多因子定价模型检验,波动和投资组合Tests of Multi-Factor Pricing Models, Volatility, and Portfolio Pe
投资组合管理中的多因子模型解析与应用
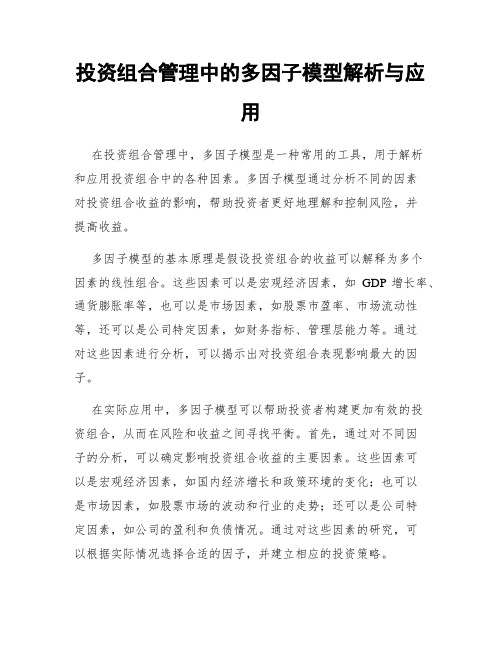
投资组合管理中的多因子模型解析与应用在投资组合管理中,多因子模型是一种常用的工具,用于解析和应用投资组合中的各种因素。
多因子模型通过分析不同的因素对投资组合收益的影响,帮助投资者更好地理解和控制风险,并提高收益。
多因子模型的基本原理是假设投资组合的收益可以解释为多个因素的线性组合。
这些因素可以是宏观经济因素,如GDP增长率、通货膨胀率等,也可以是市场因素,如股票市盈率、市场流动性等,还可以是公司特定因素,如财务指标、管理层能力等。
通过对这些因素进行分析,可以揭示出对投资组合表现影响最大的因子。
在实际应用中,多因子模型可以帮助投资者构建更加有效的投资组合,从而在风险和收益之间寻找平衡。
首先,通过对不同因子的分析,可以确定影响投资组合收益的主要因素。
这些因素可以是宏观经济因素,如国内经济增长和政策环境的变化;也可以是市场因素,如股票市场的波动和行业的走势;还可以是公司特定因素,如公司的盈利和负债情况。
通过对这些因素的研究,可以根据实际情况选择合适的因子,并建立相应的投资策略。
其次,多因子模型可以帮助投资者评估不同因素之间的相关性和重要性。
通过多因子模型的分析,可以确定哪些因素对投资组合表现的影响最大,从而优化投资策略。
例如,在股票市场中,市盈率和市净率是两个常用的因子。
如果市盈率对投资组合的收益影响较大,那么投资者可以选择更多的低市盈率股票,以获得更高的收益。
相反,如果市盈率对投资组合的影响较小,那么投资者可以重点关注其他因子,如市净率或企业盈利能力。
除了研究各个因素的影响力外,多因子模型还可以帮助投资者评估整体投资组合的风险和收益特征。
通过对不同因素的组合,可以模拟出各种不同的投资组合,并对其进行风险和收益分析。
这样一来,投资者可以根据自己的风险承受能力和收益预期,选择最适合自己的投资组合。
值得注意的是,多因子模型并不是一种完美的工具。
它建立在各个因素对投资组合收益的线性关系上,而实际市场往往存在非线性的因素和不确定性。
投资学中的多因子模型如何综合考虑多种因素进行投资决策

投资学中的多因子模型如何综合考虑多种因素进行投资决策投资是一门精密而复杂的艺术,需要投资者综合考虑多种因素来作出理智而明智的决策。
多因子模型是一种投资分析方法,旨在通过综合考虑多个影响投资回报的因子来优化投资组合的构建。
本文将探讨多因子模型在投资决策中的应用,并分析其优势和局限性。
一、多因子模型的基本原理多因子模型是基于资本资产定价模型(CAPM)的发展而来。
CAPM是通过市场因子来解释资产回报率的模型,但随着研究的深入,人们逐渐认识到市场因子并不能完全解释资产回报的波动性。
因此,基于CAPM的基础上发展出了多因子模型。
多因子模型通过引入更多的因子来解释资产回报的波动性。
这些因子可以是市场因子、行业因子、财务因子、宏观经济因子等等。
通过综合考虑多个影响因素,多因子模型能够更准确地预测资产的回报率。
二、多因子模型在投资决策中的应用多因子模型在投资决策中的应用主要通过以下几个步骤实现:1. 因子选择:在构建多因子模型之前,投资者首先需要选择适当的因子。
因子的选择需要基于理论和经验,并且需要考虑投资者的投资目标和风险承受能力。
2. 因子权重设定:不同因子对资产回报的影响可能是不同的。
投资者需要根据因子的重要性设定合适的权重。
这需要基于数据分析和统计方法来进行。
3. 模型构建:通过将选择的因子和相应的权重结合起来,投资者可以构建多因子模型。
这个模型可以用来估计不同资产的预期回报率。
4. 投资组合优化:利用多因子模型的估计结果,投资者可以通过优化方法来构建最优的投资组合。
这种方法可以帮助投资者在给定的风险水平下,实现最大的收益。
5. 跟踪与调整:一旦建立了投资组合,投资者需要不断跟踪资产的表现,并根据市场状况进行必要的调整。
这可以通过定期的投资组合再平衡来实现。
三、多因子模型的优势和局限性多因子模型相比于传统的单因子模型具有以下几个优势:1. 更准确的预测能力:多因子模型通过综合考虑多个因素,可以更准确地预测资产回报的波动性和预期收益率。
多因子资产定价模型
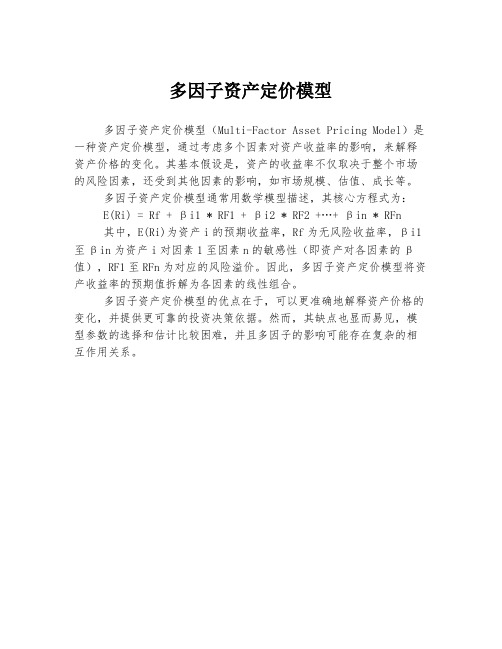
多因子资产定价模型
多因子资产定价模型(Multi-Factor Asset Pricing Model)是一种资产定价模型,通过考虑多个因素对资产收益率的影响,来解释资产价格的变化。
其基本假设是,资产的收益率不仅取决于整个市场的风险因素,还受到其他因素的影响,如市场规模、估值、成长等。
多因子资产定价模型通常用数学模型描述,其核心方程式为:
E(Ri) = Rf + βi1 * RF1 + βi2 * RF2 +…+ βin * RFn
其中,E(Ri)为资产i的预期收益率,Rf为无风险收益率,βi1至βin为资产i对因素1至因素n的敏感性(即资产对各因素的β值),RF1至RFn为对应的风险溢价。
因此,多因子资产定价模型将资产收益率的预期值拆解为各因素的线性组合。
多因子资产定价模型的优点在于,可以更准确地解释资产价格的变化,并提供更可靠的投资决策依据。
然而,其缺点也显而易见,模型参数的选择和估计比较困难,并且多因子的影响可能存在复杂的相互作用关系。
金融风险定价模型的多因子分析与优化研究

金融风险定价模型的多因子分析与优化研究一、引言金融市场的不确定性和风险性使得风险定价模型在金融领域中扮演着重要的角色。
随着时间的推移,传统的单因子模型已经不足以满足风险定价的需求。
因此,多因子分析和优化成为了研究的热点。
本文旨在探讨金融风险定价模型的多因子分析与优化研究。
二、多因子模型的概念和应用1. 多因子模型的概念多因子模型是指将金融资产的收益率分解为多个因素的线性组合,以揭示背后的经济和市场趋势。
通常,这些因子包括市场因子、公司特定因子和宏观经济因子等。
2. 多因子模型的应用多因子模型的应用广泛,包括股票选取、投资组合管理和风险分析等。
通过使用多因子模型,投资者可以更准确地评估投资组合的风险和收益,优化资产配置,并进行有效的风险管理。
三、金融风险定价模型的多因子分析1. 市场因子分析市场因子是多因子模型中最重要的因素之一,通常是指市场指数的表现。
通过分析市场因子,可以帮助我们预测市场的整体风险和收益。
一些常用的市场因子包括市场收益率、市场波动率以及市场流动性等。
2. 公司特定因子分析公司特定因子是指影响个别公司或行业的因素。
这些因素包括公司的盈利情况、行业竞争力、财务状况和管理层能力等。
通过分析公司特定因素,可以帮助我们理解某个公司或行业的风险和收益特征。
3. 宏观经济因子分析宏观经济因子是指宏观经济环境的变化对金融资产收益率的影响。
例如,利率、通货膨胀率、国内生产总值(GDP)增长率等。
通过分析宏观经济因素,可以更好地理解金融市场的风险和收益。
四、金融风险定价模型的多因子优化1. 基于协方差矩阵的优化在多因子模型中,协方差矩阵是优化构建投资组合的关键。
通过分析各个因子之间的协方差,可以更好地实现资产的多样化和风险的分散。
在优化投资组合时,可以通过最小化投资组合的方差或最大化投资组合的效用函数来优化投资组合的风险和收益。
2. 约束优化约束优化是在投资组合构建中常用的一种方法,在优化过程中引入约束条件来满足特定的投资目标。
因子模型与投资组合分析在金融资产管理中的应用与评估
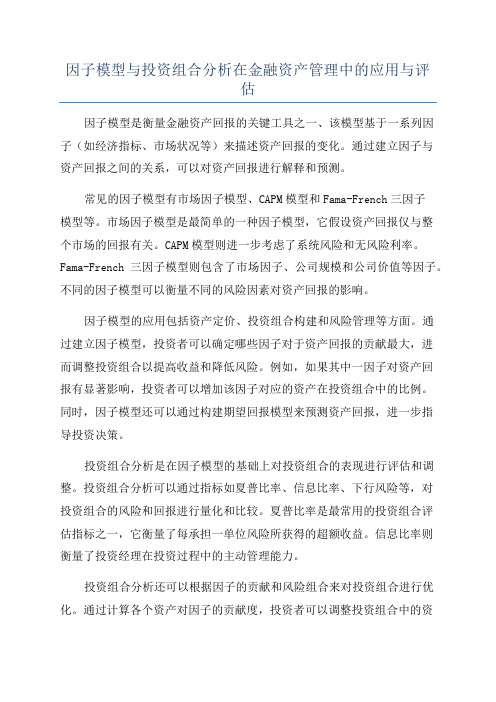
因子模型与投资组合分析在金融资产管理中的应用与评估因子模型是衡量金融资产回报的关键工具之一、该模型基于一系列因子(如经济指标、市场状况等)来描述资产回报的变化。
通过建立因子与资产回报之间的关系,可以对资产回报进行解释和预测。
常见的因子模型有市场因子模型、CAPM模型和Fama-French三因子模型等。
市场因子模型是最简单的一种因子模型,它假设资产回报仅与整个市场的回报有关。
CAPM模型则进一步考虑了系统风险和无风险利率。
Fama-French三因子模型则包含了市场因子、公司规模和公司价值等因子。
不同的因子模型可以衡量不同的风险因素对资产回报的影响。
因子模型的应用包括资产定价、投资组合构建和风险管理等方面。
通过建立因子模型,投资者可以确定哪些因子对于资产回报的贡献最大,进而调整投资组合以提高收益和降低风险。
例如,如果其中一因子对资产回报有显著影响,投资者可以增加该因子对应的资产在投资组合中的比例。
同时,因子模型还可以通过构建期望回报模型来预测资产回报,进一步指导投资决策。
投资组合分析是在因子模型的基础上对投资组合的表现进行评估和调整。
投资组合分析可以通过指标如夏普比率、信息比率、下行风险等,对投资组合的风险和回报进行量化和比较。
夏普比率是最常用的投资组合评估指标之一,它衡量了每承担一单位风险所获得的超额收益。
信息比率则衡量了投资经理在投资过程中的主动管理能力。
投资组合分析还可以根据因子的贡献和风险组合来对投资组合进行优化。
通过计算各个资产对因子的贡献度,投资者可以调整投资组合中的资产比例,以达到最优配置。
同时,通过分析不同因子对投资组合的风险贡献,投资者可以对投资组合的风险进行管理和控制。
多因子模型在投资组合中的应用
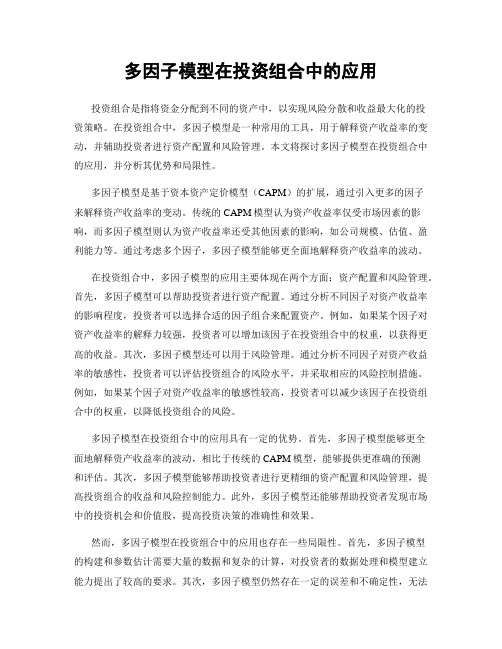
多因子模型在投资组合中的应用投资组合是指将资金分配到不同的资产中,以实现风险分散和收益最大化的投资策略。
在投资组合中,多因子模型是一种常用的工具,用于解释资产收益率的变动,并辅助投资者进行资产配置和风险管理。
本文将探讨多因子模型在投资组合中的应用,并分析其优势和局限性。
多因子模型是基于资本资产定价模型(CAPM)的扩展,通过引入更多的因子来解释资产收益率的变动。
传统的CAPM模型认为资产收益率仅受市场因素的影响,而多因子模型则认为资产收益率还受其他因素的影响,如公司规模、估值、盈利能力等。
