人脸识别英文文献
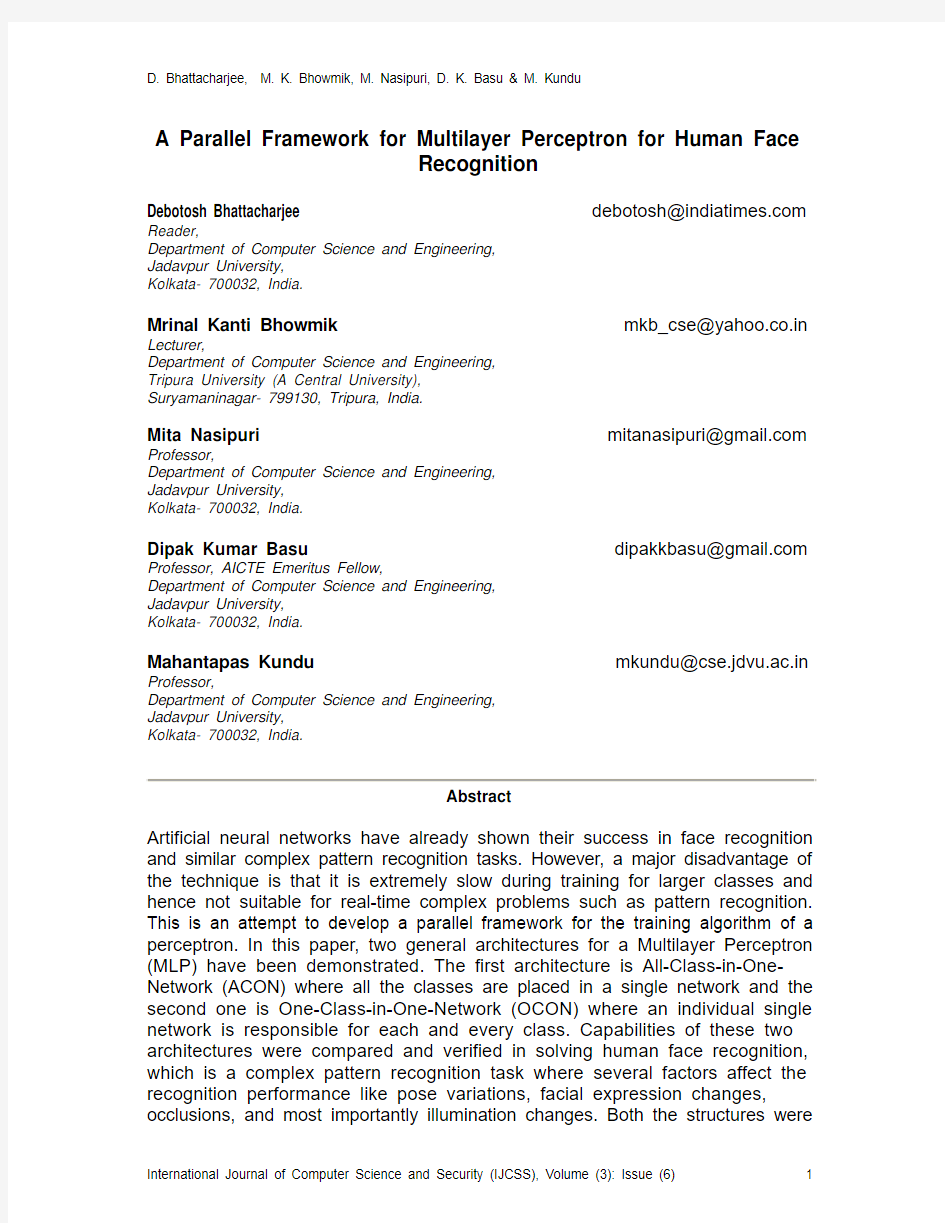

D. Bhattacharjee, M. K. Bhowmik, M. Nasipuri, D. K. Basu & M. Kundu
A Parallel Framework for Multilayer Perceptron for Human Face Recognition
Debotosh Bhattacharjee
Reader, Department of Computer Science and Engineering, Jadavpur University, Kolkata- 700032, India.
debotosh@https://www.360docs.net/doc/ac2699255.html,
Mrinal Kanti Bhowmik
Lecturer, Department of Computer Science and Engineering, Tripura University (A Central University), Suryamaninagar- 799130, Tripura, India.
mkb_cse@yahoo.co.in
Mita Nasipuri
Professor, Department of Computer Science and Engineering, Jadavpur University, Kolkata- 700032, India.
mitanasipuri@https://www.360docs.net/doc/ac2699255.html,
Dipak Kumar Basu
Professor, AICTE Emeritus Fellow, Department of Computer Science and Engineering, Jadavpur University, Kolkata- 700032, India.
dipakkbasu@https://www.360docs.net/doc/ac2699255.html,
Mahantapas Kundu
Professor, Department of Computer Science and Engineering, Jadavpur University, Kolkata- 700032, India.
mkundu@cse.jdvu.ac.in
Abstract
Artificial neural networks have already shown their success in face recognition and similar complex pattern recognition tasks. However, a major disadvantage of the technique is that it is extremely slow during training for larger classes and hence not suitable for real-time complex problems such as pattern recognition. This is an attempt to develop a parallel framework for the training algorithm of a perceptron. In this paper, two general architectures for a Multilayer Perceptron (MLP) have been demonstrated. The first architecture is All-Class-in-OneNetwork (ACON) where all the classes are placed in a single network and the second one is One-Class-in-One-Network (OCON) where an individual single network is responsible for each and every class. Capabilities of these two architectures were compared and verified in solving human face recognition, which is a complex pattern recognition task where several factors affect the recognition performance like pose variations, facial expression changes, occlusions, and most importantly illumination changes. Both the structures were
International Journal of Computer Science and Security (IJCSS), Volume (3): Issue (6) 1
D. Bhattacharjee, M. K. Bhowmik, M. Nasipuri, D. K. Basu & M. Kundu
implemented and tested for face recognition purpose and experimental results show that the OCON structure performs better than the generally used ACON ones in term of training convergence speed of the network. Unlike the conventional sequential approach of training the neural networks, the OCON technique may be implemented by training all the classes of the face images simultaneously.
Keywords: Artificial Neural Network, Network architecture, All-Class-in-One-Network (ACON), One-Classin-One-Network (OCON), PCA, Multilayer Perceptron, Face recognition.
1. INTRODUCTION
Neural networks, with their remarkable ability to derive meaning from complicated or imprecise data, can be used to extract patterns and detect trends that are too complex to be noticed by either humans or other computer techniques. A trained neural network can be thought of as an "expert" in the category of information it has been given to analyze [1]. This proposed work describes the way by which an Artificial Neural Network (ANN) can be designed and implemented over a parallel or distributed environment to reduce its training time. Generally, an ANN goes through three different steps: training of the network, testing of it and final use of it. The final structure of an ANN is generally found out experimentally. This requires huge amount of computation. Moreover, the training time of an ANN is very large, when the classes are linearly non-separable and overlapping in nature. Therefore, to save computation time and in order to achieve good response time the obvious choice is either a high-end machine or a system which is collection of machines with low computational power. In this work, we consider multilayer perceptron (MLP) for human face recognition, which has many real time applications starting from automatic daily attendance checking, allowing the authorized people to enter into highly secured area, in detecting and preventing criminals and so on. For all these cases, response time is very critical. Face recognition has the benefit of being passive, nonintrusive system for verifying personal identity. The techniques used in the best face recognition systems may depend on the application of the system. Human face recognition is a very complex pattern recognition problem, altogether. There is no stability in the input pattern due to different expressions, adornments in the input images. Sometimes, distinguishing features appear similar and produce a very complex situation to take decision. Also, there are several other that make the face recognition task complicated. Some of them are given below. a) Background of the face image can be a complex pattern or almost same as the color of the face. b) Different illumination level, at different parts of the image. c) Direction of illumination may vary. d) Tilting of face. e) Rotation of face with different angle. f) Presence/absence of beard and/or moustache g) Presence/Absence of spectacle/glasses. h) Change in expressions such as disgust, sadness, happiness, fear, anger, surprise etc. i) Deliberate change in color of the skin and/or hair to disguise the designed system. From above discussion it can now be claimed that the face recognition problem along with face detection, is very complex in nature. To solve it, we require some complex neural network, which takes large amount of time to finalize its structure and also to settle its parameters. In this work, a different architecture has been used to train a multilayer perceptron in faster way. Instead of placing all the classes in a single network, individual networks are used for each of the
International Journal of Computer Science and Security (IJCSS), Volume (3): Issue (6)
2
D. Bhattacharjee, M. K. Bhowmik, M. Nasipuri, D. K. Basu & M. Kundu
classes. Due to lesser number of samples and conflicts in the belongingness of patterns to their respective classes, a later model appears to be faster in comparison to former.
