u05
SAP实施项目_U05 SD用户考试试卷_20091226
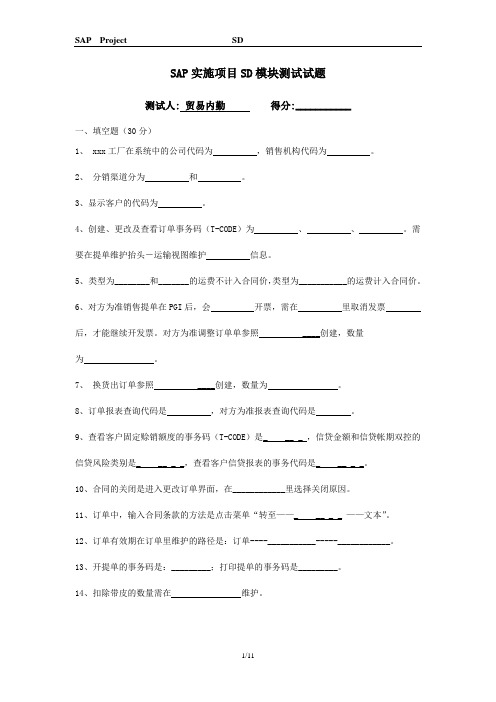
SAP实施项目SD模块测试试题测试人: 贸易内勤得分:___________一、填空题(30分)1、 xxx工厂在系统中的公司代码为,销售机构代码为。
2、分销渠道分为和。
3、显示客户的代码为。
4、创建、更改及查看订单事务码(T-CODE)为、、。
需要在提单维护抬头-运输视图维护信息。
5、类型为________和_______的运费不计入合同价,类型为___________的运费计入合同价。
6、对方为准销售提单在PGI后,会开票,需在里取消发票后,才能继续开发票。
对方为准调整订单单参照 ____创建,数量为。
7、换货出订单参照 ____创建,数量为。
8、订单报表查询代码是,对方为准报表查询代码是。
9、查看客户固定赊销额度的事务码(T-CODE)是_ __ _ ,信贷金额和信贷帐期双控的信贷风险类别是_ __ _ _,查看客户信贷报表的事务代码是_ __ _ _。
10、合同的关闭是进入更改订单界面,在____________里选择关闭原因。
11、订单中,输入合同条款的方法是点击菜单“转至——_ __ _ _ ——文本”。
12、订单有效期在订单里维护的路径是:订单----___________-----____________。
13、开提单的事务码是:_________;打印提单的事务码是_________。
14、扣除带皮的数量需在维护。
二、问答题(30分)1、在创建提单时系统提示如下操作,请解释原因。
2、我方为准的订单类型是什么?描述国内销售业务整个流程主要包括哪些步骤。
3、简述对方为准调整流程的操作4、简述换货业务在系统上需要做哪些操作?5、简述退货业务在系统上需要做哪些操作?6、赠品销售过程中根据客户接受发票中体现赠品量与发票中不体现赠品量,以及赠送同种物料和赠送不同种物料的业务,请分别简述处理流程。
7、简述什么情况下需要创建借、贷项请求,在系统上需要做哪些操作?三、操作题(40分)1、设置订单报表ZSD502的变式,要求:销售组织YCSO、分销渠道01、生产线83、默认取所有未交货的订单,写下变式名称。
小学下册U卷英语第二单元真题试卷
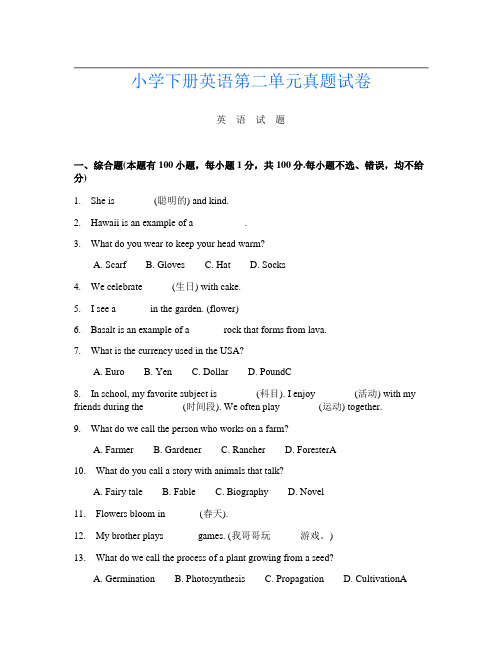
小学下册英语第二单元真题试卷英语试题一、综合题(本题有100小题,每小题1分,共100分.每小题不选、错误,均不给分)1.She is _______ (聪明的) and kind.2.Hawaii is an example of a __________.3.What do you wear to keep your head warm?A. ScarfB. GlovesC. HatD. Socks4.We celebrate _____ (生日) with cake.5.I see a ______ in the garden. (flower)6.Basalt is an example of a ______ rock that forms from lava.7.What is the currency used in the USA?A. EuroB. YenC. DollarD. PoundC8.In school, my favorite subject is _______ (科目). I enjoy _______ (活动) with my friends during the _______ (时间段). We often play _______ (运动) together.9.What do we call the person who works on a farm?A. FarmerB. GardenerC. RancherD. ForesterA10.What do you call a story with animals that talk?A. Fairy taleB. FableC. BiographyD. Novel11.Flowers bloom in ______ (春天).12.My brother plays ______ games. (我哥哥玩______游戏。
外教社国际商务礼仪简明教程PPT课件U05 United Kingdom
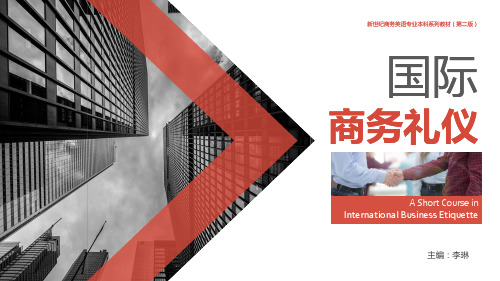
Lead-in
Cultural Values
Reading
Etiquette
Cultural Notes
Task 1: Read the text and answer the questions
Dilemma
The NHS aims to deliver comprehensive, universal and free medical service.
