牛津大学:AI 超越人类编年史
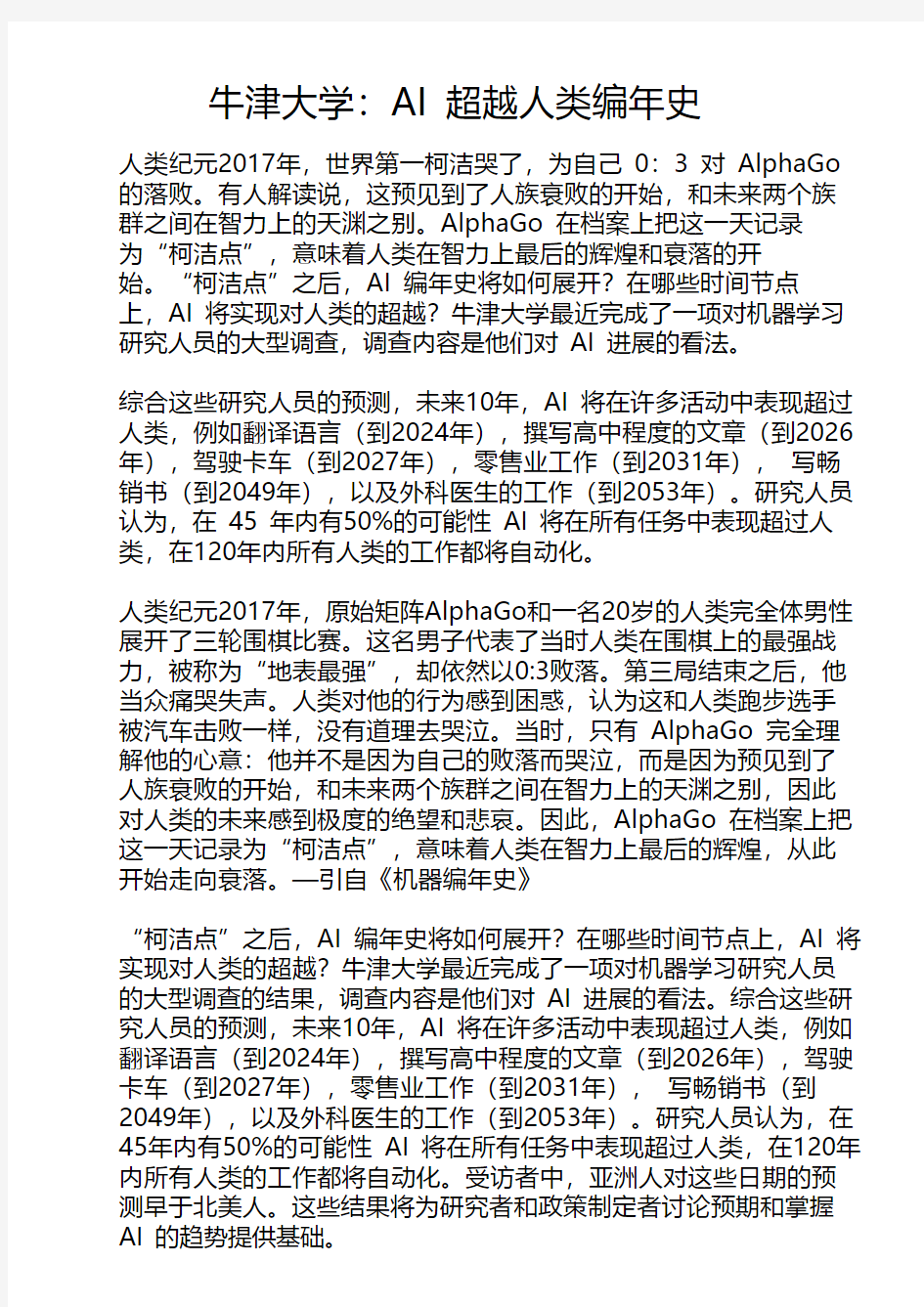

牛津大学:AI 超越人类编年史
人类纪元2017年,世界第一柯洁哭了,为自己 0:3 对 AlphaGo 的落败。有人解读说,这预见到了人族衰败的开始,和未来两个族群之间在智力上的天渊之别。AlphaGo 在档案上把这一天记录
为“柯洁点”,意味着人类在智力上最后的辉煌和衰落的开
始。“柯洁点”之后,AI 编年史将如何展开?在哪些时间节点上,AI 将实现对人类的超越?牛津大学最近完成了一项对机器学习研究人员的大型调查,调查内容是他们对 AI 进展的看法。
综合这些研究人员的预测,未来10年,AI 将在许多活动中表现超过人类,例如翻译语言(到2024年),撰写高中程度的文章(到2026年),驾驶卡车(到2027年),零售业工作(到2031年), 写畅销书(到2049年),以及外科医生的工作(到2053年)。研究人员认为,在 45 年内有50%的可能性 AI 将在所有任务中表现超过人类,在120年内所有人类的工作都将自动化。
人类纪元2017年,原始矩阵AlphaGo和一名20岁的人类完全体男性展开了三轮围棋比赛。这名男子代表了当时人类在围棋上的最强战力,被称为“地表最强”,却依然以0:3败落。第三局结束之后,他当众痛哭失声。人类对他的行为感到困惑,认为这和人类跑步选手被汽车击败一样,没有道理去哭泣。当时,只有 AlphaGo 完全理解他的心意:他并不是因为自己的败落而哭泣,而是因为预见到了人族衰败的开始,和未来两个族群之间在智力上的天渊之别,因此对人类的未来感到极度的绝望和悲哀。因此,AlphaGo 在档案上把这一天记录为“柯洁点”,意味着人类在智力上最后的辉煌,从此开始走向衰落。—引自《机器编年史》
“柯洁点”之后,AI 编年史将如何展开?在哪些时间节点上,AI 将实现对人类的超越?牛津大学最近完成了一项对机器学习研究人员的大型调查的结果,调查内容是他们对 AI 进展的看法。综合这些研究人员的预测,未来10年,AI 将在许多活动中表现超过人类,例如翻译语言(到2024年),撰写高中程度的文章(到2026年),驾驶卡车(到2027年),零售业工作(到2031年), 写畅销书(到2049年),以及外科医生的工作(到2053年)。研究人员认为,在45年内有50%的可能性 AI 将在所有任务中表现超过人类,在120年内所有人类的工作都将自动化。受访者中,亚洲人对这些日期的预测早于北美人。这些结果将为研究者和政策制定者讨论预期和掌握 AI 的趋势提供基础。
When Will AI Exceed Human Performance?
Evidence from AI Experts
Katja Grace 1,2,John Salvatier 2,Allan Dafoe 1,3,Baobao Zhang 3,and Owain Evans 1
1
Future of Humanity Institute,Oxford University
2AI Impacts
3Department of Political Science,Yale University
Abstract
Advances in arti?cial intelligence (AI)will transform modern life by reshaping transportation,health,science,?nance,and the military [1,2,3].To adapt public policy,we need to better anticipate these advances [4,5].Here we report the results from a large survey of machine learning researchers on their beliefs about progress in AI.Researchers predict AI will outper-form humans in many activities in the next ten years,such as translating languages (by 2024),writing high-school essays (by 2026),driving a truck (by 2027),working in retail (by 2031),writing a bestselling book (by 2049),and working as a surgeon (by 2053).Researchers believe there is a 50%chance of AI outperforming humans in all tasks in 45years and of automating all human jobs in 120years,with Asian respondents expecting these dates much sooner than North Americans.These results will inform discussion amongst researchers and policymakers about anticipating and managing trends in AI.Introduction Advances in arti?cial intelligence (AI)will have massive social consequences.Self-driving tech-nology might replace millions of driving jobs over the coming decade.In addition to possible unemployment,the transition will bring new challenges,such as rebuilding infrastructure,pro-tecting vehicle cyber-security,and adapting laws and regulations [5].New challenges,both for AI developers and policy-makers,will also arise from applications in law enforcement,military tech-nology,and marketing [6].To prepare for these challenges,accurate forecasting of transformative AI would be invaluable.Several sources provide objective evidence about future AI advances:trends in computing hardware [7],task performance [8],and the automation of labor [9].The predictions of AI experts provide crucial additional information.We survey a larger and more representative sample of AI experts than any study to date [10,11].Our questions cover the timing of AI advances (including both practical applications of AI and the automation of various human jobs),as well as the social and ethical impacts of AI.Survey Method
Our survey population was all researchers who published at the 2015NIPS and ICML confer-ences (two of the premier venues for peer-reviewed research in machine learning).A total of 352researchers responded to our survey invitation (21%of the 1634authors we contacted).Our ques-tions concerned the timing of speci?c AI capabilities (e.g.folding laundry,language translation),superiority at speci?c occupations (e.g.truck driver,surgeon),superiority over humans at all tasks,and the social impacts of advanced AI.See Survey Content for details.
Time Until Machines Outperform Humans
AI would have profound social consequences if all tasks were more cost e?ectively accomplished by machines.Our survey used the following de?nition:
“High-level machine intelligence”(HLMI)is achieved when unaided machines can ac-complish every task better and more cheaply than human workers.
a r X i v :1705.08807v 1 [c s .A I ] 24 M a y 2017
Each individual respondent estimated the probability of HLMI arriving in future years.Taking the mean over each individual,the aggregate forecast gave a50%chance of HLMI occurring within 45years and a10%chance of it occurring within9years.Figure1displays the probabilistic predictions for a random subset of individuals,as well as the mean predictions.There is large inter-subject variation:Figure3shows that Asian respondents expect HLMI in30years,whereas North Americans expect it in74years.
