Intuitionistic fuzzy set vs. fuzzy set application in medical pattern recognition
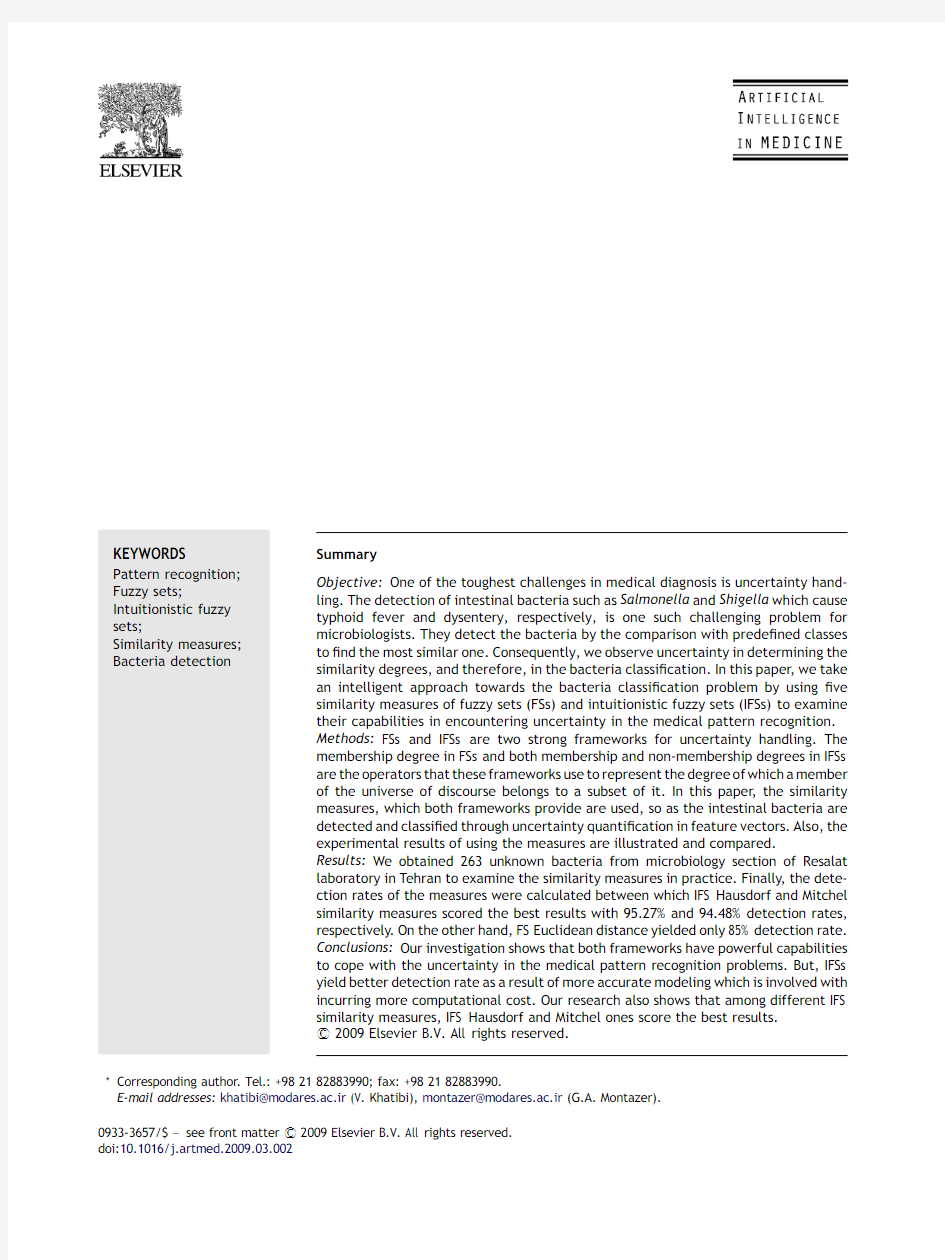

Summary
Objective:One of the toughest challenges in medical diagnosis is uncertainty hand-
ling.The detection of intestinal bacteria such as Salmonella and Shigella which cause
typhoid fever and dysentery,respectively,is one such challenging problem for
microbiologists.They detect the bacteria by the comparison with prede?ned classes
to?nd the most similar one.Consequently,we observe uncertainty in determining the
similarity degrees,and therefore,in the bacteria classi?cation.In this paper,we take
an intelligent approach towards the bacteria classi?cation problem by using?ve
similarity measures of fuzzy sets(FSs)and intuitionistic fuzzy sets(IFSs)to examine
their capabilities in encountering uncertainty in the medical pattern recognition.
Methods:FSs and IFSs are two strong frameworks for uncertainty handling.The
membership degree in FSs and both membership and non-membership degrees in IFSs
are the operators that these frameworks use to represent the degree of which a member
of the universe of discourse belongs to a subset of it.In this paper,the similarity
measures,which both frameworks provide are used,so as the intestinal bacteria are
detected and classi?ed through uncertainty quanti?cation in feature vectors.Also,the
experimental results of using the measures are illustrated and compared.
Results:We obtained263unknown bacteria from microbiology section of Resalat
laboratory in T ehran to examine the similarity measures in practice.Finally,the dete-
ction rates of the measures were calculated between which IFS Hausdorf and Mitchel
similarity measures scored the best results with95.27%and94.48%detection rates,
respectively.On the other hand,FS Euclidean distance yielded only85%detection rate.
Conclusions:Our investigation shows that both frameworks have powerful capabilities
to cope with the uncertainty in the medical pattern recognition problems.But,IFSs
yield better detection rate as a result of more accurate modeling which is involved with
incurring more computational cost.Our research also shows that among different IFS
similarity measures,IFS Hausdorf and Mitchel ones score the best results.
#2009Elsevier B.V.All rights reserved.
*Corresponding author.Tel.:+982182883990;fax:+982182883990.
E-mail addresses:khatibi@modares.ac.ir(V.Khatibi),montazer@modares.ac.ir(G.A.Montazer).
0933-3657/$—see front matter#2009Elsevier B.V.All rights reserved.
doi:10.1016/j.artmed.2009.03.002
1.Introduction
Uncertainty is an inseparable aspect of medical diagnosis problems[1,2].A symptom is an uncertain indication of a disease as it may or may not occur with the disease.Uncertainty characterizes a rela-tion between symptoms and diseases[1—3].Hence, coping ef?ciently with uncertainty leads us to more accurate decision-making and this is considered as a fundamental challenge in medicine.
Fuzzy set(FS),proposed by Zadeh[4],as a frame-work to encounter uncertainty,vagueness and par-tial truth,represents a degree of membership for each member of the universe of discourse to a subset of it.Therefore,we have a spectrum of truth values.By adding the degree of non-membership to FS,Atanassov proposed intuitionistic fuzzy set(IFS) [5]which looks more accurately to uncertainty quanti?cation and provides the opportunity to pre-cisely model the problem based on the existing knowledge and observations[6].Both of these fra-meworks are considered as soft methods which in turn,lead to soft computing and approximate rea-soning[7].
In most of the medical diagnosis problems,there exist some base patterns,and experts make deci-sions based on the similarity between unknown sample and the base patterns[8,9].Medical diag-nosis problems are inherently involved with uncer-tainty,so fuzzy approach could be appropriate to envisage these problems[6].On the other hand,the uncertainty is not just in the judgment,but also in the identi?cation.Hence,there is a need for a kind of fuzzy sets which could support the latter uncer-tainty.The concept of type II fuzzy sets provide such tools through interval valued fuzzy sets and intui-tionistic fuzzy sets as two speci?c implementations. Each of the mentioned sets has its advantages.The interval valued fuzzy sets demonstrate the uncer-tainty in the membership degree by representing an interval for it,but the IFS reveals the uncertainty by a non-membership degree.IFS is the appropriate choice when representation of the non-membership degree is simpler than the membership degree[10]. Also,Atanassov and Gargov[11]further generalized IFS in the spirit of ordinary interval-valued fuzzy sets and de?ned the notion of interval-valued intuitio-nistic fuzzy set(IVIFS).
The main advantages of using the IFS framework are[12]:
By using IFSs,we transform a vague pattern clas-si?cation problem into a precise and well-de?ned optimization problem.
IFSs,unlike ordinary FSs,retain a controlled degree of the uncertainty.
The main disadvantage of using the IFS frame-work is[12]:
The relatively high computational complexity.
Also,the intuitionistic fuzzy set theory has inde-pendently introduced by Takeuti and Titani[13]as a set theory developed in(a kind of)intuitionistic logic.Takeuti—Titani’s intuitionistic fuzzy logic is simply an extension of intuitionistic logic[14].Even if their interpretive settings and motivation are quite different,Atanassov’s construct is isomorphic to interval-valued fuzzy sets and other similar notions,the latter captures the idea of ill-known membership grade,while the former starts from the idea of evaluating degrees of membership and non-membership independently.The main objection to the terminology used by Atanassov is raised from the fact that he calls‘‘intuitionistic fuzzy set theory’’something which accepts rules and principles(such as the double negation)that,added to the intuitio-nistic logic,make it classical,i.e.nothing from intuitionism remains.In this way,calling the Ata-nassov’s theory intuitionistic leads to a misunder-standing.Hence,a solution is proposed to use the terms such as uncertain,imprecise,vague or bipolar fuzzy sets instead of intuitionistic fuzzy sets and replace intuitionistic fuzzy logic with neutrosophic logic which is in line with the terminology in this area and does not borrow its name from another ?eld(intuitionistic logic)[10].
Among diverse medical diagnosis problems,the bacteria detection is considered as a key issue in the microbiology[15].Detecting intestinal bacteria such as Shigella,Salmonella,Klebsiella,and Bacil-lus coli is an important task of microbiologists, because each of them could originate speci?c dis-eases.Salmonella and Shigella are the causes of typhoid fever and dysentery,respectively[16].
Microbiologists detect the bacteria by the special experiments to investigate their features similari-ties with the prede?ned classes’features[17].But, the detection is involved with uncertainty,so as we could not assign an unknown pattern to a speci?c class certainly.
In this paper,we applied the similarity measures of FSs and IFSs to the bacteria detection problem to examine their capabilities to cope with uncertainty in the medical pattern recognition.
