Supercapacitors and Battery Energy Management Based on New European Driving Cycle
人工智能英文参考文献(最新120个)
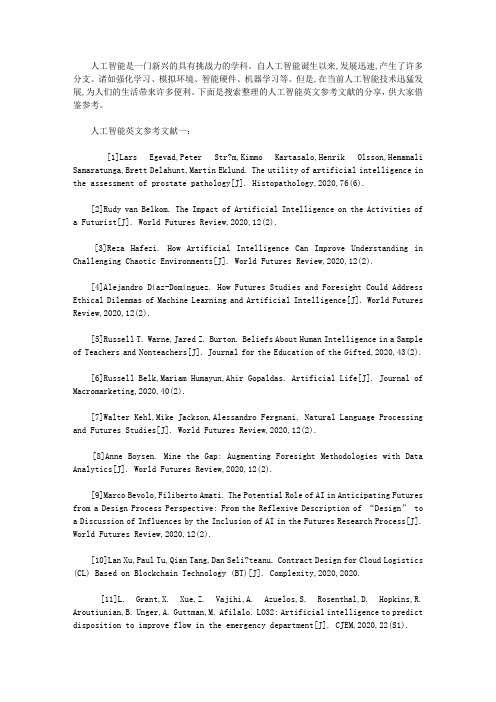
人工智能是一门新兴的具有挑战力的学科。
自人工智能诞生以来,发展迅速,产生了许多分支。
诸如强化学习、模拟环境、智能硬件、机器学习等。
但是,在当前人工智能技术迅猛发展,为人们的生活带来许多便利。
下面是搜索整理的人工智能英文参考文献的分享,供大家借鉴参考。
人工智能英文参考文献一:[1]Lars Egevad,Peter Str?m,Kimmo Kartasalo,Henrik Olsson,Hemamali Samaratunga,Brett Delahunt,Martin Eklund. The utility of artificial intelligence in the assessment of prostate pathology[J]. Histopathology,2020,76(6).[2]Rudy van Belkom. The Impact of Artificial Intelligence on the Activities ofa Futurist[J]. World Futures Review,2020,12(2).[3]Reza Hafezi. How Artificial Intelligence Can Improve Understanding in Challenging Chaotic Environments[J]. World Futures Review,2020,12(2).[4]Alejandro Díaz-Domínguez. How Futures Studies and Foresight Could Address Ethical Dilemmas of Machine Learning and Artificial Intelligence[J]. World Futures Review,2020,12(2).[5]Russell T. Warne,Jared Z. Burton. Beliefs About Human Intelligence in a Sample of Teachers and Nonteachers[J]. Journal for the Education of the Gifted,2020,43(2).[6]Russell Belk,Mariam Humayun,Ahir Gopaldas. Artificial Life[J]. Journal of Macromarketing,2020,40(2).[7]Walter Kehl,Mike Jackson,Alessandro Fergnani. Natural Language Processing and Futures Studies[J]. World Futures Review,2020,12(2).[8]Anne Boysen. Mine the Gap: Augmenting Foresight Methodologies with Data Analytics[J]. World Futures Review,2020,12(2).[9]Marco Bevolo,Filiberto Amati. The Potential Role of AI in Anticipating Futures from a Design Process Perspective: From the Reflexive Description of “Design” to a Discussion of Influences by the Inclusion of AI in the Futures Research Process[J]. World Futures Review,2020,12(2).[10]Lan Xu,Paul Tu,Qian Tang,Dan Seli?teanu. Contract Design for Cloud Logistics (CL) Based on Blockchain Technology (BT)[J]. Complexity,2020,2020.[11]L. Grant,X. Xue,Z. Vajihi,A. Azuelos,S. Rosenthal,D. Hopkins,R. Aroutiunian,B. Unger,A. Guttman,M. Afilalo. LO32: Artificial intelligence to predict disposition to improve flow in the emergency department[J]. CJEM,2020,22(S1).[12]A. Kirubarajan,A. Taher,S. Khan,S. Masood. P071: Artificial intelligence in emergency medicine: A scoping review[J]. CJEM,2020,22(S1).[13]L. Grant,P. Joo,B. Eng,A. Carrington,M. Nemnom,V. Thiruganasambandamoorthy. LO22: Risk-stratification of emergency department syncope by artificial intelligence using machine learning: human, statistics or machine[J]. CJEM,2020,22(S1).[14]Riva Giuseppe,Riva Eleonora. OS for Ind Robots: Manufacturing Robots Get Smarter Thanks to Artificial Intelligence.[J]. Cyberpsychology, behavior and social networking,2020,23(5).[15]Markus M. Obmann,Aurelio Cosentino,Joshy Cyriac,Verena Hofmann,Bram Stieltjes,Daniel T. Boll,Benjamin M. Yeh,Matthias R. Benz. Quantitative enhancement thresholds and machine learning algorithms for the evaluation of renal lesions using single-phase split-filter dual-energy CT[J]. Abdominal Radiology,2020,45(1).[16]Haytham H. Elmousalami,Mahmoud Elaskary. Drilling stuck pipe classification and mitigation in the Gulf of Suez oil fields using artificial intelligence[J]. Journal of Petroleum Exploration and Production Technology,2020,10(10).[17]Rüdiger Schulz-Wendtland,Karin Bock. Bildgebung in der Mammadiagnostik –Ein Ausblick <trans-title xml:lang="en">Imaging in breast diagnostics—an outlook [J]. Der Gyn?kologe,2020,53(6).</trans-title>[18]Nowakowski Piotr,Szwarc Krzysztof,Boryczka Urszula. Combining an artificial intelligence algorithm and a novel vehicle for sustainable e-waste collection[J]. Science of the Total Environment,2020,730.[19]Wang Huaizhi,Liu Yangyang,Zhou Bin,Li Canbing,Cao Guangzhong,Voropai Nikolai,Barakhtenko Evgeny. Taxonomy research of artificial intelligence for deterministic solar power forecasting[J]. Energy Conversion and Management,2020,214.[20]Kagemoto Hiroshi. Forecasting a water-surface wave train with artificial intelligence- A case study[J]. Ocean Engineering,2020,207.[21]Tomonori Aoki,Atsuo Yamada,Kazuharu Aoyama,Hiroaki Saito,Gota Fujisawa,Nariaki Odawara,Ryo Kondo,Akiyoshi Tsuboi,Rei Ishibashi,Ayako Nakada,Ryota Niikura,Mitsuhiro Fujishiro,Shiro Oka,Soichiro Ishihara,Tomoki Matsuda,Masato Nakahori,Shinji Tanaka,Kazuhiko Koike,Tomohiro Tada. Clinical usefulness of a deep learning‐based system as the first screening on small‐bowel capsule endoscopy reading[J]. Digestive Endoscopy,2020,32(4).[22]Masashi Fujii,Hajime Isomoto. Next generation of endoscopy: Harmony with artificial intelligence and robotic‐assisted devices[J]. Digestive Endoscopy,2020,32(4).[23]Roberto Verganti,Luca Vendraminelli,Marco Iansiti. Innovation and Design in the Age of Artificial Intelligence[J]. Journal of Product Innovation Management,2020,37(3).[24]Yuval Elbaz,David Furman,Maytal Caspary Toroker. Modeling Diffusion in Functional Materials: From Density Functional Theory to Artificial Intelligence[J]. Advanced Functional Materials,2020,30(18).[25]Dinesh Visva Gunasekeran,Tien Yin Wong. Artificial Intelligence in Ophthalmology in 2020: A Technology on the Cusp for Translation and Implementation[J]. Asia-Pacific Journal of Ophthalmology,2020,9(2).[26]Fu-Neng Jiang,Li-Jun Dai,Yong-Ding Wu,Sheng-Bang Yang,Yu-Xiang Liang,Xin Zhang,Cui-Yun Zou,Ren-Qiang He,Xiao-Ming Xu,Wei-De Zhong. The study of multiple diagnosis models of human prostate cancer based on Taylor database by artificial neural networks[J]. Journal of the Chinese Medical Association,2020,83(5).