Vmware平台上高性能计算的性能评估
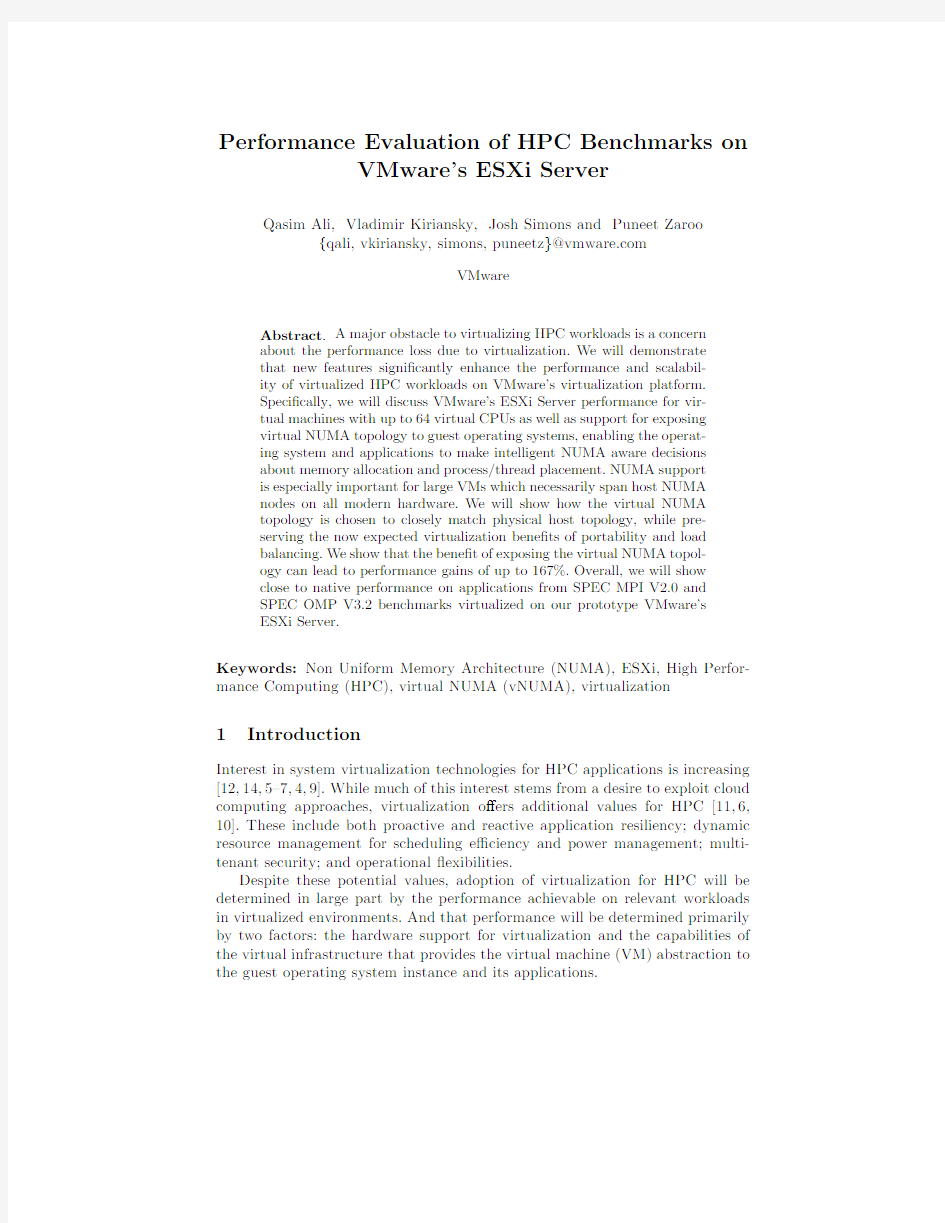

Performance Evaluation of HPC Benchmarks on
VMware’s ESXi Server
Qasim Ali,Vladimir Kiriansky,Josh Simons and Puneet Zaroo
{qali,vkiriansky,simons,puneetz}@https://www.360docs.net/doc/758884918.html,
VMware
Abstract.A major obstacle to virtualizing HPC workloads is a concern
about the performance loss due to virtualization.We will demonstrate
that new features signi?cantly enhance the performance and scalabil-
ity of virtualized HPC workloads on VMware’s virtualization platform.
Speci?cally,we will discuss VMware’s ESXi Server performance for vir-
tual machines with up to64virtual CPUs as well as support for exposing
virtual NUMA topology to guest operating systems,enabling the operat-
ing system and applications to make intelligent NUMA aware decisions
about memory allocation and process/thread placement.NUMA support
is especially important for large VMs which necessarily span host NUMA
nodes on all modern hardware.We will show how the virtual NUMA
topology is chosen to closely match physical host topology,while pre-
serving the now expected virtualization bene?ts of portability and load
balancing.We show that the bene?t of exposing the virtual NUMA topol-
ogy can lead to performance gains of up to167%.Overall,we will show
close to native performance on applications from SPEC MPI V2.0and
SPEC OMP V3.2benchmarks virtualized on our prototype VMware’s
ESXi Server.
Keywords:Non Uniform Memory Architecture(NUMA),ESXi,High Perfor-mance Computing(HPC),virtual NUMA(vNUMA),virtualization
1Introduction
Interest in system virtualization technologies for HPC applications is increasing [12,14,5–7,4,9].While much of this interest stems from a desire to exploit cloud computing approaches,virtualization o?ers additional values for HPC[11,6, 10].These include both proactive and reactive application resiliency;dynamic resource management for scheduling e?ciency and power management;multi-tenant security;and operational?exibilities.
Despite these potential values,adoption of virtualization for HPC will be determined in large part by the performance achievable on relevant workloads in virtualized environments.And that performance will be determined primarily by two factors:the hardware support for virtualization and the capabilities of the virtual infrastructure that provides the virtual machine(VM)abstraction to the guest operating system instance and its applications.
While there are many aspects of the VM abstraction that contribute to the delivered performance of an application,this paper focuses on two such capabil-ities of particular importance to HPC.The?rst is support for VMs with many virtual CPUs which is required to allow thread-parallel OpenMP and other sim-ilar codes including hybrid MPI/OpenMP applications to take full advantage of the underlying cores in modern multi-core systems.The second is support for exposing the NUMA topology of the underlying hardware to the guest operating system so that it,along with any NUMA-aware runtime libraries,can optimally co-locate compute and data where possible.
The paper presents a brief overview of ESXi server,VMware’s commercial hypervisor,with an emphasis on its scalability properties.We then describe how ESXi exposes a virtual NUMA topology to guest operating systems,and?nally present experimental results using SPEC OMP and SPEC MPI as representative workloads.
2ESXi Server Architecture
VMware ESXi Server is VMware’s bare-metal hypervisor which runs directly on physical hardware.It multiplexes physical hardware among multiple VMs and works in conjunction with the virtual machine monitor(VMM),an instance of which runs per VM.By managing hardware resources directly,ESXi Server achieves high performance by reducing virtualization overhead[13].
In about a decade of existence,VMware’s server virtualization platform has undergone many advancements to increase its scalability and performance.The scalability improvements have led to support for larger physical and virtual pro-cessor counts and memory sizes,as well as higher VM consolidation rates.These scalability increases have been enabled by advancements in both the virtual machine monitor and ESXi hypervisor.Some key features which have enabled these advances are architectural changes to support large virtual processor counts (large SMP VMs),support for64bit x86architecture and e?cient use of hard-ware virtualization support[2],along with advanced physical CPU,memory and IO management[13].Supporting large SMP VMs required careful data structure design,coscheduling improvements[3],?ne-grained locking,and best software engineering practices to enable support for64virtual CPUs in a VM with mini-mal virtualization overhead.In the next section,we discuss the presentation of a virtual NUMA topology to guest operating systems running inside VMs,which proved crucial to the performance of virtualized HPC workloads.
3Virtual NUMA support
Current generation operating systems,runtime libraries and applications are ex-pected to be NUMA-aware for improved performance,e.g.they would allocate memory and schedule execution threads to take advantage of the NUMA topol-ogy.To support this within a virtual environment,we introduce the concept of a virtual NUMA topology which can be exposed to a guest operating system.This
abstraction is then mapped by the ESXi NUMA scheduler to an intermediate level of abstraction physical NUMA node,which is in turn dynamically bound to speci?c machine NUMA node resources on the host system.This hierarchy of (virtual,physical,machine)is analogous to that used to implement virtual mem-ory in a virtualized environment.It should be noted that there is not necessarily a one-to-one correspondence between virtual and physical NUMA nodes since multiple physical NUMA nodes may be required to provision the required CPUs and interleaved memory for a virtual NUMA node.Similarly,multiple physical NUMA nodes may be scheduled on the same machine NUMA node,and even over-commit available CPU and memory resources.