通过考虑多个因子,多因子模型能够更全面地解释资产收益率的波动。
在投资组合中,多因子模型的应用主要体现在两个方面:资产配置和风险管理。
首先,多因子模型可以帮助投资者进行资产配置。
通过分析不同因子对资产收益率的影响程度,投资者可以选择合适的因子组合来配置资产。
例如,如果某个因子对资产收益率的解释力较强,投资者可以增加该因子在投资组合中的权重,以获得更高的收益。
其次,多因子模型还可以用于风险管理。
通过分析不同因子对资产收益率的敏感性,投资者可以评估投资组合的风险水平,并采取相应的风险控制措施。
例如,如果某个因子对资产收益率的敏感性较高,投资者可以减少该因子在投资组合中的权重,以降低投资组合的风险。
多因子模型在投资组合中的应用具有一定的优势。
首先,多因子模型能够更全面地解释资产收益率的波动,相比于传统的CAPM模型,能够提供更准确的预测和评估。
其次,多因子模型能够帮助投资者进行更精细的资产配置和风险管理,提高投资组合的收益和风险控制能力。
此外,多因子模型还能够帮助投资者发现市场中的投资机会和价值股,提高投资决策的准确性和效果。
然而,多因子模型在投资组合中的应用也存在一些局限性。
首先,多因子模型的构建和参数估计需要大量的数据和复杂的计算,对投资者的数据处理和模型建立能力提出了较高的要求。
其次,多因子模型仍然存在一定的误差和不确定性,无法完全准确地预测和解释资产收益率的变动。
多因子模型资产定价应用评述
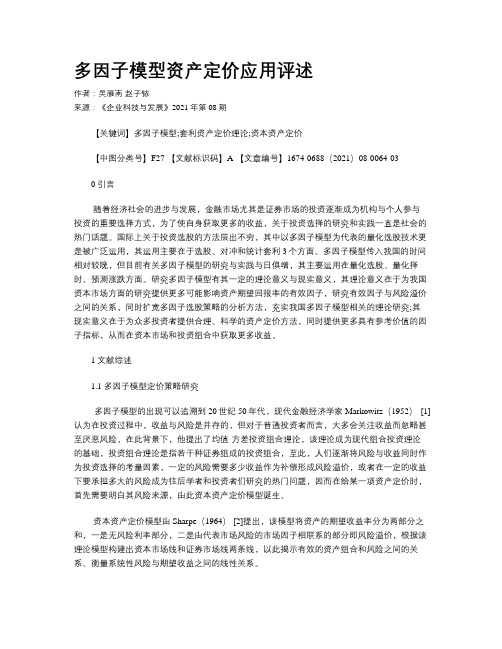
多因子模型资产定价应用评述作者:吴雁南赵子铱来源:《企业科技与发展》2021年第08期【关键词】多因子模型;套利资产定价理论;资本资产定价【中图分类号】F27 【文献标识码】A 【文章编号】1674-0688(2021)08-0064-030 引言随着经济社会的进步与发展,金融市场尤其是证券市场的投资逐渐成为机构与个人参与投资的重要选择方式,为了使自身获取更多的收益,关于投资选择的研究和实践一直是社会的热门话题。
国际上关于投资选股的方法层出不穷,其中以多因子模型为代表的量化选股技术更是被广泛运用,其运用主要在于选股、对冲和统计套利3个方面。
多因子模型传入我国的时间相对较晚,但目前有关多因子模型的研究与实践与日俱增,其主要运用在量化选股、量化择时、预测涨跌方面。
研究多因子模型有其一定的理论意义与现实意义,其理论意义在于为我国资本市场方面的研究提供更多可能影响资产期望回报率的有效因子,研究有效因子与风险溢价之间的关系,同时扩宽多因子选股策略的分析方法,充实我国多因子模型相关的理论研究;其现实意义在于为众多投资者提供合理、科学的资产定价方法,同时提供更多具有参考价值的因子指标,从而在资本市场和投资组合中获取更多收益。
1 文献综述1.1 多因子模型定价策略研究多因子模型的出现可以追溯到20世纪50年代,现代金融经济学家Markowitz(1952)[1]认为在投资过程中,收益与风险是并存的,但对于普通投资者而言,大多会关注收益而忽略甚至厌恶风险,在此背景下,他提出了均值-方差投资组合理论,该理论成为现代组合投资理论的基础,投资组合理论是指若干种证券组成的投资组合,至此,人们逐渐将风险与收益同时作为投资选择的考量因素,一定的风险需要多少收益作为补偿形成风险溢价,或者在一定的收益下要承担多大的风险成为往后学者和投资者们研究的热门问题,因而在给某一项资产定价时,首先需要明白其风险来源,由此资本资产定价模型诞生。
业绩归因报告
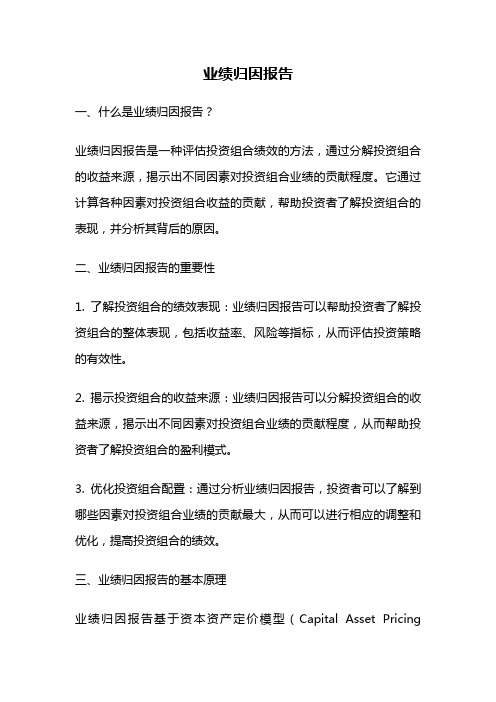
业绩归因报告一、什么是业绩归因报告?业绩归因报告是一种评估投资组合绩效的方法,通过分解投资组合的收益来源,揭示出不同因素对投资组合业绩的贡献程度。
它通过计算各种因素对投资组合收益的贡献,帮助投资者了解投资组合的表现,并分析其背后的原因。
二、业绩归因报告的重要性1. 了解投资组合的绩效表现:业绩归因报告可以帮助投资者了解投资组合的整体表现,包括收益率、风险等指标,从而评估投资策略的有效性。
2. 揭示投资组合的收益来源:业绩归因报告可以分解投资组合的收益来源,揭示出不同因素对投资组合业绩的贡献程度,从而帮助投资者了解投资组合的盈利模式。
3. 优化投资组合配置:通过分析业绩归因报告,投资者可以了解到哪些因素对投资组合业绩的贡献最大,从而可以进行相应的调整和优化,提高投资组合的绩效。
三、业绩归因报告的基本原理业绩归因报告基于资本资产定价模型(Capital Asset PricingModel,简称CAPM)和多因子模型(Multi-factor Model),通过对投资组合进行分解,计算各种因素对投资组合收益的贡献。
1. 单因子模型:单因子模型假设投资组合的收益与某一特定因子相关,如市场因子或行业因子。
通过计算该因子对投资组合收益的贡献,可以评估该因子对投资组合业绩的影响。
2. 多因子模型:多因子模型考虑了多个因素对投资组合收益的影响,如市场因子、行业因子、风格因子等。
通过计算各个因子对投资组合收益的贡献,可以更全面地评估投资组合的绩效。
四、业绩归因报告的应用1. 评估投资策略的有效性:通过分析业绩归因报告,投资者可以评估投资策略的有效性,了解哪些因素对投资组合业绩的贡献最大,从而调整投资策略,提高绩效。
2. 比较不同投资组合的表现:业绩归因报告可以帮助投资者比较不同投资组合的表现,了解其收益来源和风险特征,从而做出更明智的投资决策。
3. 优化投资组合配置:通过分析业绩归因报告,投资者可以了解哪些因素对投资组合业绩的贡献最大,从而进行相应的调整和优化,提高投资组合的绩效。
- 1、下载文档前请自行甄别文档内容的完整性,平台不提供额外的编辑、内容补充、找答案等附加服务。
- 2、"仅部分预览"的文档,不可在线预览部分如存在完整性等问题,可反馈申请退款(可完整预览的文档不适用该条件!)。
- 3、如文档侵犯您的权益,请联系客服反馈,我们会尽快为您处理(人工客服工作时间:9:00-18:30)。
NBER WORKING PAPER SERIESTESTS OF MUTLIFACTOR PRICIN G MODELS,VOLATILITY BOUNDS ANDPORTFOLIO PERFORMANCEWayne E. FersonWorking Paper9441/papers/w9441NATIONAL BUREAU OF ECONOMIC RESEARCH1050 Massachusetts AvenueCambridge, MA 02138January 2003The author acknowledges financial support from the Collins Chair in Finance at Boston College and the Pigott-PACCAR professorship at the University of Washington. He is also grateful to George Constantinides and Ludan Liu for helpful comments and suggestions. The views expressed herein are those of the authors and not necessarily those of the National Bureau of Economic Research.©2003 by Wayne E. Ferson. All rights reserved. Short sections of text not to exceed two paragraphs, may be quoted without explicit permission provided that full credit including . notice, is given to the source.Tests of Multifactor Pricing Models, Volatility Bounds and Portfolio PerformanceWayne E. FersonNBER Working Paper No. 9441January 2003JEL No. G000, G110, G120, G140ABSTRACTThree concepts: stochastic discount factors, multi-beta pricing and mean variance efficiency, are at the core of modern empirical asset pricing. This paper reviews these paradigms and the relations among them, concentrating on conditional asset pricing models where lagged variables serve as instruments for publicly available information. The different paradigms are associated with different empirical methods. We review the variance bounds of Hansen and Jagannathan (1991), concentrating on extensions for conditioning information. Hansen's (1982) Generalized Method of Moments (GMM) is briefly reviewed as an organizing principle. Then, cross-sectional regression approaches as developed by Fama