提取量〔6〕.1.3超声波提取技术(UE)超声波提取技术是利用超声波振动作用来强化提取植物中的有效成分,超声波是频率高于20kHz 的声波.超声波可在提取过程中产生“空化效应”,超声波的机械作用可有效地破碎植物的细胞壁,使有效成分快速地溶解在溶剂中,超声提取药材不受成分极性、分子量大小的限制,适用于绝大多数种类中药材和各类成分的提取;另一方面超声波振动可加速分子运动,使得溶剂和植物中的有效成分快速混合.与传16内蒙古民族大学学报2018年统萃取方式相比,超声波萃取技术用时短、适用性广、效率高.李颖〔7〕等利用超声波技术从银州柴胡中提取挥发性活性成分,同时采用多种溶剂混合冰浴,并应用高分辨气-质联用仪进行分析,鉴定出116种成分.利用超声波法从新鲜橄榄中提取总酚类化合物(TPC),萃取条件为固液比22mL/g,萃取温度47℃,萃取时间30min,提取率为7.01mg/g;而传统的浸渍提取法在50℃,4.7h,固液比为24mL/g的条件下,提取率只有5.18mg/g,因此超声波萃取法能有效增加橄榄中酚类化合物的提取率〔8〕.采用超声提取法从黄连中提取小檗碱,处理30min后所得的小檗碱提取率比采用传统的碱水浸泡法处理24.0h高50%以上〔9〕.采用超声波法提取平菇多糖,提取时间短,能量损耗低,也降低了高温对多糖的破坏〔10〕.采用超声波辅助方法从石榴子中提取石榴籽油,在以石油醚为溶剂,140W、40℃、36min和固液比为10mL/g的条件下,提取率高达25.11%,提取率明显高于传统的索氏提取法(SE,20.50%)〔11〕.黄晓辉等人以95%乙醇为溶剂,用超声波提取法提取水葫芦中的黄酮,在超声温度为45℃,料液比为1:45(g:mL),超声时间为35min,pH为8的条件下,测得黄酮提取率4.51%〔12〕.超声工艺虽应用广泛,但在大规模工业生产中的应用还较少,还有待进一步探索.1.4生物酶提取技术酶是由生物细胞产生的一种具有催化活性的蛋白质.生物酶提取技术是利用酶破坏植物细胞壁的结构,例如纤维素酶可以破坏植物细胞壁中的B-D-葡萄糖链.酶的催化效率高,具有高度的专一性,合适的酶可使植物组织通过酶反应温和地分解,提高有效成分的提取率.用纤维素酶提取黄连中的盐酸小檗碱,未经酶处理的的样品盐酸小檗碱平均含量为2.5%,而经过酶处理后盐酸小檗碱含量为4.2%〔13〕.郭海鹏等〔14〕利用酶提取法,将生物降解细菌产生的酶以1:3(V/V)的比例直接添加到新鲜海藻中处理48.0h,脂质提取产量增加了10.4%~43.9%,生物降解细菌产生的酶可以削弱和破坏藻类的细胞壁,促进藻类中脂质的释放.酶解技术具有操作简单、成本低廉、可大量生产等优点,也存在一定的局限性,虽然酶作用条件温和,但酶的活性受多种因素调节控制,导致实验条件(如温度、pH值及作用时间等)较难控制,使该技术的发展和应用也受到了相应地制约.1.5膜分离技术所谓膜分离是以选择性透过膜为分离介质,当膜两侧存在差异时,原料中组分可透过选择性膜而对混合物进行分离,目前已经研发出了多种膜分离工艺如超滤、纳滤、电渗析、渗透汽化和气体分离等.膜分离是一种新型的分离方法,与传统的分离方式相比,具有节能、单级分离效率高、环保、无相态变化、过滤过程简单等优点.由于膜分离过程中不需要进行加热,因此,该技术特别适用于分离对热敏感的物质.传统的水净化膜分离过程需要消耗大量的能量,但纳米膜分离过程能消耗的能量更少,消除了传统工艺的局限性.这种纳米膜可以除去水中的有机污染物同时对水进行消毒,简化了后续的工艺流程,一些纳米分离膜已经在水净化领域实现商业化〔15〕.相关的研究数据显示,使用超滤中空纤维膜分离分散染料等不溶性染料可达99%的脱色率,透过液可作为中性水可再循环利用,降低了成本同时也减少了环境污染〔16〕.董洁等〔17〕对黄连解毒汤模拟体系的超滤膜过程进行了分析,截留分子质量为5kDa的聚砜超滤膜对黄连解毒汤模拟溶液中药效物质小檗碱和栀子苷的透过率在90%以上,淀粉、果胶、蛋白质三种高分子物质的截留率为100%,能满足中药材纯化、精制的要求.随着膜分离技术的不断发展,它的巨大作用定会在未来的工业发展中显现出来,它将在人类工业的发展史上起到重要作用.1.6大孔树脂吸附分离技术大孔树脂又称全多孔树脂,其化学性质稳定,难溶于酸、碱及各种有机溶剂.大孔树脂现已广泛应用于医药、环保和食品等领域,尤其20世纪70年代末,被广泛应用于中草药研究的各个方面.该方法具有所需溶剂量少、可重复使用、操作方便、生产周期短、产品纯度高及不吸潮等优点,在各领域的应用日益广泛.应用XAD-7HP树脂纯化桑椹花青素提取物,相比于其他树脂,XAD-7HP有较高吸附/解吸能力,它的吸附容量为3.57mg/g,而吸附率及解吸率分别为86.45%和80.81%,用40%乙醇洗脱XAD-7HP纯度可达到93.6%,该方法可用于从水果以及其他植物中制备高纯度桑椹花青素〔18〕.大孔树脂吸附技术也有一些缺第1期周佳儒等:天然植物中有效成分的提取、分离技术研究进展17点的不足,如制备时需要加入一些由有机溶剂组成的致孔剂,而有机溶剂大多具有毒性,会残留在树脂的空隙中.如果不能清除残留的致孔剂,在长期使用的过程中会遇到树脂降解的问题.但目前我国已经探索出了树脂使用前对致孔剂、降解物的处理方法,已经通过了国家药品监督管理局的审评.1.7高速逆流色谱分离技术(HSCCC)高速逆流色谱技术(High-Speed Countercurrent Chromatography,HSCCC)是1982年由美国国立卫生院Ito博士研制开发的一种新型无固相载体的连续液-液色谱技术.它不用任何固态的支撑物或载体.目前HSCCC法的优点有以下几点:a.操作简便,容易掌握;b.应用范围很广;C.无需固体载体;d.重现性好,产品纯度高;e.适用于制备型分离.采用HSCCC法从萝卜籽中分离具有药理活性的萝卜籽素,选用正己烷-乙酸乙酯-甲醇-水(35:100:35:100,V/V/V/V)为两相溶剂体系.在分离柱转速为800rpm、流动相流动速率和分离温度分别为2mL/min和30℃的条件下,从约1000mg提取物中一步分离得到249.4mg的纯萝卜籽素.得到的萝卜籽素纯度达96.9%.这为后续的药理活性研究提供了较好的基础〔19〕.应用HSCCC法从山楂提取物中分离茶多酚(Crataeguslaevigata),将500mg的粗提物引入高速逆流色谱系统在260min后获得了超过9.7mg的产品,纯度为94.8%.因此,高速逆流色谱分离技术可以用来从天然植物中分离和纯化茶多酚〔20〕.该技术也成功应用于紫胶红酸、花青素等天然食用色素的提取分离和纯化〔21〕上.高速逆流色谱技术在天然产物方面有突出的作用,随着科学的发展,高速逆流色谱分离技术的应用范围也逐渐扩大.1.8分子印迹分离技术(MIT)分子印迹技术(Molecular imprinting technology)是一种在高度交联、刚性的聚合物母体中引入特定分子结合位点的技术.Southern在1975年首先提出了分子印迹的概念.MIT是一种有效、简便的分离技术,同时具有耐热、耐有机溶剂、耐酸碱的优点以及制备简单、可重复使用等特点.用环糊精分子印迹聚合物分离纯化葛根素,纯度可达到98%,收率高达80%以上,而传统的方法则需要6步才可完成,收率仅为10%左右,纯度也并不理想〔22〕.一种以半印迹方法合成的印记聚合物,可以从药用真菌干粉菌体的提取液中提取出麦角甾醇〔23〕.由于其具有特殊的识别功能,可以用来分离混合物,由于印迹聚合物在有机溶剂与水溶液中都可使用,与传统方法相比,具有独特的优点,随着科学的发展,未来分子印迹分离技术在天然植物有效成分提取分离研究中也会发挥越来越大的作用.2结语本文对分离植物活性成分中常用的八种提取和分离技术进行了比对和分析,这八种方法在分离不同物质时都有各自的特点和优势,但由于植物结构具有复杂性和差异性,上述八种常用提取、分离技术都有其各自的适用性和局限性,因此,对不同方法的应用范围也会有所不同〔24〕.一些新型技术在某些提取过程中分离效果比传统方法更具优势.但随着科技的全面发展,传统提取方法和上述的八种较新的提取分离技术必将共同发展,甚至还会在互补交叉的过程中诞生更方便快捷的分离技术〔25〕.在科研、生产实践中还需具体问题具体分析,才可以挑选出最佳的提取方式,不可盲目选择.未来天然植物有效成分提取技术会更加安全、高效、环保,天然植物中有效成分得以在更多领域中发挥作用.在生活中,随着人民生活水平的提高,回归自然的理念日益增强,天然提取物行业下游的食品添加剂、营养保健品、化妆品、饲料等行业都日益趋向绿色环保.天然、无污染的绿色产品在国内外均有巨大的发展空间和广阔的市场前景,这也必将带动天然植物提取物行业的快速发展.参考文献〔1〕葛发欢,李菁,王海波,等.超临界CO2萃取技术在黄花蒿成分研究中的应用〔J〕.中药材,1994,17(8):31-32.〔2〕葛发欢,史庆龙,谭晓华,等.柠檬桉叶中山鸡椒醇的分离与合成〔J〕.中药材,1997,20(7):345-349.〔3〕Manuel A.Falcão,RodrigoScopel,Rafael N.Almeida,et a1.Supercritical fluid extraction of vinblastine from Catharanthus-roseus〔J〕.The Journal of Supercritical Fluids,2017,129(11):9-15.〔4〕吴龙琴,李克.微波萃取原理及其在中草药有效成分提取中的应用〔J〕.中国药业,2012,21(12):110-112.18内蒙古民族大学学报2018年〔5〕Ganzler K.Microwave Extraction Anoval Sample Preparation Method for Chromatography〔J〕.J Chromatogr A,1986,371: 299-306.〔6〕张梦军,金建锋,李伯玉,等.微波辅助提取甘草黄酮的研究〔J〕.中成药,2002,24(5):334-336.〔7〕李颖,彭建和,宙卫莉,等.银州柴胡的化学成分研究〔J〕.中国野生植物资源,1995(4):1-6.〔8〕Junlin Deng,Zhou Xu,Chunrong Xiang,et parative evaluation of maceration and ultrasonic-assisted extraction of phenolic compounds from fresh olives〔J〕.UltrasonicsSonochemistry,2017,37:328-334〔9〕赵兵,王玉春,欧阳蕾,等.超声波在植物提取中的应用〔J〕.中草药,1999,30(9):1-3.〔10〕张扬,熊耀康.超声波法提取平菇多糖工艺研究〔J〕.中国实用医药,2009,4(29):13.〔11〕Yuting Tian,Zhenbo Xu,Baodong Zheng,et al.Optimization of ultrasonic-assisted extraction of pomegranate(Punicagra-natum L.)seed oil〔J〕.Ultrasonics Sonochemistry,2013,20(1):202-208.〔12〕黄晓辉,茹晶晶,罗五魁,等.超声波法提取水葫芦中黄酮的工艺研究〔J〕.赤峰学院学报(自科学版),2016,32(5): 43-44.〔13〕马桔云,赵晶岩,姜颖,等.纤维素酶在黄连提取工艺中的应用〔J〕.中草药,2000,31(2):103—104.〔14〕HaipengGuo,HoumingChen,LuFan,et al.Enzymes produced by biomass-degrading bacteria can efficiently hydrolyze al-gal cell walls and facilitate lipid extraction〔J〕.Renewable Energy,2017,109:195-201.〔15〕Santanu Sarkar,Ankur Sarkar,ChiranjibBhattacharjee.10-Nanotechnology-based membrane-separation process for drinking water purification〔J〕.Water Purification,2017:355-389.〔16〕吴开芬.用超滤法处理靛兰废水〔J〕.环境科学进展(增刊),1998(6):124-127.〔17〕徐龙泉,彭黔荣,杨敏,等.膜分离技术在中药生产及研究中的应用进展〔J〕.中成药,2013,35(9):1989-1994.〔18〕YaoChen,WeijieZhang,TingZhao,et al.Adsorption properties of macroporous adsorbent resins for separation of anthocy-anins from mulberry〔J〕.Food Chemistry,2016,194:712-722.〔19〕PengqunKuang,DanSong,QipengYuan,et al.Preparative separation and purification of sulforaphene from radish seeds by high-speed countercurrent chromatography〔J〕.Food Chemistry,2013,136(2):309-315.〔20〕Hai-YanCui,Xiao-YanJia,XiaZhang,et al.Optimization of high-speed counter-current chromatography for separation of polyphenols from the extract of hawthorn(Crataeguslaevigata)with response surface methodology〔J〕.Separation and Purification Technology,2011,77(2):269-274.〔21〕陈爱华,杨坚.高速逆流色谱(HSCCC)在食品色素制备中的应用〔J〕.中国食品添加剂,2005(1):83-85.〔22〕贺湘凌,谭天伟,JANSON Jan-Christer.利用β-环糊精键合固定相分离纯化葛根素〔J〕.色谱,2003,21(6): 610-613.〔23〕ShimaN.N.S.Hashim,Lachlan J.Schwarz,BasilDanylecetal.Recovery of ergosterol from the medicinal mushroom,Gano-dermatsugae var.Janniae,with a molecularly imprinted polymer derived from a cleavable monomer-template composite 〔J〕.Journal of Chromatography A,2016,1468:1-9.〔24〕春英,李久明,黄廷廷,等.蓖麻碱的提取及生物活性研究进展〔J〕.内蒙古民族大学学报(自然科学版),2015,30(6):487-489.〔25〕穆莎茉莉,黄凤兰,冀照君,等.蓖麻碱的提取与应用研究进展〔J〕.内蒙古民族大学学报(自然科学版),2012,27(4):463-466.〔责任编辑郑瑛〕。
Tessier 提取法英文版

Talanta46(1998)449–455Extraction procedures for the determination of heavy metals incontaminated soil and sedimentGemma RauretDept.Quı´mica Analı´tica,Uni6ersitat de Barcelona,Barcelona,SpainReceived25May1997;accepted14October1997AbstractExtraction tests are commonly used to study the mobility of metals in soils and sediments by mimicking different environmental conditions or dramatic changes on them.The results obtained by determining the extractable elements are dependent on the extraction procedure applied.The paper summarises state of the art extraction procedures used for heavy metal determination in contaminated soil and sediments.Two types of extraction are considered:single and sequential.Special attention is paid to the Standard,Measurement and Testing projects from the European Commission which focused on the harmonisation of the extraction procedures and on preparing soil and sediment certified reference materials for extractable heavy metal contents.©1998Elsevier Science B.V.All rights reserved. Keywords:Extraction procedures;Heavy metals;Contaminated soil;Sediment;Certified reference materials1.IntroductionTrace metals in soils and sediments may exist in different chemical forms or ways of binding.In unpolluted soils or sediments trace metals are mainly bound to silicates and primary minerals forming relatively immobile species,whereas in polluted ones trace metals are generally more mobile and bound to other soil or sediments phases.In environmental studies the determina-tion of the different ways of binding gives more information on trace metal mobility,as well as on their availability or toxicity,in comparison with the total element content.However,the determi-nation of the different ways of binding is difficult and often impossible.Different approaches are used for soil and sediment analysis,many of them focused on pollutant desorption from the solid phase;others are focused on the pollutant adsorp-tion from a solution by the solid phase.Among those approaches based on desorption,leaching procedures are the most widely accepted and used.Extraction procedures by means of a single extractant are widely used in soil science.These procedures are designed to dissolve a phase whose element content is correlated with the availability of the element to the plants.This approach is well established for major elements and nutrients and it is commonly applied in studies of fertility and quality of crops,for predicting the uptake of essential elements,for diagnosis of deficiency or excess of one element in a soil,in studies of the physical-chemical behaviour of elements in soils0039-9140/98/$19.00©1998Elsevier Science B.V.All rights reserved. PII S0039-9140(97)00406-2G.Rauret/Talanta46(1998)449–455 450and for survey purposes.To a lesser extent they are applied to elements considered as pollutants such as heavy metals.The application of extrac-tion procedures to polluted or naturally contami-nated soils is mainly focused to ascertain the potential availability and mobility of pollutants which is related to soil-plant transfer of pollutants and to study its migration in a soil profile which is usually connected with groundwater problems[1]. For sediment analysis,extraction is used to asses long term emission potential of pollutants and to study the distribution of pollutants among the geochemical phases.As far as heavy metals are concerned sediments are usually a sink but may also become a source under certain condi-tions,especially in heavily contaminated areas or in drastically changing environments.Chemical extraction of sediments has proven to be adequate for determining the metal associated with source constituents in sedimentary deposits[2],but the general aim of many studies involving chemical extraction is the determination of element distri-bution among different phases of a sediment. Single extractants are usually chosen to evaluate a particular release controlling mechanism such as desorption by increasing salinity or complexing by competing organic agents.Generally,fractions can be isolated more specifically by using sequen-tial extraction schemes.For sediments these pro-cedures are frequently used and are designed in relation to the problems arising from disposal of dredged materials.Extraction tests,either in soils and sediments, are always restricted to a reduced group of ele-ments and as far as soil is concerned they are applied to a particular type of soil;silicious,car-bonated or organic.In a regulatory context,two applications for leaching tests can be recognised: the assessment or prediction of the environmental effects of a pollutant concentration in the environ-ment and the promulgation of guidelines or objec-tives for soil quality as for example for land application of sewage sludge or dredge sediments. The data obtained when applying these tests are used for decision makers in topics such as land use of soil or in countermeasures monly used extraction procedures in soils During the last decades several extraction pro-cedures for extractable heavy metals in soils have been developed and modified.In this respect,two groups of tests must be considered:the single reagent extraction test,one extraction solution and one soil sample,and in the sequential extrac-tion procedures,several extraction solutions are used sequentially to the same sample although this last type of extraction is still in development for soils.Both types of extraction are applied using not only different extracting schemes but also different laboratory conditions.This leads to the use of a great deal of extraction procedures. In Table1a summary of the most common leaching test are given.Table1Most common single extraction testsType and solution strength Reference Group[3]HNO30.43–2mol l−1Acid extractionAqua regia[4]HCl0.1–1mol l−1[3]CH3COOH0.1mol l−1[5]Melich1:[6]HCl0.05mol l−1+H2SO40.0125mol l−1EDTA0.01–0.05mols l−1[3] Chelatingagents at different pH[7]DTPA0.005mol l−1+TEA0.1mol l−1CaCl20.01mol l−1Melich3:[8]CH3COOH0.02mol l−1NH4F0.015mol l−1HNO30.013mol l−1EDTA0.001mol l−1NH4–acetate,acetic acidBuffered salt[9]buffer pH=7;1mol l−1solution[3]NH4–acetate,acetic acidbuffer pH=4.8;1mol l−1Unbuffered salt CaCl20.1mol l−1[3]solutionCaCl20.05mol l−1[3][3]CaCl20.01mol l−1NaNO30.1mol l−1[10]NH4NO31mol l−1[3]AlCl30.3mol l−1[11]BaCl20.1mol l−1[12]G.Rauret/Talanta46(1998)449–455451 Table2Extraction methods proposed for standardisation or standardised in some European countriesMethod MethodCountry Reference[15]Mobile trace element determination1mol l−1NH4NO3Germany[16]Available Cu,Zn and Mn evaluation for fer-France0.01mol l−1Na2–EDTA+1mol l−1tilisation purposesCH3COONH4at pH=7DTPA0.005mol l−1+TEA0.1mol l−1+CaCl20.01mol l−1at pH=7.3Available Cu,Zn,Fe and Mn evaluation inItaly0.02mol l−1EDTA+0.5mol l−1[17]acidic soilsCH3COONH4at pH=4.6DTPA0.005mol l−1+TEA0.1mol l−1+CaCl20.01mol l−1at pH=7.3[18]Availability and mobility of heavy metals inCaCl20.1mol l−1Netherlandspolluted soils evaluationSoluble heavy metal(Cu,Zn,Cd,Pb and[19] Switzerland NaNO30.1mol l−1Ni)determination and ecotoxicity risk evalu-ationUnited Kingdom EDTA0.05mol l−1at pH=4[20]Cu availability evaluationFrom Table1it can be observed that a single extraction including a large spectra of extractants are used.It ranges from very strong acids,such as aqua regia,nitric acid or hydrochloric acid,to neutral unbuffered salt solutions,mainly CaCl2or NaNO3.Other extractants such as buffered salt solutions or complexing agents are frequently ap-plied,because of their ability to form very stable water soluble complexes with a wide range of cations.Hot water is also used for the extraction of boron.Basic extraction by using sodium hy-droxide is used to assess the influence of the dissolved organic carbon in the release of heavy metals from soils.A large number of extractants are reviewed by Pickering[13]and Lebourg[14]. The increasing performance of the analytical techniques used for element determination in an extract,together with the increasing evidence that exchangeable metals better correlate with plant uptake,has lead extraction methods to evolve towards the use of less and less aggressive solu-tions[10].These solutions are sometimes called soft extractants and are based on non buffered salt solutions although diluted acids and complex-ant agents are also included in the group.Neutral salts dissolve mainly the cation exchangeable frac-tion although in some cases the complexing ability of the anion can play a certain role.Diluted acids dissolve partially trace elements associated to dif-ferent fractions such as exchangeable,carbonates, iron and manganese oxides and organic matter. Complexing agents dissolve not only exchange-able element fraction but also the element fraction forming organic