[27]Matheus Calil Faleiros,Marcello Henrique Nogueira-Barbosa,Vitor Faeda Dalto,JoséRaniery Ferreira Júnior,Ariane Priscilla Magalh?es Tenório,Rodrigo Luppino-Assad,Paulo Louzada-Junior,Rangaraj Mandayam Rangayyan,Paulo Mazzoncini de Azevedo-Marques. Machine learning techniques for computer-aided classification of active inflammatory sacroiliitis in magnetic resonance imaging[J]. Advances in Rheumatology,2020,60(1078).[28]Balamurugan Balakreshnan,Grant Richards,Gaurav Nanda,Huachao Mao,Ragu Athinarayanan,Joseph Zaccaria. PPE Compliance Detection using Artificial Intelligence in Learning Factories[J]. Procedia Manufacturing,2020,45.[29]M. Stévenin,V. Avisse,N. Ducarme,A. de Broca. Qui est responsable si un robot autonome vient à entra?ner un dommage ?[J]. Ethique et Santé,2020.[30]Fatemeh Barzegari Banadkooki,Mohammad Ehteram,Fatemeh Panahi,Saad Sh. Sammen,Faridah Binti Othman,Ahmed EL-Shafie. Estimation of Total Dissolved Solids (TDS) using New Hybrid Machine Learning Models[J]. Journal of Hydrology,2020.[31]Adam J. Schwartz,Henry D. Clarke,Mark J. Spangehl,Joshua S. Bingham,DavidA. Etzioni,Matthew R. Neville. Can a Convolutional Neural Network Classify Knee Osteoarthritis on Plain Radiographs as Accurately as Fellowship-Trained Knee Arthroplasty Surgeons?[J]. The Journal of Arthroplasty,2020.[32]Ivana Nizetic Kosovic,Toni Mastelic,Damir Ivankovic. Using Artificial Intelligence on environmental data from Internet of Things for estimating solar radiation: Comprehensive analysis[J]. Journal of Cleaner Production,2020.[33]Lauren Fried,Andrea Tan,Shirin Bajaj,Tracey N. Liebman,David Polsky,Jennifer A. Stein. Technological advances for the detection of melanoma: Part I. Advances in diagnostic techniques[J]. Journal of the American Academy of Dermatology,2020.[34]Mohammed Amoon,Torki Altameem,Ayman Altameem. Internet of things Sensor Assisted Security and Quality Analysis for Health Care Data Sets Using Artificial Intelligent Based Heuristic Health Management System[J]. Measurement,2020.[35]E. Lotan,C. Tschider,D.K. Sodickson,A. Caplan,M. Bruno,B. Zhang,Yvonne W. Lui. Medical Imaging and Privacy in the Era of Artificial Intelligence: Myth, Fallacy, and the Future[J]. Journal of the American College of Radiology,2020.[36]Fabien Lareyre,Cédric Adam,Marion Carrier,Juliette Raffort. Artificial Intelligence in Vascular Surgery: moving from Big Data to Smart Data[J]. Annals of Vascular Surgery,2020.[37]Ilesanmi Daniyan,Khumbulani Mpofu,Moses Oyesola,Boitumelo Ramatsetse,Adefemi Adeodu. Artificial intelligence for predictive maintenance in the railcar learning factories[J]. Procedia Manufacturing,2020,45.[38]Janet L. McCauley,Anthony E. Swartz. Reframing Telehealth[J]. Obstetrics and Gynecology Clinics of North America,2020.[39]Jean-Emmanuel Bibault,Lei Xing. Screening for chronic obstructive pulmonary disease with artificial intelligence[J]. The Lancet Digital Health,2020,2(5).[40]Andrea Laghi. Cautions about radiologic diagnosis of COVID-19 infection driven by artificial intelligence[J]. The Lancet Digital Health,2020,2(5).人工智能英文参考文献二:[41]K. Orhan,I. S. Bayrakdar,M. Ezhov,A. Kravtsov,T. ?zyürek. Evaluation of artificial intelligence for detecting periapical pathosis on cone‐beam computed tomography scans[J]. International Endodontic Journal,2020,53(5).[42]Avila A M,Mezi? I. Data-driven analysis and forecasting of highway traffic dynamics.[J]. Nature communications,2020,11(1).[43]Neri Emanuele,Miele Vittorio,Coppola Francesca,Grassi Roberto. Use of CT andartificial intelligence in suspected or COVID-19 positive patients: statement of the Italian Society of Medical and Interventional Radiology.[J]. La Radiologia medica,2020.[44]Tau Noam,Stundzia Audrius,Yasufuku Kazuhiro,Hussey Douglas,Metser Ur. Convolutional Neural Networks in Predicting Nodal and Distant Metastatic Potential of Newly Diagnosed Non-Small Cell Lung Cancer on FDG PET Images.[J]. AJR. American journal of roentgenology,2020.[45]Coppola Francesca,Faggioni Lorenzo,Regge Daniele,Giovagnoni Andrea,Golfieri Rita,Bibbolino Corrado,Miele Vittorio,Neri Emanuele,Grassi Roberto. Artificial intelligence: radiologists' expectations and opinions gleaned from a nationwide online survey.[J]. La Radiologia medica,2020.[46]?. ? ? ? ? [J]. ,2020,25(4).[47]Savage Rock H,van Assen Marly,Martin Simon S,Sahbaee Pooyan,Griffith Lewis P,Giovagnoli Dante,Sperl Jonathan I,Hopfgartner Christian,K?rgel Rainer,Schoepf U Joseph. Utilizing Artificial Intelligence to Determine Bone Mineral Density Via Chest Computed Tomography.[J]. Journal of thoracic imaging,2020,35 Suppl 1.[48]Brzezicki Maksymilian A,Bridger Nicholas E,Kobeti? Matthew D,Ostrowski Maciej,Grabowski Waldemar,Gill Simran S,Neumann Sandra. Artificial intelligence outperforms human students in conducting neurosurgical audits.[J]. Clinical neurology and neurosurgery,2020,192.[49]Lockhart Mark E,Smith Andrew D. Fatty Liver Disease: Artificial Intelligence Takes on the Challenge.[J]. Radiology,2020,295(2).[50]Wood Edward H,Korot Edward,Storey Philip P,Muscat Stephanie,Williams George A,Drenser Kimberly A. The retina revolution: signaling pathway therapies, genetic therapies, mitochondrial therapies, artificial intelligence.[J]. Current opinion in ophthalmology,2020,31(3).[51]Ho Dean,Quake Stephen R,McCabe Edward R B,Chng Wee Joo,Chow Edward K,Ding Xianting,Gelb Bruce D,Ginsburg Geoffrey S,Hassenstab Jason,Ho Chih-Ming,Mobley William C,Nolan Garry P,Rosen Steven T,Tan Patrick,Yen Yun,Zarrinpar Ali. Enabling Technologies for Personalized and Precision Medicine.