傅里叶,小波分析,CS简介及其应用(英文版)

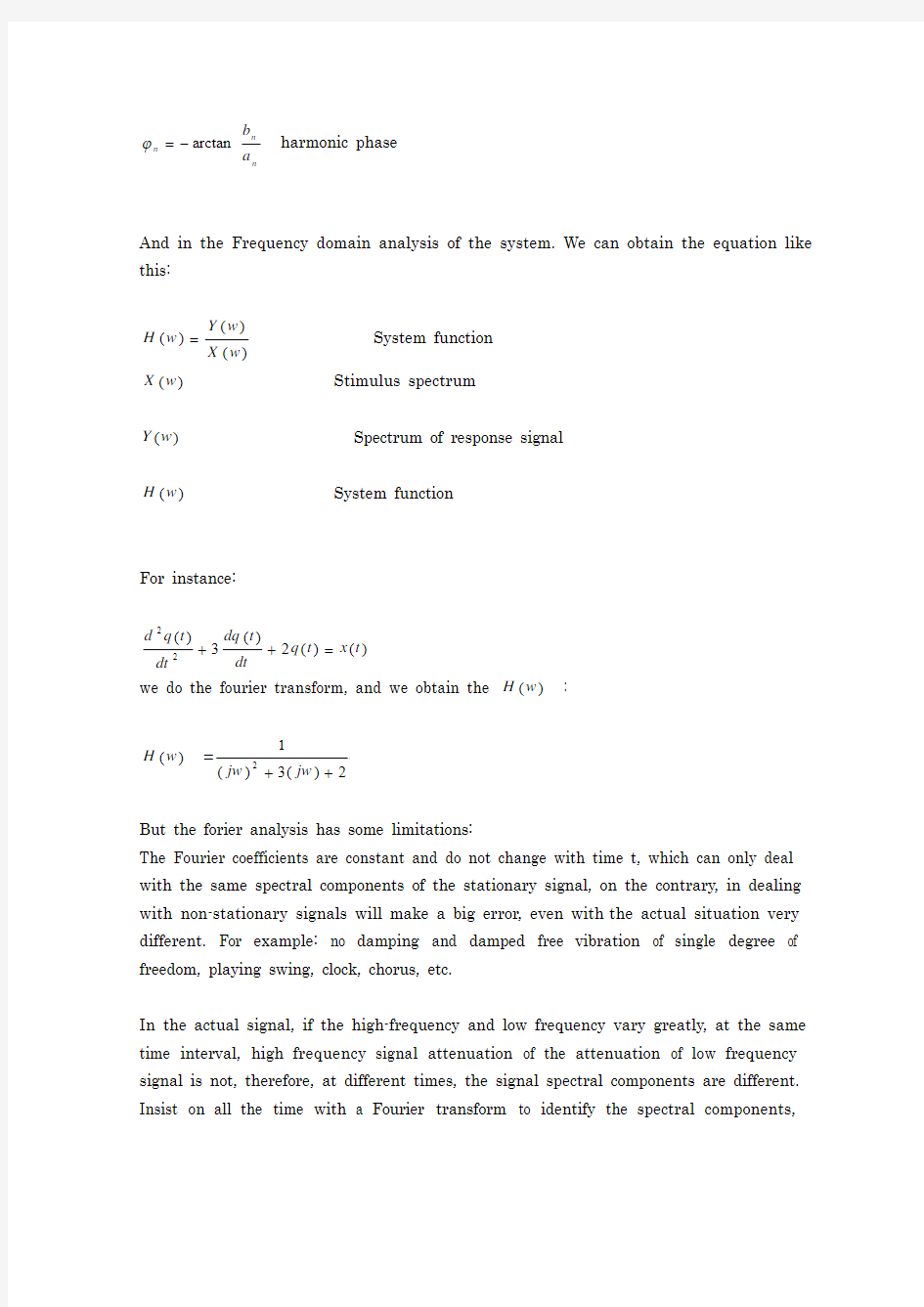
1) Fourier Analysis
Fourier analysis, also known as harmonic analysis, is a branch of mathematics field. The Fourier analysis can show how a function or a signal is expressed as the superposition of the basic waveform.
Fourier analysis is used to analyze non-sinusoidal periodic signals of a mathematical method.
In the field of signal analysis, we can use Fourier series representation of the signal, to analyze the characteristics of periodic signals in the frequency domain, and to establish the signal spectrum.
If the periodic signal f 1 (t) satisfy the Dirichlet conditions, we can make x (t) expand into triangular form of Fourier series.
The Dirichlet conditions are:
f(x) must have a finite number of extrema in any given interval f(x) must have a finite number of discontinuities in any given interval f(x) must be absolutely integrable over a period. f(x) must be bounded
direct current component
T
πω21=
fundamental frequency
0c a n = direct current range
2
2n n n b a c += harmonic amplitude
)
sin cos ()(11101t n b t n a a t f n n n ωω++=∑∞
=
?+=10
).(11
0T
t t dt t f T a ∑
∞
=++
=1
101)
cos()(n n n t n c c t f ??
n
n n a b arctan
-=? harmonic phase
And in the Frequency domain analysis of the system. We can obtain the equation like this: )
()()(w X w Y w H =
System function
)(w X Stimulus spectrum
)(w Y Spectrum of response signal
)(w H System function
For instance: )()(2)(3
)(22
t x t q dt
t dq dt
t q d =++
we do the fourier transform, and we obtain the )(w H :
)(w H =
2
)(3)(1
2
++jw jw
But the forier analysis has some limitations:
The Fourier coefficients are constant and do not change with time t, which can only deal with the same spectral components of the stationary signal, on the contrary, in dealing with non-stationary signals will make a big error, even with the actual situation very different. For example: no damping and damped free vibration of single degree of freedom, playing swing, clock, chorus, etc.
In the actual signal, if the high-frequency and low frequency vary greatly, at the same time interval, high frequency signal attenuation of the attenuation of low frequency signal is not, therefore, at different times, the signal spectral components are different. Insist on all the time with a Fourier transform to identify the spectral components,
deliberately changing the amplitude of the change with frequency to compensate for not only high-frequency Fourier coefficients of error, low-frequency Fourier coefficients are significant errors, including the frequency obtained Of course there are errors.
Seeking full-time Fourier coefficient is the weighted average domain. So it means, mutation information is the local average out, the local mutation is difficult to reflect the role of information (like eating the same big pot, egalitarianism). Very different signals, such as square wave, triangle wave, sine wave, can get the same frequency, so the processing, to capture transient signals such as the fault signal, the sensitivity is poor. Treatment should be used to capture transient signals reflect the transformation of local information.
2)Wavelet Analysis
Wavelet transforms are broadly divided into three classes: continuous, discrete and multiresolution-based.
