Multi_instancelearnin_省略_tedsystems_acas
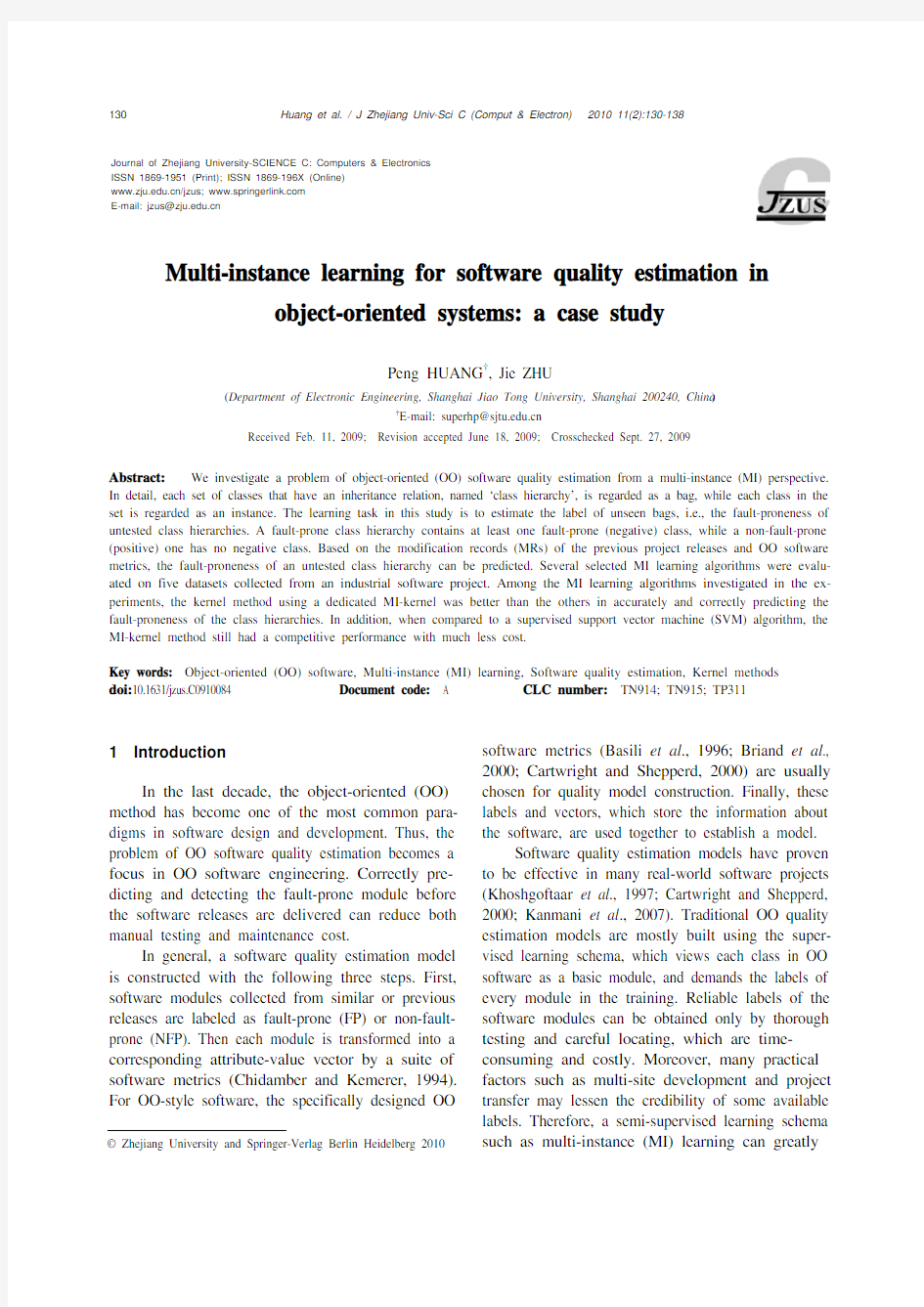

Huang et al. / J Zhejiang Univ-Sci C (Comput & Electron) 2010 11(2):130-138
130
Multi-instance learning for software quality estimation in
object-oriented systems: a case study
Peng HUANG?, Jie ZHU
(Department of Electronic Engineering, Shanghai Jiao Tong University, Shanghai 200240, China)
?E-mail: superhp@https://www.360docs.net/doc/f2945479.html,
Received Feb. 11, 2009; Revision accepted June 18, 2009; Crosschecked Sept. 27, 2009
Abstract: We investigate a problem of object-oriented (OO) software quality estimation from a multi-instance (MI) perspective. In detail, each set of classes that have an inheritance relation, named ‘class hierarchy’, is regarded as a bag, while each class in the set is regarded as an instance. The learning task in this study is to estimate the label of unseen bags, i.e., the fault-proneness of untested class hierarchies. A fault-prone class hierarchy contains at least one fault-prone (negative) class, while a non-fault-prone (positive) one has no negative class. Based on the modification records (MRs) of the previous project releases and OO software metrics, the fault-proneness of an untested class hierarchy can be predicted. Several selected MI learning algorithms were evalu-ated on five datasets collected from an industrial software project. Among the MI learning algorithms investigated in the ex-periments, the kernel method using a dedicated MI-kernel was better than the others in accurately and correctly predicting the fault-proneness of the class hierarchies. In addition, when compared to a supervised support vector machine (SVM) algorithm, the MI-kernel method still had a competitive performance with much less cost.
Key words: Object-oriented (OO) software, Multi-instance (MI) learning, Software quality estimation, Kernel methods
doi:10.1631/jzus.C0910084 Document code: A CLC number: TN914; TN915; TP311
1 Introduction
In the last decade, the object-oriented (OO) method has become one of the most common para-digms in software design and development. Thus, the problem of OO software quality estimation becomes a focus in OO software engineering. Correctly pre-dicting and detecting the fault-prone module before the software releases are delivered can reduce both manual testing and maintenance cost.
In general, a software quality estimation model is constructed with the following three steps. First, software modules collected from similar or previous releases are labeled as fault-prone (FP) or non-fault- prone (NFP). Then each module is transformed into a corresponding attribute-value vector by a suite of software metrics (Chidamber and Kemerer, 1994). For OO-style software, the specifically designed OO software metrics (Basili et al., 1996; Briand et al., 2000; Cartwright and Shepperd, 2000) are usually chosen for quality model construction. Finally, these labels and vectors, which store the information about the software, are used together to establish a model.
Software quality estimation models have proven to be effective in many real-world software projects (Khoshgoftaar et al., 1997; Cartwright and Shepperd, 2000; Kanmani et al., 2007). Traditional OO quality estimation models are mostly built using the super-vised learning schema, which views each class in OO software as a basic module, and demands the labels of every module in the training. Reliable labels of the software modules can be obtained only by thorough testing and careful locating, which are time- consuming and costly. Moreover, many practical factors such as multi-site development and project transfer may lessen the credibility of some available labels. Therefore, a semi-supervised learning schema such as multi-instance (MI) learning can greatly
Journal of Zhejiang University-SCIENCE C: Computers & Electronics ISSN 1869-1951 (Print); ISSN 1869-196X (Online)
https://www.360docs.net/doc/f2945479.html,/jzus; https://www.360docs.net/doc/f2945479.html,
E-mail: jzus@https://www.360docs.net/doc/f2945479.html,
? Zhejiang University and Springer-Verlag Berlin Heidelberg 2010
Huang et al. / J Zhejiang Univ-Sci C (Comput & Electron) 2010 11(2):130-138131
reduce the number of necessary labels, which con-tributes towards both effort reduction and reliability enhancement. Other important reasons for introduc-ing MI learning to the OO software quality estimation problem include:
1. In practice, fault prediction tools can be used only as an early auxiliary component for large-scale software projects. Manual and automatic testing is still unavoidable to assure the software quality. Thus, a fault prediction model does not need to localize the fault in a very small area.
2. Limited fault data always reduce the size of available training data due to many practical software engineering problems (Seliya and Khoshgoftaar, 2007), while MI learning can utilize plentiful attribute-value vectors and relatively few labels.
3. In OO systems, the complete definition of an inherited method is allowed to be absent in the class that inherits it. A fault or modification in a class may not be separated from other classes in the same class hierarchy, meaning that these classes should be learned as a whole.
4. Even when some inheritance-related software metrics are used, treating class as a basic module (supervised learning schema) can still partly ignore the relational information hidden in the inheritance- related classes as reported in previous research (Huang and Zhu, 2008).
In MI learning, the elemental learning units are labeled bags which are composed of unlabeled in-stances. Under the given precondition that there is at least one positive instance in a positive bag while no positive instance in the negative bags, the task of MI learning is to predict the label of unseen bags. Diet-terich et al. (1997) demonstrated that supervised learning algorithms such as the popular decision tree and neural networks do not work well in this scenario compared with their proposed MI learning framework.
The main contribution of this study is to inves-tigate the effect of MI learning in estimating OO software quality. Several popular MI learning algo-rithms were selected and experimentally evaluated on five datasets collected from an industrial optical communication software project. A supervised sup-port vector machine (SVM) algorithm was also stud-ied for comparison. The experimental results revealed that the kernel method combining a special MI kernel and SVM has a superior performance in terms of prediction accuracy and Type I and Type II error ra-tios among the studied MI learning methods. Al-though it was slightly inferior to the two-step super-vised support vector classifier (SVC), the MI-kernel method still had a competitive outcome with much less effort in obtaining labels.