The organization of this paper is as follows:in Section2,the bacteria classi?cation and the intest-inal bacteria considered in this paper are described. Then,in Section3,FS,IFS and their respective simi-larity measures are represented.In Section4,we use these tools to solve a bacteria detection problem,and then,illustrate their results comparison.
44V.Khatibi,G.A.Montazer
2.Bacteria classi?cation
Microbiologists broadly classify bacteria according to their shapes[17,18].Most bacteria come in one of three shapes:rod,sphere,or spiral.Rod-shaped bacteria are called bacilli.Spherical bacteria are called cocci,and spiral or cockscrew-shaped ones are called spirilla.Some bacteria come in more complex shapes, e.g.plemorphic bacteria can assume a variety of shapes.It must be noted that the bacterial cell wall generally determines the shape of the bacterial cell.Bacteria may be further classi?ed according to whether they require oxygen and how they react to a test with Gram’s stain. Bacteria in which alcohol washes away Gram’s stain are called Gram-negative,while bacteria in which alcohol cause the bacteria’s wall to absorb the stain, are called Gram-positive.Another system for bac-teria classi?cation depends on source of carbon which the bacteria are fed on(autotrophic or het-erotrophic)[17,18].
As noted before,bacillus is a rod-shaped bacter-ium which is active only in the presence of the oxygen(aerobic bacterium).Bacilli occur mainly in chains,produce spores,and include many sapro-phytes,some parasites,and the bacterium that causes anthrax[19].In this research,four intestinal bacteria of bacillus category named Shigella,Sal-monella,Bacillus coli and Klebsiella,shown in Fig.1,are considered which have some similarity in culture medium and are Gram-negative.
Shigella[19]is a rod-shaped Gram-negative bac-terium(bacillus)that lives in the intestinal tracts of human beings and animals and causes bacillary dysentery.There are four species,all causing dys-entery,but with varying degrees of severity.Salmo-nella[19]is also a rod-shaped bacterium,found in the intestine that can cause food poisoning,gastro-enteritis,and typhoid fever.Other two bacteria considered herein,Klebsiella and Bacillus coli are also Gram-negative and found in the intestine.
The bacteria detection is a microbiologists’task. They use experiments such as the Gram’s stain to classify unknown bacteria[17,18].Hence,they spend much time and energy to detect bacteria, whereas this repetitive task could be done by an expert system.To replace a human expert,the system exploits respective expertise in the pattern recognition.Our aim is to use FS and IFS similarity measures in the bacteria detection to examine their capabilities in encountering uncertainty in the med-ical pattern recognition.
Primal features used for the bacteria classi?ca-tion herein comprise of the macroshape,which is domical in the four studied bacteria,the single microscopic shape,the double microscopic shape, the colony size,and existence of the?agellum.In the next section,we represent the fundamental concepts of FS,IFS and their respective similarity measures which are applied to a bacteria detection problem in following.
3.Fuzzy sets,intuitionistic fuzzy sets, and their similarity measures
Fuzzy sets theory,proposed by Zadeh[4]in1965,has shown successful applications in various?elds.In this theory,the membership of an element to a fuzzy set is a single value between zero and one.But in reality,it may not always be certain that the degree of non-membership of an element to a fuzzy set is just equal to1minus the degree of membership,i.e.there may be some hesitation degree.So,as a generalization of fuzzy sets,the concept of intuitionistic fuzzy sets was introduced by Atanassov[5]in1986.Bustince and Burillo[20]showed that this notion coincides with the notion of vague sets(VSs).
The IFS was de?ned as an extension of the ordin-ary FS[21].As opposed to a fuzzy set in X,given by A?fex;m AexTTj x2X g(1)
where m A:X![0,1]is the membership function of the fuzzy set A,an intuitionistic fuzzy set B is given by
B?fex;m BexT;n BexTTj x2X g(2)
where m B:X![0,1]and n B:X!?0;1 are such that: 0m Btn B1(3)
and m B;n B2?0;1 denote degrees of membership and non-membership of x2A,respectively.For each
Intuitionistic fuzzy set vs.fuzzy set application
45 Figure1Four intestinal bacteria considered in the
detection problem.
intuitionistic fuzzy set B in X,‘‘hesitation margin’’(or‘‘intuitionistic fuzzy index’’)of x2B is given by p B?1àm Bàn B(4) which expresses a hesitation degree of whether x belongs to B or not.It is obvious that0p B(x)1, for each x2X.The illustration of these degrees has been exhibited in Fig.2.
Therefore,to describe an intuitionistic fuzzy set completely;we need at least two functions from the triplet[21,22]:
membership function;
non-membership function;
hesitation margin.
In other words,using IFS introduces another degree of freedom into a set description(i.e.in addition to m B,we also have n B or p B).Since the intuitionistic fuzzy sets are generalized from fuzzy sets and they give us an additional possibility to represent imper-fect knowledge,they can make it possible to describe many real problems in a more adequate way[6,23].
Similarity and distance measures as two main pattern recognition mechanisms can be considered as dual concepts[24].As important subjects in intuitionistic fuzzy mathematics,similarity and dis-tance measures between IFSs have attracted many researchers.Szmidt and Kacprzyk[25]proposed four distance measures between IFSs,which were in some extent based on the geometric interpreta-tion of intuitionistic fuzzy sets,and have some good geometric properties.
Let A?fex;m AexT;n AexTTx2X
j g and B?fex;m BexT;n BexTTx2X
j g be two IFSs in X?f x1;x2;...;x n g.Based on the geometric inter-pretation of IFS,Szmidt and Kacprzyk proposed the following distance measures between A and B:
Hamming distance:
d1
IFS
eA;BT?
1
2
X n
i?1
em Aex iTàm Bex iT
j j
tn Aex iTàn Bex iT
j j
tp Aex iTàp Bex iT
j jT(5) Euclidean distance:
whereas their respective measures in fuzzy sets are represented with:
Hamming distance:
d1FSeA;BT?
1
2
X n
i?1
em Aex iTàm Bex iT
j jT(7) Euclidean distance:
e1
FS
eA;BT?
????????????????????????????????????????????????
1
2
X n
i?1
em Aex iTàm Bex iTT2
v u
u t
(8)
Li and Cheng proposed some IFS similarity mea-sures and applied them to pattern recognition problems[26].For A and B as IFSs in which x2[a,b],Li and Cheng[27]proposed the follow-ing similarity measure:
which Liu[28]modi?ed it to:
But Liang and Shi[29]and Mitchell[30]pointed out that Li and Cheng’s measures are not always effective,and made some modi?cations, respectively.Also,Dengfeng and Chantian pro-posed a similarity measure between the two
46V.Khatibi,G.A.Montazer
àn Bex iTT2tep Aex iTàp Bex iTT2(6)
T c L eA;BT?1à
??????????????????????????????????????????????????????????????????????????????????????????????????????????
1
ebàaT
Z b
a
em AexTàm BexTTten AexTàn BexTT
2
!p
dx
p
s
(9)
T ceA;BT?1à
???????????????????????????????????????????????????????????????????????????????????????????????????????????????????????????????????????????????????1
2ebàaT
Z b
a
m AexTàm BexT
j j ptn AexTàn BexT
j j ptp AexTàp BexT
j j p
??
dx
p
s
(10)
IFSs,A and B as follows:
S p d eA;BT?1à
1
???
n
p
p
?????????????????????????????????????????
X n
i?1
m AeiTàm BeiT
j j p
p
v u
u t
(11)
where m AeiT?em Aex iTt1àn Aex iTT=2;m BeiT?em Bex iTt1àn Bex iTT=2;and1p<1.
Also,Liang and Shi[29]proposed the following similarity measure between IFSs.Let F tAB(i)=j m A(x i)àm B(x i)j/2and F fABeiT??e1à
j
n Aex iTT=2 à?e1àn Bex iTT=2 j.They used(F tA-B
(i)+F fAB(i))p to measure the distance between ?m Aex iT;1àn Aex iT and?m Bex iT;1àn Bex iT .Thus, the distance between IFSs A and B was given by
D p eeA;BT?
1
???
n
p
p
?????????????????????????????????????????????????
X n
i?1
eF tABeiTtF fABeiTTp
p
v u
u t
(12)
and S p eeA;BT?1àD p eeA;BTwas de?ned as a simi-larity measure between A and B.Also,Mitchell [30]adopted a statistical approach and inter-preted IFSs as ensembles of ordered fuzzy sets to modify Li and Cheng’s similarity measure.Let r m(A,B)and r neA;BTdenote the similarity mea-sures between the‘‘low’’membership functions m A and m B and between the‘‘high’’membership functions1ày A and1ày B,respectively,as follows:
r meA;BT?Sem A;m BT
?1à
1
???
n
p
p
????????????????????????????????????????????
X n
i?1
m Aex iTàm Bex iT
j j p
p
v u
u t
;(13)
and
r neA;BT?Se1àn A;1àn BT
?1à
1
???
n
p
p
??????????????????????????????????????????
X n
i?1
n Aex iTàn Bex iT
j j p
p
v u
u t
:(14)
He then,de?ned the modi?ed similarity measure
between A and B with:
S modeA;BT?
1
2
er meA;BTtr neA;BTT(15)
Hung and Yang[31]suggested some similarity
measures between IFSs.First,they use the idea
of Hausdorf distance to de?ne the distance
between IFSs A and B as follows:
d HeA;BT
?
1
n
X n
i?1
max f m Aex iTàm Bex iT
j j;n Aex iTàn Bex iT
j jg:
(16T
Furthermore,they used the distance d H to gen-
erate three similarity measures:
S leA;BT?1àd HeA;BT;(17)
S eeA;BT?
expeàd HeA;BTTàexpeà1T
1àexpeà1T
;(18)
S ceA;BT?
1àd HeA;BT
1td HeA;BT
:(19)
We can utilize the IFSs correlation measures or
association coef?cients to investigate the similar-
ity as well.Recently,a new IFS association coef?-
cient has been represented[32].Let us consider a
discrete universe of discourse,X={x1,x,...,x n},
and let A,B2F(X),then the association coef?-
cient of A and B can be de?ned as
The larger the value of c(A,B),the more the asso-
ciation between A and B.Clearly,the association
coef?cient derived from Eq.(20)takes into
account all the three parameters(membership,
non-membership,and hesitation degrees)describ-
ing IFS,and thus contains the most information.
