A clause-based heuristic for SAT solvers
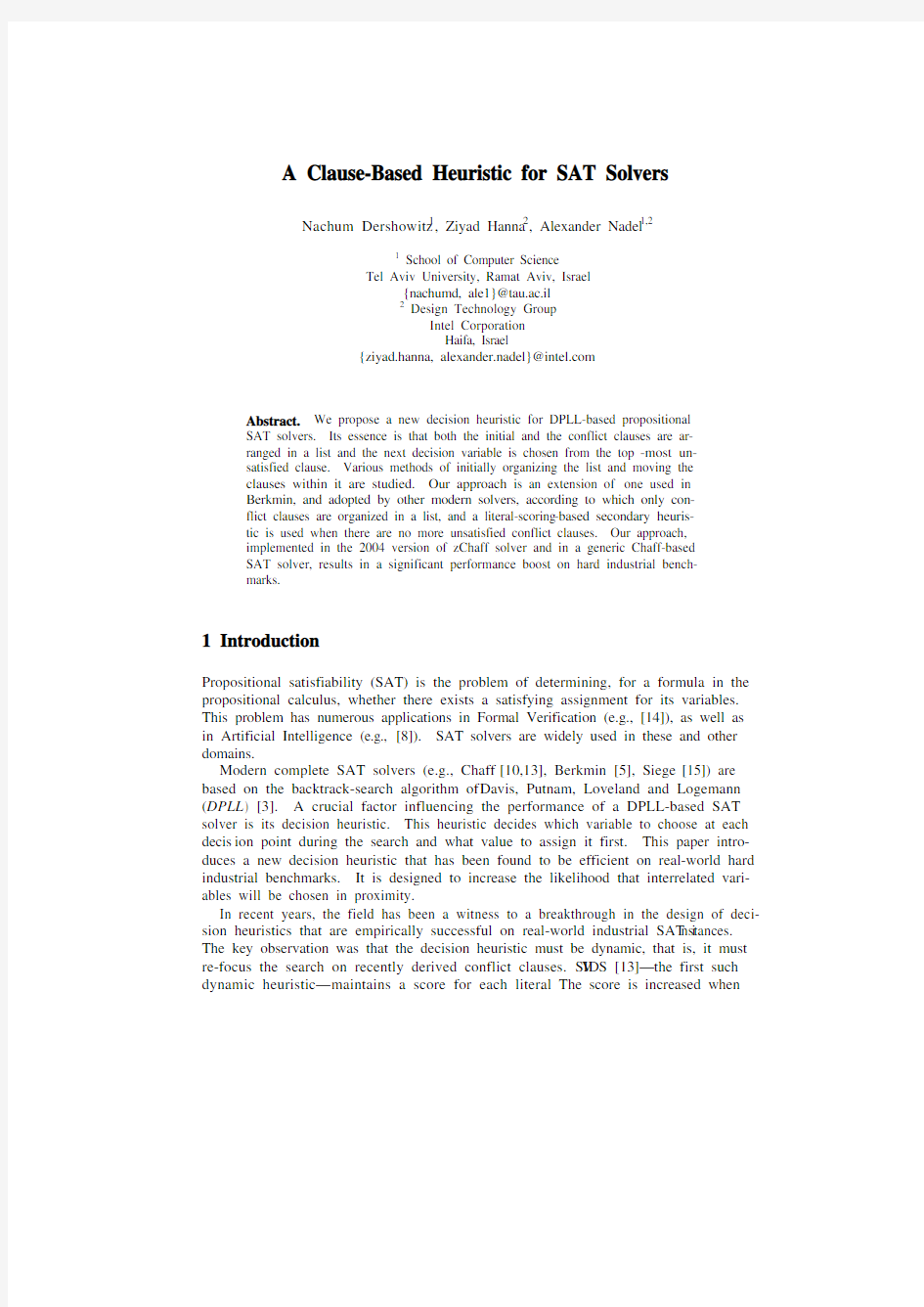
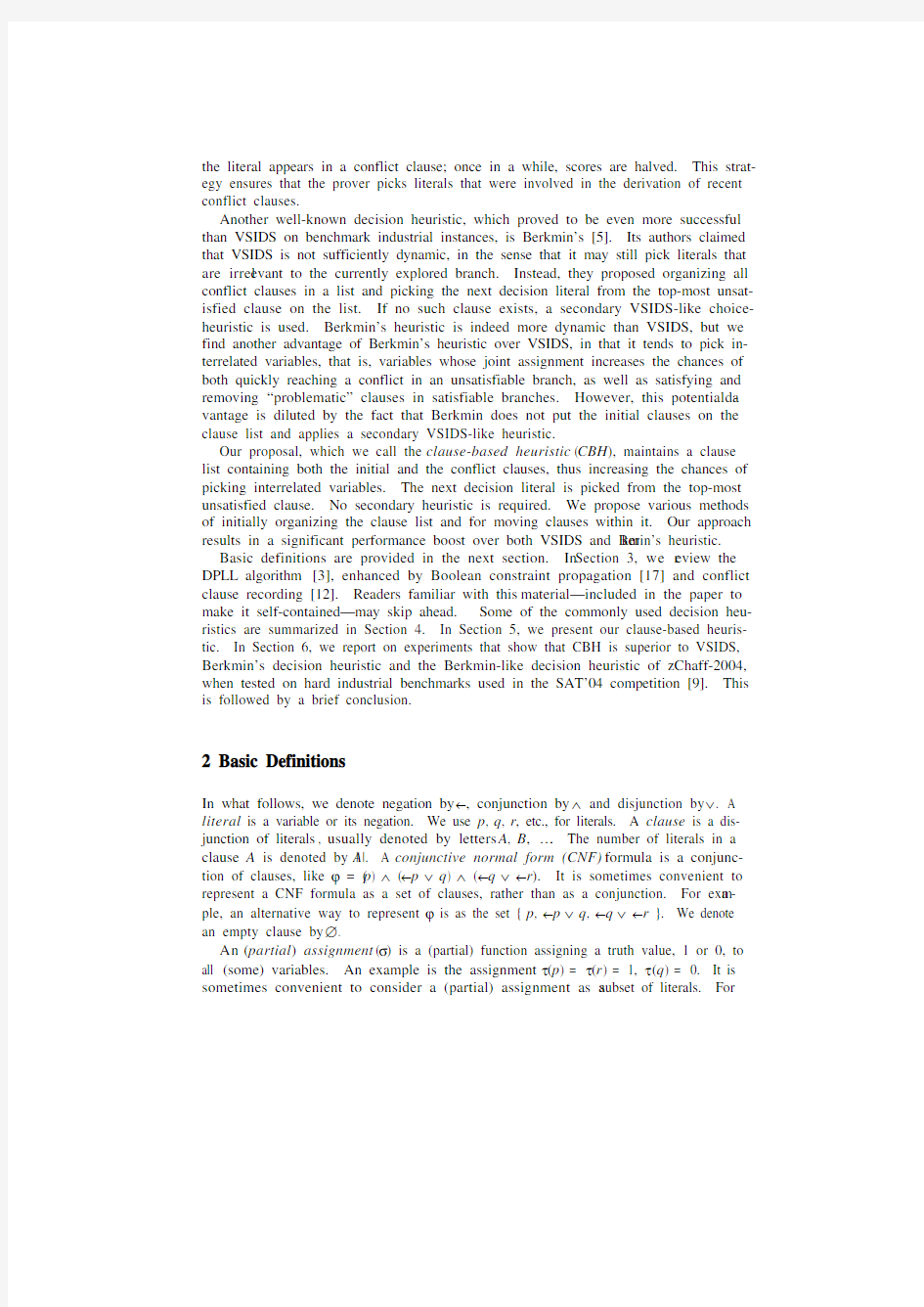
A Clause-Based Heuristic for SAT Solvers
Nachum Dershowitz1, Ziyad Hanna2, Alexander Nadel1,2
1 School of Computer Science
Tel Aviv University, Ramat Aviv, Israel
{nachumd, ale1}@tau.ac.il
2 Design Technology Group
Intel Corporation
Haifa, Israel
{ziyad.hanna, alexander.nadel}@https://www.360docs.net/doc/6e9857002.html,
Abstract. We propose a new decision heuristic for DPLL-based propositional
SAT solvers. Its essence is that both the initial and the conflict clauses are ar-
ranged in a list and the next decision variable is chosen from the top-most un-
satisfied clause. Various methods of initially organizing the list and moving the
clauses within it are studied. Our approach is an extension of one used in
Berkmin, and adopted by other modern solvers, according to which only con-
flict clauses are organized in a list, and a literal-scoring-based secondary heuris-
tic is used when there are no more unsatisfied conflict clauses. Our approach,
implemented in the 2004 version of zChaff solver and in a generic Chaff-based
SAT solver, results in a significant performance boost on hard industrial bench-
marks.
1 Introduction
Propositional satisfiability (SAT) is the problem of determining, for a formula in the propositional calculus, whether there exists a satisfying assignment for its variables. This problem has numerous applications in Formal Verification (e.g., [14]), as well as in Artificial Intelligence (e.g., [8]). SAT solvers are widely used in these and other domains.
Modern complete SAT solvers (e.g., Chaff [10,13], Berkmin [5], Siege [15]) are based on the backtrack-search algorithm of Davis, Putnam, Loveland and Logemann (DPLL) [3]. A crucial factor influencing the performance of a DPLL-based SAT solver is its decision heuristic. This heuristic decides which variable to choose at each decis ion point during the search and what value to assign it first. This paper intro-duces a new decision heuristic that has been found to be efficient on real-world hard industrial benchmarks. It is designed to increase the likelihood that interrelated vari-ables will be chosen in proximity.
In recent years, the field has been a witness to a breakthrough in the design of deci-sion heuristics that are empirically successful on real-world industrial SAT i nstances. The key observation was that the decision heuristic must be dynamic, that is, it must re-focus the search on recently derived conflict clauses. V SIDS [13]—the first such dynamic heuristic—maintains a score for each literal. The score is increased when
the literal appears in a conflict clause; once in a while, scores are halved. This strat-egy ensures that the prover picks literals that were involved in the derivation of recent conflict clauses.
Another well-known decision heuristic, which proved to be even more successful than VSIDS on benchmark industrial instances, is Berkmin’s [5]. Its authors claimed that VSIDS is not sufficiently dynamic, in the sense that it may still pick literals that are irrel evant to the currently explored branch. Instead, they proposed organizing all conflict clauses in a list and picking the next decision literal from the top-most unsat-isfied clause on the list. If no such clause exists, a secondary VSIDS-like choice-heuristic is used. Berkmin’s heuristic is indeed more dynamic than VSIDS, but we find another advantage of Berkmin’s heuristic over VSIDS, in that it tends to pick in-terrelated variables, that is, variables whose joint assignment increases the chances of both quickly reaching a conflict in an unsatisfiable branch, as well as satisfying and removing “problematic” clauses in satisfiable branches. However, this potential a d-vantage is diluted by the fact that Berkmin does not put the initial clauses on the clause list and applies a secondary VSIDS-like heuristic.