[J]. Trends in biotechnology,2020,38(5).[52]Fischer Andreas M,Varga-Szemes Akos,van Assen Marly,Griffith L Parkwood,Sahbaee Pooyan,Sperl Jonathan I,Nance John W,Schoepf U Joseph. Comparison of Artificial Intelligence-Based Fully Automatic Chest CT Emphysema Quantification to Pulmonary Function Testing.[J]. AJR. American journal ofroentgenology,2020,214(5).[53]Moore William,Ko Jane,Gozansky Elliott. Artificial Intelligence Pertaining to Cardiothoracic Imaging and Patient Care: Beyond Image Interpretation.[J]. Journal of thoracic imaging,2020,35(3).[54]Hwang Eui Jin,Park Chang Min. Clinical Implementation of Deep Learning in Thoracic Radiology: Potential Applications and Challenges.[J]. Korean journal of radiology,2020,21(5).[55]Mateen Bilal A,David Anna L,Denaxas Spiros. Electronic Health Records to Predict Gestational Diabetes Risk.[J]. Trends in pharmacological sciences,2020,41(5).[56]Yao Xiang,Mao Ling,Lv Shunli,Ren Zhenghong,Li Wentao,Ren Ke. CT radiomics features as a diagnostic tool for classifying basal ganglia infarction onset time.[J]. Journal of the neurological sciences,2020,412.[57]van Assen Marly,Banerjee Imon,De Cecco Carlo N. Beyond the Artificial Intelligence Hype: What Lies Behind the Algorithms and What We Can Achieve.[J]. Journal of thoracic imaging,2020,35 Suppl 1.[58]Guzik Tomasz J,Fuster Valentin. Leaders in Cardiovascular Research: Valentin Fuster.[J]. Cardiovascular research,2020,116(6).[59]Fischer Andreas M,Eid Marwen,De Cecco Carlo N,Gulsun Mehmet A,van Assen Marly,Nance John W,Sahbaee Pooyan,De Santis Domenico,Bauer Maximilian J,Jacobs Brian E,Varga-Szemes Akos,Kabakus Ismail M,Sharma Puneet,Jackson Logan J,Schoepf U Joseph. Accuracy of an Artificial Intelligence Deep Learning Algorithm Implementing a Recurrent Neural Network With Long Short-term Memory for the Automated Detection of Calcified Plaques From Coronary Computed Tomography Angiography.[J]. Journal of thoracic imaging,2020,35 Suppl 1.[60]Ghosh Adarsh,Kandasamy Devasenathipathy. Interpretable Artificial Intelligence: Why and When.[J]. AJR. American journal of roentgenology,2020,214(5).[61]M.Rosario González-Rodríguez,M.Carmen Díaz-Fernández,Carmen Pacheco Gómez. Facial-expression recognition: An emergent approach to the measurement of tourist satisfaction through emotions[J]. Telematics and Informatics,2020,51.[62]Ru-Xi Ding,Iván Palomares,Xueqing Wang,Guo-Rui Yang,Bingsheng Liu,Yucheng Dong,Enrique Herrera-Viedma,Francisco Herrera. Large-Scale decision-making: Characterization, taxonomy, challenges and future directions from an Artificial Intelligence and applications perspective[J]. Information Fusion,2020,59.[63]Abdulrhman H. Al-Jebrni,Brendan Chwyl,Xiao Yu Wang,Alexander Wong,Bechara J. Saab. AI-enabled remote and objective quantification of stress at scale[J]. Biomedical Signal Processing and Control,2020,59.[64]Gillian Thomas,Elizabeth Eisenhauer,Robert G. Bristow,Cai Grau,Coen Hurkmans,Piet Ost,Matthias Guckenberger,Eric Deutsch,Denis Lacombe,Damien C. Weber. The European Organisation for Research and Treatment of Cancer, State of Science in radiation oncology and priorities for clinical trials meeting report[J]. European Journal of Cancer,2020,131.[65]Muhammad Asif. Are QM models aligned with Industry 4.0? A perspective on current practices[J]. Journal of Cleaner Production,2020,258.[66]Siva Teja Kakileti,Himanshu J. Madhu,Geetha Manjunath,Leonard Wee,Andre Dekker,Sudhakar Sampangi. Personalized risk prediction for breast cancer pre-screening using artificial intelligence and thermal radiomics[J]. Artificial Intelligence In Medicine,2020,105.[67]. Evaluation of Payer Budget Impact Associated with the Use of Artificial Intelligence in Vitro Diagnostic, Kidneyintelx, to Modify DKD Progression:[J]. American Journal of Kidney Diseases,2020,75(5).[68]Rohit Nishant,Mike Kennedy,Jacqueline Corbett. Artificial intelligence for sustainability: Challenges, opportunities, and a research agenda[J]. International Journal of Information Management,2020,53.[69]Hoang Nguyen,Xuan-Nam Bui. Soft computing models for predicting blast-induced air over-pressure: A novel artificial intelligence approach[J]. Applied Soft Computing Journal,2020,92.[70]Benjamin S. Hopkins,Aditya Mazmudar,Conor Driscoll,Mark Svet,Jack Goergen,Max Kelsten,Nathan A. Shlobin,Kartik Kesavabhotla,Zachary A Smith,Nader S Dahdaleh. Using artificial intelligence (AI) to predict postoperative surgical site infection: A retrospective cohort of 4046 posterior spinal fusions[J]. Clinical Neurology and Neurosurgery,2020,192.[71]Mei Yang,Runze Zhou,Xiangjun Qiu,Xiangfei Feng,Jian Sun,Qunshan Wang,Qiufen Lu,Pengpai Zhang,Bo Liu,Wei Li,Mu Chen,Yan Zhao,Binfeng Mo,Xin Zhou,Xi Zhang,Yingxue Hua,Jin Guo,Fangfang Bi,Yajun Cao,Feng Ling,Shengming Shi,Yi-Gang Li. Artificial intelligence-assisted analysis on the association between exposure to ambient fine particulate matter and incidence of arrhythmias in outpatients of Shanghai community hospitals[J]. Environment International,2020,139.[72]Fatemehalsadat Madaeni,Rachid Lhissou,Karem Chokmani,Sebastien Raymond,Yves Gauthier. Ice jam formation, breakup and prediction methods based on hydroclimatic data using artificial intelligence: A review[J]. Cold Regions Science and Technology,2020,174.[73]Steve Chukwuebuka Arum,David Grace,Paul Daniel Mitchell. A review of wireless communication using high-altitude platforms for extended coverage and capacity[J]. Computer Communications,2020,157.