and MacBeth (1973) are reviewed and used to interpret empirical factors, such as those advocated by Fama and French (1993, 1996). Finally, we review the multivariate regression approach, popularized in the finance literature by Gibbons (1982) and others. A regression approach, with a beta pricing formulation, and a GMM approach with a stochastic discount factor formulation, may be considered competing paradigms for empirical work in asset pricing. This discussion clarifies the relations between the various approaches. Finally, we bring the models and methods together, with a review of the recent conditional performance evaluation literature, concentrating on mutual funds and pension funds.Wayne E. FersonBoston CollegeCarroll School of Management140 Commonwealth Avenue, 330BChestnut Hill, MA 02467and NBERwayne.ferson@CONTENTSI. Introduction2. Multi-factor Asset Pricing Models: Review and Integration2.1 The Stochastic Discount Factor RepresentationExpected Risk PremiumsReturn Predictability2.2 Consumption-based Asset Pricing Models2.3 Multi-beta pricing ModelsRelation to the Stochastic Discount FactorRelation to Mean variance efficiencyA "Large Markets" Interpretation2.4 Mean variance efficiency with conditioning informationConditional versus Unconditional EfficiencyImplications for Tests2.5 Choosing the factors3. Modern Variance Bounds3.1 The Hansen Jagannathan Bounds3.2 Variance bounds with conditioning informationEfficient-portfolio boundsOptimal boundsDiscussion3.3 The Hansen Jagannathan Distance4. Methodology and Tests of Multifactor Asset Pricing Models4.1 The Generalized Method of Moments Approach4.2 Cross-sectional Regression MethodsThe Fama-MacBeth approachInterpreting the estimatesA CaveatErrors in Betas4.3 Multivariate Regression and beta pricing modelsComparing the Beta Pricing and stochastic discount factor approaches35. Conditional Performance Evaluation5.1 Stochastic Discount Factor formulationInvariance to the number of funds5.2 Beta pricing formulation5.3 Using portfolio weightsConditional Performance AttributionInterim Trading Bias5.4 Conditional market timing models5.5 Empirical Evidence on Conditional Performance6. ConclusionsI. IntroductionThe asset pricing models of modern finance describe the prices or expected rates of return of financial assets, which are claims traded in financial markets. Examples of financial assets are common stocks, bonds, options, futures and other "derivatives," so named because they derive their values from other, underlying assets. Asset pricing models are based on two central concepts. The first is the no arbitrage principle, which states that market forces tend to align prices so as to eliminate arbitrage opportunities. An arbitrage opportunity arises when assets can be combined in a portfolio with zero cost, no chance of a loss and positive probability of a gain. The second central concept in asset pricing is financial market equilibrium. Investors' desired holdings of financial assets derive from an optimization problem. In equilibrium the first order conditions of the optimization problem must be satisfied, and asset pricing models follow from these conditions. When the agent considers the consequences of the investment decision for more than a single period in the future, intertemporal asset pricing models result.The present paper reviews multi-factor asset pricing models from an empiricists' perspective. Multi-factor models can be motivated by either the no arbitrage principle or by an equilibrium model. Their distinguishing feature is that expected asset returns are determined by a linear combination of their covariances with variables representing the risk factors. This paper has two main objectives. The first is to integrate the various empirical models and their tests in a self contained discussion. The second is to review the application to the problem of measuring investment performance.This paper concentrates heavily on the role of conditioning information, in the form of lagged variables that serve as instruments for publicly available information. I think that developments in this area, conditional asset pricing, represent some of the most significant advances in empirical asset pricing research in recent years.The models described in this paper