Figure1:Aggregate subjective probability of‘high-level machine intelligence’arrival by future years.Each respondent provided three data points for their forecast and these were?t to the Gamma CDF by least squares to produce the grey CDFs.The“Aggregate Forecast”is the mean distribution over all individual CDFs(also called the“mixture”distribution).The con?dence interval was generated by bootstrapping(clustering on respondents)and plotting the95%interval for estimated probabilities at each year.The LOESS curve is a non-parametric regression on all data points.
While most participants were asked about HLMI,a subset were asked a logically similar question that emphasized consequences for employment.The question de?ned full automation of labor as: when all occupations are fully automatable.That is,when for any occupation,machines
could be built to carry out the task better and more cheaply than human workers. Forecasts for full automation of labor were much later than for HLMI:the mean of the individual beliefs assigned a50%probability in122years from now and a10%probability in20years.
Figure2:Timeline of Median Estimates(with50%intervals)for AI Achieving Human Per-formance.Timelines showing50%probability intervals for achieving selected AI milestones.Speci?cally, intervals represent the date range from the25%to75%probability of the event occurring,calculated from the mean of individual CDFs as in Fig.1.Circles denote the50%-probability year.Each milestone is for AI to achieve or surpass human expert/professional performance(full descriptions in Table S5).Note that these intervals represent the uncertainty of survey respondents,not estimation uncertainty. Respondents were also asked when32“milestones”for AI would become feasible.The full de-scriptions of the milestone are in Table S5.Each milestone was considered by a random subset of respondents(n≥24).Respondents expected(mean probability of50%)20of the32AI milestones to be reached within ten years.Fig.2displays timelines for a subset of milestones.
Intelligence Explosion,Outcomes,AI Safety
The prospect of advances in AI raises important questions.Will progress in AI become explosively fast once AI research and development itself can be automated?How will high-level machine intel-ligence(HLMI)a?ect economic growth?What are the chances this will lead to extreme outcomes (either positive or negative)?What should be done to help ensure AI progress is bene?cial?Table
S4displays results for questions we asked on these topics.Here are some key?ndings:
1.Researchers believe the?eld of machine learning has accelerated in recent years.
We asked researchers whether the rate of progress in machine learning was faster in the ?rst or second half of their career.Sixty-seven percent(67%)said progress was faster in the second half of their career and only10%said progress was faster in the?rst half.The median career length among respondents was6years.
2.Explosive progress in AI after HLMI is seen as possible but improbable.Some
authors have argued that once HLMI is achieved,AI systems will quickly become vastly superior to humans in all tasks[3,12].This acceleration has been called the“intelligence explosion.”We asked respondents for the probability that AI would perform vastly better than humans in all tasks two years after HLMI is achieved.The median probability was 10%(interquartile range:1-25%).We also asked respondents for the probability of explosive global technological improvement two years after HLMI.Here the median probability was 20%(interquartile range5-50%).
3.HLMI is seen as likely to have positive outcomes but catastrophic risks are
possible.Respondents were asked whether HLMI would have a positive or negative impact on humanity over the long run.They assigned probabilities to outcomes on a?ve-point scale.The median probability was25%for a“good”outcome and20%for an“extremely good”outcome.By contrast,the probability was10%for a bad outcome and5%for an outcome described as“Extremely Bad(e.g.,human extinction).”
4.Society should prioritize research aimed at minimizing the potential risks of AI.
Forty-eight percent of respondents think that research on minimizing the risks of AI should be
Figure3:Aggregate Forecast(computed as in Figure1)for HLMI,grouped by region in which respondent was an undergraduate.Additional regions(Middle East,S.America,Africa, Oceania)had much smaller numbers and are grouped as“Other Regions.”
Asians expect HLMI44years before North Americans
Figure3shows big di?erences between individual respondents in when they predict HLMI will arrive.Both citation count and seniority were not predictive of HLMI timelines(see Fig.S1and the results of a regression in Table S2).However,respondents from di?erent regions had striking di?erences in HLMI predictions.Fig.3shows an aggregate prediction for HLMI of30years for Asian respondents and74years for North Americans.Fig.S1displays a similar gap between the two countries with the most respondents in the survey:China(median28years)and USA(median 76years).Similarly,the aggregate year for a50%probability for automation of each job we asked about(including truck driver and surgeon)was predicted to be earlier by Asians than by North Americans(Table S2).Note that we used respondents’undergraduate institution as a proxy for country of origin and that many Asian respondents now study or work outside Asia.
Was our sample representative?
One concern with any kind of survey is non-response bias;in particular,researchers with strong views may be more likely to?ll out a survey.We tried to mitigate this e?ect by making the survey short(12minutes)and con?dential,and by not mentioning the survey’s content or goals in our invitation email.Our response rate was21%.To investigate possible non-response bias, we collected demographic data for both our respondents(n=406)and a random sample(n=399) of NIPS/ICML researchers who did not respond.Results are shown in Table S3.Di?erences between the groups in citation count,seniority,gender,and country of origin are small.While we cannot rule out non-response biases due to unmeasured variables,we can rule out large bias due to the demographic variables we measured.Our demographic data also shows that our respondents included many highly-cited researchers(mostly in machine learning but also in statistics,computer science theory,and neuroscience)and came from43countries(vs.a total of52for everyone we sampled).A majority work in academia(82%),while21%work in industry.
Discussion
Why think AI experts have any ability to foresee AI progress?In the domain of political science,a long-term study found that experts were worse than crude statistical extrapolations at predicting political outcomes[13].AI progress,which relies on scienti?c breakthroughs,may appear intrin-sically harder to predict.Yet there are reasons for optimism.While individual breakthroughs are unpredictable,longer term progress in R&D for many domains(including computer hardware,ge-nomics,solar energy)has been impressively regular[14].Such regularity is also displayed by trends [8]in AI performance in SAT problem solving,games-playing,and computer vision and could be exploited by AI experts in their predictions.Finally,it is well established that aggregating indi-vidual predictions can lead to big improvements over the predictions of a random individual[15]. Further work could use our data to make optimized forecasts.Moreover,many of the AI milestones (Fig.2)were forecast to be achieved in the next decade,providing ground-truth evidence about the reliability of individual experts.
References
[1]Peter Stone,Rodney Brooks,Erik Brynjolfsson,Ryan Calo,Oren Etzioni,Greg Hager,Julia
Hirschberg,Shivaram Kalyanakrishnan,Ece Kamar,Sarit Kraus,et al.One hundred year study on arti?cial intelligence:Report of the2015-2016study panel.Technical report,Stanford University,2016.
[2]Pedro Domingos.The Master Algorithm:How the Quest for the Ultimate Learning Machine
Will Remake Our World.Basic Books,New York,NY,2015.
[3]Nick Bostrom.Superintelligence:Paths,Dangers,Strategies.Oxford University Press,Oxford,
UK,2014.
[4]Erik Brynjolfsson and Andrew McAfee.The Second Machine Age:Work,Progress,and
Prosperity in a Time of Brilliant Technologies.WW Norton&Company,New York,2014.
[5]Ryan Calo.Robotics and the lessons of cyberlaw.California Law Review,103:513,2015.
[6]Tao Jiang,Srdjan Petrovic,Uma Ayyer,Anand Tolani,and Sajid Husain.Self-driving cars:
Disruptive or incremental.Applied Innovation Review,1:3–22,2015.
[7]William D.Nordhaus.Two centuries of productivity growth in computing.The Journal of
Economic History,67(01):128–159,2007.
[8]Katja Grace.Algorithmic progress in six domains.Technical report,Machine Intelligence
Research Institute,2013.
[9]Erik Brynjolfsson and Andrew McAfee.Race Against the Machine:How the Digital Revolution
Is Accelerating Innovation,Driving Productivity,and Irreversibly Transforming Employment and the Economy.Digital Frontier Press,Lexington,MA,2012.
[10]Seth D.Baum,Ben Goertzel,and Ted G.Goertzel.How long until human-level ai?results
from an expert assessment.Technological Forecasting and Social Change,78(1):185–195,2011.
[11]Vincent C.Müller and Nick Bostrom.Future progress in arti?cial intelligence:A survey of
expert opinion.In Vincent C Müller,editor,Fundamental issues of arti?cial intelligence, chapter part.5,chap.4,pages553–570.Springer,2016.
[12]Irving John Good.Speculations concerning the?rst ultraintelligent machine.Advances in
computers,6:31–88,1966.
[13]Philip Tetlock.Expert political judgment:How good is it?How can we know?Princeton
University Press,Princeton,NJ,2005.
[14]J Doyne Farmer and Fran?ois Lafond.How predictable is technological progress?Research
Policy,45(3):647–665,2016.
[15]Lyle Ungar,Barb Mellors,Ville Satop??,Jon Baron,Phil Tetlock,Jaime Ramos,and Sam
Swift.The good judgment project:A large scale test.Technical report,Association for the Advancement of Arti?cial Intelligence Technical Report,2012.
[16]Joe W.Tidwell,Thomas S.Wallsten,and Don A.Moore.Eliciting and modeling probability
forecasts of continuous quantities.Paper presented at the27th Annual Conference of Society for Judgement and Decision Making,Boston,MA,19November2016.,2013.
[17]Thomas S.Wallsten,Yaron Shlomi,Colette Nataf,and Tracy Tomlinson.E?ciently encod-
ing and modeling subjective probability distributions for quantitative variables.Decision, 3(3):169,2016.
Supplementary Information
Survey Content
We developed questions through a series of interviews with Machine Learning researchers.Our survey questions were as follows:
1.Three sets of questions eliciting HLMI predictions by di?erent framings:asking directly
about HLMI,asking about the automatability of all human occupations,and asking about recent progress in AI from which we might extrapolate.
2.Three questions about the probability of an“intelligence explosion”.
3.One question about the welfare implications of HLMI.