[74]Yong-Hong Kuo,Nicholas B. Chan,Janny M.Y. Leung,Helen Meng,Anthony Man-Cho So,Kelvin K.F. Tsoi,Colin A. Graham. An Integrated Approach of Machine Learning and Systems Thinking for Waiting Time Prediction in an Emergency Department[J]. International Journal of Medical Informatics,2020,139.[75]Matteo Terzi,Gian Antonio Susto,Pratik Chaudhari. Directional adversarial training for cost sensitive deep learning classification applications[J]. Engineering Applications of Artificial Intelligence,2020,91.[76]Arman Kilic. Artificial Intelligence and Machine Learning in Cardiovascular Health Care[J]. The Annals of Thoracic Surgery,2020,109(5).[77]Hossein Azarmdel,Ahmad Jahanbakhshi,Seyed Saeid Mohtasebi,Alfredo Rosado Mu?oz. Evaluation of image processing technique as an expert system in mulberry fruit grading based on ripeness level using artificial neural networks (ANNs) and support vector machine (SVM)[J]. Postharvest Biology and Technology,2020,166.[78]Wafaa Wardah,Abdollah Dehzangi,Ghazaleh Taherzadeh,Mahmood A. Rashid,M.G.M. Khan,Tatsuhiko Tsunoda,Alok Sharma. Predicting protein-peptide binding sites with a deep convolutional neural network[J]. Journal of Theoretical Biology,2020,496.[79]Francisco F.X. Vasconcelos,Róger M. Sarmento,Pedro P. Rebou?as Filho,Victor Hugo C. de Albuquerque. Artificial intelligence techniques empowered edge-cloud architecture for brain CT image analysis[J]. Engineering Applications of Artificial Intelligence,2020,91.[80]Masaaki Konishi. Bioethanol production estimated from volatile compositions in hydrolysates of lignocellulosic biomass by deep learning[J]. Journal of Bioscience and Bioengineering,2020,129(6).人工智能英文参考文献三:[81]J. Kwon,K. Kim. Artificial Intelligence for Early Prediction of Pulmonary Hypertension Using Electrocardiography[J]. Journal of Heart and Lung Transplantation,2020,39(4).[82]C. Maathuis,W. Pieters,J. van den Berg. Decision support model for effects estimation and proportionality assessment for targeting in cyber operations[J]. Defence Technology,2020.[83]Samer Ellahham. Artificial Intelligence in Diabetes Care[J]. The American Journal of Medicine,2020.[84]Yi-Ting Hsieh,Lee-Ming Chuang,Yi-Der Jiang,Tien-Jyun Chang,Chung-May Yang,Chang-Hao Yang,Li-Wei Chan,Tzu-Yun Kao,Ta-Ching Chen,Hsuan-Chieh Lin,Chin-Han Tsai,Mingke Chen. Application of deep learning image assessment software VeriSee? for diabetic retinopathy screening[J]. Journal of the Formosan Medical Association,2020.[85]Emre ARTUN,Burak KULGA. Selection of candidate wells for re-fracturing in tight gas sand reservoirs using fuzzy inference[J]. Petroleum Exploration and Development Online,2020,47(2).[86]Alberto Arenal,Cristina Armu?a,Claudio Feijoo,Sergio Ramos,Zimu Xu,Ana Moreno. Innovation ecosystems theory revisited: The case of artificial intelligence in China[J]. Telecommunications Policy,2020.[87]T. Som,M. Dwivedi,C. Dubey,A. Sharma. Parametric Studies on Artificial Intelligence Techniques for Battery SOC Management and Optimization of Renewable Power[J]. Procedia Computer Science,2020,167.[88]Bushra Kidwai,Nadesh RK. Design and Development of Diagnostic Chabot for supporting Primary Health Care Systems[J]. Procedia Computer Science,2020,167.[89]Asl? Bozda?,Ye?im Dokuz,?znur Begüm G?k?ek. Spatial prediction of PM 10 concentration using machine learning algorithms in Ankara, Turkey[J]. Environmental Pollution,2020.[90]K.P. Smith,J.E. Kirby. Image analysis and artificial intelligence in infectious disease diagnostics[J]. Clinical Microbiology and Infection,2020.[91]Alklih Mohamad YOUSEF,Ghahfarokhi Payam KAVOUSI,Marwan ALNUAIMI,Yara ALATRACH. Predictive data analytics application for enhanced oil recovery in a mature field in the Middle East[J]. Petroleum Exploration and Development Online,2020,47(2).[92]Omer F. Ahmad,Danail Stoyanov,Laurence B. Lovat. Barriers and pitfalls for artificial intelligence in gastroenterology: Ethical and regulatory issues[J]. Techniques and Innovations in Gastrointestinal Endoscopy,2020,22(2).[93]Sanne A. Hoogenboom,Ulas Bagci,Michael B. Wallace. Artificial intelligence in gastroenterology. The current state of play and the potential. How will it affect our practice and when?[J]. Techniques and Innovations in Gastrointestinal Endoscopy,2020,22(2).[94]Douglas K. Rex. Can we do resect and discard with artificial intelligence-assisted colon polyp “optical biopsy?”[J]. Techniques and Innovations in Gastrointestinal Endoscopy,2020,22(2).[95]Neal Shahidi,Michael J. Bourke. Can artificial intelligence accurately diagnose endoscopically curable gastrointestinal cancers?[J]. Techniques and Innovations in Gastrointestinal Endoscopy,2020,22(2).[96]Michael Byrne. Artificial intelligence in gastroenterology[J]. Techniques and Innovations in Gastrointestinal Endoscopy,2020,22(2).[97]Piet C. de Groen. Using artificial intelligence to improve adequacy of inspection in gastrointestinal endoscopy[J]. Techniques and Innovations in Gastrointestinal Endoscopy,2020,22(2).[98]Robin Zachariah,Andrew Ninh,William Karnes. Artificial intelligence for colon polyp detection: Why should we embrace this?[J]. Techniques and Innovations in Gastrointestinal Endoscopy,2020,22(2).[99]Alexandra T. Greenhill,Bethany R. Edmunds. A primer of artificial intelligence in medicine[J]. Techniques and Innovations in Gastrointestinal Endoscopy,2020,22(2).[100]Tomohiro Tada,Toshiaki Hirasawa,Toshiyuki Yoshio. The role for artificial intelligence in evaluation of upper GI cancer[J]. Techniques and Innovations in Gastrointestinal Endoscopy,2020,22(2).