日本佐贺大学E-EMG基于控制人体上肢动力辅助的肌肉疲劳的影响研究
肌电图(EMG)在运动生物力学研究中的应用

肌电图(EMG)在运动生物力学研究中的应用王琨;李小生;宋姌;富仁杰;郭晓慧【摘要】主要通过文献研究,从应用的角度出发,对肌电图(EMG)在运动生物力学研究中的相关研究进行综述.包括EMG的测量、结果的处理与分析、应用研究成果、存在问题和应用展望.重点对目前的研究提出问题并进行探讨,为EMG在运动生物力学中的进一步研究与应用提出思考和帮助.【期刊名称】《体育科研》【年(卷),期】2014(035)001【总页数】4页(P31-33,38)【关键词】肌电图(EMG);运动生物力学;应用研究;展望【作者】王琨;李小生;宋姌;富仁杰;郭晓慧【作者单位】西安体育学院健康科学系,西安 710086;西安体育学院健康科学系,西安 710086;西安体育学院研究生部,西安 710068;西安体育学院研究生部,西安710068;西安体育学院研究生部,西安 710068【正文语种】中文【中图分类】G804.6肌肉(骨骼肌)是人体运动系统重要的组成部分,是人体主动运动的动力来源。
肌肉的生物力学研究是对人体运动产生变化进一步深入研究的基础,也最具吸引力和挑战性。
肌电图(EMG)测量与分析作为肌肉生物力学研究的重要方法与手段之一,得到了深入研究和广泛应用。
在运动生物力学研究中,肌肉的EMG测量主要是为了获得肌肉活动的信息和收缩特征,包括有肌肉(群)活动的时程(顺序)、肌肉收缩强度(力量)和肌肉疲劳。
从而,进一步探究和揭示运动的本质与特征。
本文主要依据目前的相关研究成果,从应用的角度出发,对EMG的应用测量、结果的处理与分析和应用实例进行综述,并对目前的研究提出问题进行探讨与展望。
目的在于为EMG在运动生物力学中的进一步研究与应用提出思考和帮助。
1 EMG的测量1.1 测量设备肌电图仪的种类和型号较多,从测量方式上,分为有线测量和无线遥测两种;从引导电极使用上,分为针电极肌电图测量和表面电极肌电图(sEMG)测量,前者主要用于临床或动物实验,后者广泛应用于运动人体科学研究。
绳驱动上肢康复机器人动力学建模及仿真
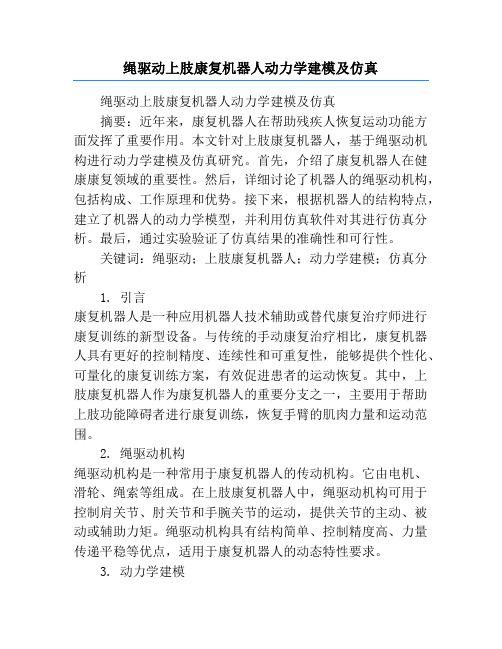
绳驱动上肢康复机器人动力学建模及仿真绳驱动上肢康复机器人动力学建模及仿真摘要:近年来,康复机器人在帮助残疾人恢复运动功能方面发挥了重要作用。
本文针对上肢康复机器人,基于绳驱动机构进行动力学建模及仿真研究。
首先,介绍了康复机器人在健康康复领域的重要性。
然后,详细讨论了机器人的绳驱动机构,包括构成、工作原理和优势。
接下来,根据机器人的结构特点,建立了机器人的动力学模型,并利用仿真软件对其进行仿真分析。
最后,通过实验验证了仿真结果的准确性和可行性。
关键词:绳驱动;上肢康复机器人;动力学建模;仿真分析1. 引言康复机器人是一种应用机器人技术辅助或替代康复治疗师进行康复训练的新型设备。
与传统的手动康复治疗相比,康复机器人具有更好的控制精度、连续性和可重复性,能够提供个性化、可量化的康复训练方案,有效促进患者的运动恢复。
其中,上肢康复机器人作为康复机器人的重要分支之一,主要用于帮助上肢功能障碍者进行康复训练,恢复手臂的肌肉力量和运动范围。
2. 绳驱动机构绳驱动机构是一种常用于康复机器人的传动机构。
它由电机、滑轮、绳索等组成。
在上肢康复机器人中,绳驱动机构可用于控制肩关节、肘关节和手腕关节的运动,提供关节的主动、被动或辅助力矩。
绳驱动机构具有结构简单、控制精度高、力量传递平稳等优点,适用于康复机器人的动态特性要求。
3. 动力学建模为了对上肢康复机器人进行控制和优化设计,需要建立其动力学模型。
根据绳驱动机构的工作原理和机器人的结构特点,可以将机器人简化为刚体连接的多关节链模型,并利用拉格朗日方程进行动力学建模。
模型的状态变量包括关节角度、关节角速度和关节力矩,可用于描述机器人的运动学和动力学特性。
4. 仿真分析利用仿真软件,可以对上肢康复机器人的动力学模型进行仿真分析。
通过设定初始条件和控制策略,可以模拟机器人在不同工况下的运动轨迹和关节力矩变化。
仿真分析可以帮助我们深入理解机器人的运动特性、力学特性和控制特性,为机器人的优化设计和运动控制提供参考。
基于肌电控制的辅助型机器人外骨骼

基于肌电控制的辅助型机器人外骨骼王杜;桂凯;曹红升;张定国【摘要】针对臂丛神经损伤患者上肢功能部分或完全丧失的问题,本文设计了一款基于肌电控制的辅助型机器人外骨骼.该外骨骼具有2个自由度,受试者穿戴上之后能完成5个上肢动作.采用腱鞘驱动方式设计外骨骼结构,减轻受试者上肢使用负担.通过提取受试者健侧手臂产生的肌电信号,使用基于贝叶斯决策的线性判别分析方法解码受试者运动意图,实现利用健侧上肢运动带动患侧运动的目的.而本研究最终目标则是通过解码患侧肌电信号控制患侧上肢运动,实现受试者在不需要任何帮助的前提下重建患侧上肢运动功能.系统对肌电信号的处理、识别均为在线进行,整个过程耗时约57 ms,完全符合外骨骼使用过程中的延时要求.对4名健康受试者进行实验,结果表明5类动作的平均在线识别率均达到95%以上,且受试者能够顺畅使用所设计外骨骼系统.【期刊名称】《燕山大学学报》【年(卷),期】2018(042)003【总页数】7页(P219-224,258)【关键词】臂丛神经损伤;外骨骼系统;表面肌电信号;线性判别分析;在线识别【作者】王杜;桂凯;曹红升;张定国【作者单位】上海交通大学机械与动力工程学院,上海201100;上海交通大学机械与动力工程学院,上海201100;上海交通大学机械与动力工程学院,上海201100;上海交通大学机械与动力工程学院,上海201100【正文语种】中文【中图分类】TP2420 引言臂丛神经损伤是属于周围神经损伤中的上肢神经损伤,多由牵拉伤、切割伤、挤压伤、医源性损伤等原因引起[1]。
由于臂丛神经主要支配肩部、肘部、手部以及胸背等的运动和感觉,其损伤后造成患者上肢功能部分的或者完全的丧失,极大地影响了患者的正常生活,臂丛神经损伤也因此被称为最严重的周围神经损伤之一。
比较幸运的是,臂丛神经损伤患者在经过神经移位术[2]并经过一定时间的主动康复训练之后,能够在其上臂相应肌肉位置处检测到微弱的表面肌电信号(sEMG)。
模拟驾驶过程中腰部疲劳的表面肌电信号分析
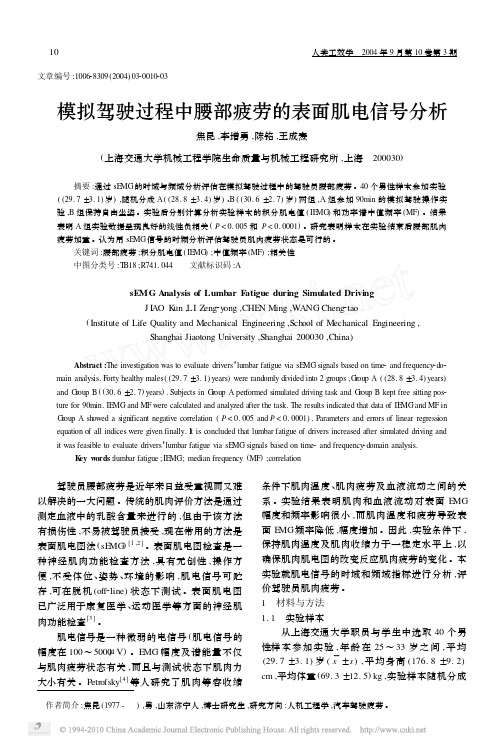
文章编号:100628309(2004)0320010203 作者简介:焦昆(1977- ),男,山东济宁人,博士研究生,研究方向:人机工程学,汽车驾驶疲劳。