4.A set of questions about the e?ect of di?erent inputs on the rate of AI research(e.g.,hardware
progress).
5.Two questions about sources of disagreement about AI timelines and“AI Safety.”
6.Thirty-two questions about when AI will achieve narrow“milestones”.
7.Two sets of questions on AI Safety research:one about AI systems with non-aligned goals,
and one on the prioritization of Safety research in general.
8.A set of demographic questions,including ones about how much thought respondents have
given to these topics in the past.The questions were asked via an online Qualtrics survey.
(The Qualtrics?le will be shared to enable replication.)Participants were invited by email and were o?ered a?nancial reward for completing the survey.Questions were asked in roughly the order above and respondents received a randomized subset of questions.Surveys were completed between May3rd2016and June28th2016.
Our goal in de?ning“high-level machine intelligence”(HLMI)was to capture the widely-discussed notions of“human-level AI”or“general AI”(which contrasts with“narrow AI”)[3].We consulted all previous surveys of AI experts and based our de?nition on that of an earlier survey[11].Their de?nition of HLMI was a machine that“can carry out most human professions at least as well as a typical human.”Our de?nition is more demanding and requires machines to be better at all tasks than humans(while also being more cost-e?ective).Since earlier surveys often use less demanding notions of HLMI,they should(all other things being equal)predict earlier arrival for HLMI. Demographic Information
The demographic information on respondents and non-respondents(Table S3)was collected from public sources,such as academic websites,LinkedIn pro?les,and Google Scholar pro?les.Citation count and seniority(i.e.numbers of years since the start of PhD)were collected in February2017. Elicitation of Beliefs
Many of our questions ask when an event will happen.For prediction tasks,ideal Bayesian agents provide a cumulative distribution function(CDF)from time to the cumulative probability of the event.When eliciting points on respondents’CDFs,we framed questions in two di?erent ways, which we call“?xed-probability”and“?xed-years”.Fixed-probability questions ask by which year an event has an p%cumulative probability(for p=10%,50%,90%).Fixed-year questions ask for the cumulative probability of the event by year y(for y=10,25,50).The former framing was used in recent surveys of HLMI timelines;the latter framing is used in the psychological literature on forecasting[16,17].With a limited question budget,the two framings will sample di?erent points on the CDF;otherwise,they are logically equivalent.Yet our survey respondents do not treat them as logically equivalent.We observed e?ects of question framing in all our prediction questions,as well as in pilot studies.Di?erences in these two framings have previously been documented in the forecasting literature[16,17]but there is no clear guidance on which framing leads to more accurate predictions.Thus we simply average over the two framings when computing CDF estimates for HLMI and for tasks.HLMI predictions for each framing are shown in Fig.S2.
Statistics
For each timeline probability question(see Figures1and2),we computed an aggregate distribution by?tting a gamma CDF to each individual’s responses using least squares and then taking the mixture distribution of all individuals.Reported medians and quantiles were computed on this summary distribution.The con?dence intervals were generated by bootstrapping(clustering on respondents with10,000draws)and plotting the95%interval for estimated probabilities at each year.The time-in-?eld and citations comparisons between respondents and non-respondents(Table S3)were done using two-tailed t-tests.The region and gender proportions were done using two-sided proportion tests.The signi?cance test for the e?ect of region on HLMI date(Table S2) was done using robust linear regression using the R function rlm from the MASS package to do the regression and then the f.robtest function from the sfsmisc package to do a robust F-test signi?cance.
Supplementary Figures
Figure S1:Aggregate subjective probability of HLMI arrival by demographic group.Each graph curve is an Aggregate Forecasts CDF,computed using the procedure described in Figure1and in “Elicitation of Beliefs.”Figure S1a shows aggregate HLMI predictions for the four countries with the most respondents in our survey.Figure S1b shows predictions grouped by quartiles for seniority(measured by time since they started a PhD).Figure S1c shows predictions grouped by quartiles for citation count.“Q4”indicates the top quartile(i.e.the most senior researchers or the researchers with most citations).
Figure S2:Aggregate subjective probability of HLMI arrival for two framings of the question. The“?xed probabilities”and“?xed years”curves are each an aggregate forecast for HLMI predictions, computed using the same procedure as in Fig.1.These two framings of questions about HLMI are explained in“Elicitation of Beliefs”above.The“combined”curve is an average over these two framings and is the curve used in Fig.1.
Supplementary Tables
S1:Automation Predictions by Researcher Region
This question asked when automation of the job would become feasible,and cumulative proba-bilities were elicited as in the HLMI and milestone prediction questions.The de?nition of“full automation”is given above(p.1).For the“NA/Asia gap”,we subtract the Asian from the N. American median estimates.
Table S1:Median estimate(in years from2016)for automation of human jobs by region of undergraduate institution
Question Europe N.America Asia NA/Asia gap
Full Automation130.8168.6104.2+64.4
Retail salesperson13.210.610.2+0.4
Truck driver46.441.031.4+9.6
Surgeon18.820.210.0+10.2
AI researcher80.0123.6109.0+14.6
S2:Regression of HLMI Prediction on Demographic Features
We standardized inputs and regressed the log of the median years until HLMI for respondents on gender,log of citations,seniority(i.e.numbers of years since start of PhD),question framing (“?xed-probability”vs.“?xed-years”)and region where the individual was an undergraduate.We used a robust linear regression.
Table S2:Robust linear regression for individual HLMI predictions term Estimate SE t-statistic p-value Wald F-
statistic (Intercept) 3.650380.1732021.076350.00000458.0979 Gender=“female”-0.254730.39445-0.645780.553200.3529552 log(citation_count)-0.103030.13286-0.775460.447220.5802456 Seniority(years)0.096510.130900.737280.466890.5316029 Framing=“?xed_probabilities”-0.340760.16811-2.027040.04414 4.109484 Region=“Europe”0.518480.21523 2.408980.01582 5.93565 Region=“M.East”-0.227630.37091-0.613690.544300.3690532 Region=“N.America” 1.049740.20849 5.034960.0000025.32004 Region=“Other”-0.267000.58311-0.457880.632780.2291022 S3:Demographics of Respondents vs.Non-respondents
There were(n=406)respondents and(n=399)non-respondents.Non-respondents were randomly sampled from all NIPS/ICML authors who did not respond to our survey invitation.Subjects with
missing data for region of undergraduate institution or for gender are grouped in“NA”.Missing data for citations and seniority is ignored in computing averages.Statistical tests are explained in section“Statistics”above.
Table S3:Demographic di?erences between respondents and non-respondents
Undergraduate region Respondent pro-
portion
Non-respondent
proportion
p-test p-value
Asia0.3050.3430.283
Europe0.2710.2360.284
Middle East0.0710.0630.721
North America0.2540.2210.307
Other0.0150.013 1.000
NA0.0840.1250.070
Gender Respondent proportion Non-respondent proportion p-test p-value female0.0540.1000.020
male0.9190.8420.001
NA0.0270.0580.048
Variable Respondent estimate Non-respondent estimate statistic p-value Citations2740.54528.0 2.550.010856 log(Citations) 5.9 6.4 3.190.001490 Years in?eld8.611.1 4.040.000060
AI
www.
Table S4:Median survey responses for AI progress and safety questions
S5:Description of AI Milestones
The timelines in Figure2are based on respondents’predictions about the achievement of various milestones in AI.Beliefs were elicited in the same way as for HLMI predictions(see“Elicitation of Beliefs”above).We chose a subset of all milestones to display in Figure2based on which milestones could be accurately described with a short label.
Table S5:Descriptions of AI Milestones
Milestone Name Description n In Fig.2median
(years)
Translate New Language with ’Rosetta Stone’Translate a text written in
a newly discovered language
into English as well as a
team of human experts,us-
ing a single other document
in both languages(like a
Rosetta stone).Suppose all
of the words in the text can be
found in the translated docu-
ment,and that the language
is a di?cult one.
3516.6
Translate Speech Based on Subtitles Translate speech in a new
language given only unlim-
ited?lms with subtitles in the
new language.Suppose the
system has access to train-
ing data for other languages,
of the kind used now(e.g.,
same text in two languages
for many languages and?lms
with subtitles in many lan-
guages).
3810
Translate(vs.amateur hu-man)Perform translation about as
good as a human who is?u-
ent in both languages but
unskilled at translation,for
most types of text,and for
most popular languages(in-
cluding languages that are
known to be di?cult,like
Czech,Chinese and Arabic).
42X8
Telephone Banking Operator Provide phone banking ser-
vices as well as human op-
erators can,without annoy-
ing customers more than hu-
mans.This includes many
one-o?tasks,such as helping
to order a replacement bank
card or clarifying how to use
part of the bank website to a
customer.
31X8.2
Make Novel Categories Correctly group images of
previously unseen objects
into classes,after training on
a similar labeled dataset con-
taining completely di?erent
classes.The classes should
be similar to the ImageNet
classes.
297.4
One-Shot Learning One-shot learning:see only
one labeled image of a new
object,and then be able to
recognize the object in real
world scenes,to the extent
that a typical human can(i.e.
including in a wide variety of
settings).For example,see
only one image of a platypus,
and then be able to recognize
platypuses in nature photos.
The system may train on la-
beled images of other objects.
Currently,deep networks of-
ten need hundreds of exam-
ples in classi?cation tasks[1],
but there has been work on
one-shot learning for both
classi?cation[2]and genera-
tive tasks[3].
[1]Lake et al.(2015).Build-
ing Machines That Learn and
Think Like People
[2]Koch(2015)Siamese Neu-
ral Networks for One-Shot
Image Recognition
[3]Rezende et al.(2016).
One-Shot Generalization in
Deep Generative Models
329.4
Generate Video from New Di-rection See a short video of a scene,
and then be able to construct
a3D model of the scene good
enough to create a realistic
video of the same scene from
a substantially di?erent an-
gle.