The number of virtual NUMA nodes,the number of virtual CPUs(vCPUs) and the amount of memory associated with the virtual nodes normally remain ?xed for the lifetime of a VM.We also support hot-plug add of CPU and memory resources,though not all OSes support hot-plug remove or dynamic changes to NUMA topology.Our virtual BIOS exposes the ACPI Static Resource A?nity Table(SRAT)[1]and ACPI PXM methods to guest OSes to reveal which mem-ory regions and vCPUs belong to which virtual NUMA node.Minimally NUMA aware OSes only distinguish between local vs remote allocation;most modern ones take into account the minimal inter node latencies(or number of hops);yet more advanced OSes need to track maximum link bandwidths,and ultimately total system bandwidth for optimal scheduling.There are no standard facilities for exposing the actual link topology connecting NUMA nodes,e.g.Intel QPI or AMD HyperTransport frequency,number of lanes,lane widths,and for par-tially connected systems the routes and congestion policies that determine the maximum total interconnect bandwidth.We don’t expose ACPI System Local-ity Information Table(SLIT)[1]information which would only provide?xed latency information.Most modern guest OSes measure during initialization the unidirectional access costs for a vCPU from one virtual NUMA node accessing memory on another virtual NUMA node.
There are several scenarios in which the NUMA topology deduced by the guest OS may become inaccurate over the lifetime of the virtual machine and the remote memory access latency and bandwidth may change.First,the VM might get powered on,suspended and resumed,or live migrated to hosts with di?erent machine NUMA topology than the original system.If di?erent number of physical nodes may be needed,e.g.if originally4nodes were used and now 8nodes are needed,memory interleaving across pairs of physical nodes will be necessary.The physical NUMA node abstraction accommodates even a VM started with8vCPUs per NUMA node after it is migrated to a node with6 PCPUs per NUMA node with best e?orts to minimize the performance impact. Second,even if the same number of physical nodes are needed they may use a di?erent NUMA topology.For example,when no subset of4machine nodes on an8-socket host is fully connected,a4-node VM would need more hops for some remote accesses,instead of symmetric accesses on a fully connected4-socket host.
It is also possible for the ESXi NUMA scheduler to choose di?erent actual NUMA nodes for placement based on the system workload.Physical NUMA
nodes belonging to the same VM which are smaller than the machine NUMA node may be either consolidated or spread over multiple machine nodes.For example,a single VM workload may bene?t from spreading over four NUMA nodes to gain higher memory bandwidth and cache capacity,but two such VMs should each be using two non-overlapping NUMA nodes and links.
Trading o?maximum single VM performance versus optimal total system performance and overall fairness across VMs can add additional constraints. While the optimal choices are speci?c to the VM workload,the best physical NUMA topology and placement on machine nodes depends on the overall load on the system and each NUMA node load.Other VMs’CPU utilization,cache capacity,and memory bandwidth consumption may impact the scheduler choices as well.We expose manually con?gurable policies that a?ect both the?xed virtual NUMA topology,initial physical NUMA topology placement,and load balancing.
If the memory bandwidth demands of the VM are very high,then using mul-tiple memory controllers will be more bene?cial and thus spreading over multiple machine NUMA nodes would be favored.If there are high levels of inter-thread communication,sharing the same last level cache will be preferred and thus consolidation over fewer machine nodes will be favored.Finally,a VM may be con?gured to favor using SMT hyperthreads instead of full cores-appropriate when the lower latency of local memory may outweigh the disadvantage of not using the full resources of a core.This bene?ts workloads with higher level of inter-thread communication or external I/O,where cores will otherwise be un-derutilized.So,for example,a VM with16vCPUs,on a host with four8-core (16SMT)sockets will perform best with either one,two,or four NUMA nodes, depending on the workload.
The above complexities and potential divergence over time of guest OS deter-mined topology are most pronounced for heterogeneous workloads consolidated on hosts in heterogeneous clusters.Maximum performance of a single VM run-ning on hosts with identical machine NUMA topology is an equally important scenario.In our experimental evaluation we discuss performance of VMs with maximum vCPU count with matching virtual,physical and machine topology. We expect these to be typical con?gurations for virtualized HPC environments which generally will not over-subscribe hardware resources and which employ homogeneous clusters.
4Experimental Evaluation
We evaluated our prototype ESXi Server using four applications from the SPEC MPI V2.0suite and seven applications from SPEC OMP V3.2suite.SPEC MPI workloads are meant to be evaluated on distributed memory machines but can be useful for performance evaluation on shared memory machines as well.SPEC OMP is designed for use on shared-memory machines and is well-suited to evaluate the performance of large SMP virtual machines for HPC. All the experimental results reported here were gathered on2.27GHz Intel(R)
Xeon(R)CPU X7560processors based on the Nehalem-EX architecture.All the benchmarks were compiled using gcc-4.3and gfortran.We present three types of results in this paper:
–Gains obtained due to vNUMA
–Native to virtual runtime ratio showing virtual performance
–Native and virtual scaling
Our virtual NUMA(vNUMA)results start at16vCPUs since this represents the two-socket case on our test system.Native to virtual ratio is obtained by di-viding the completion time of an application in the native case by the completion time of the virtual run.A ratio greater than1indicates that a VM runs faster than the native.Scaling results are reported starting at four vCPUs/processes /threads because some MPI tests failed to run using fewer than four processes. All of the SPEC benchmark applications were run for two iterations,which is not SPEC-compliant.Run-to-run variation was within1-2%.The numbers reported here are the average of the two runs.
4.1SPEC MPI Results
The SPEC MPI applications were evaluated on a Dell PowerEdge R910,which has four sockets and a total of256GB memory.RHEL5.4was used as a native and guest OS with kernel version2.6.18-164.All VMs were con?gured with128 GB of virtual RAM.We used Open MPI v1.4[8]and the SPEC MPI medium dataset for our tests.
(a)Native to Virtual Runtime Ratio(b)Default vs vNUMA Runtime Fig.1.SPEC MPI Virtualization performance,and Interleaving vs vNUMA with vCPU count=Number Of Physical Cores=32
We conducted two sets of experiments.In the?rst set,the number of vCPUs is equal to the number of physical cores in the system(32in this case)and the
number of processes spawned is the same as in the case of native.This con?g-uration is similar to the native setup in the sense that the guest OS scheduler would schedule as it would on a native system with the minor di?erence that in the native case it would schedule on64logical threads,while in the virtual case, it would schedule on32vCPUs and the32vCPUs would then be scheduled by the ESXi scheduler on the64logical threads.We do not expose hyper-threading information to the guest and for this workload we need full cores for each vCPU. While this con?guration has the best performance,we discuss alternative VM size con?gurations later in our evaluation.In the second set of experiments,the number of vCPUs con?gured for a VM is set equal to the number of processes spawned in each SPEC MPI run–we size the VM equal to the size of the MPI job.
Figure1(a)shows the native to virtual runtime ratio for four applications from the SPEC MPI V2.0suite.The ratio for most of the applications is close to one which indicates that virtualization is adding little or no overhead in this case.In certain cases we see up to20%better virtual performance than native as in the case of the milc application run with32processes.We observed that native Linux scheduler gives better performance if HT is OFF versus when it is ON (32-process SPEC MPI applications on the64logical threads).We also observed that many SPEC MPI applications on a native system with64processes on a 64logical core system like the Nehalem-EX system were not gaining much over the performance of the32-process run.Hence we sized the VM with32vCPUs (because there were32physical cores in the system).Also typically in HPC applications,processes/threads are spawned based on number of physical cores rather than logical cores.
Figure1(b)shows the performance gain obtained due to exposing to the guest OS a virtual NUMA topology matching the machine topology.In this?g-ure,Default-16means that vNUMA is disabled(memory is interleaved over the physical NUMA nodes)and the su?x16means that sixteen MPI processes were spawned within the MPI application,whereas vNUMA-16means that vNUMA is enabled(virtual NUMA nodes match physical NUMA nodes).The gain due to vNUMA for milc were14%and33%for the16and32vCPU cases,respec-tively.Similarly for LU,the gains are11%and32%for16and32vCPU VMs. Noticeable gains were also observed for the pop2and lammps applications.
In order to better understand the trade-o?s in performance,we modi?ed the virtual experiments so that the number of vCPUs con?gured for a VM is equal to the number of processes spawned in SPEC MPI applications.Figure2(a)shows the results with the number of vCPUs equal to number of processes.