模拟驾驶过程中腰部疲劳的表面肌电信号分析焦昆,李增勇,陈铭,王成焘(上海交通大学机械工程学院生命质量与机械工程研究所,上海 200030) 摘要:通过sE MG 的时域与频域分析评估在模拟驾驶过程中的驾驶员腰部疲劳。
40个男性样本参加实验((29.7±3.1)岁),随机分成A ((28.8±3.4)岁)、B ((30.6±2.7)岁)两组,A 组参加90min 的模拟驾驶操作实验,B 组保持自由坐姿。
实验后分别计算分析实验样本的积分肌电值(IE MG )和功率谱中值频率(MF )。
结果表明A 组实验数据呈现良好的线性负相关(P <0.005和P <0.0001)。
研究表明样本在实验结束后腰部肌肉疲劳加重。
认为用sE MG 信号的时频分析评估驾驶员肌肉疲劳状态是可行的。
关键词:腰部疲劳;积分肌电值(IE MG );中值频率(MF );相关性中图分类号:T B18;R741.044 文献标识码:AsEMG Analysis of Lumbar F atigue during Simulated DrivingJ I AO K un ,LI Z eng 2y ong ,CHE N Ming ,W ANG Cheng 2tao(Institute of Life Quality and Mechanical Engineering ,School of Mechanical Engineering ,Shanghai Jiaotong University ,Shanghai 200030,China ) Abstract :The investigation was to evaluate drivers ’lumbar fatigue via sE MG signals based on time 2and frequency 2do 2main analysis.F orty healthy males ((29.7±3.1)years )were randomly divided into 2groups ,G roup A ((28.8±3.4)years )and G roup B ((30.6±2.7)years ).Subjects in G roup A per formed simulated driving task and G roup B kept free sitting pos 2ture for 90min.IE MG and MF were calculated and analyzed after the task.The results indicated that data of IE MG and MF in G roup A showed a significant negative correlation (P <0.005and P <0.0001).Parameters and errors of linear regression equation of all indices were given finally.I t is concluded that lumbar fatigue of drivers increased after simulated driving and it was feasible to evaluate drivers ’lumbar fatigue via sE MG signals based on time 2and frequency 2domain analysis.K ey w ords :lumbar fatigue ;IE MG;median frequency (MF );correlation 驾驶员腰部疲劳是近年来日益受重视而又难以解决的一大问题。
英语文献翻译稿关节康复机器人上肢理疗和训练(可编辑)
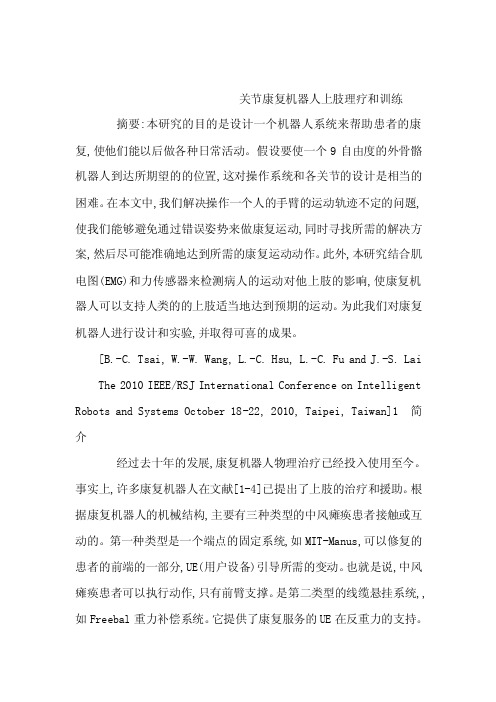
关节康复机器人上肢理疗和训练摘要:本研究的目的是设计一个机器人系统来帮助患者的康复,使他们能以后做各种日常活动。
假设要使一个9自由度的外骨骼机器人到达所期望的的位置,这对操作系统和各关节的设计是相当的困难。
在本文中,我们解决操作一个人的手臂的运动轨迹不定的问题,使我们能够避免通过错误姿势来做康复运动,同时寻找所需的解决方案,然后尽可能准确地达到所需的康复运动动作。
此外,本研究结合肌电图(EMG)和力传感器来检测病人的运动对他上肢的影响,使康复机器人可以支持人类的的上肢适当地达到预期的运动。
为此我们对康复机器人进行设计和实验,并取得可喜的成果。
[B.-C. Tsai, W.-W. Wang, L.-C. Hsu, L.-C. Fu and J.-S. LaiThe 2010 IEEE/RSJ International Conference on Intelligent Robots and Systems October 18-22, 2010, Taipei, Taiwan]1 简介经过去十年的发展,康复机器人物理治疗已经投入使用至今。
事实上,许多康复机器人在文献[1-4]已提出了上肢的治疗和援助。
根据康复机器人的机械结构,主要有三种类型的中风瘫痪患者接触或互动的。
第一种类型是一个端点的固定系统,如MIT-Manus,可以修复的患者的前端的一部分,UE(用户设备)引导所需的变动。
也就是说,中风瘫痪患者可以执行动作,只有前臂支撑。
是第二类型的线缆悬挂系统,,如Freebal重力补偿系统。
它提供了康复服务的UE在反重力的支持。
第三类是外骨骼臂系统,如AR Min。
在这项研究中,我们的康复机器人选择外骨骼类型。
类似人类的机器人臂(外骨骼)的使用已在文献中已经提出各种建议,并对人的手臂的动作的原理进行解释。
所有这些类人机器人的结构通常采用一些特殊的组成部分圆形的导环,以达到人的肩膀的内部/外部旋转。
然而,参与设计的特殊成分,这项研究的目的是在模仿人类的??运动使用的坐标功能和生物启发的标准,以实现我们的目标。
表面肌电信号分析评价肌肉疲劳的有效性和敏感性
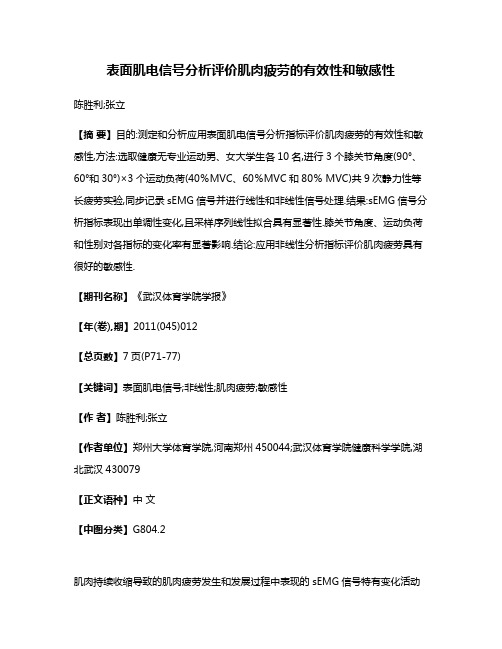