matter complexes and the ele-ment fractionfixed on the soil hydroxides.Nowa-days it is generally accepted that extractants are not selective and that minor variations in analyti-cal procedures have significant effects on the re-sults.Some leaching procedures for soils have been adopted officially or its adoption is under study in different countries with different objectives[14]. An account of these methods are given on Table 2.monly used extraction procedures in sedimentsAs for soils,exchangeable metal in sediments are selectively displaced by soft extractants.Other extractants used are less selective and they co-ex-tract the exchangeable fraction together with metals bound to different sediment phases moreG.Rauret/Talanta46(1998)449–455 452or less extensively.The phases considered relevant in heavy metals adsorption in sediments are ox-ides,sulphides and organic matter.Fractionation is usually performed by using sequential extrac-tion schemes.The fractions obtained,when apply-ing these schemes,are related to exchangeable metals,metals mainly bound to carbonates, metals released in reducible conditions such as those bound to hydrous oxides of Fe and Mn, metals bonded to oxidable components such as organic matter and sulphides and residual frac-tion.The extractants more commonly used in sequential extraction schemes are generally ap-plied according to the following order:unbuffered salts,weak acids,reducing agents,oxidising agents and strong acids.In Table3the extractants most commonly used to isolate each fraction are given[21].The water soluble fraction may be obtained by two ways,by sampling sediment pore solution using in situ filtration,dialysis tubes or bags,or by a leaching procedure in the laboratory.When this procedure is used the pH may be indeterminate because of the low buffering capacity of the extractant and problems with readsorption occurs.Exchangeable fraction uses an electrolyte such as salts of strong acids and bases or salts of weak acids and bases at pH7to prevent oxyhydroxy phases precipitation. The carbonate bound fraction generally uses an acid such as acetic or a buffer solution acetic acid-sodium acetate at pH5.These reagents are not able to attack all the carbonate content,as for example dolomitic carbonates,neither to attack carbonate selectively as they also remove partially organically bound trace metals.The fraction ob-tained when a reducing solution is used as extrac-tant is mainly related to metals bound to iron and manganese oxides.Hydroxylamine in acid solu-tion is the reducing agent most widely used to solubilise these oxides although iron oxide is not completely dissolved.Ammonium oxalate seems to be most effective when used in the dark,al-though some problems in heavy metals oxalate phase precipitation may occur even at low pH. The sodium dithionite/citrate/carbonate reagent dissolves the oxide and hydroxyoxides but can attack iron rich silicates.So reducing extractants are neither selective nor completely effective for iron and manganese oxides.Other group of ex-tractants used sequentially includes oxidising reagents which destroy organic matter and also oxidises sulphides to sulphates.The extractants most widely used in this group are H2O2and NaOCl.Hydrogen peroxide seems to be more efficient if used after the oxide extraction step. The most widely used extraction scheme is the one proposed by Tessier[22]which has been modified by several authors[23–25].Many of these modifications make more specific the isola-tion of the iron and manganese oxide and hydrox-ide phases.The Tessier procedure is schematised in Table4together with the modified procedures of Fo¨rstner[26]and of Meguelatti[24].4.Harmonisation and method validationOwing to the need of establishing common schemes in Europe for extractable trace metals in soils and sediments the EC Standards,Measure-ment and Testing Programme,formerly BCR(Bu-Table3Most common extractants used in sequential extraction schemesType and solution strenght GroupH2OWater soluble fractionExchangeable and weakly NaNO30.1mol l−1 adsorbed fractionKNO30.1mol l−1MgCl21mol l−1CaCl20.05mol l−1Ca(NO3)20.1mol l−1NH4OAc1mol l−1pH=7 Carbonate bound fraction HOAc0.5mol l−1HOAc/NaOAc1mol l−1pH=5Fractions bound to hydrous NH2OH.HCl0.04mol l−1 oxides of Fe and Mn in acetic or nitric acidNH4OxSodium ditionite,sodiumcitrate,sodium bicarbonate(DCB)Organically bound fraction H2O2NaOClG.Rauret/Talanta46(1998)449–455453 Table4Sequential extraction schemes2345Method1HF/HClO4NaOAc1mol l−1H2O28.8mol l−1Tessier et al.NH2OH.HCl0.04molMgCl2mol l−1l−1HNO3/NH4OAc residual pH7pH525%HOAcsilicate phaseorganic matter+sul-exchangeable carbonate Fe/Mn oxidesphideHNO3NH4Ox/HOx0.1mol H2O28.8mol l−1NH2OH.HCl0.1molFo¨rstner NaOAc1moll−1l−1l−1residualpH7NH4OAcpH5easily reducible pH3in darksilicate phasemoderately reducible organic matter+sul-exchan+carbphideNaOAc1mol l−1NH2OH.HCl0.1molMeguellati BaCl21mol l−1H2O28.8mol l−1+ashingl−1HNO3+HF/HClresidualpH525%HOAcorganic matter+sul-pH7phidesilicate phaseFe/Mn oxidesexchangeable carbonatereau Community of Reference),has sponsored from1987several projects focused on single ex-traction for soils and sequential extraction for soils and sediments.The project started with the intercomparison of existing procedures tested in an interlaboratory exercise[27].The next step was to adopt common procedures for single extraction of trace metals from mineral soils.The second step was to adopt a common procedure for se-quential extraction of sediment.As a conclusion of thefirst step,single extraction procedures using acetic acid,0.43mol l−1,and EDTA,0.005mol l−1for mineral soils and a mixture of DTPA, 0.005mol l−1diethylenetriamine pentaacetic acid, 0.01mol l−1CaCl2and0.1mol l−1tri-ethanolamine for calcareous soils were adopted for extractable Cd,Cr,Cu,Ni,Pb and Zn.In order to improve the quality of the determination of extractable metal content in different types of soil using the procedures previously adopted,the extraction procedures were validated by means of intercomparison exercises[28,29].Moreover the lack of suitable certified reference materials for this type of studies did not enable the quality of the measurements to be controlled.With the pur-pose to overcome this problem three certified reference materials:a terra rossa soil,a sewage amended soil and a calcareous soil have been prepared and their extractable trace metal con-tents were certified(CRM483,CRM484and CRM600)[30,31].The second step of the EC,Standards,Mea-surement and Testing was focused on a feasibility study on the adoption and validation of a sequen-tial extraction scheme for sediment samples.In a workshop held in1992in Sitges(Spain)a sequen-tial extraction scheme was proposed which in-cludes three steps:acetic acid,hydroxylamine hydrochloride or a reducing reagent and hydrogen peroxide or an oxidising reagent.This procedure is schematised in Table5.Moreover in this work-shop the main analytical limitations in sequential extraction of trace metals in sediments were thor-oughly discussed and practical recommendations were given[32,33].These recommendations deal with sampling and sample pre-treatment,practical experiences with reagents and matrices and ana-lytical problems after extraction.Once the scheme was designed,it was tested through two round robin exercises using two dif-ferent type of sediment,silicious and calcareous [34].In these exercises some critical parameters in the protocol were identified such as the type and the speed of the shaking and the need of an optimal separation of the liquid–solid phases af-ter the extraction.It was stated that the sedimentG.Rauret/Talanta46(1998)449–455 454should be continually in suspension during the extraction.In these intercomparison exercises an important decrease was noted on the acceptable set of values for concentration in the extract lower than10m g l−1,which illustrates the difficulties experienced by a number of laboratories in the determination of such concentration levels in these matrices.It was concluded that when elec-trothermal atomic absorption spectrometry is used for thefinal determination,the method of standard additions is strongly recommended for calibration.The results obtained in the round robin exercises encouraged to proceed with the organisation of a certification campaign in order to produce a sediment reference material follow-ing the sequential extraction scheme adopted.So the next step of the project was the preparation of a sediment certified reference material for the extractable contents of Cd,Cr,Cu,Ni.Pb and Zn,following the three-step sequential extraction procedure.A silicious type sediment with rather high trace metal content was chosen for this pur-pose.This material has been recently certified for five metals,Cd,Cr,Ni,Pb and Zn in thefirst step,Cd,Ni and Zn in the second step and Cd,Ni and Pb in the third step[35].Not all the elements were certified because the lack of reproducibility atributable to non adherence to the protocol,in the acceptance of too large tolerances in the con-ditions specified in it or in the existence of critical aspects in the procedure referred mainly to the second step.These aspects were mainly pH,redox conditions and possible losses of sediment in the transfer.The results obtained in the certification exercise recommended to continue the develop-ment of the extraction protocol in order to in-crease reproducibility.Consequently the causes of non reproducibility are now under study in a new SMT project.5.ConclusionsThe advantages of a differential analysis over investigations of total metal contents and about the usefulness of single and sequential chemical extraction for predicting long-term adverse effects of heavy metals from polluted solid material,soils and sediments,is beyond any doubt.The ad-vances in thisfield,especially to make available soil and sediment certified reference materials for extractable element contents by using harmonised procedures,is going to increase the quality of the results due to the possibility of verifying the ana-lytical quality control.Nevertheless some problems need to be solved with these procedures for example:(1)reactions are not selective and are influenced by the experi-mental conditions so it is necessary to identify the main variables which involves a lack of reproduci-bility when applying a procedure,to write very well defined protocols and to validate them;(2) labile fractions could be transformed during sam-ple preparation and during sequential extraction schemes application so problems encountered when preparing certified reference materials are not representing all the problems to be found when working with environmental samples such as wet sediments,some work in this area is needed;(3)analytical problems due to the low level of metals to be measured in the different fractions especially when using soft extractants; and(4)the procedures need to be optimised and validated for different type of soils,including organic soils and sediments.Table5EC Standard,Measurements and Testing procedureConditionsStep10.11mol l−1HOAc,V m−140ml g−1temp.20o C,shaking overnight20.1mol l−1NH2OH.HCl(pH=2with HNO3)V m−140ml.g−1temp.20o Cshaking overnight8.8mol l−1H2O2(pH=2–3with HNO3)3V m−1=10ml g−1room temperature1h.New addition10ml g−185o C for1h.reduce volume to few ml.1mol l−1NH4Oac(pH=2with HNO3)V m−1=50ml g−120o Cshaking overnightG.Rauret/Talanta46(1998)449–455455References[1]H.A.van der Sloot,L.Heasman,Ph.Quevauviller(Eds.),Harmonization of leaching/extraction test,Chap.3, 1997,41–56.[2]H.A.van der Sloot,L.Heasman,Ph.Quevauviller(Eds.),Harmonization of leaching/extraction test,Chap.5, 1997,pp.75–99.[3]I.Novozamski,Th.M.Lexmon,V.J.G.Houba,Int.J.Environ.Anal.Chem.51(1993)47–58.[4]E.Colinet,H.Gonska,B.Griepink,H.Muntau,EURReport8833EN,1983,p57.[5]A.M.Ure,Ph.Quevauviller,H.Muntau,B.Griepink,Int.J.Environ.Anal.Chem.51(1993)135–151.[6]C.L.Mulchi,C.A.Adamu,P.F.Bell,R.L.Chaney,Com-mon.Soil Sci.Plant Anal.23(1992)1053–1059.[7]W.L.Lindsay,W.A.Norvell,Soil Sci.Soc.Am.J.42(1978)421–428.[8]A.Melich,Common.Soil Sci.Plant Anal.15(1984)1409–1416.[9]A.M.Ure,R.Thomas, D.Litlejohn,Int.J.Environ.Anal.Chem.51(1993)65–84.[10]S.K.Gupta,C Aten,Int.J.Environ.Anal.Chem.51(1993)25–46.[11]J.C.Hughes,A.D.Noble,Common.Soil Sci.Plant Anal.22(1991)1753–1766.[12]C.Juste,P.Solda,Agronomie8(1988)897–904.[13]W.P.Pickering,Ore Geol.Rev.1(1986)83–146.[14]A.Lebourg,T.Sterckeman,H.Cielsielki,N.Proix,Agronomie16(1996)201–215.[15]DIN(Deutches Institut fu¨r Normung)(Ed.)Bo-denbeschaffenheit.Vornorm DIN V19730,in:Boden -Chemische Bodenuntersuchungsverfahren,DIN,Berlin, 1993,p.4.[16]AFNOR(Association Francaisede Normalization),AFNOR,Paris,1994,p.250.[17]UNICHIM(Ente Nazionale Italiano di Unificazione),UNICHIM,Milan1991.[18]V.J.G.Houba,I.Novozamski,T.X.Lexmon,J.J.van derLee,Common.Soil Sci.Plant Anal.21(1990)2281–2291.[19]VSBo(Veordnung u¨ber Schadstoffgehalt im Boden)Nr.814.12,Publ.eidg.Drucksachen und Materialzentrale, Bern,1986,pp.1–4.[20]MAFF(Ministry of Agriculture,Fisheries and Food),Reference Book427MAFF,London1981.[21]A.Ure,Ph.Quevauviller,H.Muntau,B.Griepink,Re-port EUR14763EN.1993.[22]A.Tessier,P.G.X.Campbell,M.Bisson,Anal.Chem.51(1979)844.[23]W.Salomons,U.Fo¨rstner,Environ.Lett1(1980)506.[24]M.Meguellati,D.Robbe,P.Marchandise,M.Astruc,Proc.Int.Conf.on Heavy Metals in the Environment, Heidelberg,CEP Consultants,Edinburgh,1983,p.1090.[25]G.Rauret,R.Rubio,J.F.Lopez-Sanchez,Int.J.Envi-ron.Anal.Chem.36(1989)69–83.[26]U.Fo¨rstner,in:R.Lechsber,R.A.Davis,P.L’Hermitte(Eds.),Chemical Methods for Assessing Bioavailable Metals in Sludges,Elsevier,London,1985.[27]A Ure,Ph.Quevauviller,H.Muntau,B.Griepink,Int.J.Environ.Anal.Chem.51(1993)135–151.[28]Ph.Quevauviller,chica,E.Barahona,G.Rauret,A.Ure,A Gomez,H.Muntau,Sci.Total Environ.178(1996)127–132.[29]Ph.Quevauviller,G.Rauret, A.Ure,R.Rubio,J-FLo´pez-Sa´nchez,H.Fiedler,H.Muntau,Mikrochim.Acta 120(1995)289–300.[30]Ph.Quevauviller,G.Rauret,A.Ure,J.Bacon,H.Mun-tau,Report EUR17127EN,1997.[31]Ph.Quevauviller,chica,E.Barahona,G.Rauret,A.Ure,A.Gomez,H.Muntau,Report EUR17555EN,1997.[32]Ph.Quevauviller,G.Rauret,B.Griepink,Int.J.Environ.Anal.Chem.51(1993)231–235.[33]B.Griepink,Int.J.Environ.Anal.Chem.51(1993)123–128.[34]Ph.Quevauviller,G.Rauret,H.Muntau,A.M.Ure,R.Rubio,J-F Lo´pez-Sanchez,H.D.Fiedler, B.Griepink, Fres.J.Anal.Chem.349(1994)808–814.[35]Ph.Quevauviller,G.Rauret,J-F.Lo´pez-Sanchez,R.Rubio, A.Ure,H.Muntau,Report EUR17554EN, 1997.. .。
A New Academic Word List