2 Related works
2.1 Supervised learning method
Most attention of related works is given to su-pervised learning methods for software quality esti-mation. Briand et al. (2000) took logistic regression to explore the relationships between four groups of OO metrics and the fault-detected system classes during testing. Khoshgoftaar et al. (1997) applied back propagation neural networks to predict the fault-prone software modules in a large telecommunication pro-ject. Kanmani et al. (2007) investigated two fault prediction models based on OO metric and neural networks using a dataset of software modules de-signed by students. Among the two neural networks, the probabilistic neural network outperformed the back propagation one in accurately predicting the fault-proneness of the OO modules. Reformat et al. (2003) exploited several techniques of computational intelligence to support the quality assessment of in-dividual software objects. These techniques covered granular computing, neural networks, self-organizing maps and evolutionary-based developed decision. Recently, Elish and Elish (2008) evaluated the capa-bility of SVMs in predicting defect-prone software modules and compared their prediction performance to eight statistical and machine learning models in the context of four National Aeronautics and Space Ad-ministration (NASA) datasets.
Several other supervised learning approaches including regression trees (Khoshgoftaar et al., 2002), Bayesian belief networks (Fenton et al., 2002), deci-sion trees (Huang et al., 2006), genetic programming (Evett et al., 1998), and random forests (Guo et al., 2004) have also been reported for software quality estimation.
2.2 Semi-supervised learning method
Semi-supervised learning for software quality estimation was first introduced by Seliya and
Huang et al. / J Zhejiang Univ-Sci C (Comput & Electron) 2010 11(2):130-138
132 Khoshgoftaar (2007). They viewed the class labels as missing values and used an expectation-maximization (EM) algorithm to estimate the labels, which repre-sented the class fault-proneness. According to their study, the semi-supervised learning using an EM algorithm provided comparable results to the com-pared supervised classifiers. Catal et al . (2008) stud-ied a software quality prediction model using a simple semi-supervised learning algorithm called YATSI (Driessens et al ., 2006). This semi-supervised ap-proach had a similar performance to the leading su-pervised classifier using random forest (RF) on some public software datasets.
In our prior work (Huang and Zhu, 2008), a kernel method with a layered kernel has been applied to quality prediction of the class hierarchy in OO systems. Compared to the statistical kernel, the lay-ered kernel showed a superior performance for both the artificial and real-life datasets. 2.3 MI learning applications
MI learning was first introduced for drug activity prediction (Dietterich et al ., 1997). As a new learning framework, MI learning has been given much atten-tion and applied to many machine learning problems, such as scene classification (Zhou and Zhang, 2006), web mining (Zhou et al ., 2005), data mining applica-tions (Ruffo, 2000), text categorization (Andrews et al ., 2003), image categorization (Tang et al ., 1999), and object class reorganization (Chen et al ., 2006). To our knowledge, MI learning for OO software quality estimation has not been reported.
3 Multi-instance learning for object-oriented software quality estimation
The MI learning problem in this paper is limited to the definition below, which is consistent with its standard form (Dietterich et al ., 1997):
Definition (MI problem) An MI concept is a func-tion c MI on 2χ→?. Here ?={+1, ?1} and χ denotes the instance space. It is defined as
MI I ()1iff :()1,c X x X c x =??∈=? (1)
where c MI is a specific concept from a concept space, and X ?χ is a set of instances. Note that compared to the usual formulation of the MI setting, positive and
negative labels have changed their roles in Eq. (1).
As shown in Fig. 1, the basic learning unit in our study is not a single class, but a bag of classes. The MI bagging schema in this study is clustering by inheri-tance relation. Preliminary experiments (Huang and Zhu, 2008) have showed that knowledge concerning the inheritance relationship can be better reserved in the class hierarchies if they are trained as a whole rather than as a group of separate instances. In our MI learning model for OO software, each set of classes that have inheritance relation, named ‘class hierarchy’ (Fig. 2), is regarded as a bag, while each class is re-garded as an instance. Each class instance is repre-sented by a vector C =[c 1, c 2, …, c n ], where c i (i =1, 2, …, n ) denotes the measurement on this class using the i th member in the suite of software metrics for this project. The suite of OO software metrics selected in our experiments is described in Section 5.2. Now the MI prediction task of our software quality estimation is to label the untested class hierarchy as FP (negative) or NFP (positive). A positive bag means that no fault- prone class exists in this class hierarchy, while a negative bag is a class hierarchy containing at least one fault-prone class.
Fig. 1 The difference between (a) supervised learning and (b) MI learning in software quality estimation
Fig. 2 An example of an MI bag for object-oriented software
Classes in a inheritance tree A bag in MI Learning
Huang et al. / J Zhejiang Univ-Sci C (Comput & Electron) 2010 11(2):130-138 133
Table 1 Selected MI learning methods for comparison
Symbol Method description MIOptimal- Ball Optimal ball algorithm for MI (Auer and Ortner, 2004) Citation- kNN Citation k -nearest neighbors algorithm (Wang and Zucker, 2000) MI-SVM Support vector machine for MI (Andrews et al ., 2003)
MI-DD Diverse density approach for MI (Maron and Lozano-Pérez, 1998) MI-EMDD Diverse density approach with expectation
maximization for MI (Zhang and Gold-man, 2001) MI-ID3 MI version of the decision tree algorithms ID3 (Chevaleyre and Zucker, 2001) MI-BPN Back propagation neural networks for MI
(Zhang and Zhou, 2004) MI-kernel An MI kernel method
It is worth mentioning that encapsulating each class hierarchy into a bag is an effective but not the only available schema for the MI learning in OO software quality modeling. Other bagging rules such as clustering by software function or by designer can also be considered.
4 Selected MI learning algorithms for evaluation
Many learning algorithms have been developed for MI problems. All these algorithms can be divided into two major categories: specifically designed ap-proaches and approaches adapted from existing su-pervised learning methods. The axis-parallel rectan-gles (APR) algorithms and its variants (Dietterich et al ., 1997), the diverse density (DD) approach (Maron and Lozano-Pérez, 1998) and its upgraded version EM-DD (Zhang and Goldman, 2001), and a boosting method devised to find the optimal ball in MI space (Auer and Ortner, 2004) belong to the first category. The lazy learning algorithms Bayesian-kNN and Citation-kNN (Wang and Zucker, 2000), the decision tree algorithm MI-ID3 (Chevaleyre and Zucker, 2001), the back propagation (BP) neural network MI-BPN (Zhang and Zhou, 2004), and the MI ver-sions of SVM (Andrews et al ., 2003) are in the second category. Recently, some MI-kernels were also intro-duced (Gartner et al ., 2002) to solve the MI problems.
Several representative algorithms (Table 1) were selected to investigate the MI learning in OO software
quality estimation. The MI-kernel method was im-plemented using the SimpleSVM (SSVM) toolkit
(Vishwanathan et al ., 2003), and other algorithms were based on the open source WEKA (Waikato En-vironment for Knowledge Analysis, http://www.cs. https://www.360docs.net/doc/f2945479.html,/~ml/weka/) learning toolkit. The ex-perimental results are detailed in Section 6.
5 An empirical study
5.1 Description of the software system
The software project used to investigate the MI learning based OO software quality estimation was taken from the optical communication domain. Writ-ten in C++, this software project implements mainly commuting and transporting data packages varying from 2 kb to 10 Gb in the synchronous digital hier-archy (SDH) networks. It was developed by the soft-ware development team in the optical networks de-partment of Alcatel-Lucent, Shanghai. Another in-dependent system verification and validation team (SVT) in the same department undertook the testing. After the codes passed the functional test performed by software team (SW) itself, they were verified and validated by the SVT in the practical communication environment. Hundreds of test-cases, including both manual and automatic tests, were executed to ensure product quality. If the test results did not meet re-quirements, corresponding modification reports (MRs) were proposed. Each MR was assigned a severity level from 1 to 5 with increasing severity.
The selected software release comprised about 170 000 lines of code and a total of 425 classes. After clustering by inheritance relation, the whole dataset had 175 bags including 33 multi-class bags. The biggest bag contained 32 instances. The mean in-heritance height of all class hierarchies (bags) and multi- class class hierarchies (bags) was 1.57 and 2.33, respectively. Because multiple inheritance mecha-nism tends to trigger code collision and misunder-standing, it was seldom used in this release. 5.2 Dataset construction
The whole dataset was constructed with the la-bels of the class hierarchies and a suite of appropriate software metrics. In our work, the fault-proneness label of the class hierarchy (bag) was determined by
Huang et al. / J Zhejiang Univ-Sci C (Comput & Electron) 2010 11(2):130-138 134
the number of MRs and their severity. In general, if a bag’s weighted sum of the related MRs is more than 2.5, it is viewed as fault-prone (negative).
Software metrics are usually used to capture the characters and the quality trends of software modules. For OO software projects, many specifically designed OO metrics have been proposed and evaluated. Bri-and et al. (2000) investigated 64 existing software metrics in OO systems. All these metrics can be di-vided into four groups, i.e., size, coupling, cohesion and inheritance measure. In this study, 21 commonly used software metrics (Table 2) in each of the four groups were taken to measure each class, which cover the core sets in Kanmani et al. (2007) and Chidamber and Kemerer (1994). In general, fewer than 10 well-selected metrics are quite powerful in applica-tion (Berard, 1998). According to Briand et al. (2000) and Kanmani et al. (2007), many existing OO metrics are based on similar ideas and hypotheses, and therefore they are almost always interrelated and somewhat redundant. Thus, a large number (e.g., 20 to 30, or more) of different metrics can store sufficient information for the vectors for classes.