Continuous wavelet transforms (continuous shift and scale parameters):
The subspace of scale a or frequency band is generated by the functions (sometimes called child wavelets):
,
where a is positive and defines the scale and b is any real number and defines the shift.
The pair (a,b) defines a point in the right half-plane .
The projection of a function x onto the subspace of scale a then has the form:
with wavelet coefficients:
.
For the analysis of the signal x, one can assemble the wavelet coefficients into a scalogram of the signal.
Discrete wavelet transforms (discrete shift and scale parameters):
∑∑
∞
-∞=∞
-∞
=><=
j k k
j k
j t f t f )(~,)(,,ψψ =
∑∑∞
-∞=∞
-∞
=j k k
j k
j t d
)(,,ψ
)2(,k t j k
j -=ψψ
Among them,
)(t ψ is the wavelet function, k
j d
, is the wavelet coefficients, and
k
j d
, =><
k
j f ,~,ψ
Wavelet series is a double sum index of wavelet coefficients not only the frequency of the target, but also the time of the index. In other words, not only as the Fourier coefficients of the wavelet coefficients, as is varies with frequency, and for the same frequency index at different times, the wavelet coefficients are different.
Because the wavelet function with compact support of nature, that is zero outside a certain range. So that the level of demand at different times of the frequency of the wavelet coefficients, only use local information near the time.
An important application of wavelet analysis is using the two-dimensional wavelet analysis to do the image compression.
It is characterized by high compression ratio and high compression speed. And it is can maintain essentially the same characteristics after the compression. Moreover, in the transmission process can resist interference.
Here is a two-dimensional image signal, using two-dimensional wavelet analysis to do the compression of the image. After the image is decomposed by the wavelet analysis, it can obtain a series of sub-images with different resolution. Different resolutions of the sub-images correspond to different frequencies.
image
%imput the image figure,the image file is wbarb.mat
load wbarb;
%show the figure
subplot(221);image(X);colormap(map)
title('Original image');
axis square
disp('The size of the image before compression X:');
whos('X')
%use the wavelet bior3.7 to do the second layer wavelet decomposition
[c,s]=wavedec2(X,2,'bior3.7');
%extract coefficients of the first layer with low-frequency and high
%frequency coefficients from the structure of wavelet decomposition.
cal=appcoef2(c,s,'bior3.7',1);
ch1=detcoef2('h',c,s,1);
cv1=detcoef2('v',c,s,1);
cd1=detcoef2('d',c,s,1);
%reconstruction of the various frequencies
a1=wrcoef2('a',c,s,'bior3.7',1);
h1=wrcoef2('h',c,s,'bior3.7',1);
v1=wrcoef2('v',c,s,'bior3.7',1);
d1=wrcoef2('d',c,s,'bior3.7',1);
c1=[a1,h1;v1,d1];
%show the various frequencies after the decomposition.
subplot(222);image(c1);
axis square
title('Decomposition of low and high frequency information');
%do the compression of the image.
%keep the first layer of low-frequency information of wavelet decomposition
%and do the compression of the figure.
%the first layer of low-frequency information is the cal,and display the
%first layer of low-frequency information.
%first,do the quantization coding for the first layer i nformation.
cal=appcoef2(c,s,'bior3.7',1);
cal=wcodemat(cal,440,'mat',0);
%change the figure height
cal=0.5*cal;
subplot(223);image(cal);colormap(map);
axis square
title('The first compression');
disp('The size of the first compression: ');
whos('cal')
%reserve the second layer of low-frequency information of wavelet
%decomposition,do the compression of the image,and the compression ratio %is more higher.
%the second layer of low-frequency information is ca2,display the second %layer of low-frequency information.
ca2=appcoef2(c,s,'bior3.7',2);
%do the quantization coding for the second layer.
ca2=wcodemat(ca2,440,'mat',0);
%change the figure height
ca2=0.25*ca2;
subplot(224);image(ca2);colormap(map);
axis square
title('The second compression');
disp('The size of the second compression: ');
whos('ca2')
The result is:
The size of the image before compression X:
Name Size Bytes Class
X 256x256 524288 double array Grand total is 65536 elements using 524288 bytes The size of the first compression:
Name Size Bytes Class
cal 135x135 145800 double array Grand total is 18225 elements using 145800 bytes The size of the second compression:
Name Size Bytes Class
ca2 75x75 45000 double array
Grand total is 5625 elements using 45000 bytes
Original image
50100150200250
Decomposition of low and high frequency information
100200300400500
The first compression
20406080100120
The second compression 10203040506070
3)Compressed Sensing
Compressed sensing, also known as compressive sensing, compressive sampling and sparse sampling, is a technique for finding sparse solutions to underdetermined linear systems. In engineering, it is the process of acquiring and reconstructing a signal utilizing the prior knowledge that it is sparse or compressible.
The main idea behind compressed sensing is to exploit that there is some structure and redundancy in the majority of interesting signals—they are not pure noise. In particular, most signals are sparse, that is, they contain many coefficients close to or equal to zero, when represented in some domain. (This is the same insight used in many forms of lossy compression.)
Compressed sensing typically starts with taking a weighted linear combination of samples also called compressive measurements in a basis different from the basis in which the signal is known to be sparse. The results found by David Donoho, Emmanuel Candès, Justin Romberg and Terence Tao showed that the number of these compressive measurements can be small and still contain all the useful information. Therefore, the task of converting the image back into the intended domain involves solving an underdetermined matrix equation since the number of compressive measurements taken is smaller than the number of pixels in the full image. However, adding the constraint that the initial signal is sparse enables one to solve this underdetermined system of linear equations.
The classical solution to such problems is to minimize the L2 norm—that is, minimize the amount of energy in the system. This is usually simple mathematically (involving only a matrix multiplication by the pseudo-inverse of the basis sampled in). However, this leads to poor results for most practical applications, as the unknown coefficients seldom have zero energy.
In order to enforce the sparsity constraint when solving for the underdetermined system of linear equations, one should be minimizing the L0 norm, or equivalently maximizing the number of zero coefficients in the new basis. However, searching a solution with this constraint is NP-hard (it contains the subset-sum problem), and so is computationally infeasible for all but the tiniest data sets. Following Tao et al., where it was shown that the L1 norm is equivalent the L0 norm, leads one to solve an easier problem. Finding
the candidate with the smallest L1 norm can be expressed relatively easily as a linear program, for which efficient solution methods already exist. These solution methods have been refined over the past few years yielding enormous gain.
A more technical insight on the different techniques employed in sampling and decoding signals with compressive sensing can be gained in.