表面肌电信号分析评价肌肉疲劳的有效性和敏感性陈胜利;张立【摘要】目的:测定和分析应用表面肌电信号分析指标评价肌肉疲劳的有效性和敏感性,方法:选取健康无专业运动男、女大学生各10名,进行3个膝关节角度(90°、60°和30°)×3个运动负荷(40%MVC、60%MVC和80% MVC)共9次静力性等长疲劳实验,同步记录sEMG信号并进行线性和非线性信号处理.结果:sEMG信号分析指标表现出单调性变化,且采样序列线性拟合具有显著性.膝关节角度、运动负荷和性别对各指标的变化率有显著影响.结论:应用非线性分析指标评价肌肉疲劳具有很好的敏感性.【期刊名称】《武汉体育学院学报》【年(卷),期】2011(045)012【总页数】7页(P71-77)【关键词】表面肌电信号;非线性;肌肉疲劳;敏感性【作者】陈胜利;张立【作者单位】郑州大学体育学院,河南郑州450044;武汉体育学院健康科学学院,湖北武汉430079【正文语种】中文【中图分类】G804.2肌肉持续收缩导致的肌肉疲劳发生和发展过程中表现的sEMG信号特有变化活动规律就其机制而言,取决于中枢神经系统控制与外周肌肉活动代谢的共同作用,并且与肌肉收缩做功能力密切相关。
因此,研究运动性肌肉疲劳过程中sEMG信号变化特征,对于了解肌肉疲劳发生机制和客观评价肌肉疲劳程度,对运动能力的影响具有重要理论和应用价值。
以往大量研究表明无论是上下肢还是躯干肌肉,静力性或是动力性运动所导致肌肉疲劳,sEMG信号活动均表现出相对一致的变化规律,即频谱曲线左移(频域分析指标随时间序列持续减小);而时域分析指标目前还没有统一认可的变化规律,大部分研究结果认为在次最大随意收缩的实验中表现为先增加后减少,而在最大随意收缩的实验中表现为持续下降[1,2]。
肌电信号来源于大脑皮层运动区及皮层下不同部位,中枢运动性神经元兴奋冲动时的依时性序列信号,是测试电极部位许多运动单元发放的动作电位的总和。
一种新型EMS智能腰围在腰腹部肌肉损伤中的康复应用

一种新型EMS智能腰围在腰腹部肌肉损伤中的康复应用作者:杨琪刘勇前曲方园李涛李卫来源:《医学食疗与健康》2021年第07期【摘要】目的:探讨一种新型EMS智能腰围在腰腹部肌肉损伤中的康复应用。
方法:选择2020年2月至2020年7月治疗的126例腰腹部肌肉损伤患者,随机均分为对照组与试验组各63例,对照组患者给予常规热红外照射加普通腰围治疗,试验组患者应用一种新型EMS智能腰围治疗,两组患者均连续治疗21 d。
结果:两组治疗7 d、21 d后视觉模拟评分、腰椎Oswestry功能障碍指数评分均显著低于治疗前,日本骨科协会评估治疗评分显著高于治疗前(P【关键词】腰腹部;肌肉损伤;EMS智能腰围;康复[中图分类号]R49;R658 [文献标识码]A [文章编号]2096-5249(2021)07-0223-02腰腹部肌肉损伤由于长期保持固定姿势、运动和分娩等引起,是一个影响终生的重要健康问题,腰腹肌损伤在运动员中很常见[1]。
近一半的球类运动员在过去的12个月内发生过腰背痛,普通民众的发生率为44.1%,女性患病率更高[2]。
腰腹部肌肉损伤诱发多种问题,包括生理、心理和生活。
对疼痛的消极信念和/或对疾病的负面信息可能会使患者想象最坏的结果是害怕和避免运动。
文献表明减少运动恐惧的干预措施有很多,但效果不一。
EMS是Electronic Muscle Simulator的缩写,是通过仿肌肉生物电信号引发肌肉收缩运动的设备,已经在治疗腰腹部肌肉损伤中显现非常好的效果。
本研究选择2020年2月至2020年7月在哈尔滨市第一医院诊治的126例腰腹部肌肉损伤患者,通过对照试验探讨新型EMS 智能腰围在腰腹部肌肉损伤中的康复应用,报道如下。
1 资料与方法1.1一般资料选择2020年2月至2020年7月于本院治疗的126例腰腹部肌肉损伤患者,随机均分为观察组与试验组各63例,对照组患者给予常规热红外照射加普通腰围治疗,试验组患者给予一种新型EMS 智能腰围治疗(日本专利号2017-005042),观察组中,男45例、女18例,腰部肌肉损伤43例,腹部肌肉损伤20例,年龄(25.14±3.08)岁,体重指数(21.24±1.32)kg/m2;对照组中,男39例、女24例,腰部肌肉损伤36例,腹部肌肉损伤27例,年龄(26.21±4.28)岁,体重指数(23.13±1.48)kg/m2。
基于表面肌电信号的上肢康复机器人变阻抗控制技术研究

基于表面肌电信号的上肢康复机器人变阻抗控制技术研究吴彤;李健;李国栋;刘路;朱立国;冯敏山
【期刊名称】《电气传动》
【年(卷),期】2024(54)1
【摘要】我国老龄人群肢体障碍者很多,运动康复技术是康复治疗的有效手段。
但医患比例严重失调难以使得人人都能享受到康复医疗服务。
康复机器人通过阻抗控制技术能够与人体进行一定的安全交互,但现有康复机器人多采用定阻抗控制,环境适应能力弱,难以应用于临床。
针对上述问题,提出一种变阻抗控制方法,以采集使用者的表面肌电信号为输入条件,通过改进随机森林算法训练识别使用者上肢的运动角度。
并配合末端力传感器,获得使用者的运动意图,再通过变阻抗控制器完成康复运动。
搭建了实验平台,并对轨迹跟踪和康复训练能力进行验证与评估。
结果表明所设计的控制器能够控制机器人完成康复运动。
【总页数】9页(P83-91)
【作者】吴彤;李健;李国栋;刘路;朱立国;冯敏山
【作者单位】北京理工大学自动化学院;中国软件测评中心;中国中医科学院望京医院中医正骨技术北京市重点实验室
【正文语种】中文
【中图分类】TP13
【相关文献】
1.基于力阻抗模型的上肢康复机器人交互控制系统设计
2.基于遗传算法和模糊神经网络的康复机器人上肢阻抗控制
3.基于变阻抗补偿的上肢康复机器人人机交互方法研究
因版权原因,仅展示原文概要,查看原文内容请购买。
- 1、下载文档前请自行甄别文档内容的完整性,平台不提供额外的编辑、内容补充、找答案等附加服务。
- 2、"仅部分预览"的文档,不可在线预览部分如存在完整性等问题,可反馈申请退款(可完整预览的文档不适用该条件!)。
- 3、如文档侵犯您的权益,请联系客服反馈,我们会尽快为您处理(人工客服工作时间:9:00-18:30)。