[101]Yahui Jiang,Meng Yang,Shuhao Wang,Xiangchun Li,Yan Sun. Emerging role of deep learning‐based artificial intelligence in tumor pathology[J]. Cancer Communications,2020,40(4).[102]Kristopher D. Knott,Andreas Seraphim,Joao B. Augusto,Hui Xue,Liza Chacko,Nay Aung,Steffen E. Petersen,Jackie A. Cooper,Charlotte Manisty,Anish N. Bhuva,Tushar Kotecha,Christos V. Bourantas,Rhodri H. Davies,Louise A.E. Brown,Sven Plein,Marianna Fontana,Peter Kellman,James C. Moon. The Prognostic Significance of Quantitative Myocardial Perfusion: An Artificial Intelligence–Based Approach Using Perfusion Mapping[J]. Circulation,2020,141(16).[103]Muhammad Asad,Ahmed Moustafa,Takayuki Ito. FedOpt: Towards Communication Efficiency and Privacy Preservation in Federated Learning[J]. Applied Sciences,2020,10(8).[104]Wu Wenzhi,Zhang Yan,Wang Pu,Zhang Li,Wang Guixiang,Lei Guanghui,Xiao Qiang,Cao Xiaochen,Bian Yueran,Xie Simiao,Huang Fei,Luo Na,Zhang Jingyuan,Luo Mingyan. Psychological stress of medical staffs during outbreak of COVID-19 and adjustment strategy.[J]. Journal of medical virology,2020.[105]. Eyenuk Fulfills Contract for Artificial Intelligence Grading of Retinal Images[J]. Telecomworldwire,2020.[106]Kim Tae Woo,Duhachek Adam. Artificial Intelligence and Persuasion: A Construal-Level Account.[J]. Psychological science,2020,31(4).[107]McCall Becky. COVID-19 and artificial intelligence: protecting health-care workers and curbing the spread.[J]. The Lancet. Digital health,2020,2(4).[108]Alca?iz Mariano,Chicchi Giglioli Irene A,Sirera Marian,Minissi Eleonora,Abad Luis. [Autism spectrum disorder biomarkers based on biosignals, virtual reality and artificial intelligence].[J]. Medicina,2020,80 Suppl 2.[109]Cong Lei,Feng Wanbing,Yao Zhigang,Zhou Xiaoming,Xiao Wei. Deep Learning Model as a New Trend in Computer-aided Diagnosis of Tumor Pathology for Lung Cancer.[J]. Journal of Cancer,2020,11(12).[110]Wang Fengdan,Gu Xiao,Chen Shi,Liu Yongliang,Shen Qing,Pan Hui,Shi Lei,Jin Zhengyu. Artificial intelligence system can achieve comparable results to experts for bone age assessment of Chinese children with abnormal growth and development.[J]. PeerJ,2020,8.[111]Hu Wenmo,Yang Huayu,Xu Haifeng,Mao Yilei. Radiomics based on artificial intelligence in liver diseases: where we are?[J]. Gastroenterology report,2020,8(2).[112]Batayneh Wafa,Abdulhay Enas,Alothman Mohammad. Prediction of the performance of artificial neural networks in mapping sEMG to finger joint angles via signal pre-investigation techniques.[J]. Heliyon,2020,6(4).[113]Aydin Emrah,Türkmen ?nan Utku,Namli G?zde,?ztürk ?i?dem,Esen Ay?e B,Eray Y Nur,Ero?lu Egemen,Akova Fatih. A novel and simple machine learning algorithm for preoperative diagnosis of acute appendicitis in children.[J]. Pediatric surgery international,2020.[114]Ellahham Samer. Artificial Intelligence in Diabetes Care.[J]. The Americanjournal of medicine,2020.[115]David J. Winkel,Thomas J. Weikert,Hanns-Christian Breit,Guillaume Chabin,Eli Gibson,Tobias J. Heye,Dorin Comaniciu,Daniel T. Boll. Validation of a fully automated liver segmentation algorithm using multi-scale deep reinforcement learning and comparison versus manual segmentation[J]. European Journal of Radiology,2020,126.[116]Binjie Fu,Guoshu Wang,Mingyue Wu,Wangjia Li,Yineng Zheng,Zhigang Chu,Fajin Lv. Influence of CT effective dose and convolution kernel on the detection of pulmonary nodules in different artificial intelligence software systems: A phantom study[J]. European Journal of Radiology,2020,126.[117]Georgios N. Kouziokas. A new W-SVM kernel combining PSO-neural network transformed vector and Bayesian optimized SVM in GDP forecasting[J]. Engineering Applications of Artificial Intelligence,2020,92.[118]Qingsong Ruan,Zilin Wang,Yaping Zhou,Dayong Lv. A new investor sentiment indicator ( ISI ) based on artificial intelligence: A powerful return predictor in China[J]. Economic Modelling,2020,88.[119]Mohamed Abdel-Basset,Weiping Ding,Laila Abdel-Fatah. The fusion of Internet of Intelligent Things (IoIT) in remote diagnosis of obstructive Sleep Apnea: A survey and a new model[J]. Information Fusion,2020,61.[120]Federico Caobelli. Artificial intelligence in medical imaging: Game over for radiologists?[J]. European Journal of Radiology,2020,126.以上就是关于人工智能参考文献的分享,希望对你有所帮助。
钴、氮共掺杂碳纳米复合材料的制备及其在锂硫电池中的应用
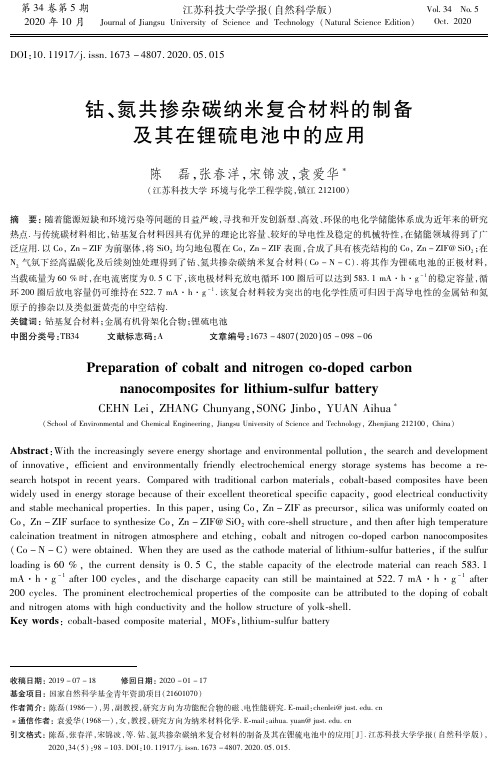
第34卷第5期2020年10月 江苏科技大学学报(自然科学版)JournalofJiangsuUniversityofScienceandTechnology(NaturalScienceEdition) Vol 34No 5Oct.2020 DOI:10.11917/j.issn.1673-4807.2020.05.015钴、氮共掺杂碳纳米复合材料的制备及其在锂硫电池中的应用陈 磊,张春洋,宋锦波,袁爱华(江苏科技大学环境与化学工程学院,镇江212100)摘 要:随着能源短缺和环境污染等问题的日益严峻,寻找和开发创新型、高效、环保的电化学储能体系成为近年来的研究热点.与传统碳材料相比,钴基复合材料因具有优异的理论比容量、较好的导电性及稳定的机械特性,在储能领域得到了广泛应用.以Co,Zn-ZIF为前驱体,将SiO2均匀地包覆在Co,Zn-ZIF表面,合成了具有核壳结构的Co,Zn-ZIF@SiO2;在N2气氛下经高温碳化及后续刻蚀处理得到了钴、氮共掺杂碳纳米复合材料(Co-N-C).将其作为锂硫电池的正极材料,当载硫量为60%时,在电流密度为0 5C下,该电极材料充放电循环100圈后可以达到583 1mA·h·g-1的稳定容量,循环200圈后放电容量仍可维持在522 7mA·h·g-1.该复合材料较为突出的电化学性质可归因于高导电性的金属钴和氮原子的掺杂以及类似蛋黄壳的中空结构.关键词:钴基复合材料;金属有机骨架化合物;锂硫电池中图分类号:TB34 文献标志码:A 文章编号:1673-4807(2020)05-098-06收稿日期:2019-07-18 修回日期:2020-01-17基金项目:国家自然科学基金青年资助项目(21601070)作者简介:陈磊(1986—),男,副教授,研究方向为功能配合物的磁、电性能研究.E mail:chenlei@just.edu.cn 通信作者:袁爱华(1968—),女,教授,研究方向为纳米材料化学.E mail:aihua.yuan@just.edu.cn引文格式:陈磊,张春洋,宋锦波,等.钴、氮共掺杂碳纳米复合材料的制备及其在锂硫电池中的应用[J].