4.FS vs.IFS in bacteria detection
As noted in Section2,microbiologists generally rely on
the experimental results such as Gram’s stain to
classify the bacteria.We extract the knowledge and
expertise of microbiologists regarding the features of
the studied intestinal bacteria to propose a soft
method for their detection.For this purpose,?rst
we obtained263unknown bacteria from microbiology
section of Resalat laboratory in Tehran.Then,exis-
Intuitionistic fuzzy set vs.fuzzy set application
47
Figure2Membership,non-membership,and hesitation
degrees.
(20)
tence of the aforementioned features was investi-gated in the unknown bacteria.Consequently ,the degrees of membership and non-membership for the features of unknown bacteria were formed.In fact,based on the physical evidence of feature exis-tence in the bacteria by microscopic investigation or experiments,microbiologists determined the mem-bership and non-membership values of the bacteria features.Some part of our data set has been exhibited in T able 1.Based on the acquired knowledge from microbiologists,values of the features in the four studied bacteria were determined which are shown in T able 2.The membership and non-membership functions for each feature indicate the feature’s exis-tence and non-existence degrees in a speci?c class,respectively.
For the colony size,no constant values were observed for membership and non-membership degrees.Instead,according to the colony size,var-iant behaviors for these functions were obtained.To set equations for these functions,several regression functions were examined to ?t the points,and at last,the following polynomial functions resulted in the best ?tness:
m eColony Size T?à0:23x 2t0:98x à0:1849(21)n eColony Size T?0:24x 2à0:97x t1:1349
(22)
The colony size membership and non-membership function curves have been shown in Figs.3and 4,respectively.To detect unknown bacteria,?rst,the fuzzy Euclidean distance was used to determine the most similar class to an unknown sample.For instance,according to Eq.(8),to compare unknown sample S 1with Shigella class,we have:Fuzzy Euc eS 1;Shigella T
????????????????????????????????????????????????????????????12X n i ?1
em Shigella ex i Tàm S 1ex i TT2
v u u t (23)
Then,the IFS Euclidean distance measure was applied to the bacteria detection problem to deter-mine the most similar class for an unknown sample.For instance,based on Eq.(6),we have:
Also,three IFS similarity measures of Mitchel,Hausdorf and Xu et al.have been applied to this problem to examine their capabilities to cope with the uncertainty.For instance,according to Eqs.(13)—(15)for Mitchel similarity measure which is itself a modi?ed version of Dengfeng and Chantian measure,we have:
r m eS 1;Shigella T?S em S 1;m Shigella T
?1à1???n p
p ????????????????????????????????????????????????????????X n
i ?1
m S 1ex i Tàm Shigella ex i T p p v u u t ;(25)48
V .Khatibi,G.A.Montazer
Table 1Some part of the data set.Sample
Domical shape Single microscopic shape Double
microscopic shape Flagellum m (A i )
n eA i Tm (B i )n eB i Tm (C i )n eC i Tm (D i )n eD i TS 10.8370.1330.7180.1590.0640.8970.0210.806S 20.9110.0290.8310.0310.0280.8940.9520.036S 30.9290.0370.8120.0330.0210.9260.0540.922S 40.8150.0910.9490.0480.0200.8800.8330.042S 50.8640.020.6100.2300.2430.6240.00040.964S 6
0.905
0.016
0.878
0.015
0.072
0.917
0.789
0.114
Table 2The features’values in the studied bacteria classes (base patterns).Classes
Domical shape
Single
microscopic shape Double
microscopic shape
Flagellum
m (A i )
n eA i Tm (B i )n eB i Tm (C i )n eC i Tm (D i )n eD i TBacillus coli 0.850.050.870.010.020.970.920.06Shigella 0.830.080.920.050.050.920.080.91Salmonella 0.790.120.780.110.110.850.870.01Klebsiella
0.82
0.15
0.72
0.15
0.22
0.75
0.12
0.85
ten Shigella ex i Tàn S 1ex i TT2tep Shigella ex i Tàp S 1ex i TT2
(24)
and
r n eS 1;Shigella T?S e1àn S 1;1àn Shigella T
?1à1???n p
p ??????????????????????????????????????????????????????X n
i ?1n S 1ex i Tàn Shigella ex i T p p v u u t ;(26)
where assuming p =1:
S mod eA ;B T?1
2
er m eA ;B Ttr n eA ;B TT:
(27)
Fig.5illustrates and compares the detection per-formance of the fuzzy Euclidean distance,IFS Eucli-dean distance and IFS Mitchel similarity measures.
Also,the comparison of the four IFS measures’results against the microbiologists’opinions has been repre-sented in Fig.6.T able 3summarizes the applied approaches’results and compares them with the microbiologists’opinions.
Having applied the ?ve aforementioned measures to all the unknown bacteria,fuzzy Euclidean dis-tance measure could distinguish 85%of the samples properly,whereas,IFS Euclidean distance scored a better result and detected 87%of the samples cor-rectly.Also,the IFS Mitchel and Hausdorf similarity measures even provided us with more accurate classi?cation,so as they could detect 94.48%and 95.27%of the samples properly,respectively.All the three Hausdorf similarity measures Eqs.(15)—(17),were applied to the problem which brought us
Intuitionistic fuzzy set vs.fuzzy set application
49
Figure 4The colony size non-membership
function.
Figure 3The colony size membership
function.
Figure 5The performance comparison of the three approaches in the bacteria detection:(a)fuzzy Euclidean distance,(b)IFS Mitchel similarity,and (c)IFS Euclidean distance.
50V .Khatibi,G.A.
Montazer
Figure 6The comparison of the four approaches with microbiologists’opinions:(a)fuzzy Euclidean distance,(b)IFS Euclidean distance,(c)IFS Mitchel similarity,and (d)IFS Hausdorf similarity.
Table 3Classi?cation results based on the FS and IFS measures vs.microbiologists’opinions.S.No.Measure
Bacillus coli Shigella Salmonella Klebsiella Microbiologists S 1
FS Euclidean distance 0.214 1.2600.2560.988Bacillus coli
IFS Euclidean distance 0.267 1.7870.353 1.360IFS Hausdorf similarity 0.9190.5930.8890.659S 2FS Euclidean distance 1.1780.190 1.1720.764Shigella
IFS Euclidean distance 1.7210.306 1.734 1.128IFS Hausdorf similarity 0.5780.9010.5980.766S 3FS Euclidean distance 0.212 1.2480.1450.923Salmonella
IFS Euclidean distance 0.288 1.7770.201 1.273IFS Hausdorf similarity 0.9010.5920.9390.744S 4FS Euclidean distance 1.0980.625 1.0590.287Klebsiella
IFS Euclidean distance 1.5880.913 1.5630.424IFS Hausdorf similarity 0.5790.7530.6290.862S 5FS Euclidean distance 0.114 1.1910.2070.950Bacillus coli
IFS Euclidean distance 0.146 1.7010.307 1.315IFS Hausdorf similarity 0.9680.6220.8980.671S 6FS Euclidean distance 1.2030.1425 1.2120.819Shigella
IFS Euclidean distance 1.7370.211 1.773 1.158IFS Hausdorf similarity
0.616
0.956
0.584
0.722
95.27%,94.4%,and86.6%detection rates,respec-tively.Also,the Xu et al.association coef?cient approach was examined to classify the unknown bacteria,but it yielded the weak detection rate of86.61%.
Considering non-membership function and incurring more computational cost in IFS frame-work led us to better results.Also,the errors in misclassi?ed samples of IFS Hausdorf similarity measure resided in the interval[0.003,0.07], whereas the same errors were in the intervals [0.007,0.7]and[0.01,0.09]for IFS and fuzzy Euclidean distance measures,respectively.This implies utilizing the IFS framework not only pro-vides us with more accurate classi?cation results, but also,the related errors are less than that the fuzzy framework generates.
5.Conclusions
Soft methods which deal with uncertainty,vague-ness and partial truth,provide us with better tol-erance to cope with uncertainty in the medical diagnosis problems.The bacteria detection by soft computing was undertaken in this paper.For this purpose,different similarity measures of FSs and IFSs were applied to a bacteria classi?cation pro-blem to investigate their capabilities to encounter the uncertainty in the medical pattern recognition.
IFSs yielded more accurate classi?cation results than FSs,so as95.27%of unknown samples were classi?ed correctly by IFS Hausdorf similarity mea-sure,while FS Euclidean distance measure detected 85%of the samples correctly.The cause of this phenomenon resides in the nature of the IFS frame-work.It considers the degree of non-membership and this leads to better detection rate,but it con-veys a drawback of more computational cost. Acknowledgements
The authors would like to thank Ms.Hoda Davarzani, Ph.D.student at the Tarbiat Modares University,for her precious assistance in data analysis of our research.This work was supported by the Iran Tele-communications Research Center(ITRC),Tehran, Iran,under grant no.TMU87-07-48. References
[1]De SK,Biswas A,Roy R.An application of intuitionistic fuzzy
sets in medical diagnosis.Fuzzy Sets and Systems2001;117(2): 209—13.
[2]Straszecka https://www.360docs.net/doc/5d14216269.html,bining uncertainty and imprecision in
models of medical https://www.360docs.net/doc/5d14216269.html,rmation Sciences 2007;176:3026—59.
[3]Han J,Kamber M.Data mining:concepts and techniques.
Morgan,San Mateo,CA:Elsevier,Kaufmann;2006. [4]Zadeh LA.Fuzzy https://www.360docs.net/doc/5d14216269.html,rmation Control1965;8:
338—53.
[5]Atanassov K.Intuitionistic fuzzy sets.Fuzzy Sets and Systems
1986;20(1):87—96.
[6]Vlachos IK,Sergiadis GD.Intuitionistic fuzzy information:
applications to pattern recognition.Pattern Recognition Letters2007;28:197—206.
[7]Zadeh LA.Fuzzy logic,neural networks,and soft computing.
Communications of ACM1994;37:77—84.
[8]Meyer-Base A.Neuro-fuzzy classi?cation.Pattern Recogni-
tion in Medical Imaging2004;282—317.
[9]Wolff JG.Medical diagnosis as pattern recognition in a
framework of information compression by multiple align-ment,uni?cation and search.Decision Support Systems 2006;42:608—25.