Abstract—It may be difficult task for physically weak elderly, disabled and injured individuals to perform the day to day activities in their life. Therefore, many assistive devices have been developed in order to improve the quality of life of those people. Especially upper-limb power-assist exoskeletons have been developed since the upper limb motions are vital for the daily activities. Electromyography (EMG) signals of the upper limb muscles have sometimes been used as a primary signal to control the power assist exoskeletons since the EMG signals directly reflect the motion intention of the user. But one of the main obstacles for EMG based controller is the muscle fatigue, because the muscle fatigue might change the EMG patterns. Itis important for power-assist exoskeleton to correctly assist the user for longer period of time. But it has high probability of user muscles been fatigued because users getting more and more exhausted at the end of the day. Therefore it is necessaryto consider the variations of EMG signals due to the effect of muscle fatigue. In this paper it demonstrates the study which was conducted to find out the effects of muscle fatigue on the three EMG features derived from the raw EMG signals of the Bicep brachii, Deltoid-posterior, Deltoid-anterior and Supinator muscles of the upper limb. Shoulder vertical flexion/extension, shoulder abduction/adduction, elbow flexion/extension and forearm pronation/supination motions were carried out before and after a set of muscle fatiguing exercises. The three features computed in this experiment were RMS (Root Mean Square), MPF (Mean Power Frequency) anda spectral feature (FInsm5) which was proposed by Dimitrov. Comparison results of these three features of all muscles before and after the fatiguing exercises showed an percentage increaseof the RMS and F Insm5 features whereas MPF showed a percentage decrease with respect to the before fatiguing conditions. The result showed that the EMG RMS may not a reliable feature to use as the only input signal in EMG based control for human upper-limb power assist in the muscle fatiguing conditions. Therefore, it is suggested that a modification method for compensating the effect of muscle fatigue is required on the EMG based control in order to have a long and reliable use of the human upper-limb power assist exoskeletons.I.I NTRODUCTIONHE upper-limb motions are important for the human daily activities. However, it is sometimes difficult for physically weak elderly, disabled, and injured individuals to perform daily upper-limb activities. Due to the modern science and advancement of the technology, many developed countries have to face the problem of aging societies. Therefore in such societies it is important to improve the quality of living of such persons to care of themselves in the present society. Recently, some assistive devices such as power assists robots have been developed to assist the daily life motions as one of the solutions to these problems [1]-[5].These power-assist robots/exoskeletons are required to generate the movements according to the user’s