It still has not achieved its goals for lack of funding, understaffing and closure of local services. Centralization drives, and an increase in reliance on privatized services. It can campaign for more funding from the government, which can be used to recruit more doctors and nurses, and increase hospital beds for patients.
Dilemma
Why did the schemes in the “People’s Budget” of 1909 fail?
They had obvious limitations like meagre pension and unemployment insurance exclusive of hospital care, and spouses and children, which has attracted criticism from the British Medical Association, middle-class households and so on.
U05-01-中级口译之旅游观光
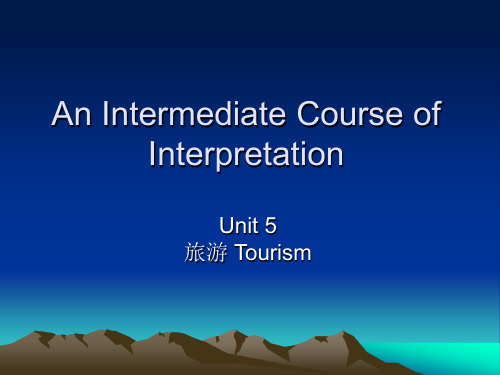
• 小旅馆,客栈 • 海边度假旅馆 • 高速公路 单人床 / 双床位/ 双人床/ • 加大床 / 特大床
• • • • • • • • • •
加长床 标准间(双床位) 水床 滚动式折叠床 暗床 露营,野营 宿营地 野营用拖车 美国 栖身之地
• 9. “long boy” • 10. double room • 11. waterbed • 12. rollaway bed • 13. hide-a-bed • 14. camping • 15. campground • 16. trailer • 17. the States • 18. shelter
新兴产业
The Chinese government has given profound attention to the tourism work, which has been undergoing steady and fast growth, as a newly emerging, dynamic and potentially strong industry.
• 6.旅游时一项集观光,娱乐,健身为一体的愉快 而美好的活动 Tourism represents a kind of popular and pleasant activity that combines sightseeing, recreation and health care. • 7.中国政府高度重视旅游工作,旅游业持续快速 发展,已经成为一个富有蓬勃活力和巨大潜力的
• 在各位做出选择之前,我想谈一下本旅行社有关团 体旅游的报价问题。 Before you make a decision on our tour, I’d like to make some remarks about the quotation policies regarding the group tours with this travel agency. • 首先,参加团体旅游的个人其报价均含交通费、住 宿费、膳食费、观光费、导游服务费以及双程国际 机票。 First, an individual's quotation for each group tour includes the cost of transportation, accommodation, meals, sightseeing, tour-guide service and round-trip (return trip) international airplane tickets.
etpb1u05_b_note
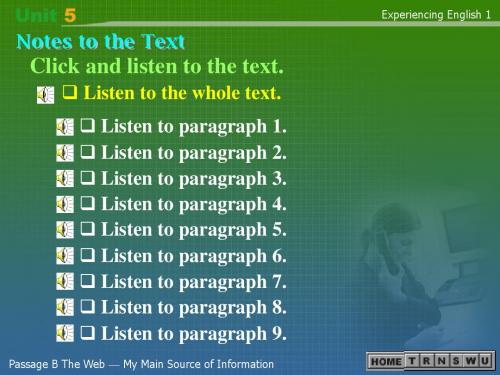
Passage B The Web — My Main Source of Information
Passage B The Web — My Main Source of Information
Experiencing English 1
Notes to the Text 4. Although it takes time to sift through the multitude of sources, I find that after years of use I can quickly analyze the search results to find the most appropriate site. It takes time to compare the many sources and find the best website, but after years of practice I’ve learned to do this quickly. 尽管筛选各种信息需要花费时间, 尽管筛选各种信息需要花费时间,但经过多年的 使用后, 使用后,我发现我能快速地分析搜寻的结果并找 到最合适的网址。 到最合适的网址。
Passage B The Web — My Main Source of Information
Experiencing English 1
Notes to the Text 9. It also provides a highly collaborative environment in which it is quick and easy to exchange ideas with colleagues. It also promotes a very convenient and cooperative approach towith colleagues. 网络也提供了高度协作的工作环境, 网络也提供了高度协作的工作环境,通过它可 以和同事们随时交换意见。 以和同事们随时交换意见。
LiBu05-03
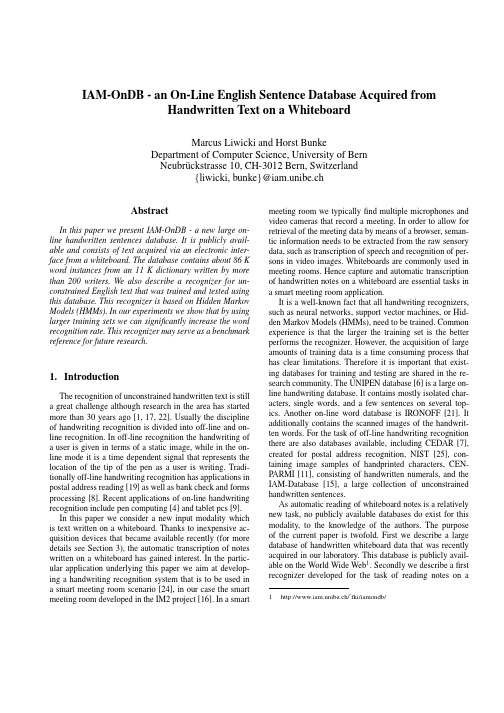
IAM-OnDB-an On-Line English Sentence Database Acquired from Handwritten Text on a WhiteboardMarcus Liwicki and Horst BunkeDepartment of Computer Science,University of BernNeubr¨u ckstrasse10,CH-3012Bern,Switzerland{liwicki,bunke}@iam.unibe.chAbstractIn this paper we present IAM-OnDB-a new large on-line handwritten sentences database.It is publicly avail-able and consists of text acquired via an electronic inter-face from a whiteboard.The database contains about86K word instances from an11K dictionary written by more than200writers.We also describe a recognizer for un-constrained English text that was trained and tested using this database.This recognizer is based on Hidden Markov Models(HMMs).In our experiments we show that by using larger training sets we can significantly increase the word recognition rate.This recognizer may serve as a benchmark reference for future research.1.IntroductionThe recognition of unconstrained handwritten text is still a great challenge although research in the area has started more than30years ago[1,17,22].Usually the discipline of handwriting recognition is divided into off-line and on-line recognition.In off-line recognition the handwriting of a user is given in terms of a static image,while in the on-line mode it is a time dependent signal that represents the location of the tip of the pen as a user is writing.Tradi-tionally off-line handwriting recognition has applications in postal address reading[19]as well as bank check and forms processing[8].Recent applications of on-line handwriting recognition include pen computing[4]and tablet pcs[9].In this paper we consider a new input modality which is text written on a whiteboard.Thanks to inexpensive ac-quisition devices that became available recently(for more details see Section3),the automatic transcription of notes written on a whiteboard has gained interest.In the partic-ular application underlying this paper we aim at develop-ing a handwriting recognition system that is to be used in a smart meeting room scenario[24],in our case the smart meeting room developed in the IM2project[16].In a smart meeting room we typicallyfind multiple microphones and video cameras that record a meeting.In order to allow for retrieval of the meeting data by means of a browser,seman-tic information needs to be extracted from the raw sensory data,such as transcription of speech and recognition of per-sons in video images.Whiteboards are commonly used in meeting rooms.Hence capture