Since the software release delivered to the sys-tem verification and validation team has already been debugged, the distribution of positive and negative instances in the whole dataset tends to be unbalanced. According to our labeling schema, only 27 instances were labeled as faulty (negative) compared with 148 labeled as non-faulty (positive). To investigate the effect of unbalanced data, five datasets were taken from the software project. D1 is the whole dataset, D2 (100 bags in total) and D3 (50 bags in total) contain all 33 multi-class instances. D4 (100 bags in total) and D5 (50 bags in total) contain all 27 negative instances. The remaining instances in D2–D5 were randomly selected. The details of the datasets are tabulated in Table 3.
5.3 Parameters for investigated algorithms
The parameters for each of the selected MI learning algorithms are listed below. If a parameter varied in a group of values in the experiment, only the best result was recorded.
1. Citation-kNN: numbers of citation (C n) and reference (R) both varied from 1 to 10.
2. MI-SVM: the regularization parameter (C) was set at 1; the kernel function was RBF (radial basis function), and its bandwidth (γ) was set at 0.5.
3. MI-BPN: a three-layered multi-layer percep-tron (MLP) was used as the network architecture. The number of hidden nodes is set to 4, the learning rate is set to 0.3, the momentum is set to 0.2, and the training time is set to 500 epochs.
4. MI-DD, MI-EMDD, MI-ID3 and MIOpti-malBall: default setting of WEKA.
5. MI-kernel: the investigated kernel function is in the form of Eq. (2), and the parameter p varied from 1 to 10.
MI I
,
(,)(,)
p
x X y Y
K X Y k x y
∈∈
=∑. (2)
Here k I(x, y) is a kernel on instance space χ, and X, Y?χ.
Table 2 Selected OO software metrics
Metric Description CBO Coupling between object classes
CSAO Class size (attributes & operations)
CSA Class size (attributes)
CSI Class specialization index
CSO Class size (operations)
DIT Depth of inheritance tree
LOC Lines of code
LOCM Lack of cohesion methods
NAAC Number of attributes added
NAIC Number of attributes inherited
NAOC Number of operations added
NOIC Number of operations inherited
NPavgC Average number of method parameters NSUB Number of sub classes
OSavg Average operation size
PA Private
attribute
usage
PPPC Percentage public/protected members
RFC Response
for
class
SLOC Source lines of code
TLOC Total lines of code
WMC Weighted methods in class
Table 3 Experimental datasets
Number of datasets
Dataset
Positive Negative Single-class Multi-class D1 148 27 142 33 D2 82 18 67 33
D3 36 14 17 33
D4 73 27 70 30
D5 23 27 22 28
Huang et al. / J Zhejiang Univ-Sci C (Comput & Electron) 2010 11(2):130-138 135
5.4 Estimation performance measures
To evaluate the performance of each prediction
model, the prediction accuracy was measured.
Moreover, Type I and Type II error ratios were also
measured because of the different positive and nega-tive ratios of the bags in the experimental datasets.
The definitions of the three measures are given in
Eqs. (3)–(5):
T T Accuracy ()/(),P N P N =++ (3) F Type I error /,P P = (4) F Type II error /.N N = (5) Here P and N denote the total number of positive and
negative bags respectively: P =P T +P F , N =N T +N F . They are derived from a confusion matrix as shown in Table 4.
6 Results and analysis
6.1 Comparison among MI methods
On each dataset, 5-fold cross validation (Kohavi, 1995) was executed to evaluate the performance of all
the selected MI learning algorithms above mentioned. Fig. 3 shows the prediction accuracy of all the MI learning methods on the datasets D 1–D 5.
The experiments indicated that the MI-kernel method always performed best in the prediction ac-curacy on all the five datasets (Fig. 3). Although compared to other MI learning algorithms, the MI-kernel method had a slightly better performance on D 2 and D 5, it significantly gave a best overall pre-diction (average 92.03% accuracy) on D 1–D 5 (Table 5). Moreover, the MI-kernel method also showed a competitive performance in terms of Type I (lowest) and Type II (second lowest) error ratios on D 1–D 5.
A close inspection of the detailed experiment results in Table 6 indicates that as the proportion of multi-class bags increased on D 1–D 3, the MI-kernel’s performance improved and attained the best result
(94.00% accuracy, with 5.56% Type I and 7.14%
Type II errors) on D 3 while other MI methods showed
no obvious improvement or even degraded.
As the ratios of the negative and positive bags in D 1, D 4, and D 5 tended to be balanced, some MI methods (MIOptimalBall, Citation-kNN, MI-EMDD, MI-ID3) had a decrease in prediction accuracy while the MI-kernel had stable discrimination accuracy. At the same time, the Type I and Type II error ratios of the MI-kernel were still at a relatively low level.
In general, the specific MI-kernel method has a solid performance concerning accuracy and Type I and Type II error ratios over all the datasets. This means that the MI-kernel method was effective and robust for the datasets with unbalanced distributions of bag labels or bag size in this study. Thus, this characteristic makes the MI-kernel method a poten-tially suitable approach in predicting the quality of large-scale and highly complex OO software projects.
Table 5 Average accuracy and Type I and Type II error
ratios over all datasets (MI methods)
Method Accuracy (%) Type I error (%) Type II error (%)
Citation-kNN 87.80 11.25 20.95 MI-SVM 87.77
11.49 21.06 MI-DD 84.09
11.54 36.19 MI-EMDD 87.14 14.49 13.97 MI-ID3 85.34 11.14 31.75 MI-BPN 89.06
10.81 16.24 MI-kernel 92.03 6.98 11.43 Table 4 A confusion matrix Matrix element Non-fault-prone (positive)** Fault-prone (negative)** Non-fault-prone (positive)* True positive, P T False positive, P F Fault-prone
(negative)*
False negative, N F True negative, N T * Actual; ** Predicted
A c c u r a c y (%)
Datasets
12345Fig. 3 Comparison of the prediction accuracy on D 1–D 5(MI methods)
Huang et al. / J Zhejiang Univ-Sci C (Comput & Electron) 2010 11(2):130-138 136
6.2 Comparison with a supervised SVM classifier
A supervised SVM learning algorithm with an RBF kernel was also evaluated on D1–D5 for com-parison. Since supervised learning algorithms cannot be applied directly to MI problems, different training and testing strategies were used. In the training phase, the supervised SVM was trained using labeled in-stances in the training bags. In the testing phase, the SVM classifier was utilized to predict the labels of every instance in the testing bags. Then the bag-labels were calculated using the predicted instance-labels according to Eq. (1). The parameters for this super-vised SVM were the same as those for MI-SVM and all instance-labels were also given.
Table 7 reveals that the MI-kernel method was a little less effective in the prediction accuracy than the supervised SVM. However, this supervised SVM requires the labels of every instance in the training set and indirectly predicts the bag-labels. Considering the cost of obtaining the labels, the MI learning algo-rithms such as the MI-kernel method are at least competitive if no better choices.
7 Conclusion and future work
This paper proposed a novel MI learning ap-proach for software quality estimation in OO systems. With an MI perspective, this approach attempted to apply an MI-kernel method to the fault-proneness prediction of OO software modules. In detail, the OO software quality estimation was considered as an MI problem by regarding each ‘class hierarchy’, which is a set of inheritance-related classes, as a bag and each class as an instance. The MI learning schema is suit-able for non-thoroughly tested or in-progress OO software projects for which it is hard and unnecessary to ascertain the fault-proneness of every relatively small module.
Several representative MI learning algorithms were evaluated on five datasets from an optical communication software project. The experimental results showed that the MI-kernel method performs better when compared to other MI learning ap-proaches in terms of overall predication accuracy and type I and type II error ratios, and was consistently efficient for the datasets with unbalanced size or label distribution. When compared to a supervised SVM learning algorithm in the same experiments, the MI-kernel method still had close and encouraging results with far fewer labels.