[10]Dubois D,Gottwald S,Ha-jek P,Kacprzyk J,Prade H.Termi-
nological dif?culties in fuzzy set theory–—the case of intui-tionistic fuzzy sets.Fuzzy Sets and Systems2005;156:485—
91.
[11]Atanassov K,Gargov G.Interval valued intuitionistic fuzzy
sets.Fuzzy Sets and Systems1989;31:343—9.
[12]Mitchell HB.Pattern recognition using type-II fuzzy sets.
Information Sciences2005;170:409—18.
[13]Takeuti G,Titani S.Intuitionistic fuzzy logic and intuitionis-
tic fuzzy set theory.Journal of Symbolic Logic1984;49:851—
66.
[14]Dalen DV.Intuitionistic logic,handbook of philosophical
logic.Dordrecht:Kluwer;2002.
[15]Singleton P.Bacteria in biology,biotechnology,and medi-
cine.Chichester:Wiley;1999.
[16]Garrett L.The coming plague:newly emerging di-
seases in a world out of balance.New York:Penguin Group;1994.
[17]Facklam H,Facklam M.Bacteria.Kent:T wenty-First Cen-
tury;1995.
[18]Ricciuti ER.Microorganisms:the unseen world.San Diego:
Blackbirch;1993.
[19]Sankaran N.Microbes and people:an A—Z of microorganisms
in our lives.Phoneix:Oryx;2001.
[20]Bustince H,Burillo P.Vague sets are intuitionistic fuzzy sets.
Fuzzy Sets and Systems1996;79(3):403—5.
[21]Atanassov KT.Intuitionistic fuzzy sets.Heidelberg:Physica-
Verlag;1999.
[22]Atanassov K.More on intuitionistic fuzzy sets.Fuzzy Sets and
Systems1989;33(1):37—46.
[23]Abbas SE.On intuitionistic fuzzy https://www.360docs.net/doc/5d14216269.html,rmation
Sciences2005;173:75—91.
[24]Wang W,Xin X.Distance measure between intuitionis-
tic fuzzy sets.Pattern Recognition Letters2005;26:2063—9.
[25]Szmidt E,Kacprzyk J.Distances between intuitioni-
stic fuzzy sets.Fuzzy Sets and Systems2000;114(3):505—
18.
[26]Li D,Cheng C.New similarity measures of intuitionistic fuzzy
sets and application to pattern recognition.Pattern Recog-nition Letters2002;23(1-3):221—5.
[27]Li DF,Cheng CT.New similarity measures of intuitionistic
fuzzy sets and application to pattern recognition.Pattern Recognition Letters2002;23:221—5.
[28]Liu H-W.New similarity measures between intuitionistic
fuzzy sets and between elements.Mathematical and Com-puter Modelling2005;42:61—70.
Intuitionistic fuzzy set vs.fuzzy set application51
[29]Liang Z,Shi P.Similarity measures for intuitionistic fuzzy
sets.Pattern Recognition Letters2003;24:2687—93. [30]Mitchell HB.On the Dengfeng—Chuntian similarity measure
and its application to pattern recognition.Pattern Recogni-tion Letters2003;24:3101—4.[31]Hung W-L,Yang M-S.Similarity measures of intuitionistic
fuzzy sets based on Hausdorff distance.Pattern Recognition Letters2004;25:1603—11.
[32]Xu Z,Chen J,Wuc J.Clustering algorithm for intuitionistic
fuzzy https://www.360docs.net/doc/5d14216269.html,rmation Sciences2008;178:3775—90.
52V.Khatibi,G.A.Montazer
找等量关系列方程专题练习
一、填空 1、a×b×6的简便写法是() 2、甲数是12.5,比乙数的x倍少6,乙数是() 3、四(2)班有男生a人,比女生多6人,这个班共有学生()人。 4、30盒饼干共花了 a元,平均每盒饼干()元。 5、小丽有a块巧克力,给妹妹2块后,两人就同样多,原来妹妹有()块 6、三个连续自然数,中间的数是m, 两个数是()() 7、三个连续偶数,中间的数是n,它们的和是() 8、……摆一个正方形需要4根小棒,摆2个正方形需要7根小棒,3个需要 10根……摆n个正方形需要()小棒 9、一个两位数,十位上的数字为a,个位上的数字为b,用字母式子表示这个两位数是() 二、看图找出等量关系,列方程 方程一: 方程二:(挑战试一试) 三、根据题意找出等量关系,列方程。 【基础部分】注:一般在列方程时,未知数要参与运算。 1.小明原有一些故事书,送给小红4本,妈妈又给他买了9本,现在还有56本,小明原有故事书多少本? 解:设 3、大楼高29.2米,一楼准备开商店,商店层高4米,上面9层是住宅。住宅每层高多少米? 解:设2、一块长方形菜地的面积是180平方米,它的宽是12米,长是多少米? 解:设 4、猎豹是世界最快的动物,能达到每小时110km,比大象的2倍还多30km。大象最快能达到每小时多少千米? 解:设 5、一辆双层巴士共有乘客51人,下层人数是上层的2倍,上层有多少人? 1 / 21 / 2
解:设 6 、单价分别是:《科学家》2.5元/本,《发明家》3元 /本,两套丛书的本数相同,共花了22元。每套丛书多 少本? 解:设 【提高部分】 1、一个数的3倍加上这个数的2倍等于1.5,求这个数是多少?。 解:设 3、小红家到小明家距离是560米,小明和小红在校门口分手,7分钟后他们同时到家,小明平均每分钟走45m,小红平均每分钟走多少米? 解:设2、建筑工地用一辆卡车运60吨沙子,每次运4.6吨,运了几次后还剩14吨? 解:设 4、一根铁丝可以做成一个边长为25厘米的正方形,如果改折成一个长是32厘米的长方形,这个长方形的宽是多少厘米? 解:设 四、灵活运用 下面是小明编的一个计算程序。 1、假设输入的数是a,请用式子表示输出结果。 2、当a=2.6时,求出输出结果。 3、输入的数为y,输出的结果是10,y是多少? 五、能力提升 甲、乙两地仓库存有化肥,甲仓库存有化肥50吨,乙仓库存有62吨。每次从甲仓库运出5吨,同时从乙仓库运出8吨,运了多少次后,两个仓库所存化肥的吨数相等? 2 / 22 / 2
小信号模型及环路设计
开关电源的小信号模型及环路设计 文章作者:万山明吴芳 文章类型:设计应用文章加入时间:2004年8月31日22:9 文章出处:电源技术应用 摘要:建立了Buck电路在连续电流模式下的小信号数学模型,并根据稳定性原则分析了电压模式和电流模式控制下的环路设计问题。 关键词:开关电源;小信号模型;电压模式控制;电流模式控制 引言 设计一个具有良好动态和静态性能的开关电源时,控制环路的设计是很重要的一个部分。而环路的设计与主电路的拓扑和参数有极大关系。为了进行稳定性分析,有必要建立开关电源完整的小信号数学模型。在频域模型下,波特图提供了一种简单方便的工程分析方法,可用来进行环路增益的计算和稳定性分析。由于开关电源本质上是一个非线性的控制对象,因此,用解析的办法建模只能近似建立其在稳态时的小信号扰动模型,而用该模型来解释大范围的扰动(例如启动过程和负载剧烈变化过程)并不完全准确。好在开关电源一般工作在稳态,实践表明,依据小信号扰动模型设计出的控制电路,配合软启动电路、限流电路、钳位电路和其他辅助部分后,完全能使开关电源的性能满足要求。开关电源一般采用Buck电路,工作在定频PWM控制方式,本文以此为基础进行分析。采用其他拓扑的开关电源分析方法类似。 1 Buck电路电感电流连续时的小信号模型