and automatic transcription of handwritten notes on a whiteboard are essential tasks in a smart meeting room application.It is a well-known fact that all handwriting recognizers, such as neural networks,support vector machines,or Hid-den Markov Models(HMMs),need to be mon experience is that the larger the training set is the better performs the recognizer.However,the acquisition of large amounts of training data is a time consuming process that has clear limitations.Therefore it is important that exist-ing databases for training and testing are shared in the re-search community.The UNIPEN database[6]is a large on-line handwriting database.It contains mostly isolated char-acters,single words,and a few sentences on several top-ics.Another on-line word database is IRONOFF[21].It additionally contains the scanned images of the handwrit-ten words.For the task of off-line handwriting recognition there are also databases available,including CEDAR[7], created for postal address recognition,NIST[25],con-taining image samples of handprinted characters,CEN-PARMI[11],consisting of handwritten numerals,and the IAM-Database[15],a large collection of unconstrained handwritten sentences.As automatic reading of whiteboard notes is a relatively new task,no publicly available databases do exist for this modality,to the knowledge of the authors.The purpose of the current paper is twofold.First we describe a large database of handwritten whiteboard data that was recently acquired in our laboratory.This database is publicly avail-able on the World Wide Web1.Secondly we describe afirst recognizer developed for the task of reading notes on a 1http://www.iam.unibe.ch/˜fki/iamondb/whiteboard.This recognizer may serve as a reference for further research in this field.The rest of the paper is organized as follows.Sec-tion 2describes the design of IAM-OnDB.In Section 3the data acquisition process is presented.Section 4gives an overview of the system for whiteboard note recog-nition,including some optimization steps.Experiments and results are presented in Section 5,and finally Sec-tion 6draws some conclusions and gives an outlook for future work.2.The databaseThe design of the database described in this paper,called IAM-OnDB,is inspired by the IAM-Database presented in [15].However,while the IAM-Database is an off-line database,the IAM-OnDB consists of on-line data acquired from a whiteboard.All texts included in the IAM-OnDB are taken from the Lancaster-Oslo/Bergen corpus (LOB),which is a large electronic corpus of text [10].Using the LOB corpus as the underlying source of text makes it pos-sible to automatically generate language models,such as statistical n-grams and stochastic grammars [18].Conse-quently linguistic knowledge beyond the lexicon level can be integrated in a recognizer.The LOB corpus contains 500English texts,each consisting of about 2,000words.These texts are of quite diverse nature.They are divided into 15categories ranging from press and popular literature to learned and scientific writing.To acquire a database of handwritten sentences con-tained in the corpus we split the texts in the corpus into frag-ments of about 50words each.These fragments were copied onto forms on paper and each writer was asked to write down the text of eight forms on the whiteboard.To make sure that many different word samples are obtained from each writer,we have chosen these eight texts from different text categories in the LOB corpus.The resulting database consists of more than 1,700handwritten forms from 221writers.It contains 86,272word instances from a 11,059word dictionary written down in 13,049text lines.In addition to the recorded data and its transcription some informations about the writers,which could be use-ful for future work,are stored in the IAM-OnDB.These include,for each writer,the native country and language,other mastered languages,age and gender,and the writing style,i.e.right-or left-handed writing style.The writers who contributed to the database were all volunteers.Most of them are students and staff members of the University of Bern.Both genders are about equally represented in the database and about 10%of the writers have left-handedwriting.Figure 1.Illustration of the recording;note the data acquisition device in the left upper corner of thewhiteboardFigure 2.Interface of the recording software3.AcquisitionThe eBeam 2interface is used to record the handwriting of a user.It allows us to write on a whiteboard with a nor-mal pen in a special casing,which sends infrared signals to a triangular receiver mounted in one of the corners of the whiteboard.The acquisition interface outputs a sequence of (x,y)-coordinates representing the location of the tip of the pen together with a time stamp for each location.An illus-tration of the data acquisition process is shown in beling of the data is a prerequisite for recognition ex-periments.It is advisable to do as much as possible automat-ically because labeling is expensive,time consuming and2eBeam System by Luidia,error prone.During the recordings an operator observes the received data with a special recording software written at our laboratory.The softwarefirst loads the ASCII transcrip-tion of the text to be written.While the writer renders