In Figure2(a),milc and LU actually lose performance for4,8and16rank runs when compared to results in Figure1(a).This is because the scheduling decisions in native and virtual environments were di?erent.For example,in the native four-process case the Linux scheduler spreads the workload across multiple sockets,which increases the e?ective cache size and delivers better performance for applications that bene?t from larger cache sizes.ESXi instead will schedule the four virtual processors on a single socket for better consolidation and to
(a)vCPU count=Num processes(b)vCPU count=32with HT OFF Fig.2.SPEC MPI Virtualization Performance with vCPUs=processes,and with hyper-threading o?
increase cache sharing e?ects.That is the reason why LU’s native to VM ratio is in between0.7and0.9for4,8and16vCPU runs.In the light of these results, the best practice would be to size your VMs depending on your application’s cache footprint.
Both Figure1(a)and Figure2(a)show that native su?ers performance degra-dation with HT.To test whether HT is responsible for this degradation,we dis-abled HT from the system BIOS.Figure2(b)shows the native to virtual ratio with HT OFF in both the VM and the native case with vCPU count equal to32. The ratio is less than one for almost all cases(expect lammps which is slightly higher).Given the fact that both native and virtual con?gurations represented in Figure2(b)have same amount of cache,we conclude that the ESXi scheduler is more optimal than the native scheduler in this case.
Figure3(a)shows native and virtual scaling of the four applications from the SPEC MPI V2.0suite.The su?x”-N”means native run and”-V”means virtual run.In this?gure,HT is ON,which explains why virtual numbers are sometimes better than native,e.g.,the32-process data point.
In the light of the above results,for the virtual runs,HT ON and number of vCPUs being equal to the physical cores in the system is the best con?guration. We used this con?guration for SPEC OMP V3.2benchmarks in the next section.
4.2SPEC OMP Results
SPEC OMP applications were evaluated on an HP ProLiant DL980G7,with eight sockets and512GB RAM.RHEL6.0was used as a native and guest OS with kernel version2.6.32-71.el6.All VMs were con?gured with128GB of virtual RAM.The large data sets were used for all SPEC OMP experiments.Figure4(a) shows the virtualization performance for SPEC OMP V3.2benchmarks.The native to virtual runtime ratio was close to one for all applications expect for
(a)SPEC MPI V2.0Applications(b)SPEC OMP V3.2Applications
Fig.3.Native and Virtual Scaling with vCPUs=No.Of Physical Cores.
(a)Native to Virtual Runtime Ratio(b)Default vs vNUMA Runtime Fig.4.SPEC OMP Virtualization performance,and Interleaving vs vNUMA with vCPU count=Number Of Physical Cores=64
equake(four process and64process run)and fma3d(4process run)where the ratio was greater than one.As was explained earlier,this is because native scheduling with HT ON is sub-optimal.
Figure4(b)shows performance gains due to vNUMA for seven di?erent ap-plications from the SPEC OMP V3.2suite.
Strikingly,swim with16threads and virtual NUMA performs better than 32or even64threads without virtual NUMA topology!This shows the memory bandwidth demands of this benchmark are much more critical than the thread count.With16threads the gains on swim from vNUMA are167%,but with 64threads the bene?t is down to72%,probably because of saturated memory bandwidth.
Art starts with56%vNUMA gains at16threads,and the bene?ts increase to 71%at64threads.Most likely memory bandwidth becomes a bigger bottleneck at higher thread counts and bandwidth is still available.
Fma3d and Wupwise have a relatively constant gain from vNUMA at di?er-ent thread counts respectively17%and30%where both peak at32threads. Given that the increased memory bandwidth demand of higher number of threads doesn’t a?ect performance,these applications are probably latency-sensitive but not bandwidth-sensitive.Our hypothesis is that instead of12.5%locality when using8sockets,the lower latency for accessing up to100%local memory is the main reason for these gains.
Applu and Equake gain signi?cantly at16-threads respectively114%and 30%,but at64-threads the vNUMA gains drop to2%and5%.This implies that initially memory bandwidth was the biggest bottleneck but new bottle-necks emerge at higher thread counts.Little gain in Apsi was observed probably because of a smaller memory bandwidth demand.
Overall at64threads two applications get more than70%gains from vNUMA, and the geometric mean of the performance gains over all applications is25%. All applications achieve their best performance at64threads but don’t scale equally well.
Figure3(b)shows native and virtual scaling for the seven applications.All applications show similar trends for virtual and native scaling.Some virtual and scaling data points were not close due to the di?erence in HT scheduling.One important point to note here is that Apsi,Swim and Equake are not scaling as nicely as other applications beyond16threads.Evaluating a multi-VM scenario on a single host as well on a cluster of hosts will be covered in future work.
5Conclusion
We demonstrated that HPC workloads on VMware’s virtualization platform achieve close to native performance(in some cases even20%better than na-tive)with applications from SPEC MPI and SPEC OMP benchmarks.We eval-uated the new features in VMware’s ESXi Server that signi?cantly enhance the performance and scalability of HPC workloads.
Exposing a virtual NUMA topology that closely matches the physical host topology is a major feature,bridging the gap between native and virtual per-formance for VMs with large memory and high vCPUs counts.Gains of up to 2.67x were observed.
Virtual machines with up to64virtual CPUs now achieve their best perfor-mance when a virtual NUMA topology is exposed to guest operating systems. This allows operating systems and applications to make intelligent decisions about memory allocation and process/thread placement.We discussed the fea-tures required for a production system that exposes virtual NUMA topology.We preserve the now-expected virtualization bene?ts of portability and load balanc-ing both within a host and a cluster,yet with with minimal overhead for a single VM.
Acknowledgments We’d like to thank our colleagues Je?rey Buell,Ishaan Joshi,and Seongbeom Kim for helpful experiments and suggestions,and our monitor and VMkernel group colleagues for making this work possible. References
1.Advanced Con?guration and Power Interface speci?cation,rev4.0a,2009.http:
//https://www.360docs.net/doc/758884918.html,/spec40.htm.
2.O.Agesen,A.Garthwaite,J.Sheldon,and P.Subrahmanyam.The evolution of
an x86virtual machine monitor.Operating Systems Review,44(4),2010.
3.Scalable Infrastructure with the CPU scheduler in VMware ESX
4.1.http://www.
https://www.360docs.net/doc/758884918.html,/files/pdf/techpaper/VMW vSphere41cpu schedule ESX.pdf.
4. A.Gavrilovska and S.K.et al.High-Performance Hypervisor Architectures:Vir-
tualization in HPC Systems.In In HPCVirt2007:1st Workshop on System-level Virtualization for High Performance Computing,2007.
5.W.Huang,Q.Gao,J.Liu,and D.K.Panda.High performance virtual machine
migration with RDMA over modern interconnects.In Proceedings of the2007 IEEE International Conference on Cluster Computing,CLUSTER’07,pages11–20,Washington,DC,USA,2007.IEEE Computer Society.
6.M.F.Mergen,V.Uhlig,O.Krieger,and J.Xenidis.Virtualization for high-
performance computing.SIGOPS Oper.Syst.Rev,40:8–11,2006.
7. A.B.Nagarajan and F.Mueller.Proactive fault tolerance for HPC with Xen
Virtualization.In In Proceedings of the21st Annual International Conference on Supercomputing(ICS07),pages23–32.ACM Press,2007.
8.Open MPI:Open Source High Performance Computing,http://www.open-mpi.
org,2011.
9. A.Ranadive,M.Kesavan,A.Gavrilovska,and K.Schwan.Performance implica-
tions of virtualizing multicore cluster machines.In Proceedings of the2nd workshop on System-level virtualization for high performance computing,HPCVirt’08,pages 1–8,New York,NY,USA,2008.ACM.
10. D.Rao and K.Schwan.vNUMA-mgr:Managing VM memory on NUMA platforms.
In High Performance Computing(HiPC),2010International Conference on,pages 1–10,dec.2010.
11.J.E.Simons and J.Buell.Virtualizing high performance computing.SIGOPS
Oper.Syst.Rev.,44:136–145,December2010.
12.G.Valle,C.Engelmann,S.L.Scott,T.Naughton,and H.Ong.System-Level
Virtualization for High Performance Computing,Feb.13-15,2008.
13. C.A.Waldspurger.Memory resource management in VMware ESX server.In
OSDI’02:Proceedings of the5th symposium on Operating systems design and implementation,pages181–194,New York,NY,USA,2002.ACM Press.