图1为典型的Buck电路,为了简化分析,假定功率开关管S和D1为理想开关,滤波电感L为理想电感(电阻为0),电路工作在连续电流模式(CCM)下。Re为滤波电容C的等效串联电阻,Ro为负载电阻。各状态变量的正方向定义如图1中所示。 S导通时,对电感列状态方程有 L(dil/dt)=Uin-Uo (1) S断开,D1续流导通时,状态方程变为 L(dil/dt)=-Uo (2) 占空比为D时,一个开关周期过程中,式(1)及式(2)分别持续了DTs和(1-D)Ts的时间(Ts为开关周期),因此,一个周期内电感的平均状态方程为 L(dil/dt)=D(Uin-Uo)+(1-D)(-Uo)=DUin-Uo (3) 稳态时,=0,则DUin=Uo。这说明稳态时输出电压是一个常数,其大小与占空比D和输入电压Uin成正比。 由于电路各状态变量总是围绕稳态值波动,因此,由式(3)得
列方程解应用题(写出等量关系式)
列方程解应用题 (写出等量关系式) 1、甲乙两辆客车同时从两地相向而行,5小时后在距离中点30千米处相遇,快车每小时行60千米,慢车每小时行多少千米 2、甲地到乙地是斜坡路,一辆卡车上坡每小明行30千米,下坡每小时40千米,往返一次共用7小时,甲乙两地相距多少千米 3、10元和5元的人民币共有405元,已知10元的张数是5元张数的4倍,那么两种票面的钱各有多少张 4、一轮船从甲港 往乙港,第一天行了全程的1/2多16千米,第二天行的路程是第一天的7/8,这时离乙港还有15千米,甲、乙港之间的距离是多少千米 5、买一辆汽车,分期付款要多付出10%,若现金付款能打九折,王叔叔算了一下,两种方式有9000元的差价,这辆车原价是多少元 6、两个小组共种树200棵,甲组种的棵树的1/3比乙组种的1/10多19棵,两组各种多少棵 7、现在浓度为75%和45%的酒各一种,若要配制酒精含量65%的酒300克,应当从这两种酒中各取多少克 8、有两筐香蕉一共重80千克。从大筐取出4 1,从小筐取出21,从两筐取出的香蕉正好25千克,原来两筐香蕉各重多少千克 9、一次数学考试有10道题,评分规则对一题得10分,错一题倒扣2分,小明回答了10道题,但只得了76分,他答对了几题 10、第一个正方形的边长比第二个的2倍多1厘米,它们的周长相差24厘米。求这两个正方形的面积各多少。 11、弟弟今年5岁,哥哥今年18岁,几年后哥哥的年龄是弟弟的2倍 12、兄妹两人各有钱若干,如果兄给妹20元两人钱数就相等,如果妹给兄25元,则兄的钱是妹的2倍,问兄妹两人各有多少钱 13、一个通讯员骑自行车要在规定的时间内把信件送到某地,他每小时15千米就会早到24分钟,每小时骑12千米要迟到15分钟,规定时间是多少他去某地的路程有多远 14、食堂买的白菜比萝卜的3倍少20千克,萝卜比白菜少70千克,白菜、萝卜食堂各买了多少千克
找等量关系式的四种方法
找等量关系式的方法 1、根据题目中的关键句找等量关系。 应用题中反映等量关系的句子,如“合唱队的人数比舞蹈队的3倍多15人”、“桃树和杏树一共有18 0棵”这样的句子叫做应用题的关键句。在列方程解应用题时,同学们可以根据关键句来找等量关系。 例如:买3支钢笔比买5支圆珠笔要多花0.9元。每 支圆珠笔的价钱是0.6元,每支钢笔多少钱? 我们可以根据题目中的关键句“ 3支钢笔比5支圆珠笔要多花0.9元”找出等量关系: 3支钢笔的价钱一5支圆珠笔的价钱= 0.9元解:设每支钢笔X元。 3X —0.6 X5 = 0.9 2、用常见数量关系式作等量关系。 我们已学过了如“工效X工时=工作总量”、“速度X时 间=路程”、“单价X数量=总价”、“单产量X数量=总产量”等常见数量关系式,可以把这些常见数量关系式作为等量关系式来列方程。 例如:甲乙两辆汽车同时从相距237千米的两个车站 相向开出,经过3小时两车相遇,甲车每小时行3 8千米,乙车每小时行多少千米? 我们可以根据“速度(和)X时间=路程”找出等量关系:(甲速+乙速)X相遇时间=路程 解:设乙车每小时行X千米 (38+X)X3 = 23 7 3、把公式作为等量关系。 在解答一些几何形体的应用题时,我们可以把有关的公式作为等量关系。 例如:一个梯形的面积是30平方分米,它的上底是4 公式作为等量关系即:"(上底+下底)X高-2=梯形的面积”列出方程。 解:设梯形的高是X分米 (4 + 8)XX-2 = 3 0 4、画出线段图找等量关系 对于数量关系比较复杂,等量关系不够明显的应用题我们可以先画出线段图,再根据线段图找出等量关系。 例如:东乡农场计划耕6420公顷耕地,已经耕了5天, 平均每天耕780公顷,剩下的要3天耕完,平均每天要耕多少公顷? 根据题意画出线段图: 从图中我们可以看出等量关系是:“已耕的公顷数+剩下的公顷数=6420”列出方程: 解:设平均每天要耕X公顷 780 X 5 + 3 X= 6420 想一想:根据上面的线段图还可以找出哪些等量关系。 分米,下底是8分米。求梯形的高。我们就把梯形的面积
常见等量关系
常见等量关系 列方程解应用题的一般步骤: 1.认真审题,找出已知量和未知量,以及它们之间的关系; 2.设未知数,可以直接设未知数,也可以间接设未知数; 3.列出方程中的有关的代数式; 4.根据题中的相等关系列出方程; 5.解方程; 6.答题。 一、行程问题: 基本相等关系:速度×时间=路程 (一)相遇问题 相遇问题的基本题型及等量关系 1.同时出发(两段)甲的路程+乙的路程=总路程 2.不同时出发(三段)先走的路程+甲的路程+乙的路程=总路程 (二)追及问题 追及问题的基本题型及等量关系 1.不同地点同时出发快者行驶的路程-慢者行驶的路程=相距的路程 2.同地点不同时出发快者行驶的路程=慢者行驶的路程慢者所用时间=快者所用时间+多用时间 (三)飞行、航行的速度问题等量关系: 顺水速度=静水速度+水流速度 (顺风飞行速度=飞机本身速度+风速) 逆水速度=静水速度-水流速度 (逆风飞行速度=飞机本身速度-风速) 顺水(顺风)的路程=逆水(逆风)的路程 二、商品的利润率: 基本相等关系 利润利润=售价-进价实际售价=折扣数×10%×标价利润率= 进价
利润率=进价 进价售价- 销售额=售价×销售量 售价=进价×(1+利润率) 利息-利息税=应得利息 利息=本金×利率×期数 利息税=本金×利率×期数×税率 本息和=本金+本金×年利率×年数 三、变化率的问题: 1、 基本相等关系(增长率、下降率问题) a(1±x )n =b (其中a 为变化前的量,x 为变化率,n 为变化次数,b 为变化后的量) 四、工程问题: 1、 基本相等关系 工作效率=工作总量/工作时间 工作量=工作效率×工作时间 各工作量之和=总工作量 甲、乙一起合做:1+=合做天数合做天数甲独做天数乙独做天数 甲先做a 天,后甲乙合做:1++=a 合做天数合做天数甲独做天数甲独做天数乙独做天数 全部工作量之和=各队工作量之和,各队合作工作效率=各队工作效率之和 五、不等式问题: 1、 友情提醒 注意审清题意,不要列成方程来解题。留意“至少”、“多于”、“少于”、“不超过”、“不低于”等字眼,通常包含这些字词的题目都要列不等式(组)解题,并且要理解这些字词所代表的数学意义。六、方案问题(方程与不等式结合型):
初中应用题常用等量关系式整合
应用题常用等量关系式 列方程解应用题的一般步骤: 1. 认真审题,找出已知量和未知量,以及它们之间的关系; 2. 设未知数,可以直接设未知数,也可以间接设未知数; 3. 列出方程中的有关的代数式; 4. 根据题中的相等关系列出方程; 5. 解方程; 6. 答题。 一、行程问题:速度×时间=路程 (一)相遇问题::相遇问题的基本题型及等量关系 1、同时出发(两段):甲的路程+乙的路程=总路程 2、不同时出发(三段):先走的路程+甲的路程+乙的路程=总路程 (二)追及问题:追及问题的基本题型及等量关系 (快者的速度-慢者的速度)×追及所用的时间=两者相距的路程 1、不同地点出发:慢者行驶的路程+两者相距的路程=快者行驶的路程 同地不同时出发:快者行驶的路程=慢者行驶的路程 慢者所用时间=快者所用时间+多用时间 慢着先走的路程+慢者后走的路程=快者走的路程 2、追及问题:甲、乙同向不同地: 追者走的路程=前者走的路程+两地间的距离。 (三)飞行、航行的速度问题 顺水速度=静水速度+水流速度顺风飞行速度=飞机本身速度+风速 逆水速度=静水速度-水流速度逆风飞行速度=飞机本身速度-风速 顺水速度-逆水速度=2×水速顺风速度-逆风速度=2×风速 (四):环形跑道题: ①甲、乙两人在环形跑道上同时同地同向出发:快的必须多跑一圈才能追上慢 的。 ②甲、乙两人在环形跑道上同时同地反向出发:两人相遇时的总路程为环形跑道一圈的长度。 二、利润、利率问题: (一)利润问题: 售价=标价×打折数售价=进价×(1+利润率)利润=售价-进价 利润率=(利润÷进价)×100℅=(售价-进价)÷进价×100﹪ 进价=利润÷利润率利润=进价×利润率 售价-进价=进价×利润率=利润销售额=售价×销售量 (二)利率问题:
数学方程找等量关系式的几种方法
找等量关系式的几种方法 1、根据题目中的关键句找等量关系。 应用题中反映等量关系的句子,如“合唱队的人数比舞蹈队的3倍多15人”、“桃树和杏树一共有180棵”这样的句子叫做应用题的关键句。在列方程解应用题时,同学们可以根据关键句来找等量关系。 2、用常见数量关系式作等量关系。 我们已学过了如“工效×工时=工作总量”、“速度×时间=路程”、“单价×数量=总价”、“单产量×数量=总产量”等常见数量关系式,可以把这些常见数量关系式作为等量关系式来列方程。 3、把公式作为等量关系。 在解答一些几何形体的应用题时,我们可以把有关的公式作为等量关系。 4、画出线段图找等量关系 对于数量关系比较复杂,等量关系不够明显的应用题我们可以先画出线段图,再根据线段图找出等量关系。 例如:东乡农场计划耕6420公顷耕地,已经耕了5天,平均每天耕780公顷,剩下的要3天耕完,平均每天要耕多少公顷? 根据题意画出线段图: 780×5 3X X 6420公顷 从图中我们可以看出等量关系是:“已耕的公顷数+剩下的公顷数=6420”列出方程: 设:平均每天要耕X公顷 780×5+3X=6420 想一想:根据上面的线段图还可以找出哪些等量关系。