The field of compressive sensing has direct connections to Underdetermined Linear Systems, Group Testing, Heavy-hitters, Sparse Coding, Multiplexing, Sparse Sampling, Finite Rate of Innovation. Imaging techniques having a strong affinity with compressive sensing include Coded aperture and Computational Photography. As a generic rule of thumb, any two stage techniques or indirect imaging involving the use of a computer for the reconstruction of a signal or an image is bound to find a use for compressive sensing techniques.
Starting with the famous single-pixel camera from Rice University an up-to-date list of the most recent implementations of compressive sensing in hardware at different technology readiness level is listed in. Some hardware implementation like the one used in MRI or Compressed Genotyping do not require an actual physical change whereas other hardware require substantial re-engineering to perform this new type of sampling. Similarly, a number of hardware implementation already existed before 2004 but while they were acquiring signals in a compressed manner, they generally did not use compressive sensing reconstruction techniques to reconstruct the original signal. The result of these reconstruction were suboptimal and have been greatly enhanced thanks to compressive sensing. Hence there is a large disparity of implementation of compressive sensing in different areas of engineering and science.
References:
1, Hayes, Brian, The Best Bits, American Scientist, July 2009
2, Donoho, D. L., Compressed Sensing, IEEE Transactions on Information Theory, V. 52(4), 1289–1306, 2006 [1]
3, Candès, E.J., & Wakin, M.B., An Introduction To Compressive Sampling, IEEE Signal Processing Magazine, V.21, March 2008 [2]
Etc.
傅里叶(Fourier)级数的指数形式与傅里叶变换
傅里叶(Fourier )级数的指数形式与傅里叶变换 专题摘要:根据欧拉(Euler )公式,将傅里叶级数三角表示转化为指数表示,进而得到傅里叶积分定理,在此基础上给出傅里叶变换的定义和数学表达式。 在通信与信息系统、交通信息与控制工程、信号与信息处理等学科中,都需要对各种信号与系统进行分析。通过对描述实际对象数学模型的数学分析、求解,对所得结果给以物理解释、赋予其物理意义,是解决实际问题的关键。这种数学分析方法主要针对确定性信号的时域和频域分析,线性时不变系统的描述以及信号通过线性时不变系统的时域分析与变换域分析。所有这些分析方法都离不开傅里叶变换、拉普拉斯变换和离散时间系统的z 变换。而傅里叶变换的理论基础是傅里叶积分定理。傅里叶积分定理的数学表达式就是傅里叶级数的指数形式。 不但傅里叶变换依赖于傅里叶级数,就是纯数学分支的调和分析也来源于函数的傅里叶级数。因此,傅里叶级数无论在理论研究还是在实际应用中都占有非常重要的地位。我们承认满足狄里克莱(Dirichlet )条件下傅里叶级数的收敛性结果,不去讨论和深究傅里叶展式的唯一性问题。 傅里叶级数的指数形式 一个以T 为周期的函数)(t f ,在]2 ,2[T T 上满足狄里克莱条件:1o
)(t f 连续或只有有限个第一类间断点;2o 只有有限个极值点。那么)(t f 在]2 ,2[T T - 上就可以展成傅里叶级数。在连续点处 ∑∞ =++=1 )sin cos (2)(n n n t n b t n a a t f ωω, (1) 其中 T πω2= , ),2,1,0(,cos )(2 22Λ==?-n dt t n t f T a T T n ω, (2) ),3,2,1(,sin )(2 22 Λ==?-n dt t n t f T b T T n ω, (3) 根据欧拉(Euler )公式:θθθsin cos j e j +=,(1)式化为 ∑∞=--?? ????-+++=10222)(n t jn t jn n t jn t jn n j e e b e e a a t f ωωωω ∑∞=-?? ? ???++-+=10222n t jn n n t jn n n e jb a e jb a a ωω, (4) 若令 dt t f T c T T ?-=22 0)(1 Λ,3,2,1,)(1 ]sin )[cos (1 sin )(1cos )(1222 2222 22==-=-=-=????-----n dt e t f T dt t n j t n t f T dt t n t f T j dt t n t f T jb a c T T t jn T T T T T T n n n ωωωωω Λ,3,2,1,)(1 22 ==?--n dt e t f T c T T t jn n ω 综合n n c c c -,,0,可合并成一个式子 Λ,2,1,0,)(1 22 ±±==?--n dt e t f T c T T t jn n ω, (5)
傅里叶变换在信号处理中的应用
傅里叶变换在信号处理中的应用 傅里叶变换在物理学、电子类学科、数论、组合数学、信号处理、
概率论、统计学、密码学、声学、光学、海洋学、结构动力学等领域都有着广泛的应用(例如在信号处理中,傅里叶变换的典型用途是将信号分解成幅值谱——显示与频率对应的幅值大小)。 尽管最初傅立叶分析是作为热过程的解析分析的工具,但是其思想方法仍然具有典型的还原论和分析主义的特征。"任意"的函数通过一定的分解,都能够表示为正弦函数的线性组合的形式,而正弦函数在物理上是被充分研究而相对简单的函数类,这一想法跟化学上的原子论想法何其相似!奇妙的是,现代数学发现傅立叶变换具有非常好的性质,使得它如此的好用和有用,让人不得不感叹造物的神奇: 1.傅立叶变换是线性算子,若赋予适当的范数,它还是酉算子; 2.傅立叶变换的逆变换容易求出,而且形式与正变换非常类似; 3.正弦基函数是微分运算的本征函数,从而使得线性微分方程的求解可以转化为常系数的代数方程的求解.在线性时不变的物理系统内,频率是个不变的性质,从而系统对于复杂激励的响应可以通过组合其对不同频率正弦信号的响应来获取; 4.著名的卷积定理指出:傅立叶变换可以化复杂的卷积运算为简单的乘积运算,从而提供了计算卷积的一种简单手段; 5.离散形式的傅立叶变换可以利用数字计算机快速的算出(其算法称为快速傅立叶变换算法(FFT)). 正是由于上述的良好性质,傅里叶变换在物理学、数论、组合数学、信号处理、概率、统计、密码学、声学、光学等领域都有着广泛的应用。