14.L.Youse?,K.Seymour,H.You,J.Dongarra,and R.Wolski.The impact of paravir-
tualized memory hierarchy on linear algebra computational kernels and software.In Proceedings of the17th international symposium on High performance distributed computing,HPDC’08,pages141–152,New York,NY,USA,2008.ACM.
基于大数据和云计算平台与应用
基于大数据和云计算平台与应用 发表时间:2018-08-20T16:09:00.780Z 来源:《基层建设》2018年第21期作者:全仲谋 [导读] 摘要:大数据应用的发展对信息系统及其应用提出了更高要求,而基于云计算的大计算平台技术已成为现代建模仿真领域的核心技术,尤其是当前社会各领域开始注重对基于数据的应用,大数据的兴起引发了社会各领域研究、应用大数据的热潮。 中国移动通信集团广东有限公司湛江分公司 524033 摘要:大数据应用的发展对信息系统及其应用提出了更高要求,而基于云计算的大计算平台技术已成为现代建模仿真领域的核心技术,尤其是当前社会各领域开始注重对基于数据的应用,大数据的兴起引发了社会各领域研究、应用大数据的热潮。本文详细阐述了大数据和云计算平台应用的基本概念,病态系讨论了大数据和云计算平台的实际应用。 关键词:大数据;云计算;平台;应用 引言 “大数据”这个词在世界上的地位日益显著,甚至隐约可以成为这个时代的代名词。对于数据信息的采集和处理已然成为各行各业创造经济突破的新增长点,是企业战略目标制定和实施的关键依据。大数据的概念决定了它需要在一个特殊的平台上才能够发挥作用,庞大的信息量并不是以往的单机处理系统可以“吃得消”的。而云计算平台的建立正好弥补了这一方面的短板,其新颖的信息处理模式与大数据概念有着很好的契合度。但是目前大多数研究者的目光都是集中在大数据分析上,关于大数据与云计算平台应用的研究尚处于初级阶段。不过可以预期,未来大数据和云计算平台必将成为社会的发展核心。 一、大数据与云计算平台概述 1、大数据的特征。大数据又被IT业称之为巨量数据集合,具体是指无法在某个特定时间范围内用常规的软件工具进行捕捉、管理和处理的数据集合,是一种海量、多样化、高增长率的信息资产。大数据的特征主要体现在如下几个方面:超大的容量、繁多的种类、获取数据的高速、数据质量真实可靠、数据来源渠道复杂等等。信息时代到来的今天,数据信息在生产生活中的重要性日益凸显,大数据的发展速度也变得越来越快,对信息处理提出了更高的要求,即需要在短时间内对数据库进行有关的操作与处理,为满足这一需求,大数据技术应运而生。 2、云计算平台的优势。云计算是以网络为平台,利用远程连接的计算机获取所需计算服务,该计算机可供给弹性伸缩的计算资源,可提高资源利用效率,节省因重复配置资源增加的成本。云计算的优点:1.计算能力强。云计算可对计算机集群中的CPU进行远程调用,使其具备强大的计算能力,每秒高达10万亿次运算。2.可靠性高。云计算使用数据容错技术和计算节点同构可互换措施,能够保证云计算服务的可靠性。3.使用成本低。云计算采用自动化集中式管理,按需分配使用硬件资源,无需支付数据管理成本。 3、大数据与云计算平台的关系。大数据与云计算的联系紧密,两者均能够为数据资源提供存储、访问和计算的平台。对于云计算而言,其核心技术为数据处理技术,最终目的是为国家、企业和个人提供便捷服务,这与大数据的发展目的一致。大数据拥有丰富的数据资源,能够与云计算平台共同一个平台,进行大数据分析与计算,两者的相似度极高。 二、大数据与云计算平台优势分析 数据处理是大数据的基础要求,新时代下的“大数据”理念已经是无法用传统计算机处理方式来满足的,因而需要一种新的计算方式作为支持。容量大、种类多、价值高、更新快的特点使得大数据看起来像是一座高楼大厦,有着巨大的价值等待人们的开发利用,而云计算所提供的安全、高效的数据应用服务可以有力地支撑这座楼房。 大数据与云计算平台是一个由众多技术融合的综合体,其主要包括虚拟化技术、分布式海量数据存储与管理和分布式并行编程技术。大数据与云计算平台充分利用云计算适用于数据密集型计算的特点,很好地贴合了大数据对数据量和数据类型的要求;云计算分散到集群电脑的处理方式能够实现数据的及时调用和动态调整,达到高效、快速处理数据信息的目的;平台可以利用虚拟化处理方式对电脑本地资源、网络资源等进行整合、按照要求进行统一调度,实现信息价值最大化。同时大数据与云计算平台具有良好的相容性,能够与各种系统应用做到有效契合。以云计算为核心的数据处理平台能够满足更加复杂的操作要求,同时其容量大、运行稳定、安全性高的特点能够适应现在对数据处理的需求;大数据可以为云计算的运行提供指导,对云计算的资源进行有效的调配。 三、基于云计算的大数据平台应用研究 3.1基于云计算的大数据平台优点分析 目前社会各领域所采用的传统单机处理模式成本较高,而且无法根据用户的使用要求进行扩展,随着用户应用数据量的不断增加及数据处理复杂程度的不断提高,这便会导致单机处理模式的性能无法满足用户的实际需求,而基于云计算技术构建而成的大数据平台可以有效解决上述问题,可以为不同层次用户提供安全、高效、便捷的应用数据服务,对提高用户对应用数据的使用效率和使用质量有着重要作用。云计算在实际运用中具备良好的弹性伸缩及动态调配等功能,对资源的虚拟化处理及系统的透明性处理可以满足用户按需使用要求,其绿色节能可以最大程度上契合新型大数据处理技术的诸多要求,而以云计算为代表的新一代计算处理模式具有更强大的处理功能,其存储空间、可靠性、安全性、便捷性都可以满足用户需求,并且大数据平台在应用中具有优秀的可平滑迁移、可弹性伸缩等有点,并且可以实现对云计算资源的统一管理和调度等诸多优势特性,所以基于云计算的大数据平台应用已成为未来计算技术的主要发展方向。 3.2基于云计算的大数据平台实际应用 基于云计算技术的大数据平台可以提供聚合大规模分布式系统中,对通讯、存储、处理等能力的需求,并可以为上层平台通过灵活、可靠的方式提供各类应用,并且其在实际应用中可以针对海量多格式、多模式大数据的跨系统、跨平台等操作,提供统一管理手段和敏捷的响应机制,对支持大数据快速变化的功能目标、系统环境以及应用配置有着重要作用。例如,基于云计算技术构建而成的企业信息系统,该新型系统在建设过程中采用了分布式集群技术来构建一个大数据平台,该平台在实际运行中可以支持不同业务应用中多种格式、多种访问模式的大数据统一存储,并采用分布式工作流和调度系统框架来构建一个数据分析系统,利用分布式计算手段实现大数据的转换、关联、提取以及聚合等功能,该类大数据平台在实际应用中可以满足企业各种业务的实际需求。 基于云计算技术的大数据平台可以实现企业决策支撑、销售预测等功能,这是因为其在实际应用中可以利用上层应用数据,通过大数据平台分析系统的功能及附加业务的逻辑功能对其进行分析,从而为现代企业利用数据决策提供科学、准确、有效的参考依据。云计算平台技术与云计算服务技术在新时期的高速发展,使大数据平台应用技术成为可能,如果没有云计算技术作为大数据平台的技术支撑,大数
Vmware-Horizon-View搭建
Vmware vSphere ?服务器虚拟化 ?桌面虚拟化 ?客户端
目录 目录-------------------------------------------------------------------------------------------------------- 2 一、ESXi安装 ---------------------------------------------------------------------------------------------- 3 1、安装过程 ------------------------------------------------------------------------------------------- 3 2、通过浏览器访问---------------------------------------------------------------------------------- 8 3、安装vSphere Client ------------------------------------------------------------------------------ 9 4、登录ESXi主机---------------------------------------------------------------------------------- 