are set in the classical world of perfectly efficient financialmarkets, and perfectly rational economic agents. Of course, a great deal of research is devoted to understanding asset prices under market imperfections like information and transactions costs. The perfect markets models reviewed here represent a baseline, and a starting point for understanding these more complex issues.Work in empirical asset pricing over the last few years has provided a markedly improved understanding of the relations among the various asset-pricing models. Bits and pieces of this are scattered across a number of published papers, and some is "common" knowledge, shared by aficionados. This paper provides an integrative discussion, refining the earlier review in Ferson (1995) to reflect what I hope is an improved understanding.Much of our understanding of how asset pricing models' empirical predictions are related flows from representing the models as stochastic discount factors. Section 2 presents the stochastic discount factor approach, briefly illustrates a few examples of stochastic discount factors, and then relates the representation to beta pricing and to mean variance efficiency. These three concepts: stochastic discount factors, beta pricing and mean variance efficiency, are at the core of modern empirical asset pricing. We show the relation among these three concepts, and a "large-markets" interpretation of these relations. The discussion then proceeds to refinements of these issues in the presence of conditioning information. Section 2 ends with a brief discussion of how the risk factors have been identified in the empirical literature, and what the empirical evidence has to say about the selection of factors.Section 3 begins with a fundamental empirical application of the stochastic discount factor approach - the variance bounds originally developed by Hansen and Jagannathan (1991). Unlike the case where a model identifies a particular stochastic discount factor, the question in the Hansen-Jagannathan bounds is: Given a set of asset returns, and some conditioning information, what can besaid about the set of stochastic discount factors that could properly "price" the assets? By now, a number of reviews of the original Hansen-Jagannathan bounds are available in the literature. The discussion here is brief, quickly moving on to focus on less well-known refinements of the bounds to incorporate conditioning information.Section 4 discusses empirical methods, starting with Hansen's (1982) Generalized Method of Moments (GMM). This important approach has also been the subject of several review articles and textbook chapters. We briefly review the use of the GMM to estimate stochastic discount factor models. This section is included only to make the latter parts of the paper accessible to a reader who is not already familiar with the GMM. Section 4 then discusses two special cases that remain important in empirical asset pricing. The first is the cross-sectional regression approach, as developed by Fama and MacBeth (1973), and the second is the multivariate regression approach, popularized in the finance literature following Gibbons (1982).Once the mainstay of empirical work on asset pricing, cross-sectional regression continues to be used and useful. Our main focus is on the economic interpretation of the estimates. The discussion attempts to shed light on recent studies that employ the empirical factors advocated by Fama and French (1993, 1996), or generalizations of that approach. The multivariate regression approach to testing portfolio efficiency can be motivated by its immunity to the errors-in-variables problem that plagues the two step, cross-sectional regression approach. The multivariate approach is also elegant, and provides a nice intuition for the statistical tests.A regression approach, with a beta pricing formulation, and a GMM approach with a stochastic discount factor formulation, may be considered as competing paradigms for empirical work in asset pricing. However, under the same distributional assumptions, and when the same moments are estimated, the two approaches are essentially equivalent. The present discussion attempts to clarifythese points, and suggests how to think about the choice of empirical