有関傅立叶变换的FPGA实现 傅立叶变换是数字信号处理中的基本操作,广泛应用于表述及分析离散时域信号领域。但由于其运算量与变换点数N的平方成正比关系,因此,在N较大时,直接应用DFT算法进行谱变换是不切合实际的。然而,快速傅立叶变换技术的出现使情况发生了根本性的变化。本文主要描述了采用FPGA来实现2k/4k/8k点FFT的设计方法。 离散傅里叶变换的应用 DFT在诸多多领域中有着重要应用,下面仅是颉取的几个例子。需要指出的是,所有DFT的实际应用都依赖于计算离散傅里叶变换及其逆变换的快速算法,即快速傅里叶变换(快速傅里叶变换(即FFT)是计算离散傅里叶变换及其逆变换的快速算法。)。 1.频谱分析 DFT是连续傅里叶变换的近似。因此可以对连续信号x(t)均匀采样并截断以得到有限长的离散序列,对这一序列作离散傅里叶变换,可以分析连续信号x(t)频谱的性质。前面还提到DFT应用于频谱分析需要注意的两个问题:即采样可能导致信号混叠和截断信号引起的频谱泄漏。可以通过选择适当的采样频率(见奈奎斯特频率)消减混叠。选择适当的序列长度并加窗可以抑制频谱泄漏。 2.数据压缩 由于人类感官的分辨能力存在极限,因此很多有损压缩算法利用
近代数学 小波 简答题+答案
1什么是小波函数?(或小波函数满足什么条件?) 答:设)()(2R L t ∈?,且其Fourier 变换)(ω? 满足可允许性(admissibility )条件 +∞∞ +∞-ωω?d w |||)(|2 ,则称)(t ?为小波函数。 2 Fourier 变换的不足? Fourier 分析的不足,主要表现在以下两点: 1) Fourier 分析不能刻画时域信号的局部特性;(只知道信号所含有的频率信息,但不能知道各种不同频率信息在什么时候/位置出现) 2) Fourier 分析对非平稳信号的处理效果不好。(如音乐、语言、地震、电脉冲等) 3 什么是加窗Fourier 变换? 用一个时间函数g(t)做窗口函数,该时间函数在有限区间外恒等于零,或很快趋近与零。 用g(t –τ)与待分析函数f(t)相乘,然后对乘积进行Fourier 变换,乘积作用相当与在 t =τ处开了个“窗口”。即 ),(τωf G = dt e t g t f R t i ? --ωτ)()( 其反演公式为: ττωτωπωd G t g e d t f f R R t i ),()(21)(-= ?? ),(τωf G ),(+∞<<-∞+∞<<-∞τω确实包含了f(t)的全部信息。 4.什么是分数傅里叶变换? 分数傅里叶变换是傅里叶变换的广义化,傅里叶变换通常指变换整数次,而分数傅里叶变换的变换次数不一定是整数,而是分数,其定义式为 ,当a=1时,分数傅里叶变换就变成了傅里叶变换。 一、写出离散小波、二进小波的表达式 答:(1)离散小波:)2(2 2 ,k t j j k j -=--ψψ (2)二进小波:))2(2(2)(2 ,2τψψτj j j t t j -=-- 三、二进小波满足什么样的条件时,它的小波变换及其逆变换是存在的? 设小波函数)(2R L t ∈)(ψ,若存在两个常数A,B,满足0<+∞<≤B A ,使得 B A j Z ≤∑≤∈2 j ) 2(ωψ 成立,则称)(,2t j τψ小波是)(2R L 上的二进小波,称上式为二进小波的稳定条件,
小波分析考试题(附答案)
《小波分析》试题 适用范围:硕士研究生 时 间:2013年6月 一、名词解释(30分) 1、线性空间与线性子空间 解释:线性空间是一个在标量域(实或复)F 上的非空矢量集合V ;设V1是数域K 上的线性空间V 的一个非空子集合,且对V 已有的线性运算满足以下条件 (1) 如果x 、y V1,则x +y V1; (2) 如果x V1,k K ,则kx V1, 则称V1是V 的一个线∈∈∈∈∈性子空间或子空间。2、基与坐标 解释:在 n 维线性空间 V 中,n 个线性无关的向量,称为 V 的一组n 21...εεε,,,基;设是中任一向量,于是 线性相关,因此可以被基αn 21...εεε,,,线性表出:,其中系数 αεεε,,,,n 21...n 21...εεε,,,n 2111an ...a a εεεα+++=是被向量和基唯一确定的,这组数就称为在基下的坐标,an ...a a 11,,,αn 21...εεε,,,记为 () 。an ...a a 11,,,3、内积 解释:内积也称为点积、点乘、数量积、标量积。,()T n x x x x ,...,,21= ,令,称为x 与y 的内积。 ()T n y y y y ,...,,21=[]n n y x y x y x y x +++=...,2211[]y x ,4、希尔伯特空间 解释:线性 完备的内积空间称为Hilbert 空间。线性(linearity ):对任意 f , g ∈H ,a ,b ∈R ,a*f+b*g 仍然∈H 。完备(completeness ):空间中的任何柯西序列都收敛在该空间之内。内积(inner product ):
傅里叶级数及其应用.
毕业论文 题目:傅里叶级数及其应用作者:姜广辉 指导教师:李博 职称:讲师 院系:理学院数学系 专业:数学与应用数学 班级:10级1班 日期: 2014年5月
傅里叶级数及其应用 摘要:傅里叶级数是数学分析中的一个重要概念,具有较好的几何和代数性质,伴随着科技的进步与发展,涉及了许多数学命题的讨论和应用,傅里叶级数的相关知识已经成为从事科学研究和工程设计等科技人员必备的数学基础.通过对傅里叶、拉格朗日、狄利克雷、黎曼等人在傅里叶级数方面的贡献,介绍了傅里叶级数起源和发展历程.同时文章以在图案设计和铁路客运量预测上的应用说明了傅里叶级数的价值.在图案设计设计方面,运用MATLAB软件,编写傅里叶级数的程序语言,通过自定义函数、编写画图函数程序、对图形多余部分处理、图形线条加粗等步骤,进而得到傅里叶级数的图形.通过对最基本的傅里叶级数的图形的组合、排列可以构成丰富的图案.在铁路客运量预测方面,基于傅里叶级数预测模型,以我国2004—2009年铁路客运量为数据基础,通过将时间序列划分为趋势性和季节性部分,分别采用最小二乘法和傅里叶级数预测法对两者进行拟合,应用MATLAB软件,求出预测模型,并进行预测.通过对预测结果的误差分析,表明:采用傅里叶级数预测法预测我国铁路客运量的效果较好.因此傅里叶级数在一定程度上受到了很多数学家的欢迎. 关键词:傅里叶级数;收敛性;MATLAB软件;图案设计;预测模型
Fourier series and its applications Abstract:Fourier series is a mathematical analysis of an important concept,and has good geometry and algebraic properties,along with the progress and development of technology,involving a lot of discussion and application of mathematical propositions,Fourier series of relevant knowledge has become a mathematical foundation for scientific research and engineering design and other technical personnel necessary. Through Fourier,Lagrange,Dirichlet, Riemann,who contribute in terms of Fourier series,Fourier series introduces the origin and