2. ARTIFICIAL NEURAL NETWORK
Artificial neural networks (ANN) have been developed as generalizations of mathematical models of biological nervous systems. A first wave of interest in neural networks (also known as connectionist models or parallel distributed processing) emerged after the introduction of simplified neurons by McCulloch and Pitts (1943).The basic processing elements of neural networks are called artificial neurons, or simply neurons or nodes. In a simplified mathematical model of the neuron, the effects of the synapses are represented by connection weights that modulate the effect of the associated input signals, and the nonlinear characteristic exhibited by neurons is represented by a transfer function. The neuron impulse is then computed as the weighted sum of the input signals, transformed by the transfer function. The learning capability of an artificial neuron is achieved by adjusting the weights in accordance to the chosen learning algorithm. A neural network has to be configured such that the application of a set of inputs produces the desired set of outputs. Various methods to set the strengths of the connections exist. One way is to set the weights explicitly, using a priori knowledge. Another way is to train the neural network by feeding it teaching patterns and letting it change its weights according to some learning rule. The learning situations in neural networks may be classified into three distinct sorts. These are supervised learning, unsupervised learning, and reinforcement learning. In supervised learning, an input vector is presented at the inputs together with a set of desired responses, one for each node, at the output layer. A forward pass is done, and the errors or discrepancies between the desired and actual response for each node in the output layer are found. These are then used to determine weight changes in the net according to the prevailing learning rule. The term supervised originates from the fact that the desired signals on individual output nodes are provided by an external teacher [3]. Feed-forward networks had already been used successfully for human face recognition. Feed-forward means that there is no feedback to the input. Similar to the way that human beings learn from mistakes, neural networks also could learn from their mistakes by giving feedback to the input patterns. This kind of feedback would be used to reconstruct the input patterns and make them free from error; thus increasing the performance of the neural networks. Of course, it is very complex to construct such types of neural networks. These kinds of networks are called as auto associative neural networks. As the name implies, they use back-propagation algorithms. One of the main problems associated with backpropagation algorithms is local minima. In addition, neural networks have issues associated with learning speed, architecture selection, feature representation, modularity and scaling. Though there are problems and difficulties, the potential advantages of neural networks are vast. Pattern recognition can be done both in normal computers and neural networks. Computers use conventional arithmetic algorithms to detect whether the given pattern matches an existing one. It is a straightforward method. It will say either yes or no. It does not tolerate noisy patterns. On the other hand, neural networks can tolerate noise and, if trained properly, will respond correctly for unknown patterns. Neural networks may not perform miracles, but if constructed with the proper architecture and trained correctly with good data, they will give amazing results, not only in pattern recognition but also in other scientific and commercial applications [4]. 2A. Network Architecture The computing world has a lot to gain from neural networks. Their ability to learn by example makes them very flexible and powerful. Once a network is trained properly there is no need to devise an algorithm in order to perform a specific task; i.e. no need to understand the internal mechanisms of that task. The architecture of any neural networks generally used is All-Class-inOne-Network (ACON), where all the classes are lumped into one super-network. Hence, the implementation of such ACON structure in parallel environment is not possible. Also, the ACON structure has some disadvantages like the super-network has the burden to simultaneously satisfy all the error constraints by which the number of nodes in the hidden layers tends to be large. The structure of the network is All-Classes-in-One-Network (ACON), shown in Figure 1(a) where one single network is designed to classify all the classes but in One-Class-in-One-Network
International Journal of Computer Science and Security (IJCSS), Volume (3): Issue (6)
3
D. Bhattacharjee, M. K. Bhowmik, M. Nasipuri, D. K. Basu & M. Kundu
(OCON), shown in Figure 1(b) a single network is dedicated to recognize one particular class. For each class, a network is created with all the training samples of that class as positive examples, called the class-one, and the negative examples for that class i.e. exemplars from other classes, constitute the class-two. Thus, this classification problem is a two-class partitioning problem. So far, as implementation is concerned, the structure of the network remains the same for all classes and only the weights vary. As the network remains same, weights are kept in separate files and the identification of input image is made on the basis of feature vector and stored weights applied to the network one by one, for all the classes.
Classes 1, 2, ……, C or not
ANN
Input
(a)
Class 1 or not?
Class 2 or not?
Class C or not?
ANN 1
ANN 2
… … … ..
ANN C
Input
Input
Input
(b) Figure 1: a) All-Classes-in-One-Network (ACON) b) One-Class-in-One-Network (OCON). Empirical results confirm that the convergence rate of ACON degrades drastically with respect to the network size because the training of hidden units is influenced by (potentially conflicting) signals from different teachers. If the topology is changed to One Class in One Network (OCON) structure, where one sub-network is designated and responsible for one class only then each sub-network specializes in distinguishing its own class from the others. So, the number of hidden units is usually small. 2B. Training of an ANN In the training phase the main goal is to utilize the resources as much as possible and speed-up the computation process. Hence, the computation involved in training is distributed over the system to reduce response time. The training procedure can be given as:
International Journal of Computer Science and Security (IJCSS), Volume (3): Issue (6)
4
D. Bhattacharjee, M. K. Bhowmik, M. Nasipuri, D. K. Basu & M. Kundu
(1) Retrieve the topology of the neural network given by the user, (2) Initialize required parameters and weight vector necessary to train the network, (3) Train the network as per network topology and available parameters for all exemplars of different classes, (4) Run the network with test vectors to test the classification ability, (5) If the result found from step 4 is not satisfactory, loop back to step 2 to change the parameters like learning parameter, momentum, number of iteration or even the weight vector, (6) If the testing results do not improve by step 5, then go back to step 1, (7) The best possible (optimal) topology and associated parameters found in step 5 and step 6 are stored. Although we have parallel systems already in use but some problems cannot exploit advantages of these systems because of their inherent sequential execution characteristics. Therefore, it is necessary to find an equivalent algorithm, which is executable in parallel. In case of OCON, different individual small networks with least amount of load, which are responsible for different classes (e.g. k classes), can easily be trained in k different processors and the training time must reduce drastically. To fit into this parallel framework previous training procedure can be modified as follows: (1) Retrieve the topology of the neural network given by the user, (2) Initialize required parameters and weight vector necessary to train the network, (3) Distribute all the classes (say k) to available processors (possibly k) by some optimal process allocation algorithm, (4) Ensure the retrieval the exemplar vectors of respective classes by the corresponding processors, (5) Train the networks as per network topology and available parameters for all exemplars of different classes, (6) Run the networks with test vectors to test the classification ability, (7) If the result found from step 6 is not satisfactory, loop back to step 2 to change the parameters like learning parameter, momentum, number of iteration or even the weight vector, (8) If the testing results do not improve by step 5, then go back to step 1, (9) The best possible (optimal) topology and associated parameters found in step 7 and step 8 are stored, (10) Store weights per class with identification in more than one computer [2]. During the training of two different topologies (OCON and ACON), we used total 200 images of 10 different classes and the images are with different poses and also with different illuminations. Sample images used during training are shown Figure 2. We implemented both the topologies using MATLAB. At the time of training of our systems for both the topologies, we set maximum number of possible epochs (or iterations) to 700000. The training stops if the number of iterations -6 exceeds this limit or performance goal is met. Here, performance goal was considered as 10 . We have taken total 10 different training runs for 10 different classes for OCON and one single training run for ACON for 10 different classes. In case of the OCON networks, performance goal was met for all the 10 different training cases, and also in lesser amount of time than ACON. After the completion of training phase of our two different topologies we tested our both the network using the images of testing class which are not used in training. 2C. Testing Phase During testing, the class found in the database with minimum distance does not necessarily stop the testing procedure. Testing is complete after all the registered classes are tested. During testing some points were taken into account, those are:
International Journal of Computer Science and Security (IJCSS), Volume (3): Issue (6)
5
D. Bhattacharjee, M. K. Bhowmik, M. Nasipuri, D. K. Basu & M. Kundu
(1) The weights of different classes already available are again distributed in the available computer to test a particular image given as input, (2) The allocation of the tasks to different processors is done based on the testing time and interprocessor communication overhead. The communication overhead should be much less than the testing time for the success of the distribution of testing, and (3) The weight vector of a class matter, not the computer, which has computed it. The testing of a class can be done in any computer as the topology and the weight vector of that class is known. Thus, the system can be fault tolerant [2]. At the time of testing, we used total 200 images. Among 200 images 100 images are taken from the same classes those are used during the training and 100 images from other classes are not used during the training time. In the both topology (ACON and OCON), we have chosen 20 images for testing, in which 10 images from same class those are used during the training as positive exemplars and other 10 images are chosen from other classes of the database as negative exemplars. 2D. Performance measurement Performance of this system can be measured using following parameters: (1) resource sharing: Different terminals remain idle most of the time can be used as a part of this system. Once the weights are finalized anyone in the net, even though not satisfying the optimal testing time criterion, can use it. This can be done through Internet attempting to end the “tyranny of geography”, (2) high reliability: Here we will be concerned with the reliability of the proposed system, not the inherent fault tolerant property of the neural network. Reliability comes from the distribution of computed weights over the system. If any of the computer(or processor) connected to the network goes down then the system works Some applications like security monitoring system, crime prevention system require that the system should work, whatever may be the performance, (3) cost effectiveness: If we use several small personal computers instead of high-end computing machines, we achieve better price/performance ratio, (4) incremental growth: If the number of classes increases, then the complete computation including the additional complexity can be completed without disturbing the existing system. Based on the analysis of performance of our two different topologies, if we see the recognition rates of OCON and ACON in Table 1 and Table 2 OCON is showing better recognition rate than ACON. Comparison in terms of training time can easily be observed in figures 3 (Figure 3 (a) to (k)). In case of OCON, performance goals met for 10 different classes are 9.99999e-007, 1e-006, 9.99999e-007, 9.99998e-007, 1e-006, 9.99998e-007,1e-006, 9.99997e-007, 9.99999e-007 respectively, whereas for ACON it is 0.0100274. Therefore, it is pretty clear that OCON requires less computational time to finalize a network to use.