江苏科技大学学报(自然科学版),2020,34(5):98-103.DOI:10.11917/j.issn.1673-4807.2020.05.015.Preparationofcobaltandnitrogenco dopedcarbonnanocompositesforlithium sulfurbatteryCEHNLei,ZHANGChunyang,SONGJinbo,YUANAihua(SchoolofEnvironmentalandChemicalEngineering,JiangsuUniversityofScienceandTechnology,Zhenjiang212100,China)Abstract:Withtheincreasinglysevereenergyshortageandenvironmentalpollution,thesearchanddevelopmentofinnovative,efficientandenvironmentallyfriendlyelectrochemicalenergystoragesystemshasbecomearesearchhotspotinrecentyears.Comparedwithtraditionalcarbonmaterials,cobalt basedcompositeshavebeenwidelyusedinenergystoragebecauseoftheirexcellenttheoreticalspecificcapacity,goodelectricalconductivityandstablemechanicalproperties.Inthispaper,usingCo,Zn-ZIFasprecursor,silicawasuniformlycoatedonCo,Zn-ZIFsurfacetosynthesizeCo,Zn-ZIF@SiO2withcore shellstructure,andthenafterhightemperaturecalcinationtreatmentinnitrogenatmosphereandetching,cobaltandnitrogenco dopedcarbonnanocomposites(Co-N-C)wereobtained.Whentheyareusedasthecathodematerialoflithium sulfurbatteries,ifthesulfurloadingis60%,thecurrentdensityis0.5C,thestablecapacityoftheelectrodematerialcanreach583 1mA·h·g-1after100cycles,andthedischargecapacitycanstillbemaintainedat522 7mA·h·g-1after200cycles.Theprominentelectrochemicalpropertiesofthecompositecanbeattributedtothedopingofcobaltandnitrogenatomswithhighconductivityandthehollowstructureofyolk shell.Keywords:cobalt basedcompositematerial,MOFs,lithium sulfurbattery 为了满足日益增长的能源需求和大型储能设备市场的应用,人们对长寿命、高能量密度的可充电锂离子电池(LIBs)提出了更高的要求.在各类可充电电池系统中,锂硫电池因其高理论容量(1675mA·h·g-1)和能量密度(2600W·h·kg-1)而备受关注[1].在锂电池材料中,硫正极材料的含量丰富、成本低廉和环保型使LIBs在商业上具有更大的竞争力.但是,锂硫电池具有低实际容量、快速的容量衰减和低库仑效率等缺点.另外,硫及其放电产物的绝缘性会限制了硫的电化学利用,而且生成的中间多硫化物容易扩散到电解液中,导致绝缘性差以及较低的硫的利用率,从而在充放电过程中产生穿梭效应[2].为了解决上述问题,国内外研究者采取了各种方法来减少穿梭效应,包括开发正极材料、电解质和保护阳极的思路,以提高锂电池的整体性能.目前最有前景的方法是将硫与各种多孔碳基质高效结合,包括微/介孔碳、多孔空心碳纳米球、碳纳米纤维/纳米管和石墨烯等.具有高表面积的多孔碳可以提供大的孔体积来封装硫和作为电子传输的导电网络将多硫化物中间产物困与孔内.文献[3]使用ZIF-8衍生的微孔碳多面体作为载硫基质,其初始容量高达1500mA·h·g-1.文献[4]通过在氧化石墨烯上原位生长ZIF-8和ZIF-67,在高温处理后形成氮掺杂多孔碳/石墨烯(NPC/G)混合物.高导电石墨烯不仅提供了一个相互连接的导电框架,以促进快速的电子传输,而且作为一个建筑单元以支撑金属有机骨架材料(MOF)衍生的碳.由于多孔碳具有丰富的孔结构和氮掺杂特性,使其对多硫化物具有物理限制和化学吸附两种性质.将其作为锂硫电池正极材料,循环超过300次仍能维持良好的稳定性,比容量高达1372mA·h·g-1,说明MOF衍生碳材料和石墨烯复合结构的设计可以提高锂硫电池的电性能.最近有报道称,导电金属具有高效的聚硫介质,能够影响表面聚硫穿梭过程,从而增强氧化还原化活性.因此当导电金属被用于锂硫电池时具有较好的循环稳定性.文献[5]合成了一种含有钴和氮掺杂石墨碳的ZIF-67衍生硫宿主,作为高效基质来截存多硫化物,在大电流下500圈循环后仍具有良好的循环稳定性.文献[6]通过回流法制备了新型双金属Zn,Co-MOF-5,将其碳化转化为具有较大表面积的钴@石墨碳多孔复合材料(Co@GC-PC).Co@GC-PC具有较大的表面积和足够的介孔,使其能够吸附多硫化物,而电子传导则来源于分布良好的钴和石墨碳.密度泛函理论计算也进一步表明,钴单质促进了硫化物的分解.当其作为硫的载体时,在0 2C(1C=1675mA·h·g-1)的电流密度下,经过220圈循环后仍能维持高可逆容量(790mA·h·g-1).文中利用Co,Zn-ZIF前驱体和二氧化硅保护煅烧策略合成了钴、氮共掺杂碳纳米复合材料(Co-N-C).在合成的过程中(图1),先将Co,Zn-ZIF前驱体表面包覆二氧化硅后再进行高温热解,在氮气氛围下高温(900℃)碳化处理,单质锌将随之挥发.同时,其包覆MOF的方法可以有效防止高温条件下产物的聚集.最后,通过氢氟酸刻蚀表面的二氧化硅和裸露在外面未被保护的钴单质,进而制备Co-N-C并将该材料作为硫的载体.S/Co-N-C的复合物在应用为锂硫电池的正极材料时,显示出优异的电化学性能.图1 基于SiO2保护煅烧策略的Co-N-C合成示意Fig.1 SyntheticprocedureoftheCo-N-CbytheSiO2-protectedcalcinationstrategy1 实验1 1 试剂硝酸钴,硝酸锌,2-甲基咪唑,无水甲醇,十六烷基三甲基溴化铵,硅酸四乙酯(上海萨恩化学技术有限公司,分析纯);氢氧化钠,氢氟酸,无水乙醇(国药集团有限公司,分析纯);聚偏氟乙烯(PVDF);双三氟甲基磺酸亚酰胺锂(LiTFSI);去离子水.1 2 材料制备1 2 1 Co,Zn-ZIF的合成将16mmol的Co(NO3)2·6H2O和16mmol的Zn(NO3)2·6H2O溶解在200mL的无水甲醇中,将128mmol的2-甲基咪唑溶解于200mL的无水甲醇中,分别搅拌30min.然后将金属盐溶液缓慢添加到2-甲基咪唑溶液中,室温搅拌4h.通过离心收集产物并用无水甲醇清洗.1 2 2 Co,Zn-ZIF@SiO2的合成将所得样品(300mg)超声分散在120mL的H2O中,搅拌30min后加入75mg的十六烷基三甲基溴化铵和30mg的氢氧化钠.然后继续搅拌30min,将0 6mL的硅酸四乙酯逐滴加入上述溶液中,反应1h后迅速离心.产物用水和无水乙醇99第5期 陈磊,等:钴、氮共掺杂碳纳米复合材料的制备及其在锂硫电池中的应用分别清洗3次.1 2 3 Co-N-C的合成将干燥好的样品置于管式炉中,在氮气氛围下,以1℃/min的加热速率缓慢升至900℃并维持4h.将所得到的黑色产物Co-N-C@SiO2超声分散在氢氟酸(5%)的水溶液中刻蚀6h,用来去除二氧化硅以及表面未被保护的钴单质.用大量的水和无水乙醇润洗Co-N-C,抽滤至中性,烘干待用.1 2 4 S/Co-N-C的合成将Co-N-C与硫单质按质量比4∶6进行混合,在玛瑙研钵中充分研磨30min使其混合均匀,然后转移至密闭的小瓶中155℃加热12h,获得S/Co-N-C.1 3 材料表征采用X射线衍射仪(XRD,Cu靶(λ=1 5418),U=40kV,I=30mA)测定产物的物质结构,扫描角度为10~80°之间,扫率为5°/min.用场发射扫描电子显微镜(SEM)和透射电子显微镜(TEM)分别观察表面形貌和微观结构.X射线光电子能谱(XPS)用来分析样品组成和价态结构.使用热重分析仪器(TG)分析产物的热分解行为,测试温度为室温~600℃之间,升温速度10℃/min,在氮气氛围下进行.1 4 电化学性能测试将S/Co-N-C样品与科琴黑和PVDF在8∶1∶1的质量比混合均匀,与N-甲基吡咯烷酮(NMP)为分散剂,混合成粘度适当的浆料.随后把浆料均匀的涂在铝箔上,并在真空干燥箱中55℃下干燥.利用打孔机将铝箔裁成圆片电极( =12mm),组装成纽扣式半电池,该半电池组装在充满氩气的手套箱中,水和氧浓度均低于1×10-6.以金属锂作为对电极,Celgard2400薄膜作为隔膜,电解液由1 0mol/LLiTFSI的乙二醇二甲醚(DME)和1,3-二氧戊环(DOL)溶液组成(体积比为1∶1,其中含有0 2%的添加剂LiNO3).将组装完的电池静置16h后待测,在1 7~2 8V(相对于Li/Li+)的电压范围内,通过蓝电测试系统(LANDCT-2001A)进行恒流充放电测试.所有的容量都是根据正极材料的硫质量来计算.2 结果与讨论2 1 Co-N-C和S/Co-N-C的物相与结构分析 图2为Co,Zn-ZIF和Co,Zn-ZIF@SiO2的XRD图谱.Co,Zn-ZIF的特征峰比较尖锐,表明成功合成了高结晶度的MOF,与文献[7]报道的图谱相吻合.经过正硅酸四乙酯在碱性条件下的水解缩合,由图可以看出包覆完二氧化硅后的XRD图仍呈现的是Co,Zn-ZIF的衍射峰,这主要是由于二氧化硅是无定型材料.图3为单质硫、Co-N-C以及S/Co-N-C的XRD图谱.Co-N-C的衍射峰在2θ=26°和45°处有两个明显的宽峰,对应于石墨碳的(002)和(100)晶面[8].S/Co-N-C和单质硫具有相同的物相,复合物中所有的衍射峰与单质硫粉的衍射峰相匹配.图2 Co,Zn-ZIF与Co,Zn-ZIF@SiO2的XRD图谱Fig.2 XRDpatternsofCo,Zn-ZIFandCo,Zn-ZIF@SiO2图3 单质硫粉、Co-N-C以及S/Co-N-C的XRD图谱Fig.3 XRDpatternsofsulfur,Co-N-CandS/Co-N-C图4为所制备样品的扫描电镜和透射电镜图片以及元素分析.图4 样品的扫描电镜和透射电镜图片以及元素分析Fig.4 SEMandTEMimagesofthesamplesandtheelementalmappingdistributionofS/Co-N-C如图4(a)中,Co,Zn-ZIF显示出均匀的菱形多面体形貌和光滑的颗粒表面.从透射图片(图4(b))可以看出其平均尺寸约为100nm.通过二氧001江苏科技大学学报(自然科学版)2020年化硅的包覆之后,Co,Zn-ZIF@SiO2没有发生结构的变化,仍然维持着原有的颗粒感,显示出核壳结构且表面趋于球形形貌,外壳的厚度约为10nm(图4(c)、(d)和(f)).随后在惰性气体下高温碳化和刻蚀后将Co,Zn-ZIF@SiO2转化为Co-N-C,由图4(e)的扫描图片可以看出前驱体经过煅烧处理以及除去二氧化硅后保持着较好的分散性且大小均一.从图4(f)的透射电镜图像可以看出Co-N-C仍保持完整的Co,Zn-ZIF骨架结构.值得注意的是该材料转化为类似蛋黄壳结构,这种中空结构更有利于较大的体积进行载硫,其平均粒径约为100nm.图4(g)是S/Co-N-C的元素分布图,从图中可以看出该样品均匀分布着C、Co、N、S4种元素,进一步证实成功制备了该复合材料.利用X射线光电子能谱(XPS)分析了S/Co-N-C的表面化学组成.从全谱图中(图5(a))表明主要元素为钴、氧、氮、碳、硫.钴的特征峰并不是很清晰,这是由于大部分钴都处于碳基体的内部且含量较少.Co2p的精细谱中位于780 8eV和795 9eV处的两个特征峰归因于金属钴[9],如图5(b).图5(c)中N1s的精细谱可分成3个组分,包括吡啶氮(398 5eV)、吡咯氮(400 0eV)、石墨化氮(400 7eV),其中吡咯型氮和吡啶型氮主要的作用来束缚多硫化锂来减小穿梭效应,进而提高锂硫电池的性能[10].S2p通过分峰拟合可以分成3个峰(图5(d)),S2p3/2和S2p1/2的组分与S-S物种的存在有关,它们的结合能分别为163 8eV和165 0eV.168 6eV处出现的宽峰与硫酸盐物种有关[11-12].图5 S/Co-N-C的XPS图谱Fig.5 XPSspectraoftheS/Co-N-C图6为S/Co-N-C复合材料在氮气氛围下,以10℃/min的加热速率下所测试的热重曲线.从图可以看出在150~280℃之间有着明显的质量损失,这主要归因于大孔和表面硫的蒸发.在280~400℃之间也有较小的质量损失平台,这主要是由于内部或者较小孔内硫分子的蒸发.