Our proposal, which we call the clause-based heuristic (CBH), maintains a clause list containing both the initial and the conflict clauses, thus increasing the chances of picking interrelated variables. The next decision literal is picked from the top-most unsatisfied clause. No secondary heuristic is required. We propose various methods of initially organizing the clause list and for moving clauses within it. Our approach results in a significant performance boost over both VSIDS and Ber kmin’s heuristic.
Basic definitions are provided in the next section. In Section 3, we r eview the DPLL algorithm [3], enhanced by Boolean constraint propagation [17] and conflict clause recording [12]. Readers familiar with this material—included in the paper to make it self-contained—may skip ahead. Some of the commonly used decision heu-ristics are summarized in Section 4. In Section 5, we present our clause-based heuris-tic. In Section 6, we report on experiments that show that CBH is superior to VSIDS, Berkmin’s decision heuristic and the Berkmin-like decision heuristic of zChaff-2004, when tested on hard industrial benchmarks used in the SAT’04 competition [9]. This is followed by a brief conclusion.
2 Basic Definitions
In what follows, we denote negation by ?, conjunction by ∧ and disjunction by ∨. A literal is a variable or its negation. We use p, q, r, etc., for literals. A clause is a dis-junction of literals, usually denoted by letters A, B, …. The number of literals in a clause A is denoted by |A|. A conjunctive normal form (CNF) formula is a conjunc-tion of clauses, like ? = (p) ∧ (?p∨q) ∧ (?q∨?r). It is sometimes convenient to represent a CNF formula as a set of clauses, rather than as a conjunction. For exa m-ple, an alternative way to represent ? is as the set { p, ?p∨q, ?q∨?r }. We denote an empty clause by ?.
An(partial) assignment (σ) is a (partial) function assigning a truth value, 1 or 0, to all (some) variables. An example is the assignment τ(p) = τ(r) = 1, τ(q) = 0. It is sometimes convenient to consider a (partial) assignment as a subset of literals. For
example, an alternative way to represent τ is as {p, ?q, r}. A CNF formula ? evalu-ates under partial assignment σ to σ[?] by applying the following rules for each vari-able p:
1. If σ(p) = 1, remove ?p from ?’s clauses and remove clauses containing p from
?.
2. If σ(p) = 0, remove p from ?’s clauses and remove clauses containing ?p from
?.
If σ[?] contains no clauses, then σ[?] = 1 and σ is a satisfying assignment or a model for ?, and we say that ?=1 under σ. If ?∈σ[?], then σ is an unsatisfying assign-ment for ?, and we say that ?=0 underσ. Formula ? is satisfiable if there exists an assignment σ, such that ?=1 under σ; otherwise, it is unsatisfiable.
3 Enhanced DPLL
The DPLL algorithm [3] is the basic backtrack-search algorithm for SAT. DPLL is based upon the validity of the following two rules:
1. Splitting Literal Rule: Let σ= {p} and τ = {?p}. Formula ? is satisfiable iff σ[?] or τ[?] is satisfiable.
2. Unit Clause Rule: Let σ = {p}. If there is a clause A∈? such that A = p, then ? is satisfiable iff σ[?] is.
Boolean constraint propagation (BCP) [17] is a process of extending a partial as-signment by repeatedly applying the unit-clause rule. A decision variable is a vari-able assigned as a result of an application of the splitting rule. A decision literal is the assigned literal of a decision variable. An implied variable is a variable assigned as a result of the BCP process. An implied literal is the assigned literal of an implied variable. The decision level of an assigned variable p is one more than the number of decision variables assigned prior to p.
Let ? be an input formula. Modern complete SAT solvers implement the DPLL algorithm in the following way: A partial assignment σ, initialized to the empty par-tial assignment, is maintained. The partial assignment σ is extended by executing the BCP process. Then, if the input formula ? is neither 1 nor 0 under σ, the splitting rule is used, that is, the satisfiability of ? is recursively checked under σ∪{p} and then under σ∪{?p}, as required. The literal p is picked using some decision heuristic.
Next we describe a powerful technique, used by all the modern SAT solvers, namely conflict clause recording [12]. A conflict is a situation during DPLL invoca-tion when a formula ? evaluates to 0 under the current assignment σ. After such a conflict, it is possible to identify an a ssignment τ?σ, such that if χ is an assignment containingτ, extended by invoking BCP on τ[?], then? is 0 under χ. In other words, applying τ to ? is sufficient to create the same conflict as applying σ to ? up to BCP. We refer to such a partial assignment τ as a conflict-sufficient assignment. Let τ be a conflict-sufficient assignment. Then a clause A consisting of the negations of all the literals contained in τ is referred to as a conflict clause. The process of adding con-flict clauses to the formula after each conflict is referred to as conflict clause r e-cording. Conflict-clause recording prevents DPLL from re-exploring the same sub-
space during the subsequent search. Diffe rent conflict recording schemes add conflict clauses corresponding to different conflict-sufficient assignments. The 1UIP conflict recording scheme [12,13] has empirically been shown to be the most efficient [15,18]. In what follows, we describe the 1UIP conflict clause identification.
First, we define an “implication clause” for an assigned literal p. Let σ be a partial assignment held by DPLL prior to assigning p. The implication clause of an implied literal p, denoted by ic(p), is the clause that became a unit under σ and made the im-plication of p possible, during BCP. The implication clause of a decision literal p is the empty clause ?. After a conflict, a conflicting variable r is the variable that had been assigned last, before it was identified that σ is an unsatisfying assignment. Both literals of a conflicting variable are referred to as conflicting literals. According to the definition of an unsatisfying a ssignment, an empty clause must belong to σ[?]. It means that there is a clause F∈? that is 0 under σ. One of the conflicting literals must belong to F, otherwise r would not have been the last variable that was assigned a value. We denote a conflicting literal that belongs to F by ecl(r). For example, if ?= (p∨r) ∧ (p∨?r); σ = {?p, r}, then σ[?] = 0; p∨?r is the 0-clause under σ; r is the conflicting variable and ecl(r) = ?r. Observe that the implication clause is well defined for ?ecl(r), since ?ecl(r) is an assigned literal. However, the implication clause is not defined for ecl(r), since ecl(r) is not an assigned literal. We extend the definition of the implication clause by setting ic(ecl(r)) =F. Note that by the ex-tended definition, the conflicting variable has two implication clauses (one for each literal.)
An implication graph is a directed acyclic graph, in which each vertex is associated with a literal corresponding to an assignment along with its decision level. There is a directed edge from vertex p to vertex q if ic(q) = A and ?p∈A. Consider the example in Fig. 1, which illustrates concepts presented in this section. DPLL has been invoked on formula ? and the situation at the time of the first conflict is shown in Table 1. The implication graph is shown at the bottom. The decision literals ?y, ?p and ?s do not have incoming edges. Any implied literal y has | ic(y) | – 1 incoming edges.
Now we are ready to describe the concept of conflict recording schemes. Any con-flict clause is generated by a conflict cut of the implication graph. All the decision lit-erals are on one side of the conflict cut (called the reason side). Both conflicting lit-erals are on the other side of the conflict cut (called the conflict side). All vertices on the reason side that have at least one edge to the vertices of the conflict side comprise the conflict’s reason. Let τ?σbe a partial assignment containing only the assigned literals corresponding to the reason of a conflict cut. The partial assignment τ is a conflict-sufficient assignment.
Next, we describe the 1UIP learning scheme. Let p and q be two literals assigned at the same decision level dl. Then, in an implication graph, p is said to dominate q, if and only if any path from the decision variable corresponding to level dl to q contains p. A unique implication point (UIP)[12] is an assigned literal of the highest decision level dominating both literals of the conflicting variable. Observe that the decision literal corresponding to the highest decision level is always a UIP. The 1UIP learning scheme requires that any literal assigned after the first UIP (counting from the con-flicting literals) will be on the conflict side of the corresponding conflict cut and all others will be on the reason side. Consider again the exa mple in Fig. 1. The literals t
and ?s are UIP’s, since both dominate x and ?x . The literal t is the first UIP, since it is closer than ?s to the conflicting literals. The 1UIP cut is shown on Fig. 1. The corresponding conflict clause is ?q ∨ ?t .
A 1 = ?w ∨ s ∨ v ∨ ?q ; A 2 = p ∨ q ; A 3 = ?q ∨ r ∨ s ∨ ?w ; A 4 = ?q ∨ ?t ∨ x ; A 5 = s ∨ ?r ∨ ?v ∨ t ; A 6 = ?t ∨ ?x ; A 7 = y ∨ w ;
? = A 1 ∧ A 2 ∧ A 3 ∧ A 4 ∧ A 5 ∧ A 6 ∧ A 7.
(1)
Fig. 1. An example of an implication graph and 1UIP conflict clause identification. The impli-cation graph corresponds to a DPLL invocation on Formula (1). The related variable values, implication clauses and decision level of the variables are shown on Table 1
Table 1. The implication graph and 1UIP conflict clause identification principles. The value, decision level and implication clause for each variable of formula (1) are shown. The corre-sponding implication graph and 1UIP conflict cut are displayed in Fig. 1
p q r s t v w x y σ
0 1 1 0 1 1 1 1
0 DL
2 2
3 3 3 3 1 3 1 ic ? 2 3 ? 5 1 7
4 ?
4 Existing Decision Heuristics
In this section, we describe the most widely used decision heuristics, known to be ef-ficient on real-world industrial benchmarks.
Early static heuristics (e.g. Jeroslaw-Wang [7], Literal Count [11]) picked the next variable based on the number of appearances (scores) of different variables in unsatis-fied clauses. A major drawback of such an approach is that score calculation requires visiting all the clauses at each node of the search tree, which implies a very significant
x [3] 1UIP cut. Conflict
clause: ?q ∨ ?t .
overhead. Another disadvantage of static heuristics is that they do not consider i n-formation that can be retrieved after a conflict during implication graph analysis. Heuristics based upon such analyses were found to be several orders of magnitude faster [5,13].
The first dynamic heuristic is called Variable State Independent Decaying Sum (VSIDS) [13]. According to VSIDS, each literal is associated with a counter cl(p), whose value is increased once a new clause containing p is added to the database. Counters are initialized to 0. Every once in a while, all counters are halved. The next literal to be picked is the one with the largest counter. Ties are broken random ly. Two major advantages of VSIDS over the previous heuristics are that: (1) VSIDS is characterized by a negligible computational cost; (2) VSIDS gives preference to liter-als that participate in recent conflicts, i.e. it is dynamic. MiniSat SAT solver [4] im-plements a variant of VSIDS. Instead of infrequent halving of the scores, MiniSat multiplies the scores after each conflict by 0.95. This makes the heuristic more dy-namic.