motion intention. Some of these power-assist robotic systems use a biological signal such as EMG signals of muscles of the users which have been identified as an input signals to estimate the user’s motion intention in real time. Hence, it is important to analyze the relationships between the upper-limb motions and related muscle activities to perform the power-assist of the upper-limb motion. In [6], an electromyography study has been carried out for upper-limb adduction force with varying shoulder and elbow postures. In [7], a study has been conducted to research about the muscle activity and coordination in the normal shoulder. In the recent study [8], the results of human upper–limb muscle activities during daily upper limb motions were elaborated. However, it is important to consider about the time varying characteristics of the EMG signal which is known to be a non-linear signal as well as non stationary signal with respect to time. One ofA Study on Effects of Muscle Fatigue onEMG-Based Control for Human Upper-LimbPower-AssistThilina Dulantha Lalitharatne1, Yoshikai Hayashi2, Kenbu Teramoto3, Kazuo Kiguchi41,2,3 Department of Advanced Technology Fusion,Saga University, Saga, JapanEmail: 1thilina@mech.mrt.ac.lk,2hayashi@me.saga-u.ac.jp,3tera@me.saga-u.ac.jp4 Department of Mechanical Engineering,Kyushu University, Fukuoka, JapanEmail: 4kiguchi@mech.kyushu-u.ac.jpT978-1-4673-1975-1/12/$31.00 ©2012 IEEE ICIAfS’12the main reasons behind the EMG non-stationary is themuscle fatigue. It has a high probability of getting muscles fatigued of the physically weak elderly people or disable individuals as they are more and more exhausted during the day to day life activities throughout the day. However, it is important for power-assist exoskeleton to behave in a reliable and accurate manner as long as it is used. But the alterations to EMG signals due to muscle fatiguing conditions may cause controller of such power-assist exoskeleton to generate an incorrect output. In [9], myoelectric measurements have been carried out for shoulder muscle fatigue during the intermittent dynamic exertions. In the study [10], the results have shown the variation of EMG signals of bicep brachii muscle during the fatiguing dynamic contractions. In this paper, it demonstrates the study on effect of muscle fatigue on EMG based control in human upper-limb power assist. Three EMG features are calculated from the raw EMG signals of four muscles of human upper-limb namely bicep brachii, Deltoid-anterior, Deltoid-posterior and Supinator which are often use in EMG based control of human upper-limb power assist exoskeletons. Three features interested in this study are EMG RMS (Root Mean Square), MPF (Mean Power Frequency) and a spectral feature (FInsm5) explained in [11]. Four