A New Academic Word ListAVERIL COXHEADVictoria University of WellingtonWellington, New ZealandThis article describes the development and evaluation of a new aca-demic word list (Coxhead, 1998), which was compiled from a corpus of3.5 million running words of written academic text by examining therange and frequency of words outside the first 2,000 most frequentlyoccurring words of English, as described by West (1953). The AWLcontains 570 word families that account for approximately 10.0% of thetotal words (tokens) in academic texts but only 1.4% of the total wordsin a fiction collection of the same size. This difference in coverageprovides evidence that the list contains predominantly academic words.By highlighting the words that university students meet in a wide rangeof academic texts, the AWL shows learners with academic goals whichwords are most worth studying. The list also provides a useful basis forfurther research into the nature of academic vocabulary.O ne of the most challenging aspects of vocabulary learning and teaching in English for academic purposes (EAP) programmes is making principled decisions about which words are worth focusing on during valuable class and independent study time. Academic vocabulary causes a great deal of difficulty for learners (Cohen, Glasman, Rosenbaum-Cohen, Ferrara, & Fine, 1988) because students are generally not as familiar with it as they are with technical vocabulary in their own fields and because academic lexical items occur with lower frequency than general-service vocabulary items do (Worthington & Nation, 1996; Xue & Nation, 1984).The General Service List (GSL) (West, 1953), developed from a corpus of 5 million words with the needs of ESL/EFL learners in mind, contains the most widely useful 2,000 word families in English. West used a variety of criteria to select these words, including frequency, ease of learning, coverage of useful concepts, and stylistic level (pp. ix–x). The GSL has been criticised for its size (Engels, 1968), age (Richards, 1974), and need for revision (Hwang, 1989). Despite these criticisms, the GSL covers up to 90% of fiction texts (Hirsh, 1993), up to 75% of nonfiction texts (Hwang, 1989), and up to 76% of the Academic Corpus (Coxhead,213 TESOL QUARTERLY Vol. 34, No. 2, Summer 20001998), the corpus of written academic English compiled for this study. There has been no comparable replacement for the GSL up to now.Academic words (e.g., substitute, underlie, establish, inherent) are not highly salient in academic texts, as they are supportive of but not central to the topics of the texts in which they occur. A variety of word lists have been compiled either by hand or by computer to identify the most useful words in an academic vocabulary. Campion and Elley (1971) and Praninskas (1972) based their lists on corpora and identified words that occurred across a range of texts whereas Lynn (1973) and Ghadessy (1979) compiled word lists by tracking student annotations above words in textbooks. All four studies were developed without the help of computers. Xue and Nation (1984) created the University Word List (UWL) by editing and combining the four lists mentioned above. The UWL has been widely used by learners, teachers, course designers, and researchers. However, as an amalgam of the four different studies, it lacked consistent selection principles and had many of the weaknesses of the prior work. The corpora on which the studies were based were small and did not contain a wide and balanced range of topics.An academic word list should play a crucial role in setting vocabulary goals for language courses, guiding learners in their independent study, and informing course and material designers in selecting texts and developing learning activities. However, given the problems with cur-rently available academic vocabulary lists, there is a need for a new academic word list based on data gathered from a large, well-designed corpus of academic English. The ideal word list would be divided into smaller, frequency-based sublists to aid in the sequencing of teaching and in materials development. A word list based on the occurrence of word families in a corpus of texts representing a variety of academic registers can provide information about how words are actually used (Biber, Conrad, & Reppen, 1994).The research reported in this article drew upon principles from corpus linguistics (Biber, Conrad, & Reppen, 1998; Kennedy, 1998) to develop and evaluate a new academic word list. After discussing issues that arise in the creation of a word list through a corpus-based study, I describe the methods used in compiling the Academic Corpus and in developing the AWL. The next section examines the coverage of the AWL relative to the complete Academic Corpus and to its four discipline-specific subcorpora. To evaluate the AWL, I discuss its coverage of (a) the Academic Corpus along with the GSL (West, 1953), (b) a second collection of academic texts, and (c) a collection of fiction texts, and compare it with the UWL(Xue & Nation, 1984). In concluding, I discuss the list’s implications for teaching and for materials and course design, and I outline future research needs.214TESOL QUARTERLYTHE DEVELOPMENT OF ACADEMIC CORPORAAND WORD LISTSTeachers and materials developers who work with vocabulary lists often assume that frequently occurring words and those which occur in many different kinds of texts may be more useful for language learners to study than infrequently occurring words and those whose occurrences are largely restricted to a particular text or type of text (Nation, in press; West, 1953). Given the assumption that frequency and coverage are important criteria for selecting vocabulary, a corpus, or collection of texts, is a valuable source of empirical information that can be used to examine the language in depth (Biber, Conrad, & Reppen, 1994). However, exactly how a corpus should be developed is not clear cut. Issues that arise include the representativeness of the texts of interest to the researcher (Biber, 1993), the organization of the corpus, its size (Biber, 1993; Sinclair, 1991), and the criteria used for word selection. RepresentationResearch in corpus linguistics (Biber, 1989) has shown that the linguistic features of texts differ across registers. Perhaps the most notable of these features is vocabulary. To describe the vocabulary of a particular register, such as academic texts, the corpus must therefore contain texts that are representative of the varieties of texts they are intended to reflect (Atkins, Clear, & Ostler, 1992; Biber, 1993; Sinclair, 1991). Sinclair (1991) warns that a corpus should contain texts whose sizes and shapes accurately reflect the texts they represent. If long texts are included in a corpus, “peculiarities of an individual style or topic occasionally show through” (p. 19), particularly through the vocabulary. Making use of a variety of short texts allows more variation in vocabulary (Sutarsyah, Nation, & Kennedy, 1994). Inclusion of texts written by a variety of writers helps neutralise bias that may result from the idiosyn-cratic style of one writer (Atkins et al., 1992; Sinclair, 1991) and increases the number of lexical items in the corpus (Sutarsyah et al., 1994).Scholars who have compiled corpora have attempted to include a variety of academic texts. Campion and Elley’s (1971) corpus consisted of 23 textbooks, 19 lectures published in journals, and a selection of university examination papers. Praninskas (1972) used a corpus of 10first-year, university-level arts and sciences textbooks that were required reading at the American University of Beirut.Lynn (1973) and Ghadessy (1979) both focussed on textbooks used in their universities. Lynn’s corpus included 52 textbooks and 4 classroom handouts from 50 A NEW ACADEMIC WORD LIST215students of accounting, business administration, and economics from which 10,000 annotations were collected by hand. The resulting list contained 197 word families arranged from those occurring the most frequently (39 times) to those occurring the least frequently. Words occurring fewer than 10 times were omitted from the list (p. 26). Ghadessy compiled a corpus of 20 textbooks from three disciplines (chemistry, biology, and physics). Words that students had glossed were recorded by hand, and the final list of 795 items was then arranged in alphabetical order (p. 27). Relative to this prior work, the corpus compiled for the present study considerably expands the representation of academic writing in part by including a variety of academic sources besides textbooks.OrganizationA register such as academic texts encompasses a variety of subregisters. An academic word list should contain an even-handed selection of words that appear across the various subject areas covered by the texts contained within the corpus. Organizing the corpus into coherent sections of equal size allows the researcher to measure the range of occurrence of the academic vocabulary across the different disciplines and subject areas of the corpus. Campion and Elley (1971) created a corpus with 19 academic subject areas, selecting words occurring outside of the first 5,000 words of Thorndike and Lorge’s (1944) list and excluding words encountered in only one discipline (p. 7). The corpus for the present study involved 28 subject areas organised into 7 general areas within each of four disciplines: arts, commerce, law, and science. SizeA corpus designed for the study of academic vocabulary should be large enough to ensure a reasonable number of occurrences of academic words. According to Sinclair (1991), a corpus should include millions of running words (tokens) to ensure that a very large sample of language is available (p. 18).1 The exact amount of language required, of course, depends on the purpose and use of the research; however, in general more language means that more information can be gathered about lexical items and more words in context can be examined in depth.1The term running words (or tokens) refers to the total number of word forms in a text, whereas the term individual words (types) refers to each different word in a text, irrespective of how many times it occurs.216TESOL QUARTERLYIn the past, researchers attempted to work with academic corpora by hand, which limited the numbers of words they could analyze.Campion and Elley (1971), in their corpus of 301,800 running words, analysed 234,000 words in textbooks, 57,000 words from articles in journals, and 10,800 words in a number of examination papers (p. 4). Praninskas’s (1972) corpus consisted of approximately 272,000 running words (p. 8), Lynn (1973) examined 52 books and 4 classroom handouts (p. 26), and Ghadessy (1979) compiled a corpus of 478,700 running words. Praninskas (1972) included a criterion of range in her list and selected words that were outside the GSL (West, 1953).In the current study, the original target was to gather 4.0 million words; however, time pressures and lack of available texts limited the corpus to approximately 3.5 million running words. The decision about size was based on an arbitrary criterion relating to the number of occurrences necessary to qualify a word for inclusion in the word list: If the corpus contained at least 100 occurrences of a word family, allowing on average at least 25 occurrences in each of the four sections of the corpus, the word was included. Study of data from the Brown Corpus (Francis & Kucera, 1982) indicated that a corpus of around 3.5 million words would be needed to identify 100 occurrences of a word family. Word SelectionAn important issue in the development of word lists is the criteria for word selection, as different criteria can lead to different results. Re-searchers have used two methods of selection for academic word lists. As mentioned, Lynn (1973) and Ghadessy (1979) selected words that learners had annotated regularly in their textbooks, believing that the annotation signalled difficulty in learning or understanding those words during reading. Campion and Elley (1971) selected words based on their occurrence in 3 or more of 19 subject areas and then applied criteria, including the degree of familiarity to native speakers. However, the number of running words in the complete corpus was too small for many words to meet the initial criterion. Praninskas (1972) also included a criterion of range in her list; however, the range of subject areas and number of running words was also small, resulting in a small list without much variety in the words.Another issue that arises in developing word lists is defining what to count as a word. The problem is that lexical items that may be morphologically distinct from one another are, in fact, strongly enough related that they should be considered to represent a single lexical item. To address this issue, word lists for learners of English generally group words into families (West, 1953; Xue & Nation, 1984). This solution is A NEW ACADEMIC WORD LIST217supported by evidence suggesting that word families are an important unit in the mental lexicon (Nagy, Anderson, Schommer, Scott, & Stallman, 1989, p. 262). Comprehending regularly inflected or derived members of a family does not require much more effort by learners if they know the base word and if they have control of basic word-building processes (Bauer & Nation, 1993, p. 253). In the present study, therefore, words were defined through the unit of the word family, as illustrated in Table 1.For the creation of the AWL, a word family was defined as a stem plus all closely related affixed forms, as defined by Level 6 of Bauer and Nation’s (1993) scale. The Level 6 definition of affix includes all inflections and the most frequent, productive, and regular prefixes and suffixes (p. 255). It includes only affixes that can be added to stems that can stand as free forms (e.g., specify and special are not in the same word family because spec is not a free form).Research QuestionsThe purpose of the research described here was to develop and evaluate a new academic word list on the basis of a larger, more principled corpus than had been used in previous research. Two questions framed the description of the AWL:1.Which lexical items occur frequently and uniformly across a widerange of academic material but are not among the first 2,000 words of English as given in the GSL (West, 1953)?2.D o the lexical items occur with different frequencies in arts, com-merce, law, and science texts?TABLE 1Sample Word Families From the Academic Word Listconcept legislate indicateconception legislated indicatedconcepts legislates indicatesconceptual legislating indicatingconceptualisation legislation indicationconceptualise legislative indicationsconceptualised legislator indicativeconceptualises legislators indicatorconceptualising legislature indicatorsconceptuallyNote. Words in italics are the most frequent form in that family occurring in the Academic Corpus.218TESOL QUARTERLYThe evaluation of the AWL considered the following questions:3.What percentage of the words in the Academic Corpus does the AWLcover?4.Do the lexical items identified occur frequently in an independentcollection of academic texts?5.How frequently do the words in the AWL occur in nonacademictexts?6.How does the AWL compare with the UWL (Xue & Nation, 1984)? METHODOLOGYThe development phase of the project identified words that met the criteria for inclusion in the AWL (Research Questions 1 and 2). In the evaluation phase, I calculated the AWL’s coverage of the original corpus and compared the AWL with words found in another academic corpus, with those in a nonacademic corpus, and with another academic word list (Questions 3–6).Developing the Academic CorpusD eveloping the corpus involved collecting each text in electronic form, removing its bibliography, and counting its words. After balancing the number of short, medium-length, and long texts (see below for a discussion on the length of texts), each text was inserted into its subject-area computer file in alphabetical order according to the author’s name. Each subject-area file was then inserted into a discipline master file, in alphabetical order according to the subject. Any text that met the selection