the handwritten text the operator adjusts the line feeds during recording.He or she is also able to make corrections if the handwritten text does not correspond to the printed text,for example,if the writer leaves out some words.Fig.2shows a screen shot of the interface.The transcription produced by the operator in the lower window is saved together with the recorded on-line data in one xml-file.The raw data stored in one xml-file usually includes sev-eral consecutive lines of text.For the recognizer and the experiments described in Section4and5,respectively,we need to segment the text into individual lines.The line seg-mentation process of the on-line data is guided by heuris-tic rules.If there is a pen-movement to the left and verti-cally down that is greater than a predefined threshold,a new line is started.This method succeeds on more than99%of the text forms.There are only two cases where a line is too short and a few cases where the writer moved back and forth across different text lines to render an i-dot.To ensure that the automatic line segmentation has been done correctly the resulting lines are checked by the operator and corrected if necessary.Consecutive lines are highlighted in different colors on the screen so that an error can be easily detected.4.Recognition system overviewA basic cursive handwriting recognition system has been trained and tested on the database described in the previ-ous sections.A preliminary version of this recognition sys-tem was introduced in[13],and its adaptation to a different training modality described in[12].The recognizer is de-rived from the Hidden Markov Model(HMM)based system proposed in[14].Although the handwriting captured in the database described in this paper is in the on-line mode,the recognizer takes off-line handwritten lines of text as its ing off-line rather than on-line data has two reasons. First,the existing recognizer[14]has been designed for off-line data,and secondly,it is straightforward to convert on-line data to the off-line modality.Eventually we plan to ad-ditionally build an on-line recognizer and combine it with the existing off-line system.From such a combination,en-hanced recognition performance can be expected[20,23].An overview of our whiteboard data handwriting recog-nition system is shown in Fig.3.The system consists of six main modules:the on-line preprocessing,where noise in the raw data is reduced;the transformation,where the on-line data is transformed into off-line format;the off-line pre-processing,where various normalization steps take place; the feature extraction,where the normalized image is trans-formed into a sequence of feature vectors;the recognition,Figure3.Recognition system overview where the HMM-based classifier generates an n-best list of word sequences;and the post-processing,where a statisti-cal language model is applied to improve the results gener-ated by the HMM.In the remainder of this section more de-tails of the individual modules will be provided.The recorded on-line data usually contain noisy points and gaps within strokes.Thus two on-line preprocessing steps are applied to the data,to recover from artifacts of this kind.Let p1,...,p n be the points of a given stroke and q1be thefirst point of the succeeding stroke,if any.To identify noisy points,we check whether the distance be-tween two consecutive points p i,p i+1,is larger than afixed threshold.In this case one of the points is deleted.To de-cide which point has to be deleted,the number of points within a small neighborhood of p i and p i+1are determined, and the point with a smaller number of neighbors is deleted. To recover from artifacts of the second type,i.e.from gaps within strokes,we check if the distance between the times-tamps of p n and q1is under afixed threshold.If the condi-tion is true the strokes are merged into one stroke.Since the preprocessed data is still in the on-line format, it has to be transformed into an off-line image,so that it can be used as input for the off-line recognizer.The recognizer was originally designed for the off-line IAM-Database[15] and optimized on gray-scale images scanned with a resolu-tion of300dpi.To get good recognition results in the con-sidered application,the produced images should be similar to these off-line images.Consequently the following steps are applied to generate the images.First,all consecutivepoints within the same stroke are connected.This results in one line segment per stroke.Then the lines are dilated to a width of eight pixels.The center of each line is colored black and the pixels are getting lighter towards the periph-ery.The basic recognizer is a Hidden Markov Model(HMM) based cursive handwriting recognizer similar to the one de-scribed in[14].It takes,as an input unit,the image of a complete text line,which isfirst normalized with respect to skew,slant,writing width and baseline location.Normaliza-tion of the baseline location means that the body of the text line(the part which is located between the upper and lower baselines),the ascender part(located above the upper base-line),and the descender part(below the lower baseline)will be vertically scaled to a predefined size each.Writing width normalization is performed by a horizontal scaling