数据结果进一步表明其载硫量为60%[13].图6 S/Co-N-C复合材料的热重曲线Fig.6 TGAcurveofS/Co-N-Ccomposites2 2 电化学性能分析将S/Co-N-C复合材料作为锂硫电池的正极材料,平均载硫量为1 2mg·cm-2,组装成纽扣式半电池对其进行相应的电化学性能测试,如图7.图7 S/Co-N-C复合材料的电化学性能Fig.7 ElectrochemicalpropertiesofthecompositesofS/Co-N-C101第5期 陈磊,等:钴、氮共掺杂碳纳米复合材料的制备及其在锂硫电池中的应用图7(a)为电压区间在1 7~2 8V,电流密度为0 5C(1C=1675mA·h·g-1)时,电极材料的循环性能图.结果表明,S/Co-N-C展现了优异的循环稳定性,当其载硫量为60%时,该正极材料可提供高达803 9mA·h@g-1的初始可逆容量,100圈充放电循环之后仍能维持583 1mA·h·g-1的稳定容量,循环200圈后放电比容量可以达到522 7mA·h·g-1.该正极材料的每圈容量衰减在0 17%左右,可逆容量不可避免的衰减主要是由于穿梭效应.为了进一步评估该电极材料的电化学性能,也对其在不同电流密度下进行了倍率性能的测试(图7(b)).S/Co-N-C在0 1C的电流密度下可提供高达1265 7mA·h·g-1的放电比容量,第二圈略有下降至1072 5mA·h·g-1.在电流密度增加至0 2C、0 5C、1 0C、2 0C时,电极材料的可逆容量分别为804 2mA·h·g-1、693 5mA·h·g-1、601 5mA·h·g-1、525 5mA·h·g-1.当电流密度降至0 2C下,循环50圈后,其放电比容量可恢复至787 7mA·h·g-1.从结果可以发现该材料在不同电流密度下均表现出良好的电化学稳定性.图7(c)是电压窗口为1 7~2 8V,大电流密度为0 5C时,前三圈的充放电曲线图,用来分析该正极材料在充放电过程的电化学反应.首圈充放电时放电平台与后两圈的平台存在较大的差异,这是在第一次充放电过程中正极材料存在比较大的极化现象,之后的两圈充放电时极化反应减小,可以看到随后两圈的放电曲线也恢复至正常的平台.第二圈的放电曲线显示出典型的两个平台,分别处于2 3V和2 1V[14].在2 3V的电压平台与S8向可溶性长链多硫化物(Li2Sn,4≤n≤8)的转变有关,而在2 1V的电压平台则与可溶性长链Li2Sn进一步还原为不溶性短链多硫化物(Li2Sn,n<4)相对应[15-16].另外,第二圈充电曲线平台与上述相反的形式有关,代表着多硫化物向硫转变的过程[17].尽管在0 5C的大电流密度下,第二圈和第三圈的充放电曲线较好的重合且平台清晰可见,说明该材料的优异循环稳定性[18].锂硫电池中低的硫含量会展现出较高的放电比容量,然而极片的载硫量小于2mg/cm2会降低其实际能量密度[19].考虑到高面积容量对于锂硫电池实际应用的重要性,文中也探究了高载硫量电极的循环性能,如图8.将S/Co-N-C材料涂覆成厚膜电极(载硫量约2 4mg/cm2),在0 1C电流密度下循环100圈后其放电比容量为350 4mA·h·g-1,将电流密度增至0 2C再循环100圈后放电比容量仍能达到278 4mA·h·g-1.S/Co-N-C正极材料的优良电化学性能主要是由于其特殊的组分和结构.首先,嵌入的钴纳米粒子提供了吸附多硫化物的强相互作用,可大大提高多硫化物的氧化还原反应动力学.其次,类似蛋黄壳的中空结构对于硫的大装载率的包封和物理约束很有效.最后,氮掺杂碳具有高导电性,能够高效的束缚多硫化锂的溶出,减小穿梭效应,从而提高硫的利用率,进一步稳定锂硫电池的电化学循环性能和高比容量.图8 在0 1C和0 2C的电流密度下,载硫量为2 4mg/cm2的循环性能Fig.8 Cyclingperformanceof2 4mg/cm2sulfurloadingatcurrentdensitiesof0 1Cand0 2C3 结论以双金属Co,Zn-ZIF为前驱体,通过高温碳化和刻蚀工艺合成了类似蛋黄壳中空结构的Co-N-C复合材料.将其作为高性能锂硫电池载硫体,S/Co-N-C由于合理的纳米结构和组分,具有很高的可逆比容量,良好的倍率性能和超长的循环稳定性.值得注意的是,S/Co-N-C正极材料(高面积载硫量为2 4mg·cm-2)在200圈后仍显示出稳定的循环性能.在该工作中,金属源和氮掺杂的碳材料有利于提高复合材料的导电性,而中空的结构则有利于存储单质硫和缓冲体积的膨胀,为获得高性能锂硫电池提供了新的思路.参考文献(References)[1] LIZhen,ZHANGJintao,GUANBuyuan,etal.Asulfurhostbasedontitaniummonoxide@carbonhollowspheresforadvancedlithium sulfurbatteries[J].Na tureCommunications,2016(7):13065-13076.DOI:10.1038/ncomms13065.[2] BAOWZ,LIUL,WANGCY,etal.Facilesynthesisofcrumplednitrogen dopedmxenenanosheetsasanewsulfurhostforlithium sulfurbatteries[J].Ad201江苏科技大学学报(自然科学版)2020年vancedEnergyMaterials,2018,8(13):1702485.DOI:10.1002/aenm.201702485.[3] LIXX,ZHENGSS,JINL,etal.Metal organicframework derivedcarbonsforbatteryapplications[J].AdvancedEnergyMaterials,2018,8(23):1800716.DOI:10.1002/aenm.201800716.[4] CHENK,SUNZH,FANGRP,etal.Metal OrganicFrameworks(MOFs) derivednitrogen dopedporouscarbonanchoredongraphenewithmultifunctionaleffectsforlithium sulfurbatteries[J].AdvancedFunctionalMaterials,2018,28(38):1707592.DOI:10.1002/adfm.201707592.[5] LIYJ,FANJM,ZHENGMS,etal.Anovelsyner gisticcompositewithmulti functionaleffectsforhighperformanceLi-Sbatteries[J].Energy&EnvironmentalScience,2016,9(6):1998-2004.DOI:10.1039/C6EE00104A.[6] LUYQ,WUYJ,SHENGT,etal.NovelsulfurhostcomposedofcobaltandporousgraphiticcarbonderivedfromMOFsforthehigh performanceLi-Sbattery[J].ACSAppliedMaterials&Interfaces,2018,10(16):13499-13508.DOI:10.1021/acsami.8b00915.[7] SHANGL,YUHJ,HUANGX,etal.Well dispers edZIF derivedCo,N-Co-dopedcarbonnanoframesthroughmesoporous silica protectedcalcinationasefficientoxygenreductionelectrocatalysts[J].AdvancedMaterials,2016,28(8):1668-1674.DOI:10.1002/adma.201505045.[8] LIJ,CHENC,QINFR,etal.MesoporousCo-N-Ccompositeasasulfurhostforhigh capacityandlonglifelithium sulfurbatteries[J].JournalofMaterialsScience,2018,53(18):13143-13155.DOI:10.1007/s10853-018-2566-z.[9] BAIQ,SHENFC,LISL,etal.Cobalt@Nitrogen dopedporouscarbonfiberderivedfromtheelectrospunfiberofbimetal organicframeworkforhighlyactiveoxygenreduction[J].SmallMethods,2018,2(12):1800049.DOI:10.1002/smtd.201800049.[10] CHENGXL,LIDJ,LIUFF,etal.Bindingnanos izedcobaltchalcogenidesinB,N codopedgrapheneforenhancedsodiumstorage[J].SmallMethods,2018,3(4):1800170.DOI:10.1002/smtd.201800170.[11] ZHANGJH,HUANGM,XIBJ,etal.Systematicstudyofeffectonenhancingspecificcapacityandelectrochemicalbehaviorsoflithium sulfurbatteries[J].AdvancedEnergyMaterials,2018,8(2):1701330.DOI:10.1002/aenm.201701330.[12] CHENT,CHENGBR,ZHUGY,etal.Highlyeffi cientretentionofpolysulfidesin“seaurchin” likecarbonnanotube/nanopolyhedrasuperstructuresascathodematerialforultralong lifelithium sulfurbatteries[J].NanoLetters,2017,17(1):437-444.DOI:10.1021/acs.nanolett.6b04433.[13] YEC,ZHANGL,GUOCX,etal.A3Dhybridofchemicallycouplednickelsulfideandhollowcarbonspheresforhighperformancelithium sulfurbatteries[J].AdvancedFunctionalMaterials,2017,27(33):1702524.DOI:10.1002/adfm.201702524.[14] JIANGHQ,LIUXC,WUYS,etal.Metal OrganicFrameworksforhighcharge dischargeratesinlithiumsulfurbatteries[J].AngewandteChemieInternationalEdition,2018,57(15):3916-3921.DOI:10.1002/anie.201712872.[15] MANOJM,JASNAM,ANILKUMARKM,etal.Sulfur polyanilinecoatedmesoporouscarboncompositeincombinationwithcarbonnanotubesinterlayerasasuperiorcathodeassemblyforhighcapacitylithiumsulfurcells[J].AppliedSurfaceScience,2018,458:751-761.DOI:10.1016/j.apsusc.2018.07.113.[16] QUYH,ZHANGZA,WANGXW,etal.AsimpleSDS assistedself assemblymethodforthesynthesisofhollowcarbonnanospherestoencapsulatesulfurforadvancedlithium sulfurbatteries[J].JournalofMaterialsChemistryA,2013,1(45):14306-14310.DOI:10.1039/C3TA13306K.[17] ZHANGJT,LIZ,CHENY,etal.Nickel ironlay ereddoublehydroxidehollowpolyhedronsasasuperiorsulfurhostforlithium sulfurbatteries[J].AngewChemIntEdEngl,2018,57(34):10944-10948.DOI:10.1002/anie.201805972.[18] CHENT,ZHANGZW,CHENGBR,etal.Self templatedformationofinterlacedcarbonnanotubesthreadedhollowCo3S4nanoboxesforhigh rateandheat resistantlithium sulfurbatteries[J].JournaloftheAmericanChemicalSociety,2017,139(36):12710-12715.DOI:10.1021/jacs.7b06973.[19] HEJR,CHENYF,MANTHIRAMA.MOF derivedcobaltsulfidegrownon3Dgraphenefoamasanefficientsulfurhostforlong lifelithium sulfurbatteries[J].Science,2018,4:36-43.DOI:10.1016/j.isci.2018.05.005.(责任编辑:顾琳)301第5期 陈磊,等:钴、氮共掺杂碳纳米复合材料的制备及其在锂硫电池中的应用。