The authors of the Berkmin SAT solver [5] proposed a successful decision heuris-tic that has been partially or fully adopted by the most modern SAT solvers, such as the 2004 version of zChaff [10], Satzoo [4], and Oepir [1]. We show, in the experi-mental section, that Berkmin’s heuristic is indeed faster than VSIDS on hard indus-trial benchmarks. The main diffe rence of Berkmin’s heuristic, when compared to VSIDS, is as follows: Conflict clauses are organized in a list, and every new conflict clause is appended to the head of the list. The next decision variable is picked from the top-most unsatisfied clause. If no such clause exists, the next decision variable is chosen according to a VSIDS-like heuristic. We will describe Berkmin’s heuristic in detail and analyze why it is advantageous over VSIDS.
Berkmin maintains a counter cl′(p) measuring the contribution of each literal to the search. Unlike VSIDS, Berkmin augments cl′(p), not only for literals that belong the conflict clause itself, but also for literals that belong to one of the clauses that were traversed in the implication graph during 1UIP conflict clause identification. At in-tervals, Berkmin divides all the counters by 4 (compared to 2 for VSIDS). Let cv′(p) be a counter measuring the contribution of each variable to the conflicts, defined as cl′(p) + cl′(?p). Berkmin m aintains all conflict clauses in a list. After each conflict, the new conflict clause is appended to the top of the list. The next decision variable is one with the highest cv′(p) out of all the variables of the topmost unsatisfied clause. If no conflict clauses exist yet, or if all the conflict clauses are satisfied, then the vari-able with the highest cv′(p) of all unassigned variables is chosen.
Next, we describe how Berkmin decides which literal, out of the two possible liter-als of the already chosen variable, to pick. Berkmin maintains a counter gcl(p), measuring the global contribution of each literal to the conflicts. The counter gcl(p) is initialized to 0 and is increased whenever cl′(p) is increased, but is not divided by a constant. If a topmo st unsatisfied clause exists, Berkmin picks a literal with the high-est global score gcl(p). Ties are broken randomly. If there is no unsatisfied topmost clause, then Berkmin picks the literal with the highest value of two(p), where two(p) approximates the number of binary clauses in the neighborhood of literal p. The func-tion two(p) is computed as follows: First, the number of binary clauses containing p is calculated. Then, for each binary clause B, containing p, the number of binary clauses containing ?q is computed, where q is the other literal of B. The sum of all computed
numbers gives the value of two(p). To reduce the amount of time spent computing two(p), a threshold value of 100 is used. As soon as the value of two(p) exceeds the threshold, its computation is stopped. Once again, ties are broken randomly.
The most important advantage of Berkmin's approach over VSIDS, as stated by the authors of Berkmin, is its additional dynamicity. It quickly adjusts itself to reflect changes in the set of variables relevant to the currently explored branch. Indeed, Berkmin picks variables from fresh conflict clauses and thus uses very recent data. Our understanding is that there is another important advantage of Berkmin’s heuristic over VSIDS: newly assigned variables tend to embrace more interrelated variables. By “interrelated,” we mean variables whose joint assignment increases the chances of both quickly reaching a conflict in an unsatisfiable branch and satisfying out “prob-lematic” clauses in satisfiable branches. According to Berkmin’s heuristic, a series of new decision variables appear in recent conflict clauses. Hence, these variables were recently traversed during conflict analysis and consequently contributed to conflict derivation. Moreover, even if the top-most conflict clauses were recorded a long time ago, the fact that their variables appeared closely together during conflict analysis, hints that they are interrelated. However, the impact of this advantage is diluted by the fact that Berkmin does not put the initial clauses on the list, but instead uses VSIDS as a secondary heuristic. The novel CBH heuristic, described in the next sec-tion, takes advantage of this observation.
5 The Clause-Based Heuristic
In our clause-based heuristic (CBH), all clauses (both the initial and the conflict clauses) are organized in a list. After each conflict, the conflict clause is prepended to the top of the list. Conflict-responsible clauses, that is, clauses visited during 1UIP conflict-clause identification, are placed just after the new conflict clause. The next decision literal is picked from the topmost unsatisfied clause of the list. One can see that CBH is highly dynamic, since recently visited clauses are placed at the top of the list. Also, CBH organizes the list in such way that clauses that were responsible for a recent conflict are placed together. Hence, when one picks a series of decision vari-ables after backtracking, it will tend to embrace interrelated variables. Indeed, when literals are picked from the same clause they must be related, even if the clause is an initial clause. When literals are picked from closely-placed clauses, they also tend to be related, since the list is organized in such a way that interrelated clauses are near each other, by placing conflict clauses at the top and moving conflict-responsible clauses towards the top.
As a variant, CBH can also move clauses found to have exactly two unassigned lit-erals during BCP to the top of the list. We refer to this strategy as 2LitFirst. The added value of this strategy is that: (1) more implications are learned during BCP; (2) short and potentially contradictory clauses tend to be immediately satisfied. The first point guides the solver to find conflicts in an unsatisfiable area, and the second one is useful to eliminate conflicts in a satisfiable area. The disadvantages of 2LitFirst are that: (1) it tends to separate between clauses that contain interrelated variables; (2) it may promote clauses that have never been responsible for conflicts.
We found experimentally that while usually 2LitFirst hurts performance, it may be helpful for instances having high clause/variable ratio. This can be explained by the fact that, in instances having a high clause/variable ratio, variables tend to appear in a greater number of clauses, since there are fewer variables per clause overall. Hence, two chains of decisions taken using different decision strategies tend to contain more common variables. This gives more weight to the order between variables and the lo-cal context of the search. One should prefer variables whose assignment can have an immediate impact; this is exactly what 2LitFirst does. The default version of CBH invokes 2LitFirst on instances where the clause/variable ratio exceeds 10.
One can see that the major differences of CBH comparing with Berkmin’s heuristic are the following:
(1)Both the initial and the conflict clauses, rather than only conflict clauses, are
organized in a list; therefore, a second choice heuris tic is not required. Thus,
any set of decision variables picked by CBH tends to contain more variables
from the same clause.
(2)After a conflict, in addition to the conflict clause, a number of clauses r e-
sponsible for the conflict (including initial clauses) are moved towards the
head of the list. Thus, clauses that are placed nearby are likely interrelated.
(3)As a variant, clauses that were discovered to have two unassigned literals are
moved towards the top of the list.
CBH can be easily implemented using a doubly-linked list. A pointer to the cur-rently watched clause C, initialized to the top-most clause, is maintained. When a de-cision is required, we seek the top-most unsatisfied clause A, starting from C towards the bottom of the list, and pick a literal from A (as described in Section 5.1). Observe that if no top-most unsatisfied clause exists, then we have a satisfying assignment, since all the clauses, including the original ones are satisfied. After each conflict, the solver updates the clause list and sets the currently watched clause to point to the top of the list.
The next subsection explains how CBH chooses the decision literal from the top-most unsatisfiable clause. Subsection 5.2 explains the initial organization of the clause list. Subsection 5.3 describes conflict-responsible clause identification in greater detail.
5.1 Choosing the Decision Literal from the Top-Most Clause
CBH maintains two counters, lcl(p) and gcl(p), measuring the local and global contri-butions of each literal to the conflicts, respectively. The counter lcl(p) is initialized to 0 for each p, while gcl(p) is initialized with the number of p’s appearances in initial clauses. Both counters are incremented whenever a literal belongs to one of the clauses traversed in the implication graph during 1UIP conflict-clause identification. Occasionally, the value of lcl(p) is divided by 2.
CBH also maintains two counters for variables lcv(p) and gcv(p), measuring the contribution of each variable to the conflicts. We define:
lcv(p) = (lcl (p) + lcl (?p)) + 3 ?min(lcl (p), lcl (?p)).
(2)
The first term gives preference to variables for which both literals are important, and the second term eliminates variables where only one literal is important. In a similar manner, we have:
gcv(p) = (gcl (p) + gcl (?p)) + 3 ?min(gcl (p), gcl (?p)).
(3)
CBH chooses the decision variable from the topmost unsatisfied clause using the following algorithm: A variable p with maximal lcv(p) is chosen, so as to give prefer-ence to variables that participated in recent conflicts. Ties are broken by preferring variables with maximal global score gcv(p). According to the next criterion, variables that used to have the maximal decision level when assigned the last time are pre-ferred. (If there still is a tie, it is broken by picking the lexicographically smallest variable.)
CBH chooses the decision literal out of the two possible, based on the global con-tribution value gcl(p).
5.2 Initial Clause-List Organization
In general, we aim to:
(1)place clauses containing frequently appearing literals near the top of the list,
and
(2)place clauses containing common literals nearby.
Point (1) guides the solver to start the search using frequent literals, and the second point increases the chances of picking interrelated literals.
First, the initial global score igs(p) is calculated for each literal p. The function igs(p) is initialized to 0 and is augmented for each clause that contains the literal p. The initial global score reflects the overall frequency of a literal.
In the process of clause list construction, we also maintain the initial l o cal score ils(p) for each literal p. It is calculated similarly to igs(p), except that only clauses that were already placed on the clause lis t are considered. The local score reflects the involvement of p in clauses that have been already appended to the clause list. Ini-tially, no clauses are included in the clause list, hence ils(p)=0 for each literal p. We also define the initial overall score ios(p) = igs(p) + ils(p) for each literal p. The ini-tial overall score takes into consideration both the local and global influence of each literal.
So far, we have defined three functions for each literal reflecting its global, local and overall influence. Now, we can define the initial variable overall score for each variable p:
iosv(p) = (ios(p) + ios(?p)) + 3 ? min(ios(p), ios(?p)).
(4)
The clause list is constructed by repeating the following procedure until all the clauses are placed on the clause list: Let p be the variable having the maximal variable overall score amongst all variables that have not already been picked. (Ties are bro-ken by preferring the smaller variable according to lexicographical order.) We append clauses containing the variable p that have not yet been a ppended to the end of the
clause list. Local and overall scores are updated for each literal participating in clauses that have been appended to the list. Such dynamic update of scores does not require any overhead, given that we use a priority queue, indexed by the scores. L it-erals can be moved within the queues in constant time.
5.3 Conflict-Responsible Clauses
Recall that after a conflict, conflict-responsible clauses are placed at the head of the list, after the new conflict clause. A clause is responsible for a conflict (in the context of CBH) if it appears in the implication clause of either a literal that appears on the conflict side of the 1UIP cut, or else of a literal whose negation appears in the 1UIP conflict clause.
Consider the example in Fig. 1. Clauses A4, A6, A2 and A5 are responsible for the conflict. Indeed, clauses A4 and A6 are the implication clauses of the two literals x and ?x that appear on the conflict side of the 1UIP cut, and clauses A2 and A5 are the im-plication clauses of the literals q and t, whose negation appear in the conflict clause.
From a practical point of view, it is not hard to identify clauses that are responsible for the conflict, since these are all the clauses that were visited during 1UIP conflict-clause construction.