3. PRINCIPAL COMPONENT ANALYSIS
The Principal Component Analysis (PCA) [5] [6] [7] uses the entire image to generate a set of features in the both network topology OCON and ACON and does not require the location of individual feature points within the image. We have implemented the PCA transform as a reduced feature extractor in our face recognition system. Here, each of the visual face images is projected into the eigenspace created by the eigenvectors of the covariance matrix of all the training images for both the ACON and OCON networks. Here, we have taken the number of eigenvectors in the eigenspace as 40 because eigenvalues for other eigenvectors are negligible in comparison to the largest eigenvalues.
4. EXPERIMENTS RESULTS USING OCON AND ACON
This work has been simulated using MATLAB 7 in a machine of the configuration 2.13GHz Intel Xeon Quad Core Processor and 16 GB of Physical Memory. We have analyzed the performance of our method using YALE B database which is a collection of visual face images with various poses and illumination.
International Journal of Computer Science and Security (IJCSS), Volume (3): Issue (6)
6
D. Bhattacharjee, M. K. Bhowmik, M. Nasipuri, D. K. Basu & M. Kundu
4A. YALE Face Database B This work has been simulated using MATLAB 7 in a machine of the configuration 2.13GHz Intel Xeon Quad Core Processor and 16 GB of Physical Memory. We have analyzed the performance of our method using YALE B database which is a collection of visual face images with various poses and illumination. This database contains 5760 single light source images of 10 subjects each seen under 576 viewing conditions (9 poses x 64 illumination conditions). For every subject in a particular pose, an image with ambient (background) illumination was also captured. Hence, the total number of images is 5850. The total size of the compressed database is about 1GB. The 65 (64 illuminations + 1 ambient) images of a subject in a particular pose have been "tarred" and "gzipped" into a single file. There were 47 (out of 5760) images whose corresponding strobe did not go off. These images basically look like the ambient image of the subject in a particular pose. The images in the database were captured using a purpose-built illumination rig. This rig is fitted with 64 computer controlled strobes. The 64 images of a subject in a particular pose were acquired at camera frame rate (30 frames/second) in about 2 seconds, so there is only small change in head pose and facial expression for those 64 (+1 ambient) images. The image with ambient illumination was captured without a strobe going off. For each subject, images were captured under nine different poses whose relative positions are shown below. Note the pose 0 is the frontal pose. Poses 1, 2, 3, 4, and 5 were about 12 degrees from the camera optical axis (i.e., from Pose 0), while poses 6, 7, and 8 were about 24 degrees. In the Figure 2 sample images of per subject per pose with frontal illumination. Note that the position of a face in an image varies from pose to pose but is fairly constant within the images of a face seen in one of the 9 poses, since the 64 (+1 ambient) images were captured in about 2 seconds. The acquired images are 8bit (gray scale) captured with a Sony XC-75 camera (with a linear response function) and stored in PGM raw format. The size of each image is 640(w) x 480 (h) [9]. In our experiment, we have chosen total 400 images for our experiment purpose. Among them 200 images are taken for training and other 200 images are taken for testing purpose from 10 different classes. In the experiment we use total two different networks: OCON and ACON. All the recognition results of OCON networks are shown in Table 1, and all the recognition results of ACON network are shown in Table 2. During training, total 10 training runs have been executed for 10 different classes. We have completed total 10 different testing for OCON network using 20 images for each experiment. Out of those 20 images, 10 images are taken form the same classes those were used during training, which acts as positive exemplars and rest 10 images are taken from other classes that acts as negative exemplars for that class. In case of OCON, system achieved 100% recognition rate for all the classes. In case of the ACON network, only one network is used for 10 different classes. During the training we achieved 100% as the highest recognition rate, but like OCON network not for all the classes. For ACON network, on an average, 88% recognition rate was achieved.
Figure 2: Sample images of YALE B database with different Pose and different illumination.
International Journal of Computer Science and Security (IJCSS), Volume (3): Issue (6)
7
D. Bhattacharjee, M. K. Bhowmik, M. Nasipuri, D. K. Basu & M. Kundu
Class
Total number of testing images 20 20 20 20 20 20 20 20 20 20
Class-1 Class-2 Class-3 Class-4 Class-5 Class-6 Class-7 Class-8 Class-9 Class-10
Number of images from the training class 10 10 10 10 10 10 10 10 10 10
Number of images from other classes 10 10 10 10 10 10 10 10 10 10
Recognition rate 100% 100% 100% 100% 100% 100% 100% 100% 100% 100%
Table 1: Experiments Results for OCON.
Class
Total number of testing images 20 20 20 20 20 20 20 20 20 20
Class - 1 Class - 2 Class - 3 Class - 4 Class - 5 Class - 6 Class - 7 Class - 8 Class - 9 Class-10
Number of images from the training class 10 10 10 10 10 10 10 10 10 10
Number of images from other classes 10 10 10 10 10 10 10 10 10 10
Recognition rate 100% 100% 90% 80% 80% 80% 90% 100% 90% 70%
Table 2: Experiments results for ACON.
In the Figure 3, we have shown all the performance measure and reached goal during 10 different training runs in case of OCON network and also one training phase of ACON network. We set highest epochs 700000, but during the training, in case of all the OCON networks, performance goal was met before reaching maximum number of epochs. All the learning rates with required epochs of OCON and ACON networks are shown at column two of Table 3. In case of the OCON network, if we combine all the recognition rates we have the average recognition rate is 100%. But in case of ACON network, 88% is the average recognition rate i.e.
International Journal of Computer Science and Security (IJCSS), Volume (3): Issue (6)
8
D. Bhattacharjee, M. K. Bhowmik, M. Nasipuri, D. K. Basu & M. Kundu
we can say that OCON showing better performance, accuracy and speed than ACON. Figure 4 presents a comparative study on ACON and OCON results. Total no. of iterations Learning Rate (lr)
-4
Class
Figures
Network Used
290556 248182 260384 293279 275065 251642 273819 263251 295986 257019 Highest epoch reached (7, 00, 000)
lr > 10 lr =10 lr =10
Class – 1 Class – 2 Class – 3 Class - 4 Class - 5 Class – 6 Class – 7 Class – 8 Class – 9 Class - 10 For all Classes (class -1,…,10)
Figure 3(a) Figure 3(b) Figure 3(c) Figure 3(d) Figure 3(e) Figure 3(f) Figure 3(g) Figure 3(h) Figure 3(i) Figure 3(j) Figure 3(k) ACON OCON
-4 -5 -4
lr < 10 lr =10 lr =10 lr =10
-4 -3 -4 -3 -3 -6
lr < 10 lr < 10 lr > 10
Performance goal not met
Table 3: Learning Rate vs. Required Epochs for OCON and ACON.
Figure 3 (a) Class – 1 of OCON Network.
International Journal of Computer Science and Security (IJCSS), Volume (3): Issue (6)
9
D. Bhattacharjee, M. K. Bhowmik, M. Nasipuri, D. K. Basu & M. Kundu
Figure 3 (b) Class – 2 of OCON Network.
Figure 3 (c) Class – 3 of OCON Network.
International Journal of Computer Science and Security (IJCSS), Volume (3): Issue (6)
10
D. Bhattacharjee, M. K. Bhowmik, M. Nasipuri, D. K. Basu & M. Kundu
Figure 3 (d) Class – 4 of OCON Network.
Figure 3 (e) Class – 5 of Ocon Network.
International Journal of Computer Science and Security (IJCSS), Volume (3): Issue (6)
11
D. Bhattacharjee, M. K. Bhowmik, M. Nasipuri, D. K. Basu & M. Kundu
Figure 3 (f) Class – 6 of OCON Network.
Figure 3 (g) Class – 7 of OCON Network.
International Journal of Computer Science and Security (IJCSS), Volume (3): Issue (6)
12
D. Bhattacharjee, M. K. Bhowmik, M. Nasipuri, D. K. Basu & M. Kundu
Figure 3 (h) Class – 8 of OCON Network.
Figure 3 (i) Class – 9 of OCON Network.
International Journal of Computer Science and Security (IJCSS), Volume (3): Issue (6)
13
D. Bhattacharjee, M. K. Bhowmik, M. Nasipuri, D. K. Basu & M. Kundu
Figure 3 (j) Class – 10 of OCON Network.
3 (k) of ACON Network for all the classes.
International Journal of Computer Science and Security (IJCSS), Volume (3): Issue (6)
14
D. Bhattacharjee, M. K. Bhowmik, M. Nasipuri, D. K. Basu & M. Kundu
Figure 4: Graphical Representation of all Recognition Rate using OCON and ACON Network. The OCON is an obvious choice in terms of speed-up and resource utilization. The OCON structure of neural network makes it most suitable for incremental training, i.e., network upgrading upon adding/removing memberships. One may argue that compared to ACON structure, the OCON structure is slow in retrieving time when the number of classes is very large. This is not true because, as the number of classes increases, the number of hidden neurons in the ACON structure also tends to be very large. Therefore ACON is slow. Since the computation time of both OCON and ACON increases as number of classes grows, a linear increasing of computation time is expected in case of OCON, which might be exponential in case of ACON.
5. CONCLUSION
In this paper, two general architectures for a Multilayer Perceptron (MLP) have been demonstrated. The first architecture is All-Class-in-One-Network (ACON) where all the classes are placed in a single network and the second one is One-Class-in-One-Network (OCON) where an individual single network is responsible for each and every class. Capabilities of these two architectures were compared and verified in solving human face recognition, which is a complex pattern recognition task where several factors affect the recognition performance like pose variations, facial expression changes, occlusions, and most importantly illumination changes. Both the structures were implemented and tested for face recognition purpose and experimental results show that the OCON structure performs better than the generally used ACON ones in term of training convergence speed of the network. Moreover, the inherent non-parallel nature of ACON has compelled us to use OCON for the complex pattern recognition task like human face recognition.