11 二、域控制器【Domain Controller】的安装 ------------------------------------------------------- 13 1、系统 ------------------------------------------------------------------------------------------------ 13 2、其他搭建域控前的操作 ----------------------------------------------------------------------- 13 3、开始搭建 ------------------------------------------------------------------------------------------ 14 4、搭建完成后登录--------------------------------------------------------------------------------- 26 三、vCenter Server安装 --------------------------------------------------------------------------------- 28
云计算和大数据基础知识12296
精心整理 云计算与大数据基础知识 一、云计算是什么? 云计算就是统一部署的程序、统一存储并由相关程序统一管理着的数据! 云计算cloudcomputing是一种基于因特网的超级计算模式,在远程的数据中心里,成千上万台电脑和服务器连接成一片电脑云。因此,云计算甚至可以让你体验每秒超过10万亿次的运算能力,拥有这么强大的计算能力可以模拟核爆炸、预测气候变化和市场发展趋势。用户通过电脑、笔记本、手机等方式接入数据中心,按自己的需求进行运算。 二、 三、 1 );软件2 任一资源节点异常宕机,都不会导致云环境中的各类业务的中断,也不会导致用户数据的丢失。这里的资源节点可以是计算节点、存储节点和网络节点。而资源动态流转,则意味着在云计算平台下实现资源调度机制,资源可以流转到需要的地方。如在系统业务整体升高情况下,可以启动闲置资源,纳入系统中,提高整个云平台的承载能力。而在整个系统业务负载低的情况下,则可以将业务集中起来,而将其他闲置的资源转入节能模式,从而在提高部分资源利用率的情况下,达到其他资源绿色、低碳的应用效果。 3、支持异构多业务体系 在云计算平台上,可以同时运行多个不同类型的业务。异构,表示该业务不是同一的,不是已有的或事先定义好的,而应该是用户可以自己创建并定义的服务。这也是云计算与网格计算的一个重要差异。 4、支持海量信息处理 云计算,在底层,需要面对各类众多的基础软硬件资源;在上层,需要能够同时支持各类众多的异构的业务;
而具体到某一业务,往往也需要面对大量的用户。由此,云计算必然需要面对海量信息交互,需要有高效、稳定的海量数据通信/存储系统作支撑。 5、按需分配,按量计费 按需分配,是云计算平台支持资源动态流转的外部特征表现。云计算平台通过虚拟分拆技术,可以实现计算资源的同构化和可度量化,可以提供小到一台计算机,多到千台计算机的计算能力。按量计费起源于效用计算,在云计算平台实现按需分配后,按量计费也成为云计算平台向外提供服务时的有效收费形式。 四、云计算按运营模式分类 1、公有云 公有云通常指第三方提供商为用户提供的能够使用的云,公有云一般可通过Internet使用,可能是免费或成本低廉的。 烦。B 2 3 五、 六、 1、传统的IT部署架构是“烟囱式”的,或者叫做“专机专用”系统。 图2传统IT基础架构 这种部署模式主要存在的问题有以下两点: 硬件高配低用。考虑到应用系统未来3~5年的业务发展,以及业务突发的需求,为满足应用系统的性能、容量承载需求,往往在选择计算、存储和网络等硬件设备的配置时会留有一定比例的余量。但硬件资源上线后,应用系统在一定时间内的负载并不会太高,使得较高配置的硬件设备利用率不高。 整合困难。用户在实际使用中也注意到了资源利用率不高的情形,当需要上线新的应用系统时,会优先考虑部署在既有的基础架构上。但因为不同的应用系统所需的运行环境、对资源的抢占会有很大的差异,更重要的是考虑到可靠性、稳定性、运维管理问题,将新、旧应用系统整合在一套基础架构上的难度非常大,更多的用户往往选择新增与应用系统配套的计算、存储和网络等硬件设备。
大数据与云计算研究报告
(说明:此文为WORD文档,下载后可直接使用)
摘要:近年来,大数据和云计算已经成为社会各界关注的热点话题。秉承“按需服务”理念的“云计算(Cloudcomputing)”正高速发展,“数据即资源”的“大数据(bigdata)”时代已经来临[1]。大数据利用对数据处理的实时性、有效性提出了更高要求,需要根据大数据特点对传统的常规数据处理技术进行技术变革,形成适用于大数据收集、存储、管理、处理、分析、共享和可视化的技术。如何更好地管理和利用大数据已经成为普遍关注的话题。大数据的规模效应给数据存储、管理以及数据分析带来了极大的挑战,数据管理方式上的变革正在酝酿和发生。本文所提到的大数据包含着云计算,因为云计算是支撑大数据的平台。 关键词:大数据云计算数据分析数据挖掘
引言 在学术界,大数据这一概念的提出相对较早。2008年9月,《自然》杂志就推出了名为“大数据”(bigdata)的专刊。2011年5月,麦肯锡全球研究院发布了名为《大数据:创新、竞争和生产力的下一个前沿》(Bigdata:Thenextfrontierforinnovation,competition,andproductivity)的研究报告,指出大数据将成为企业的核心资产,对海量数据的有效利用将成为企业在竞争中取胜的最有力武器。2012年,联合国发布大数据政务白皮书,指出大数据可以使用极为丰富的数据资源来对社会经济进行前所未有的实时分析,帮助政府更好地响应社会和经济运行。2012年3月29日,奥巴马政府发布了《大数据研究与发展计划倡议》,宣布启动对大数据的研发计划,标志着美国把大数据提高到国家战略层面,将“大数据研究”上升为国家意志,对未来的科技与经济发展必将带来深远影响。 大数据应用正在风靡全球,大数据精准营销成为企业掌舵者的口头禅,那么大数据真的是无懈可击吗?答案显然是否定的。随着互联网和移动设备的普及,大数据已经在我们的生活中无处不在,而有关大数据与隐私的问题也日益受到关注。毫无疑问,未来可以获得的个人数据量越多,其中的信息量就越大。只要拥有了足够多的数据,我们甚至可能发现有关于一个人的未来信息。另外市场是变化无常并且不可预期的,决策者的创造性思维并不能通过数据得以体现,相反,大数据在压制创新。大数据搜集到的数据的真实性也有待检验。一个人获得的数据和事实越多,预测就越有意义,人的判断也就显得愈发
高性能计算云平台解决方案
高性能计算云平台 解决方案
目录 1概述 (3) 1.1建设背景 (3) 1.2设计范围 (3) 1.3总体设计原则 (3) 2系统平台设计 (4) 2.1项目需求 (4) 2.2设计思想 (5) 2.3云存储系统方案 (6) 2.4系统优势和特点 (6) 2.5作业调度系统方案 (8) 3系统架构 (9) 3.1cStor系统基本组成 (9) 3.2cStor系统功能描述 (10) 3.3Jobkeeper系统基本组成 (17) 4系统安全性设计 (20) 4.1安全保障体系框架 (20) 4.2云计算平台的多级信任保护 (21) 4.3基于多级信任保护的访问控制 (25) 4.4云平台安全审计 (28) 5工作机制 (31) 5.1数据写入机制 (31) 5.2数据读出机制 (32) 6关键技术 (33) 6.1负载自动均衡技术 (33) 6.2高速并发访问技术 (33) 6.3高可靠性保证技术 (33) 6.4高可用技术 (34) 6.5故障恢复技术 (34) 7接口描述 (35) 7.1POSIX通用文件系统接口访问 (35) 7.2应用程序API接口调用 (35) 8本地容错与诊断技术 (36) 8.1 cStor高可靠性 (36) 8.2 cStor数据完整性 (36) 8.3 cStor快照技术 (37) 8.4 Jopkeeper故障处理技术 (37) 9异地容灾与恢复技术 (39) 9.1cStor数据备份与恢复系统功能 (39) 9.2cStor异地文件恢复 (40)