method.Section 5 brings the models and methods together, in a review of the relatively recent literature on conditional performance evaluation. The problem of measuring the performance of managed portfolios has been the subject of research for more than 30 years. Traditional measures use unconditional expected returns, estimated by sample averages, as the baseline. However, if expected returns and risks vary over time, this may confuse common time-variation in fund risk and market risk premiums with average performance. In this way, traditional methods can ascribe abnormal performance to an investment strategy that trades mechanically, based only on public information. Conditional performance evaluation attempts to control these biases, while delivering potentially more powerful performance measures, by using lagged instruments to control for time-varying expectations. Section 5 reviews the main models for conditional performance evaluation, and includes a summary of the empirical evidence. Finally, Section 6 of this paper offers concluding remarks.2. Multifactor Asset Pricing Models: Review and Integration2.1 The Stochastic Discount Factor RepresentationVirtually all asset pricing models are special cases of the fundamental equation:P t = E t {m t+1 (P t+1 + D t+1)}, (1)where P t is the price of the asset at time t and D t+1 is the amount of any dividends, interest or other payments received at time t+1. The market-wide random variable m t+1 is the stochastic discount factor (SDF).1 The prices are obtained by "discounting" the payoffs using the SDF, or multiplying by m t+1, so1 The random variable mis also known as the pricing kernel, benchmark pricing variable, ort+1that the expected "present value" of the payoff is equal to the price.The notation E t {.} denotes the conditional expectation, given a market-wide information set, Ωt . Since empiricists don't get to see Ωt , it will be convenient to consider expectations conditioned on an observable subset of instruments, Z t . These expectations are denoted as E(.|Z t ). When Z t is the null information set, we have the unconditional expectation, denoted as E(.). Empirical work on asset pricing models like (1) typically relies on rational expectations , interpreted as the assumption that the expectation terms in the model are mathematical conditional expectations. Taking the expected values of equation (1), rational expectations implies that versions of (1) must hold for the expectations E(.|Z t ) and E(.).Assuming nonzero prices, equation (1) is equivalent to:E(m t+1 R t+1 - 1 |Ωt )=0, (2)where R t+1 is the N-vector of primitive asset gross returns and 1 is an N-vector of ones. The gross return R i,t+1 is defined as (P i,t+1 + D i,t+1)/P i,t . We say that a SDF "prices" the assets if equations (1) and(2) are satisfied. Empirical tests of asset pricing models often work directly with equation (2) and the relevant definition of m t+1.Without more structure the equations (1-2) have no content because it is almost always possible to find a random variable m t+1 for which the equations hold. There will be some m t+1 that "works," in this sense, as long as there are no redundant asset returns.2 With the restriction that m t+1 is intertemporal marginal rate of substitution, depending on the context. The representation (1) goes at least back to Beja (1971), while the term "stochastic discount factor" is usually ascribed to Hansen and Richard (1987).2 For example, take a sample of assets with a nonsingular second moment matrix and let m t+1 bea strictly positive random variable, equation (1) becomes equivalent to the no arbitrage principle, which says that all portfolios of assets with payoffs that can never be negative, but which are positive with positive probability, must have positive prices [Beja (1971), Rubinstein (1976), Ross (1977), Harrison and Kreps (1979), Hansen and Richard (1987)].The no arbitrage condition does not uniquely identify m t+1 unless markets are complete. In that case, m t+1 is equal to primitive state prices divided by state probabilities. To see this write equation (1) as P i,t = E t{m t+1X i,t+1}, where X i,t+1 = P i,t+1 + D i,t+1. In a discrete-state setting, P it =Σsπs X i,s =Σs q s(πs/q s)X i,s, where q s is the probability that state