ACKNOWLEDGEMENT
Second author is thankful to the project entitled “Development of Techniques for Human Face Based Online Authentication System Phase-I” sponsored by Department of Information Technology under the Ministry of Communications and Information Technology, New Delhi110003, Government of India Vide No. 12(14)/08-ESD, Dated 27/01/2009 at the Department of Computer Science & Engineering, Tripura University-799130, Tripura (West), India for providing
International Journal of Computer Science and Security (IJCSS), Volume (3): Issue (6)
15
D. Bhattacharjee, M. K. Bhowmik, M. Nasipuri, D. K. Basu & M. Kundu
the necessary infrastructural facilities for carrying out this work. The second author is also grateful to the Honorable Vice Chancellor Prof. A. Saha and Prof. B.K. De, Dean of Science of Tripura University (A Central University), Tripura, India for their kind support to carry out this research work.
REFERENCES
1. M. K. Bhowmik, ”Artificial Neural Network as a Soft Computing Tool – A case study”, In Proceedings of National Seminar on Fuzzy Math. & its application, Tripura University, November 25 – 26, 2006, pp: 31 – 46. 2. M. K. Bhowmik, D. Bhattacharjee and M. Nasipuri, “Topological Change in Artificial Neural Network for Human Face Recognition”, In Proceedings of National Seminar on Recent Development in Mathematics and its Application, Tripura University, November 14 – 15, 2008, pp: 43 – 49. 3. A. Abraham, “Artificial Neural Network”, Oklahoma Tate University, Stillwater, OK, USA. 4. https://www.360docs.net/doc/ac2699255.html,/ubiquity/homepage.html. 5. I. Aleksander and H. Morton, “An introduction to Neural Computing,” Chapman & Hall, London, 1990.
6. R. Hecht-Nielsen, “Neurocomputing,” Addison-Wesley, 1990. 7. M. Turk and A. Pentland, “Eigenfaces for recognition”, Journal of Cognitive Neuro-science, March 1991. Vol. 3, No-1, pp. 71-86. 8. L. Sirovich and M. Kirby, “A low-dimensional procedure for the characterization of human faces,” J. Opt. Soc. Amer. A 4(3), pp. 519-524, 1987. 9. A. S. Georghiades, P. N. Belhumeur and D. J. Kriegnab, “From Few to Many: Illumination Cone Models for face Recognition under Variable Lighting and Pose”, IEEE Trans. Pattern Anal. Mach. Intelligence, 2001, vol. 23, No. 6, pp. 643 – 660.
10. D. Bhattacharjee, “Exploiting the potential of computer network to implement neural network in solving complex problem like human face recognition,” Proc. of national Conference on Networking of Machines, Microprocessors, IT, and HRD-need of the nation in the next millennium, Kalyani Engg. College, Kalyani, West Bengal, 1999. 11. Pradeep K. Sinha, “Distributed Operating Systems-Concepts and Design,” PHI, 1998. [8] M. K. Bhowmik, D. Bhattacharjee, M. Nasipuri, D. K. Basu and M. Kundu; “Classification of Fused Face Images using Multilayer Perceptron Neural Network”, proceeding of International Conference on Rough sets, Fuzzy sets and Soft Computing, Nov 5–7, 2009, organized by Department of Mathematics, Tripura University pp. 289-300. 12. M.K. Bhowmik, D. Bhattacharjee, M. Nasipuri, D.K. Basu and M. Kundu, “Classification of Polar-Thermal Eigenfaces using Multilayer Perceptron for Human Face Recognition”, rd proceedings of the 3 IEEE Conference on Industrial and Information Systems (ICIIS-2008), IIT Kharagpur, India, Dec 8-10, 2008, pp. 118. 13. M.K. Bhowmik, D. Bhattacharjee, M. Nasipuri, D.K. Basu and M. Kundu, “Classification of Log-Polar-Visual Eigenfaces using Multilayer Perceptron for Human Face Recognition”, nd proceedings of The 2 International Conference on Soft computing (ICSC-2008), IET, Alwar, Rajasthan, India, Nov 8–10, 2008, pp.107-123.
International Journal of Computer Science and Security (IJCSS), Volume (3): Issue (6)
16
D. Bhattacharjee, M. K. Bhowmik, M. Nasipuri, D. K. Basu & M. Kundu
14. M.K. Bhowmik, D. Bhattacharjee, M. Nasipuri, D.K. Basu and M. Kundu, “Human Face Recognition using Line Features”, proceedings of National Seminar on Recent Advances on Information Technology (RAIT-2009), Indian School of Mines University, Dhanbad, Feb 67,2009, pp. 385-392. 15. P. Raviram, R.S.D. Wahidabanu Implementation of artificial neural network in concurrency control of computer integrated manufacturing (CIM) database, International Journal of Computer Science and Security (IJCSS), Volume 2, Issue 5, pp. 23-25, September/October 2008. 16. Teddy Mantoro, Media A. Ayu, “Toward The Recognition Of User Activity Based On User Location In Ubiquitous Computing Environments,” International Journal of Computer Science and Security (IJCSS)Volume 2, Issue 3, pp. 1-17, May/June 2008. 17. Sambasiva Rao Baragada, S. Ramakrishna, M.S. Rao, S. Purushothaman , “Implementation of Radial Basis Function Neural Network for Image Steganalysis,” International Journal of Computer Science and Security (IJCSS) Volume 2, Issue 1, pp. 12-22, January/February 2008.
International Journal of Computer Science and Security (IJCSS), Volume (3): Issue (6)
17
NMF综述报告
人脸识别的非负矩阵分解(NMF)方法文献综述 摘要:人类对整体的感知是基于对部分的感知,NMF(非负矩阵分解,Non-negative matrix factorization)的思想正是源于此。通过对矩阵分解因子加入了非负性约束,使得对高维非负原始数据矩阵的分解结果不存在负值,且具有一定的稀疏性,因而得到了相对低维、纯加性、拥有一定稀疏特性的分解结果。与PCA(主成分分析,principal components analysis)等传统人脸识别方法相比,NMF的基图像就是人脸的各个局部特征,并且通过对经典算法的一系列优化,改进的NMF算法的识别率和鲁棒性较传统方法有着显著优势。此外,NMF在机器学习、语义理解等领域也有着重要应用。 关键词:非负矩阵分解(NMF)稀疏性改进的NMF 语义理解 一、引言 在实际中的许多数据都具有非负性,而现实中对数据的处理又要求数据的低秩性经典的数据处理方法一般不能够确保非负性的要求,如何找到一个非负的低秩矩阵来近似原数据矩阵成为一个关键问题。在这样的背景下,NMF方法应运而生。 NMF方法思想最早可以追溯到由Paatero和Tapper在1994年提出的正矩阵分解(Positive Matrix Factorization,PMF)[1];此后1999年,Lee和Seung提出了一个以广义KL散度为优化目标函数的基本NMF模型算法,并将其应用于人脸图像表示[2];2001年,Lee和Seung通过对基本NMF算法进行深入研究,又提出了两个经典的NMF算法,即基于欧氏距离测度的乘性迭代算法和基于广义KL散度的乘性迭代算法,并给出了收敛性证明[3],这两种算法称为NMF方法的基准算法,广泛应用于各个领域。 但是在实际应用中,由于经典的基准NMF算法存在收敛速度较慢,未利用统计特征,对光线、遮挡等敏感,以及无法进行增量学习等问题,各种改进的NMF算法被提出。其中包括Lin提出的基于投影梯度(Projected Gradient,PG)的NMF方法[3],该方法有着很高的分解精度;Berry提出的基于投影非负最小二乘(Projected Non-negative Least Square,PNLS)的NMF方法[5],通过这种方法得到的基矩阵的稀疏性、正交性叫基准NMF方法都更好;此外还有牛顿类方法[6]和基于有效集[7]的NMF方法等。 二、NMF的基准算法 1.NMF模型 给定一个非负矩阵(即),和一个正整数,求未知非负矩阵和,使得 用表示逼近误差矩阵。可以用下图表示该过程:
人脸识别英文专业词汇教学提纲
人脸识别英文专业词 汇
gallery set参考图像集 Probe set=test set测试图像集 face rendering Facial Landmark Detection人脸特征点检测 3D Morphable Model 3D形变模型 AAM (Active Appearance Model)主动外观模型Aging modeling老化建模 Aging simulation老化模拟 Analysis by synthesis 综合分析 Aperture stop孔径光标栏 Appearance Feature表观特征 Baseline基准系统 Benchmarking 确定基准 Bidirectional relighting 双向重光照 Camera calibration摄像机标定(校正)Cascade of classifiers 级联分类器 face detection 人脸检测 Facial expression面部表情 Depth of field 景深 Edgelet 小边特征 Eigen light-fields本征光场 Eigenface特征脸 Exposure time曝光时间
Expression editing表情编辑 Expression mapping表情映射 Partial Expression Ratio Image局部表情比率图(,PERI) extrapersonal variations类间变化 Eye localization,眼睛定位 face image acquisition 人脸图像获取 Face aging人脸老化 Face alignment人脸对齐 Face categorization人脸分类 Frontal faces 正面人脸 Face Identification人脸识别 Face recognition vendor test人脸识别供应商测试Face tracking人脸跟踪 Facial action coding system面部动作编码系统 Facial aging面部老化 Facial animation parameters脸部动画参数 Facial expression analysis人脸表情分析 Facial landmark面部特征点 Facial Definition Parameters人脸定义参数 Field of view视场 Focal length焦距 Geometric warping几何扭曲
人脸识别技术发展及应用分析解读
人脸识别技术发展及应用分析 人脸识别是基于人的脸部特征信息进行身份识别的一种生物识别技术。用摄像机采集人脸图像,并自动在图像中检测和跟踪人脸,进而对检测到的人脸进行脸部的一系列相关技术处理,包括人脸图像采集、人脸定位、人脸识别预处理、记忆存储和比对辨识,达到识别不同人身份的目的。 市场现状 人脸识别技术的研究始于20世纪60年代末期。 20世纪90年代后期以来,一些商业性的人脸识 别系统逐渐进入市场,但是,这些技术和系统离 实用化都有一定距离,性能和准确率也有待提高。 美国遭遇恐怖袭击后,这一技术引起了广泛关 注。作为非常容易隐蔽使用的识别技术,人脸识 别逐渐成为国际反恐和安全防范重要的手段之一。 近年来,人脸识别在中国市场,也经历着迅速的 发展,而且发展的脚步也越来越快。主要原因有以下两方面。 科技的进步 国际上,美国标准与技术研究院(NIST)举办的Face Recognition Vendor Test 2006,通过大规模的人脸数据测试表明,当今世界上人脸识别方法的识别 精度比2002年的FRVT2002至少提高了一个数量级(10倍),而对于高清晰,高质量人脸图像识别,机器的识别精度几乎达到100%。在我国,近年来科技界和社会各个方面都认识到人脸识别技术的重要性,国家政策对人脸识别技术研究给予了很大支持,使得我国人脸识别技术也得到了迅速的发展。 应用需求的增加 越来越趋向于高科技的犯罪手段使得人们对各种场合的安全机制要求也近乎 苛刻,各种应用需求不断涌现。人脸识别市场的快速发展一方面归功于生物识别需求的多元化,另一方面则是由于人脸识别技术的进步。从需求上来说,除了传统的考勤、门禁等应用外,视频监控环境下的身份识别正成为一种迫切的需求,
基于matlab人脸识别技术 开题报告
毕业设计(论文)开题报告 毕业设计(论文)课题情况,根据所查阅的文献资料,每人撰写2500字以上的文献综述,文后应列出所查阅的文献资料。 基于matlab人脸识别技术的实现 文献综述 一、MATLAB概述 MATLAB是矩阵实验室(Matrix Laboratory)的简称,是美国MathWorks公司出品的商业数学软件,用于算法开发、数据可视化、数据分析以及数值计算的高级技术计算语言和交互式环境,主要包括MATLAB和Simulink两大部分。MATLAB主要面对科学计算、可视化以及交互式程序设计的高科技计算环境。它将数值分析、矩阵计算、科学数据可视化以及非线性动态系统的建模和仿真等诸多强大功能集成在一个易于使用的视窗环境中,为科学研究、工程设计以及必须进行有效数值计算的众多科学领域提供了一种全面的解决方案,并在很大程度上摆脱了传统非交互式程序设计语言(如C、Fortran)的编辑模式,代表了当今国际科学计算软件的先进水平。应用于工程计算、控制设计、信号处理与通讯、图像处理、信号检测、金融建模设计与分析等领域。而在本文中主要用到的功能是图像处理功能。 二、BP神经网络概述 人工神经网络(Artificial Neural Net works,简写为ANNs)也简称为神经网络(NNs)或称作连接模型(Connectionist Model),它是一种模范动物神经网络行为特征,进行分布式并行信息处理的算法数学模型。这种网络依靠系统的复杂程度,通过调整内部大量节点之间相互连接的关系,从而达到处理信息的目的。 人工神经网络发展的主要历程有:20世纪50年代末,Rosenblatt提出的感知器模型和Widrow提出的自适应线性元件,出现了简单的线性分类器;1986年,Rumelhart和Mcllelland 提出了层网络“误差反向传播算法(BP)”,使有导师学习多层感知器网络(ML PN)模式分类器走向实用化,在此基础上又派生出若干前向网络,如径向基函数网络( RBFN)和函数链网络等;1982年,美国加州工学院的物理学家Hopfield提出的一种用于联想记忆和优化计算的反馈网络模型,由于引进了“能量函数” 的概念,使网络走向具体电路有了保证;20世纪70年代,Watanabe 提出了使用模式子空间的概念来设计不同类别对应的子空间,由不同类别聚类的子空间实现模式识别; Kohonen提出的自组织特征映射网络模型等都为神经网络模式识别理论提供了进一步的根据。 构成人工神经网络的三个基本要素是:神经元、络拓扑结构和网络的训练(学习)方法。神经元(节点)的作用是把若干输入加权求和,并对这种加权和进行非线性处理后输出。神经元的选择一般有以下特点:每个神经元都具有多个输入、个输出,具有闭值,采用非线性函数。 1、神经元
人脸识别发展趋势及应用领域分析
人脸识别发展趋势及应用领域分析 人脸识别技术是一种高精度、易于使用、稳定性高、难仿冒的生物识别技术,具有极其广阔的市场应用前景。在公安、国防、海关、交通、金融、社保、医疗及其他民用安全控制等行业和部门存在着广泛的需求。 一、人脸识别何以瞬间爆发? 其实对于整个生物识别领域来说,由于指纹识别应用时间早,价格低廉,而且使用便利,因此早早地便占据了国内的大部分市场,在顶峰时期,甚至可以达到90%左右。但是,又是什么力量,使人脸识别在短短五六年的时间里,就实现了如此迅猛的腾飞呢? (一)政策因素:抛开技术因素,国家政策的支持可以说是人脸识别崛起的重要因素之一。尤其随着近些年来,平安城市等技术的大力推广,我国安防市场的需求也随之迅速升温,各领域安保的等级也就随之实现明显的提升。甚至在部分地区的部分领域,人脸识别已经开始被列为使用过程中的强制标准。这种情况的出现,对于人脸识别的推广无疑是一种非常有力的推动。因此,虽然政策的角色只是一种诱导的作用,但是这种诱导对于人脸识别的爆发,却又是不可或缺的。 (二)社会需求:在我国,随着城镇化的进程加快,流动人口比例大大增加,面临的突发事件和异常事件越来越复杂。因此这也就给城市的安保工作,以及视频监控带来了更大的压力。传统依靠人工来“盯”的方式难免会因疲劳或精神不集中等原因影响监视效果,难以胜任对庞大数据库的分析与理解,从而影响事后查找证据,更难以满足时代的需求,于是,人们对于具有智能分析的视频监控应用的呼声越来越高。 二、人脸识别发展趋势 (一)与视频监控相结合:随着人脸识别技术的进一步发展,将人脸识别技术将实现与数字监控系统的进一步融合,将成为人脸识别技术的另外一大应用领域。据统计数据显示,仅在中国大陆,在未来三年内有望形成年销售额过百亿,并在未来十年内则有望形成年销售额过千亿的市场规模。(二)逐步取代指纹考勤:人脸识别考勤,通过对人脸一些独一无二的特征识别对验进行考
人脸识别论文文献翻译中英文
人脸识别论文中英文 附录(原文及译文) 翻译原文来自 Thomas David Heseltine BSc. Hons. The University of York Department of Computer Science For the Qualification of PhD. -- September 2005 - 《Face Recognition: Two-Dimensional and Three-Dimensional Techniques》 4 Two-dimensional Face Recognition 4.1 Feature Localization Before discussing the methods of comparing two facial images we now take a brief look at some at the preliminary processes of facial feature alignment. This process typically consists of two stages: face detection and eye localisation. Depending on the application, if the position of the face within the image is known beforehand (for a cooperative subject in a door access system for example) then the face detection stage can often be skipped, as the region of interest is already known. Therefore, we discuss eye localisation here, with a brief discussion of face detection in the literature review(section 3.1.1). The eye localisation method is used to align the 2D face images of the various test sets used throughout this section. However, to ensure that all results presented are representative of the face