1概述 1.1建设背景 云存储平台与作业调度为本次高性能计算总体解决方案的一部分。主要针对海量的数据的集中存储、共享、计算与挖掘,建立一套具有高可靠、可在线弹性伸缩,满足高吞吐量并发访问需求的云存储与计算平台。为数据存储和高效计算提供便捷、统一管理和高效应用的基础平台支撑。 1.2设计范围 本技术解决方案针对海量数据集中存储、共享与计算,提供从系统软硬件技术架构、原理、硬件选型、网络接入以及软件与应用之间的接口等方面的全面设计阐述。 1.3总体设计原则 针对本次工程的实际情况,充分考虑系统建设的建设发展需求,以实现系统统一管理、高效应用、平滑扩展为目标,以“先进、安全、成熟、开放、经济”为总体设计原则。 1.3.1先进性原则 在系统总体方案设计时采用业界先进的方案和技术,以确保一定时间内不落后。选择实用性强产品,模块化结构设计,既可满足当前的需要又可实现今后系统发展平滑扩展。 1.3.2安全性原则 数据是业务系统核心应用的最终保障,不但要保证整套系统能够7X24运行,而且存储系统必须有高可用性,以保证应用系统对数据的随时存取。同时配置安全的备份系统,对应用数据进行更加安全的数据保护,降低人为操作失误或病毒袭击给系统造成的数据丢失。 在进行系统设计时,充分考虑数据高可靠存储,采用高度可靠的软硬件容错设计,进行有效的安全访问控制,实现故障屏蔽、自动冗余重建等智能化安全可靠措施,提供
感统训练-感觉统合能力测量表
儿童感觉统合能力发展评估量表 各位家长: 儿童学习能力的发展主要是大脑和身体运动神经系统的良好协调,为了了解儿童学习能力存在哪些障碍,首先要了解他们脑生理和行为发展状况。请家长根据孩子的日常表现认真填写下了问卷 (一)大肌肉及平衡能力 1、特别爱玩旋转的凳椅或游乐设施而不会晕。 2、喜欢旋转或绕圈子跑而不晕不累。 3、虽然看到了仍然碰撞桌椅、旁人、柱子、门墙。 4、行动、吃饭、敲鼓、画画时双手协调不良,常忘了另一边。 5、手脚笨拙,容易跌倒,拉他时仍显得笨重。 6、俯卧地板和床上,头、颈、胸无法抬高。 7、爬上爬下,跑进跑出,不听劝阻。 8、不安地乱动,东摸西扯,不听劝阻,处罚无效。 9、喜欢惹人,捣蛋,恶作剧。 10、经常自言自语,重复别人的话,并且喜欢背诵广告语言。 11、表面左撇子,其实左右手都用,无固定使用哪只手。 12、分不清左右方向,鞋子、衣服常穿反。 13、对陌生地方的电梯或楼梯,不敢坐或动作缓慢。 14、组织力不佳,经常弄乱东西,不喜欢整理自己的环境。 (二)触觉敏感及情绪稳定 15、对亲人特别暴躁,强词夺理,到陌生环境则害怕。
16、害怕到新场合,常常不及就要求离开。 17、偏食、挑食、不吃青菜或软皮。 18、害羞不安,喜欢孤独,不爱和别人玩。 19、容易粘妈妈或固定某个人,不喜欢陌生环境,喜欢恐怖镜头。 20、看电视或听故事,容易大受感动,大叫或大笑。 21、严重怕黑,不喜欢在空房,到处要人陪。 22、早上懒床,晚上不睡,上学前常拒绝到校,放学后又不想回家。 23、容易生小病,生病后常不想上学,常常没有原因拒绝上学。 24、常吸吮手指或咬指甲,不喜欢别人帮助剪指甲。 25、换床睡不着,不能换被或睡衣,出外常担心睡眠问题。 26、独占性强,不让别人碰他的东西,常会无缘无故发脾气。 27、不喜欢同别人谈天和玩碰触游戏,视洗脸和洗澡为痛苦。 28、过分保护自己的东西,尤其讨厌别人由后面接近他。 29、怕玩沙土、水,有洁癖倾向。 30、不喜欢直接视觉接触,常需用手来表达需要。 31、对危险和疼痛反应迟钝或反应过于激烈。 32、听而不见,过分安静,表情冷漠又无故嬉笑。 33、过分安静或坚持奇怪玩法。 34、喜欢咬人,并且常咬固定的友伴,并无故碰坏东西。 35、内向、软弱、爱哭,又常会触摸生殖器。 (三)本体感及协调能力 36、穿脱衣裤、扣纽扣、拉拉链、系鞋带,动作缓慢、笨拙。
VMware Horizon View 6.0复杂安装
目录 第一章、部署环境介绍。 (3) 第二章、安装ESXI5.5 (3) 第三章、AD安装 (3) 第四章、SQL服务器安装部署. (12) 第五章、安装VCenter Server (23) 5.1 关于SQL Server数据库的准备工作 (23) 5.2 安装vCenter Single Sign-on。 (33) 5.3 安装vSphere WEB Client。 (39) 5.4 安装vCenter清单服务。 (44) 5.5 安装vCenter Server。 (49) 第六章、安装Horizon View 6.0 (58) 6.1 安装View Connection Server (58) 6.2 安装View Composer (64) 第七章、Horizon View初始配置 (70) 7.1 vSphere 初始配置 (70) 7.2 View 初始配置 (70)
第一章、部署环境介绍。 找一台PC机做管理端,安装VMware vSphere Client,通过VMware vSphere Client链接到一台ESXI主机并部署4台Windows Server 2008 R2 虚拟机。 一、安装exsi主机 二、建4台Windows 2008 R2虚拟机,AD\VC\SQL\Connection 三、部署AD域服务器 a)192.168.123.221 administrator qwer@1234 b)https://www.360docs.net/doc/758884918.html, 四、SQL服务器(不加域) a)192.168.132.223 administrator qwer@1234 b)Sa qwer@1234 五、部署VCenter服务器(加域) a)192.168.132.222 administrator qwer@1234 b)采用手动安装不采用简单安装 六、Composer服务器(加域) 七、Connection服务器(加域) a)如果环境较小可以将VCenter 、SQL、Composer安装在一台虚拟机中。SQL 也可以 使用vmware安装程序自带的Express SQL。 八、本次环境将每个组件分开。 第二章、安装ESXI5.5 第三章、AD安装 环境:Windows Server 2008 R2 设置IP为:192.168.123.221,根据情况配置网关. 设置DNS为本机IP或127.0.0.1 设置帐号密码为:Administrator qwer@1234 1.在服务器上运行dcpromo进行域控制器的安装部署“使用高级模式安装”, 下一步
云计算中的HPC高性能计算
1.背景: 云计算的优势 共享的计算设备 多租户的使用模型 可高度适配的资源分配 按需定制的HPC环境开始流行 2.挑战 虚拟化的开销 CPU, 内存, 驱动等 通信网络的区别 万兆以太网vs. Infiniband 并行IO的配置选项 设备, 文件系统和IO库的选择 3.CCI: Amazon的HPC解决方案 4. 虚拟化对HPC的影响 虚拟设备和物理设备有巨大的性能差别 虚拟机并没有引入很大的开销 对于直接分配给客户机的千兆网卡结论如此, 我们正在研究万兆网卡和IB 网卡的性能结果 5. 性能评价——结论 本地集群在通信上有巨大优势 对于CPU和内存密集型程序,CCI的性能和本地集群相似 究竟使用云还是本地集群,需要研究二者的性价比 6. I/O系统的可配置性:背景 I/O是很多高性能应用程序的性能瓶颈 应用程序的读写密集和并发度差别较大 传统高性能平台只提供通用的、统一的I/O系统 一些高性能程序开始考虑向云计算平台迁移 云计算平台可以带来I/O系统的高可配性 完全受控的虚拟机环境,自定义配置成为可能
弹性的资源申请和方便的部署方式 可选多种存储资源进行搭配 I/O系统的可配置性在于 可以在虚拟集群上选择不同的文件系统 可以利用多种底层存储设备进行组合 可以充分调节文件系统参数,专门为特定的某一个高性能应用程序进行配置 I/O系统可配置性的挑战 最优配置需要根据不同应用程序进行选择 需要平衡性能和总成本 7. I/O系统的可配置性:文件系统 网络文件系统(NFS) 使用简单,只有POSIX系统调用接口 对I/O需求较低的应用程序已经足够 存在单点瓶颈,扩展性差 并行文件系统(如PVFS) MPI-IO接口,对并行读写支持良好 可以使用更多的IO节点,扩展性好 8. I/O系统的可配置性:存储设备、 单实例临时存储设备(Ephemeral) 块设备,每节点2*800 GB, 非持久化存储 弹性块设备(EBS) 每个实例可挂载任意多块,可跨实例挂载 持久化,生命期与虚拟机实例无关 云端数据库存储服务(S3) 键值存储,面向数据库和互联网应用 9. I/O系统的可配置性:文件系统参数 10. I/O系统的可配置性:结论 针对不同的HPC应用配置I/O系统很有必要 不同HPC应用对I/O的需求不一样 性能和价格需要折中 I/O配置的挑战
VMware View 4桌面虚拟化应用(一)-----简介