Table 6 Experimental results of different MI methods over all datasets
Accuracy (%)
Method
D1D2D3D4D5 MIOptimalBall 90.29 91.00 90.00 83.0078.00 Citation-kNN 92.00 88.00 90.00 83.0086.00 MI-SVM 90.86 82.00 90.00 84.0092.00
MI-DD 83.43 83.00 88.00 86.0080.00 MI-EMDD 89.71 90.00 86.00 88.0082.00 MI-ID3 89.71 83.00 88.00 84.0082.00 MI-BPN 90.29 84.00 90.00 89.0092.00 MI-kernel 93.14 91.00 94.00 90.0092.00
Type I error (%)
Method
D1D2D3D4D5 MIOptimalBall 9.46
10.98
13.89
16.4434.78 Citation-kNN 5.41 10.98 5.56 8.2226.09 MI-SVM 9.46 8.54 11.11 10.9617.39 MI-DD 8.11 8.54 11.11 8.2221.74 MI-EMDD 8.78 9.76 13.89 9.5930.74 MI-ID3 4.73 8.54 11.11 9.5921.74 MI-BPN 6.76
12.20
11.11
10.9613.04 MI-kernel 5.41 9.76 5.56 5.48 8.70
Type II error (%)
Method
D1D2D3D4D5 MIOptimalBall 11.11 0.00 0.00 18.5211.11 Citation-kNN 22.22 16.67 21.43 40.74 3.70 MI-SVM 7.41 61.11 7.14 29.63 0.00 MI-DD 62.96 55.56 14.29 29.6318.52 MI-EMDD 18.52 11.11 14.29 18.52 7.41 MI-ID3 40.74 55.56 14.29 33.3314.81 MI-BPN 25.93 33.33 7.14 11.11 3.70 MI-kernel 14.81 5.56 7.14 22.22 7.41Table 7 Comparison of experimental results of the MI-kernel method and a supervised SVM
Dataset Method
Accuracy
(%)
Type I
error (%)
Type II
error (%)
MI-kernel
1
SVM (supervised)95.43 2.03 18.52
MI-kernel 91.00 9.76 5.56
D2
SVM (supervised)93.00 3.66 22.22
MI-kernel 94.00 5.56 7.14
D3
SVM (supervised)94.00 0.00 21.43
MI-kernel 90.00 5.48 22.22 D4
SVM (supervised)91.00 6.85 14.81
MI-kernel 92.00 8.70 7.41
D5
SVM (supervised)92.00 4.35 11.11
Huang et al. / J Zhejiang Univ-Sci C (Comput & Electron) 2010 11(2):130-138137
In the presented work, the OO software quality estimation was limited to a standard MI form, which is relatively naive. That is, the non-fault-proneness class hierarchy must have no fault-prone class in-stance. In fact, this decision criterion may vary for different software releases; e.g., newly started pro-jects could have a higher threshold than the main-tained release. Future work may extend the standard MI model to a more generalized one (Weidmann et al., 2003). Moreover, other kernels for multi-instance or structured data may also be studied for the MI based quality estimation in OO software systems. References
Andrews, S., Tsochantaridis, I., Hofmann, T., 2003. Support Vector Machines for Multiple-Instance Learning. Proc.
15th Advances in Neural Information Processing Systems, p.561-568.
Auer, P., Ortner, R., 2004. A Boosting Approach to Multiple Instance Learning. Proc. 15th European Conf. on Ma-
chine Learning, p.63-74. [doi:10.1007/b100702]
Basili, V., Briand, L., Melo, W., 1996. A validation of object- oriented design metrics as quality indicators. IEEE Trans.
Software Eng., 22(10):751-761. [doi:10.1109/32.544352] Berard, E.V., 1998. Metrics for Object-Oriented Software Engineering. Available at http://www.ipipan.gda.pl/ ~marek/objects/TOA/moose.html [Accessed on Dec. 10,
2009].
Briand, L., Wust, J., Daly, J., Victor, P.D., 2000. Exploring the relationships between design measures and software quality in object-oriented systems. J. Syst. Software,
51(3):245-273. [doi:10.1016/S0164-1212(99)00102-8] Cartwright, M., Shepperd, M., 2000. An empirical investiga-tion of an object-oriented software system. IEEE Trans.
Software Eng., 26(8):786-796. [doi:10.1109/32.879814] Catal, C., Diri, B., 2008. A fault prediction model with limited fault data to improve test process. LNCS, 5089:244-257.
[doi:10.1007/978-3-540-69566-0_21]
Chen, Y., Bi, J., Wang, J.Z., 2006. MILES: multiple-instance learning via embedded instance selection. IEEE Trans.
Pattern Anal. Mach. Intell., 28(12):1931-1947. [doi:10.
1109/TPAMI.2006.248]
Chevaleyre, Y., Zucker, J.D., 2001. Solving multiple-instance and multiple-part learning problems with decision trees
and decision rules. LNCS, 2056:204-214. [doi:10.1007/
3-540-45153-6]
Chidamber, S., Kemerer, C., 1994. A metrics suite for object- oriented design. IEEE Trans. Software Eng., 20(6):
476-493. [doi:10.1109/32.295895]
Dietterich, T.G., Lathrop, R.H., Lozano-Pérez, T., 1997.
Solving the multiple instance problem with axis-parallel
rectangles. Artif. Intell., 89(1-2):31-71. [doi:10.1016/
S0004-3702(96)00034-3]
Driessens, K., Reutemann, P., Pfahringer, B., Leschi, C., 2006.
Using weighted nearest neighbor to benefit from unla-
beled data. LNCS, 3918:60-69. [doi:10.1007/11731139] Elish, K.O., Elish, M.O., 2008. Predicting defect-prone soft-ware modules using support vector machines. J. Syst.
Software, 81(5):649-660. [doi:10.1016/j.jss.2007.07.040] Evett, M., Khoshgoftar, T., Chien, P.D., Allen, E., 1998.
GP-Based Software Quality Prediction. Proc. 3rd Annual Genetic Programming Conf., p.60-65.
Fenton, N., Krause, P., Neil, M., 2002. Software measurement: uncertainty and causal modeling. Software,19(4):116- 122. [doi:10.1109/MS.2002.1020298]
Gartner, T., Flach, P.A., Kowalczyk, A., Smola, A.J., 2002.
Multi-Instance Kernels. Proc. 19th Int. Conf. on Machine Learning, p.179-186.
Guo, L., Ma, Y., Cukic, B., Singh, H., 2004. Robust Prediction of Fault-Proneness by Random Forests. Proc. 15th Int.
Symp. on Software Reliability Engineering, p.417-428.
[doi:10.1109/ISSRE.2004.35]
Huang, P., Zhu, J., 2008. Predicting the fault-proneness of class hierarchy in object-oriented software using a layered kernel. J. Zhejiang Univ. Sci. A, 9(10):1390-1397. [doi:10.
1631/jzus.A0720073]
Huang, S.J., Lin, C.Y., Chiu, N.H., 2006. Fuzzy decision tree approach for embedding risk assessment information into software cost estimation model. J. Inf. Sci. Eng., 22(2): 297-313.
Kanmani, S., Uthariaraj, V.R., Sankaranarayanan, V., 2007.
Object-oriented software fault prediction using neural networks. Inf. Software Technol., 49(5):483-492. [doi:10.
1016/j.infsof.2006.07.005]
Khoshgoftaar, T.M., Allen, E.B., Hudepohl, J.P., Aud, S.J., 1997. Application of neural networks to software quality modeling of a very large telecommunications systems.
IEEE Trans. Neur. Networks, 8(4):902-909. [doi:10.1109/
72.595888]
Khoshgoftaar, T.M., Allen, E.B., Deng, J., 2002. Using re-gression trees to classify fault-prone software modules.
IEEE Trans. Rel., 51(4):455-462. [doi:10.1109/TR.2002.
804488]
Kohavi, R., 1995. A Study of Cross-Validation and Bootstrap for Accuracy Estimation and Model Selection. Proc. 4th Int. Joint Conf. on Artificial Intelligence, p.1137-1143. Maron, O., Lozano-Pérez, T., 1998. A Framework for Multiple Instance Learning. Proc. 10th Advances in Neural In-
formation Processing Systems, p.570-576.
Reformat, M., Pedrycz, W., Pizzi, N.J., 2003. Software quality analysis with the use of computational intelligence. Inf.
Software Technol., 45(7):405-417. [doi:10.1016/S0950- 5849(03)00012-0]
Ruffo, G., 2000. Learning Single and Multiple Instance Deci-sion Trees for Computer Security Applications. PhD Thesis, Department of Computer Science, University of Turin, Torino, Italy, p.425-432.
Seliya, N., Khoshgoftaar, T.M., 2007. Software quality esti-mation with limited fault data: a semi-supervised learning perspective. Software Qual. J., 15(3):327-344. [doi:10.
1007/s11219-007-9013-8]
Huang et al. / J Zhejiang Univ-Sci C (Comput & Electron) 2010 11(2):130-138 138
Tang, M.H., Kao, M.H., Chen, M.H., 1999. An Empirical Study on Object Oriented Metrics. Proc. 6th Int. Conf. on Software Metrics Symp., p.242-249.
Vishwanathan, S.V.N., Smola, A.J., Murty, M.N., 2003. Sim-ple SVM. Proc. 20th Int. Conf. on Machine Learning, p.760-767.
Wang, J., Zucker, J.D., 2000. Solving Multiple-Instance Problem: A Lazy Learning Approach. Proc. 17th Int. Conf.
on Machine Learning, p.1119-1125.
Weidmann, N., Frank, E., Pfahringer, B., 2003. A Two-level Learning Method for Generalized Multi-Instance Prob-
lem. Proc. European Conf. on Machine Learning,p.468- 479. [doi:10.1007/b13633] Zhang, M.L., Zhou, Z.H., 2004. Improve multi-instance neural networks through feature selection. Neur. Process. Lett., 19(1):1-10. [doi:10.1023/B:NEPL.0000016836.03614.9f] Zhang, Q., Goldman, S.A., 2001. EM-DD: An Improved Multiple-Instance Learning Technique. Proc. 14th Ad-
vances in Neural Information Processing Systems, p.1073-1080.
Zhou, Z.H., Zhang, M.L., 2006. Multi-Instance Multi-Label Learning with Application to Scene Classification. Proc.
Advances in Neural Information Processing Systems, p.1609-1616.
Zhou, Z.H., Jiang, K., Li, M., 2005. Multi-instance learning based Web mining. Appl. Intell., 22(2):135-147. [doi:10.