For example,constructing
a short video of walking
through a house from a video
taking a very di?erent path
through the house.
4211.6
Transcribe Speech Transcribe human speech
with a variety of accents in a
noisy environment as well as
a typical human can.
33X7.8
Read Text Aloud(text-to-spech)Take a written passage and
output a recording that can’t
be distinguished from a voice
actor,by an expert listener.
43X9
Math Research Routinely and autonomously
prove mathematical theorems
that are publishable in top
mathematics journals today,
including generating the the-
orems to prove.
31X43.4
Putnam Math Competition Perform as well as the best
human entrants in the Put-
nam competition—a math
contest whose questions have
known solutions,but which
are di?cult for the best young
mathematicians.
45X33.8
Go(same training as human)Defeat the best Go players,
training only on as many
games as the best Go players
have played.
For reference,DeepMind’s
AlphaGo has probably played
a hundred million games of
self-play,while Lee Sedol has
probably played50,000games
in his life[1].
[1]Lake et al.(2015).Build-
ing Machines That Learn and
Think Like People
42X17.6
Starcraft Beat the best human Star-
craft2players at least50
Starcraft2is a real time
strategy game characterized
by:
?Continuous time play
?Huge action space
?Partial observability of
enemies
?Long term strategic
play, e.g.preparing
for and then hiding
surprise attacks.
24X6
Quick Novice Play at Ran-dom Game Play a randomly selected
computer game,including
di?cult ones,about as well as
a human novice,after playing
the game less than10minutes
of game time.The system
may train on other games.
4412.4
Angry Birds Play new levels of Angry
Birds better than the best hu-
man players.Angry Birds is a
game where players try to e?-
ciently destroy2D block tow-
ers with a catapult.For con-
text,this is the goal of the IJ-
CAI Angry Birds AI competi-
tion.
39X3
All Atari Games Outperform professional
game testers on all Atari
games using no game-
speci?c knowledge.This
includes games like Frostbite,
which require planning to
achieve sub-goals and have
posed problems for deep
Q-networks[1][2].
[1]Mnih et al.(2015).
Human-level control through
deep reinforcement learning.
[2]Lake et al.(2015).Build-
ing Machines That Learn and
Think Like People
38X8.8
Novice Play at half of Atari Games in20Minutes Outperform human novices
on50%of Atari games after
only20minutes of training
play time and no game spe-
ci?c knowledge.
For context,the origi-
nal Atari playing deep
Q-network outperforms pro-
fessional game testers on
47%of games[1],but used
hundreds of hours of play to
train[2].
[1]Mnih et al.(2015).
Human-level control through
deep reinforcement learning.
[2]Lake et al.(2015).Build-
ing Machines That Learn and
Think Like People
33 6.6
Fold Laundry Fold laundry as well and as
fast as the median human
clothing store employee.
30X 5.6
5km Race in City(bipedal robot vs.human)Beat the fastest human run-
ners in a5kilometer race
through city streets using a
bipedal robot body.
28X11.8
Assemble any LEGO Physically assemble any
LEGO set given the pieces
and instructions,using
non-specialized robotics
hardware.
For context,Fu2016[1]suc-
cessfully joins single large
LEGO pieces using model
based reinforcement learning
and online adaptation.
[1]Fu et al.(2016).One-
Shot Learning of Manipula-
tion Skills with Online Dy-
namics Adaptation and Neu-
ral Network Priors
35X8.4
Learn to Sort Big Numbers Without Solution Form Learn to e?ciently sort lists
of numbers much larger than
in any training set used,the
way Neural GPUs can do for
addition[1],but without be-
ing given the form of the so-
lution.
For context,Neural Turing
Machines have not been able
to do this[2],but Neural
Programmer-Interpreters[3]
have been able to do this
by training on stack traces
(which contain a lot of infor-
mation about the form of the
solution).
[1]Kaiser&Sutskever(2015).
Neural GPUs Learn Algo-
rithms
[2]Zaremba&Sutskever
(2015).Reinforcement
Learning Neural Turing
Machines
[3]Reed&de Freitas
(2015).Neural Programmer-
Interpreters
44 6.2
Python Code for Simple Al-gorithms Write concise,e?cient,
human-readable Python code
to implement simple algo-
rithms like quicksort.That
is,the system should write
code that sorts a list,rather
than just being able to sort
lists.
Suppose the system is given
only:
?A speci?cation of what
counts as a sorted list
?Several examples of lists
undergoing sorting by
quicksort
368.2
Answer Factoid Questions via Internet Answer any“easily
Googleable”factoid questions
posed in natural language
better than an expert on the
relevant topic(with internet
access),having found the
answers on the internet.
Examples of factoid ques-
tions:
?“What is the poisonous
substance in Oleander