6 Experimental Results
We implemented CBH within two SAT solvers. The first is the newest version of the famous zChaff solver, namely zchaff_2004.11.15 [10]. zChaff won first place in the Industrial-Overall category of the SAT’04 competition [9]. This new version of zChaff implements Berkmin’s heuristic, in contrast with the old version of zChaff [13] which used VSIDS. The performance of zChaff was measured on a m achine with 4Gb of memory and two Intel? Xeon TM CPU 3.06GHz processors with hyper-threading. The second solver we used in our experiments is SE—a Chaff-like solver, implementing 1UIP conflict-clause recording [13], non-chronological backtracking [12], frequent search restarts [5,6,10] and aggressive clause deletion [2,5,10] strate-gies. We designed SE to implement t he aforementioned enhancements of DPLL, since they play a crucial role for the performance of a modern SAT solver, tuned for industrial benchmarks, as explained in papers on Berkmin [5] and the newest zChaff [10]. We did not check whether CBH boosts performance when one or more of the above mentioned techniques is not used. The performance of SE was measured on a stronger machine with 4Gb of memory and two Intel? Xeon TM CPU 3.20GHz proces-sors with hyper-threading.
In what follows, we first analyze the overall performance of CBH versus Berk-min’s heuristic, VSIDS and Berkmin-like new zChaff’s heuristic. We show that CBH outperforms both Berkmin’s heuristic and VSIDS within the SE SAT solver, and also that CBH significantly outperforms zChaff’s new Berkmin-like heuristic. Then, we analyze how various strategies used by CBH contribute to its performance. We tested CBH inside two solvers to ensure that its measured impact on performance is inde-
pendent of the implementation details of a particular solver. The main measure for success in our experiments is the number of solved instances within an hour on hard industrial fam ilies used during SAT’04 competition [9]. We find this m easure, which was used during the SAT’04 competition, more convincing than a comparison of the number of decisions or conflicts (omitted for lack of space), since reducing the run-ning time is the final goal of any practical heuristic. Our experiments required a p-proximately 35 days of computation.
Table 3 compares the performance of CBH, VSIDS, VSIDSM—MiniSat-like VSIDS with frequent scores decay and Berkmin’s heuristic, implemented in SE, on eight hard industrial families used during the SAT’04 competition (downloaded from the competition’s website [16]). The description of these families is provided in Ta-ble 2. VSIDSM mult iplies the score by 0.95 after every 10 conflicts, rather than with each conflict. The latter rate is used within MiniSat, but the former is preferable within SE. Other heuristics decay the scores each 6000 conflicts. CBH solves at least as many instances within each family, when compared to either Berkmin’s heuristic or either version of VSIDS. CBH solves more instances than both version of VSIDS in 7 out of 8 cases, and solves more instances than Berkmin’s heuristic in 5 out of 8 cases.
Table 4 shows the performance of CBH within the new version of zChaff. T he performance of a version of CBH that does not use 2LitFirst is also provided. One can see that zChaff, enabled with CBH, outperforms zChaff in a very convincing manner for 6 out of 8 families and is inferior in only once case. Moreover, if the 2LitFirst strategy is not used, CBH is never inferior.
One can conclude that CBH definitely contributes to modern SAT-solver perform-ance, outperforming both VSIDS and Berkmin’s heuristic. Our experiments also con-firm that Berkmin’s heuristic is preferable to VSIDS, though the gap narrows if VSIDS uses frequent scores decay. This is to be expected, but—to the best of our knowledge—has never been reported, despite the fact that Berkmin’s heuristic has been partially or fully adopted by the most modern SAT solvers [1,4,5,10].
What remains is to analyze the performance of CBH when disabling some of its specific strategies. Accordingly, we consider CBH_NM, a version that does not move conflict-responsible clauses to the top of the list (but still appends the conflict clause itself to the top of the list), and CBH_NI, which does not use the initial strategy, de-scribed in Section 5.2, but rather appends all clauses to the list in the order of appear-ance in the input instance. We also experimented with CBH_2L_A, which always uses 2LitFirst, and with CBH_2L_N, which never does. Table 5 and 6 compare the performance of CBH within SE and zChaff respectively.
Switching off the initial strategy results in a performance degradation for 3 families within SE, and in performance gain for one family. In zChaff, switching off the ini-tial strategy resulted in performance degradation for 2 families. In general, the initial strategy improves the performance within both SE and zChaff, although it is not the most crucial factor contributing to CBH’s performance. E ven if the initial strategy is switched off, CBH performs better than other decision heuristics. This can be ex-plained by the fact that during the search, CBH quickly reorganizes the clause list to contain groups of interrelated clauses.
Switching off the moving of conflict-responsible clauses to the top of the list r e-sults in a performance degradation for 4 families and performance gain on 3 families
in zChaff. T he overall number of solved instances is higher when the strategy is switched on. Switching off the moving of conflict-responsible clauses to the top of the list leads to mixed results in the case of SE. Performance seriously degrades for the SCH family, and also for the ST2 family; however, there is a performance-boost for the GR, ST2B and VUN families. The overall number of solved instances r e-mains the same. One can conclude that moving the conflict-responsible clauses to the top of the list can be useful for some families, but hurt others. We recommend invok-ing it by default, since it results in an overall performance boost in the case of zChaff and does not hurt overall performance of SE.
Table 2. Description of the hard industrial benchmark families used in our experiments. Fam-ily name, number of instances in each family as well as the average, and maximal and minimal clause/variable ratios are provided
Abbrevia-tion Family Name Inst.
Num.
Cls/Var
Avrg
Cls/Var
Max
Cls/Var
Min
HEQ goldberg03-hard_eq_check 13 6.4 6.7 6.1
GR maris03-gripper 10 9.1 9.7 8.5
SCH schuppan03-l2s 11 3.2 3.3 3.0
ST2 simon03-sat02 9 3.3 4.2 2.7
ST2B simon03-sat02bis 10 23.9 71.9 2.9
CLR vangelder-cnf-color 12 42.9 195.4 4.0
PST velev-pipe-sat-1-1 10 33.7 33.8 33.7
VUN velev-vliw_unsat_2.0 8 15.6 20.1 10.7 Table 3. Performance of CBH vs. two versions of VSIDS and Berkmin’s heuristic, imple-mented in the SE SAT solver, on eight hard industrial families. The first column contains an abbreviated family name. Each pair of subsequent columns is dedicated to a specific heuristic,. The number of instances, solved within an hour, is provided
Family CBH solved Berkmin heur. VSIDSM VSIDS
HEQ 5 4 4 3
GR 1 1 0 1
SCH 5 2 2 0
ST2 5 4 4 2
ST2B 2 2 2 1
CLR 6 4 4 4
PST 10 10 4 5
VUN 4 2 1 0
ALL 38 29 2116 Regarding the impact of 2LitFirst strategy, first observe that according to Tables 5 and 6, invoking 2LitFirst on every instance does not pay off. Note that the default strategy used by CBH invokes 2LitFirst if the clause/variable rate is greater than 10. The motivating experimental observation for designing CBH in this manner is that SE without 2LitFirst performs the same as the default version on all families, except VUN, where performance seriously degrades. VUN is the only family, other than PST, for which the clause/variable ratio is greater than 10 for all instances. To con-
firm that SE performs better when 2LitFirst is invoked on instances with high clause/variable ratio, we launched SE on 26 handmade families that were submitted to SAT’04 (and are available at [16]). SE was able to solve at least 1 instance from 13 families. The default CBH strategy performed better than a strategy with 2LitFirst disabled on 2 out of 13 families, and it performed the same on other families. In the case of zChaff, we found that 2LitFirst invocation hurts performance in a dramatic manner on families with low clause/variable ratio, and leaves the performance the same or slightly degraded on families having high clause/variable ratio. The families HEQ, GR, SCH and ST2 are those with a ratio for all its instances lower than 10. If 2LitFirst is always used, zChaff is able to solve only 4 instances of these 4 families, comparing to 20 i nstances solved by the version that never uses 2LitFirst. The other 4 families have e ither mixed or high clause/variable ratio. On these families, when 2LitFirst is always used, zChaff is able to solve 12 instances, compared to 16 by a version that never uses 2LitFirst. One can conclude that within zChaff, 2LitFirst us-age is not justified. Overall, 2LitFirst performs much better on instances having a high clause/variable ratio.
Table 4. CBH vs. the default heuristic within zChaff_2004.11.15. CBH_2L_N is a version of CBH that does not use the 2LitFirst strategy
Family zChaff + CBH zChaff+ CBH_2L_N zChaff default
HEQ 8 8 4
GR 3 3 0
SCH 5 5 2
ST2 4 4 1
ST2B 2 2 1
CLR 3 4 1
PST 5 8 8
VUN 2 2 2
ALL 32 36 19
Table 5. Performance of different configurations of CBH in terms of solved instances within an hour in SE solver
Family CBH CBH_NM CBH_NI CBH_2L_A CBH_2L_N
HEQ 5 4 5 3 5
GR 1 2 2 0 1
SCH 5 2 4 5 5
ST2 5 4 5 2 5
ST2B 2 3 1 2 2
CLR 6 7 5 5 6
PST 10 10 10 10 10
VUN 4 6 4 4 1
ALL 38 38 36 31 35
Table 6. Performance of different configurations of CBH in terms of solved instances within an hour in zChaff solver
Family CBH CBH_NM CBH_NI CBH_2L_A CBH_2L_N
HEQ 8 4 8 4 8
GR 3 1 3 0 3
SCH 5 2 4 0 5
ST2 4 2 4 0 4
ST2B 2 3 2 2 2
CLR 3 3 3 3 4
PST 5 10 3 5 8
VUN 2 3 2 2 2
ALL 32 28 29 16 36
7. Conclusions
We have presented a novel clause-based heuristic, CBH. It maintains a clause list or-ganized in a manner that allows the algorithm to choose sequences of interrelated variables that were responsible for recent conflict derivation.
CBH maintains both the initial and the conflict clauses in a single list. The next decision literal is picked from the topmost u nsatisfied clause of the list. After each conflict, the conflict clause is prepended to the top of the list. Clauses visited during conflict-clause identification, are placed just after the new conflict clause. As a vari-ant, if the clause/variable ratio of the input instance is greater than a predefined value (10 is a reasonable choice), newly identified binary clauses are moved to the top of the list.
We have demonstrated that using CBH results in a significant performance boost on hard industrial families, when compared with Berkmin’s heuristic or VSIDS. Acknowledgements
The work of the third author was carried out in partial fulfillment of the requirements for a Ph.D. We thank Ranan Fraer for his careful reading and helpful comments. References
[1] J. Alfredsson. The SAT solver Oepir. URL
http://www.lri.fr/~simon/contest/results/ONLINEBOOKLET/OepirA.ps (viewed Janu-ary, 16 2005).
[2] R. Bayardo, Jr., and R. Schrag. Using CSP look-back techniques to solve real-world
SAT instances. In Proceedings of the National Conference on Artificial Intelligence, pp.
203-208, 1997.
[3] M. Davis, G. Logemann and D. Loveland. A machine program for theorem proving.
In Communications of the ACM, (5): 394-397, 1962.
[4] N. Eén and N. S?rensson. An extensible SAT-solver. In Proceedings of Sixth Interna-
tional Conference on Theory and Applications of Satisfiability Testing (SAT2003), May 2003.