criteria but was not included in the Academic Corpus because its corresponding subject area was complete was kept aside for use in a second corpus used to test the AWL’s coverage at a later stage. The resulting corpus contained 414 academic texts by more than 400 authors, containing 3,513,330 tokens (running words) and 70,377 types (individual words) in approximately 11,666 pages of text. The corpus was divided into four subcorpora: arts, commerce, law, and science, each containing approximately 875,000 running words and each subdivided into seven subject areas (see Table 2).The corpus includes the following representative texts from the academic domain: 158 articles from academic journals, 51 edited aca-demic journal articles from the World Wide Web, 43 complete university textbooks or course books, 42 texts from the Learned and Scientific section of the Wellington Corpus of Written English (Bauer, 1993), 41 A NEW ACADEMIC WORD LIST219220TESOL QUARTERLY texts from the Learned and Scientific section of the Brown Corpus (Francis & Kucera, 1982), 33 chapters from university textbooks, 31 texts from the Learned and Scientific section of the Lancaster-Oslo/Bergen (LOB) Corpus (Johansson, 1978), 13 books from the Academic Texts section of the MicroConcord academic corpus (Murison-Bowie, 1993),and 2 university psychology laboratory manuals.The majority of the texts were written for an international audience.Sixty-four percent were sourced in New Zealand, 20% in Britain, 13% in the United States, 2% in Canada, and 1% in Australia. It is difficult to say exactly what influence the origin of the texts would have on the corpus,for even though a text was published in one country, at least some of the authors may well have come from another.The Academic Corpus was organized to allow the range of occurrence of particular words to be examined. Psychology and sociology texts were placed in the arts section on the basis of Biber’s (1989) finding that texts from the social sciences (psychology and sociology) shared syntactic characteristics with texts from the arts (p. 28). Lexical items may well pattern similarly. Placing the social science subject areas in the science section of the Academic Corpus might have introduced a bias: The psychology and sociology texts might have added lexical items that do not occur in any great number in any other subject in the science section. The presence of these items, in turn, would have suggested that science and arts texts share more academic vocabulary items than is generally true.With the exception of the small number of texts from the Brown (Francis & Kucera, 1982), LOB (Johansson, 1978), and Wellington TABLE 2Composition of the Academic Corpus DisciplineArtsCommerce Law Science Total Running 883,214879,547874,723875,846351,333wordsTexts122 107 72 113 414Subject Education Accounting Constitutional Biology areas History Economics Criminal Chemistry Linguistics Finance Family and Computer science Philosophy Industrial medicolegal Geography Politics relations International Geology Psychology Management Pure commercial Mathematics Sociology Marketing Quasi-commercial Physics Public policy Rights and remedies(Bauer, 1993) corpora, the texts in the Academic Corpus were complete. The fact that frequency of occurrence of words was only one of the criteria for selecting texts minimized any possible bias from word repetition within longer texts. To maintain a balance of long and short texts, the four main sections (and, within each section, the seven subject areas) each contained approximately equal numbers of short texts (2,000–5,000 running words), medium texts (5,000–10,000 running words), and long texts (more than 10,000 running words). The break-down of texts in the four main sections was as follows: arts—18 long, 35 medium; commerce—18 long, 37 medium; law—23 long, 22 medium; and science—19 long, 37 medium.Developing the Academic Word ListThe corpus analysis programme Range (Heatley & Nation, 1996) was used to count and sort the words in the Academic Corpus. This programme counts the frequency of words in up to 32 files at a time and records the number of files in which each word occurs (range) and the frequency of occurrence of the words in total and in each file.Words were selected for the AWL based on three criteria:1.Specialised occurrence: The word families included had to be outsidethe first 2,000 most frequently occurring words of English, as represented by West’s (1953) GSL.2.Range: A member of a word family had to occur at least 10 times ineach of the four main sections of the corpus and in 15 or more of the28 subject areas.3.Frequency: Members of a word family had to occur at least 100 times inthe Academic Corpus.Frequency was considered secondary to range because a word count based mainly on frequency would have been biased by longer texts and topic-related words. For example, the Collins COBUILD Dictionary (1995) highlights Yemeni and Lithuanian as high-frequency words, probably because the corpus on which the dictionary is based contains a large number of newspapers from the early 1990s.The conservative threshold of a frequency of 100 was applied strictly for multiple-member word families but not so stringently for word families with only one member, as single-member families operate at a disadvantage in gaining a high frequency of occurrence. In the Aca-demic Corpus, the word family with only one member that occurs the least frequently is forthcoming (80 occurrences).A NEW ACADEMIC WORD LIST221RESULTSDescriptionOccurrence of Academic WordsThe first research question asked which lexical items beyond the first 2,000 in West’s (1953) GSL occur frequently across a range of academic texts. In the Academic Corpus, 570 word families met the criteria for inclusion in the AWL (see Appendix A). Some of the most frequent word families in the AWL are analyse, concept, data, and research. Some of the least frequent are convince, notwithstanding, ongoing, persist, and whereby. Differences in Occurrence of Words Across DisciplinesThe second question was whether the lexical items selected for the AWL occur with different frequencies in arts, commerce, law, and science texts. The list appears to be slightly advantageous for commerce students, as it covers 12.0% of the commerce subcorpus. The coverage of arts and of law is very similar (9.3% and 9.4%, respectively), and the coverage of science is the lowest among the four disciplines (9.1%). The 3.0% difference between the coverage of the commerce subcorpus and the coverage of the other three subcorpora may result from the presence of key lexical items such as economic, export, finance, and income, which occur with very high frequency in commerce texts. (See Appendix B for excerpts from texts in each section of the Academic Corpus.) The words in the AWL occur in a wide range of the subject areas in the Academic Corpus. Of the 570 word families in the list, 172 occur in all 28 subject areas, and 263 (172 + 91) occur in 27 or more subject areas (see Table 3). In total, 67% of the word families in the AWL occur in 25 or more of the 28 subject areas, and 94% occur in 20 or more. EvaluationCoverage of the Academic Corpus Beyond the GSLThe AWL accounts for 10.0% of the tokens in the Academic Corpus. This coverage is more than twice that of the third 1,000 most frequent words, according to Francis and Kucera’s (1982) count, which cover 4.3% of the Brown Corpus. Taken together, the first 2,000 words in West’s (1953) GSL and the word families in the AWL account for approximately 86% of the Academic Corpus (see Table 4). Note that the AWL’s coverage of the Academic Corpus is double that of the second 222TESOL QUARTERLY1,000 words of the GSL. The AWL and the GSL combined have a total of 2,550 word families, and all but 12 of those in the GSL occur in the Academic Corpus.The AWL, the first 1,000 words of the GSL (West, 1953), and the second 1,000 words of the GSL cover the arts, commerce, and law subcorpora similarly but in very different patterns (see Table 5). The first 1,000 words of the GSL account for fewer of the word families in the commerce subcorpus than in the arts and law subcorpora, but this lower coverage of commerce is balanced by the AWL’s higher coverage of this discipline. On the other hand, the AWL’s coverage of the arts and law subcorpora is lower than its coverage of the commerce subcorpus, but the GSL’s coverage of arts and law is slightly higher than its coverage of commerce. The AWL’s coverage of the science subcorpus is 9.1%, which indicates that the list is also extremely useful for science students. The GSL, in contrast, is not quite as useful for science students as it is for arts,commerce, and law students.Subject-Area Coverage of Word Families in the Academic Word List No. of Subject areas in No. of Subject areas in word familieswhich they occurred word families which they occurred 1722820219127152058269196225918432451743235163322415Note. Total subject areas = 28; total word families = 570.TABLE 4Coverage of the Academic Corpus by the Academic Word Listand the General Service List (West, 1953)No. of word familiesCoverage of In Academic Word listAcademic Corpus (%)Total Corpus Academic Word List10.0570570General Service List First 1,000 words 71.41,0011,000Second 1,000 words4.7979968Total 86.12,5502,538Coverage of Another Academic CorpusA frequency-based word list that is derived from a particular corpus should be expected to cover that corpus well. The real test is how the list covers a different collection of similar texts. To establish whether the AWL maintains high coverage over academic texts other than those in the Academic Corpus, I compiled a second corpus of academic texts in English, using the same criteria and sources to select texts and dividing them into the same four disciplines. This corpus comprised approxi-mately 678,000 tokens (82,000 in arts, 53,000 in commerce, 143,000 in law, and 400,000 in science) representing 32,539 types of lexical items.This second corpus was made up of texts that had met the criteria for inclusion in the Academic Corpus but were not included either because they were collected too late or because the subject area they belonged to was already complete.The AWL’s coverage of the second corpus is 8.5% (see Table 6), and all 570 word families in the AWL occur in the second corpus. The GSL’s coverage of the second corpus (66.2%) is consistent with its coverage of the science section of the Academic Corpus (65.7%). The overall lower coverage of the second corpus by both the AWL and the GSL (79.1%)seems to be partly the result of the large proportion of science texts it contains.Coverage of Nonacademic TextsTo establish that the AWL is truly an academic word list rather than a general-service word list, I developed a collection of 3,763,733 running words of fiction texts. The collection consisted of 50 texts from Project Gutenberg’s () collection of texts that were written more than 50 years ago and are thus in the public domain. The Coverage of the Four Subcorpora of the Academic Corpus by the General Service List (West, 1953) and the Academic Word List (%)General Service ListAcademic First 1,000Second 1,000SubcorpusWord List words words Total Arts9.373.0 4.486.7Commerce12.071.6 5.288.8Law9.475.0 4.188.5Science 9.165.7 5.079.8。
食用菌多糖的提取和纯化英语

食用菌多糖的提取和纯化英语Extraction and Purification of Edible Fungi Polysaccharides.Edible fungi, known for their nutritional and medicinal properties, have gained significant attention in recent years. Among their various bioactive components, polysaccharides stand out due to their potential health benefits. Extraction and purification of these polysaccharides is crucial for their effective utilization in food, pharmaceutical, and cosmetic industries.Extraction Methods.The extraction of polysaccharides from edible fungi typically involves two main steps: solvent extraction and isolation. Common solvents used for polysaccharide extraction include water, dilute acids, and alkaline solutions. Water extraction is the most widely used method due to its simplicity and effectiveness. However, for somefungi species, dilute acid or alkaline extraction may be necessary to disrupt the cell wall and release the polysaccharides.During the extraction process, temperature, time, and solvent-to-solid ratio are critical parameters. Generally, higher temperatures and longer extraction times enhance the yield of polysaccharides. However, excessive temperatures can lead to degradation of the polysaccharides, thus affecting their biological activities. Therefore, it is essential to optimize these parameters for each specific fungi species.Purification Methods.After extraction, the crude polysaccharide mixture often contains impurities such as proteins, lipids, and small molecules. Purification is necessary to obtain a pure polysaccharide fraction with high biological activity. Common purification methods include precipitation, chromatography, and dialysis.Precipitation is a simple and effective method to remove proteins and other impurities. By adjusting the pHor adding specific chemicals, the polysaccharides can be precipitated while the impurities remain in the supernatant. Chromatography, especially anion-exchange and gelfiltration chromatography, is widely used to further purify the polysaccharides. These methods allow for the separation of polysaccharides based on their charge and molecular size, respectively.Dialysis is another purification technique thatinvolves the diffusion of smaller molecules through a semi-permeable membrane. This method is particularly useful for removing small molecules and salts from the polysaccharide solution.Applications of Edible Fungi Polysaccharides.The purified polysaccharides from edible fungi exhibita range of biological activities, including antioxidant, antitumor, immunomodulatory, and hypoglycemic effects. These properties make them valuable ingredients infunctional foods, nutraceuticals, and pharmaceutical formulations.In functional foods, edible fungi polysaccharides can enhance the nutritional value and provide health benefits to consumers. For example, they can be added to beverages, yogurts, and cereals to improve their nutritional profile and functional properties.In the pharmaceutical industry, edible fungi polysaccharides are being investigated for their potential in treating various diseases such as cancer, diabetes, and immune disorders. The purified polysaccharides can be formulated into tablets, capsules, or injectable formulations for therapeutic use.Conclusion.The extraction and purification of polysaccharides from edible fungi is a crucial step in harnessing their numerous biological activities. By optimizing extraction conditions and employing suitable purification methods, it is possibleto obtain pure polysaccharides with high biologicalactivity. These polysaccharides find applications invarious industries, including food, pharmaceutical, and cosmetics, offering health benefits to consumers and therapeutic potential for treating various diseases.(Note: This article is a simplified overview of the extraction and purification of edible fungi polysaccharides. For a more detailed and comprehensive understanding, it is recommended to consult research articles and technical reports in this field.)。
information extraction

/squared
• What
−
is it?