1007/s10489-005-5602-z]
日语省略音、音便解释
日语省略音、音便解释 ◆在口语中,接在「て」之后所出现的「い」音常会被省略掉。如:原文口语表现 ~ていないで→ ~てないで ~ている→ ~てる ~ていられない→ ~てられない ~ていく→ ~てく 聞いていれば→ 聞いてれば もっていったら→ もってったら ◆在口语中所出现的「ん」音。 1. ら行常会音便成「ん」音。如: 原文口语表现 信じられない→ 信じらんない てる→ てん ふぎけるな→ ふぎけんな なってるの→ なってんの 分からなくて→分かんなくて *「の」之前所接若为「る」时,此时的「る」通常会发「ん」音。 2. 「の」的口语形式也常会发「ん」音。如: 原文口语表现 ~のだ→ ~んだ ~ので→ ~んで もの→ もん ◆口语简略形ちゃ/じゃ 原文口语表现 ~てはいけない→ ちゃいけない ~ではいけない→ じゃいけない ~ではない→ じゃない ◆口语简略形ちゃ/きゃ 原文口语表现 ~なくてはいけない→ なくちゃいけない ~なければならない→ なきゃならない ◆口语简略形りゃ 原文口语表现 これは→ こりゃ それは→ そりゃ あれは→ ありゃ すれば→ すりゃ
聞いていれば→ 聞いてりゃ ◆形容词~くって—其后省略了「たまらない/非常」。为口语强调形。如:忙しくてたまらない→ 忙しくって/忙死了。 美味しくてたまらない→ おいしくって/太好吃了。 ◆って:[副助词] 1. 提示话题。有「というのは」、「というものは」的意思。例: *見習いというのは大変だね。 →見習いって大変だね。 /当学徒很辛苦吧! 2. 表达听来的传言或他人的想法。 *あの人、首になったという話よ。 →あの人、首になったんだってよ。 /听说他被炒鱿鱼了! *川瀬さんが日本に行くと聞いたよ。 →川瀬さんが日本に行くんだってよ。 /听说川濑先生要去日本喔! *美味しくないと思ってるんだよ。 →美味しくないって思ってるんだよ。 /他觉得不好吃! 3. 表示指定的内容,有「~という」、「~といって」、「~ということ」的意思。 *トムさんという人はどこの国の人? →トムさんって人はどこの国の人? /Tom是哪一国人啊? *ちょっと行ってくるといって出掛けたよ。 →ちょっと言ってくるって出掛けたよ。 /他说我出去一下就出门了。 *友達と約束してたということ、忘れてた。 →友達と約束だったって忘れてた。 /我忘了和朋友有约。 4. 重复对方问话。 *誰かって言われても知らないよ。 /你问我他是谁,我不知道啊。 *花子ちゃんの前で花子ちゃんのこと好きかって聞かれても答えられるわけないだろう。 /在花子面前问你喜欢她吗,你不好回答吧! 5. 「って」放在句尾,可当终助词,无意义,用来加强语气。 *あの人を見た覚えはないって。 /我不记得见过那个人。 ◆とく 「とく」是「ておく/预先……、保持某种状态」的口语简略形。
双引号、省略号、破折号的作用
双引号、省略号、破折号的作用省略号的作用:①表引文或引述的话有所省略;②表重复词语的省略;③表列举同类事物和序数词语的省略;④表静默或思考;⑤表说话断断续续;⑥表语言的中断;⑦表话未说完,语意未尽。 破折号的作用:1.表示解释说明 2.表示语音的延长3.表示意思的转换,跳跃或转折 4.表示插说 5.电脑应用 6.加强重点7.分行举例8.用在副标题前9.标明作者10.补充说明11.表示总结上文12.表示语言的延续 引号的作用:a.表示引语b.表示特定称谓c.表示特殊含义需要强调d.表示否定和讽刺 引号最基本的用法是引用,引用包括直接引用和间接引用.直接引用是把人所说的话完整地记录下来;间接引用是把某人的原话非正式地、不完整地、随语意拈来放在自己的话中的引用.这类引述如果不作明白交代,就很难发现它是引用。引号除了引用外,还具有强调的作用。
省略号作用(……) 1.表示引文或引述的话里有所省略.例如: ①就在那样不平凡的时刻,在那个可纪念的地方,我第一次听见唱:二月里来,好风光,家家户户种田忙.…… ②听筒里传来十分响亮而焦灼的声音:“时间来不及了,请马上设法空运……空运!” 2.表示重复词语的省略.例如: 孔乙己低声说道:"跌断,跌、跌……,他的脸色,很象恳求掌柜,不要再提. 3.表示列举同类事物和序数词语的省略.例如: (场长)介绍给我们看董昆他们打的野物皮子:狐狸、貉子、獾、水獭、野猫……种类实在不少. 4.表示静默或思考.例如: 何为:不行!梅伯母的身体已经经不起路上的颠簸了! 欧阳平:…… 5.表示说话断断续续.例如: 他……直指着正北方向,“好,好同志……你……你把它带给……” 6.表示语言的中断.例如: ①“我并没有阔哩,我须卖了这些,再去……” ②“啊呀呀,你放了道台了,还说不阔?你现在有三房姨太太;
英语语法中的省略现象
外教一对一https://www.360docs.net/doc/f2945479.html, 英语语法中的省略现象 并列复合句中的省略 在并列句中后边的分句可以省略与前边分句中相同的成分。如: a) The boy picked up a coin in the road and (the boy ) handed it to a policeman. 这个男孩在马路上拾起一枚硬币并把他交给了警察。 b) Your advice made me happy but(your advice made) Tom angry .你的建议使我高兴但使汤姆生气。 c) Tom must have been playing basketball and Mary (must have been)doing her homework. 汤姆肯定一直在打篮球,玛丽一直在写作业。 d) Gao Xiumin was born in 1959 and Fu Biao (was born) in 1963.高秀敏出生于 1959 年,傅彪出生于 1963 年。 其他一些省略结构 1.名词所有格修饰的名词,若表示住宅、店铺、教堂或上下文已暗示或明确指出过的事物时,常常可以省略。如: We spent the weekend at the Mary's. 我们在玛丽家过的周末。 2.What 和 how 引导的感叹句中,常可省略主语 it 和 be 动词如: a) What a wonderful victory (it is ) for Tom ! 这对 Tom 来说是个多么大的胜利呀! b) How beautiful (it is ) to be treated like a normal child. 被当作一个正常孩子对待对他而言是多么美妙的一件事呀。
谈英汉翻译技巧中的增补与省略
谈英汉翻译技巧中的增补与省略 【摘要】增补法是英汉翻译中常用的方法之一。所谓增补,就是为了使译文准确和符合汉语习惯,译者应该添加必要的词句。所谓省略法,就是将不必译的冠词、物主代词、连系动词等省略不译。 【关键词】翻译技巧增补法省略法 英汉翻译是英语学习中最基本的技能之一,在今天的飞速发展的世界中越来越显示出它的重要性。一篇好的译文应该是既忠实于原文,又符合汉语的用法习惯,并且在内容与形式上与原文达到辩证的统一。忠实于原文是翻译中最重要的一点。但由于英汉两种语言的巨大差异,“忠实”的涵义并不是逐字逐句地照搬原文,而是“忠实地表达”原作者的思想,准确地理解和恰当地表现原文。因此,译者必须在理解原文的基础上,运用恰当的翻译技巧,将原文译出。 英汉翻译技巧一般来说有8种,即“分清主从”,“选词用字”,“增补法”,“省略法”,“词性转换”,“词序调整”,“正说反译和反说正译”以及“长句拆译”。本文侧重谈谈英汉翻译技巧中的增补与省略这两种方法。 1.增补法 增补法是英汉翻译中常用的方法之一。增补不是给原文增加某些内容,而只是翻译中的一个必要的规则。它实际上主张,为了使译文准确和符合汉语习惯,译者应该添加必要的词句。增补法在下面几种情况下经常使用: A.当某些词在英语的句子中被省略时 例如:We can get rid of a bad style and keep the good. 虽然,在good 一词后省略了style. 这句可译成:我们能够去掉不良作风,保持优良作风。 He majors in English and l in French. 在I 后面省略了动词major. 这句应译成:他主修英语,我主修法语。 在这类句子中,原文省略的部分应在译文中写出来。 B. 当英文中用代词来避免重复时
高考英语语法专项突破训练专题16-倒装句和省略句
专题十六倒装句和省略句重难点分析 一、倒装句 倒装句主要考查以下几个方面: ◆含有否定意味的词置于句首,用部分倒装; ◆“only+状语/状语从句”置于句首,用部分倒装; ◆so/such…that句型中,“so+形容词/副词”提前,用部分倒装; ◆表示方位的副词或介词短语放在句首,要用完全倒装。 1. 倒装句用法一览表:
2. 特别提示 (1) there be结构的倒装句型中,除了be动词之外,there后还可接lie, live, seem to be等。如:There lived an old man in the village long long ago. 很久很久以前,村子里住着一位老人。 (2) here, there, now, then, up, down, away, out等副词位于句首,主语为代词时不到装。如:Away it flew. 它飞走了。 (3) 直接引语的一部分或全部位于句首,主句倒装,主句倒装,但是主语为代词时不倒装。如:“You have to finish it tonight,” she said. “今晚你必须完成它,”她说。 (4) so位于句首不倒装的情况:
① 主语与前句相同,表赞同,译为“确实如此”。如: — Mike studies hard. 迈克学习很刻苦。 — So he does. 确实是。 ② 表示前句内容也适用于另外的人或事,前句如果列举了两种事实以上,用“so it is/was with sb./sth.”回答。如: — Tom is kind and often helps those in trouble. 汤姆很友好,经常帮助处于困境中的人。 — So it is with his father. 他父亲也是。 (5) 使用as/though进行倒装时注意: ①句首有名词,名词不能带任何冠词。如:Child as he is, he can tell right from wrong. 尽管他是个孩子,却能够明辨是非。 ②句首是实义动词时,其他助动词则放在主语之后。如果实义动词有宾语和状语,则宾语和状语随实义动词一起放在主语之前。如: Try hard as he will, he never seems to be able to do the work satisfactorily. 虽然他尽力了,但他的工作总做得不尽如人意。 (6) not until位于句首的倒装结构中,如果是复合句,只有主句用倒装,从句不用。如: Not until he loses his health will he give up smoking. 他直到失去健康才会戒烟。 二、省略句 高考命题导向:省略是一种避免重复,保持简洁的语法手段。缺少一个或一个以上的必要语言成分,但在一定语境中能够独立存在,意义明确,并且能发挥交际功能的句子叫作省略句。高考主要是考查省略在固定结构中的运用。 省略句用法一览表:
日语中の的类型和用法的用法学习资料
一、「の」的类型和用法 领格助词 接续方法:体言+の+体言 前面的[体言和の]一起形成定语,作后面体言的连体形修饰语。 例如:「私は日本语の教师です。」 这里「日本语」是体言,与领格助词「の」一起构成定语,来修饰「教师」。 全句译成:“我是日语(的)教师。” 主格助词--作定语句的主语 接续方法:体言+の+谓语+体言 前面的[体言+の+谓语]形成句子,构成后面体言的定语。这里要注意:句子要以连体形出 例如:「ここは私の勉强している大学です。」 这里「私の勉强している」是定语句,是用来修饰「大学」的。定语句中「私」是「勉强している」的主语。这时主语后面可加「の」和「が」,而不能加「は」。 「ここは私が勉强している大学です。」(正确) 「ここは私は勉强している大学です。」(错误) 「勉强している」是连体形,不过和基本形是一样的。 全句译成:“这里是我学习的大学。” 又如:「器の奇丽な料理は食欲が出る。」 这里「器の奇丽な」是描写句「器が奇丽だ」作定语句时的变化,作「料理」的定语。这
里「器」是句子的主语,「奇丽だ」是谓语。当它作定语时,主语用「の」或「が」表示,「奇丽だ」变成连体形「奇丽な」。 整个句子译成“器皿好看的菜能引起食欲。” 同位语 接续方法:体言+の+体言 从接续方法上看和领格助词一样,但作同位语时,在「の」前后的体言必须表达同一事物。例如:「留学生の山田さんは今上海にいます。」 这里的「留学生」和「山田さん」是一个人,之间的「の」就是表示前后是个同位关系。全句译成:“留学生山田先生现在在上海。” (1)在日语句中,主语、宾语、补语等都要求是体言,如果不是体言的用言或句子作这些 例如:「私が勉强しているのはこの学校です。」 这里的「の」代表了「私が勉强している」的地方,在句子中是主语。 全句译成:“我学习的地方是这个学校。” 又如:「借りた本は全部返さないといけないと言うので、私は借りたのを全部返しました。」 这里的「の」代表了我借的书,在句子中是宾语。 全句译成:“说是必须全部还清借的书,所以我把我借的(书)全部还掉了。”
省略号和破折号的作用和例句
省略号的作用和例句 省略的内容主要有四:一是引文;二是重复性词语;三是类似语句;四是列举。 除省略外,省略号还有以下几种用法: 1、表示沉默: 例:何为:梅伯母的身体已经经不起路上的颠簸了!欧阳平:…… 2、表语言中断: 例:穿长袍的问:"这位是……""我的兄弟。"戴礼帽的回答。 破折号也可表语言中断,但两者有不同:破折号表语言戛然而止,省略号表余音未尽。3、表语言断断续续。 例:上级……派我……到这儿……工作,我没……有……完成……上级……交给我的……任务。 4、表含糊其辞,欲言又止 例:我即刻胆怯了起来,便想全翻过先前的话来,"那是……实在,……我说不清……。其实,究竟有没有魂灵,我也说不清。" 5、表明语意未尽。 例:"可恶!然而……。"四叔说。 使用省略号时应注意以下问题: (1)不可滥用省略号。一是省略号后语境不明的不能用省略号,而是没有上述特殊语意不能用省略号。 (2)、用了"等"、"等等"就不能再用省略号。(省略号和"等"、"等等"有不同,省略号表示的可以是各种需要省略的,而后者只能是类似的词语或意思。) (3)要正确处理省略号前后的点号问题:一是省略号前是个完整的句子的,省略号前应使用句末标点,表示省略的是句子;二是省略号前不是完整句子的,省略号前一般不用点号,表示省略的是词语、短语;三是省略号后一般不用标点。 (4)、如果省略的是一大段或几段文字,就用十二个圆点表示,单独成行,不顶格。 破折号的作用和例句 破折号是一个用途比较广的标号。它的主要用法可以归纳如下。 1. 用以表示对上文的解释说明或补充。例如:“亚洲大陆有世界上最高的山系——喜玛拉雅山,有目前地球上最高的山峰——珠穆朗玛峰。” 括号也表示解释说明,但是破折号引出的解释说明是正文的一部分,是较重要的信息,要读出来的。而括号里的解释说明不是正文,只是注释,没有它不影响句子的完整性,读时也不必连着正文念出来。 解释说明或补充的语句如果是插在句子中间的,可以前后各用一个破折号。例如:“太阳系除了九个大行星——水星、金星、地球、火星、木星、土星、天王星、海王星和冥王星——以外,还有40个卫星以及为数众多的小行星、彗星和流星体等。”“直觉——尽管它并不总是可靠的——告诉我,这事可能出了什么问题。” 2. 表示话题的转变。例如:“我在珠海的公司干得挺顺心。老板对我不错,工资也挺高,每月三千多呢!——我能抽支烟吗?”破折号前是讲述自己情况, 后面突然转而询问对方别的事。 3. 表示话语的中断。例如:“…班长他牺——?小马话没说完就大哭起来。”“他很大了,——(顿)并且他以为他母亲早就死了的。”前一例的破折号表示下面的话没说出来。后一例表示说了半句暂时中断,停顿了一下又接着说下去。 4. 表示声音的延长。例如:“…顺——山——倒——?林子里传出我们伐木连小伙子的喊声。” 5. 表示引出下文。例如:“在一篇题为《论气节》的文章里,他对…五四?以来中国知识分子
英语中省略现象
高中英语语法之省略 英语中省略现象较为普遍,对省略的考查已成为高考中的热点。句子成分的省略,可分为以下几种情况: 为了使话说得简明扼要,英语句子中某个单词、短语甚至从句或主句都可以省去。Ⅰ、状语从句中的省略用法 一、如果从句的主语和主句的主语一致,且从句的谓语含有be动词的某种形式 (am/is/are/was/were),可同时省略从句的主语和be动词的某种形式。 1、when,while引导的时间状语从句 e.g. Do be careful when (you are) crossing the street. When/While (I was) on my way to work, I met her. 2、if,unless,once引导的条件状语从句 e.g. If (it is) properly treated, waste will do no harm to the environment. I’ll not go to the party unless (I am) invited. Once (you are) caught stealing in a supermarket, you will be punished. 3、though,although,whether,no matter whether/what/how/who等引导的 让步状语从句 e.g. He was happy, though/although (he was) poor. Whether (she is) sick or well, she is always cheerful. No matter how/However hard the task (is), we must fulfill it in time. (注:从句的主语和主句的主语不一致时,只省略从句中的be动词形式) 4、as if,as though引导的方式状语从句 e.g. He rubbed his eyes and yawned as if/though (he was) waking up after a long sleep. He stood up as if/though (he wanted) to leave.(as if/though + to do表示一个将来的动作) 二、than,as引导的比较状语从句中的省略用法:当不同的主语进行比较时, 一般省略从句中的谓语;当从句中的主语与谓语(be动词除外)和主句中的主语与谓语相同时,通常省略从句中的主语和谓语,只保留比较部分。 e.g. He is taller than his brother (is).