[5] E. Goldberg and Y. Novikov. BerkMin: A fast and robust SAT-solver. In Design,
Automation, and Test in Europe (DATE '02), p. 142-149, March 2002.
[6] C.P. Gomes, B. Selman and H. Kautz. Boosting combinatorial search through ran-
domization. In Proceedings of the National Conference on Artificial Intelligence, July 1998.
[7] R.G. Jeroslaw and J. Wang. Solving propositional satisfiability problems. In Annals of
mathematics and Artificial Intelligence, (1):167-187, 1990.
[8] H. Kautz, B. Selman. Planning as satisfiability. In Proceedings of the 10th European
conference on Artificial intelligence, 1992.
[9] D. Le Berre and L. Simon. Fifty-five solvers in Vancouver: The SAT 2004 competition.
In Proceedings of the Seventh International Conference on Theory and Applications of Satisfiability Testing (SAT2004), volume Lecture Notes in Computer Science, 2004. Ac-cepted for publication.
[10] Y.S. Mahajan, Z. Fu, S. Malik. ZChaff2004: an efficient SAT solver. In Proceedings of
the Seventh International Conference on Theory and Applications of Satisfiability Test-ing (SAT2004), volume Lecture Notes in Computer Science, 2004. Accepted for publica-tion.
[11] J.P. Marques-Silva. The impact of branching heuristics in propositional satisfiability
algorithms. In Proceedings of the 9th Portuguese Conference on Artificial Intelligence (EPIA), September 1999.
[12] J.P. Marques-Silva and K.A. Sakallah. GRASP: A search algorithm for propositional
satisfiability. IEEE Transactions on Computers, (48):506-521, 1999.
[13] M. Moskewicz, C. Madigan, Y. Zhao, L. Zhang and S. Malik. Chaff: Engineering
an efficient SAT solver. In Proceedings of the Design Automation Conference, 2001. [14] M. R. Prasad, A. Biere, A. Gupta. A survey of recent advances in SAT-based formal
verification, Intl. Journal on Software Tools for Technology Transfer (STTT), (7):156-173, January 2005.
[15] L. Ryan, Efficient algorithms for clause-learning SAT solvers. Masters thesis, Simon
Fraser University, February 2004.
[16] Seventh International Conference on Theory and Applications of Satisfiability Testing
(SAT2004), May 2004. URL https://www.360docs.net/doc/6e9857002.html,/SATCompetition/2004/ (viewed Septem-ber 1, 2004).
[17] R. Zabih and D.A. McAllester. A rearrangement search strategy for determining pro-
positional satisfiability. In Proceedings of National Conference on Artificial Intelli-gence, p. 155-160, 1988.
[18] L. Zhang, C.F. Madigan, M.H. Moskewicz and S. Malik. Efficient conflict driven
learning in a boolean satisfiability solver. International Conference on Computer-Aided Design (ICCAD'01), p. 279-285, November 2001.
人力资源管理系统测试报告
人力资源管理系统
一. 引言 (1) 1.1编写目的 (1) 1.2项目背景 (1) 1.3定义 (1) 1.4项目简介 (1) 1.5操作系统 (1) 1.6参考资料 (2) 二.测试范围 (2) 三.测试策略 (3) 3.1 测试完成标准 (3) 3.2 测试类型 (3) 功能测试 (3) 性能测试 (4) 用户界面(UI)测试 (4) 安全性与访问控制测试 (5) 兼容性测试 (6) 回归测试 (6) 测试实施阶段 (7) 四.测试计划 (7) 4.1测试阶段 (7) 4.2测试进度 (8) 4.3测试软件 (9) 4.4测试环境 (10) 五.测试项目说明 (10) 5.1单位测试 (10) 5.2测试用例 (15) 5.3安全性与访问控制测试 (23) 5.4兼容性测试 (23) 5.5 回归测试 (23) 六.报道总结 (24)
一.引言 1.1 编写目的 根据软件的功能及结构设计出相应的测试用例,目的在于尽可能发现程序中的存在的错误,并加以改正,以达到减低系统运行的故障,使交付到用户手中的系统是一个尽可能安全的、可靠的和有效地系统。本文档将为测试人员提供测试用例,对测试人员进行测试指导,使测试工作顺利进行。 1.2 项目背景 小组名称:ABC小组 项目名称:人力资源管理系统 1.3 定义 人力资源管理系统 1.4项目简介 1.4.1平台:主要使用.net平台用来完成人力资源管理系统。 1.4.2功能:本系统为员工、部门经理和管理员提供职工和 部门信息的填加,修改和删除功能,包括职工档案、员工履历、员工合同、部门名称、部门经理等,可以记录奖惩情况,包括获奖人员、奖惩时间、奖惩内容等,并能够维护和查询教育培训信息,最后还可以让系统管理员进行后台管理,包括组织管理、安全控制。 1.5操作系统:
SAT 2 数学重点大全
函数,方程 ● asymptote 渐近线。当在X 的某一个值时,分式的分母为0而分子不为0, 则函数有vertical asymptote 。当X 趋向于无限大(小)的时候,Y 趋近某一个数值,则函数有horizontal asymptote 。 ● 当在X 的某一个值,分式的分子分母同时是0,则函数有一个洞。 ● 在三角函数中,注意多角度的情况 ● 极坐标polar coordinates P(r ,θ)中,r 指的是长度,θ指的是角度。 ● ))(())((x g f x g f = ● 奇偶函数应该用负X 代入计算。 ● 解等式解方程的题目都可以用图像,左边和右边两个图像的交点就是解。 ● 等式()()()05322 =-+-x x x 所有解的和是多少? 注意2要加两次,因为它是双根。 ● 在图像计算机的window 里面,Yscl=50表示y 轴每一格间距是50。 ● 如果函数的对称轴是3-=x ,那么)3(-x f 的图像关于y 轴对称。 ● 求 21>-x x 的解集。应该分两大类0>x 和0
实验四 控制系统频率特性的测试(实验报告)
实验四 控制系统频率特性的测试 一. 实验目的 认识线性定常系统的频率特性,掌握用频率特性法测试被控过程模型的原理和方法,根据开环系统的对数频率特性,确定系统组成环节的参数。 二.实验装置 (1)微型计算机。 (2)自动控制实验教学系统软件。 三.实验原理及方法 (1)基本概念 一个稳定的线性定常系统,在正弦信号的作用下,输出稳态与输入信号关系如下: 幅频特性 相频特性 (2)实验方法 设有两个正弦信号: 若以)(t x ω为横轴,以)(y t ω为纵轴,而以t ω作为参变量,则随t ω的变化,)(t x ω和 )(y t ω所确定的点的轨迹,将在 x--y 平面上描绘出一条封闭的曲线(通常是一个椭圆)。这 就是所谓“李沙育图形”。 由李沙育图形可求出Xm ,Ym ,φ,
四.实验步骤 (1)根据前面的实验步骤点击实验七、控制系统频率特性测试菜单。 (2)首先确定被测对象模型的传递函数, 预先设置好参数T1、T2、ξ、K (3)设置好各项参数后,开始仿真分析,首先做幅频测试,按所得的频率范围由低到高,及ω由小到大慢慢改变,特别是在转折频率处更应该多取几个点 五.数据处理 (一)第一种处理方法: (1)得表格如下: (2)作图如下: (二)第二种方法: 由实验模型即,由实验设置模型根据理论计算结果绘制bode图,绘制Bode图。
(三)误差分析 两图形的大体趋势一直,从而验证了理论的正确性。在拐点处有一定的差距,在某些点处也存在较大的误差。 分析: (1)在读取数据上存在较大的误差,而使得理论结果和实验结果之间存在。 (2)在数值应选取上太合适,而使得所画出的bode图形之间存在较大的差距。 (3)在实验计算相角和幅值方面本来就存在着近似,从而使得误差存在,而使得两个图形之间有差异 六.思考讨论 (1)是否可以用“李沙育”图形同时测量幅频特性和想频特性 答:可以。在实验过程中一个频率可同时记录2Xm,2Ym,2y0。 (2)讨论用“李沙育图形”测量频率特性的精度,即误差分析(说明误差的主要来源)答:用“李沙育图形”测量频率特性的精度从上面的分析处理上也可以看出是比较高的,但是在实验结果和理论的结果之间还是存在一定的差距,这些误差主要来自于从“李沙育图形”上读取数据的时候存在的误差,也可能是计算机精度方面的误差。 (3)对用频率特性测试系统数学模型方法的评测 答:用这种方法进行此次实验能够让我们更好地了解其过程,原理及方法。但本次实验的数据量很大,需要读取较多坐标,教学软件可以更智能一些,增加一些自动读取坐标的功能。 七.实验总结 通过本次实验,我加深了对线性定常系统的频率特性的认识,掌握了用频率特性法测试被控过程模型的原理和方法。使我把书本知识与实际操作联系起来,加深了对课程内容的理解。在处理数据时,需要进行一定量的计算,这要求我们要细心、耐心,作图时要注意不能用普通坐标系,而是半对数坐标系进行作图。
sat2 math level2
MATH LEVEL2 抱歉这个看得比较少…… 如何使用CASIO 学生用计算器……(本人认为完全不用TI等高级货…中国小孩儿~手绘快~) MODE 2SD统计, 输入方法:A;B M+ 一组……………………之后SHIFT 【S-VAR】 standard deviation 标准偏差Xon-1 其他不用说了 MODE3REG求回归函数吧…… 常用的是LIN线性,LOG对数,QUAD二次,(废话……) 输入方法A,B M+ 一组…………………………之后SHIFT 【S-VAR】 线性:Y= a+ bX 二次:Y=a+bX+cX2 对数:Y=a+bInX indirect proof反证In an indirect proof of ―if p, then q,‖ you assume the negative of the conclusion rhombus 菱形 parallelogram 平行四边形 slant height斜高 pentagon五边形 挑几个我当时觉得有问题的给大家找找自信…… 41. If and , then AB =