opera-tion,and its purpose is to scale the characters so that they have a predefined average width.To extract the feature vectors from the normalized im-ages,a sliding window approach is used.The with of the window is one pixel and nine geometrical features are com-puted at each window position.Thus an input text line is converted into a sequence of feature vectors in a9-dimensional feature space.An HMM is built for each of the58characters in the character set,which includes all small and capital letters and some other special characters,e.g.punctuation marks.In all HMMs the linear topology is used,i.e.there are only two transitions per state,one to itself and one to the next state. In the emitting states,the observation probability distribu-tions are estimated by mixtures of Gaussian components. The character models are concatenated to represent words and sequences of words.For training,the Baum-Welch al-gorithm[2]is applied.In the recognition phase,the Viterbi algorithm[3]is used tofind the most probable word se-quence.Note that the difficult task of explicitly segmenting a line of text into isolated words is avoided,and the segmen-tation is obtained as a byproduct of the Viterbi decoding ap-plied in the recognition phase.The output of the recognizer is a sequence of words.In the experiments described in Sec-tion5,the recognition rate will always be measured on the word level.In[5]it has been pointed out that the number of Gaus-sians and training iterations have an effect on the recogni-tion results of an HMM recognizer.Often the optimal value increases with the amount of training data because more variations are encountered.The system described in this pa-per has been trained with up to36Gaussian components and the classifier that performed best on a validation set has been taken as thefinal one in each of the experiments de-scribed in Section5.Another optimization step proposed in[26]is the inclu-sion of a language model,which corresponds to the post-processing step illustrated in Fig.3.Since the system de-scribed in this paper is performing handwritten text recog-nition on text lines and not only on single words,it is in fact reasonable to integrate a statistical language model.For fur-ther details we refer to[26].5.Experiments and resultsIn this section we report on a number of experiments with the database and the recognizer introduced in this pa-per.These experiments were conducted for the purpose of getting afirst impression of how difficult the reading of whiteboard notes is.Intuitively one can expect that the qual-ity of handwriting on a whiteboard is lower than on-line handwriting produced on an electronic writing tablet or off-line handwriting scanned in from paper,for at least two rea-sons.First,most people are much more used to writing with a normal pen on paper or even with an electronic pen on a writing tablet than to writing on a whiteboard.Secondly, when using a normal pen on paper or an electronic pen on a tablet,the writer’s arm usually rests on a table.By contrast, when writing on a whiteboard,one usually stands in front of the whiteboard and the arm does not rest on any surface, which puts much more stress on the writers’hand.There-fore we must expect more noise and distortions in white-board handwriting than in normal on-line or off-line hand-written data.To investigate the effect of a growing amount of train-ing data,wefirst trained and tested the recognition system on a small data set produced by20writers.Next,we used a larger training set produced by50writers and tested the rec-ognizer under the same conditions as on the small data set. Finally,we used the full IAM-OnDB for the experiments. For all these experiments the same test set was used always under the same conditions.The language model was gen-erated from the LOB-Corpus,which contains500printed texts of about2,000words each.In thefirst three experiments a dictionary of size2,337 words was used.It contains exactly those words which oc-cur in the test set.In the experiment with the small train-ing set,6,204words in1,258lines from20different writers were available.This data set was randomly divided intofive disjoint sets of approximately equal size(sets s0,...,s4). On these sets,5-fold cross validation was performed in the following way(combinations c0,...,c4).For i=0, (4)sets s i⊕2,s i⊕3and s i⊕4were taken for training the recog-nizer,set s i⊕1was used as a validation set,i.e.for optimiz-ing the parameters in the optimization steps,and set s i was used as a test set for measuring the system performance.No writer appeared in more than one set.Consequently,writer-independent recognition experiments were conducted.The average word recognition rate of this recognizer is59.54% on the validation sets and59.59%on the test sets.By in-5055 6065 70r e c o g n i t i o n r a t etraining set sizeFigure 4.Recognition rate on the test set by using a small dictionary tegrating a language model as described in Section 4the recognition rate could be increased to 65.56%on the vali-dation sets and to 64.27%on the test sets.For the next experiment we added the texts of 30more writers to each training set.We validated the optimization parameters on the same validation sets and tested the per-formance on the same