1.牢记计算公式,根据公式来找等量关系。 这种方法一般适用于几何应用题,教师要让学生牢记周长公式、面积公式、体积公式等,然后根据公式来解决问题。 2.熟记数量关系,根据数量关系找等量关系。 这种方法一般适用于工程问题、路程问题、价格问题,教师在教学这三类问题时,不但要让学生理解,还应让学生记熟 工作效率×工作时间=工作总量; 速度×时间=路程; 单价×件数=总价” 等关系式。 如“汽车平均每小时行45千米,从甲地到乙地共225千米,汽车共需行多少小时?”就可以根据“速度×时间=路程”这一数量关系,列出方程45X=225。 3.抓住关键字词,根据字词的提示找等量关系。 这种方法一般适用于和差关系、倍数关系的应用题,在题中常有这样的提示:“一共有”、“比……多(少)”、“是……的几倍”、“比……的几倍多(少)”等。在解题时,可根据这些关键字词来找等量关系,按叙述的顺序列出方程。 如“四年级有学生250人,比三年级的2倍少70人,三年级有学生多少人?”,根据题中“比……少”可知:三年级的2倍减去70人等于四年级的人数,从而列出方程2X-70=250。 4.找准单位“1”,根据“量率对应”找等量关系。 这种方法一般适用于分数应用题,有时也适用“倍比关系”应用题。对于分数应用题来说,每一个分率都对应着一个具体的量,而每一个具体的量也都对应着一个分率。在倍比关系的应用题中,也应找准标准量。因此,正确地确定“量率对应”是解题的关键。 5.补充缺省条件,根据句子意思找等量关系。 这类应用题的特征是含有“比……多(少)”、“比……增加(减少)”等特定词,如:甲比乙多“几分之几”、少“几分之几”、增加“几分之几”、减少“几分之几”等类型的语句,题目中由于常缺少主语,造成学生理解上的困难。因此,
蔡琴歌词大全
爱断情伤 作曲:张弘毅 作词:林秋离 演唱:蔡琴 等待不难 时间总是不长不短 心中有渴望 和你静静谈一谈 而雷声轰传 却让人心慌意乱 终于我冷却了心情 窗外的天色已晚 开口之前 泪光已在眼里旋转 你无波的心情 比我的泪还冰凉 而再三思量 避开你又能怎样 想走却没有方向 迷乱在狂想的路上 夜那么长 足够我把每一盏灯都点亮 守在门旁 换上我最美丽的衣裳 夜那么长 所以人们都梦的神魂飘荡 不会再有空间 听我的爱断情伤 把月光射下来 蔡琴 作词:邬裕康作曲/编曲:鲍比达 深深地爱上一个人 才发现心脏在哪儿 砰然的隐隐的它就像被小火慢慢炖 捕捉你眼里的光和热呼着贴背贴心的闷你才转身我就昏了该怎么好呢 今晚的月色特别烦人 它不放过小小的一点灰尘 让眼底手心发汗的情侣们变得慢吞吞 好心人快把月光射下来 就算只有半秒黑暗 好心人快把月光射下来善妒的月光快快把它射下来 花草的香才上的来 好心人快把月光射下来我要你爱 被遗忘的时光 蔡琴> 词:曲: 是谁在敲打我窗 是谁在撩动琴弦 那一段被遗忘的时光 渐渐地回升出我心坎 记忆中那欢乐的情景 慢慢地浮现在我的脑海 那缓缓飘落的小雨 不停地打在我窗 只有那沉默无语的我 不时地回想过去 菜根谭 蔡琴> 词:曲: 想起了菜根甜呀 菜根甜菜根香 退一步嘛心放宽 吃得菜根心甜甘 不闻车马喧 不见尘土扬 知足常乐笑怡然 你若是不求名呀 不求名不求利 世事本是一盘棋 吃得菜根好福气 不为五斗米 只穿青步衣 道理全在菜根里
出人头地 蔡琴 那个不想出人头地 说起来简单做起来不易 那个不愿登峰造极 首先要问一问你自己 哎哟朋友你有没有自私自利 哎哟朋友你有没有自暴自弃 哎哟朋友你有没有尽心尽力 不努力怎会出人头地 那个不想出人头地 那万丈高楼由平地造起 不要空想登峰造极 大海洋源流于小水滴 哎哟朋友你必须要先人后自 哎哟朋友你必须要自食其力 哎哟朋友你必须要自强不息 到头来终会出人头地 哎哟朋友你有没有自私自利 哎哟朋友你有没有自暴自弃 哎哟朋友你有没有尽心尽力 不努力怎会出人头地 出塞曲 LRC EDIT:洪枫sunhfone@https://www.360docs.net/doc/5d14216269.html, 请为我唱一首出塞曲 用那遗忘了的古老言语 请用美丽的颤音轻轻呼唤 我心中的大好河山 那只有长城外才有的清香 谁说出塞歌的调子太悲凉 如果你不爱听 那是因为歌中没有你的渴望 而我们总是要一唱再唱 想着草原千里闪着金光 想着风沙呼啸过大漠 想着黄河岸啊阴山旁 英雄骑马壮 骑马荣归故乡初恋 演唱:蔡琴 夜已深沉月儿昏昏 寂寞地等侯你 等侯你诉一诉 别后岁月凄凉 我要你常记在你心里 我要你永远不忘记 我俩相依蔷薇花底 好想鸟儿双栖枝连李 千万种的情义 我要你快回到我身边 我要你相爱在一起 趁着今夜星月依稀 我俩重回蔷薇花底 大约在冬季 词、曲:齐秦 轻轻的我将离开你 请将眼角的泪拭去 漫漫长夜里未来日子里亲爱的你别为我哭泣 前方的路虽然太凄迷 请在笑容里为我祝福 虽然迎著风虽然下著雨我在风雨之中念著你 没有你的日子里 我会更加珍惜自己 没有我的岁月里 你要保重你自己 你问我何时归故里 我也轻声地问自己 不是在此时不知在何时我想大约会是在冬季 不是在此时不知在何时我想大约会是在冬季
基于BUCK电路的电源设计(DOC)
现代电源技术 基于BUCK电路的电源设计 学院: 专业: 姓名: 班级: 学号: 指导教师: 日期:
目录 摘要 (3) 一、设计意义及目的 (4) 二、Buck电路基本原理和设计指标 (4) 2.1 Buck电路基本原理 (4) 2.2 Buck电路设计指标 (6) 三、参数计算及交流小信号等效模型建立 (6) 3.1 电路参数计算 (6) 3.2 交流小信号等效模型建立 (10) 四、控制器设计 (11) 五、Matlab电路仿真 (17) 5.1 开环系统仿真 (17) 5.2 闭环系统仿真 (18) 六、设计总结 (21)
摘要 Buck电路是DC-DC电路中一种重要的基本电路,具有体积小、效率高的优点。本次设计采用Buck电路作为主电路进行开关电源设计,根据伏秒平衡、安秒平衡、小扰动近似等原理,通过交流小信号模型的建立和控制器的设计,成功地设计了Buck电路开关电源,通过MATLAB/Simulink进行仿真达到了预设的参数要求,并有效地缩短了调节时间和纹波。通过此次设计,对所学课程的有效复习与巩固,并初步掌握了开关电源的设计方法,为以后的学习奠定基础。 关键词:开关电源设计 Buck电路
一、设计意义及目的 通常所用电力分为直流和交流两种,从这些电源得到的电力往往不能直接满足要求,因此需要进行电力变换。常用的电力变换分为四大类,即:交流变直流(AC-DC),直流变交流(DC-AC),直流变直流(DC-DC),交流变交流(AC-AC)。其中DC-DC电路的功能是将直流电变为另一固定电压或可调电压的直流电,包过直接直流变流电路和间接直流变流电路。直接直流变流电路又称斩波电路,它的功能是将直流电变为另一固定电压或可调电压的直流电,主要包括六种基本斩波电路:Buck电路,Boost电路,Buck-Boost电路,Cuk电路,Sepic电路,Zeta 电路。其中最基本的一种电路就是Buck电路。 因此,本文选用Buck电路作为主电路进行电源设计,以达到熟悉开关电源基本原理,熟悉伏秒平衡、安秒平衡、小扰动近似等原理,熟练的运用开关电源直流变压器等效模型,熟悉开关电源的交流小信号模型及控制器设计原理的目的。这些知识均是《线代电源设计》课程中所学核心知识点,通过本次设计,将有效巩固课堂所学知识,并加深理解。 二、Buck电路基本原理和设计指标 2.1 Buck电路基本原理 Buck变换器也称降压式变换器,是一种输出电压小于输入电压的单管不隔离直流变换器,主要用于电力电路的供电电源,也可拖动直流电动机或带蓄电池负载等。其基本结构如图1所示:
开关电源的小信号建模详解
详解:开关电源的小信号建模 开关电源的反馈环路设计是开关电源设计的一个非常重要的部分,它关系到一个电源性能的好坏。要设计一个好的环路,必须要知道主回路的数学模型,然后根据主回路的数学模型,设计反馈补偿环路。本文想重点介绍下主回路的数学建模方法。 首先来介绍下小信号的分析法。开关电源是一个非线性系统,但可以对其静态工作点附近进行局部线性化。这种方法称为小信号分析法。 以一个CCM模式的BOOST电路为例, 其增益为: 其增益曲线为: 其中M和D之间的关系是非线性的。但在其静态工作点M附近很小的一个区 域范围内,占空比的很小的扰动和增益变化量之间的关系是线性的。因此在这个很小的区域范围内,我们可以用线性分析的方法来对系统进行分析。这就是小信号分析的基本思路。因此要对一个电源进行小信号建模,其步骤也很简单,第一步就是求出其静态工作点,第二步就是叠加扰动,第三步就是分离扰动,
进行线性化,第四步就是拉氏变换,得到其频域特性方程,也就是我们说的传递函数。要对一个变换器进行小信号建模,必须满足三个条件。 首先要保证得到的工作点是“静”态的。因此有两个假设条件: 1,一个开关周期内,不含有低频扰动。因此叠加的交流扰动小信号的频率应该 远远小于开关频率。这个假设称为低频假设 2,电路中的状态变量不含有高频开关纹波分量。也就是系统的转折频率要远远 小于开关频率。这个假设称为小纹波假设。其次为了保证这个扰动是在静态工作 点附近,因此有第三个假设条件: 3,交流小信号的幅值必须远远小于直流分量的幅值。这个称为小信号假设。 对于PWM模式下的开关电源,通常都能满足以上三个假设条件,因此可以使用小 信号分析法进行建模。对于谐振变换器来说,由于谐振变换器含有一个谐振槽路。 在一个开关时区或多个开关时区内,谐振槽路中各电量为正弦量,或者其有效成 分是正弦量。正弦量的幅值是在大范围变化的,因此在研究PWM型变换器所使用 的“小纹波假设”在谐振槽路的小信号建模中不再适用。对于谐振变换器,通常 采用数据采样法或者扩展描述函数法进行建模。 以一个CCM模式下的BUCK电路为例,应用上面的四个步骤,来建立一个小信号 模型。 对于一个BUCK电路 当开关管开通时,也就是在(0-DTs)区间 其状态方程为