(A) (B) (C) (D) (E) You left this question blank. You should have selected B. Explanation The number of rows in A equals the number of columns in form product matrix AB , multiply each row of B by each c arranging the six resulting entries in a 3-row, 2-column ma (B row 1)(A col. 1) = (3)(4) + (0)(1) = 12 (B row 1)(A = –9 (B row 2)(A col. 1) = (1)(4) + (5)(1) = 9 (B row 2)(A = 7 (B row 3)(A col. 1) = (–2)(4) + (4)(1) = –4 (B row 3)(A (4)(2) = 14 Topic:
过程控制系统实验报告
实验一过程控制系统的组成认识实验 过程控制及检测装置硬件结构组成认识,控制方案的组成及控制系统连接 一、过程控制实验装置简介 过程控制是指自动控制系统中被控量为温度、压力、流量、液位等变量在工业生产过程中的自动化控制。本系统设计本着培养工程化、参数化、现代化、开放性、综合性人才为出发点。实验对象采用当今工业现场常用的对象,如水箱、锅炉等。仪表采用具有人工智能算法及通讯接口的智能调节仪,上位机监控软件采用MCGS工控组态软件。对象系统还留有扩展连接口,扩展信号接口便于控制系统二次开发,如PLC控制、DCS控制开发等。学生通过对该系统的了解和使用,进入企业后能很快地适应环境并进入角色。同时该系统也为教师和研究生提供一个高水平的学习和研究开发的平台。 二、过程控制实验装置组成 本实验装置由过程控制实验对象、智能仪表控制台及上位机PC三部分组成。 1、被控对象 由上、下二个有机玻璃水箱和不锈钢储水箱串接,4.5千瓦电加热锅炉(由不锈钢锅炉内胆加温筒和封闭外循环不锈钢锅炉夹套构成),压力容器组成。 水箱:包括上、下水箱和储水箱。上、下水箱采用透明长方体有机玻璃,坚实耐用,透明度高,有利于学生直接观察液位的变化和记录结果。水箱结构新颖,内有三个槽,分别是缓冲槽、工作槽、出水槽,还设有溢流口。二个水箱可以组成一阶、二阶单回路液位控制实验和双闭环液位定值控制等实验。 模拟锅炉:锅炉采用不锈钢精致而成,由两层组成:加热层(内胆)和冷却层(夹套)。做温度定值实验时,可用冷却循环水帮助散热。加热层和冷却层都有温度传感器检测其温度,可做温度串级控制、前馈-反馈控制、比值控制、解耦控制等实验。 压力容器:采用不锈钢做成,一大一小两个连通的容器,可以组成一阶、二阶单回路压力控制实验和双闭环串级定值控制等实验。 管道:整个系统管道采用不锈钢管连接而成,彻底避免了管道生锈的可能性。为了提高实验装置的使用年限,储水箱换水可用箱底的出水阀进行。 2、检测装置 (液位)差压变送器:检测上、下二个水箱的液位。其型号:FB0803BAEIR,测量范围:0~1.6KPa,精度:0.5。输出信号:4~20mA DC。 涡轮流量传感器:测量电动调节阀支路的水流量。其型号:LWGY-6A,公称压力:6.3MPa,精度:1.0%,输出信号:4~20mA DC 温度传感器:本装置采用了两个铜电阻温度传感器,分别测量锅炉内胆、锅炉夹套的温度。经过温度传感器,可将温度信号转换为4~20mA DC电流信号。 (气体)扩散硅压力变送器:用来检测压力容器内气体的压力大小。其型号:DBYG-4000A/ST2X1,测量范围:0.6~3.5Mpa连续可调,精度:0.2,输出信号为4~20mA DC。 3、执行机构 电气转换器:型号为QZD-1000,输入信号为4~20mA DC,输出信号:20~100Ka气压信号,输出用来驱动气动调节阀。 气动薄膜小流量调节阀:用来控制压力回路流量的调节。型号为ZMAP-100,输入信号为4~20mA DC或0~5V DC,反馈信号为4~20mA DC。气源信号 压力:20~100Kpa,流通能力:0.0032。阀门控制精度:0.1%~0.3%,环境温度:-4~+200℃。 SCR移相调压模块:采用可控硅移相触发装置,输入控制信号0~5V DC或4~20mA DC 或10K电位器,输出电压变化范围:0~220V AC,用来控制电加热管加热。 水泵:型号为UPA90,流量为30升/分,扬程为8米,功率为180W。
sat2考试准备时间要多久
https://www.360docs.net/doc/6e9857002.html, SAT2考试准备时间要多久 SAT2考试一共有20个考试科目,包括中国考生最难受的数理化以及语言等科目,在20们考试科目中中国考生可以选择其实并没有那么多,之所以选择参加SAT2考试其实目的就是为自己的大学申请增添竞争力,所以一定要选择适合自己的科目,下面我们来看下SAT2考试准备时间要多久。 1.中国考生准备的SAT2考试科目 SAT2考试一共20个科目包括文学,生物,化学,物理,美国历史,世界历史,数学,法语,德语,西班牙语,现代希伯来语,意大利语,拉丁语,法语(有听力),德语(有听力),西班牙语(有听力),中文(有听力),日语(有听力),韩语(有听力)。但是其中语言的科目是不适合中国考生选择的,包括小语种,英语和汉语,无论是哪一种考生都不具有优势,即使考也不会为自己的申请增加分数的。其实是历史和生物和文学,这三个科目对于考生的专业知识和英语功底都要求比较高,所以不建议大家选择,那么适用于大部分考生选择的考试就是物理,化学和数学科目,下面我们就来为大家介绍这三科目准时间要多久。 2.SAT2数学备考时间 SAT2数学包括数学1和数学2两个科目但是数学1比较简单,考也是没有多大意义的,SAT数学2包括代数,平面几何,立体几何,坐标几何。代数和函数,三角学和坐标Level 2中所考比重较大,但是他所涉及的范围都是包括在初中数学范围以及高二之前的数学内容之内的,所以知识点都是大家熟悉的,可以不用担心。但是考生一定不能轻视,要有一套专门的备考资料进行练习,花费两个月的时间,每天规定出做题时间和数量进行练习,了解数学题型,掌握做题方法和做题技巧。 3.SAT2物理备考时间 物理考试内容包括运动学,动力学,能力和动量,圆周运动,简谐运动,重力,电磁学,波和光学,热力学,现代物理学,这个科目的考试要求考生熟练掌握物理学中的重要概念并能够灵活的解决物理中的实际问题,物理设计范围比较广,所以大家在备考要花费多一点的时间大约在2-3个月内完成物理的备考。》》更多详情点击SAT2物理备考 4.SAT2化学备考时间 化学所涉及到的考点包括结构,物质的状态,反应类型,化学计量学,热力学,描述化学,化学部分的知识点对于中国考生来说并不难,从初中就开始接触,高中更加深入一些,所以基础好点的考生不必担心化学的难度,但是练习还是一定要进行准备的,建议大家用2个月的时间进行化学的备考。》》更多详情点击SAT2化学备考 以上就是给大家分享的关于与SAT2考试准备时间多长的问题,更多内容请大家进入前程百利SAT2考试频道,小编会为大家持续更新最权威的考试资讯,预祝大家取得理想的SAT考试成绩。
软件系统测试报告(二)
软件系统测试报告 ——网上招聘系统 学院:计算机科学学院 背景: 如今网上招聘越来越普遍,但有些招聘系统的综合性能不是很好,
比如系统的冗余、系统的性能、安全性、完整性等等都有待提高,本次测试的目的就是针对本系统的性能进行测试。 一.实验目的 1、通过对测试结果的分析,得到对软件质量的评价 2、分析测试的过程,产品,资源,信息,为以后制定测试计划提供参考 3、评估测试测试执行和测试计划是否符合 4、分析系统存在的缺陷,为修复和预防bug提供建议 二、实验内容 该文档的目的是描述网上招聘系统项目客户端系统测试的总结报告,其主要内容包括: ●系统环境简介 1、软件名称:网上招聘求职系统 2、软件功能:为求职者提供求职、收藏、信息交互等功能;为招聘单位提供招聘、收藏、信息交互等功能;为管理员提供管理网站公告、友情链接和网站会员的管理功能。 3、用户:求职者、招聘单位、管理员 4、开发者:ZSS ●系统数据度量 ●系统结果评估 用户群:1、项目管理人员 2、测试人员 范围:该文档定义了客户端系统测试的结果,总结了测试客户端的
职位查询、网上提交简历、在线答题的基本功能,以及支持大数据量并发访问的性能,给出了测试的结论。 2.1严重bug:出现以下缺陷,测试定义为严重bug 系统无响应,处于死机状态,需要其他人工修复系统才可复原。 点击某个菜单后出现“The page cannot be displayed”或者返回 异常错误。 进行某个操作(增加、修改、删除等)后,出现“The page cannot be displayed”或者返回异常错误 2.2缩写说明 HR--- Human Resource(人力资源管理)的缩写。 MVC---Model-View-Control(模式-视图-控制)的缩写,表示一个三层的结构体系。 2.3测试类型 a、功能性测试:按照系统需求定义中的功能定义部分对系统实行的系统级别的测试。 b、非功能性测试:按照系统需求定义中的非功能定义部分(如系统的性能指标,安全性能指标等)对系统实行的系统级别的测试。 c、测试用例:测试人员设计出来的用来测试软件某个功能的一种情形 2.4参考资料 [1] 《LoadRunner使用手册》北京长江软件有限公司编制 [2] 《网上招聘客户端需求说明》北京长江软件有限公司编制
2020年SAT考试内容
2020年SAT考试内容 2020年SAT考试内容 一是通用考试----推理测验(Reasoning Test),包括阅读、写 作和数学,被称为SAT或NEW SAT; 第二个是单科考试-----专项测验(Subject Tests),有数学、 物理、化学、生物、外语(包括汉语、日语、德语、法语、西班牙语)等,被统称为SAT2。 SAT1主要测验考生的写作、阅读和数学水平,每部分满分是800分,总分是2400分;SAT2每科满分为800分。绝绝大部分美国名校只要求中国留学申请人提供SAT通用考试的成绩即可,个别院校及专业 要求申请人提供SAT2的单科考试成绩。 考试内容: SAT1考试时间三小时四十五分钟 题型为选择题及写作,主要测验考生的阅读、数学及写作水平 满分 2400分 SAT2考试时间一小时 题型绝大部分为选择题,主要考察考生某一专业的知识。 SAT2是单科考试,有数学、物理、化学、生物、外语(包括汉语、日语、德语、法语、西班牙语)等,根据各专业和学校的要求参加。 下面主要介绍SAT通用考试: SAT (Reasoning Test)的考察重点 写作(writing)三个部分 第一部分 writing test 议论文写作
第二部分改错,句子错误和语篇错误 阅读(Critical reading) 第一部分句子补充 19道题 第二部分阅读文章理解分析 48道题 数学(Math section) 第一部分44道选择题 第二部分 10道解答题 SAT考试包括10部分,考试部分包括5个25分钟的选择题部分(2个数学、2个阅读和1个写作语法技能部分),2个20分钟的选择题部分(1个数学、1个阅读部分)、1个10分钟的选择题部分(写作语法技能部分)、以及1个25分钟的写作部分。其余的一部分是加试题;加试题是一个25分钟的选择题,内容可能涉及数学、阅读或者写作技能。加试题不计入成绩,但是考生无法判断哪一部分是加试题。 阅读部分包括:句子填空和阅读理解,一共67道题,阅读理解部分分长文章和短文章 每个部分的句子填空题大致是按难度顺序出现的,先出现的题目比较简单;阅读理解部分不按难度排序。 数学部分包括:选择题和10道主观填空题,共有54道题目 每个部分的数学部分大致是按难度顺序出现的,先出现的题目比较简单; 写作部分包括:语篇写作、句子找错、修改句子、修改语篇 除了10道数学部分的主观题填空题,其余的部分都是选择题。
测试过程控制及样例
测试过程控制及样例
测试过程控制及样例 1目的 确保测试的有效性和验证结果的可靠性,从而保证软件实现阶段质量和最终质量。并作为验证及确认软件版本发布、项目验收的依据。 2适用范围 部门:应用开发事业部总监、系统测试部、软件部门、业务部门。 业务:模块测试、系统测试,β测试及试运行测试结果的收集。 3职责 1)1)系统测试部经理负责组织测试人员编写测试工作计划和测试大纲,审 核测试记录和测试报告,申请发布β测试版或软件试运行。 2)2)测试人员按照测试工作计划和测试大纲进行测试,填写测试记录,编 写系统测试报告和用户测试报告。 3)3)业务部门负责提供用户测试名单,系统测试部收集β测试结果 4)4)应用开发事业部(副)总监审批测试报告,批准β测试版发布或软件 试运行,通知业务部门。 5)5)市场部为产品发布做准备。 6)6)总经理批准紧急放行。 7)7)系统测试部负责解释和修订本程序文件。 4工作程序 1)1)测试准备 除单元测试外,在进行各种测试前应准备做好如下准备: ●●配备测试用硬件环境; ●●建立相应的运行环境和网络环境; ●●准备测试数据; 2)2)测试依据 测试依据主要包括:测试工作计划、测试大纲、上阶段测试记录、上版软件产品用户反馈意见记录等。 3)3)测试工作计划及测试大纲 系统测试部经理组织测试人员按照/3-07/QR/001《测试工作计划》编写测试工作计划,测试工作计划应主要包括测试进度、人员安排、设备环境的建立等。测试工作计划经应用开发事业部(副)总监批准后实施。 系统测试部经理组织测试人员,根据软件《需求分析规格说明书》、《软件设计说明书》,按照/3-07/QR/002《测试大纲编写指南》编写测试大纲。 测试大纲作为测试的主要依据,测试大纲经应用开发事业部(副)总监批准后实施。