test sets.The average recognition rate on the validation sets is 61.17%without a language model.It increases to 66.22%by including a language model.On the test sets it is 60.39%without and 64.81%with inclu-sion of a language model.In the last experiments all data from the 201writers that do not appear in the test sets was used for training.There the average recognition rate is 61.75%on the validation sets and 61.03%on the test sets.By integrating a language model the performance could be increased to 68.07%on the validation sets and 66.4on the test sets.Fig.4gives a summary of the experimental results on the test set.The performance could be increased by 2.1%by us-ing the large data sets for training.This increase is statisti-cally significant (α=1%).We also tested the trained recognizers on the large 11K word dictionary that includes all words in the database to study the effect of increasing the dictionary size (see Fig.5).The average recognition rate of the optimized system which has been trained on the small database is 62.80%on the test sets when the language model is included.The effect of using a larger training database is greater than on the small dictionary.The recognition rate increased by 0.6%to 63.38%for the medium size and statistically significantly (α=1%)by 3.1%to 65.90%for the large training set.This performance is only 0.5%below the performance on the small dictionary which has only about one fifth of the size.In Figs.4and 5can be observed that the inclusion of a5055606570r e c o g n i t i o n r a t etraining set sizeFigure 5.Recognition rate on the same test set by using a large dictionarylanguage model has a larger effect if the word dictionary contains more words.While the performance of the system trained on the large database increases by 5.4%on the 2.3K dictionary,it increases by 8.3%on the 11K dictionary.This is because many errors that could have been corrected by using a dictionary are now corrected by using linguis-tic information.6.Conclusions and future workIn this paper we have addressed a new task in cursive handwriting recognition,which is the automatic reading of cursive text from a whiteboard.This modality is emerging in new applications,for example,in the context of smart meeting rooms.First a new database of handwritten white-board text has been described.To the knowledge of the au-thors,this is the first public handwritten sentence database which is based on a whiteboard as input modality.It consists of 86,272word instances over an 11,059word dictionary written by 221writers,where each writer wrote approxi-mately the same number of words.It is planned to make this IAM-OnDB a part of the UNIPEN database [6]soon.Furthermore we have introduced a recognizer for white-board handwriting.It is based on HMMs and includes a sta-tistical bigram language model.This recognizer may serve as a benchmark for future research.In a number of ex-periments it was confirmed that increasing the size of the training set leads in fact to higher recognition rates.On the 11K word dictionary the recognition rate could be in-creased by 3.1%to 65.9%.This increase is statistically sig-nificant.From this point of view the database described in this paper,which is publicly available,may be useful to the research community for improving the quality of handwrit-ing recognition systems,particularly in the context of hand-writing data acquired from a whiteboard.AcknowledgmentsThis work was supported by the Swiss National Science Foundation program“Interactive Multimodal Information Management(IM)2”in the Individual Project“Scene Anal-ysis”,as part of NCCR.The authors thank all volunteers who took the time for participating in the recordings.We also thank Dr.Darren Moore for providing us a basic driver software for the eBeam system.References[1]H.Bunke.Recognition of cursive roman handwriting-pastpresent and future.In Proc.7th ICDAR,volume1,pages 448–459,2003.[2] A.Dempster,ird,and D.Rubin.Maximum likelihoodfrom incomplete data via the EM algorithm.Journal of Royal Statistical Society B,39(1):1–38,1977.[3]G.D.Forney.The Viterbi algorithm.In Proc.IEEE,vol-ume61,pages268–278,1973.[4]N.Furukawa,H.Ikeda,Y.Kato,and H.Sako.D-pen:A dig-ital pen system for public and business enterprises.In Proc.9th IWFHR,pages269–274,2004.[5]S.G¨u nter and H.Bunke.HMM-based handwritten wordrecognition:on the optimization of the number of states, training iterations and Gaussian components.Pattern Recog-nition,37:2069–2079,2004.[6]I.Guyon,L.Schomaker,R.Plamondon,M.Liberman,andS.Janet.Unipen project of on-line data exchange and recog-nizer benchmarks.In Proc.12th ICPR,pages29–33,1994.[7]J.J.Hull.A database for handwritten text recognition re-search.IEEE TPAMI,16(5):550–554,1994.[8]S.Impedovo,P.Wang,and H.Bunke.Automatic BankcheckProcessing.World Scientific,1997.[9]N.Iwayama,K.Akiyama,H.Tanaka,H.Tamura,andK.Ishigaki.Handwriting-based learning materials on a tablet pc:A prototype and its practical studies in an elementary school.In Proc.9th IWFHR,pages533–538,2004. [10]S.Johansson.The tagged LOB Corpus:User’s Manual.Norwegian Computing Centre for the Humanities,Norway, 1986.[11]S.-W.Lee.Off-line recognition of totally unconstrainedhandwritten numerals using multilayer cluster neural net-work.IEEE Trans.Pattern Anal.Mach.Intell.,18(6):648–652,1996.