常用应用题常用等量关系式
常用应用题常用等量关系式 列方程解应用题的一般步骤: 1. 认真审题,找出已知量和未知量,以及它们之间的关系; 2. 设未知数,可以直接设未知数,也可以间接设未知数; 3. 列出方程中的有关的代数式; 4. 根据题中的相等关系列出方程; 5. 解方程; 6. 答题。 一、行程问题:速度×时间=路程 (一)相遇问题:相遇问题的基本题型及等量关系 1、同时出发(两段):甲的路程+乙的路程=总路程 2、不同时出发(三段):先走的路程+甲的路程+乙的路程=总路程 (二)追及问题:追及问题的基本题型及等量关系 (快者的速度-慢者的速度)×追及所用的时间=两者相距的路程 1、不同地点出发:慢者行驶的路程+两者相距的路程=快者行驶的路程 同地不同时出发:快者行驶的路程=慢者行驶的路程 慢者所用时间=快者所用时间+多用时间 慢着先走的路程+慢者后走的路程=快者走的路程 2、追及问题:甲、乙同向不同地: 追者走的路程=前者走的路程+两地间的距离。 (三)飞行、航行的速度问题 顺水速度=静水速度+水流速度顺风飞行速度=飞机本身速度+风速 逆水速度=静水速度-水流速度逆风飞行速度=飞机本身速度- 风速 顺水速度-逆水速度=2×水速顺风速度-逆风速度=2×风速 (四):环形跑道题: ①甲、乙两人在环形跑道上同时同地同向出发:快的必须多跑一圈才能追上慢的。 ②甲、乙两人在环形跑道上同时同地反向出发:两人相遇时的总路程为环形跑道一圈长度。 二、利润、利率问题: (一)利润问题: 售价=标价×打折数售价=进价×(1+利润率)利润=售价-进价 利润率=(利润÷进价×100℅利润率=(售价-进价)÷进价×100﹪ 进价=利润÷利润率利润=进价×利润率 利润=售价-进价利润=进价×利润率销售额=售价×销售量 (二)利率问题: 利息=本金×利率×存期(年数、月数)利息税=本金×利率×期数×税率 本息和=本金+利息=本金+本金×利率×存期利息- 利息税=应得利息 (三)工程问题(一般把工作总量设为单位1) 工作总量=工作效率×工作时间 各工作量之和=总工作量 各队合作工作效率=各队工作效率之和 工作量并不是具体数量,因而常常把工作总量看作整体1, 工作效率=工作总量除以工作时间 甲、乙一起合做:合做天数除以甲独做天数+合做天数除以乙独做天数=1 甲先做a 天,后甲乙合做:a 除以甲独做天数+合做天数除以甲独做天数+合做天数除以乙独做天数=1
(完整版)解方程等量关系式的四种方法
找等量关系式的四种方法 1、根据题目中的关键句找等量关系。 应用题中反映等量关系的句子,如“合唱队的人数比舞蹈队的3倍多15人”、“桃树和杏树一共有180棵”这样的句子叫做应用题的关键句。在列方程解应用题时,同学们可以根据关键句来找等量关系。 例如:买3支钢笔比买5支圆珠笔要多花0.9元。每支圆珠笔的价钱是0.6元,每支钢笔多少钱? 我们可以根据题目中的关键句“3支钢笔比5支圆珠笔要多花0.9元”找出等量关系:3支钢笔的价钱-5支圆珠笔的价钱=0.9元 设:每支钢笔X元。3X-0.6×5=0.9 2、用常见数量关系式作等量关系。 我们已学过了如“工效×工时=工作总量”、“速度×时间=路程”、“单价×数量=总价”、“单产量×数量=总产量”等常见数量关系式,可以把这些常见数量关系式作为等量关系式来列方程。 例如:甲乙两辆汽车同时从相距237千米的两个车站相向开出,经过3小时两车相遇,甲车每小时行38千米,乙车每小时行多少千米? 我们可以根据“速度(和)×时间=路程”找出等量关系:“(甲速+乙速)×相遇时间=路程” 设:乙车每小时行X千米 (38+X)×3=237 3、把公式作为等量关系。 在解答一些几何形体的应用题时,我们可以把有关的公式作为等量关系。 例如:一个梯形的面积是30平方分米,它的上底是4分米,下底是8分米。求梯形的高。我们就把梯形的面积公式作为等量关系即:“(上底+下底)×高÷2=梯形的面积”列出方程。 设:梯形的高是X分米 (4+8)×X÷2=30 4、画出线段图找等量关系 对于数量关系比较复杂,等量关系不够明显的应用题我们可以先画出线段图,
再根据线段图找出等量关系。 例如:东乡农场计划耕6420公顷耕地,已经耕了5天,平均每天耕780公顷,剩下的要3天耕完,平均每天要耕多少公顷? 根据题意画出线段图: 从图中我们可以看出等量关系是:“已耕的公顷数+剩下的公顷数=6420”列出方程: 设:平均每天要耕X公顷 780×5+3X=6420 想一想:根据上面的线段图还可以找出哪些等量关系。
恒定导通时间电流模式控制buck电路小信号分析
过u逼题 ---------------------------------------------------------------------------------------------------------------------------------------------------------------------------------------------------------------------------------------满y Do路釐r 满y Do路釐r 满y Do路釐r 恶描获 提得获 度00获 D点 度得获 得感获 感得获 描0获 补度言 综难量l釐y D釐s逼r难过难高g 环u高逼t难o高 补恶言 获s高获s高 离T点恶方度得补得言 电获难高模感方获如获o模恶实恶获如To高模0实得描μs实fs模得抑0题hz 离s模恶实度u电实点o模得型度抑0u环实 综逼o模是展得 mΩ实 ()*()()*(1)*()*()s f Ton L i f fs Vin Gic f e Vc f sf Lf s f ?= =? 度 *Vo sf Ri Lf = fs 补得言 ()2***s f j f π=如 系路量釐 ( )*2 2 ()*1()()11*11s f T o n s f T o n e s f s f Q ωω?=? ++ 得 2 1Q π = 1Ton π ω=
()*()*(1()**) ()*(1)**()*()1()*()*s f Ton Vo f fs Vin Rl s f Rco Co Gvc f e Vc f sf Lf s f s f Rl Rco Co ?+= =?++ 恶 22 11*(1()**) ()*()()1()*()*11*11Rl s f Rco Co Gvc f s f s f Ri s f Rl Rco Co Q ωω+= ++++ 感 得 1*1()* 2Ton Ri k f Ls = 1*2()*2Ton Ri k f Ls =? 题得(f噪如 22()(1()**)1 1()** 2()*2()()()()*(1****11*111()* *21Vo f Rl s f Rco Co Gvc f k f Rl k f s f s f Vc f Ri Rl Co Ro Rco Co Ri Ri Q s f Rl k Ri ωω+= =??++ +? 题得(f噪 ()1()**) 2()*()1()**Vo f Rl s f Rco Co Gvc f Vc f Ri s f Rl Co +==+ 抑
反激变换器小信号模型Gvd(s)推导__1210
一、反激变换器小信号模型的推导 1.1 DCM 1.1.1 DCM buck-boost 小信号模型的推导 根据状态空间平均法推导DCM buck-boost 变换器小信号模型如下: +-v in (t)v o (t)一般开关网络 图1 1理想Buck-Boost 变换器开关网络 1231d d d ++= (1) 首先,定义开关网络的端口变量1122,,,v i v i ,建立开关周期平均值 1 1 2 2 ,,,s s s s T T T T v i v i 之间的关系: 11()s g T g pk s s v t v i d T d T L L <>= = (2) 根据工作模态:113()()()0s s s L T g T T v t d v t d v t d <>=<>+<>+ (3) []1 1 ()()()s s s t T t T L T L s t t s s s di L v t v d L d i t T i t T T d T τττ++<>= = =+-? ? (4) DCM 下,()()0s i t T i t +==,所以()0s L T v t <>=,结合(3)式: 11()()0s s g T T d v t d v t <>+<>= (5) 21()(t)=-(t)()s s g T T v t d d v t <><> (6) 根据工作模态:1123()()0()(()())()()s s s s T g T T g T v t d t d t v t v t d t v t <>=+<>-<>+<>(7) 消去上式的2d 和3d 得:1()()s s T g T v t v t <>=<> (8) 根据工作模态:2123()()(()())()0(()) s s s s T g T T g T v t d t v t v t d t d v t <>=<>-<>++-<>
一元一次方程如何找等量关系