英语语法省略句
英语语法省略句 省略句 1)不定式在love, mean, want, like, wish, expect, try, intend, plan, refuse, prefer, seem等动词后面 2)在happy,glad,eager,ready,willing等形容词后面。 1.---will you join us? --- I should love to(join you). 2.I asked him to see the film, but he didn’t want to(s ee the film). 3.--- Would you like to go with us? --- Yes, I’m glad to(go with you) 4.He doesn’t get up early as he used to. (get up) 注意: 如果不定式中含有be, have(助动词), have been, 通常保留be, have(助动词), have been. 1.--- Are you a sailor? --- No, but I used to be. 2.---He hasn’t finished yet. ---well, he ought to have. 1. —I'll be away on a business trip. Would you mind looking after my cat? —Not at all, ________ . (NMET 1995) A. I have no time B. I'd rather not C. I‘d like it D. I'd be happy to 2. —Does your brother intend to study German? —Yes, he intends ________ . (NMET 1998上海卷)
英语倒装和省略
1、Not until the early years of the 19th century _________what heat is. A、man did know B、man knew C、didn’t man know D、did man know 【解题分析】not until意为“直到……才”,后面的句子要用倒装语序。句意为:“直到19世纪早期,人们才认识到热是什么。” 2、Mary doesn’t speak French, and ____________ does John. A、either B、so C、no D、neithe 【解题分析】neither用在句首表示“……也不”时,句子用倒装语序。正确答案:D 3、On the wall _______________some famous paintings. A、am B、is C、are D、be 【解题分析】[正确答案:C句意:墙上有一些著名的油画。在主谓倒装的句子中,动词的单复式形式应和它后面的主语在数上保持一致。 4、—Did you finish doing your homework in time? —I am afraid _______. A、doing B、so C、no D、not 【解题分析】正确答案:D I am afraid not表示“恐怕不能”的意思。 5、If you go to the park tomorrow morning, _________. A、so will he B、so he will C、so he does D、so does he 【解题分析】正确答案:A这是—个含有条件状语从句的主从复合句,条件状语从句中谓语用—般现在时,主句的谓语常用—般将来时,故C和D被排除。主句所表示的意义是“他也将去。”因此,主句的主语和if条件状语从句中的主语所做的事—致,主谓要倒装。 6、—Hello, Zhu Hua. I’ll have to return to Canada because I’ve worked here for a year. —__________! A、What time flies B、How time flies C、What does time fly D、How does time fly 【解题分析】正确答案:B how time flies=how fast time flies“时间过得真快”。因为被感叹的部分是副词fast,因此感叹词用how,感叹句需用陈述语序。 7、Never before that night _________ the extent of my own power. A、had I felt B、I felt C、did I feel D、I had felt 【解题分析】正确答案:A. never before that…意为“直到……才……”,常与完成时态连用。never放在句首时,后面的语序要部分倒装。句意为:“直到那天晚上我才意识到我的权力范围。” 8、During the war, __________but also he lost his wife and his child A、not was his job in the lab taken away B、not only was his job in the lab taken away C、not merely his job in the lab was taken away D、not just was taken away his job in the lab 【解题分析】正确答案:B not only. . . but also连接两个并列分句,且not only位于句首时,该分句主谓部分倒装。 9、—I would never come to this restaurant again. The food is terrible. —____________. A、Same with me B、So do I C、Nor am I D、Neither would I 【解题分析】正确答案:D neither用在句首表示“……也不”时,句子用倒装语序;而且要使用与上文相同的助动词。 10、Hardly had she walked out of the woods __________ she heard the strange scream coming from behind a tree. A、than B、until C、since D、when 【解题分析】正确答案:D hardly. . . when. . . 表示“一……就”。注意,主句主谓要用部分倒装。 11、Little _______________ that the district was very rich in resources. A、we suspected B、we did suspect C、did we suspect D、do we suspect 【解题分析】正确答案:C little意为“很少,几乎没有”放在句首表示强调时,其后的语序要用倒装。句意为:“我们从未怀疑过这个地区资源很丰富。” 12、My cousin read a history book. _______. A、So John does B、So did John C、So does John D、So John did 【解题分析】正确答案:B此句为—般过去时,应用助动词did完成,故选B。 13、Only when one is away from home ____________ how nice home is. A、he realizes B、does he realizes C、does he realize D、he will realize 【解题分析】正确答案:C only用在句首修饰状语时句子倒装。 14、He is strict in everything and strict with everyone. __________. A、My father is always such B、My father is always so a strict man C、Such is my father D、So a strict man is my father 【解题分析】正确答案:C 当such作句子的表语,位于句首代替上文情况时,句子通常用倒装语序。 15、—You seem to like sweets. —________. A、So do I B、So I do C、So am I D、So I am 【解题分析】正确答案:B本题考查句型“So+主语+助动词”与“So+助动词+主语”的区别。—些考生对这两种结构混淆不清,以致误选:“So+主语+助动词”作“的确如此”解,是对上文所言之事的进—步肯定,本题译作“你好像喜欢吃糖”,“我就是这样”。故选B。而“So+助动词+(另—个)主语”作“…也是如此”解,表示上文所言同样适合另—个人或物,如:I like sweets, and so does Lucy. 我喜欢吃糖,露西也是如此。 16、Li Xin thinks Yao Ming is becoming the most famous basketball player in the world. _________.
破折号、省略号的作用
破折号得作用 “——”,破折号就是小学课文中常见得标点符号,它一般有以下几种用法: 1、表示破折号后面就是解释说明得部分。 (1)各国政府——无论专制政府或共与政府都驱逐她。 (2)带工老板或者打杂得拿着一叠叠得名册,懒散地站在正门口——好像火车站剪票处一般得木栅子前面2、表示意思得递进。 每年——特别就是水灾、旱灾得时候,这些在日本厂里有门路得带工…… 3、表示意思得转换、跳跃或转折。 (1)“今天好热啊!——您什么时候去上海?” (2)我本来不想去,可就是俺婆婆非叫我再去瞧瞧她——有什么瞧头啊! 4、表示语音得延长 (1)“小林——,我来了!”她大喊着 (2)“呜——呜——呜”小男孩大声哭起来。 (3)我们在天安门前深情得呼唤:周——总——理——5、表示语音较大得停顿或中断。 那个时候在无锡得人,我倒问过,可就是——(表示说话中断) 人教版六年级上册破折号汇总
1、先就是听见它得声音从,从很远得山林里传来,从很高得山坡山传来——沙啦啦,沙啦啦……( 1、表示破折号后面就是解释说明得部分。) 2、来得突然——跟着一阵阵湿润得山风,跟着一缕缕轻盈得云雾,鱼,悄悄地来了。( 1、表示破折号后面就是解释说明得部分。) 3、丁——冬——丁——冬……( 4、表示语音得延长) 4、它们散居在两棵大树下面——这就是两簇野灌丛……(1、表示破折号后面就是解释说明得部分。) 5、这样得“路”,还可以过汽车——汽车吼叫着,车身摇晃着,卵石挤碰着…… (1、表示破折号后面就是解释说明得部分。) 6、孩子虽然不多——只有两个,可就是全靠她一个人张罗。( 1、表示破折号后面就是解释说明得部分。) 7、我瞧见一堆焦黄得马草——这些日子我卖给她所有得马草。( 1、表示破折号后面就是解释说明得部分。) 8、她就是一位老太太,非常瘦,满头白发,不过——她就是聋子。( 4、表示语音得延长) 9、巴拉那河上有一条世界著名得大瀑布——赛特凯达
公示语翻译中的“情景省略”
公示语翻译中的“情景省略” 摘要:每个公示语都是一个完整的语篇,意义很大程度上依靠特定情景语境来传达。公示语的翻译应该追求情景语境的对等,而非形式的对等,而情景语境的对等主要是通过不同形式的“情景省略”来实现的。 关键词:公示语;翻译;情景语境 一、前言 公示语是在公共场合给特定人群观看,以达到某种特定交际目的的特殊应用文体。社会公示语的范围很广,包括公共场所的宣传标语、指示牌、告示语和旅游景点的介绍等,在对外宣传中发挥着不容忽视的作用。和普通文本相比,公示语有一个明显的特点是每个公示语虽然简短,但都是一个完整的语篇,而语篇的实现意义很大程度上依赖于情景语境,信息主要依靠特定情境或场合来传达,语篇功能也主要依靠情景语境来实现,不一定要体现在字面上。这种基于情景语境的省略简称为“情景省略”,在公示语翻译中非常普遍,也是公示语翻译的一大特色,但却没有专文探讨。而语篇语言学中的情景语境理论,对解释公示语翻译中的“情景省略”有参考价值。 二、情景语境理论 “情景语境”最早是马林诺夫斯基(malinowski)提出来的。马林诺夫斯基的语境包括文化语境和情景语境两大类。马氏所说的