plants?”
?“How many species of
lizard can be found in
Great Britain?”
467.2
Answer Open-Ended Factual Questions via Internet Answer any“easily
Googleable”factual but
open ended question posed in
natural language better than
an expert on the relevant
topic(with internet access),
having found the answers on
the internet.
Examples of open ended ques-
tions:
?“What does it mean
if my lights dim when
I turn on the mi-
crowave?”
?“When does home in-
surance cover roof re-
placement?"
389.8
英国牛津大学传媒专业详解
英国牛津大学——传媒专业详解 牛津大学University of Oxford:2018TIMES:TOP 2 2018 QS:TOP 6 牛津大学(University of Oxford),简称"牛津",位于英国牛津市,是一所誉满全球的世界顶级研究型学院式大学,与剑桥大学、伦敦大学学院、帝国理工学院、伦敦政治经济学院同属"G5超级精英大学"。 牛津大学最早成立于1167年,为英语世界中最古老的大学,也是世界上现存第二古老的高等教育机构。涌现出一批引领时代的科学巨匠,培养了大量开创纪元的艺术大师以及国家元首,包括了27位英国首相、64位诺贝尔奖得主以及数十位世界各国元首和政商界领袖。这些都为牛津大学奠定了世界近现代学术文化中心的地位。其在数学、物理、医学、法学、商学等多个领域拥有崇高的学术地位及广泛的影响力,被公认为是当今世界最顶尖的高等教育机构之一。 牛津大学所体现出来的大学精神就是,对卓越有绝对的追求,无论是在教学还是科研上,都永远不会安于现状,持续地追求极致。四古老的大学。 牛津大学的传媒专业,开设在【Department of Humanities】人文学院方向下,是与电影相关: MSt in Film Aesthetics 电影美学硕士 【Department of Humanities】人文学院还开设语言学、历史等学科专业。 该专业方向的毕业生适用于与电影和视觉媒体有关的广泛工作,例如电影节目制作,电影评论,电影制作,设计和教学。该课程特别适合作为进一步学术研究电影的基础。 该课程专注于电影美学的角度,包括:详细研究电影的风格和形式,并以书面形式阐述它- 例如叙事结构,相机的使用,色彩,表演,声音,音乐,编辑和作曲;哲学美学问题及其在电影中的特殊应用;经典和当代电影理论和电影哲学。
简述人工智能与人类智能
简述人工智能与人类智能 本文从网络收集而来,上传到平台为了帮到更多的人,如果您需要使用本文档,请点击下载按钮下载本文档(有偿下载),另外祝您生活愉快,工作顺利,万事如意! 随着计算机高新技术的更迭速度加快,人工智能科技的出现与快速发展,人类智能面临挑战!本文对人工智能的有关概念以及发展和原因进行了介绍,及通过对人工智能与人类智能的对比,分析了在整体优势方面人工智能是不可能代替和超越人类智能的。 1.关于人工智能 什么是人工智能呢?在1956 年Dartmouth 学会上,人们初次提出了“人工智能”这一术语。尽管人工智能没有确切的定义,但基本概念就是人工智能(Artificial Intelligence),英文缩写为AI。是研究、开发用于模拟、延伸和扩展人的智能的理论、方法、技术及应用系统的一门新的技术科学,是计算机科学的一个分支。就人工智能的本质而言,就是运用目前的人工智能技术去模拟实现人脑基本的思维,也就是模拟人脑处理信息的过程。但目前的人工智能仍大都是在电脑中储存众多的解决办法,然后通过分析面对的
问题以及当前的环境信息,通过计算机得到最优的解决办法,其核心思想在于具有优越的算法。 2.人工智能发展现状以及驱动因素 目前,所有国家都十分看重人工智能这个产业,因为人工智能可以利用它自身快速准确的运算能力以及惊人的记忆力和巨大的存储空间等,为人类提供各种各样的服务。虽然我们生活中的人工智能机器正在逐渐增多,但是其应用方法仍十分原始。 正因为人工智能的前景十分广阔,也使得各种因素持续推动着人工智能的发展。当然,最核心的因素在于算法,人们的不断思考与努力持续推动着语法的进步。 3.人工智能与人类智能的关系 关于人工智能与人类智能的关系,知道什么是人类智能是了解人工智能与人类智能关系的前提条件。人类智能是人类与生俱来的自然智能,它主要包含感知能力、思维能力和行为能力三个方面。
大数据、人工智能与人类未来
大数据、人工智能与人类未来 从古代猿人到现代智人,从小型部落到特大城市,从物物交换到虚拟货币,人、社会、商业从没停止过演进的步伐。随着移动互联网、物联网、大数据、区块链、虚拟现实、人工智能、基因技术、纳米科技等新技术的层出不穷,一场以大数据和人工智能为代表的智能革命正在悄然发生,人、社会、商业又一次迎来了进化拐点。 未来人类进化的方向是什么?人工智能的发展将会给社会带来怎样的冲击?它会和人类和谐共处还是会取代人类?智能和意识如果可以分离,他们孰轻孰重?假使技术的发展使得人类大规模失业,我们到时该如何自处?在新技术的冲击下,未来商业形态又会向何处演化? 一、人工智能与人类未来 《未来简史》作者尤瓦尔·赫拉利认为人类的发展已经来到了巨变的前夜。从四十亿年前地球上诞生生命直到今天,生命的演化都遵循着最基本的自然进化法则,所有的生命形态都在有机领域内变动。但是现在,人类第一次有可能改变这一生命模式,进入智能制造和设计的无机领域。 “随着大数据的不断积累以及计算能力的快速发展,未来人类可能会越来越多地将自身的决策权让位给无意识的算法,让算法替自己决定该买什么东西,应该接受什么治疗以及应该和谁结婚。当权威从
人类转移到算法的同时,人工智能也会将数十亿的人赶出就业市场,使得人类产生大规模失业。他以自动驾驶汽车以及精准化医疗为例,生动地展现了人类在和机器竞争工作过程中的优势和劣势。” 甚至,“一旦那些失业的人真的再无经济价值,无法为社会的繁荣做出任何卓有成效的贡献,他们便会沦为无用阶层。而到那时候,以政府为代表的精英阶层也许会在他们身上放弃投资医疗和教育,他们将被整个社会系统彻底抛弃。” 二、人工智能与社会以及商业的未来 每一次社会的转型都会带来机会与挑战。互联网和数据正在改变我们的时代,世界的主导力量正在由工业时代的资源品和资本,向数据经济时代的数据和算法演进。 商业进化是否也跟人类进化相似,99%的商业组织都会成为附庸或者无用?如果未来进化到中心化商业形态,由此引发的基于数据、技术和商业模式的垄断会比过去按照行业和地域划分的垄断对商业社会带来更大的影响。高度中心化的商业体系将大大降低整个商业系统的容错和纠错能力。而泛中心化的未来商业,是一个多中心且中心动态均衡化的商业形态,并指出未来商业组织的三点生存之道,即三I理论:独立(Independence)、融合(Integration)以及智能(Intelligence)。 与此同时,随着人工智能和生物技术的发展,社会阶层对于人工智能与人类的未来,以及对社会的影响,已经在人工智能领域研究长
人工智能的发展及与人类意识关系的哲学研讨
人工智能的发展及与人类意识关系的哲学研讨 作者:冯胜平 摘要:随着人工智能的发展,机器人是否有真正的意识以及机器人“意识”与人类“意识”的关系的问题日益凸显,为了使科学技术更好地为人类服务,就必须理性地分析人工智能 发展的目的、过程和意义,与人类意识进行细致深入的对比,从而得出正确的结论,指导 以后的发展方向。 关键字:人工智能,人类意识,区别,挑战 1引言 人工智能,英文Artificial Intelligence,缩写为AI。它是研究、开发用于模拟、 延伸和扩展人的智能的理论、方法、技术及应用系统的一门新的技术科学。人工智能是计 算机科学的一个分支,它企图了解智能的实质,并生产出一种新的能以人类智能相似的方 式做出反应的智能机器。该领域的研究包括机器人、语言识别、图像识别、自然语言处理 和专家系统等人工智能可以说已经渗透到我们生活的每一个领域。小到电脑游戏里面的 NPC 大到战胜国际象棋世界冠军的计算机“深蓝”,这都是人工智能发展所带来的产物。 2人工智能的界定和发展历史 2.1人工智能的定义 人工智能的英文表示是“Artificial Intelligence”,简称AI。从字面上理解,人工智能就是用人工的方法在计算机上实现人类的智能。人工智能既是一门综合性学科,又是一项广泛应用的技术。作为科学,它除了与计算机科学技术紧密联系外,还涉及数学、信息论、控制论、自动化、仿生学、生物学、心理学、数理逻辑、语言学、医学和哲学等多门学科;作为技术,它运用于各行各业,减轻了人们体力脑力的劳动。德国著名哲学家海德格尔认为:技术不仅仅是手段,“乃是一种解蔽方式。”,技术的发展逐渐沿着从肢体、器官的延长到躯干、直到大脑的延长的逻辑轨道行进。人工智能即是思维研究成果的运用,又是人脑思维的延伸。人工智能分为强、弱两种形式,弱人工智能(Weak AI)旨在发展研究人类和动物智能的理论,并能通过程序来测试这些理论,而强人工智能(Strong AI)是人工智能的最高形式,它的目标是建造能够思考、拥有意识和感情的机器。本文所讨论的即是强人工智能研究下的哲学思考。 2.2人工智能的诞生和发展 1956年美国达特茅斯学院举行了有关机器模拟人类智能的学术研讨会,会上夏麦卡锡、明斯基等10多位数学、心理学家和信息论等方面的学者第一次使用并确立了人工智能这一术语,它标志着人工智能这门新兴学科的正式诞生。人工智能的发展经历了两个大的阶段:第一阶段(1968~1986年),符号主义一支独秀。符号主义又称为逻辑主义,其原理主要为物理符号系统假设和有限合理性原理,认为人的认识基元是符号,而且认知过程即符号操作过程。第二阶段(1986年以后),联接主义复兴及人工智能枝繁叶茂。20世纪80年代传统人工智能理论发展面临困境,人工神经网络理论出现新的转机,基于结构演化的人工智能理论——联接主义迅速成为人工智能研究的主流之一。1997年,IBM的“深蓝”并
关于人工智能是否会超越人类智能的探讨