basic motions of upper-limb namely Shoulder abduction/adduction, Shoulder vertical flexion/extension, Elbow flexion/extension and Forearm pronation/supination are carried in the beginning of the experiments. In order to get the muscle fatigue conditions a set of muscle fatiguing exercises are conducted. Immediately after the fatiguing exercises, the same motions which conducted before the fatiguing exercises are carried out to measure the effect of muscle fatigue on each of the EMG features in each different motions of the upper-limb.II.M ETHODA.Data AcquisitionIn order to measure the EMG changes due to fatigue, EMG signals of muscles of the upper limb were measured with EMG electrodes with bipolar montage [NE-101A, Nihon Koden Co.] through an amplifier [MEG-6108, Nihon Koden Co.]. The EMG signals of the muscles bicep brachii, deltoid-anterior, deltoid-posterior and supinator of the right upper-limb were measured. The locations of the electrodes can be found in [8].Two 3-axis accelerometers attached on the shoulder and the forearm using adhesive tapes were used to measure the shoulder angles and forearm angle respectively. A rotary encoder attached to elbow using two links was used to measure the elbow angle (Fig. 1).B.Experimental ProcedureThree healthy men (age: 24-27), who were not suffering from any previous muscle disorders of upper limb participated in this study. Each subject was given the full instructions about the experimental procedure before the experiments. The subjects were asked to follow four different upper limb motions (shoulder abduction/adduction, shoulder vertical flexion/extension, elbow flexion/extension and forearm pronation/supination). The initial positions and the motion ranges for shoulder vertical movement and shoulder abduction/adduction movement are shown in Fig. 2(a) and Fig. 2(b) respectively. The elbow flexion/extension and the forearm supination/pronation movements are shown in the Fig. 3(a) and Fig. 3(b) respectively. The experimental procedure of the elbow flexion/extension movement is described here, and other experiments were carried out in the same routine. A t the first phase of the elbow motion experiment, subjects were asked to do three repetitions of(a) (b)Fig. 2 Motion ranges of shoulder movements(a) (b)Fig. 3 Motion ranges of elbow and forearm movementsFig. 1 Placement of Sensorscondition. FInsm5 feature showed a significant increase in bicep brachii muscle of subject B. Fig. 7(e) and 7(f) show the variations of FInsm5 features in before and after fatiguing exercise respectively. Similar kinds of changes were observed for other muscles in each subject. In order to compare the percentage variation of EMG features of bicep brachii muscle followed by the muscles fatiguing exercises to initial EMG