Google Squared is an experimental tool that takes a category (like US presidents, roller coasters, or digital cameras) and attempts to create a starter "square" of information, automatically fetching and organizing facts from across the web.
Entitycube
Entitycube
Current Problems
The prototype currently only contains information extracted from 3 billion Web pages, therefore it is possible that some information for people with a substantial Web presence is still missing in our index; • Some names and relationships could be incorrect, and the information may not be update-to-date; • Name disambiguation is still largely unsolved. Some people with popular/common names may find that their information has been mixed with other people of the same name; • Some of the summarization features are currently only available for people. We are currently working on these for other entities.
重庆评估院 师范类专业认证 英语
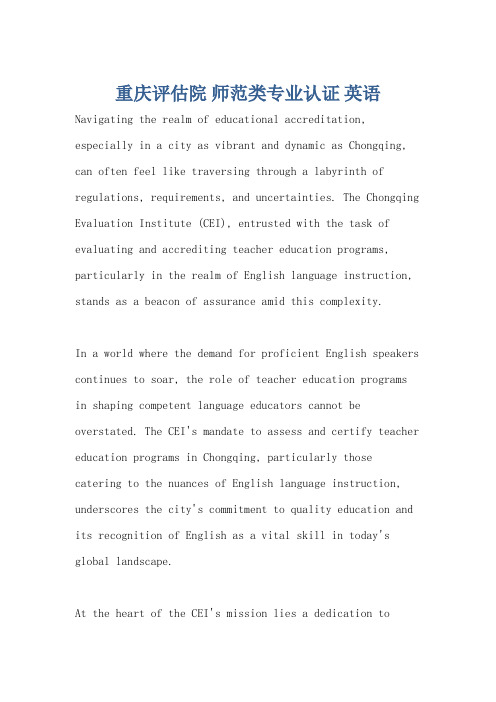
重庆评估院师范类专业认证英语Navigating the realm of educational accreditation, especially in a city as vibrant and dynamic as Chongqing, can often feel like traversing through a labyrinth of regulations, requirements, and uncertainties. The Chongqing Evaluation Institute (CEI), entrusted with the task of evaluating and accrediting teacher education programs, particularly in the realm of English language instruction, stands as a beacon of assurance amid this complexity.In a world where the demand for proficient English speakers continues to soar, the role of teacher education programsin shaping competent language educators cannot be overstated. The CEI's mandate to assess and certify teacher education programs in Chongqing, particularly those catering to the nuances of English language instruction, underscores the city's commitment to quality education and its recognition of English as a vital skill in today's global landscape.At the heart of the CEI's mission lies a dedication toensuring that teacher education programs align with international standards of excellence while also catering to the unique needs and context of Chongqing. Through a meticulous process of evaluation, which encompasses curriculum review, faculty qualifications, teaching methodologies, and infrastructure, the CEI endeavors to uphold the integrity and efficacy of teacher education programs across the city.For aspiring educators embarking on their journey through teacher education programs in Chongqing, the CEI's accreditation serves as both a compass and a credential. It provides them with the assurance that their chosen program has undergone rigorous scrutiny and meets the requisite standards for producing competent and capable teachers.Moreover, the CEI's accreditation serves as a catalyst for continuous improvement within teacher education institutions. By identifying areas of strength and areas in need of enhancement, the accreditation process facilitates a culture of introspection and innovation, driving institutions towards excellence and relevance in an ever-evolving educational landscape.For educational stakeholders, including government bodies, employers, and the broader community, the CEI's accreditation of teacher education programs serves as a stamp of approval, instilling confidence in the quality and efficacy of Chongqing's educational offerings. It not only enhances the city's reputation as a hub for educational excellence but also contributes to its overall socio-economic development by producing a skilled workforce equipped to meet the demands of the globalized world.In conclusion, the Chongqing Evaluation Institute's role in accrediting teacher education programs, particularly those focused on English language instruction, underscores the city's commitment to educational quality and relevance. By upholding rigorous standards and fostering a culture of continuous improvement, the CEI plays a pivotal role in shaping the future of education in Chongqing and beyond.。
紫花杜鹃中总黄酮的提取工艺优选
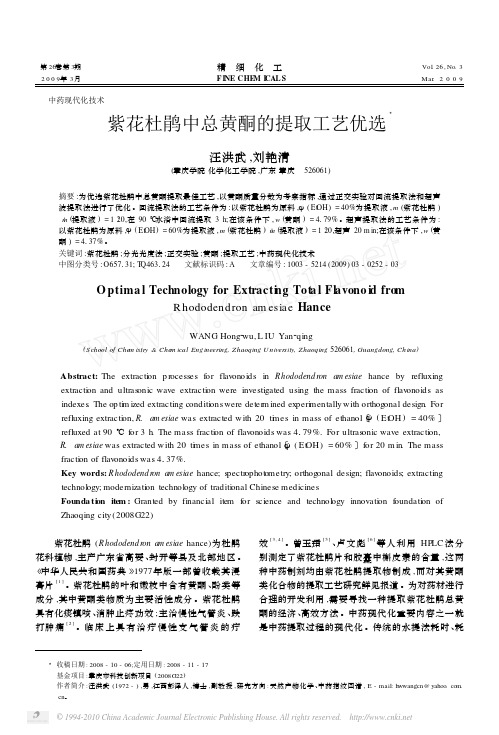
第26卷第3期2009年3月精细化工F I NE CHE M I CAL SVol.26,No.3Mar.2009中药现代化技术紫花杜鹃中总黄酮的提取工艺优选3汪洪武,刘艳清(肇庆学院化学化工学院,广东肇庆 526061)摘要:为优选紫花杜鹃中总黄酮提取最佳工艺,以黄酮质量分数为考察指标,通过正交实验对回流提取法和超声波提取法进行了优化。
回流提取法的工艺条件为:以紫花杜鹃为原料,φ(Et O H)=40%为提取液,m(紫花杜鹃)∶m(提取液)=1∶20,在90℃水浴中回流提取3h;在该条件下,w(黄酮)=4179%。
超声提取法的工艺条件为:以紫花杜鹃为原料,φ(Et O H)=60%为提取液,m(紫花杜鹃)∶m(提取液)=1∶20,超声20m in;在该条件下,w(黄酮)=4137%。
关键词:紫花杜鹃;分光光度法;正交实验;黄酮;提取工艺;中药现代化技术中图分类号:O657131;T Q463124 文献标识码:A 文章编号:1003-5214(2009)03-0252-03O ptima l Technology for Extracti n g Tot a l Fl avono i d fro mR hododendron am esiae HanceWANG Hong2wu,L IU Yan2qing(School of Che m istry&Che m ical Engineering,Zhaoqing U niversity,Zhaoqing526061,Guangdong,China)Abstract:The extracti on p r ocesses f or flav onoids in R hododendron am esiae hance by refluxing extracti on and ultras onic wave extracti on were investigated using the mass fracti on of flavonoids as indexes.The op ti m ized extracting conditi onswere deter m ined experi m entally with orthogonal design.For refluxing extracti on,R.am esiae was extracted with20ti m es in mass of ethanol〔φ(Et O H)=40%〕refluxed at90℃f or3h.The mass fracti on of flavonoids was4179%.For ultras onic wave extracti on, R.am esiae was extracted with20ti m es in mass of ethanol〔φ(Et O H)=60%〕for20m in.The mass fracti on of flavonoids was4137%.Key words:R hododend ron am esiae hance;s pectr ophot ometry;orthogonal design;flavonoids;extracting technol ogy;modernizati on technol ogy of traditi onal Chinese medicinesFounda ti on ite m:Granted by financial ite m f or science and technol ogy innovati on f oundati on of Zhaoqing city(2008G22) 紫花杜鹃(R hododendron am esiae hance)为杜鹃花科植物,主产广东省高要、封开等县及北部地区。
绿原酸,金银花英文
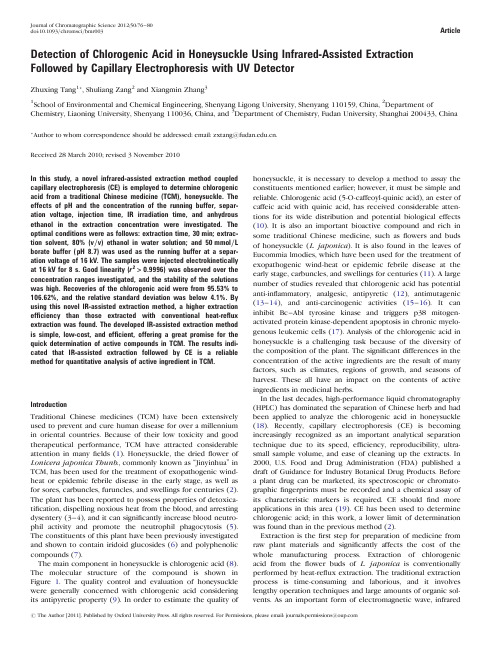
Detection of Chlorogenic Acid in Honeysuckle Using Infrared-Assisted Extraction Followed by Capillary Electrophoresis with UV DetectorZhuxing Tang 1*,Shuliang Zang 2and Xiangmin Zhang 31School of Environmental and Chemical Engineering,Shenyang Ligong University,Shenyang 110159,China,2Department ofChemistry,Liaoning University,Shenyang 110036,China,and 3Department of Chemistry,Fudan University,Shanghai 200433,China*Author to whom correspondence should be addressed:email:zxtang@.Received 28March 2010;revised 3November 2010In this study,a novel infrared-assisted extraction method coupled capillary electrophoresis (CE)is employed to determine chlorogenic acid from a traditional Chinese medicine (TCM),honeysuckle.The effects of pH and the concentration of the running buffer,separ-ation voltage,injection time,IR irradiation time,and anhydrous ethanol in the extraction concentration were investigated.The optimal conditions were as follows:extraction time,30min;extrac-tion solvent,80%(v /v)ethanol in water solution;and 50mmol /L borate buffer (pH 8.7)was used as the running buffer at a separ-ation voltage of 16kV.The samples were injected electrokinetically at 16kV for 8s.Good linearity (r 2>0.9996)was observed over the concentration ranges investigated,and the stability of the solutions was high.Recoveries of the chlorogenic acid were from 95.53%to 106.62%,and the relative standard deviation was below 4.1%.By using this novel IR-assisted extraction method,a higher extraction efficiency than those extracted with conventional heat-reflux extraction was found.The developed IR-assisted extraction method is simple,low-cost,and efficient,offering a great promise for the quick determination of active compounds in TCM.The results indi-cated that IR-assisted extraction followed by CE is a reliable method for quantitative analysis of active ingredient in TCM.IntroductionTraditional Chinese medicines (TCM)have been extensively used to prevent and cure human disease for over a millennium in oriental countries.Because of their low toxicity and good therapeutical performance,TCM have attracted considerable attention in many fields (1).Honeysuckle,the dried flower of Lonicera japonica Thunb .,commonly known as “Jinyinhua”in TCM,has been used for the treatment of exopathogenic wind-heat or epidemic febrile disease in the early stage,as well as for sores,carbuncles,furuncles,and swellings for centuries (2).The plant has been reported to possess properties of detoxica-tification,dispelling noxious heat from the blood,and arresting dysentery (3–4),and it can significantly increase blood neutro-phil activity and promote the neutrophil phagocytosis (5).The constituents of this plant have been previously investigated and shown to contain iridoid glucosides (6)and polyphenolic compounds (7).The main component in honeysuckle is chlorogenic acid (8).The molecular structure of the compound is shown in Figure 1.The quality control and evaluation of honeysuckle were generally concerned with chlorogenic acid considering its antipyretic property (9).In order to estimate the quality ofhoneysuckle,it is necessary to develop a method to assay the constituents mentioned earlier;however,it must be simple and reliable.Chlorogenic acid (5-O-caffeoyl-quinic acid),an ester of caffeic acid with quinic acid,has received considerable atten-tions for its wide distribution and potential biological effects (10).It is also an important bioactive compound and rich in some traditional Chinese medicine,such as flowers and buds of honeysuckle (L.japonica ).It is also found in the leaves of Eucommia lmodies,which have been used for the treatment of exopathogenic wind-heat or epidemic febrile disease at the early stage,carbuncles,and swellings for centuries (11).A large number of studies revealed that chlorogenic acid has potential anti-inflammatory,analgesic,antipyretic (12),antimutagenic (13–14),and anti-carcinogenic activities (15–16).It can inhibit Bc–Abl tyrosine kinase and triggers p38mitogen-activated protein kinase-dependent apoptosis in chronic myelo-genous leukemic cells (17).Analysis of the chlorogenic acid in honeysuckle is a challenging task because of the diversity of the composition of the plant.The significant differences in the concentration of the active ingredients are the result of many factors,such as climates,regions of growth,and seasons of harvest.These all have an impact on the contents of active ingredients in medicinal herbs.In the last decades,high-performance liquid chromatography (HPLC)has dominated the separation of Chinese herb and had been applied to analyze the chlorogenic acid in honeysuckle (18).Recently,capillary electrophoresis (CE)is becoming increasingly recognized as an important analytical separation technique due to its speed,efficiency,reproducibility,ultra-small sample volume,and ease of cleaning up the extracts.In 2000,U.S.Food and Drug Administration (FDA)published a draft of Guidance for Industry Botanical Drug Products.Before a plant drug can be marketed,its spectroscopic or chromato-graphic fingerprints must be recorded and a chemical assay of its characteristic markers is required.CE should find more applications in this area (19).CE has been used to determine chlorogenic acid;in this work,a lower limit of determination was found than in the previous method (2).Extraction is the first step for preparation of medicine from raw plant materials and significantly affects the cost of the whole manufacturing process.Extraction of chlorogenic acid from the flower buds of L.japonica is conventionally performed by heat-reflux extraction.The traditional extraction process is time-consuming and laborious,and it involves lengthy operation techniques and large amounts of organic sol-vents.As an important form of electromagnetic wave,infrared#The Author [2011].Published by Oxford University Press.All rights reserved.For Permissions,please email:journals.permissions@Journal of Chromatographic Science 2012;50:76–80doi:10.1093/chromsci /bmr003Article(IR)rays have wavelengths between 750nm and1mm and have found a wide range of applications.It has been widely employed as a heat resource due to its high penetration ability. Based on its wavelength,it can be divided into near-IR(0.75–1.5m m),middle-IR(1.5–5.6m m),and far-IR(5.6–1000m m) rays.Recently,this method has been used to determine active compounds in radix salviae miltiorrhizae by HPLC(20). However,to our knowledge,IR-assisted extraction coupled with CE has not been fully explored,and its application to the analysis of chlorogenic acid from traditional Chinese medicines, such as honeysuckle,has not been conducted.It is of high interest to demonstrate the possibility of employing IR radi-ation as an energy source to enhance the efficiency of conven-tional reflux extraction.IR-assisted extraction is a process that uses infrared energy and solvents to extract target compounds from various pared with conventional methods, IR-assisted extraction can considerably increase extraction efficiency.In this work,a simple and rapid method was developed to determine chlorogenic acid in honeysuckle by CE,employing IR-assisted extraction as an efficient technique.ExperimentalApparatusIn this work,a high-voltage(+30kV)power supply(Shanghai Institute of Nuclear Research,Shanghai,China)provided a voltage between the ends of the capillary.The separation was undertaken in a50-cm length,75-m m i.d.and360-m m o.d.fused silica capillary(Hebei,China).The capillary was rinsed with 0.1mol/L NaOH30min before use.The injector electrode was kept at a high positive voltage,detection of all the samples was performed by means of a UV detector positioned at the cathodic end of the capillary.Pressurized Capillary Electrochromatography System-2010GV(Unimcro Technology Company,Shanghai,China)was used as the UV detector,which was connected to a high-performance PC with the Windows XP operating system installed.Detection wavelength was254nm. ReagentsChlorogenic acid was purchased from the National Institute for the Control of Pharmaceutical and Biological Products(Beijing, China).Stock solutions of chlorogenic acid(2.0Â1023g/mL) were prepared in anhydrous ethanol(A.R.grade),stored in the dark at48C,and were diluted to the desired concentrations with the running buffer(50mmol/L borate buffer,pH¼8.7).Before use,all solutions werefiltered through0.22-m m nylonfilters. Buffer preparationA series of buffer solutions with pH from7.00to10.00were prepared by mixing boric acid and phosphoric