VMware View 4桌面虚拟化应用(一)-----简介 一、概述 VMware View 4 是领先的桌面虚拟化解决方案,旨在将桌面以托管服务的形式交付(从平台到协议)。此解决方案使企业IT 部门可以将操作系统、应用程序和用户角色彼此独立地进行管理。VMware View 4 可以简化桌面管理、降低桌面操作成本和加强对IT 的控制,同时为最终用户提供灵活的访问,使最终用户能够通过任何网络获得卓越的桌面体验。 包含PCoIP(一种高性能显示协议)的VMware View专为通过广域网或局域网交付虚拟桌面构建,以便从任务工作人员到设计人员的所有最终用户都能获得卓越的体验。播放多媒体内容丶选择任意数量的监视器配置(包括真正的多监视器),以及无缝访问本地连接的打印机丶扫描仪或大容量存储等外设。 VMware vSphere 集成,唯一针对桌面工作负载进行了调整和优化的虚拟化平台,能够同时启动数千个桌面而不会造成任何性能下降。为桌面赋予数据中心的强大功能,并使用一个通用平台来同时管理从数据中心到云的服务器和桌面。 二、VMware View 的工作原理 当今的桌面环境将桌面组件(硬件、操作系统、应用程序、用户配置文件和数据)联系在一起,给支持和维护带来了很大困难。因此,一个层发生问题往往会导致连锁反应,最终破坏整个桌面,并使得IT 部门恢复本地存储的用户数据和设置非常困难并且成本昂贵。 VMware View 使用虚拟化切断了桌面与关联的操作系统丶应用程序和硬件之间的联系,并且使用各自桌面的个性化视图向用户提供桌面和应用程序并将它们动态地组合在一起。VMware View 通过将桌面操作系统、应用程序和用户
云计算和大数据基础知识
云计算与大数据基础知识 一、云计算是什么? 云计算就是统一部署的程序、统一存储并由相关程序统一管理着的数据! 云计算cloud computing是一种基于因特网的超级计算模式,在远程的数据中心里,成千上万台电脑和服务器连接成一片电脑云。因此,云计算甚至可以让你体验每秒超过10万亿次的运算能力,拥有这么强大的计算能力可以模拟核爆炸、预测气候变化和市场发展趋势。用户通过电脑、笔记本、手机等方式接入数据中心,按自己的需求进行运算。 云计算是一种按使用量付费的模式,这种模式提供可用的、便捷的、按需的网络访问,进入可配置的计算资源共享池(资源包括网络,服务器,存储,应用软件,服务),这些资源能够被快速提供,只需投入很少的管理工作,或与服务供应商进行很少的交互。 通俗的理解是,云计算的“云”就是存在于互联网上的服务器集群上的资源,它包括硬件资源(服务器、存储器、CPU等)和软件资源(如应用软件、集成开发环境等),所有的处理都在云计算提供商所提供的计算机群来完成。 用户可以动态申请部分资源,支持各种应用程序的运转,无需为繁琐的细节而烦恼,能够更加专注于自己的业务,有利于提高效率、降低成本和技术创新。 云计算的核心理念是资源池。 二、云计算的基本原理 云计算的基本原理是,在大量的分布式计算机集群上,对这些硬件基础设施通过虚拟化技术构建不同的资源池。如存储资源池、网络资源池、计算机资源池、数据资源池和软件资源池,对这些资源实现自动管理,部署不同的服务供用户应用,这使得企业能够将资源切换成所需要的应用,根据需求访问计算机和存储系统。 打个比方,这就好比是从古老的单台发电机模式转向了电厂集中供电的模式。它意味着计算能力也可以作为一种商品进行流通,就像煤气、水电一样,取用方便,费用低廉。最大的不同在于,它是通过互联网进行传输的。 三、云计算的特点 1、支持异构基础资源 云计算可以构建在不同的基础平台之上,即可以有效兼容各种不同种类的硬件和软件基础资源。硬件基础资源,主要包括网络环境下的三大类设备,即:计算(服务器)、存储(存储设备)和网络(交换机、路由器等设备);软件基础资源,则包括单机操作系统、中间件、数据库等。 2、支持资源动态扩展 支持资源动态伸缩,实现基础资源的网络冗余,意味着添加、删除、修改云计算环境的任一资源节点,或者任一资源节点异常宕机,都不会导致云环境中的各类业务的中断,也不会导致用户数据的丢失。这里
基于大数据的云计算支撑平台IOP
基于大数据的云计算支撑平台 IOP 浪潮IOP(Inspur Open Platform)是一个云计算架构的开放平台,采用大数据处理、社交网络、情景感知、服务化架构等关键技术和理念开放的应用支撑和资源整合平台,通过共享平台强化企业信息资源的有机整合和高效利用,构建开放、协同、智能、互联、弹性可扩展的IT基础软件环境,使客户有机会利用新技术的解决传统IT系统规划和建设存在的诸多难题,实现信息化从传统架构向云计算架构的平滑转型。 IOP平台采用“平台+应用”的总体思路,采用支持分布式、高并发和大数据处理的云计算架构设计。开放的架构为各种应用提供分布式计算、分布式存储、大数据分析、统一用户认证、统一消息引擎、统一资源管理等基础支撑服务能力,通过IOP可以整合来自内外部的各类信息资源,实现信息资源共享,开放业务能力和数据资源,创新应用开发和IT服务模式。 IOP平台的应用领域包括:基于云计算的信息化应用支撑、大数据处理和资源整合以及面向公众的互联网服务和电子商务。
IOP具备四个方面的关键特性,以满足之上承载应用的稳定运行。 1、满足百万级以上用户海量数据快速存取,并能够支持水平扩展, 基于大数据可弹性扩展的技术架构。 2、利用Open API整合与共享信息资源,对基础共性服务统一构建, 基于开放平台为多应用提供公共服务。 3、制定应用开发统一的标准规范,采用应用商店模式搭建应用生 态环境,促进应用创新。。 4、IOP产品研发始终坚持安全可控的技术路线,所有底层架构和 组件均为自主研发。 目前平台研发工作已经有了初步的成果,并在浪潮实施的包括智慧城市、警务云等一些重大项目中进行应用,浪潮IOP平台的应用极 大的提高了行业IT整体的计算能力、整合能力和创新能力,下一步将
云计算大数据试题
云计算大数据试题 一、单选题(30%) 1、我公司大数据对外服务品牌是下面哪一项(A) A.智慧洞察 B.精确营销 C.智慧数据 D.和数据 答案:A 2、目前中国移动已经开展的大数据对外服务不包括下面的哪一项(D) A.旅游景区客源分析 B.交通OD系统 C.商铺选址 D.互联网广告营销 答案:D 3、大数据金融征信是对外服务一个重要的领域,下面说法错误的是(C) A.要严格保护用户信息安全 B.数据结果脱敏加工 C.可以输出用户的位置信息 D.必须获得用户授权 答案:C 4、大数据技术的战略意义不在于掌握庞大的数据信息,而在于对这些含有意义的数据进行(B)。 A. 数据信息 B. 专业化处理 C.速度处理 D. 内容处理 答案:B 5、与运营商数据相比,互联网数据有以下几点局限性,除了( D )。 A. 数据局部性 B. 数据封闭性 C. 数据割裂性 D.数据全面性 答案:D 6、推荐系统为客户推荐商品,自动完成个性化选择商品的过程,满足客户的个性化需求,推荐基于网站最热卖商品、客户所处城市、( D ),推测客户将来可能的购买行为。 A.客户的朋友 B.客户的个人信息 C.客户的兴趣爱好 D. 客户过去的购买行为和购买记录 答案:D 7、社交网络产生了海量用户以及实时和完整的数据,同时社交网络也记录了用户群体的( C ),通过深入挖掘这些数据来了解用户,然后将这些分析后的数据信息推给需要的品牌商家或是微博营销公司。 A.地址 B.行为 C.情绪 D.来源 答案:C 8、在云生态环境中,用户需求相当于( D ),云数据中心相当于( C ),云服务相当于( B )。 A. 降水 B. 水滴 C. 水库 D. 阳光 答案:D\C\B
高性能计算与云计算—教学大纲
《高性能计算与云计算》教学大纲 总学时:48 理论课学时:32实验课学时:16 一、课程的性质 《高性能计算与云计算》是计算机专业的专业领域课程。高性能计算技术是计算机技术发展中的一项重要技术。通过该课程的学习,学生应该学习和掌握高性能计算的基础理论知识和实践经验,并为后续课程打下坚实基础。 二、课程的目的与教学基本要求 本课程以高性能计算和云计算为主题,分为三大部分,第一部分是高性能计算的基础知识,第二部分是云计算的概念及核心技术,第三部分是高性能计算与云计算的软件支持-并行程序的设计原理与方法。主要课程内容包括高性能计算机的系统结构模型,对称多处理机(SMP)、大规模并行处理机(MPP)、集群系统(Cluster)和并行计算的性能评测并行算法的一般设计策略、基本设计技术和一般设计过程;云计算的分布式大规模数据处理和云存储技术;共享存储编程、分布存储编程和Map/Reduce编程等面向高性能计算与云计算应用的并行程序设计环境与工具。 课程强调融合高性能计算机结构、云计算技术、并行算法和并行编程为一体,力图反映高性能计算和云计算领域的最新成就和发展趋势。 学生除完成基本理论课程学习外,课程将通过在高性能计算机系统上的实践,学习和掌握高性能计算和云计算的基础知识。 三、课程适用专业 计算机科学与技术、软件技术、信息安全、网络工程 四、课程的教学内容、要求与学时分配 1.理论教学部分: 按各章节列出主要内容,注明课程教学的难点和重点,对学生掌握知识的要求,以及学时的分配。 主要章节及其学时分配: 第一章绪论(3学时) 重点:高性能计算和云计算的概念、基础架构、现状及发展、应用前景 难点:云计算的架构、高性能计算技术的发展以及和云计算技术的衔接 要求:了解基本概念,基础架构,以及相关的技术和应用