[12]M.Liwicki and H.Bunke.Enhancing training data for hand-writing recognition of whiteboard notes with samples from a different database.Accepted for publication,2005.[13]M.Liwicki and H.Bunke.Handwriting recognition of white-board notes.In Proc.12th Conf.of the International Grapho-nomics Society,2005.Accepted for publication.[14]U.-V.Marti and ing a statistical language modelto improve the performance of an HMM-based cursive hand-writing recognition system.IJPRAI,15:65–90,2001. [15]U.-V.Marti and H.Bunke.The IAM-database:an Englishsentence database for offline handwriting recognition.IJ-DAR,5:39–46,2002.[16] D.Moore.The IDIAP smart meeting room.Technical re-port,IDIAP-Com,2002.[17]R.Plamondon and S.N.Srinhari.On-line and off-linehandwriting recognition:A comprehensive survey.In IEEE TPAMI,volume22,pages63–84,2000.[18]R.Rosenfeld.Two decades of statistical language modeling:Where do we go from here?In Proc.IEEE88(8),2000. [19]S.N.Srihari.Handwritten address interpretation:A task ofmany pattern recognition problems.IJPRAI,14(5):663–674, 2000.[20]O.Velek,S.J¨a ger,and M.Nakagawa.Accumulated-recognition-rate normalization for combining multiple on/off-line Japanese character classifiers tested on a large database.In Proc.4th Multiple Classifier Systems,pages 196–205,2003.[21] C.Viard-Gaudin,llican,P.Binter,and S.Knerr.TheIRESTE on/off(IRONOFF)dual handwriting database.In Proc.5th ICDAR,pages455–458,1999.[22] A.Vinciarelli.A survey on off-line cursive script recogni-tion.Pattern Recognition,35(7):1433–1446,2002.[23] A.Vinciarelli and bining online and of-fline handwriting recognition.In Proc.7th ICDAR,pages 844–848,2003.[24] A.Waibel,T.Schultz,M.Bett,R.Malkin,I.Rogina,R.Stiefelhagen,and J.Yang.Smart:The smart meeting room task at isl.In Proc.IEEE ICASSP,volume4,pages752–755, 2003.[25]R.Wilkinson,J.Geist,S.Janet,P.Grother,C.Burges,R.Creecy,B.Hammond,J.Hull,rsen,T.V ogl,andC.Wilson,editors.1st census optical character recognitionsystems conf.#NISTIR4912,1992.[26]M.Zimmermann and H.Bunke.Optimizing the integrationof a statistical language model in HMM-based offline hand-written text recognition.In Proc.17th ICPR,pages541–544,2004.。
U05-A大学英语新视野
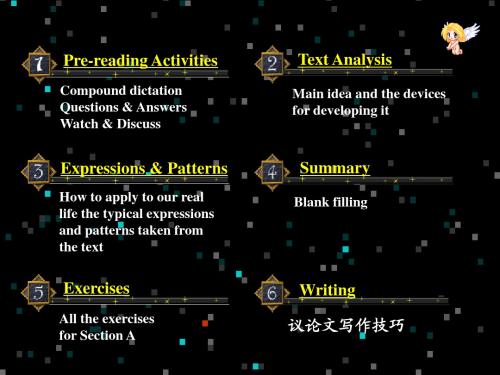
Ⅵ.
Writing
My Way of Seeing Something
1. 人们从不同的角度看待某一事物 2. 我的看法是··(说明理由) ·· ·· (理由陈述类)
写作模式 参考范文
Ⅵ.
Writing
Back
写作模式(理由陈述类) 1) Something may be many things to many people. 2) Some may see it as …while others may view it as …(人们 的看法举例) 3) As far as I am concerned, however, I would prefer to value it as … (我的看法) (Para. I)
(划线部分可替换)
上一页 下一页
Ⅵ.
Writing
Back
My Way of Seeing Life
1. 人们从不同的角度看待人生 2. 我所推崇的看法是··(说明理由) ·· ··
上一页
下一页
Ⅵ.
Writing
Back
My Way of Seeing Life 1) Life may be many things to many people. 2) Some may see it as building up as much material wealth as possible while others may view it as enjoying to the fullest the pleasures each day offers. 3) As far as I am concerned, however, I would prefer to value it as my sole chance to enrich myself with knowledge first, then to serve the society with all my heart, and finally to leave the world without any regrets.
U05NU44中文资料
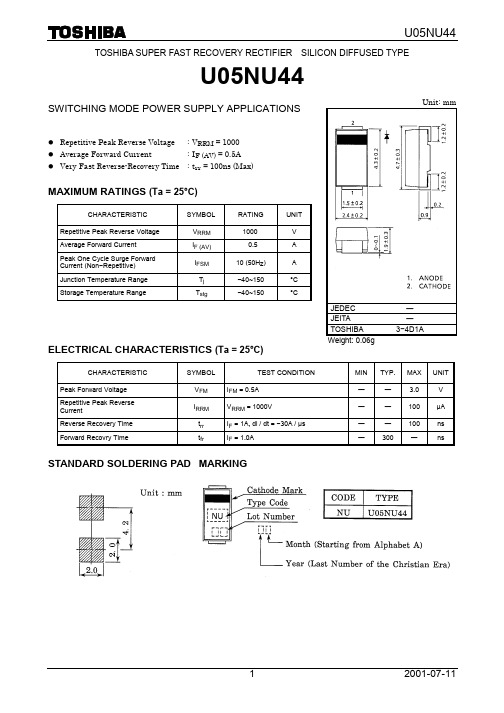
TOSHIBA SUPER FAST RECOVERY RECTIFIER SILICON DIFFUSED TYPEU05NU44SWITCHING MODE POWER SUPPLY APPLICATIONSl Repetitive Peak Reverse Voltage : V RRM = 1000l Average Forward Current : I F (AV) = 0.5Al Very Fast Reverse-Recovery Time : t rr = 100ns (Max)MAXIMUM RATINGS (Ta = 25°C)CHARACTERISTIC SYMBOLRATINGUNITRepetitive Peak Reverse Voltage V RRM1000 VAverage Forward Current I F (AV)0.5 APeak One Cycle Surge ForwardCurrent (Non−Repetitive)I FSM10 (50H z) AJunction Temperature Range T j−40~150 °CStorage Temperature Range T stg−40~150 °CELECTRICAL CHARACTERISTICS (Ta = 25°C)CHARACTERISTIC SYMBOL TESTCONDITION MINTYP.MAX UNIT Peak Forward Voltage V FM I FM = 0.5A ―― 3.0 V Repetitive Peak ReverseCurrentI RRM V RRM = 1000V ―― 100µAReverse Recovery Time t rr I F = 1A, di / dt = −30A / µs ―― 100ns Forward Recovry Time t fr I F = 1.0A ― 300 ― ns STANDARD SOLDERING PAD MARKINGJEDEC ―JEITA ―TOSHIBA 3−4D1AWeight: 0.06gUnit: mm· TOSHIBA is continually working to improve the quality and reliability of its products. Nevertheless, semiconductor devices in general can malfunction or fail due to their inherent electrical sensitivity and vulnerability to physical stress. It is the responsibility of the buyer, when utilizing TOSHIBA products, to comply with the standards of safety in making a safe design for the entire system, and to avoid situations in which a malfunction or failure of such TOSHIBA products could cause loss of human life, bodily injury or damage to property.In developing your designs, please ensure that TOSHIBA products are used within specified operating ranges as set forth in the most recent TOSHIBA products specifications. Also, please keep in mind the precautions and conditions set forth in the “Handling Guide for Semiconductor Devices,” or “TOSHIBA Semiconductor Reliability Handbook” etc.. · The TOSHIBA products listed in this document are intended for usage in general electronics applications (computer, personal equipment, office equipment, measuring equipment, industrial robotics, domestic appliances, etc.). These TOSHIBA products