features, averages across the each contraction cycle were calculated. Since, there were set of three contraction cycles carried out before and after muscle fatiguing exercise, two grand averages were calculated respectively for before and after conditions. Finally ratio between differences of grand averages to initial average was calculated as the percentage variation for each feature in each muscle. Similar procedure was implemented for data of every subject. The final results are shown in Table I, Table II and Table III for subject A, subject B and subject C respectively. It can be considered as these changes on each EMG features due to muscles fatiguing conditions, since all other conditions prior and posterior the fatiguing experiment were approximately maintained at the same levels. Results show that EMG RMS feature is affected by the muscle fatigue. Therefore these results emphasize the need of attention to be focus on muscle fatigue conditions whenever using EMG RMS signals in controlling exoskeletons. For an example, in Fig. 6(a) shows RMS feature changes with respect to elbow angle before fatiguing exercise conducted. A System which has trained for calculating the elbow torque using RMS changes with the elbow angle for before fatiguing exercise will not give accurate results due to different pattern between EMG RMS and the elbow angle after fatiguing exercise. Therefore, it may not accurate to use only the EMG RMS of muscles as the features to calculate the torques, since the subjects who are using the exoskeletons in day to day life can be suffered by muscle fatigue due to long term use and exhaustion. It is necessary to modify the torque calculation for each joint according to the muscle fatigue condition in order to achieve a long term use of the exoskeleton system. On the other hand, MPF and FInsm5 can be used as measures of the muscle fatigue conditions. These two features combine with EMG RMS can be used to accurately calculate the joint torque for long use of the exoskeleton. Since the variation of each EMG features in this study are shown to be non linear signals, a fuzzy reasoning method can be applied as a non linear algorithm to calculate the joint torque. Then a method such as artificial neural network can be used for teaching the system to work in the muscle fatigued conditions and adaption for each subject to use in practical systems.IV.C ONCLUSIONIn this paper, the objective was to study about the effects of muscle fatigue on EMG based control for human upper-limb power assist. The effect of muscle fatigue on the three EMG features namely RMS, MPF and FInsm5 were analyzed in this study. EMG RMS and FInsm5 features were increased with the fatiguing conditions whereas MPF showed a decreasing pattern. It is suggested that only use