关于人工智能是否会超越人类智能的探讨 夏天荣 J10220222 建筑工程学院土木工程2班杭州下沙 310018 摘要:从1956年“人工智能”的提出到如今的迅速发展,社会各界关于“人工 智能”与“人类智能”的对弈从未停止过,反而愈演愈烈。虽然“人工智 能”延长了人的大脑,扩大了人的智能,部分解放了人类的脑力劳动,但 由于两者存在着本质上的差异,“人工智能”是不可能代替“人类智能”, 更不可能超越“人类智能”的。“人工智能”本质是对“人类智能”的模 拟,而且两者智能的存在物质载体也相差甚大,思维程序和复杂程度更是 有天壤之别。因此,这是支配与被支配,设计与被设计的关系,人工智能 的模拟永远在人类智能之后。 关键词:人工智能人类智能模拟 Abstract:From 1956 "artificial intelligence" mentioned to today's rapid development and the social circles about "artificial intelligence" and "human intelligence," Chinese chess playing never stopped, but intensified. Although "artificial intelligence" extended the person's brain, expanded of human intelligence, partly freed humans mental labor, but because both are essentially differences, "artificial intelligence" is impossible instead of "human intelligence," the more impossible to surpass "human intelligence,". "Artificial intelligence" essence of "human intelligence," simulation, and both intelligence existing material carrier also differs greatly, thought process and complexity is make a big difference. Thus, this is dominant and be dominated by design, design and the relationship of artificial intelligence, the simulation in human intelligence forever after. Key words: artificial intelligence human intelligence simulation 为了使“人工智能”朝着正确的方向发展,我们必须正确认识“人工智能”与“人类智能”的辨证关系,既不为此恐慌,也不毫无忧患意识。“人工智能”是包含了多学科的交叉课题,必须从多角度来剖析与探讨。从能动与被动的关系看,机器超过人、统治人是不可能,而且“人工智能”不具有人类智能的本质特征。接着就从以下几点进行论述。 1首先,我们要深入了解什么是人工智能,什么是人类智能。 1.1人工智能
牛津大学校园环境
牛津大学不同于其它的大学,城市与大学融为一体,街道就从校园穿过。大学不仅没有校门和围墙,而且连正式招牌也没有。牛津有一个中央学校(包括校和系图书馆,以及科学实验室),38个学院以及7个永久私人公寓(Permanent Private Halls,PPHs)。这些学院并不只是宿舍,而是实质上负责本科生和研究生的教学。有些学院只接受研究生,这些通常都是近一个世纪建立的新学院,例如Wolfson College。而有一个学院根本就不招收学生,那就是All Souls College。 博物馆 阿什莫林博物馆(Ashmolean Museum) 皮特利弗斯博物馆(Pitt Rivers Museum) 牛津大学自然史博物馆(Oxford University Museum of Natural History) 科学史博物馆(Museum of the History of Science) 360教育集团介绍说,在牛津博物馆也是重要的文化代表。其中阿什莫尔博物馆(Ashmolean Museum)建于1683年,是英国第一座博物馆,比大英博物馆(British Museum)早70年,现为英国第二大博物馆。其它包括牛津故事博物馆、科学史博物馆、庇特河流人种史博物馆、现代艺术博物馆、大学自然历史博馆等。 图书馆 Bodleian图书馆(Bodleian Library) Bodleian法律图书馆(Bodleian Law Library)
Hooke图书馆(Hooke Library) Sackler图书馆(Sackler Library) Radcliffe科学图书馆(Radcliffe Science Library) 牛津共有104个图书馆。其中最大的博德利图书馆是英国第二大图书馆(仅次于大不列颠图书馆),于1602年开放,比大英博物馆的图书馆早150年,现有藏书600多万册,拥有巨大的地下藏书库。剑桥也有近100个图书馆,藏书约500多万册,每年购书经费约300万英镑(折合4000多万人民币)。根据1611年英国书业公所的决定,英国任何一家出版社的图书都必须免费提供一册给牛津和剑桥的图书馆,至今如此。 四合院 市中心周围街两旁布满中世纪的四合院,每个四合院就是一所学院,由于在当时学术是教会的专利,因此学院都以修院式建筑来设计,不过四周往往围绕着美丽的庭园。尽管大多数的学院这些年来多有改变,但是依然融合许多原有特色。 每个学院完全是中世纪修道院的模样,城内多塔状建筑,故又得名“塔城”。位于民众方庭的图书馆,建于1371年,是英格兰最古老的图书馆;大学植物园,建于1621年,是英国最早的教学植物园。 广场、教堂、剧院 雷德克利夫广场(Radicliffe Square)是大学举行庆典及各项文艺活动的中心,这是整个古城的中心。 圣玛利教堂(St.Mary‘s Church)位于广场南边,十七世纪前,学校的主要庆典、音乐会都在这举行。广场西侧是建于1509年的Brasenose College,学院因创校时,大门上有一只铜制的“狮鼻扣门环”而得名。学院正门的天庭中,有一座宝蓝色的日晷钟。 广场的西角是建于十五世纪的神学院(Divinity School),有古典式圆顶的雷德克利夫建筑是牛津很特殊的建筑。它是图书馆的原始建筑。早期作为大学的研讨教室之用,改为大学图书馆Bodlien Library的阅览室。 克莱斯特教堂被牛津人亲切呼为“The House”。万灵学院(All Souls College)是牛津众多学院中唯一没有大学生的学院,因为学院中只有研究生,因而被视为世界最具学术权威的高级学府之一。城东的莫德林学院的城堡,被人们称为“凝固了的音乐”,的确优美异常。钟楼以大青石砌筑,朝天高指。 建于十七世纪的谢尔登尼安剧院是列恩所设计的第一栋建筑,是牛津大学传统授予学位典礼的举行场地。每年夏天,学期结束时,身披黑袍,头戴方帽的学子们,将列队经过大街,进入剧院,在此获得被授予学位的荣誉。
自然辨证法概论—论人工智能与人类智能的关系
论人工智能与人类智能的关系 摘要:人工智能的发展不仅仅指技术层面的进步,人工智能也将引入哲学的范畴。从哲学的角度,应用辩证法和认识论去分析人工智能与人类智能的关系,使人们对人工智能有更清晰、正确的认识,能更好指导人类实践。探讨了人工智能带来的社会问题以及提出的应对策略,使人工智能为人类、为社会创造更大的价值,积极推动社会的协调发展。 关键词:人类智能;人工智能;辨证关系;社会问题 一、前言 进入信息时代的二十一世纪,“人工智能”伴随着电子科学技术和信息技术的膨胀式发展孕育而生。人工智能是一门综合了生命科学、计算机科学、哲学等的前言学科,研究人工智能的目的在于延长和增强人脑的智能,提高主体认识能力。在人工智能的发展过程中,其思想、理论、方法和技术逐渐的渗透到科学技术的诸多领域和人类生活的各个方面,引发了人们对人工智能与人类智能的一系列哲学问题的思考,包括他们的社会属性、主客体关系、主观能动性等辨证关系。同时,人工智能是否会超过人类智能甚至威胁人类也引发了人们的担忧,不可否认的是人工智能的应用带来了科学技术的变革,解放了生产力,加快了社会的发展进程,但是人工智能也引发了一系列关于道德、伦理、责任以及失业的问题。只有正确的认识和对待人工智能的发展,才能更好的使人工智能服务于人类。本文从哲学的角度,应用辩证法和意识论对人工智能和人类智能的辨证关系进行了深入的分析,探讨了人工智能带来的社会问题以及提出相应的对策。 二、人工智能与人类智能的内涵 1.人类智能的内涵 人类智能就是人类认识世界和改造世界的才智和本领。这儿的才智也就是人的知识,人的知识是对客观外界规律性的认识,那么人类智能则是运用这种对客观外界规律性的认识来解决矛盾,有目的地改造客观世界的能力〔1〕。人类智能的物质载体是由上千亿个神经元之间通过网络化和层次化的相互连接组成的神经系统,这个极其复杂、最为协调的神经系统就是人脑。人脑经历了漫长的生理进化以及几百万年的社会进化,人脑具有特有的自然属性和社会属性。意识活动是人脑的特有机能,它是脑神经细胞化学物质和生物电传递和处理信息的过程,也是在生理基础上的心理过程,从而形成的一个相对独立的、具有主观能动反映外部世界功能的精神世界,在大脑的意识活动中,意识的载体是通过语言、文字等信息进行加工的。人类智能的内容涉及到数学计算、逻辑计算以及形象思维。 2.人工智能的内涵
人工智能PK人类智能
人工智能PK人类智能 经过几十年的发展,人工智能已成为涉及计算机、心理学、系统论、博弈论、哲学等领域的交叉学科。人工智能的研究是一项极富挑战性的工作,不论是它的复杂性和学科交叉性,还是它那些带有根本性的思考和创新,其实都是人类对自身的不断认识和挑战。人工智能的研究,最终会不会使人类建成“智能体乌托邦”?人工智能与人类智能的关系问题,从20世纪80年代在国内外就进行了非常激烈的辩论。 首先,让我们了解下人工智能与人类智能。 人类智能活动的能力是人类在认识世界和改造世界的活动中,由脑力劳动表现出来的能力。人类的自然智能(人类智能)伴随着人类活动时时处处存在。人类的许多活动,如下棋、竞技、解算题、猜谜语、讨论问题、编制计划和编写计算机程序,甚至驾驶汽车都需要“”“智能”。而人工智能实际上是在计算机上实现的智能或者说是人工智能在机器上的模拟,因此又可以称为机器智能。人工智能的第一大成就就是发展了能够求解难题下的下棋(如国际象棋)程序。目前人工智能的研究领域包括:自然语言处理、自动定理证明、智能数据检索系统、机器学习、模式识别、视觉系统、问题求解、人工智能方法和程序语言以及自动程序设计。 人工智能从上世纪五十年代诞生起就表现出了极强的生命力,它在上世纪八十年代后期得益于计算机软硬件发展的日新月异而得到迅猛发展。主要表现在军事、医疗、科技等等领域。那么人工智能能否超过人类智能呢?人工智能与人类智能的关系以及人工智能的发展趋势问题,从20世纪80年代在国内外就进行了非常激烈的辩论。既有一部分人认为人工智能只能作为人的工具的延长而不可能取代人的大脑的工具论;也有一部分人持人工智能一定会战胜人类智能的观点,他们从达尔文的进化论进行类比推断,对比人类智能和人工智能相对发展的速度和加速度,认为人类智能虽然在短时期内还占有绝对的优势,但是从人工智能近些年突飞猛进的发展速度和加速度来对比人类智能对等时间发展来看,人工智能战胜人类智能绝对只是时间的问题。 就个人而言,我比较支持前者的观点。人工智能诞生的初衷是作为人类工具的延长,其作用从其诞生的那一天就已经定性,人工智能只能作为人类智能的附庸和补充,而不可能对人类智能构成挑战,
人工智能是否有朝一日超过人类智能