VMware-Horizon-View-6.0环境部署详细教程
目录 第一章、部署环境介绍。 (2) 第二章、安装ESXI5.5 (2) 第三章、AD安装 (2) 第四章、SQL服务器安装部署. (11) 第五章、安装VCenter Server (22) 5.1 关于SQL Server数据库的准备工作 (22) 5.2 安装vCenter Single Sign-on。 (32) 5.3 安装vSphere WEB Client。 (38) 5.4 安装vCenter清单服务。 (43) 5.5 安装vCenter Server。 (48) 第六章、安装Horizon View 6.0 (57) 6.1 安装View Connection Server (57) 6.2 安装View Composer (63) 第七章、Horizon View初始配置 (69) 7.1 vSphere 初始配置 (69) 7.2 View 初始配置 (69)
第一章、部署环境介绍。 找一台PC机做管理端,安装VMware vSphere Client,通过VMware vSphere Client链接到一台ESXI主机并部署4台Windows Server 2008 R2 虚拟机。 一、安装exsi主机 二、建4台Windows 2008 R2虚拟机,AD\VC\SQL\Connection 三、部署AD域服务器 a)192.168.123.221 administrator qwer@1234 b)https://www.360docs.net/doc/758884918.html, 四、SQL服务器(不加域) a)192.168.132.223 administrator qwer@1234 b)Sa qwer@1234 五、部署VCenter服务器(加域) a)192.168.132.222 administrator qwer@1234 b)采用手动安装不采用简单安装 六、Composer服务器(加域) 七、Connection服务器(加域) a)如果环境较小可以将VCenter 、SQL、Composer安装在一台虚拟机中。SQL 也可以 使用vmware安装程序自带的Express SQL。 八、本次环境将每个组件分开。 第二章、安装ESXI5.5 第三章、AD安装 环境:Windows Server 2008 R2 设置IP为:192.168.123.221,根据情况配置网关. 设置DNS为本机IP或127.0.0.1 设置帐号密码为:Administrator qwer@1234 1.在服务器上运行dcpromo进行域控制器的安装部署“使用高级模式安装”, 下一步
vmware view功能版本对比
目前销售的Horizon 6(包含View)共有三个版本:VMware Horizon?View?Standard 版;VMware Horizon?Advanced 版; VMware Horizon?Enterprise 版。 免费下载试用版在线购买查找合作伙伴 Horizon 6(包含View)版本对比
功能特性 借助Horizon(包含View),组织可以通过单一平台提供虚拟化或远程桌面及应用,并为终端用户提供支持,使之能够在一个地方访问自己的所有桌面和应用。 通过单一平台交付桌面和应用 通过单一平台交付虚拟或远程桌面及应用,从而简化管理、轻松为终端用户授权并以终端用户需要的速度快速交付他们需要的Windows 资源。 Horizon 6 现在支持: 通过单一平台交付托管应用及运行Microsoft Windows 远程桌面服务的桌面。
通过统一工作空间提供出色用户体验 借助Horizon 6,IT 部门可以通过一个统一的工作空间及Blast Performance 向终端用户交付桌面和应用(包括托管的RDS 应用、打包的ThinApp、SaaS应用甚至来自Citrix 的虚拟化应用),使终端用户可以在任何位置使用任何设备、介质和连接获得一致的出色体验。 ?XenApp 5.0 及以上版本、适用于Windows Server 2008 及以上版本的Microsoft 托管RDS 应用和桌面、SaaS应用以及使用ThinApp 5.0打包的应用 Blast Performance 包括: ?Blast Adaptive UX - 优化使用HTML 浏览器或我们的专用桌面协议PCoIP 通过WAN 和LAN 进行的访问 ?Blast Multi-media - 可实现丰富用户体验的高性能多媒体流式传输 ?Blast 3D - 可达到工作站级性能的丰富虚拟化图形 ?Blast Live Communications - 经过全面优化的统一通信与实时音频和视频支持。Horizon 6 现在可支持在Windows 8 上运行Microsoft Lync ?Blast Unity Touch - 在各种设备上向用户提供与具体环境相关的直观体验,便于在移动设备上运行Windows ?Blast Local Access - 访问本地设备、USB 和外围设备
感觉功能的评价标准
感觉功能的评价标准 感觉功能的检查方法如下: 1.触觉:①vonFrey试验:Semmes-Weins-tein尼龙单丝,根据不同号的尼龙单丝对皮肤的压力不同,测定皮肤触觉敏感程度。②两点鉴别能力(twopointdiscri-mination,2PD):Weber/Moberg2PD测定法是测定静止两点鉴别觉(s2PD),Weber/Dellon2PD测定法是测定移动两点鉴别觉(m2PD)。③振动觉检查:使用音叉测知。④砂纸试验:使用不同粗糙程度的砂纸,测定手的移动触觉。⑤拾物试验:比较闭眼和睁眼时拾起数件小物品的能力。⑥精确感觉抓握试验(precisionsensorygriptest):闭眼时将螺母从螺丝上旋下能力的测定。⑦五物试验:使用5种小而硬的物件的边缘刺激指尖,测定敏感程度。 2.痛觉:用针尖和针头刺皮肤而测知。 3.Tinel征。 4.温、冷觉。 5.位置觉。 常用感觉功能评价方法如下: 1.Werner和Omer的vonFrey评价法(1980)(见下表)。 2.Weber和Moberg的2PD试验的评判标准(见下表)。 3.Moberg的2PD评价(仅指正中神经、尺神经支配的绝对供应区): S4:良好:<12mm。 尚可:12~15mm。
S+3:不良:15~20mm,粗大把持,防御反射(+)。 S3:差:>20mm,防御反射(+)。 S2:感觉过敏、错感觉、把持障碍。 S1:轻触觉、针刺觉(+)。 S0:感觉丧失。 4.Highet评价标准: S0:绝对供应区内感觉丧失。 S1:绝对供应区内深感觉恢复。 S2:绝对供应区内浅表痛觉和触觉一定程度的恢复。 S3:绝对供应区内浅表痛觉和触觉恢复,“过反应”消失。 S4:在S3的基础上有2PD的恢复。 5.津山判定标准(1970): 优:绝对供应区内浅表痛觉和触觉恢复,无“过反应”,有定位能力,两点鉴别能力存在。 良:绝对供应区内浅表痛觉和触觉恢复,无“过反应”,有定位能力,但无两点鉴别能力,亦不能辨别粗滑。 可:绝对支配区内浅表痛觉和触觉在一定程度上恢复,“过反应”残存。 差:绝对支配区内感觉丧失或仅深痛觉恢复。 6.Sundland感觉功能评价法(1978): (1)针刺感(pinprick): P.0──皮肤感觉消失。 P.1──感觉到皮肤上有物接触,但不能区别是针尖还是针头在触及皮肤,感觉能或不能定位。 P.2──能区分是针尖还是针头触及皮肤,针尖刺皮肤引起:①钝痛感。②不愉快感,有明显的放射和假性牵涉(falsereference)。 P.3──钝刺感或锐刺感,伴放射和假性牵涉,除知道痛在手部或手指、腿部或足外,不能具体定位。 P.4──锐感觉存在,伴或不伴有刺感或刺痛,无或有很轻的放射,能定位到2.0cm内。 P.5──对针刺有正常感觉,定位精确。 (2)轻触觉(lighttouch): L.0──对轻触觉不能意识到。 L.1──意识到皮肤上有物接触。 L.2──轻触引起放射性麻感,对刺激点不能定位。 L.3──轻触觉仅能被察觉到,除知道在手部、掌面、手背或手指,下肢的腿和足外,不能具体定位。 L.4──对轻触能意识到,但敏锐度较弱,能定位到2.0cm内。 L.5──对轻触有正常感觉。 (3)两点辨别觉: D.0──无两点辨别能力。 D.1──2PD部分存在。 D.2──2PD正常。 (4)温度觉: T°0──无温度觉。