“文化语境”是指说话者生活的社会文化环境;而“情景语境”是指说话时实际发生的事情,即语言发生的具体情境。弗斯(firth)对语境因素进行了总结,将“语境”分成由语言内因素构成的语境和由语言外因素(即参加者、事物、事件、言语效果)构成的语境。这种理论主张直接影响了韩礼德(halliday),成为系统功能语法的理论基石。韩礼德将情景因素归纳为三种:语场、语旨和语式,也即语域的三个变项,这三个变项决定了具体的语言形式。语场包括了谈话的话题和场地等情境因素;语旨指参与讲话者之间的角色关系,包括他们的社会地位,以及说话者的态度和想要实施的意图;语式指的是语言活动的媒介或渠道,分为口头语和书面语。语域的这三个变量可以用来预测在特定情景语境中所使用的具体词汇和 语法类型,即什么样的情景语境因素决定什么样的语言形式。情景语境因素,即语域,不仅影响着语言的使用,也制约着翻译策略的选择和译文的质量。和普通文本相比,公示语也有三个层次的语境,但公示语有一个明显的特点,那就是对情景语境的依赖性,信息很大程度上依靠交际的参与者、时间、地点、话题、参与者的身份等特定情境因素来传达,不一定体现在字面上,因此公示语有清晰明确、通俗易懂的效果。翻译过程中译者应恰当再现原文赖以产生的语场、语式、语旨,使译文与原文在情景语境的层次上趋于一致。在这一过程中,经常需要根据情景语境进行适当省略,即“情景省略”。
2019小升初英语语法省略句知识点:空缺与增补.doc
2019 小升初英语语法省略句知识点:空缺与增补 小升初英语语法省略句知识点:空缺与增补 两个主语不同的句子,谓语有相同部分,也有不同部分,如果用 连词连接起来实行对比,则其相同部分在后面分句中能够省略,这样,后 面分句中就出现“空缺”现象。“空缺” 处通常被省略的是动词, 或动词及其宾语、补足语等。汉译时不妨采用“补齐”的方法。例如: A sound must be heard ,a colour seen ,a flavour tasted , an odour inhaled.,颜色必须目视,滋味必须口尝,气味必须鼻吸。( 后三句省略 must be) Histories make men wise;mathematic logic and rhetoric able to contend. ;数学使人精细;逻辑和修辞 使人善辩。( 原文后两句省略make men) 注意 than 引出的比较从句中,套有 when引导的时间从句或 if 引导的条 件从句,而且该比较从句中的省略部分与整个句子的主要结构一致时则 比较从句的省略部分可省略不译。例如: My uncle is better than when I wrote to 我伯父的身体比我上 次给你去信时好些了(...than 后面省略了he was) 灵活使用 对英语中一些特殊省略结构译法需灵活,指的是按正常语法规则 分析,有的无法增补,有的要用不同的方式来增补,使之成为完全句。 所以,汉译英时,视上下文加以灵活处理。 1.“宾语 +主语 +谓语 and + 谓语”结构 其中 and 连接的,是用作不同成分的同一个词,这个词既是第一分 句的宾语,又是第二分句的主语。此结构的特点是,把宾语提到句
倒装句难点
倒装句难点:关于复合句和并列句的部分倒装所在的位置 掌握部分倒装并不难,从此再也不必为纠结到底是“前倒后不倒”或“后倒前不倒”而抓破头皮啦。 确定倒装位置紧抓两点:1 主句所在的位置需倒装 2 否定所在的句子需倒装 主从复合句中,主句需部分倒装,例: No sooner had I reached home than the phone began to ring. So fast did he walk that I couldn’t catch up with him. Not until she saw the present did she feel happy. 并列句中,否定所在的句子需部分倒装,例: Not only do I know her,but I am also her friend. Neither have I seen him recently, and nor have I heard from him.(两个并列分句中都有否定词,故两个分句都需进行部分倒装) 没有了传统的语法选择题,倒装句真的在高考中毫无价值吗?肯定不会毫无价值,反之倒装句一定可以在高考中为我们加分。即使语法填空不考倒装,我们也可以将倒装句运用在写作中,为我们的写作加分。 除了not only…but also…的倒装句我们还有其它选择吗? 在实际的写作阅卷中,不难发现学生所用倒装句型中,使用频率最高的非not only…but also…莫属了。 Not only should we pay more attention to the balanced diets, but also we ought to regularly do exercises. 除了only in this can we…的倒装句我们还有其它选择吗? 除了以上提及的not only…but also…句型,在写作阅卷中另一种常见的倒装句就是Only+状语/状语从句置前的倒装句。 Only in this way can we protect our environment. Only when we are faced with ever-increasing pollutions, would we place more emphasis on environment protection. 要想在写作中凭借倒装句为自己的文章增加亮点,以上两个句型未免有些捉襟见肘。如何升级我们的倒装句式,让我们的倒装句从屌丝型一跃为高富帅呢?如何能够让我们能得心应手地在任何语境下都能用上倒装句呢?
概述日语中常见的省略表达方式
概述日语中常见的省略表达方式 本文从委婉的省略表达、人称代词的省略、双方共知部分的省略三个方面分析了在日语中经常出现的一些 省略表达方式。 [摘要]日语的表达有个显著的特点,那就是省略表达比较多。在日常生活中省略表达处处可见。本文通过具体的例子分析了委婉的省略表达、人称代词的省略表达和谈话双方共知部分的省略表达,最后分析了日语 中存在众多的省略表达的原因。 [关键词]省略表达;委婉省略;人称代词省略;共知部分省略 省略是任何语言中都存在的一种语言现象,一般来说,讲话中往往省略大家共知的部分,以求简明扼要。 由于日语特有的语言氛围和日本人独有的“以心伝心”、“腹芸”的文化背景,日语中的省略现象尤为突出,古典文法学家松尾拾治郎把省略作为日语三大特征之一,著名国语学家金田一春彦也认为日语具有“中国語以上の省略表現”:日本語の簡略表現は、時に中国語を上回るということは注意しておきたい?日本人は中 国語こそ簡略表現の極致のように思っているが、時には日本語にかなわないようだ?极多的省略表达现象,更增加了日语的暧昧性。下面我们来看一些在日语中经常出现的一些省略表达。 一、委婉的省略表达 委婉的省略,有时处于礼貌,有时处于自身为难,故意将后半句省略,任对方去琢磨、去理解。这种省略 往往出现在诸如向对方提出不满,拒绝对方或请求对方帮助等不说不行但说了又怕给对方带来不快或伤害 的场合。如:请求帮助时说:ご面倒でなければ……お忙しいところですが……提出不满时说:ちょっと、ご相談いたしたいことがございますが……あのう、申し訳ありませんが、あの、ステレオですが、音が……拒绝对方时说:お手伝いできるといいんですが……明日はちょっと……用省略的方式将自己不好讲 或不愿讲的话巧妙地“移交”给对方,看似模糊,听者稍加留心便可明其所言,这种省略的方式常给人一种 如释重负的良好感觉。因此,不仅在以上场合,就连在接电话时也经常会说“はい、鈴木ですが……”这种 情况下省略的多是一个句子单位,这是建立在日语本身的结构和共通代码———文化的共同理解的基础上的。要完成这种意会的交流,就要利用特殊的语言标志来暗示,使某些不便言表的信息,在一定的文化背 景下,通过特殊的语言标志和文化上的共通的理解,传送到听话人的意识构造里去。 二、人称代词的省略 按照日语的习惯,尽可能不使用人称代词,以敬词、惯用结构、形态变化等使之隐含在句子中,即省略”。有些一看便明白了省略了何种人称代词,而有的比较含糊,须细心观察(这一点与汉语的差异更为突出)。看下面的例句:①兄に連れてもらったのがきっかけで、(わたしは)釣りが好きになった?②(彼は)秘密にしてくれと言われていたのに、(私は)つい人に話してしまった?③(私は)いってきます。(あなたは)いってらっしゃい。(注:括号中为日语表达中的省略部分)从以上例句不难看出,日语和汉语在
中考文言文翻译实用方法经典讲解(带试题实例!)
一.增就是增补,在翻译时增补文言文省略句中的省略成分。 注意:补出省略的成分或语句,要加括号。 1、增补原文省略的主语、谓语或宾语 例1:“一鼓作气,再而衰,三而竭。”“再”“三”后省略了谓语“鼓”,翻译时要补上。 例2:“见渔人,乃大惊,问所从来。”译句:“(桃源中人)一见渔人,大为惊奇,问他是从哪里来的。” 例3:“君与具来。”“与”后省略了宾语“之”。 2、增补使语义明了的关联词 例:“不治将益深”是一个假设句,译句:“(如果)不治疗就会更加深入”。 二 .删就是删除,凡是古汉语中的发语词、在句子结构上起标志作用的助词和凑足音节的助词等虚词,因在现代汉语中是没有词能代替,故翻译时无须译出,可删去。 例1:“师道之不传也久矣。”译句:“从师学习的风尚已经很久不存在 了。”“也”为句中语气助词,起到舒缓语气的作用,没有实在意义。在翻译时,完全可以去掉。 例2:“孔子云:何陋之有?”译句:“孔子说:有什么简陋的呢?”“之”为宾语前置的标志,删去不译。 例3:“夫战,勇气也。”译句:“战斗,靠的是勇气”。“夫”为发语词,删去不译。 三.调就是调整,在翻译文言文倒装句时,应把古汉语倒装句式调整为现代汉语句式,使之符合现代汉语的表达习惯、译句通顺。这就需要调整语句语序, 大体有三种情况:
1、后置定语前移例:“群臣吏民,能面刺寡人之过者,受上赏。”可调成“能面刺寡人之过群臣吏民,受上赏。” 2、前置谓语后移例:“甚矣!汝之不惠。”可调成“汝之不惠甚矣。” 3、介宾短语前移。例:“还自扬州”可调成“自扬州还”。 4、前置宾语后移例:“何以战?”可调成“以何战?” 四.留就是保留,凡是古今意义相同的词、专有名词、国号、年号、人名、物名、人名、官职、地名等,在翻译时可保留不变。 例:“庆历四年春,滕子京谪守巴陵郡。”译句:“庆历四年的春天,滕子京被贬到巴陵郡做太守。”“庆历四年”为年号,“巴陵郡”是地名,可直接保留。 五.扩就是扩展。 1、言简义丰的句子,根据句义扩展其内容。 例:“怀敌附远,何招而不至?”译句:“使敌人降服,让远方的人归附,招抚谁,谁会不来呢?” 2、单音节词扩为同义的双音节词或多音节词。 例:“更若役,复若赋,则如何?”译句:“变更你的差役,恢复你的赋税,那么怎么样呢?”“役”“赋”扩展为双音节词。 六.缩就是凝缩,文言文中的有些句子,为了增强气势,故意实用繁笔,在翻译时应将其意思凝缩。 例:“有席卷天下,包举宇内,囊括四海之意,并吞八荒之心。”译句:“(秦)有吞并天下,统一四海的雄心。” 七.直即直译,就是指紧扣原文,按原文的词句进行对等翻译的今译方法。对于文言文的实词、大部分虚词、活用词和通假字,一般是要直接翻译的,否则, 在考查过程中是不能算作准取得翻译。 例:“清荣峻茂,良多趣味。”译句:“水清,树茂,山高,草盛,实在是趣味无穷。”