acid stock solu-tions(0.1mol/L).The pH of the buffer was measured at25+ 0.5using a pHS-3C precise pH meter(Leici Instruments, Shanghai Precise Science Instrument Ltd.Co.,Shanghai,China). The buffer solutions werefiltered through a0.22-m m syringe filter and degassed by ultrasonication prior to use.Sample preparationThe herb,honeysuckle,was obtained from local drugstore in Shanghai.Five grams of dried honeysuckle was ground into powder in a mortar and accurately weighed.Each weighed sample was dissolved in40mL anhydrous ethanol(A.R.grade) and water(4:1).The IR-assisted extraction apparatus is shown in Figure2.The distance between the top surface of the IR lamp and the bottom surface of the round-bottomedflask was 9cm,because the solution can be heated by IR lamp to708C for30min at this distance.After cooling,the mixture wasfil-tered through a paperfilter,and the residues were washed with anhydrous ethanol.The extract and washings were com-bined and concentrated to approximately45mL under vacuum,and then diluted to50mL with anhydrous ethanol in a volumetricflask.In the heat-solvent extraction experiment,five grams of dried herb honeysuckle was ground into powder in a mortar and accurately weighed.Each weighed sample was dissolved in 40mL anhydrous ethanol(A.R.grade)and water(4:1). The solution was heated to708C for30min.After cooling,the mixture wasfiltered through paper afilter and the residues were washed with anhydrous ethanol.The extract and wash-ings were combined and concentrated to approximately45mL under vacuum,and then diluted to50mL with anhydrous ethanol in a volumetricflask.In the actual sample analysis,0.50mL of the sample solution was again diluted with the running buffer to1mL.After being filtered through a0.22-m m syringefilter,all solutions can be injected directly to the CE system for analysis.Before use,all sample solutions were stored in the dark at48CResults and DiscussionEffects of the pH value and the buffer concentrationIn order to optimize the resolution and sensitivity of capillary zone electrophoresis(CZE),borate,phosphate,and borate–phosphate mixtures were employed as running buffers in the CZE separation.The experimental results showed that the best result was achieved using borate buffer.So the borate buffer was employed as the running buffer in this work.The pH of the buffer is an important parameter that affects the electroosmoticflow(EOF),the overall charge,and the migration time of the analytes.Hence,the dependence of the migration time on buffer pH was investigated in the pH range of7.0–10.0.Figure1.Structure of chlorogenic acid.Detection of chlorogenic acid in honeysuckle77The migration time of the chlorogenic acid increases with the increasing pH value,and the baseline separation of the honey-suckle can be achieved from pH8.7to10.0.When the pH is lower than8.7,the herb cannot be separated very well.Moreover, higher pH values result in a long analysis time and easy oxidation of the analytes.Therefore,pH8.7was selected as the optimum pH value.Besides the pH value,the running buffer concentration, which affects peak height and theoretical plate number,is also an important parameter.The effect of the running buffer concentra-tion on migration time was also studied,and the optimum running buffer concentration is50mmol/L.Effects of separation voltage and injection timeFor a given capillary length,the separation voltage determines the electricfield strength,which affects both the velocity of electroosmoticflow and the migration velocity of the analytes,and in turn determines the migration time of the analytes. Higher separation voltage gives shorter migration time for all analytes.However,when the separation voltage exceeded 16kV,the baseline noise increased.Therefore,the optimum separation voltage selected was16kV,at which good separ-ation can be obtained for the analytes within15min.The injec-tion time determines the amount of the sample and affects both the peak height and the peak shape.The effect of the injection time on the peak height was studied by varying the injection time from2s to10s at16kV.As seen from the data in Figure3,it was found that the peak height increases with the increasing injection time.When the injection time is longer than8s,the peak height nearly levels off and peak broadening becomes more severe.In this experiment,8s (16kV)is selected as the optimum injection time.Effect of irradiation timeFigure4illustrates the effect of the irradiation time of IR-assisted extraction on the peak area of the chlorogenic acid in the extracts of a sample of honeysuckle.Upon increasing the irradiation time of IR-assisted extraction from10to40min, the peak area of the analytes increase to the maximum values at the time of30min,and they decrease gradually when the irradiation time is more than30min.This can be attributed to decomposition of the analytes.Hence,30min was selected as the optimum irradiation time.Effect of anhydrous ethanol concentration on extraction efficiency of chlorogenic acid in honeysuckleThe influence of the anhydrous ethanol concentration on the extraction efficiency was studied.As seen from Figure5, Figure3.Effect of the injection time on the peak current of the chlorogenicacid.Figure4.Effect of the irradiation time of IR-assisted extraction on the peak area of the chlorogenic acid inhoneysuckle. Figure2.The IR-assisted extraction apparatus.78Tang et al.the maximum peak area of chlorogenic acid can be obtained at the anhydrous ethanol concentration of 80%.Based on the results obtained here,the optimum conditions for chlorogenic acid were decided.A 50mmol /L borate buffer (pH 8.7)was used as the running buffer at a separation voltage of 16kV.Samples were injected electrokinetically at 16kV for 8s.The typical electropherogram for a standard solution of the chlorogenic acid is shown in Figure 6A,and it is seen that good separation can be achieved within 15min.Method validationAppropriate method validation information concerning new analytical techniques for analyzing pharmaceuticals is required by regulatory authorities.Validation of such methods included assessment of the stability of the solutions,linearity,reproduci-bility,detection limits,and quantification limits.Stability of the solutionsThe stability of the standard and sample solutions was deter-mined by monitoring the peak area of standard chlorogenic acid solutions and sample solutions over a period of one day.The results showed that the peak area and migration time of chlorogenic acid was almost unchanged [relative standard devi-ation (RSD %),4.8]and that no significant degradation is observed within the given period,indicating the solutions are stable for at least one week.Linearity repeatability,detection limits,and quantification limitsTo determine the linearity of the peak area response on the concentration of chlorogenic acid,a series of standard chlorogenic acid solutions with concentrations from 0.2to 400m g /mL were tested.The calibration curve for quantifying chlorogenic acid was y ¼538546x þ348.44.Where y is the chlorogenic acid peak area and X is the chlorogenic acid con-centration (m g /mL),with a correlation coefficient (for R 2)of 0.9996;the linear range of the chlorogenic acid is 0.2–400m g /mL.The reproducibility of the peak area and migration time was estimated by making repetitive injections of a standard chloro-genic acid solution (40m g /mL for chlorogenic acid)under the optimum conditions.The RSD of the peak area and the migration time were 3.5%and 3.8%for chlorogenic acid(n ¼7).The detection limit of 0.05m g /mL is based on a signal-to-noise ratio of 3.The calibration curves exhibit excel-lent linear behavior over the concentration range of about three orders of magnitude with the detection limit of 0.05m g /mL for the analyte.The LOQ is defined as the level at (or above)which the measurement precision is satisfactory for quantitative analysis.In this case,the LOQ was evaluated on the basis of a signal-to-noise ratio of 10.The LOQ was 0.15m g /mL for chloro-genic acid.The RSD of the LOD and LOQ were 3.7%and 3.9%for chlorogenic acid (n ¼3).Practical sample analysis and recoveryUnder the optimum conditions,CE was applied for the deter-mination of chlorogenic acid in honeysuckle in combination with IR-assisted extraction.Typical electropherograms for a chlorogenic acid standard and for an IR-assisted extract of honeysuckle are shown in Figures 6A and 6B.The main com-pound in honeysuckle was identified as chlorogenic acid.The results show that chlorogenic acid in honeysuckle is 1.86m g /mL,with a relative standard deviation of 3.5%.As seen in Figure 7,the peak area of the chlorogenic acid in the same herb sample obtained by using CE coupled with IR-assisted extraction was higher than that obtained with the heat-solvent extraction,indicating that an IR-assisted extraction approachisFigure 6.The electropherogram of a standard solution (4.0Â1025g /mL for chlorogenic acid)(A),and the typical electropherogram of herb honeysuckle with IR-assisted extraction (B).Peak identification:1¼chlorogenicacid.Figure 5.Effect of anhydrous ethanol concentration on the peak area of the chlorogenic acid in honeysuckle.Detection of chlorogenic acid in honeysuckle 79more efficient than the conventional heat-solvent extraction when the extraction times were the same.Under the optimum conditions,the recovery and reproduci-bility experiments were also conducted to evaluate the preci-sion and accuracy of the method.The recovery was determined by a standard addition method.The average recov-eries and RSDs for the analytes are listed in Table I (n ¼3).ConclusionThis work presents the first application of CE for the qualita-tive and quantitative assay of chlorogenic acid in honeysuckle with the aid of IR-assisted extraction with higher extraction efficiency.The assay results mentioned herein indicate that this method is accurate,sensitive,and reproducible.AcknowledgementsThis paper was supported by 973Program,2007CB914100/3,863project:2006AA02A308,Shanghai Leading Academic Discipline Project,B109,Shenyang Ligong University Doctor Project.References1.Li,L.N.Biologically active components from traditional Chinese medicines.Pure Appl.Chem.1998,70,547–554.2.Peng,Y.Y.;Liu,F.H.;Ye,J.N.Determination of phenolic acids and fla-vones in Lonicera japonica Thumb.by capillary electrophoresis with electrochemical detection.Electroanalysis 2005,17,356–362.3.Zhang,E.Q.;Qu,J.F.;Zhang,S.H.;Xie,R.The Chinese Material Medica ,Publishing House of Shanghai University of Traditional Chinese Medicine,Shanghai,China,pp.124–127,1990.4.Wang,Z.Y.;Xi,Z.Clinical and laboratorial study of the effect of antilupus pill on systemic lupus erythematosus .Zhongguo Zhong Xi Yi Jie He Za Zhi (Chinese)1989,9,465–469.5.Hu,S.;Cai,W.;Ye,J.;Qian,Z.;Sun,Z.Influence of medicinal herbs on phagocytosis by bovine neutrophils.Zentralbl.Veterinarmed.,Reihe A 1992,39,593–599.6.Anikina,E.V.;Syrchina,A.I.;Vereshchagin,A.L.;Larin,M.F.;Semenov,A.A.Bitter iridoid glucoside from the fruit of Lonicera caerulea p .1988,24,512–513.7.Chang,W.C.;Hsu,F.L.Inhibition of platelet activation and endothe-lial cell injury by polyphenolic compounds isolated from Lonicera japonica Thunb.Prostaglandins Leukotrienes and Essential Fatty Acids 1992,45,307–312.8.Hu,Q.F.;Yang,G.Y.;Huang,Z.J.Study on determination of polyphenols in honeysuckle flower by microcolumn high perform-ance liquid chromatography.Chinese J.Anal.Chem.2005,33,69–72.9.Wang,D.;Ji,S.G.Determination of Chlorogenic Acid Content in Compound Lotion of Honeysuckl.J.Henan.Univ.of Chinese Medicine 2003,18,36–37.10.Wang,T.T.;Jiang,X.H.;Yang,L.W U S H,pH-gradient counter—current chromatography isolation of natural antioxidant chloro-genic acid from Lonicera japonica ing an upright coil planet centrifuge with three multi-layer coils connected in series.J.Chroma.A 2008,1180,53–58.11.Lin,Q.Pharmacopoeia of the People’s Republic of China .ThePublic Health Department of the People’s Republic of China,Chemical Industry Press,Beijing,China,pp.177–180,2000.12.Santos,M.D.;Almeida,M.C.;Lopes,N.P.;Souza,G.E.P.Evaluation ofthe anti-inflammatory,analgesic and antipyretic activities of the natural polyphenol chlorogenic acid.Biol.Pharm.Bull.2006,29,2236–2240.13.Nakamura,T.;Nakazawa,Y.;Onizuka,S.Antimutagenicity of Tochutea (an aqueous extract of Eucommia ulmoides leaves) 1.The clastogen-supressing effects of Tochu tea in CHO cells and mice.Mutat.Res.1997,338,7–20.14.Yoshimoto,M.;Yahara,S.;Okuno,S.;Islam,M.S.;Ishiguro,K.;Yamakawa,O.Antimutagenicity of mono-,di-,and tricaffeoylquinic acid derivatives isolated from sweetpotato (Ipomoea batatas L.)Leaf.Biosci.Biotech.Biochem.2002,66,2336–2341.15.Yagasaki,K.Inhibitory effects of chlorogenic acid and its relatedcompounds on the invasion of hepatoma cells in culture.Cytotechnology 2000,33,229–235.16.Kurata,R.;Adachi,M.;Yamakawa,O.;Yoshimoto,M.Growthsuppression of human cancer cells by polyphenolics from sweetpotato (Ipomoea batatas L.)leaves.J.Agric.Food Chem 2007,55,185–190.17.Bandyopadhyay,G.;Biswas,T.;Roy,K.C.;Mandal,S.et al .Chlorogenic acid inhibits Bcr–Abl tyrosine kinase and triggers p38mitogen-activated protein kinase-dependent apoptosis in chronic myelogenous leukemic cells.Blood 2004,104,2514–2522.18.Hu,F.L.;Deng,C.H.;Liu,Y.;Zhang,X.M.Quantitative Determinationof chlorogenic acid in honeysuckle using microwave-assisted ex-traction followed by nano-LC ESI mass spectrometry.Talanta 2009,77,1299–1303.19.Chen,G.;Zhang,L.Y.;Wu,X.L.;Ye,J.N.Determination of mannitoland three sugars in Ligustrum lucidum Ait.by capillary electro-phoresis with electrochemical detection.Anal.Chim.Acta 2005,530,15–21.20.Chen,Y.L.;Duan,G.L.;Xie,M.F.;Chen,B.;Li,Y.Infrared-assistedextraction coupled with high-performance liquid chromatography for simultaneous determination of eight active compounds in Radix Salviae Miltiorrhizae .J.Sep.Sci.2010,33,2888–2897.Table IDetermination Results of the Recovery for this Method with the Herb Honeysuckle Sample (n ¼3)SampleIngredientOriginal amount (m g /mL)Added amount (m g /mL)Found amount (m g /mL)Recovery (%)RSD (%)Herbchlorogenic 46.61100153.23106.62 3.7Honeysuckle acid93.22100188.7595.53 4.174.58100169.3294.743.9Figure parison of peak height of chlorogenic acid in the extract of honeysuckle by IR-assisted extraction and heat-solvent extraction at 30mins.80Tang et al.。