s will occur and πs is the state price, equal to the value at time t of one unit of the numeraire to be paid at time t+1 if state s occurs at time t+1. X i,s is the total payoff of the security i at time t+1 if state s occurs. Comparing this expression with equation (1) shows that m s = πs/q s > 0 is the value of the SDF in state s.While the no arbitrage principle places some restrictions on m t+1, empirical work typically explores the implications of equilibrium models for the SDF, based on investor optimization. Consider the Bellman equation for a representative consumer-investor's optimization:J(W t,s t) ≡ Max E t{ U(C t,.) + J(W t+1,s t+1)}, (3)where U(C t,.) is the direct utility of consumption expenditures at time t, and J(.) is the indirect utility of wealth. The notation allows the direct utility of current consumption expenditures to depend on variables such as past consumption expenditures or other state variables, s t. The state variables are sufficient statistics, given wealth, for the utility of future wealth in an optimal consumption-investment plan. Thus, changes in the state variables represent future consumption-investment opportunity risk. [1' (E t{R t+1R t+1'})-1]R t+1.The budget constraint is: W t+1 = (W t - C t) x'R t+1, where x is the portfolio weight vector, subject to x'1 = 1.If the allocation of resources to consumption and investment assets is optimal, it is not possible to obtain higher utility by changing the allocation. Suppose an investor considers reducing consumption at time t to purchase more of (any) asset. The expected utility cost at time t of the foregone consumption is the expected marginal utility of consumption expenditures, U c(C t,.) > 0 (where a subscript denotes partial derivative), multiplied by the price P i,t of the asset, measured in the numeraire unit. The expected utility gain of selling the investment asset and consuming the proceeds at time t+1 is E t{(P i,t+1+D i,t+1) J w(W t+1,s t+1)}. If the allocation maximizes expected utility, the following must hold: P i,t E t{U c(C t,.)} = E t{(P i,t+1+D i,t+1) J w(W t+1,s t+1)}, which is equivalent to equation (1), withm t+1 = J w(W t+1,s t+1)/E t{U c(C t,.)}. (4)The m t+1 in equation (4) is the intertemporal marginal rate of substitution (IMRS) of the consumer-investor, and equations (2) and (4) combined are the intertemporal Euler equation.Asset pricing models typically focus on the relation of security returns to aggregate quantities. To get there, it is necessary to aggregate the Euler equations of individuals to obtain equilibrium expressions in terms of aggregate quantities. Theoretical conditions which justify the use of aggregate quantities are discussed by Wilson (1968), Rubinstein (1974) and Constantinides (1982), among others. Some recent empirical work does not assume aggregation, but relies on panels of disaggregated data. Examples include Zeldes (1989), Brav, Constantinides and Geczy (2002) and Balduzzi and Yao (2001).Multiple factor models for asset pricing follow when m t+1 can be written as a function ofseveral factors. Equation (4) suggests that likely candidates for the factors are variables that proxy for consumer wealth, consumption expenditures or the state variables -- the sufficient statistics for the marginal utility of future wealth in an optimal consumption-investment plan.Expected Risk PremiumsTypically, empirical work focuses on expressions for expected returns and excess rates of return. Expected excess returns are related to the risk factors that create variation in m t+1. Consider any asset return R i,t+1 and a reference asset return, R0,t+1. Define the excess return of asset i, relative to the reference asset as r i,t+1 = R i,t+1 - R0,t+1. If equation (2) holds for both assets it implies:E t{m t+1 r i,t+1} = 0 for all i. (5)Use the definition of covariance to expand equation (5) into the product of expectations plus the covariance, obtaining:E t{r i,t+1} = Cov t(r i,t+1; -m t+1) / E t{m t+1}, for all i, (6)where Cov t(.;.) is the conditional covariance. Equation (6) is a general expression for the expected excess return, from which most of the expressions in the literature can be derived. The conditional covariance of return with the SDF, m t+1, is a very general measure of systematic