recognition accuracy and not a product of the performance of the eye localisation routine, all image alignments are manually checked and any errors corrected, prior to testing and evaluation. We detect the position of the eyes within an image using a simple template based method. A training set of manually pre-aligned images of faces is taken, and each image cropped to an area around both eyes. The average image is calculated and used as a template. Figure 4-1 - The average eyes. Used as a template for eye detection. Both eyes are included in a single template, rather than individually searching for each eye in turn, as the characteristic symmetry of the eyes either side of the nose, provides a useful feature that helps distinguish between the eyes and other false positives that may be picked up in the background. Although this method is highly susceptible to scale(i.e. subject distance from the
人脸识别文献综述
文献综述 1 引言 在计算机视觉和模式识别领域,人脸识别技术(Face Recognition Technology,简称FRT)是极具挑战性的课题之一。近年来,随着相关技术的飞速发展和实际需求的日益增长,它已逐渐引起越来越多研究人员的关注。人脸识别在许多领域有实际的和潜在的应用,在诸如证件检验、银行系统、军队安全、安全检查等方面都有相当广阔的应用前景。人脸识别技术用于司法领域,作为辅助手段,进行身份验证,罪犯识别等;用于商业领域,如银行信用卡的身份识别、安全识别系统等等。正是由于人脸识别有着广阔的应用前景,它才越来越成为当前模式识别和人工智能领域的一个研究热点。 虽然人类能够毫不费力的识别出人脸及其表情,但是人脸的机器自动识别仍然是一个高难度的课题。它牵涉到模式识别、图像处理及生理、心理等方面的诸多知识。与指纹、视网膜、虹膜、基因、声音等其他人体生物特征识别系统相比,人脸识别系统更加友好、直接,使用者也没有心理障碍。并且通过人脸的表情/姿态分析,还能获得其他识别系统难以获得的一些信息。 自动人脸识别可以表述为:对给定场景的静态或视频序列图像,利用人脸数据库验证、比对或指认校验场景中存在的人像,同时可以利用其他的间接信息,比如人种、年龄、性别、面部表情、语音等,以减小搜索范围提高识别效率。自上世纪90年代以来,人脸识别研究得到了长足发展,国内外许多知名的理工大学及TT公司都成立了专门的人脸识别研究组,相关的研究综述见文献[1-3]。 本文对近年来自动人脸识别研究进行了综述,分别从人脸识别涉及的理论,人脸检测与定位相关算法及人脸识别核心算法等方面进行了分类整理,并对具有典型意义的方法进行了较为详尽的分析对比。此外,本文还分析介绍了当前人脸识别的优势与困难。 2 人脸识别相关理论 图像是人们出生以来体验最丰富最重要的部分,图像可以以各种各样的形式出现,我们只有意识到不同种类图像的区别,才能更好的理解图像。要建立一套完整的人脸识别系统(Face Recognetion System,简称FRS),必然要综合运用以下几大学科领域的知识: 2.1 数字图像处理技术 数字图像处理又称为计算机图像处理,它是指将图像信号转换成数字信号并利用计算机
2019年我国人脸识别技术发展情况及发展趋势综合分析
2019年我国人脸识别技术发展情况 及发展趋势综合分析 2019年2月14日 一、全球生物识别细行业市场占比情况分析 生物识别指的是通过计算机与光学、声学、生物传感器和生物统计学原理等高科技手段相结合,利用人体固有的生理特性来进行个人身份鉴定技术。按不同的识别方式,生物识别可分为指纹识别、虹膜识别、语音识别、静脉识别和人脸识别。 伴随着生物识别产品逐渐从单一的PC处理转变为分布式计算。 用独立的前端独立设备来完成生物特征的采集、预处理、特征提取和比对,通过中心PC或服务器完成与业务相关的处理。随着生物特征 识别技术的不断发展和提高,生物特征识别技术的应用场景不断拓展,预计2015-2020年全球生物识别细分行业复合增长率分别为:人脸识别复合增长率为167%;语音识别为100%;虹膜识别为100%;指纹识别复合增长率为73%。
全球生物识别细行业市场占比情况 二、中国人脸识别技术发展情况分析 1、中国人脸识别行业发展历程 人脸识别技术在中国的发展起步于上世纪九十年代末,经历了技术引进-专业市场导入-技术完善-技术应用-各行业领域使用等五个阶段。其中,2014年是深度学习应用于人脸识别的关键一年,该年FaceBook发表一篇名为“DeepFace系统:达到肉眼级别的人脸识别系统”(翻译名),之后Face++创始人印奇团队以及香港中文大学汤晓鸥团队均在深度学习结合人脸识别领域取得优异效果,两者在LFW数据集上识别准确度均超过了99%,而肉眼在该数据集上的识别准确度仅为97.52%,可以说深度学习技术让计算机人脸识别能力超越人类的识别程度。
人脸识别与其他生物识别方式相比,优势在于自然性、不被察觉性等特点。自然性即该识别方式同人类进行个体识别时所利用的生物特征相同。指纹识别、虹膜识别等均不具有自然性。不被察觉的特点使该识别方法不易使人抵触,而指纹识别或虹膜识别需利用电子压力传感器或红外线采集指纹、虹膜图像,在采集过程中体验感不佳。目前人脸识别需要解决的难题是在不同场景、脸部遮挡等应用时如何保证识别率。此外,隐私性和安全性也是值得考虑的问题。 2、3D人脸识别与2D人脸识别数据对比 目前,国内的人脸识别技术已经相对发展成熟,该技术越来越多的被推广到安防领域,延伸出考勤机、门禁机等多种产品,产品系列达20多种类型,可以全面覆盖煤矿、楼宇、银行、军队、社会福利 保障、电子商务及安全防务等领域,人脸识别的全面应用时代已经到来。 中游人脸识别技术的进步,是推动下游场景应用拓展的关键所在。目前,人脸识别市场的解决方案主要包括2D识别、3D识别技术。市场上主流的识别方案是采用摄像头的2D方案,但由于人的脸部并非 平坦,因此2D识别在将3D人脸信息平面化投影的过程中存在特征信息损失。3D识别使用三维人脸立体建模方法,可最大程度保留有效 信息,因此3D人脸识别技术的算法比2D算法更合理并拥有更高精度。
人脸识别实验报告解读
人脸识别——特征脸方法 贾东亚12346046 一、实验目的 1、学会使用PCA主成分分析法。 2、初步了解人脸识别的特征法。 3、更熟练地掌握matlab的使用。 二、原理介绍 1、PCA(主成分分析法介绍) 引用一个网上的例子。假设有一份对遥控直升机操作员的调查,用表示飞行员i的 飞行技能,表示飞行员i喜欢飞行的程度。通常遥控直升飞机是很难操作的,只有那些 非常坚持而且真正喜欢驾驶的人才能熟练操作。所以这两个属性和相关性是非常强的。我们可以假设两者的关系是按正比关系变化的。如下图里的任意找的向量u1所示,数据散布在u1两侧,有少许噪声。 现在我们有两项数据,是二维的。那么如何将这两项变量转变为一个来描述飞行员呢?由图中的点的分布可知,如果我们找到一个方向的U,所有的数据点在U的方向上的投影之和最大,那么该U就能表示数据的大致走向。而在垂直于U的方向,各个数据点在该方向 的投影相对于在U上的投影如果足够小,那么我们可以忽略掉各数据在该方向的投影,这 样我们就把二维的数据转化成了在U方向上的一维数据。 为了将u选出来,我们先对数据进行预处理。先求出所有数据的平均值,然后用数据与平均值的偏差代替数据本身。然后对数据归一化以后,再代替数据本身。 而我们求最大的投影和,其实就是求各个数据点在U上的投影距离的方差最大。而X T u 就是投影的距离。故我们要求下式的最大值: 按照u是单位向量来最大化上式,就是求的特征向量。而此式是数据集的协方差矩阵。
在实际应用中,我们不止面临二维的数据。因此不能使用几何的形式呈现,但原理也是一样。就是找到一组相互正交的单位向量,然后根据贡献率考虑选择其中的部分作为考量的维数,这也就实现了数据的降维。 三、实验步骤 1、将库里的400张照片分成两组。一组作为训练,一组作为库。每个人的前五张照片作为 训练,后五张作为库。训练的照片按照顺序的数字重命名。库的照片名字不变。 2、库照片处理。 ①将每一张库的照片转化成N维的向量。(库里的照片是112*92,故将转化成的矩阵按列或行展开,就是个10304维的向量)我们稍后要对如此多维的向量用PCA进行降维。然后把这些向量存入一个矩阵里。而我是将这200个向量以列的形式存在了矩阵里。 即 ,,, ②将这200个向量的每个元素相加起来求出平均值。再用Z里的每一个向量减去这个平均值得到每个的偏差。 平均值,每个向量的偏差 即最后 ,,, ③接下来我们就要针对这些预处理后的数据进行降维。我们要求的N个相互正交的向量就是协方差矩阵的特征向量,而对应的特征值就是各个向量所占的比重。但是Z是个10304*200的矩阵,那么就是个10304*10304的矩阵。使用matlab直接求其特征值与特征向量不太实际。 所以我们考虑一个简单的运算方法: 协方差矩阵的秩受到训练图像的限制:如果有N个训练样本,则最多有N? 1 个对应非零特征值的特征向量,其他的特征向量对应的特征值都是0。如果训练样本的数目比图像的维数低,则可以通过如下方法简化主成份的计算。 设 Z是预处理图像的矩阵,每一列对应一个减去均值图像之后的图像。则,协方差矩阵为,并且对S的特征值分解为
(完整版)2019年我国人脸识别技术发展情况及发展趋势综合分析.doc
2019 年我国人脸识别技术发展情况 及发展趋势综合分析 2019 年 2 月 14 日 一、全球生物识别细行业市场占比情况分析 生物识别指的是通过计算机与光学、声学、生物传感器和生物统计学原理等高科技手段相结合,利用人体固有的生理特性来进行个人身份鉴定技术。按不同的识别方式,生物识别可分为指纹识别、虹膜识别、语音识别、静脉识别和人脸识别。 伴随着生物识别产品逐渐从单一的PC处理转变为分布式计算。用独立的前端独立设备来完成生物特征的采集、预处理、特征提取和比对,通过中心PC或服务器完成与业务相关的处理。随着生物特征 识别技术的不断发展和提高,生物特征识别技术的应用场景不断拓展,预计 2015-2020 年全球生物识别细分行业复合增长率分别为:人脸识别复合增长率为167%;语音识别为 100%;虹膜识别为 100%;指纹识别复合增长率为73%。
全球生物识别细行业市场占比情况 二、中国人脸识别技术发展情况分析 1、中国人脸识别行业发展历程 人脸识别技术在中国的发展起步于上世纪九十年代末,经历了技术引进 - 专业市场导入 - 技术完善 - 技术应用 - 各行业领域使用等五个阶段。其中, 2014 年是深度学习应用于人脸识别的关键一年,该年FaceBook发表一篇名为“ DeepFace系统:达到肉眼级别的人脸识别系统”(翻译名),之后Face++创始人印奇团队以及香港中文大学汤晓鸥团队均在深度学习结合人脸识别领域取得优异效果,两者在LFW数据集上识别准确度均超过了99%,而肉眼在该数据集上的识别准确度仅为 97.52%,可以说深度学习技术让计算机人脸识别能力超 越人类的识别程度。
模式识别文献综述报告
指导老师:马丽 学号:700 班级: 075111 姓名:刘建 成绩: 目录 ............................................................ 一、报告内容要点............................................................ 二、《应用主成分分解(PCA)法的图像融合技术》............................................................ 三、《基于类内加权平均值的模块 PCA 算法》............................................................