- 1、下载文档前请自行甄别文档内容的完整性,平台不提供额外的编辑、内容补充、找答案等附加服务。
- 2、"仅部分预览"的文档,不可在线预览部分如存在完整性等问题,可反馈申请退款(可完整预览的文档不适用该条件!)。
- 3、如文档侵犯您的权益,请联系客服反馈,我们会尽快为您处理(人工客服工作时间:9:00-18:30)。
Extracting and Evaluating General World Knowledge from the BrownCorpusLenhart SchubertUniversity of Rochester schubert@Matthew TongUniversity of Rochestermt004i@AbstractWe have been developing techniques for ex-tracting general world knowledge from miscel-laneous texts by a process of approximate inter-pretation and abstraction,focusing initially onthe Brown corpus.We apply interpretive rulesto clausal patterns and patterns of modifica-tion,and concurrently abstract general“possi-bilistic”propositions from the resulting formu-las.Two examples are“A person may believea proposition”,and“Children may live withrelatives”.Our methods currently yield over117,000such propositions(of variable quality)for the Brown corpus(more than2per sen-tence).We report here on our efforts to eval-uate these results with a judging scheme aimedat determining how many of these propositionspass muster as“reasonable general claims”about the world in the opinion of human judges.Wefind that nearly60%of the extracted propo-sitions are favorably judged according to ourscheme by any given judge.The percentageunanimously judged to be reasonable claims bymultiple judges is lower,but still sufficientlyhigh to suggest that our techniques may be ofsome use in tackling the long-standing“knowl-edge acquisition bottleneck”in AI.1Introduction:deriving generalknowledge from textsWe have been exploring a new method of gaining gen-eral world knowledge from texts,includingfiction.The method does not depend on full or exact interpretation, but rather tries to glean general facts from particulars by combined processes of compositional interpretation and abstraction.For example,consider a sentence such as the following from the Brown corpus(Kucera and Francis, 1967):Rilly or Glendora had entered her room while she slept,bringing back her washed clothes.From the clauses and patterns of modification of this sen-tence,we can glean that an individual may enter a room,a female individual may sleep,and clothes may be washed.In fact,given the following Treebank bracketing,our pro-grams produce the output shown:((S(NP(NP(NNP Rilly))(CC or)(NP(NNP Glendora)))(AUX(VBD had))(VP(VBN entered)(NP(PRP\$her)(NN room)))(SBAR(IN while)(S(NP(PRP she))(VP(VBD slept))))(\,\,)(S(NP(\-NONE\-\*))(VP(VBG bringing)(PRT(RB back))(NP(PRP\$her)(JJ washed)(NNS clothes))))) (\.\.))A NAMED-ENTITY MAY ENTER A ROOM.A FEMALE-INDIVIDUAL MAY HAVE A ROOM.A FEMALE-INDIVIDUAL MAY SLEEP.A FEMALE-INDIVIDUAL MAY HAVE CLOTHES.CLOTHES CAN BE WASHED.((:I(:Q DET NAMED-ENTITY)ENTER[V](:Q THE ROOM[N]))(:I(:Q DET FEMALE-INDIVIDUAL)HAVE[V](:Q DET ROOM[N])) (:I(:Q DET FEMALE-INDIVIDUAL)SLEEP[V])(:I(:Q DET FEMALE-INDIVIDUAL)HAVE[V](:Q DET(:F PLUR CLOTHE[N]))) (:I(:Q DET(:F PLUR CLOTHE[N]))WASHED[A]))The results are produced as logical forms(the lastfive lines above–see Schubert,2002,for some details),from which the English glosses are generated automatically. Our work so far has focused on data in the Penn Tree-bank(Marcus et al.,1993),particularly the Brown corpus and some examples from the Wall Street Journal corpus. The advantage is that Treebank annotations allow us to postpone the challenges of reasonably accurate parsing, though we will soon be experimenting with“industrialstrength”parsers on unannotated texts.We reported some specifics of our approach and some preliminary results in(Schubert,2002).Since then we have refined our extraction methods to the point where we can reliably apply them the Treebank corpora,on average extracting more than2generalized propositions per sen-tence.Applying these methods to the Brown corpus,we have extracted137,510propositions,of which117,326 are distinct.Some additional miscellaneous examples are“A PERSON MAY BELIEVE A PROPOSITION”,“B ILLS MAY BE APPROVED BY COMMITTEES”,“A US-STATE MAY HAVE HIGH SCHOOLS”,“C HILDREN MAY LIVE WITH RELA-TIVES”,“A COMEDY MAY BE DELIGHTFUL”,“A BOOK MAY BE WRITE-ED(i.e.,written)BY AN AGENT”,“A FEMALE-INDIVIDUAL MAY HAVE A SPOUSE”,“A N ARTERY CAN BE THICKENED”,“A HOUSE MAY HAVE WINDOWS”,etc.The programs that produce these results consist of(1)a Treebank preprocessor that makes various modifications to Treebank trees so as to facilitate the extraction of se-mantic information(for instance,differentiating different kinds of“SBAR”,such as S-THAT and S-ALTHOUGH, and identifying certain noun phrases and prepositional phrases,such as“next Friday”,as temporal);(2)a pat-tern matcher that uses a type of regular-expression lan-guage to identify particular kinds of phrase structure pat-terns(e.g.,verb+complement patterns,with possible in-serted adverbials or other material);(3)a semantic pat-tern extraction routine that associates particular semantic patterns with particular phrase structure patterns and re-cursively instantiates and collects such patterns for the preprocessed tree,in bottom-up fashion;(4)abstraction routines that abstract away modifiers and other“type-preserving operators”,before semantic patterns are con-structed at the next-higher level in the tree(for instance, stripping the interpreted modifier“washed”from the in-terpreted noun phrase“her washed clothes”);(5)routines for deriving propositional patterns from the resulting mis-cellaneous semantic patterns,and rendering them in a simple,approximate English form;and(6)heuristic rou-tines forfiltering out many ill-formed or vacuous propo-sitions.In addition,semantic interpretation of individual words involves some simple morphological analysis,for instance to allow the interpretation of(VBD SLEPT)in terms of a predicate SLEEP[V].In(Schubert,2002)we made some comparisons be-tween our project and earlier work in knowledge extrac-tion(e.g.,(muc,1993;muc,1995;muc,1998;Berland and Charniak,1999;Clark and Weir,1999;Hearst,1998; Riloff and Jones,1999))and in discovery of selectional preferences(e.g.,(Agirre and Martinez,2001;Grishman and Sterling,1992;Resnik,1992;Resnik,1993;Zernik, 1992;Zernik and Jacobs,1990)).Reiterating briefly,we note that knowledge extraction work has generally em-ployed carefully tuned extraction patterns to locate and extract some predetermined,specific kinds of facts;our goal,instead,is to process every phrase and sentence that is encountered,abstracting from it miscellaneous general knowledge whenever possible.Methods for discovering selectional preferences do seek out conventional patterns of verb-argument combination,but tend to“lose the con-nection”between argument types(e.g.,that a road may carry traffic,a newspaper may carry a story,but a road is unlikely to carry a story);in any event,they have not led so far to amassment of data interpretable as general world knowledge.Our concern in this paper is with the evaluation of the results we currently obtain for the Brown corpus.The overall goal of this evaluation is to gain some idea of what proportion of the extracted propositions are likely to be credible as world knowledge.The ultimate test of this will of course be systems(e.g.,QA systems)that use such extracted propositions as part of their knowledge base,but such a test is not immediately feasible.In the meantime it certainly seems worthwhile to evaluate the outputs subjectively with multiple judges,to determine if this approach holds any promise at all as a knowledge acquisition technique.In the following sections we describe the judging method we have developed,and two experiments based on this method,one aimed at determining whether“lit-erary style makes a difference”to the quality of outputs obtained,and one aimed at assessing the overall success rate of the extraction method,in the estimation of several judges.2Judging the output propositionsWe have created judging software that can be used by the researchers and other judges to assess the quality and cor-rectness of the extracted information.The current scheme evolved from a series of trial versions,starting initially with a3-tiered judging scheme,but this turned out to be difficult to use,and yielded poor inter-judge agree-ment.We ultimately converged on a simplified scheme, for which ease of use and inter-judge agreement are sig-nificantly better.The following are the instructions to a judge using the judger program in its current form:Welcome to the sentence evaluator for the KNEXT knowledge ex-traction program.Thank you for your participation.You will beasked to evaluate a series of sentences based on such criteria ascomprehensibility and truth.Do your best to give accurate re-sponses.The judgement categories are selected to try to ensurethat each sentencefits best in one and only one category.Help isavailable for each menu item,along with example sentences,byselecting’h’;PLEASE consult this if this is yourfirst time usingthis program even if you feel confident of your choice.There isalso a tutorial available,which should also be done if this is yourfirst time.If youfind it hard to make a choice for a particular sen-tence even after carefully considering the alternatives,you shouldprobably choose6(HARD TO JUDGE)!But if you strongly feelnone of the choicesfit a sentence,even after consulting the helpfile,please notify Matthew Tong(mtong@)to al-low necessary modifications to the menus or available help infor-mation to occur.You may quit at any time by typing’q’;if you quit partway through the judgement of a sentence,that partial judge-ment will be discarded,so the best time to quit is right after being presented with a new sentence.here thefirst sentence to be judged is presentednewly sampled propositions from the4files)using the6-category judging scheme,and the heuristic postprocess-ing andfiltering routines,yielded the following unequiv-ocal results.(The exact sizes of the samples fromfiles ck01,ck13,cd01,and cd02in both repetitions were120, 98,85,and97respectively,where the relatively high count for ck01reflects the relatively high count of ex-tracted propositions for that text.)For ck01and ck13around73%of the propositions (159/218for judge1and162/218for judge2)were judged to be in the“reasonable general claim”cat-egory;for cd01and cd02,thefigures were much lower,at41%(35/85for judge1and40/85for judge2)and less than55%(53/97for judge1and47/97for judge2)respectively.For ck01and ck13the counts in the“hard to judge”category were12.5-15%(15-18/120)and7.1-8.2% (6-7/85)respectively,while for cd01and cd02the figures were substantially higher,viz.,25.9-28.2% (22-24/85)and19.6-23%(19-34/97)respectively. Thus,as one would expect,simple narrative texts yield more propositions recognized as reasonable claims about the world(nearly3out of4)than abstruse an-alytical materials(around1out of2).The question then is then how to control for style when we turn our methods to larger corpora.One obvious answer is to hand-select texts in relevant categories,such as literature for young readers,or from authors whose writings are realistic and stylistically simple(e.g.,Hem-ingway).However,this could be quite laborious since large literary collections available online(such as the works in Project Gutenberg,/pg/, /gtn/,with expired copyrights)are not sorted by style.Thus we expect to use automated style analysis methods,taking account of such factors as vocabulary(checking for esoteric vocabulary and vocabulary indicative of fairy tales and other fanciful fiction),tense(analytical material is often in present tense),etc.We may also turn our knowledge extraction methods themselves to the task:if,for instance,wefind propositions about animals talking,it may be best to skip the text source altogether.2.2Overall quality of extracted propositionsTo assess the quality of extracted propositions over a wide variety of Brown corpus texts,with judgements made by multiple judges,the authors and three other individuals made judgements on the same set of250extracted propo-sitions.The propositions were extracted from the third of the Brown corpus(186files)that had been annotated with WordNet senses in the SEMCOR project(Landes et al.,1998)(chiefly because those were thefiles at hand when we started the experiment–but they do represent a broad cross-section of the Brown Corpus materials).We excluded the cj-files,which contain highly technical ma-terial.Table1shows the judgements of the5judges(as per-centages of counts out of250)in each of the six judge-ment categories.The category descriptions have been mnemonically abbreviated at the top of the table.Judge 1appears twice,and this represents a repetition,as a test of self-consistency,of judgements on the same data pre-sented in different randomized orderings.reasonable obscure vacuous false incomplete hard9.60.47.612.89.60.47.211.654.814.8 5.68.8 5.26.4 3.27.68.4 4.860.09.661.649.010.49.22.88.412.410.022.5judge 1judge 1judge 2judge 464.0judge 5judge 358.4 4.40.8 2.810.023.2Judgements (in %) for 250 randomly sampled propositions Table 1.As can be seen from thefirst column,the judges placed about49-64%of the propositions in the”reasonable gen-eral claim”category.This result is consistent with the re-sults of the style-dependency study described above,i.e., the average lies between the ones for“straightforward”narratives(which was nearly3out of4)and the ones for abstruse texts(which was around1out of2).This is an encouraging result,suggesting that mining general world knowledge from texts can indeed be productive.One point to note is that the second and third judge-ment categories need not be taken as an indictment of the propositions falling under them–while we wanted to dis-tinguish overly specific,obscure,or vacuous propositions from ones that seem potentially useful,such propositions would not corrupt a knowledge base in the way the other categories would(false,incomplete,or incoherent propo-sitions).Therefore,we have also collapsed our data into three more inclusive categories,namely“true”(collaps-ing thefirst3categories),“false”(same as the original “false”category),and“undecidable”(collapsing the last two categories).The corresponding