一元一次方程如何找等量关系 列方程找等量关系的关键就是找到题目中的不变量,不变量有不同的表现形式分为两种,题目中的已知数,也就是具体的数值,这种是比较简单的,一眼就能看出来的;有的是通过未知数与题目中的数字运算结果作不变量。当然理解题意非常重要,只有理解了,才能分清等量关系。好,下面我就一些例题详细作以讲解 1.找题目中已知数或者是题目中的一个或多个数字的运算结果作为不变量,让它作为等量关系的一边,把它放在方程的右边(也可以在左边,为了方便叙述,就把它放在右边),然后设未知数,通过未知数和题目中数字的运算列出代数式,使代数式的意义和右边不变量的意义相同,把代数式放在方程的左边,这样方程就会轻而易举的列了出来。 例题1.甲乙两班共有学生98人,甲班比乙班多6人,求两班各有多少人? 这个题目中有两个数字,这两个数字都是不变量,任何题目中的数字都是不变量,找到一个不变量,放在方程的右边,我们再用x与题目中的数字把它表示出来。这个题目中的我们把98作为不变量放在方程的右边,98代表的含义是甲乙两班共有学生的人数,根据题意可以设甲班人数为x,根据第二个条件“甲班比乙班多6人”,就可以用x表示出乙班的人数为x-6,这样就可以用x把98所代表的含义表示出来x+(x-6),这样就可以把方程列出来了: x+(x-6)=98 同样,我们可以把6作为不变量来列方程,这里不再叙述,同学们自己可以根据这个思路列出方程来。 例题2.甲、乙两人同时从A地前往相距25.5千米的B地,甲骑自行车,乙步行,甲的速度比乙的速度的2倍还快2千米/时,甲先到达B地后,立即由B 地返回,在途中遇到乙,这时距他们出发时已过了3小时。求两人的速度。 这个题目中的不变量就是两地之间的距离,这里不做过多解释了。 解:设乙的速度是x 千米/时, 3x+3 (2x+2)=25.5×2 2.先把未知数设出来,然后直接把它放在方程的右边或者与题目中的一个或多个数字的运算结果(代数式)放在方程的右边(也可以在左边,为了方便叙述,
等量关系教案
四年级《等量关系》教学设计 教学内容:北师大版小学数学四年级下册第五单元第64-65页 教材分析:本节课是在学生学会用字母表示数功能的基础上教学的,教材通过跷跷板情境,引导学生用语言描述具体情境中的等量关系,通过反复体验感知找出等量关系,本节课的教学对学生学习方程、解方程及运用方程解决简单的实际问题起着承上启下的作用,它是学生学习用方程解决问题的起始课,在本单元中具有重要的地位。 教学目标: 1、结合具体情境,在用多种方法表示等量关系的活动中了解等量关系,知道同一个等量关系可以有不同的表示形式。 2、初步体会等量关系在日常生活中的广泛存在,体会数学的应用价值。 3、培养学生自主探究和合作交流的能力。 教学重点:能够在具体情境中找出等量关系 教学难点:找等量关系 教法:通过具体情境引导学发现等量关系,并能用语言和算式来表述,并在反复体会和深入探究中多角度理解等量关系。 学法:以自主探究、小组合作作为学习的主要方式。由直观到抽象,在探索和交流中感受、体会和理解。 教学过程: 一、创设情境 1、谈话导入: 师:同学们周末都喜欢去哪儿玩?为什么? 生:公园、游乐场等。 2、出示跷跷板: ①师:喜欢玩吗?说说玩跷跷板的感受? 生:起、落,有意思。 ②师:看图并说说三幅图分别是什么意思。 生:(1)1只鹅比2只鸭重 (2)3只鸭比1只鹅重
(3)1只鹅与2只鸭子和1只鸡一样重 二、合作探究 1、初步感知等量关系 师:跷跷板怎样就平衡了?你能尝试表示这组相等的关系吗? 生:1只鹅的质量等于2只鸭子和1只鸡的质量。 1只鹅的质量=2只鸭子+1只鸡的质量 师:像这样的关系,我们就称之为等量关系。 2、进一步体会等量关系 ①师:生活中有很多的数量关系,我们一起去看看吧!看,著名的篮球运动员姚明也来到了我们的课堂,他最大的特点是什么?(特别高)对呀,他的身高是226厘米。笑笑和妹妹跟姚明比了一下身高。(出示妹妹、姚明和笑笑身高关系) ②读懂信息:哪两个人之间的身高有关系?什么关系? ③你能表示出妹妹、姚明和笑笑身高的关系吗? 合作要求: 1、借助体现数量关系的句子,理解、抓住关键句子。 2、可以用文字、画图等形式来表示,选你们喜欢的方式。 ④展示汇报: 师:哪一组愿意汇报你们组的合作结果? 生:文字、式子、画图。 ⑤小结 说说怎样找等量关系? 3、多角度认识等量关系 师:老师从刚才的信息中也找到了一些等量关系式,我们一起来看看,你能看懂吗? 姚明身高÷2=妹妹身高笑笑身高—20厘米=妹妹身高 姚明身高÷2=笑笑身高—20厘米 师:观察这3个等量关系式你从中有什么发现? 生:妹妹身高有两种表示形式,通过妹妹身高的两种形式我们得出了又一个等量关系即:
经典感恩歌曲推荐《念亲恩》
经典感恩歌曲推荐《念亲恩》《念亲恩》是由陈百强演唱的一首歌曲,由杨继兴作曲和作词,收录在同名专辑《念亲恩》中。 【歌词】 长夜空虚使我怀旧事 明月朗相对念母亲 父母亲爱心 柔善像碧月 怀念怎不悲莫禁 长夜空虚枕冷夜半泣 遥路远碧海示我心 父母亲爱心 柔善像碧月 常在心里问何日报 亲恩应该报 应该惜取孝道 惟独我离别 无法慰亲旁 轻弹曲韵梦中送 长夜空虚枕冷夜半泣 遥路远碧海示我心 父母亲爱心
柔善像碧月 常在心里问何日报长夜空虚使我怀旧事明月朗相对念母亲父母亲爱心 柔善像碧月 怀念怎不悲莫禁 长夜空虚枕冷夜半泣遥路远碧海示我心父母亲爱心 柔善像碧月 常在心里问何日报亲恩应该报 应该惜取孝道 惟独我离别 无法慰亲旁 轻弹曲韵梦中送 长夜空虚枕冷夜半泣遥路远碧海示我心父母亲爱心 柔善像碧月 常在心里问何日报
【歌手简介】 陈百强(Danny Chan)1958年9月7日生于香港,籍贯广东台山,中国香港歌手、演员。 1979年以专辑《First Love》在香港出道,同年凭借歌曲《眼泪为你流》获得香港十大中文金曲奖;1981年主演电影《失业生》。1983年凭借《今宵多珍重》获得香港十大劲歌金曲奖以及AGB观众抽签调查最受欢迎奖;同年他演唱的歌曲《偏偏喜欢你》入选香港十大中文金曲。1984年主演电影《圣诞快乐》。1985年推出个人专辑《深爱着你》。1986年主演电影《秋天的童话》。1987年由陈百强作曲的《我的故事》入选香港十大中文金曲。1988年、1989年获得两届叱咤乐坛流行榜“叱吒乐坛男歌手铜奖”。1989年凭借歌曲《一生何求》获得香港十大中文金曲以及十大劲歌金曲奖。 陈百强擅长演绎浪漫情歌,亦能自己创作歌曲。1993年10月25日,陈百强因为逐渐性脑衰竭而去世,终年35岁。XX年1月30日,在香港电台第三十二届十大中文金曲颁奖礼上,陈百强被追颁“金针奖”。 XX年8月31日,陈奕迅、梁咏琪、杨千、张敬轩、苏永康、梁汉文众星齐聚一堂以歌声纪念陈百强。
Buck电路小信号模型及环路设计
开关电源(Buck电路)的小信号模型及环路 设计 华中科技大学电气与电子工程学院作者:万山明,吴芳 0 引言 设计一个具有良好动态和静态性能的开关电源时,控制环路的设计是很重要的一个部分。而环路的设计与主电路的拓扑和参数有极大关系。为了进行稳定性分析,有必要建立开关电源完整的小信号数学模型。在频域模型下,波特图提供了一种简单方便的工程分析方法,可用来进行环路增益的计算和稳定性分析。由于开关电源本质上是一个非线性的控制对象,因此,用解析的办法建模只能近似建立其在稳态时的小信号扰动模型,而用该模型来解释大范围的扰动(例如启动过程和负载剧烈变化过程)并不完全准确。好在开关电源一般工作在稳态,实践表明,依据小信号扰动模型设计出的控制电路,配合软启动电路、限流电路、钳位电路和其他辅助部分后,完全能使开关电源的性能满足要求。开关电源一般采用Buck电路,工作在定频PWM控制方式,本文以此为基础进行分析。采用其他拓扑的开关电源分析方法类似。 1 Buck电路电感电流连续时的小信号模型 图1为典型的Buck电路,为了简化分析,假定功率开关管S和D1为理想开关,滤波电感L为理想电感(电阻为0),电路工作在连续电流模式(CCM)下。R e为滤波电容C的等效串联电阻,R o为负载电阻。各状态变量的正方向定义如图1中所示。 图1 典型Buck电路 S导通时,对电感列状态方程有 L=U in-U o (1) S断开,D1续流导通时,状态方程变为 L=-U o (2) 占空比为D时,一个开关周期过程中,式(1)及式(2)分别持续了DT s和(1-D)T s的时间(T s为开关周期),因此,一个周期内电感的平均状态方程为 L=D(U in-U o)+(1-D)(-U o)=DU in-U o(3) 稳态时,=0,则DU in=U o。这说明稳态时输出电压是一个常数,其大小与占空比D 和输入电压U in成正比。 由于电路各状态变量总是围绕稳态值波动,因此,由式(3)得 L=(D+d)(U in+)-(U o+) (4)
七八十年代歌曲
上面是一个70-80年代电视剧插曲、主题曲的网址,歌实在太多了,其中有些还不错,你可以挑你熟悉的歌名上百度MP3网再搜一下,(网页中给的歌曲网址好像不能点击)。 另外: 刘文正:兰花草、梅兰梅兰我爱你、三月里的小雨、乡间的小路、外婆的澎湖湾、雨中即景、童年、送你一朵勿忘我 徐小凤:风的季节、每一步、顺流逆流、漫漫前路、明月千里寄相思、 陈百强:偏偏喜欢你、一生何求、念亲恩、今宵多珍重、 邓丽君:漫步人生路、四季歌、天涯歌女、甜蜜蜜、我只在乎你、小城故事、月亮代表我的心、美酒加咖啡、一帘幽梦、阿里山的姑娘、北国之春、何日君再来、又见炊烟、夜来香、恰似你的温柔、你怎么说、路边的野花不要采、但愿人长久、何日君再来、千言万语、goodbye,my love、采红菱、南海姑娘、船歌、原乡人、东山飘雨西山晴、在水一方、。。。 许冠杰:沧海一声笑、阿郎恋曲、浪子心声、 罗文:铁血丹心、万里长城永不倒、尘缘、狮子山下、几许风雨、掌声响起、奚秀兰:牧羊曲、雁南飞、苏州河边、月儿弯弯照九州、 费玉清:一剪梅、梦驼铃、天上人间、花好月圆、凤凰于飞、今宵多珍重、我要为你歌唱、水长流、春风吻上我的脸、南屏晚钟、 蔡琴:读你、不了情、祈祷、把悲伤留给自己、康定情歌、绿岛小夜曲、情人的眼泪、月满西楼、出塞曲、我有一段情、三年、 张国荣:倩女幽魂、沉默是金、当爱已成往事 陈慧娴:千千阙歌、飘雪、人生何处不相逢、红茶馆、 罗大佑:东方之珠、滚滚红尘、恋曲1990、 还有像齐秦、齐豫、千百惠、林忆莲、叶倩文、四大天王、小虎队、梅艳芳、草蜢等都有几首脍炙人口的代表作,像橄榄树、大约在冬季、今夜你会不会来、晚秋、吻别、选择、女人花、失恋阵线联盟。。。 我把我喜欢的90年代经典歌曲列举一下: 孟庭苇:你究竟有几个好妹妹风中有朵雨做的云你看你看月亮的脸 周蕙:约定 许茹芸:美梦成真如果云知道 王菲:容易受伤的女人红豆我愿意 刘若英:为爱痴狂很爱很爱你当爱在靠近 陈淑桦:滚滚红尘梦醒时分 陈慧娴:飘雪千千阙歌 林忆莲:至少还有你伤痕 周子寒:吻和泪 王馨平:别问我是谁 辛晓琪:俩俩相望味道 高胜美:哭砂 许美静:城里的月光 李翊君:雨蝶 赵咏华:最浪漫的事