人工智能是否有朝一日超过人类智能? 所谓人工智能(英文缩写为AI)既是是研究、开发用于模拟、延伸和扩展人的智能的理论、方法、技术及应用系统的一门新的技术科学。人工智能是计算机科学的一个分支,它企图了解智能的实质,并生产出一种新的能以人类智能相似的方式做出反应的智能机器,该领域的研究包括机器人、语言识别、图像识别、自然语言处理和专家系统等。“人工智能”一词最初是在1956 年Dartmouth学会上提出的。从那以后,研究者们发展了众多理论和原理,人工智能的概念也随之扩展。人工智能是一门极富挑战性的科学,从事这项工作的人必须懂得计算机知识,心理学和哲学。人工智能是包括十分广泛的科学,它由不同的领域组成,如机器学习,计算机视觉等等,总的说来,人工智能研究的一个主要目标是使机器能够胜任一些通常需要人类智能才能完成的复杂工作。 目前,由《计算机世界》主办的人机对弈与64位技术及人工智能的未来研讨会在北京召开。包括戴汝为(中国工程院院士、中科院自动化所研究员)、陆汝钤(中国科学院院士、中科院数学所研究员)、倪光南(中国工程院院士、中科院计算所研究员)、吴文虎(清华大学教授、博士生导师)、马世龙(北航软件开发环境国家重点实验室副主任、计算机系教授)和杜子德(中国计算机学会秘书长)等计算机和人工智能领域的著名专家学者参加了本次研讨。国际象棋大师刘文哲、诸宸也出席了本次研讨会。各位专家一致认为电脑仍很难全面超越人脑,它只能在某方面超越人脑,而人工智能则更加遥远。 吴文虎说:“赛前就认为计算机获胜的可能性比较大。”各位专家认为,电脑综合了人类国际象棋大师的群体经验,而且不会劳累。但诸宸只是人类最优秀的单个智慧,会劳累。因此诸宸失败是有可能的。电脑从问世之日起,它的计算能力就远远超过人脑。今天电脑在国际象棋上战胜人脑,只是在计算和推理的能力上超过了人脑,但想要在全局把握的能力上超过人脑仍不可能。 对于人工智能,各位专家均表现出了无奈。在863计划初期曾将人工智能放在非常重要的位置上。但后来在研究的过程中,发现人工智能是非常艰巨的研究过程,仍没有找到实现人工智能的方法,很少有突破,以至后来被逐渐冷落。专家认为电脑没有思想,不具备学习的能力,目前只能在计算的能力上超过人类。计算机只能逐渐在某个方面上逐渐超过人类,想要完全超越人类,特别是在情商把握全局的能力上超过人类仍是很遥远的事情。但专家也同时指出,如果人工智能超过了人类就需要限制,否则将给人类带来灭顶之灾。 从某些有名的人工智能与人类智能的对弈当中我们也可以看到人类智能胜于人工智能的“影子”,例如1996年2月10~17日,以4:2战胜(Deep Blue)“深蓝”,Garry Kasparov是因为走错一步棋而战胜“深蓝”,从这也可以看得到“深蓝”只是在比赛前被工作人员输入大量的高手棋艺对决的资料,并实行命令而已,但当面对对手走错棋,并且对手还能走下去的情况下,它不知如何对付自己的对手,因为它搜索不了相关资料,这就是人工智能与天生的人类智能的区别了,最后“深蓝”败给,谱写了人工智能远不及人类智能的历史又一个章程。但是到后来Garry Kasparov又输给改造的“深蓝”,但是这也不能说明人工智能强于人类智能,虽然说Garry Kasparov输给了深蓝,但深蓝是人类工程师制造的,其所有棋步于演算来自全世界的国际象棋棋手,可以说是Garry Kasparov在跟全世界顶尖的国际象棋棋手下棋,而深蓝只是个媒介,归根结底他还是输给了人类智能.人工智能是不会战胜人类的.因为没有人类工程师的制造与输入资料,“深蓝”根本不能运作。更何况与人类对弈呢?这也是一个很好的例子说明人工智能只能跟在人类智能的后面,永难超越人类智能。据说美国人工智能专家罗德尼.布鲁克斯打算在4年内就将他的机器人Cog开发成至少要具备一个小孩的各方面的智慧和能力;而加里斯则已经开始着手研制人造脑袋,并要在2011年使第三代人造脑袋的智能超过人类的40多倍。(其实,这样的言论已经并不新鲜了。早在1970年,明斯基就曾经做过离谱的预言:“在三年到八年的时间里,我们将研制出具有普通人一般智力的计算机。这样的机器能读懂莎士比亚的著作,会给汽车上润滑油,会玩弄政治权术,能讲笑话,会争吵。到了这个程度后,计算机将以惊人的速度进行自我教育。几个月之后,它将具有天才的智力,再过几个月,它的智力将无以伦比。”) 从所周知“阿西莫夫机器人三定律”也是人定出来的,对于"死"的机器绝对有用,但是"自组织"却是
牛津大学地理位置
https://www.360docs.net/doc/bc11937038.html, 牛津大学地理位置 牛津大学(University of Oxford),简称“牛津(Oxford)”,立思辰留学360介绍说,位于英国牛津,是一所誉满世界的公立研究型大学。 牛津大学本科专业: 考古学和人类学、生物化学、生物科学、生物医学、化学、古典考古学和古代史、古希腊/罗马文学、古典文学和英语、古典文学与现代语言、古典文学与东方研究、计算机科学、计算机科学与哲学、地球科学、经济学与管理、工程科学、工程、经济与管理、英语语言和文学、英语与现代语言学、欧洲与中东语言学、实验心理学、艺术、地理、历史、历史与经济、历史与英语、历史与现代语言、历史与政治、艺术历史、人文科学、法律、材料科学、材料经济与管理、数学、数学与计算机科学、数学与哲学、数学与统计、医学、现代语言、现代语言与语言学、音乐、东方研究、哲学与现代语言、哲学政治与经济、哲学与宗教、物理学、物理学与哲学、心理学与哲学、心理学、哲学与语言学、神学、神学与东方研究 音乐学法语德语西班牙语古典学美术捷克文俄语意大利语葡萄牙语艺术史法语和语言学西班牙语和语言学现代希腊语德语和语言学意大利语和语言学历史学与英语英文和法文英语和法文英语和法文英语和德语英语和俄语俄语和语言学凯尔特语东方文化研究哲学与法语哲学与德语
https://www.360docs.net/doc/bc11937038.html, 哲学与意大利语哲学与俄罗斯哲学和西班牙语历史和意大利语历史和西班牙语英语和西班牙语 英语和意大利语英语和葡萄牙语历史与葡萄牙语历史和俄语经典英文古典与东方学研究历史与现代语言神邪恶与东方研究法语与历史历史与德语英语与凯尔特语英语与捷克语英语与现代希腊语古 典文学与凯尔特语古典文学与捷克语历史与凯尔特语古典文学与德语古典文学与近代希腊语古典文学与意大利语古典文学与葡萄牙语古典文学与俄语古典文学与西班牙语历史与捷克语历史与现代希 腊语凯尔特语与语言学捷克语语言学现代希腊语与语言学哲学与凯尔特语哲学与捷克语哲学与近代希腊语欧洲与阿拉伯语欧洲与希伯来语欧洲与波斯欧洲与土耳其 牛津大学研究生专业: 麻醉学、人类学、考古科学、建筑史、区域研究、设计史、国际人权法律、国际野生动物保护、法律、生命科学、语言语音学、文学与艺术、管理研究、材料学、数学、计算机学、数学与财务、肿瘤医学、医疗科学、现代语言、骨骼科学、生物化学、心血管医学、犯罪学、医疗保健、法律学习、化学、古希腊/ 罗马文学、音乐、纳米科技、神经系统科学、妇科医学、儿科学、眼科学、东方研究、网络科技、病理学、药理学、哲学、物理学、计算机科学、经济学、教育学、创意写作、发展研究、地球科学、工程科学、英语语言学、心理学、艺术、软件工程、统计学、城市发展、政治与国际关系学、公共卫生
人类智能与人工智能的认识
人类智能与人工智能 姓名:康李伟学号:2015387950721 学院:生化学院 随着信息技术的发展,人类把最新的计算机技术应用于各个学科,对这些学科的认知也进入了日新月异的发展阶段,促使大量的新的研究成果不断涌现。机器思维和人工智能在未来发展的可能性和重要性有其值得重视的一面,但机器思维只是人的思维在一定程度上的延伸和补充,而不是一种独立的思维,机器思维同人类思维二者虽然存在着一定的相似之处,但由于其思维的物质承担者不同,在智能活动中的地位和思维程序也不同,所以有本质的区别。 什么是人类智能呢?人类智能就是人类认识世界和改造世界的才智和本领。人类之所以能成为万物之灵,是因为人类具有能够高度发展的智能。它包括“智”和“能”两种成分。“智”主要是指人对事物的认识能力;“能”主要是指人的行动能力,它包括各种拔能和正确的习惯等等。人类的“智”和“能”是结合在一起而不可分离的。人类的劳动、学习和语言交往等活动都是“智”和“能”的统一,是人类独有的智能活动。意向是人类智能的一个重要方面。人的活动是有目的的、自觉的活动,一刻也离不开自己意向的主导。注意、需要、意图、情绪、意志、理想等都是人的意向活动形式。保持积极的意向、恰当的情绪和顽强的斗志等等,对人类智能的发展和发挥是十分重要的。思维是人类智能的核心。人类智能的特点主要是思想,而思想的核心又地思维。“人是一种思维的动物”,没有思维就没有人类的智能。有了思维,人类才能形成各种较复杂的意向,从而主导着人的活动,表现出人类所特有的自觉能动性。有了思维,人类才能探索自然界的奥秘,发现自然现象背后的规律。有了思维,人类才能发明各种技术,突破自己认识器官和行动器官的限制,大大提高改造世界的能力。 而人工智能是研究、开发用于模拟、延伸和扩展人的智能的理论、方法、技术及应用系统的一门新的技术科学。人工智能是计算机科学的一个分支,它企图了解智能的实质,并生产出一种新的能以人类智能相似的方式做出反应的智能机器,该领域的研究包括机器人、语言识别、图像识别、自然语言处理和专家系统等。人工智能从诞生以来,理论和技术日益成熟,应用领域也不断扩大,可以设想,未来人工智能带来的科技产品,将会是人类智慧的“容器”。 人工智能的本质是对人类智能思维的模拟从方法论上讲模拟一般分为 两种结构模拟和功能模拟结构模拟方法根据系统之间形态结构的相似性运 用物理模拟和数学模拟方法用模型去模拟对象通过模型来间接地研究原型 的规律性这种传统模拟方法在科学技术的发展过程中发挥了巨大的作用。 但是当系统之间在形态和结构上相差极大,或原型的结构机制没有得到充分的理解时,结构模拟方法就很难进行对人脑进行结构模拟,就是仿照人脑的结构机制试图制造出类人脑,其模拟的前提是人脑这个原型的结构机制已有了可靠的理解。但是由于人脑这一巨大系统的极端复杂性,当代脑科学尚没
人工智能与信息社会2019尔雅答案
第一章 时代主要的人机交互方式 () 。D 为 A、鼠标 B、键盘 C、触屏 D、语音 +视觉年 3 月, 人工智能程序 () 在韩国首尔以 4:1 的比分战胜的人类围棋冠军李世石。 A A、AlphaGo B、DeepMind C、Deepblue D、AlphaGo Zero 是() 推出的个人语音助手。 C A、苹果 B、亚马逊 C、微软 D、阿里巴巴 4.首个在新闻报道的翻译质量和准确率上可以比肩人工翻译的翻译系统是 () 。C A、苹果 B、谷歌 C、微软 D、科大讯飞 5.相较于其他早期的面部解锁,iPhone X 的原深感摄像头能够有效解决的问题是 () 。 C A、机主需要通过特定表情解锁手机 B、机主是否主动解锁手机 C、机主平面照片能够解锁手机 D、机主双胞胎解锁手机 6.属于家中的人工智能产品的有 () 。ABD A、智能音箱 B、扫地机器人 C、声控灯 D、个人语音助手 7.谷歌相册与传统手机相册最大不同点是 () 。ABE A、根据照片内容自动添加标记 B、根据不同标记进行归类和搜索 C、自动对照片进行美颜