development process,while the article in the graphic design and rail application passenger traffic forecast illustrates the value of the Fourier series. In the design of graphic design,the use of MATLAB software program written in the language of Fourier series,via a custom function,the preparation process of drawing functions,the excess part of the graphics processing,graphics,bold lines and other steps,then get the Fourier series pattern by the combination of the basic pattern of the Fourier series,the arrangement may constitute a rich patterns. Railway passenger traffic forecast,prediction model based on Fourier series to the railway passenger traffic volume of 2004-2009 data base,by the time series into trend and seasonal part,respectively,using the least squares method and fourier Fourier series prediction method for both fitting using MATLAB software,find the prediction model and predict the outcome of the prediction error by analysis showed that:Fourier series prediction method to predict the effect of China's railway passenger volume better. So to some extent,the Fourier series has been welcomed by many mathematicians. Keywords:Fourier series;convergence;MATLAB software;graphic design;prediction model
小波分析考试题及答案
一、叙述小波分析理论发展的历史和研究现状 答:傅立叶变换能够将信号的时域和特征和频域特征联系起来,能分别从信号的时域和频域观察,但不能把二者有机的结合起来。这是因为信号的时域波形中不包含任何频域信息,而其傅立叶谱是信号的统计特性,从其表达式中也可以看出,它是整个时间域内的积分,没有局部化分析信号的功能,完全不具备时域信息,也就是说,对于傅立叶谱中的某一频率,不能够知道这个频率是在什么时候产生的。这样在信号分析中就面临一对最基本的矛盾——时域和频域的局部化矛盾。 在实际的信号处理过程中,尤其是对非常平稳信号的处理中,信号在任一时刻附近的频域特征很重要。如柴油机缸盖表明的振动信号就是由撞击或冲击产生的,是一瞬变信号,单从时域或频域上来分析是不够的。这就促使人们去寻找一种新方法,能将时域和频域结合起来描述观察信号的时频联合特征,构成信号的时频谱,这就是所谓的时频分析,亦称为时频局部化方法。 为了分析和处理非平稳信号,人们对傅立叶分析进行了推广乃至根本性的革命,提出并开发了一系列新的信号分析理论:短时傅立叶变换、时频分析、Gabor 变换、小波变换Randon-Wigner变换、分数阶傅立叶变换、线形调频小波变换、循环统计量理论和调幅—调频信号分析等。其中,短时傅立叶变换和小波变换也是因传统的傅立叶变换不能够满足信号处理的要求而产生的。 短时傅立叶变换分析的基本思想是:假定非平稳信号在不同的有限时间宽度内是平稳信号,从而计算出各个不同时刻的功率谱。但从本质上讲,短时傅立叶变换是一种单一分辨率的信号分析方法,因为它使用一个固定的短时窗函数,因而短时傅立叶变换在信号分析上还是存在着不可逾越的缺陷。 小波变换是一种信号的时间—尺度(时间—频率)分析方法,具有多分辨
信号与系统matlab实验傅里叶分析及应用报告答案
实验二傅里叶分析及应用 姓名学号班级 一、实验目的 (一)掌握使用Matlab进行周期信号傅里叶级数展开和频谱分析 1、学会使用Matlab分析傅里叶级数展开,深入理解傅里叶级数的物理含义 2、学会使用Matlab分析周期信号的频谱特性 (二)掌握使用Matlab求解信号的傅里叶变换并分析傅里叶变换的性质 1、学会运用Matlab求连续时间信号的傅里叶变换 2、学会运用Matlab求连续时间信号的频谱图 3、学会运用Matlab分析连续时间信号的傅里叶变换的性质 (三)掌握使用Matlab完成信号抽样并验证抽样定理 1、学会运用MATLAB完成信号抽样以及对抽样信号的频谱进行分析 2、学会运用MATLAB改变抽样时间间隔,观察抽样后信号的频谱变化 3、学会运用MATLAB对抽样后的信号进行重建 二、实验条件 需要一台PC机和一定的matlab编程能力 三、实验内容 2、分别利用Matlab符号运算求解法和数值计算法求下图所示信号的FT,并画出其频谱图(包括幅度谱和相位谱)[注:图中时间单位为:毫秒(ms)]。
符号运算法: Ft= sym('t*(Heaviside(t+2)-Heaviside(t+1))+Heaviside(t+1)-Heaviside(t-1)+(-t)*(Heavi side(t-1)-Heaviside(t-2))'); Fw = fourier(Ft); ezplot(abs(Fw)),grid on; phase = atan(imag(Fw)/real(Fw)); ezplot(phase);grid on; title('|F|'); title('phase'); 3、试用Matlab 命令求ω ωωj 54 -j 310)F(j ++= 的傅里叶反变换,并绘出其时域信号图。
傅里叶变换在信号处理中的应用
傅里叶变换在信号处理中的应用 姓名董柱班级电气工程及其自动化学号1109141013 摘要: 傅里叶变换是一种特殊的积分变换。通过傅里叶变换把信号的从时域变换到频域研究,采用频域法较之经典时域的方法有很多突出的优点,虽然傅里叶分析不是信息科学与技术领域中唯一的变换域方法,但是不得不承认,在此领域中,傅里叶变换分析始终有着广泛的应用,通过傅里叶变换实现信号的滤波,调制,抽样是傅里叶变换在信号处理中最主要的作用。通过对信号的调制可以将信号的低频成分调制到高频,实现频谱搬移,减少马间串扰,提高抗噪声新能,有利于信号的远距离传输,另外,对信号采样可以使连续信号离散化,有利于用计算机对信号进行处理,总之,傅里叶变换在信号处理中有着非常重要的作用。傅里叶变换是学习其他频域变换的基础。 关键词: 傅里叶变换,时域,频域,信号处理,信息科学与技术,滤波,调制,抽样。 一傅里叶变换 1.定义 f(t)是t的函数,如果t满足狄里赫莱条件:具有有限个间断点;具有有限个极值点;绝对可积。则有下图①式成立。称为积分运算f(t)的傅立叶变换, ②式的积分运算叫做F(ω)的傅立叶逆变换。F(ω)叫做f(t)的像函数,f(t)叫做 F(ω)的像原函数。F(ω)是f(t)的像。f(t)是F(ω)原像。 ① 傅里叶变换 傅里叶逆变换 2.分类 连续傅立叶变换:一般情况下,若“傅立叶变换”一词的前面未加任何限定语,则指的是“连续傅立叶变换”。“连续傅立叶变换”将平方可积的函数f(t) 表示成复指数函数的积分或级数形式。 f(t) = \mathcal^[F(ω)] = \frac{\sqrt{2π}} \int\limits_{-\infty}^\infty F(ω)e^{iωt}\,dω.