risk. Asset pricing models say that assets earn expected return premiums for their systematic risk, not their total risk (i.e., variance of return). The covariance with -m t+1 is systematic risk because it measures the component of the return that contributes to fluctuations in the marginal utility of wealth. If we regressed the assetreturn on the SDF, the residual in the regression would capture the "unsystematic" risk and would not be "priced," or command a risk premium.If the conditional covariance with the SDF is zero for a particular asset, the expected excess return of that asset should be zero.3 The more negative is the covariance with m t+1 the less desireable is the distribution of the random return, as the larger payoffs tend to occur when the marginal utility is low. The expected compensation for holding assets with this feature must be higher than for those with a more desireable distribution. Expected risk premiums should therefore differ across assets in proportion to their conditional covariances with -m t+1.Return PredictabilityRational expectations implies that the difference between return realizations and the expectations in the model should be unrelated to the information that the expectations in the model are conditioned on. For example, equation (2) says that the conditional expectation of the product of m t+1 and R i,t+1 is the constant, 1.0. Therefore, 1-m t+1R i,t+1 should not be predictably different from zero using any information available at time t. If we run a regression of 1-m t+1R i,t+1 on any lagged variable, Z t, the regression coefficients should be zero. If there is predictability in a return R i,t+1 using instruments Z t, the model implies that the predictability is removed when R i,t+1 is multiplied by the correct m t+1. This is the sense in which conditional asset pricing models are asked to "explain" predictable variation in asset returns. This view generalizes the older "random walk" model of stock values, which states that stock returns should be completely unpredictable. That model is a special case which can be motivated by 3 Equation (6) is weaker than equation (2), since equation (6) is equivalent to E{m t+1R i,t+1} = ∆t, all i,twhere ∆t is a constant across assets, while equation (2) restricts ∆t=1. Therefore, empirical tests based on equation (6) do not exploit all of the restrictions implied by a model that may be stated in the form of equation (2).risk neutrality. Under risk neutrality the IMRS, m t+1, is a constant. Therefore, in this case the model implies that the return R i,t+1 should not differ predictably from a constant.Conditional asset pricing presumes the existence of some return predictability. There should be instruments Z t for which E(R t+1|Z t) or E(m t+1|Z t) vary over time, in order for the equation E(m t+1R t+1-1|Z t)=0 to have empirical bite.4 Interest in predicting security market returns is about as old as the security markets themselves. Fama (1970) reviews the early evidence.One body of literature uses lagged returns to predict future stock returns, attempting to exploit serial dependence. High frequency serial dependence, such as daily or intra-day patterns, are often considered to represent the effects of market microstructure, such as bid-ask spreads (e.g. Roll, 1984) and nonsynchronous trading of the stocks in an index (e.g. Scholes and Williams, 1977). Serial dependence at longer horizons may represent predictable changes in the expected returns.Conrad and Kaul (1989) report serial dependence in weekly returns. Jegadeesh and Titman (1993) find that relatively high return, "winner" stocks tend to repeat their performance over three to nine-month horizons. DeBondt and Thaler (1985) find that past high-return stocks perform poorly over the next five years, and Fama and French (1988) find negative serial dependence over two to five-year horizons. These serial dependence patterns motivate a large number of studies which attempt to assess the economic magnitude and statistical robustness of the implied predictability, or to explain the predictability as an economic phenomenon. For more comprehensive reviews, see Campbell, Lo and MacKinlay (1997) or Kaul (1996). Research in this area continues, and its fair to say that the jury is