四、《PCA-LDA 算法在性别鉴别中的应用》 ............................................................ 五、《一种面向数据学习的快速PCA算法》 ............................................................ 六、《Theory of fractional covariance matrix and its applications in PCA and 2D-PCA》 ............................................................ 七、课程心得体会 ............................................................ 八、参考文献 ............................................................ 一、报告内容要点 ①每篇论文主要使用什么算法实现什么 ②论文有没有对算法做出改进(为什么改进,原算法存在什么问题,改进方法是什么) ③论文中做了什么对比试验,实验结论是什么?可以加入自己的分析和想法,例如这篇论文还存在什么问题或者缺点,这篇论文所作出的改进策略是否好,你自己对算法有没有什么改进的想法? 二、《应用主成分分解(PCA)法的图像融合技术》 第一篇《应用主成分分解(PCA)法的图像融合技术》,作者主要是实现用PCA可以提取图像数据中主要成分这一特点,从元图像获得协方差矩阵的特征值和特征向量,据此确定图像融合算法中的加权系数和最终融合图像。 作者在图像融合的算法上进行改进,用PCA获得待融合的每幅图像的加权系数Wi。是这样实现的:计算待融合的i幅图像数据矩阵的协方差矩阵,从中获
2018年人脸识别行业市场调研分析报告
2018年人脸识别行业市场调研分析报告
目录 1、市场规模不断提升,政策支持力度加强 (5) 1.1、市场规模不断提升 (5) 1.2、政策支持力度不断加强 (7) 1.3、一级市场火热,国内专利不断攀升 (7) 2、 CNN 算法解决识别精度,人脸识别优势明显 (9) 2.1、发展历史悠久, CNN 算法助力识别率大幅提升 (9) 2.2、 2D 人脸识别为主, 3D 人脸识别还未成熟 (11) 2.3、生物识别技术中人脸识别优势明显 (12) 3、行业发展迅速, B 端百亿市场有望率先爆发 (13) 3.1、 B 端增量市场核心动力在于构建大安防体系 (14) 3.2、 C 端市场还未充分打开 (16) 4、多方逐鹿,综合能力至关重要 (18) 4.1、创业公司:基于技术优势切入市场 (19) 4.2、上市公司:技术+资金+渠道、综合实力强劲 (20) 4.3、互联网巨头:C 端影响力强大,探索 B 端落地 (22) 5、行业评级 (23) 6、企业分析 (24) 7、风险提示 (26)
图 1:生物识别领域未来五年复合增长率 (5) 图 2:全球生物识别市场规模(亿美元) (6) 图 3:2007-2015年人脸识别新增专利主要国家分布 (8) 图 4:人脸识别专利总量主要国家分布 (9) 图 5:人脸识别发展阶段 (9) 图 6:人脸识别算法流程 (11) 图 7:移动人脸识别系统 (15) 图 8:How-old-do-i-look (17) 图 9:ibaby婴儿监视器 (18) 图 10:云从科技部分金融案例 (20)
表 1:人脸识别相关政策 (7) 表 2:人脸识别部分企业融资情况 (8) 表 3:2D、3D人脸识别对比实验结果 (12) 表 4:模式识别对比 (13) 表 5:典型应用场景 (13) 表 6:人脸识别相关创业公司 (19) 表 7:人脸识别相关上市公司 (21) 表 8:互联网巨头人脸识别相关布局 (22)
人脸识别系统的原理与发展解读
人脸识别系统的原理与发展 一、引言 人脸识别系统以人脸识别技术为核心,是一项新兴的生物识别技术,是当今国际科技领域攻关的高精尖技术。它广泛采用区域特征分析算法,融合了计算机图像处理技术与生物统计学原理于一体,利用计算机图像处理技术从视频中提取人像特征点,利用生物统计学的原理进行分析建立数学模型,具有广阔的发展前景。2012年4月,铁路部门宣布车站安检区域将安装用于身份识别的高科技安检系统人脸识别系统;可以对人脸明暗侦测,自动调整动态曝光补偿,人脸追踪侦测,自动调整影像放大; 二、概述 人脸识别系统概述 广义的人脸识别实际包括构建人脸识别系统的一系列相关技术,包括人脸图像采集、人脸定位、人脸识别预处理、身份确认以及身份查找等;而狭义的人脸识别特指通过人脸进行身份确认或者身份查找的技术或系统。生物特征识别技术所研究的生物特征包括脸、指纹、手掌纹、虹膜、视网膜、声音(语音)、体形、个人习惯(例如敲击键盘的力度和频率、签字)等,相应的识别技术就有人脸识别、指纹识别、掌纹识别、虹膜识别、视网膜识别、语音识别(用语音识别可以进行身份识别,也可以进行语音内容的识别,只有前者属于生物特征识别技术)、体形识别、键盘敲击识别、签字识别等。 人脸识别系统功能模块 人脸捕获与跟踪功能:人脸捕获是指在一幅图像或视频流的一帧中检测出人像并将人像从背景中分离出来,并自动地将其保存。人像跟踪是指利用人像捕获技术,当指定的人像在摄像头拍摄的范围内移动时自动地对其进行跟踪。 人脸识别比对:人脸识别分核实式和搜索式二种比对模式。核实式是对指将捕获得到的人像或是指定的人像与数据库中已登记的某一对像作比对核实确定其是否为同一人。搜索式的比对是指,从数据库中已登记的所有人像中搜索查找是否有指定的人像存在。 人脸的建模与检索:可以将登记入库的人像数据进行建模提取人脸的特征,并将其生成人脸模板(人脸特征文件)保存到数据库中。在进行人脸搜索时(搜索式),将指定的人像进行建模,再将其与数据库中的所有人的模板相比对识别,最终将根据所比对的相似值列出最相似的人员列表。
人脸检测和识别技术的文献综述
人脸识别技术综述 摘要:在阅读关于人脸检测识别技术方面文献后,本文主要讨论了人脸识别技术的基本介绍、研究历史,人脸检测和人脸识别的主要研究方法,人脸识别技术的应用前景,并且总结了人脸识别技术的优越性和当下研究存在的困难。 关键词:人脸识别;人脸检测;几何特征方法;模板匹配方法;神经网络方法;统计方法;模板匹配;基于外观方法; 随着社会的发展,信息化程度的不断提高,人们对身份鉴别的准确性和实用性提出了更高的要求,传统的身份识别方式已经不能满足这些要求。人脸识别技术(FRT)是当今模式识别和人工智能领域的一个重要研究方向.虽然人脸识别的研究已有很长的历史,各种人脸识别的技术也很多,但由于人脸属于复杂模式而且容易受表情、肤色和衣着的影响,目前还没有一种人脸识别技术是公认快速有效的[1]基于生物特征的身份认证技术是一项新兴的安全技术,也是本世纪最有发展潜力的技术之一[2]。 1. 人脸识别技术基本介绍 人脸识别技术是基于人的脸部特征,一个完整的人脸识别过程一般包括人脸检测和人脸识别两大部分,人脸检测是指计算机在包含有人脸的图像中检测出人脸,并给出人脸所在区域的位置和大小等信息的过程[3],人脸识别就是将待识别的人脸与已知人脸进行比较,得
出相似程度的相关信息。 计算机人脸识别技术也就是利用计算机分析人脸图象, 进而从中出有效的识别信息, 用来“辨认”身份的一门技术.人脸自动识别系统包括三个主要技术环节[4]。首先是图像预处理,由于实际成像系统多少存在不完善的地方以及外界光照条件等因素的影响,在一定程度上增加了图像的噪声,使图像变得模糊、对比度低、区域灰度不平衡等。为了提高图像的质量,保证提取特征的有有效性,进而提高识别系统的识别率,在提取特征之前,有必要对图像进行预处理操作;人脸的检测和定位,即从输入图像中找出人脸及人脸所在的位置,并将人脸从背景中分割出来,对库中所有的人脸图像大小和各器官的位置归一化;最后是对归一化的人脸图像应用人脸识别技术进行特征提取与识别。 2. 人脸识别技术的研究历史 国内关于人脸自动识别的研究始于二十世纪80年代,由于人脸识别系统和视频解码的大量运用,人脸检测的研究才得到了新的发展利用运动、颜色和综合信息等更具有鲁棒性的方法被提出来变形模板,弹性曲线等在特征提取方面的许多进展使得人脸特征的定位变得更为准确。 人脸识别的研究大致可分为四个阶段。第一个阶段以Bertillon,Allen和Parke为代表,主要研究人脸识别所需要的面部特征;第二个阶段是人机交互识别阶段;第三个阶段是真正的机器自动识别阶段;第四个阶段是鲁棒的人脸识别技术的研究阶段。目前,国外多所