实验四 控制系统频率特性的测试 实验报告
实验四控制系统频率特性的测试 一.实验目的 认识线性定常系统的频率特性,掌握用频率特性法测试被控过程模型的原理和方法,根据开环系统的对数频率特性,确定系统组成环节的参数。二.实验装置 (1)微型计算机。 (2)自动控制实验教学系统软件。 三.实验原理及方法 (1)基本概念 一个稳定的线性定常系统,在正弦信号的作用下,输出稳态与输入信号关系如下: 幅频特性相频特性 (2)实验方法 设有两个正弦信号: 若以) (y tω为纵轴,而以tω作为参变量,则随tω的变xω为横轴,以) (t 化,) (y tω?所确定的点的轨迹,将在 x--y平面上描绘出一条封闭的xω和) (t 曲线(通常是一个椭圆)。这就是所谓“李沙育图形”。 由李沙育图形可求出Xm ,Ym,φ, 四.实验步骤 (1)根据前面的实验步骤点击实验七、控制系统频率特性测试菜单。(2)首先确定被测对象模型的传递函数, 预先设置好参数
T1、T2、ξ、K (3)设置好各项参数后,开始仿真分析,首先做幅频测试,按所得的频率范围由低到高,及ω由小到大慢慢改变,特别是在转折频率处更应该多取几个点 五.数据处理 (一)第一种处理方法: (1)得表格如下: (2)作图如下: (二)第二种方法: 由实验模型即,由实验设置模型根据理论计算结果绘制bode图,绘制Bode图。 (三)误差分析 两图形的大体趋势一直,从而验证了理论的正确性。在拐点处有一定的差距,在某些点处也存在较大的误差。 分析: (1)在读取数据上存在较大的误差,而使得理论结果和实验结果之间存在。 (2)在数值应选取上太合适,而使得所画出的bode图形之间存在较大的差距。 (3)在实验计算相角和幅值方面本来就存在着近似,从而使得误差存在,而使得两个图形之间有差异 六.思考讨论 (1)是否可以用“李沙育”图形同时测量幅频特性和想频特性
自动控制完整系统综合实验综合实验报告
综合实验报告 实验名称自动控制系统综合实验 题目 指导教师 设计起止日期2013年1月7日~1月18日 系别自动化学院控制工程系 专业自动化 学生姓名 班级 学号 成绩
前言 自动控制系统综合实验是在完成了自控理论,检测技术与仪表,过程控制系统等课程后的一次综合训练。要求同学在给定的时间内利用前期学过的知识和技术在过程控制实验室的现有设备上,基于mcgs组态软件或step7、wincc组态软件设计一个监控系统,完成相应参数的控制。在设计工作中,学会查阅资料、设计、调试、分析、撰写报告等,达到综合能力培养的目的。
目录 前言 (2) 第一章、设计题目 (4) 第二章、系统概述 (5) 第一节、实验装置的组成 (5) 第二节、MCGS组态软件 (11) 第三章、系统软件设计 (14) 实时数据库 (14) 设备窗口 (16) 运行策略 (19) 用户窗口 (21) 主控窗口 (30) 第四章、系统在线仿真调试 (32) 第五章、课程设计总结 (38) 第六章、附录 (39) 附录一、宇光智能仪表通讯规则 (39)
第一章、设计题目 题目1 单容水箱液位定值控制系统 选择上小水箱、上大水箱或下水箱作为被测对象,实现对其液位的定值控制。 实验所需设备:THPCA T-2型现场总线控制系统实验装置(常规仪表侧),水箱装置,AT-1挂件,智能仪表,485通信线缆一根(或者如果用数据采集卡做,AT-4 挂件,AT-1挂件、PCL通讯线一根)。 实验所需软件:MCGS组态软件 要求: 1.用MCGS软件设计开发,包括用户界面组态、设备组态、数据库组态、策略组态等,连接电路, 实现单容水箱的液位定值控制; 2.施加扰动后,经过一段调节时间,液位应仍稳定在原设定值; 3.改变设定值,经过一段调节时间,液位应稳定在新的设定值。
东南大学自控实验报告实验三闭环电压控制系统研究
东南大学自控实验报告实验三闭环电压控制系统研究
东南大学 《自动控制原理》 实验报告 实验名称:实验三闭环电压控制系统研究 院(系):专业: 姓名:学号: 实验室: 416 实验组别: 同组人员:实验时间:年 11月 24日评定成绩:审阅教师:
实验三闭环电压控制系统研究 一、实验目的: (1)经过实例展示,认识自动控制系统的组成、功能及自动控制原理课程所要解决的问题。 (2)会正确实现闭环负反馈。 (3)经过开、闭环实验数据说明闭环控制效果。 二、实验原理: (1)利用各种实际物理装置(如电子装置、机械装置、化工装置等)在数学上的“相似性”,将各种实际物理装置从感兴趣的角度经过简化、并抽象成相同的数学形式。我们在设计控制系统时,不必研究每一种实际装置,而用几种“等价”的数学形式来表示、研究和设计。又由于人本身的自然属性,人对数学而言,不能直接感受它的自然物理属性,这给我们分析和设计带来了困难。因此,我们又用替代、模拟、仿真的形式把数学形式再变成“模拟实物”来研究。这样,就能够“秀才不出门,遍知天下事”。实际上,在后面的课程里,不同专业的学生将面对不同的实际物理对象,而“模拟实物”的实验方式能够做到举一反三,我们就是用下列“模拟实物”——电路系统,替代各种实际物理对象。 (2)自动控制的根本是闭环,尽管有的系统不能直接感受到它的
闭环形式,如步进电机控制,专家系统等,从大局看,还是闭环。闭环控制能够带来想象不到的好处,本实验就是用开环和闭环在负载扰动下的实验数据,说明闭环控制效果。自动控制系统性能的优劣,其原因之一就是取决调节器的结构和算法的设计(本课程主要用串联调节、状态反馈),本实验为了简洁,采用单闭环、比例调节器K。经过实验证明:不同的K,对系性能产生不同的影响,以说明正确设计调节器算法的重要性。 (3)为了使实验有代表性,本实验采用三阶(高阶)系统。这样,当调节器K值过大时,控制系统会产生典型的现象——振荡。本实验也能够认为是一个真实的电压控制系统。 三、实验设备: THBDC-1实验平台 四、实验线路图: 五、实验步骤: (1)如图接线,建议使用运算放大器U8、U10、U9、U11、U13。
SAT2数学词汇
SAT Ⅱ数学词汇 assume that 假设approximation 近似,近似值absolute value 绝对值 acute 锐(角) alternate angles 内错角angle 角 area 面积 arithmetic sequence 等差数列assumption 假设 asymptote 渐近线 at random 随机地 average 平均的 axis 轴 circle 圆 circumference 周长clockwise 顺时针的common divisor 公约数common multiple 公倍数composite number 合数cone 圆锥 congruent 全等的coordinate 坐标 cube 立方体 cube root 立方根 cylinder 圆柱体 diagonal 对角线 digit 数字,位 divide 除 divisible 可整除的 domain 定义域 eccentricity 离心率 elipse 椭圆 equilateral 等边的 even 偶的 expotent 指数 function 函数 geometric sequence 等比数列hyperbola 双曲线 infinite 无穷的 integer 整数 intercept 截距
intersect 相交 irrational number 无理数isosceles 等腰的 major axis 长轴 mean 平均数 median 中数 minor axis 短轴 minus 减 mode 众数 multiply 乘 negative 负的 number line 数轴obtuse 钝(角) odd 奇的 origin of coordinates 原点parallel 平行的parallelogram 平行四边形perimeter 周长perpendicular 垂直的plus 加 polynomial 多项式positive 正的 prime number 质数probability 概率 product 积 proportion 比例quadruple 四倍的quotient 商 radian 弧度 radius 半径 range 值域 rational number 有理数rectangle 矩形 right 直(角) section 截面 sector 扇形 segment 线段 similar 相似的 slope 斜率 square 正方形 square root 平方根standard deviation 标准差
风力摆控制系统设计报告
2015 全国大学生电子设计竞赛 风力摆控制系统(B题) 【本科组】 2015年8月15日
摘要:本设计是基于STM32F103VE单片机为核心的简易风力摆控制系统,该系统由电源供电模块,直流风机及驱动模块、角度检测模块、信息处理模块、继电器及驱动模块、蜂鸣指示模块和液晶显示模块构成。STM32F103VE通过改变PWM占空比来实现对直流风机速度及方向的控制,该风力摆控制系统能够实现题目要求,简单做直线运动、复杂做圆周运动。 关键字:风力摆角度传感器单片机自动控制系统 一.方案论证: 1.系统结构 1)机械结构如图1所示。 一长约67cm的吸管上端用万向节固定在支架上,下方悬挂4只直流风机,中间安装陀螺仪,构成一风力摆。风力摆下安装一向下的激光笔,静止时,激光笔下端距离地面18cm。 图 1 2)测控电路结构 测控电路结构如图2所示。 编码器按键
图2 2.方案比较与选择 其实整体电路架构上图已经给定,主要是几个关键部分————直流风机选型及架构、直流风机驱动电路、传感器、主控芯片选择,我们分析如下: 1)直流风机的选型 方案一:采样大电流成品直流风机,虽然风力够大,但驱动多个风机所需电流过大,单个电源难以满足要求,而且比较重,多个电机使得惯性过大难以控制。鉴于以上两点,弃用。 方案二:采用小型高速电机加螺旋桨自制直流风机,风力大,体积小,质量轻,而且性价比高。 风力摆控制系统风机质量轻,减小惯性,容易起摆;风力大,风速控制范围大,摆动角度大;体积小,减少外部的干扰;鉴于以上几点,本设计采用方案二。 STM32微处理器 角度传感器 直流风机 电机驱动电路 风机供电 OLED 液晶显示 蜂鸣器
SAT2 化学考纲分析
SAT2化学主要考察对化学概念的理解能力。要求你必须会运用比率、正比例和反比例函数、科学计数法、一些简单的指数函数解决问题。考试时间为一小时,这就意味着你有平均42秒的时间来解答85道题目中的每一道题。这个考试更加注重于测试对基本化学概念的理解和领悟程度。众所周知,化学和数学是分不开的。然而如果掌握了这些化学概念,数学上的劣势就不会影响你在化学上的发挥。考试时,会提供一张元素周期表,但是它要比平常所使用的更简洁,只包括元素符号、原子序数、原子量。 考试会考什么内容?难度如何? 题目所占分值的大 概比例 问题的 数量 结构?原子理论和结构;周期性 ?核反应 ?化学键和分子结构 25 21 物质的状态?气体动力论和气体定律 ?液体、固体和状态变化 ?溶液,浓度单位,溶解度,传导率,溶液的依数性 15 13 反应类型?酸碱反应 ?氧化还原反应;化学电池 ?沉淀反应 14 12 化学计量学摩尔内容,阿伏伽德罗常数,经验公式,化学计算,百分组成12 10 化学平衡和化学反应速率化学平衡;质量(浓度)的表达,离子平衡,勒复特列原理;影响反应速率的因素 7 热力学化学反应中的能量转换;赫士定律 6 5 描述化学元素的物理化学性质以及常见的化合物,活泼性和化学反应产物, 有机和环境化学中的简单例子 13 11 实验部分设备,测量,过程,观察,安全事项,计算, 实验结果的解释7 6 考察的思维技能大致比例 基本概念,基本知识,基本术语(低级技能)20 理解基本概念并能运用到实际问题中,解决一些定性 和定量问题(中级技能) 45 运用所学知识分析已有信息,判断使用何种方法得出 结论,解决问题 35 第一个表格表述了考试的大致内容。考试通过85道多项选择题来考察你对知识的掌握情况和综合运用能力。每次考试都会涉及表中所列题目,但是每次的侧重点是不同的。由于各个学校的教学不是绝对统一的,考试中会有一些题目你是不熟悉的,甚至根本没有接触过。从整体上讲,SAT2化学难度不是很大。如果认真准备,就能取得很好的成绩。 SAT2化学考试需要具备哪些常识?