are neither intended nor warranted for usage in equipment that requires extraordinarily high quality and/or reliability or a malfunction or failure of which may cause loss of human life or bodily injury (“Unintended Usage”). Unintended Usage include atomic energy control instruments, airplane or spaceship instruments, transportation instruments, traffic signal instruments, combustion control instruments, medical instruments, all types of safety devices, etc.. Unintended Usage of TOSHIBA products listed in this document shall be made at the customer’s own risk. · The information contained herein is presented only as a guide for the applications of our products. No responsibility is assumed by TOSHIBA CORPORATION for any infringements of intellectual property or other rights of the third parties which may result from its use. No license is granted by implication or otherwise under any intellectual property or other rights of TOSHIBA CORPORATION or others. · The information contained herein is subject to change without notice.000707EAARESTRICTIONS ON PRODUCT USE。
- 1、下载文档前请自行甄别文档内容的完整性,平台不提供额外的编辑、内容补充、找答案等附加服务。
- 2、"仅部分预览"的文档,不可在线预览部分如存在完整性等问题,可反馈申请退款(可完整预览的文档不适用该条件!)。
- 3、如文档侵犯您的权益,请联系客服反馈,我们会尽快为您处理(人工客服工作时间:9:00-18:30)。
− ( P {X ≤ x 2 , Y ≤ y1 } − P {X ≤ x 1 , Y ≤ y1 }) = F ( x 2 , y 2 ) − F ( x 1 , y 2 ) − F ( x 2 , y1 ) + F ( x 1 , y1 )
y
( x1 , y 2 )
y2 y1
( x1 , y1 )
( x2 , y2 )
第五章 二维随机变量及其分布
第一节 二维随机变量及分布函数 第二节 二维离散型随机变量 第三节 二维连续型随机变量 第四节 边缘分布 第五节 随机变量的独立性
第一节 二维随机变量及分布函数
1、二维随机变量的定义 、
定义1.1 设E为一个随机试验 其样本空间 为一个随机试验, 定义 为一个随机试验 ={ω}, X=X(ω)和Y=Y(ω)是定义在 上的两个 和 是定义在 随机变量, 则由它们构成的联合变量(X,Y)称为 随机变量 则由它们构成的联合变量 称为 二维随机变量或二维随机向量。 二维随机变量或二维随机向量。
2、二维离散型随机变量的分布律
定义2.2 设二维离散型随机变量 设二维离散型随机变量(X,Y)的所有可 定义 的所有可 能取值为(x , 能取值为 i,yj),i,j=1,2,…,令 ,
pij = P {X = x i , Y = y j } 1° 2°
∞ ∞
i , j = 1, 2, ⋯ i , j = 1, 2, ⋯
y
F ( x1 , y )
F ( x2 , y )
0
x1
x2
x
性质2 性质
0≤F(x,y)≤1 ≤ ≤
对固定的 x , F ( x , −∞ ) = y, 对固定的 y , F ( −∞ , y ) = 0
F ( −∞ , −∞ ) = 0,F ( +∞ , +∞ ) = 1
性质3 F(x,y)关于变量 和y均是右 关于变量x和 均是右 性质 关于变量 连续的。 连续的。
求常数A, 求常数 B, C。 。
解:
π π F(+∞+∞ = A B+ C + =1 , ) 2 2
π y F(−∞ y) = A B− C +ar , ctan = 0 2 2
x π F(x,−∞ = A B ar ) - =0 + ctan C 2 2
例2.2 一射手向一目标射击, 击中目标的概率为p 一射手向一目标射击 击中目标的概率为 (0<p<1), 射击到击中两次目标为止。设以 表示首次 射击到击中两次目标为止。设以X表示首次 击中目标所进行的射击次数, 击中目标所进行的射击次数 以Y表示总共进行的射 表示总共进行的射 击次数, 为二维离散型随机变量, 击次数 则(X,Y)为二维离散型随机变量 其可能取值 为二维离散型随机变量 为: (X,Y)=(m,n) m=1,2,…,n–1; n=2,3,…
( X , Y )可能取值为 ( x , y ) ∈ R 2,即其值域 若 B ⊂ R 2,则 D = {( x , y ) X (ω ) = x , Y (ω ) = y, ∀ ω ∈ Ω} ⊂ R 2
ω 为一随机事件。 集合 { ( x , y ) = ( X (ω ), Y (ω ) ) ∈ B} ⊂ Ω 为一随机事件。
不满足性质4, 故F(x,y)不能作为分布函数。 不满足性质 不能作为分布函数。 不能作为分布函数 不右连续, 不满足性质3。 且F(x,y)不右连续 如(0,0), 不满足性质 。 不右连续
设二维随机变量(X,Y)的分布函数为 例1.2 设二维随机变量 的分布函数为
x y F(x, y) = A B+arctan C +arctan 2 2 −∞< x < +∞, −∞< y < +∞
1 1 1 × = 4 3 12 1 2 1 p 32 = P {X = 3, Y = 2} = P {X = 3}P { = 2 X = 3} = × = Y 4 3 6 1 p 33 = P {X = 3, Y = 3} = P {X = 3}P { = 3 X = 3} = × 0 = 0 Y 4 即:
y
1 4
(1,1)
一口袋中有四个球, 例2.3 一口袋中有四个球 其上分别标有 1, 2, 2, 3, 从中任取一球后 不放回袋中 从中任取一球后, 不放回袋中, 再从袋中任取一球, 依次用X, 表示第一 表示第一、 再从袋中任取一球 依次用 Y表示第一、 二次取得的球上标有的数字。 二次取得的球上标有的数字。 (1)求(X,Y)的分布律; 求 的分布律; 的分布律 (2)求P(X≥Y)。 求 ≥ 。
其中P{X≤x,Y≤y}=P{ω|X(ω)≤x,Y(ω)≤y}可视为随 ≤ ≤ 其中 ≤ ≤ 可视为随 机点(X,Y)落在下图所示的,以(x,y)为顶点的位 落在下图所示的, 机点 落在下图所示的 为顶点的位 于该点左下方的无穷矩形域内的概率。 于该点左下方的无穷矩形域内的概率。
y
F(x, y)
( x, y)
即 ∀x , y ∈ ( −∞ ,+∞ ) F ( x + 0, y ) = lim F ( x + ε , y ) = F ( x , y )
ε →0 +
F ( x , y + 0 ) = lim F ( x , y + ε ) = F ( x , y )
ε →0 +
性质4 对于任意的 1≤x2,y1≤y2, 对于任意的x 性质 下述不等式成立: 下述不等式成立:
F ( x 2 , y2 ) − F ( x1 , y2 ) − F ( x 2 , y1 ) + F ( x1 , y1 ) ≥ 0
注:满足上述性质1~4的二元函数 满足上述性质 的二元函数 可作为某个二维随机变量的分布函 数。
0 x + y ≤ 0 例1.1 二元函数 F ( x , y ) = 1 x + y > 0 可否为某个二维随机变量的分布函数。 可否为某个二维随机变量的分布函数。
解: 先求(X,Y)的分布律 X,Y的可能取值为 先求 的分布律, 的可能取值为1,2,3。 。 的分布律 的可能取值为
p11 = P {X = 1, Y = 1} = P {X
p12 = P {X = 1, Y = 2} = P {X p13 = P {X = 1, Y = 3} = P {X p 21 = P {X = 2 , Y = 1} = P {X p 22 = P {X = 2 , Y = 2} = P {X p 23 = P {X = 2 , Y = 3} = P {X
0 1/6 1/12 1/6 1/6 1/6 1/12 1/6 0
第三节
二维连续型随机变量
1、二维连续型随机变量的定义
定义3.1 如果二维随机变量 如果二维随机变量(X,Y)的分布函数 定义 的分布函数 F(x,y), 存在一个非负可积的二元函数 存在一个非负可积的二元函数f(x,y), 使 对于任意实数x,y, 均有 对于任意实数
0
x
定义, 由 F ( x , y ) 定义,容易得出随机点 ( X , Y ) 落入有限矩 内的概率, 形域 {x 1 < X ≤ x 2 , y1 < Y ≤ y 2 } 内的概率,即 P {x 1 < X ≤ x 2 , y1 < Y ≤ y 2 } = P {X ≤ x 2 , Y ≤ y 2 } − P {X ≤ x 1 , Y ≤ y 2 }
p 31 = P {X = 3, Y = 1} = P {X = 3}P { = 1 X = 3} = Y
Y X 1 2 3
P{X≥Y}=P{X=1,Y=1}+P{X=2,Y=1} ≥
1
2
3
+P{X=2,Y=2}+P{X=3,Y=1} +P{X=3,Y=2}+P{X=3,Y=3} =0+1/6+1/6+1/12+1/6+0 =7/12
ij
( 2.1)
若其满足
pij ≥ 0
∑∑ p
i =1 j =1
=1
则称(2.1)式为二维离散型随机变量 式为二维离散型随机变量(X,Y)的概率 则称 式为二维离散型随机变量 的 分布(或分布律),或称为随机变量X和 的 分布 或分布律 ,或称为随机变量 和Y的联合 分布或联合概率分布。 分布或联合概率分布。
分布律通常用表格表示为 X Y
y1 ⋯
p11
yj ⋯
⋯
x1 ⋮ xi
⋮
⋯ p1j
⋮
pi1
⋯ ⋮ ⋯ ⋯ pij ⋯
⋯
⋮
⋮ ⋯
如例2.1中 如例 中,(X,Y)分布律为 分布律为
p ij
Y X 0 1 0 1/4 1/4 1 1/4 1/4
x
1 即P {X = 0, Y = 0} = = P {X = 0, Y = 1} 4 1 1 = P {X = 1, Y = 0} = P {X = 1, Y = 1} = × 2 2
1 Y = 1}P { = 1 X = 1}= × 0 = 0 4 1 2 1 Y = 1}P { = 2 X = 1}= × = 4 3 6 1 1 1 Y = 1}P { = 3 X = 1}= × = 4 3 12 2 1 1 Y = 2}P { = 1 X = 2}= × = 4 3 6 2 1 1 Y = 2}P { = 2 X = 2}= × = 4 3 6 2 1 1 Y = 2}P { = 3 X = 2}= × = 4 3 6
2、二维随机变量的分布函数
定义1.2 设(X,Y)是定义在 上的二维随 定义 是定义在 机变量, 对于任意实数x,y, 二元函数 机变量 对于任意实数 F(x,y)=P{X≤x,Y≤y} x,y∈R ≤ ≤ ∈ 称为二维随机变量 的分布函数, 称为二维随机变量(X,Y)的分布函数 或 二维随机变量 的分布函数 称为随机变量 随机变量X与 的联合分布函数 的联合分布函数。 称为随机变量 与Y的联合分布函数。