of EMG RMS as input signal to measure the motion intention in EMG based controller in exoskeleton may not accurate for long-term use, because of the muscle fatiguing conditions. Therefore, a combination of the other two features with EMG RMS can be used as inputs to EMG-based power-assist exoskeleton controllers to compensate the effect of muscle fatiguing condition. Implementation of a fuzzy-neuro modifier which uses the above mentioned EMG features as the inputs is currently underway to compensate the effect of muscle fatigue and ensure the long–term use of the 7-DOF upper limb power assist exoskeleton [3].R EFERENCES[1]S.Lee and Y.Sankai, “ Power A ssist Control for Walking A id withHA L-3 Based on EMG and Impedance A djustment around Knee Joint,” Proc. of IEEE/RSJ International Conf. on Intelligent Robots and Systems, 2002, pp.1499-1504.[2]J.L. Pons, et el., “Upper-Limb Robotic Rehabilitation Exoskeleton:Tremor Suppression,” Rehabilitation Robotics, Book edited by S.S Kommu, pp.453-470, 2007.[3]R.A.R.C. Gopura and K.Kiguchi, “ SUEFUL-7: A 7 DOF Upper-LimbExoskeleton Robot with Muscle-Model-Oriented EMG-Based Control”, Proc. of IEEE/RSJ International Conf. on Intelligent Robots and Systems, pp.1126-1131,2009.[4]K.Kiguchi and M.Liyanage, Y. Kose, Y. Hayashi and D.Jeon, “3DPerception-A ssist for an Upper-Limb Power-A ssist Exoskeleton”, Proc. of the 14th IASTED International Conf. on Robotics and Applications, pp.60-66, 2009.[5]Perry J.C., Rosen J.,” Upper-Limb Powered ExoskeletonDesign.”IEEE/ASME Trans. on Mechatronics, 12(4): 408-417, 2007 [6]Gil Coury H., Kumar S., Narayan Y. “An Electromyographic Study ofUpper Limb A dduction Force with Varying Shoulder and Elbow Postures”, Journal of Electromyography and Kinesiology 8: 157-168, 1998.[7]Kronberg M., Nemeth G., Brostrom L.A.,”Muscle A ctivity andCoordination in the Normal Shoulder”, Clinical Orthopedics and Related Research, 257: 76-85, 1990.[8]R.A.R.C. Gopura, Kazuo Kiguchi, Etsuo Horikawa,” A Study onHuman Upper-Limb Muscles A ctivities during Daily Upper-Limb Motions”, International Journal of Bioelectromagnetism vol. 12, No.2,pp.54-61,2010[9]Maury A. Nussbaum, “Static and dynamic myoelectric measures ofshoulder muscle fatigue during intermittent dynamic exertions of low to moderate intensity”, European Journal of Applied Physiology, vol 85,No 3-4, pp 299-309,2001.[10]J. R. Potvin,” Effects of muscle kinematics on surface EMG amplitudeand frequency during fatiguing dynamic contractions”, Journal of Applied Physiology, vol.82 , no. 1, pp.144-151, 1997.[11]Dimitrov G.V, Arabadzhiv T.I, Mileva K.N, Bowtell J.L, Crichton N.,Dimitrova N.A,” Muscle Fatigue During Dynamic Contractions Assessed by New Spectral Indices”, Medicine & Science in Sports andExercise: vol. 38, no 38, pp.1971-1979, 2006。