still out on the issue of predictability using lagged returns.4 At one level this is easy. Since E(m|Z t) should be the inverse of a risk-free return, all we need ist+1observable risk free rates that vary over time. Ferson (1989) shows that the behavior of stock returns and short term interest rates imply that conditional covariances of returns with m t+1 must also vary over time.A second body of literature studies predictability using other lagged variables as instruments. Fama and French (1989) assemble a list of variables from studies in the early 1980's, that as of this writing remain the workhorse instruments for conditional asset pricing models. These variables include the lagged dividend yield of a stock market index, a yield spread of long-term government bonds relative to short term bonds, and a yield spread of low-grade (high default risk) corporate bonds over high-grade bonds. In addition, studies often include the level of a short term interest rate (Fama and Schwert (1977), Ferson, 1989) and the lagged excess return of a medium-term over a short-term Treasury bill (Campbell (1987), Ferson and Harvey, 1991). Recently proposed instruments include an aggregate book-to-market ratio (Pontiff and Schall, 1998) and lagged consumption-to-wealth ratios (Lettau and Ludvigson, 2000). Of course, many other predictor variables have been proposed and more will doubtless be proposed in the future.Predictability using lagged instruments remains controversial, and there are some good reasons the question the predictability. Studies have identified various statistical biases in predictive regressions (e.g. Hansen and Hodrick (1980), Stambaugh (1999), Ferson, Sarkissian and Simin, 2002), questioned the stability of the predictive relations across economic regimes (e.g. Kim, Nelson and Startz, 1991) and raised the possibility that the lagged instruments arise solely through data mining (e.g. Campbell, Lo and MacKinlay (1990), Foster, Smith and Whaley, 1997).A reasonable response to these concerns is to see if the predictive relations hold out-of-sample. This kind of evidence is also mixed. Some studies find support for predictability in step-ahead or out-of-sample exercises (e.g. Fama and French (1989), Pesaran and Timmerman, 1995). Similar instruments show some ability to predict returns outside the U.S. context, where they arose (e.g. Harvey (1991), Solnik (1993), Ferson and Harvey, 1993, 1999). However, other studies conclude that predictability using the standard lagged instruments does not hold (e.g. Goyal and Welch (1999),Simin, 2002). It seems that research on the predictability of security returns will always be interesting, and conditional asset pricing models should be useful in framing many future investigations of these issues.2.2 Consumption-based Asset Pricing ModelsIn these models the economic agent maximizes a lifetime utility function of consumption (including possibly a bequest to heirs). Consumption models may be derived from equation (4) by exploiting the envelope condition, U c (.) = J w (.), which states that the marginal utility of consumption must be equal to the marginal utility of wealth if the consumer has optimized the tradeoff between the amount consumed and the amount invested.Breeden (1979) derived a consumption-based asset pricing model in continuous time, assuming that the preferences are time-additive. The utility function for the lifetime stream of consumption is Σt βt U(C t ), where β is a time preference parameter and U(.) is increasing and concave in current consumption, C t . Breeden's model is a linearization of equation (2) which follows from the assumption that asset values and consumption follow diffusion processes [Bhattacharya (1981), Grossman and Shiller (1982)]. A discrete-time version follows Rubinstein (1976) and Lucas (1978), assuming a power utility function:αα−−=−11)(1C C U , (7) where α > 0 is the concavity parameter. This function displays constant relative risk aversion 5 equal to α. Using (7) and the envelope condition, the IMRS in equation (4) becomes: 5 Relative risk aversion in consumption is defined as -Cu''(C)/u'(C). Absolute risk aversion is -u''(C)/u'(C). Ferson (1983) studies a consumption-based asset pricing model with constant absolute risk aversion.。