小波分析基础及应用期末习题
题1:设{},j V j Z ∈是依尺度函数()x φ的多分辨率分析,101()0x x φ≤=??其它,请利用Haar 尺度关系式将信号 ()(4)2(41)2(42)(43)f x x x x x φφφφ=+-+---分解为10,0 ,w w v 分量。 题2:简述信号分解和重构的Mallat 算法(要求写出算法步骤并列出分解重构公式。) 题3:设{},,,φφψψ构成双正交多分辨分析: (1) 写出双正交条件; (2) 写出4个双尺度方程(尺度系数分别为 ,,,k k k k h h g g ); (3) 写出尺度系数间的对应关系。 题4:设{} ,j V j Z ∈是依尺度函数()x φ的正交多分辨率分析,k p 是尺度系数,证明: (1)202k l k l k Z p p δ-∈=∑ (2)2||2k k Z p ∈=∑ (3)2k k Z p ∈=∑ 题5:令 2C H =,),(),,(),1,0(212332123 21-=--==e e e , H v v v ∈=?),(21 验证},,{321e e e 是一紧框架,指出其框架界并求出其对偶框架. 题6:列出二维可分离小波的4个变换基。 题3: 0()k h k p =已知为低通分解滤波器,
11()3.k k h k p -=为高通分解滤波器,写出个双倍平移正交关系等式 题6:列出二维可分离小波的4个变换基。 题8:要得到“好”的小波,除要求滤波器0()h n 满足规范、双正交平移性、低通等最小条件外,还可以对0()h n 加消失矩条件来得到性能更优良的小波。 (1) 请写出小波函数()t ψ具有p 阶消失矩的定义条件: (2) 小波函数()t ψ具有p 阶消失矩,要求0()h n 满足等式: (3) 在长度为4的滤波器0()h n 设计中,将下面等式补充完整: 222200000000(0)(1)(2)(3)1 (0)(2)(1)(3)0 ,1 2h h h h h h h h n ?+++=???+==??? 规范性低通双平移正交阶消失矩
MATLAB实验二傅里叶分析及应用
M A T L A B实验二傅里叶 分析及应用 Document serial number【UU89WT-UU98YT-UU8CB-UUUT-UUT108】
实验二傅里叶分析及应用 一、实验目的 (一)掌握使用Matlab进行周期信号傅里叶级数展开和频谱分析 1、学会使用Matlab分析傅里叶级数展开,深入理解傅里叶级数的物理含义 2、学会使用Matlab分析周期信号的频谱特性 (二)掌握使用Matlab求解信号的傅里叶变换并分析傅里叶变换的性质 1、学会运用Matlab求连续时间信号的傅里叶变换 2、学会运用Matlab求连续时间信号的频谱图 3、学会运用Matlab分析连续时间信号的傅里叶变换的性质 (三)掌握使用Matlab完成信号抽样并验证抽样定理 1、学会运用MATLAB完成信号抽样以及对抽样信号的频谱进行分析 2、学会运用MATLAB改变抽样时间间隔,观察抽样后信号的频谱变化 3、学会运用MATLAB对抽样后的信号进行重建 二、实验条件 Win7系统,MATLAB R2015a 三、实验内容 1、分别利用Matlab符号运算求解法和数值计算法求下图所示信号的FT,并画出其频谱图(包括幅度谱和相位谱)[注:图中时间单位为:毫秒(ms)]。
符号运算法
t (20 π ex p(-3 t) heaviside(t) - 8 π ex p(-5 t) heaviside(t))/(2 π) 数值运算法 2、试用Matlab 命令求ω ωωj 54 -j 310)F(j ++=的傅里叶反变换,并绘出其时域信 号图。 两个单边指数脉冲的叠加 3、已知门函数自身卷积为三角波信号,试用Matlab 命令验证FT 的时域卷积定理 。
傅里叶变换及应用
傅里叶变换在MATLZB里的应用 摘要:在现代数学中,傅里叶变换是一种非常重要的变换,且在数字信号处理中有着广泛的应用。本文首先介绍了傅里叶变换的基本概念、性质及发展情况;其次,详细介绍了分离变数法及积分变换法在解数学物理方程中的应用。傅立叶变换将原来难以处理的时域信号转换成了易于分析的频域信号,再利用傅立叶反变换将这些频域信号转换成时域信号。应用MATLAB实现信号的谱分析和对信号消噪。 关键词:傅里叶变换;MA TLAB软件;信号消噪 Abstract: In modern mathematics,Fourier transform is a transform is very important ,And has been widely used in digital signal processing.This paper first introduces the basic concepts, properties and development situation of Fourier transform ;Secondly, introduces in detail the method of separation of variables and integral transform method in solving equations in Mathematical Physics.Fourier transformation makes the original time domain signal whose analysis is difficult easy, by transforming it into frequency domain signal that can be transformed into time domain signal by inverse transformation of Fourier. Using Mat lab realizes signal spectral analysis and signal denoising. Key word: Fourier transformation, software of mat lab ,signal denoising 1、傅里叶变换的提出及发展 在自然科学和工程技术中为了把较复杂的运算转化为较简单的运算,人们常常采用所谓变换的方法来达到目的"例如在初等数学中,数量的乘积和商可以通过对数变换化为较简单的加法和减法运算。在工程数学里积分变换能够将分析运算(如微分,积分)转化为代数运算,正是积分变换这一特性,使得它在微分方程和其它方程的求解中成为重要方法之一。 1804年,法国科学家J-.B.-J.傅里叶由于当时工业上处理金属的需要,开始从事热流动的研究"他在题为<<热的解析理论>>一文中,发展了热流动方程,并且指出如何求解"在求解过程中,他提出了任意周期函数都可以用三角级数来表示的想法。他的这种
博士复试题目+答案