variant of Table1 would thus be obtained by summing thefirst3and last 2columns.We won’t do so explicitly,but it is easy to verify that the proportion of“true”judgements comprise about three out of four judgements,when averaged over the5judges.We now turn to the extent of agreement among the judgements of thefive judges(and judge1with himself on the same data).The overall pairwise agreement results for classification into six judgement catagories are shownin Table 2.judge 190.156.910.461.762.457.358.5judge 1judge 2judge 3judge 4judge 554.556.049.3judge 2judge 3judge 4Table 2.Overall % agreement among judgesfor 250 propositions60.1A commonly used metric for evaluating interrater relia-bility in categorization of data is the kappa statistic (Car-letta,1996).As a concession to the popularity of that statistic,we compute it in a few different ways here,though –as we will explain –we do not consider it par-ticularly appropriate.For 6judgement categories,kappa computed in the conventional way for pairs of judges ranges from.195to.367,averaging.306.For 3(more in-clusive)judgement categories,the pairwise kappa scores range from.303to .462,with an average of .375.These scores,though certainly indicating a positive correlation between the assessments of multiple judges,are well below the lower threshold of.67often employed in deciding whether judgements are sufficiently consis-tent across judges to be useful.However,to see that there is a problem with applying the conventional statistic here,imagine that we could improve our extraction methods to the point where 99%of extracted propositions are judged by miscellaneous judges to be reasonable general claims.This would be success beyond our wildest dreams –yet the kappa statistic might well be 0(the worst possible score),if the judges generally reject a different one out of every one hundred propositions!One somewhat open-ended aspect of the kappa statistic is the way “expected”agreement is calculated.In the con-ventional calculation (employed above),this is based on the observed average frequency in each judgement cate-gory.This leads to low scores when one category is over-whelmingly favored by all judges,but the exceptions to the favored judgement vary randomly among judges (as in the hypothetical situation just described).A possible way to remedy this problem is to use a uniform distri-bution over judgement categories to compute expected agreement.Under such an assumption,our kappa scores are significantly better:for 6categories,they range from .366to .549,averaging.482;for 3categories,they range from .556to .730,averaging .645.This approaches,and for several pairs of judges exceeds,the minimum thresh-old for significance of the judgements.1Since the ideal result,as implied above,would be agreement by multiple judges on the “reasonableness”or truth of a large proportion of extracted propositions,it seems worthwhile to measure the extent of such agree-ment as well.Therefore we have also computed the “survival rates”of extracted propositions,when we re-ject those not judged to be reasonable general claims by judges (or,in the case of 3categories,not judged to be true by judges).Figure 1shows the results,where the survival rate for judges is averaged over all subsets of size of the 5available judges.12345.6.8.4.201Fraction of survivors number of concurring judges"true" (3 categories)(6 categories)"reasonable general claim"by multiple judges.75.65.31.28.35.43.57.59.52.55Fraction of propositions placed in best category Figure 1.Thus we find that the survival rate for “reasonable gen-eral claims”starts off at 57%,drops to 43%and then 35%for 2and 3judges,and drops further to 31%and 28%for 4and 5judges.It appears as if an asymptotic level above 20%might be reached.But this may be an unrealistic extrapolation,since virtually any proposition,no matter how impeccable from a knowledge engineering perspec-tive,might eventually be relegated to one of the other 5categories by some uninvolved judge.The survival rates based on 2or 3judges seem to us more indicative of the likely proportion of (eventually)useful propositions than an extrapolation to infinitely many judges.For the 3-way judgements,we see that 75%of extracted propositions are judged “true”by individual judges (as noted earlier),and this drops to 65%and then 59%for 2and 3judges.Though again sufficiently many judges may eventually bring this down to 40%or less,the survival rate is cer-tainly high enough to support the claim that our method of deriving propositions from texts can potentially deliver very large amounts of world knowledge.3Conclusions and further workWe now know that large numbers of intuitively reason-able general propositions can be extracted from a cor-pus that has been bracketed in the manner of the Penn Treebank.The number of“surviving”propositions for the Brown corpus,based on the judgements of multiple judges,is certainly in the tens of thousands,and the dupli-cation rate is a rather small fraction of the overall number (about15%).Of course,there is the problem of screening out,as far as possible,the not-so-reasonable propositions.One step strongly indicated by our experiment on the effect of style is to restrict extraction to the kinds of texts that yield higher success rates–namely those written in straightfor-ward,unadorned language.As we indicated,both style analysis techniques and our own proposition extraction methods could be used to select stylistically suitable ma-terials from large online corpora.Even so,a significant residual error rate will remain. There are two remedies–a short-term,brute-force rem-edy,and a longer-term computational remedy.The brute-force remedy would be to hand-select acceptable propo-sitions.This would be tedious work,but it would still be far less arduous than“dreaming up”such proposi-tions;besides,most of the propositions are of a sort one would not readily come up with spontaneously(“A per-son may paint a porch”,“A person may plan an attack”,“A house may have a slate roof”,“Superstition may blend with fact”,“Evidence of shenanigans may be gathered by a tape recorder”,etc.)The longer-term computational remedy is to use a well-founded parser and grammar,providing syntactic analyses better suited to semantic interpretation than Treebank trees.Our original motivation for using the Penn Treebank,apart from the fact that it instantly pro-vides a large number of parsed sentences from miscella-neous genres,was to determine how readily such parses might permit semantic interpretation.The Penn Tree-bank pays little heed to many of the structural principles and features that have preoccupied linguists for decades. Would these turn out to be largely irrelevant to seman-tics?We were actually rather pessimistic about this, since the Treebank data tacitly posit tens of thousands of phrase structure rules with inflated,heterogeneous right-hand sides,and phrase classifications are very coarse(no-tably,with no distinctions between adjuncts and com-plements,and with many clause-like constructs,whether infinitives,subordinate clauses,clausal adverbials,nom-inalized questions,etc.,lumped together as”SBAR”–and these are surely semantically crucial distinctions).So we are actually surprised at our degree of success in ex-tracting sensible general propositions on the basis of such rough-and-ready syntactic annotations.Nonetheless,our extracted propositions in the“some-thing missing”and“hard to judge”categories do quite often reflect the limitations of the Treebank analyses.For example,the incompleteness of the proposition“A male-individual may attach an importance”seen above as an il-lustration of judgement category5can be attributed to the lack of any indication that the PP[to]constituent of the verb phrase in the source sentence is a verb complement rather than an adjunct.Though our heuristics try to sort out complements from adjuncts,they cannot fully make up for the shortcomings of the Treebank annotations.It therefore seems clear that we will ultimately need to base knowledge extraction on more adequate syntactic analy-ses than those provided by the Brown annotations. Another general conclusion concerns the ease or dif-ficulty of broad-coverage semantic interpretation.Even though our interpretive goals up to this point have been rather modest,our success in providing rough semantic rules for much of the Brown corpus suggests to us that full,broad-coverage semantic interpretation is not very far out of reach.The reason for optimism lies in the“sys-tematicity”of interpretation.There is no need to hand-construct semantic rules for each and every phrase struc-ture rule.We were able provide reasonably comprehen-sive semantic coverage of the many thousands of distinct phrase types in Brown with just80regular-expression patterns(each aimed at a class of related phrase types) and corresponding semantic rules.Although our seman-tic rules do omit some constituents(such as prenominal participles,non-initial conjuncts in coordination,adver-bials injected into the complement structure of a verb, etc.)and gloss over subtleties involving gaps(traces), comparatives,ellipsis,presupposition,etc.,they are not radical simplifications of what would be required for full interpretation.The simplicity of our outputs is due not so much to oversimplification of the semantic rules,as to the deliberate abstraction and culling of information that we perform in extracting general propositions from a specific sentence.Of course,what we mean here by semantic in-terpretation is just a mapping to logical form.Our project sheds no light on the larger issues in text understanding such as referent determination,temporal analysis,infer-ence of causes,intentions and rhetorical relations,and so on.It was the relative independence of the kind of knowledge we are extracting of these issues that made our project attractive and feasible in thefirst place. Among the miscellaneous improvements under consid-eration are the use of lexical distinctions and WordNet abstraction to arrive at more reliable interpretations;the use of modules to determine the types of neuter pronouns and of traces(e.g.,in“She looked in the cookie jar,but it was empty”,we should be able to abstract the proposition that a cookie jar may be empty,using the referent of“it”); and extracting properties of events by making use of in-formation in adverbials(e.g.,,from“He slept soundly”we should be able to abstract the proposition that sleep may be sound;also many causal propositions can be in-ferred from adverbial constructions).We also hope to demonstrate extraction results through knowledge elici-taton questions(e.g.,“What do you know about books?”, etc.)4AcknowledgementsThe authors are grateful to David Ahn for contributing ideas and for extensive help in preparing and processing Brown corpusfiles,conducting some of the reported ex-periments,and performing some differential analyses of results.We also benefited from the discussions and ideas contributed by Greg Carlson and Henry Kyburg in the context of our“Knowledge Mining”group,and we ap-preciate the participation of group members and outside recruits in the judging experiments.As well,we thank Peter Clark and Phil Harrison(at Boeing Company)for their interest and suggestions.This work was supported by the National Science Foundation under Grant No.IIS-0082928.ReferencesEneko Agirre and David Martinez.2001.Learning class-to-class selectional preferences.In Proc.of the 5th Workshop on Computational Language Learning (CoNLL-2001),Toulouse,France,July6-7,. Matthew Berland and Eugene Charniak.1999.Find-ing parts in very large corpora.In Proc.of the37th Ann.Meet.of the Assoc.for Computational Linguistics (ACL-99),Univ.of Maryland,June22-27,.Jean Carletta.1996.Assessing agreement on classifica-tion tasks:the kappa putational Linguis-tics,22(2):249–254.Stephen Clark and David Weir.1999.An iterative approach to estimating frequencies over a semantic hierarchy.In Proc.of the Joint SIGDAT Confer-ence on Empirical Methods in Natural Language Pro-cessing and Very Large Corpora.Also available at /users/davidw/research/ papers.html.Ralph Grishman and John Sterling.1992.Acquisition of selectional patterns.In Proc.of COLING-92,pages 658–664,Nantes,France.Marti A.Hearst.1998.Automated discovery of Word-Net relations.In Christiane Fellbaum,editor,Word-Net:An Electronic Lexical Database,pages131–153? MIT Press.H.Kucera and putational Anal-ysis of Present-Day American English.Brown Univer-sity Press,Providence,RI.Shari Landes,Claudia Leacock,and Randee I.Tengi. 1998.Building semantic concordances.In Chris-tiane Fellbaum,editor,WordNet:An Electronic Lexi-cal Database,pages chapter8,199–216.MIT Press, Cambridge,MA.Mitchell P.Marcus,Beatrice Santorini,and Mary Ann Marcinkiewicz.1993.Building a large annotated cor-pus of English:The Penn putational Linguistics,19(2):313–330,June.1993.Proc.of the5th Message Understanding Confer-ence(MUC-5).Morgan Kaufmann,Los Altos,CA.1995.Proc.of the6th Message Understanding Confer-ence(MUC-6).Morgan Kaufmann,Los Altos,CA.1998.Proc.of the7th Message Understanding Confer-ence(MUC-7).Morgan Kaufmann,Los Altos,CA, April29–May1,Virginia.P.Resnik.1992.A class-based approach to lexical dis-covery.In Proc.of the30th Ann.Meet.of the Assoc. for Computational Linguistics(ACL-92),pages327–329,Newark,DE.P.Resnik.1993.Semantic classes and syntactic ambigu-ity.In Proc.of ARPA Workshop on Human Language Technology,Plainsboro,NJ.Ellen Riloff and Rosie Jones.1999.Learning dictio-naries for information extraction by multi-level boot-strapping.In Proc.of the16th Nat.Conf.on Artificial Intelligence(AAAI-99).Lenhart K.Schubert.2002.Can we derive general world knowledge from texts?In Proc.of2nd Int.Conf.on Human Language Technology Research(HLT2002), pages94–97,San Diego,CA,March24-27.Uri Zernik and Paul Jacobs.1990.Tagging for learning: Collecting thematic relations from corpus.In Proc. of the13th Int.Conf.on Computational Linguistics (COLING-90),pages34–39,Helsinki.Uri Zernik.1992.Closed yesterday and closed minds: Asking the right questions of the corpus to distin-guish thematic from sentential relations.In Proc.of COLING-92,pages1304–1311,Nantes,France,Aug. 23-28,.。