人脸识别技术综述解读
人脸识别研究 代上 (河南大学环境与规划学院河南开封 475004) 摘要:现今世界经济发展迅速,而面对繁杂的社会安全问题却显得有些捉襟见肘,人脸识别技术能够因通过面部特征信息识别身份而受到广泛关注。人脸识别通常使用采集含有人脸图像或视频流的设备,将收集到的人脸信息进行脸部检测,进而与数据库中已有信息进行对比确定被识别对象的身份,已经广泛的应用于公共安全、教育等多个方面,且在以后的社会发展中具有很大的应用前景。本文主要对人脸识别的发展历程、主要识别方法予以总结概括,并对其应用范围与发展趋势进行分析。 关键词:人脸识别;方法;应用;发展 1引言 人脸识别是基于人的脸部特征信息进行身份识别的一种生物识别技术。该项技术目前应用到社会的各个领域,例如个人家庭自动门的安全系统、犯罪人的身份识别系统、银行自动取款的服务系统等。 人脸识别系统给人带了很多方便,应用能力很强,但是人脸识别仍然有很多阻碍其发展的困难之处。主要表现在:在收集图像中目标自身的影响;在系统收集图像的过程中容易受到各种外界因素以及系统收集图像之后由于其它因素造成的面部损伤所带来的影响;随着时间的变迁,人的面部逐步发生变化的影响。这些都对人脸识别技术的发展造成了一定的困难,也使得该项技术面临着多种挑战性。 2 人脸识别研究的发展历史与研究现状 2.1发展历史 很早在19世纪80年代就有关于通过人脸对人类的身份进行辨别的论文发表,但是由于技术水平与设备的限制,人脸识别技术并没有受到重视。直到20世纪60年代末,Blcdsoc[1]提出了人脸识别研究的雏形,人脸识别技术才被人们接受。 在人脸识别研究的早期阶段,人们主要研究的是人脸识别的各种方法,但是在实际应用方面却没有得到实质性的进展。 进入20世纪90年代末的时候,人脸识别技术进入了一个快速发展阶段,在这个时期各种新的人脸识别方法相继出现,并创建了人脸图像数据库,对人脸识别的发展起到了巨大的促进作用。在实际应用方面也取得了很大的进展,运用人脸识别技术的产品逐渐进入了社会市场。 进入21世纪以后,人脸识别技术已经逐步发展成熟,但是由于非理想条件如(光照、天气、姿态)的影响,对人脸识别技术的要求也更高。为了解决这些不利因素所造成的影响,研究者们一直努力寻找更加趋于完美的方法,从而减少这些因素所带来的不利影响。 2.2研究现状 近几年来,人脸识别技术已经从以前的认知阶段发展到了实际应用阶段。但是由于每个人的面部都会因为各种不同的原因发生改变,这给人脸识别带来了不小的影响。如光照不同
参考——人脸检测文献综
附件一: 基于肤色的人脸检测系统的研究与实现文献综述一、人脸检测技术研究背景和意义 随着当今世界的发展,各个国家和地区间的人员流动越发频繁,安全部门都希望通过身份自动验证技术对往来人员进行管理,尤其是9.11事件之后,世界各国更是在人员的身份验证方面投入了大量的人力、物力。因此,身份验证技术在安全控制和金融贸易等方面的应用得到了飞速发展。在诸多的身份验证技术中,生物特征识别技术得到了新的重视,被公认为是最具应用潜力的识别技术之一。其中,人脸识别(Face Recognition )技术较其它生物特征识别方法有很大的优越性,特别是在在90 年代,随着微电子和视觉系统方面取得的新进展,使该领域中高性能自动识别技术的实现代价降低到了可以接受的程度。人脸识别从而得到快速发展,并成为研究热点,主要表现在它的方便性、快速性和非侵扰性。我们所研究的人脸检测( Face Detection)正是自动人脸识别系统中的一个关键环节,它是指在输入图像中确定所有人脸(如果存在)的位置和大小的过程【1】。 近几年来,人脸识别越来越广泛的应用于国家安全、金融、海关、民航等诸多领域。这种应用背景要求自动人脸识别系统能够对一般环境图像具有一定的适应能力,由此所面临的一系列问题使得人脸检测开始作为一个独立课题受到研究者的重视。今天,人脸检测的应用背景己经远远超出了人脸识别系统的范畴,在基于内容的检索、数字视频处理、视觉监测等方面有着重要的应用价值。人脸检测是智能人机接口技术研究的基本内容。不仅是智能接口系统的重要功能模块,而且是其他智能感知系统的基础和前提。人脸检测技术为其他感知系统(人脸识别、表情识别、唇读等)的技术研究提供了前提条件,即有效、快速的人脸定位。人脸检测还是其他领域中的一项关键技术,例如、视频会议、基于对象的编码、应用视觉系统(如安全检查、保安管理)、Web搜索、二维人脸合成、视频检索、基于内容的图像检索等。在数字音频领域有广泛影响的MPEG 系列国际标准也在征求人脸检测算法【2】。 二、人脸检测系统的组成 对于人类来说,可以毫不费力地识别出人脸以及每个人脸部位的不同特征,但对于计算机来说,这却是一件比较困难的工作。让机器从复杂的图像中自动检测出人脸覆盖了众多科学领域的知识,如图像处理、模式识别、计算机视觉和神经网络等等。图1.1现实的是人脸检测的一般过程,它主要有图像获取、图像预处理、人脸检测定位、人脸区域验证四大部分组成:
基于图像处理的人脸识别系统
东北大学 硕士学位论文 基于图像处理的人脸识别系统 姓名:周丹 申请学位级别:硕士 专业:控制理论与控制工程指导教师:王建辉 20050701
东北大学硕士学位论文第一章引言 如何能正确识别大量的人并满足实时性要求是迫切需要解决的问题。总之,要让计算机象人一样方便准确地识别大量的人脸尚需不同学科研究领域的科学家共同做出不懈的努力。 1.3模式识别的基本概念 模式识别研究的内容是利用计算机对客观物体进行分类,在错误概率最小的条件下,使识别的结果尽量与客观物体相符合。 在模式识别学科中,就“模式”与“模式类”而言,模式类是一类事物的代表,而“模式”则是某一事物的具体体现,如数字0,l,2,3,4,5,6,7,8,9是模式类,而用户任意手写的一个数字或任意一个印刷数字则是“模式”,是数字的具体化。 在模式识别技术中,被观测的每个对象称为样品,用x表示,样品的数量用N表示。 对于一个样品来说,必须确定一些与识别有关的因素作为研究的根据,侮‘ 个因素称为一个特征。模式就是样品所具有的特征描述。模式的特征集由处于同 一个特征空间的特征向量表示,特征向量的每个元素成为特征,该向量也因此称为特征向量。一般地用小写英文字母x,个特征,则可把X看作一个n维列向量,x榧 Y,z来表示特征。如果一个样品Ⅳ有n该向量x称为特征向量,记作: ’,X月 模式识别问题就是根据x的几个特征来判别模式工属于q,国:,...,∞。类中的 哪一类。 1.4模式识别系统组成 一个典型的模式识别系统如图3.1所示,由数据获取、预处理、特征提取、分类决策及分类器设计五部分组成。一般分为上下两部分。上半部分完成未知类别模式的分类;下半部分属于设计分类器的训练过程,利用样品进行训练,确定分 2 XG 一一
人脸识别门禁方案解读
人脸识别门禁、考勤安全管理系统 建设方案书 深圳市北电正光科技有限公司 日期:2014/3/10
第一部分:门禁方案设计 一、项目描述 传统的门禁管理系统是建立在机械锁、安保人员的增加、简单的报警系统等,如果有不方便管理及出现危险事件及时发现和处理,那么就需要有一套先进、科学、实用、性能稳定可靠的安全防范监控系统来实现。为了更好的进行通道管理及保护财产安全。 出入口控制系统是安全技术防范领域的重要组成部分,是现代信息科技发展的产物,是数字化社会的必然需求,是人们对社会公共安全与日常管理的双重需要。是发展最快的新技术应用之一。而我们所说的出入口控制系统通常是指:采用现代电子与信息技术,在出入口对人和物这两类目标的进、出进行放行、拒绝、记录和报警等操作的控制系统。它是以识别人和物的数字化编码信息、数字化特征信息为技术核心,具有典型的“数字化安防”技术特征。 XXX涉及到公司员工办公环境、客户接待、员工通行的方便及个人财产人生安全场所,所以XXX的通道管理就显得非常之重要。为了保障XXX通道使用的方便性、安全性、高端性,我公司为XXX设计了一套人脸识别门禁控制及通道控制系统。系统设计以科学性、先进性、稳定性、合理可靠性为原则,因此关键硬件设备器材均采用国际国内知名品牌。 本方案根据对XXX实地勘测,并根据实际需求,参照有关国际标准和国家标准,并结合我公司从事过的多个尖端智能安防工程项目所积累的经验,编制而出。若对本方案的内容或其它方面有不详尽之处,我们随时欢迎您的宝贵意见。 二、斯顿洛克人脸识别智能化门禁系统 此方案为企业门禁管理方案,是依据具备国家级发明专利的STONE LOCK人脸识别算法开发的产品: STONE LOCK人脸识别门禁系统,主要特点为智能化、高安全、分布式存储架构、一体式管理。主要应用于高档写字楼、看守所、弹药库等高端、高安全性场所。 (人脸识别门禁实物图)