1、小波变换在图像处理中有着广泛的应用,请简述其在图像压缩中的应用原理? 答:一幅图像经过一次小波变换之后,概貌信息大多集中在低频部分,而其余部分只有微弱的细节信息。为此,如果只保留占总数数量1/4的低频部分,对其余三个部分的系数不存储或传输,在解压时,这三个子块的系数以0来代替,则就可以省略图像部分细节信息,而画面的效果跟原始图像差别不是很大。这样,就可以得到图像压缩的目的。 2、给出GPEG数据压缩的特点。 答:(1)一种有损基本编码系统,这个系统是以DCT为基础的并且足够应付大多数压缩方向应用。 (2)一种扩展的编码系统,这种系统面向的是更大规模的压缩,更高精确性或逐渐递增的重构应用系统。 (3)一种面向可逆压缩的无损独立编码系统。 3、设计雪花检测系统 答:1)获得彩色雪花图像。2)灰度雪花图像。3)图像的灰度拉伸,以增强对比度。4)阈值判断法二值化图像。5)图像的梯度锐化。6)对图像进行自定义模板中值滤波以去除噪声。7)用梯度算子对雪花区域的定位。8)利用hough变换截下雪花区域的图片。 9)雪花图片几何位置调整。 4、用图像处理的原理设计系统,分析木材的年轮结构。 答:1)获得彩色木材年轮图像。2)灰度木材年轮图像。3)灰度拉伸以增加对比度。4)阈值判定法二值化图像。5)图像的梯度锐化。6)对图像进行自定义模板中值滤波以去除噪声。7)用梯度算子对木材年轮圈进行定位。8)图片二值化。9)利用边界描述子对木材的年轮结构进行识别。 5、给出生猪的尺寸和形貌检测系统。 答:1)获得彩色生猪图像。2)灰度生猪图像。3)图像的灰度拉伸,以增强对比度。4)阈值判定法二值化图像。5)图像的梯度锐化。6)对图像进行自定义模板中值滤波以除去噪声。 7)用梯度算子对生猪区域的定位。8)利用hough变换截下生猪区域的图片。9)生猪图片几何位置调整。10)生猪图片二值化。11)利用边界描述子对生猪尺寸和形貌的识别。 第二种答案:(类似牌照检测系统) 1)第一步定位牌照 由图像采集部件采集生猪的外形图像并将图像存储在存储器中,其特征在于:数字处理器由存储器中读入并运行于生猪外形尺寸检测的动态检测软件、从存储器中依次读入两幅车辆外形图像数据、经过对生猪外形图像分析可得到生猪的高度,宽度和长度数据即生猪的外形尺寸。通过高通滤波,得到所有的边对边缘细化(但要保持连通关系),找出所有封闭的边缘,对封闭边缘求多边形逼近,在逼近后的所有四边形中,找出尺寸与牌照大小相同的四边形。生猪形貌被定位。 2)第二步识别 区域中的细化后的图形对象,计算傅里叶描述子,用预先定义好的决策函数,对描述子进行计算,判断到底是数字几。 6、常用的数字图像处理开发工具有哪些?各有什么特点? 答:目前图像处理系统开发的主流工具为Visual C++(面向对象可视化集成工具)和MATLAB的图像处理工具箱(lmage processing tool box)。两种开发工具各有所长且有相互间的软件接口。 微软公司的VC++是一种具有高度综合性能的面向对象可视化集成工具,用它开发出来
傅里叶变换和拉普拉斯变换的性质及应用
1.前言 1.1背景 利用变换可简化运算,比如对数变换,极坐标变换等。 类似的,变换也存在于工程,技术领域,它就是积分变换。 积分变换的使用,可以使求解微分方程的过程得到简化, 比如乘积可以转化为卷积。什么是积分变换呢?即为利用 含参变量积分,把一个属于A函数类的函数转化属于B函 数类的一个函数。傅里叶变换和拉普拉斯变换是两种重要 积分变换。分析信号的一种方法是傅立叶变换,傅里叶变换能 够分析信号的成分,也能够利用成分合成信号。可以当做信号 的成分的波形有很多,例如锯齿波,正弦波,方波等等。傅立 叶变换是利用正弦波来作为信号的成分。 拉普拉斯变换最早由法国数学家天文学家 Pierre Simon Laplace (拉普拉斯)(1749-1827)在他的与概率论相关科学研究 中引入,在他的一些基本的关于拉普拉斯变换的结果写在 他的著名作品《概率分析理论》之中。即使在19世纪初, 拉普拉斯变换已经发现,但是关于拉普拉斯变换的相关研 究却一直没什么太大进展,直至一个英国数学家,物理学 家,同时也是一位电气工程师的Oliver Heaviside奥利 弗·亥维赛(1850-1925)在电学相关问题之中引入了算 子运算,而且得到了不少方法与结果,对于解决现实问题 很有好处,这才引起了数学家对算子理论的严格化的兴 趣。之后才创立了现代算子理论。算子理论最初的理论依 据就是拉普拉斯变换的相关理论,拉普拉斯变换相关理论 的继续发展也是得益于算理理论的更进一步发展。这篇文 章就是针对傅里叶变换和拉普拉斯变换的相关定义,相关 性质,以及相关应用做一下简要讨论,并且分析傅里叶变 换和拉普拉斯变换的区别与联系。 1.2预备知识
傅里叶级数和应用毕业论文
傅里叶级数及其应用 专业:数学与应用数学 班级: 姓名:
目录 引言 (3) 1 傅立叶级数的计算 (5) 1.1 傅立叶级数的几何意义 (5) 1.2 傅里叶级数的敛散性问题 (10) 1.3 傅里叶级数的展开 (11) 1.4 关于傅里叶级数展开的个别简便算法 (16) 1.5 利用二元函数微分中值定理研究函数性质 (19) 2 傅里叶级数的相关定理及其应用 (21) 2.1 n元函数中值定理及其几何意义 (21) 2.2 利用n元函数微分中值定理研究函数的性质 (28) 3 微分中值定理在复数域上的推广 (32) 3.1 复数域上的中值定理 (32) 3.2 利用复数域内中值定理研究函数性质 (36) 结论 (39) 致谢 (40) 参考文献 (41)
为了更好地认识和应用微分中值定理,使微分中值定理能够最大的发挥其重要作用,在深刻理解和掌握教材内微分中值定理的基础上,将微分中值定理在n元函数以及复数域内推广及应用加以探讨.首先根据一元函数微分中值定理的内容,给出了罗尔定理、拉格朗日定理、柯西中值定理、泰勒中值定理公式的统一形式.而后又仿照一元函数微分中值定理的形式对教材中二元函数微分中值定理进行补充,给出了二元函数罗尔定理、柯西中值定理和二元函数泰勒中值定理的表述,并且构造“辅助函数”给出了证明过程,然后讨论了二元函数罗尔定理与拉格朗日定理的几何意义.接着通过对比一元函数与二元函数微分中值定理,给出了n元函数罗尔定理、拉格朗日定理、柯西中值定理和泰勒中值定理的表述形式,而后同样借助构造的“辅助函数”把n元函数转化为一元函数,进而给出了四个定理的证明,并通过几个典型例题验证了n元函数微分中值定理的可用性.最后从二元函数微分中值定理着手,给出了复数域上的罗尔定理、拉格朗日定理、柯西中值定理的表述形式,同时通过几个例题验证了复数域上微分中值定理的可用性. 关键词: n元函数;微分中值定理;几何意义;复数域
数字图像处理复习题(选择题及相应答案)解析
第一章 1.1.1可以用f(x,y)来表示:(ABD) A、一幅2-D数字图像 B、一个在3-D空间中的客观景物的投影; C 2-D空间XY中的一个坐标的点的位置; D、在坐标点(X,Y)的某种性质F的数值。 提示:注意3个符号各自的意义 1.1.2、一幅数字图像是:(B) A、一个观测系统; B、一个有许多像素排列而成的实体; C、一个2-D数组中的元素 D、一个3-D空间的场景。 提示:考虑图像和数字图像的定义 1.2.2、已知如图1.2.2中的2个像素P和Q,下面说法正确的是:(C) A、2个像素P和Q直接的De距离比他们之间的D4距离和D8距离都短: B、2个像素p和q之间的D4距离为5; C、2个像素p和q之间的D8距离为5; D、2个像素p和q之间的De距离为5。 1.4.2、半调输出技术可以:(B) A、改善图像的空间分辨率; B、改善图像的幅度分辨率; C、利用抖动技术实现; D、消除虚假轮廓现象。 提示:半调输出技术牺牲空间分辨率以提高幅度分辨率 1.4.3、抖动技术可以(D) A、改善图像的空间分辨率; B、改善图像的幅度分辨率; C、利用半输出技术实现; D、消除虚假轮廓现象。 提示:抖动技术通过加入随即噪声,增加了图像的幅度输出值的个数 1.5.1、一幅256*256的图像,若灰度级数为16,则存储它所需的比特数是:(A) A、256K B、512K C、1M C、2M 提示:表达图像所需的比特数是图像的长乘宽再乘灰度级数对应的比特数。1.5.2、图像中虚假轮廓的出现就其本质而言是由于:(A)(平滑区域内灰度应缓慢变化,但当图像的灰度级数不够多时会产生阶跃) A、图像的灰度级数不够多造成的; B、图像的空间分辨率不够高造成; C、图像的灰度级数过多造成的 D、图像的空间分辨率过高造成。 提示:图像中的虚假轮廓最易在平滑区域内产生。 1.5.3、数字图像木刻画效果的出现是由于下列原因所产生的:(A) A、图像的幅度分辨率过小; B、图像的幅度分辨率过大; C、图像的空间分辨率过小; D、图像的空间分辨率过大;