风力摆控制系统 设计报告
摘要:本系统主要是以STM32单片机为控制芯片控制4只直流轴流风机,从而调节风机转速来控制使风力摆呈现不同状态的控制系统。该系统主要由主控板,无线遥控器,直流轴流风机,摆架框架等四大部分组成风力摆控制系统。 关键字:风力摆无线 STM32单片机直流轴流风机
一.系统方案 1.系统方案论证 本系统主要由遥控模块、控制模块、陀螺仪模块、直流轴流风机组成,添加一些辅助电路作为扩展功能。系统工作有六种工作模式,使用无线遥控切换模式并显示。下面分别论证这几个模块的选择。 1.1直流风机的论证与选择 方案一:使用直流鼓风机。直流鼓风机的机械摩擦非常小,具有较大的精度,并能提供足够的风力进行运动。但在实验过程中,风机启动速度较慢,且由于其自身重量过大,风摆在运动过程中受惯性影响极大,不能有效的完成任务要求。 方案二:采用直流轴流风机。直流轴流风机是在固定位置使空气流动,自身重量和体积都比较小,且出风口大,能够很好的提供动力与控制。在实验过程中能够较快的启动,并能较好的实现任务要求,符合实验需要。 综合以上两种方案,风力摆在运动过程中需要进行实时控制摆杆的姿态,且需要风机启停反应快,故选择方案二。 1.2控制器模块的论证与选择 根据设计要求,控制器主要用于计算摆杆姿态、控制直流轴流风机PWM、使摆杆能完成相应等功能。 方案一:采用STC89C51作为系统控制器。它的技术成熟,成本低。STC89C51是8位的单片机,数据传输速度慢,在用于精密的操作时,不能满足实时控制的要求,且复杂的控制算法难以实现,不利于控制。 方案二:采用意法半导体公司的STM32F103单片机作为控制器。STM32系列单片机是32位、RISC、低功耗的处理器。在进行高精密的操作时,处理能力非常强,运算速度快,具有很好的控制能力,且成本低,更符合实验要求。 综合考虑以上两种方案,采用方案二。 2.系统结构 根据上述方案的论证,我们确定以STC32F103作为控制核心,采用型号为PFB0812XHE的直流轴风机控制摆杆运动,用陀螺仪MPU6050检测状态数据,并将采集到的数据传输给控制板,然后通过单片机计算处理得出摆杆的姿态并调整直
SAT2数学考试知识点介绍
SAT2数学考试知识点介绍 以下是小编为大家整理的SAT2数学考试知识点介绍,希望对大家sat考试备考有帮助,需要的同学赶快下载吧。 SAT2数学考试知识点中,Level 1和Level 2各个知识点的问题数目对比: Content Area (涉及范围)Level 1Level 2 Algebra (代数)1310 Plane Geometry(平面几何)90 Solid Geometry(立体几何)33 Coordinate Geometry(坐标几何)56 Trigonometry(三角学)37 Functions(函数)714 Statistics/ Probability(统计/概率)44 Miscellaneous(杂项)66 根据以上SAT2数学考试知识点的范围以及各范围所占的题目数量,我们可以发现:当我们突破了LEVEL2之后,LEVEL1将迎刃而解 SAT2数学考试中,LEVEL2 知识点总结: Algebra (代数)部分 根据考试方的官方分类,Algebra大概占MATH2考试所有问题的18%,代数是MATH2的基础,没有对它熟练的掌握很难拿到高分,当然这点对于中国学生来说,简直是小菜一碟。 对代数部分,Kaplan的介绍分为四个方面 Expressions and exponents (表达式和指数) Operations with polynomials and factoring (多项式和因数分解的应用) The Golden Rule of equations (等式的黄金规则) Quadratic equations (二次方程式) 练习时的时间控制在:12分钟10道题
SAT2数学知识点集锦
一、Arithmetic(大约5道题) 1、ComplexNumber复数 Operation复数运算 ComplexPlane复平面 Magnitudeof Complex Number复数的模 2、Ratio,Proportion and Percentage比例和百分数 复利和人口增长率问题 体积或面积变化率问题 3、NumberTheory数论 奇偶数(Odd and Even)、质数(Prime Number)、约数倍数(Factor and Multiple)、余数(Remainder) 4、Sequence数列 等差数列(Arithmetic Sequence)、等比数列(Geometric Sequence) 5、Determinantand Matrix行列式和矩阵 Determinant——行列式表示符号,2x2、3x3行列式的计算法则,行列式的数乘 Matrix——矩阵的表示符号,矩阵的加减、数乘法,矩阵的乘法 二、Algebra(大约30道题) 1、Function函数 函数的定义 函数的性质,包括定义域值域、奇偶性、单调性、周期性和图像 2、InverseFunction反函数
反函数定义 反函数性质,包括反函数存在条件、图像对称等 3、CompositeFunction复合函数 f(x)、g(x)、f[g(x)]三者相互转化 4、ElementaryFunction初等函数 (1)Power Function幂函数 LinearFunction一次函数,包括斜率、截距、两直线平行和垂直,一次不等式及线性规划 QuadraticFunction二次函数,包括一般式、顶点式、顶点坐标和对称轴,二次方程和不等式 High-DegreeFunction高次函数,包括多项式除法、复数根、高次函数图像特点 (2)Exponential Function指数函数 指数运算公式 指数函数性质,包括定义域值域、图像 (3)Logarithm Function对数函数 对数运算公式 对数函数性质,包括定义域值域、图像 (4)Trigonometry Function三角函数 a.角度制(Degree)和弧度制(Radian) sinx,cosx,tanx,cotx,secx,cscx等六个基本三角函数的相互转换; 诱导公式
路灯控制系统测试报告
路灯控制系统测试报告 1、镇流器 1)通信测试 项目要求结论 串口通信单灯控制器连接镇流器,通过USB无 正常 线模块查询数据,返回的镇流器数据应 与实际一致 2)调光测试 项目要求结论 调光控制单灯控制器连接镇流器,通过USB无线 正常 模块调光50%,通过功率计查看镇流器 输出功率值应该相应调整。 2、单灯控制器 1)通信测试 项目要求结论 正常无线通信通过USB无线模块设置网络ID,能返 回成功指令 串口通信连接镇流器,通过USB无线模块查询 正常 数据,返回的镇流器数据应与实际一致2)时钟测试 项目要求结论 正常时间设置、读取设置当前时间成功后,将单灯控制器断 电10分钟,重新上电后读出的时间应 与当前时间一致 3)存储测试 项目要求结论 正常方案设置、读取设置4个方案成功后,断电重启单灯控 制器,读取方案应与设置的方案一致3)温度测试 项目要求结论 正常灯壳温度采集读取数据,返回的灯壳温度应与单灯控 制器的板上温度一致
3、路由器 1)通信测试 项目要求结论 无线通信通过USB无线模块发送单灯控制器指 令,应能接收到路由器发出的数据,且 数据与下发的数据一致 正常 4、集中控制器 1)通信测试 项目要求结论 维护口通过计算机串口发送路灯控制器参数 设置指令,应有正确返回 正常 无线通信正确执行单灯控制器参数设置指令后, USB无线模块应能接收到集中器发出 的查询单灯控制器数据指令,按照单灯 控制器数据格式正确返回,再通过维护 口查询数据,数据应与下发的单灯控制 器数据一致 正常 GPRS 在带有GPRS模块的集中控制器上正 确设置上行通信口通信参数,等待查看 是否跟设置的服务器连接成功 正常 RJ45 在带有RJ45模块的集中控制器上正确 设置上行通信口通信参数,等待查看是 否跟设置的服务器连接成功 正常 2)时钟测试 项目要求结论 时间设置、读取设置当前时间成功后,将集中控制器断 电10分钟,重新上电后读出的时间应 与当前时间一致 正常 3)存储测试 项目要求结论 上行通信口通信参数设置、读取设置上行通信口通信参数成功后,断电 重启集中控制器,读取上行通信口通信 参数应与设置的一致 正常 4)交流采样测试 项目要求结论交流采样在带有交流采样功能的集中控制器上 加稳定的电压、电流。通过交流采样数 据查询功能查看,集中控制器采集的电 正常