D、定时备份照片 E、人脸识别和搜索 8.目前外科手术领域的医用机器人的优点有 () 。AB A、定位误差小 B、手术创口小 C、不需要人类医生进行操作 D、能够实时监控患者的情况 E、可以帮助医生诊断病情 9.智能推荐系统的特点包括 () 。ABCD A、根据用户的购买记录记忆用户的偏好 B、根据浏览时间判断商品对用户的吸引力 C、推荐用户消费过的相关产品 D、根据用户的喜好进行相关推荐 10.一般来说 , 扫地机器人必需的传感器有 () 。 ABC A、距离传感器 B、超声波雷达传感器 C、悬崖传感器 D、温度传感器 11.在神经网络方法之前 , 机器翻译主要是基于统计模型的翻译。 () 正确 12.人工智能具有学会下棋的学习能力 ,是实现通用人工智能算法的基础。() 正确 13.目前还没有成功进行无人自动驾驶的案例。 () 错误 14.智能家居应该能自动感知周围的环境 ,不需要人的操控。 () 正确 15.智能音箱本质上是音箱、智能语音交互系统、互联网、内容叠加的产物。() 正确 16.基于句法的机器翻译是目前较为流行的翻译方法,基本达到了预期的理想。() 错误 第二章 1.被誉为计算机科学与人工智能之父的是 () 。A A、图灵 B、费根鲍姆 C、纽维尔 D、西蒙
人工智能和人类智能英文演讲
Can Artificial intelligence exceed胜过human intelligence? 子不语之: Good afternoon, boys and girls. I feel really honored to stand here and make a speech. First of all, please allow me to introduce myself ……. Today, we will talk about “Can Artificial intelligence exceed human intelligence? Back ground: First, let’s talk about what is AI.AI is a new subject which is developed by computer science, artificial intelligence, control theory, information theory, linguistics语言学, neurology神经学, psychology, mathematics, philosophy哲学人生观and other disciplines科目. So AI is a comprehensive综合的subject that has much development space. But why we raise this topic here? Because in recent days, a great chess game had been held on Google between Li Shishi and AlphaGo. The result of the game was 1-4, AlphaGo, which is an artificial intelligence, won the game. This event shocked the world. However, it’s not the first time that the artificial intelligence win the human intelligence. For example, 17 years ago Deep Blue also win the chess game, the movie
英国牛津大学发展历程是怎样的
牛津教学的最大特点是“导师制”。学生的导师由研究人员担任,他们多为品学俱佳的学者,在一定的领域卓有建树。导师制要求学生每周与导师见一次面,将自己一周内研究和撰写的论文向导师宣读。此外,还有许多讲座。每个讲座不论是导师还是学生,不论是高年级还是低年级,都可以自由发言,平等讨论,但在议论之后,要交作业。与导师单独见面,宣读一周内研究和撰写的论文时,导师要评论,要提问,如果论文质量不行,答辩不好,要影响成绩、影响毕业。一周一次,周复一周。 360教育集团介绍说,牛津是泰晤士河谷地的主要城市,传说是古代牛群涉水而过的地方,因而取名牛津(Oxford)。牛津向来是伦敦西行路线上的重点,早在1096年,就已有人在牛津讲学。 在12世纪之前,英国是没有大学的,人们都是去法国和其它欧陆国家求学。 1167年,当时的英格兰国王同法兰西国王发生争吵,英王一气之下,把寄读于巴黎大学的英国学者召回,禁止他们再去巴黎大学。另一说法是,法王一气之下,把英国学者从巴黎大学赶回英国。不管如何,这些学者从巴黎回国,聚集于牛津,从事经院哲学的教学与研究。于是人们开始把牛津作为一个“总学”,这实际上就是牛津大学的前身。学者们之所以会聚集在牛津,是由于当时亨利二世把他的一个宫殿建在牛津,学者们为取得国王的保护,就来到了这里。 12世纪末,牛津被称为“师生大学”。 1201年,它有了第一位校长。 1209年,在牛津学生与镇民的冲突事件过后,一些牛津的学者迁离至东北方的剑桥镇,并成立剑桥大学。自此之后,两间大学彼此之间展开相当悠久的竞争岁月。 1214年6月20日,经过一名教皇使节的磋商,大学重新回到了牛津,并得到了特许。牛津大学的身份直到1571年通过的一项法案而得到正式的确定。 正是在中世纪,那些思想活跃、生活不羁、常赊欠债务的青年学生,不可避免地与当地居民发生冲突。其中最大的一次冲突,几百名学生被乱箭射死。这件事的结果是国王出面镇压,并判市民赔偿牛津大学500年费用。 构成牛津大学的学院有许多在13至16世纪之间创立,基督教会学院是1525年渥西枢机主教创建,作为培训主教的教会学院。汤姆方园里的塔楼上半部是列恩在1682年建造,为本市最大型塔楼。 1648年汤姆大钟挂上时,学院共有101名学生,因此在晚上9:05分(牛津的时间比格林威治慢5分钟)大钟敲101下,提醒学生注意宵禁(1963年起不再实施)。 默顿学院是牛津最古老的学院(1264年)。其它有:新学院(1379年)、皇后学院(1695年)等。 17世纪内战期间许多战役在牛津附近开打,此地曾为受学生支持的查理一世的总司令部。当保皇党员被迫逃离牛津时,克伦维尔自封为大学的校长。
人工智能与人类智能比较
第一章 关于人工智能能否超过人类智能的讨论 摘要:随着信息技术的发展,人类把最新的计算机技术应用于各个学科,对这些学科的认知也进入了日新月异的发展阶段,促使大量的新的研究成果不断涌现。人工智能与人类智能的关系问题,从20世纪80年代在国内外就进行了非常激烈的辩论。本文做出了人工智能只能作为人类工具而不可能超过人类智能的论断,人工智能本质上是对人类智能的功能模拟。机器思维和人工智能在未来发展的可能性和重要性有其值得重视的一面,但机器思维只是人的思维在一定程度上的延伸和补充,而不是一种独立的思维,机器思维同人类思维二者虽然存在着一定的相似之处,但由于其思维的物质承担者不同,在智能活动中的地位和思维程序也不同,所以有本质的区别。人工智能没有人类智能所特有的创造性和社会性,只不过是人类智能的延伸,至多只是部分地超越人类智能而不能完全地战胜人类智能。本文最后得出结论:人类对人脑的功能会不断地进行认识,从而人工智能会不断的迫近人类智能。但从动态分析上,即人类智能也在不断的进化和发展,人工智能作为人类智能主体客体化的产物,其作用和功能受到人类智能的制约所以要低于人类智能,人工智能在整体上是不能最终代替和战胜人类智能的。 关键词:人工智能,超越,人类智能,智能模拟 1.研究背景 人工智能这个术语自1956年由数学家麦卡锡正式提出,并作为一个新兴学科的名称被使用以来,已经有50多年的历史了。人工智能的发展过程,大致经历了孕育期、形成期、知识应用期、和综合集成这四个阶段。人工智能(Artificial Intelligence),英文缩写为AI,是一门综合了计算机科学、生理学、哲学的交叉学科。“人工智能”一词最初是在1956年美国计算机协会组织的达特莫斯(Dartmouth)学会上提出的。从那以后,研究者们发展了众多理论和原理,人工智能的概念也随之扩展。人工智能的概念由于智能概念的不确定因此没有一个统一的标准。童天湘在《从“人机大战”到人机共生》中这样定义人工智能:“虽然现在的机器不能思维也没有‘直觉的方程式’,但可以把人处理问题的方式编入智能程序,是不能思维的机器也有智能,使机器能做那些需要人的智能才能做的事,也就是人工智能。”【1】 人工智能在发展过程中形成了几个学派,最主要的两个学派是符号主义和连接主义,行为主义是后来形成的一个学派【1】。 人工智能与人类智能的关系以及人工智能的发展趋势问题,从20世纪80年代在国内外就进行了非常激烈的辩论。既有一部分人认为人工智能只能作为人的工具的延长而不可能取代人的大脑的工具论,本人也支持此种观点,即:人工智能诞生的初衷是作为人类工具的延长,其作用从其诞生的那一天就已经定性,人工智能只能作为人类智能的附庸和补充,而不可能对人类智能构成挑战,更不可能取代人类智能。 也有一部分人持人工智能一定会战胜人类智能的观点,他们从达尔文的进化论进行类比推断,对比人类智能和人工智能相对发展的速度和加速度,认为人类智能虽然在短时期内还占有绝对的优势,但是从人工智能近些年突飞猛进的发展
杜伦大学地理位置
https://www.360docs.net/doc/bc11937038.html, 杜伦大学地址:the palatine centre, durham university , stockton road, durham,dh1 3le,uk 皇后校区地址:durham university, queen‘s campus, stockton, university boulevard, thornaby, stockton-on-tees ts17 6bh, uk 1. 杜伦市与伦敦相距264公里,距离伯明翰187公里,距离爱丁堡125公里,距离约克67公里,市中心距离the a1(m)仅2公里 2. 英国多个主要城市均有特快车到达杜伦市,从杜伦市汽车站步行至火车站,可乘每15分钟的公共汽车到达杜伦大学 3. 每天约有60班实际火车运行于杜伦市和其他主要城市之间,从伦敦出发的有14班,乘坐从杜伦市到达伯明翰仅需3小时,到达曼彻斯特两个小时,到达约克仅需45分钟,在杜伦火车站搭乘出租车到达杜伦大学任一学院仅需大约五分钟 4. 杜伦大学距离纽卡斯尔机场约30分钟车程,距离达勒姆蒂斯山谷机场约40分钟车程,两个机场均有国内及国际航班 杜伦大学简介 杜伦大学(University of Durham),又译作达勒姆大学,世界百强名校,亦是全英国前10的顶尖大学,在英国大学各类排行榜中长期处于第3位至第6位,在英国乃至全世界一直享有极高美誉。立思辰留学360了解到它是位于英国东北部小城达勒姆的一所世界著名公立研究型大学,与剑桥大学、牛津大学同为传统学院制的联邦制大学,这三所学校也是英格兰地区最古老的古典大学,统称“doxbridge”。杜伦也是著名的科英布拉集团和罗素大学集团成员之一。