快速傅里叶变换原理及其应用(快速入门)
快速傅里叶变换的原理及其应用 摘要 快速傅氏变换(FFT),是离散傅氏变换的快速算法,它是根据离散傅氏变换的奇、偶、虚、实等特性,对离散傅立叶变换的算法进行改进获得的。它对傅氏变换的理论并没有新的发现,但是对于在计算机系统或者说数字系统中应用离散傅立叶变换,可以说是进了一大步。傅里叶变换的理论与方法在“数理方程”、“线性系统分析”、“信号处理、仿真”等很多学科领域都有着广泛应用,由于计算机只能处理有限长度的离散的序列,所以真正在计算机上运算的是一种离散傅里叶变换. 虽然傅里叶运算在各方面计算中有着重要的作用,但是它的计算过于复杂,大量的计算对于系统的运算负担过于庞大,使得一些对于耗电量少,运算速度慢的系统对其敬而远之,然而,快速傅里叶变换的产生,使得傅里叶变换大为简化,在不牺牲耗电量的条件下提高了系统的运算速度,增强了系统的综合能力,提高了运算速度,因此快速傅里叶变换在生产和生活中都有着非常重要的作用,对于学习掌握都有着非常大的意义。 关键词快速傅氏变换;快速算法;简化;广泛应用
Abstract Fast Fourier Transform (FFT), is a discrete fast Fourier transform algorithm, which is based on the Discrete Fourier Transform of odd and even, false, false, and other characteristics of the Discrete Fourier Transform algorithms improvements obtained. Its Fourier transform theory has not found a new, but in the computer system or the application of digital systems Discrete Fourier Transform can be said to be a big step into. Fourier transform theory and methods in the "mathematical equation" and "linear systems analysis" and "signal processing, simulation," and many other areas have a wide range of applications, as the computer can only handle a limited length of the sequence of discrete, so true On the computer's operation is a discrete Fourier transform. Fourier Although all aspects of computing in the calculation has an important role, but its calculation was too complicated, a lot of computing system for calculating the burden is too large for some Less power consumption, the slow speed of operation of its system at arm's length, however, have the fast Fourier transform, Fourier transform greatly simplifying the making, not in power at the expense of the conditions to increase the speed of computing systems, and enhance the system The comprehensive ability to improve the speed of operation, the Fast Fourier Transform in the production and life have a very important role in learning to master all have great significance. Key words Fast Fourier Transform; fast algorithm; simplified; widely used
傅里叶变换的应用
傅立叶变换在图像处理中有非常非常的作用。因为不仅傅立叶分析涉及图像处理的很多方面,傅立叶的改进算法, 比如离散余弦变换,gabor与小波在图像处理中也有重要的分量。 印象中,傅立叶变换在图像处理以下几个话题都有重要作用: 1.图像增强与图像去噪 绝大部分噪音都是图像的高频分量,通过低通滤波器来滤除高频——噪声; 边缘也是图像的高频分量,可以通过添加高频分量来增强原始图像的边缘; 2.图像分割之边缘检测 提取图像高频分量 3.图像特征提取: 形状特征:傅里叶描述子 纹理特征:直接通过傅里叶系数来计算纹理特征 其他特征:将提取的特征值进行傅里叶变换来使特征具有平移、伸缩、旋转不变性 4.图像压缩 可以直接通过傅里叶系数来压缩数据;常用的离散余弦变换是傅立叶变换的实变换; 傅立叶变换 傅里叶变换是将时域信号分解为不同频率的正弦信号或余弦函数叠加之和。连续情况下要求原始信号在一个周期内满足绝对可积条件。离散情况下,傅里叶变换一定存在。冈萨雷斯版<图像处理>里面的解释非常形象:一个恰当的比喻是将傅里叶变换比作一个玻璃棱镜。棱镜是可以将光分解为不同颜色的物理仪器,每个成分的颜色由波长(或频率)来决定。傅里叶变换可以看作是数学上的棱镜,将函数基于频率分解为不同的成分。当我们考虑光时,讨论它的光谱或频率谱。同样,傅立叶变换使我们能通过频率成分来分析一个函数。 傅立叶变换有很多优良的性质。比如线性,对称性(可以用在计算信号的傅里叶变换里面); 时移性:函数在时域中的时移,对应于其在频率域中附加产生的相移,而幅度频谱则保持不变; 频移性:函数在时域中乘以e^jwt,可以使整个频谱搬移w。这个也叫调制定理,通讯里面信号的频分复用需要用到这个特性(将不同的信号调制到不同的频段上同时传输); 卷积定理:时域卷积等于频域乘积;时域乘积等于频域卷积(附加一个系数)。(图像处理里面这个是个重点) 信号在频率域的表现 在频域中,频率越大说明原始信号变化速度越快;频率越小说明原始信号越平缓。当频率为0时,表示直流信号,没有变化。因此,频率的大小反应了信号的变化