纯电动汽车空调与电池综合热管理仿真研究

doi: 10.3969/j.issn.2095-4468.2022.02.103 纯电动汽车空调与电池综合热管理仿真研究
孙港国,魏名山*,郑思宇,宋盼盼 (北京理工大学机械与车辆学院,北京 100081)
[摘 要] 为了实现电动汽车动力电池热管理和车舱制冷,本文基于AMEsim软件搭建了纯电动汽车空调与电池综合热管理系统仿真模型,研究了压缩机转速、电子膨胀阀的开度对综合热管理系统性能的影响。结果表明:当压缩机转速由1 000 r/min升至2 800 r/min,电池温度下降9.8 ℃,车舱温度下降至8.8 ℃;随着电子膨胀阀1开度增大50%,电池温度升高11.5 ℃,车舱温度下降5 ℃;当电池的放电电流由40 A升高至65 A时,将压缩机转速由1 250 r/min升至2 700 r/min和电子膨胀阀的开度比从1.20升至3.89,可以使车舱温度保持在23 ℃,电池温度保持在35 ℃,从而实现电动汽车综合热管理系统的控制。 [关键词] 电动汽车;综合热管理系统;直冷系统;仿真研究 中图分类号:TU831; U469.72 文献标识码:A
Simulation Research on Integrated Thermal Management of Air-Conditioning and Battery for Electric Vehicles
SUN Gangguo, WEI Mingshan*, ZHENG Siyu, SONG Panpan (School of Mechanical Engineering, Beijing Institute of Technology, Beijing 100081, China)
[Abstract] In order to realize the battery and cabin thermal management of electric vehicles, a model of an integrated thermal management system for electric vehicle air-conditioning and battery is proposed in AMEsim. The effect of compressor rotational speed and the opening of electronic expansion valve on the system performance are studied. The results showed that, the battery and cabin temperature separately decreased by 9.8 ℃ and 8.8 ℃ with the compressor rotational speed increasing from 1 000 r/min to 2 800 r/min. In addition, with the opening of the electronic expansion valve 1 increasing 50%, the battery temperature is increased by 11.5 ℃, while the cabin temperature is decreased by 5 ℃. When the discharge current varies from 40 A to 65 A, the rotational speed simultaneously is increased from 1 250 r/min to 2 700 r/min and the opening ratio of the electric expansion valves is magnified from 1.2 to 3.89, the cabin and battery temperature are kept at 23 ℃ and 35 ℃ respectively. [Keywords] Electrical vehicle; Integrated thermal management system; Direct cooling system; Numerical Simulation
What Are Batteries, Fuel Cells, and Supercapacitors 锂离子电池、燃料电池、超级电容器
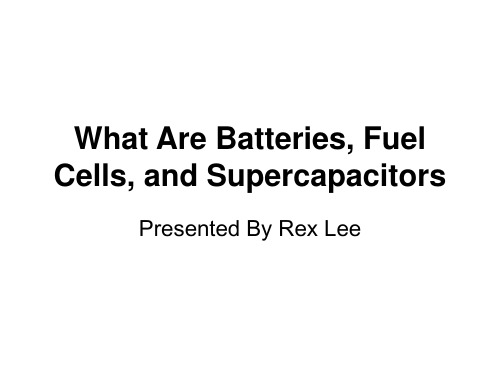
Applications
• Fuel cells established their usefulness in space applications with the advent of the Gemini and Apollo space programs • Fuel cells cannot compete today with combustion engines and gas/steam turbines because of much higher costs, inferior power and energy performance, and insufficient durability and lifetime
Batteries
• Electrical energy is generated by conversion of chemical energy via redox reactions at the anode and cathode • Closed systems, with the anode and cathode being the charge-transfer medium and taking an active role in the redox reaction as “active masses” • Energy storage and conversion occur in the same compartment • Batteries have intermediate power and energy characteristics • Batteries with thin film electrodes exhibit power characteristics similar to those of supercapacitors
Adaptive energy management of a plug-in hybrid electric vehicle混合动力汽车能量管理
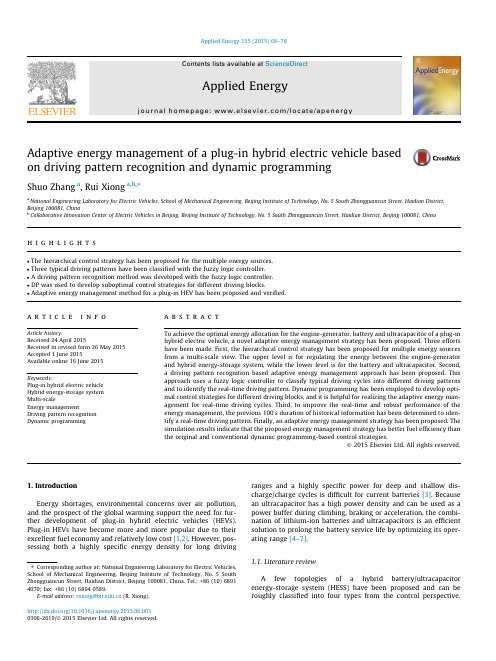
Adaptive energy management of a plug-in hybrid electric vehicle based on driving pattern recognition and dynamicprogrammingShuo Zhang a ,Rui Xiong a ,b ,⇑aNational Engineering Laboratory for Electric Vehicles,School of Mechanical Engineering,Beijing Institute of Technology,No.5South Zhongguancun Street,Haidian District,Beijing 100081,China bCollaborative Innovation Center of Electric Vehicles in Beijing,Beijing Institute of Technology,No.5South Zhongguancun Street,Haidian District,Beijing 100081,Chinah i g h l i g h t sThe hierarchical control strategy has been proposed for the multiple energy sources. Three typical driving patterns have been classified with the fuzzy logic controller. A driving pattern recognition method was developed with the fuzzy logic controller. DP was used to develop suboptimal control strategies for different driving blocks. Adaptive energy management method for a plug-in HEV has been proposed and verified.a r t i c l e i n f o Article history:Received 24April 2015Received in revised form 26May 2015Accepted 1June 2015Available online 16June 2015Keywords:Plug-in hybrid electric vehicle Hybrid energy-storage system Multi-scaleEnergy managementDriving pattern recognition Dynamic programminga b s t r a c tTo achieve the optimal energy allocation for the engine-generator,battery and ultracapacitor of a plug-in hybrid electric vehicle,a novel adaptive energy management strategy has been proposed.Three efforts have been made.First,the hierarchical control strategy has been proposed for multiple energy sources from a multi-scale view.The upper level is for regulating the energy between the engine-generator and hybrid energy-storage system,while the lower level is for the battery and ultracapacitor.Second,a driving pattern recognition based adaptive energy management approach has been proposed.This approach uses a fuzzy logic controller to classify typical driving cycles into different driving patterns and to identify the real-time driving pattern.Dynamic programming has been employed to develop opti-mal control strategies for different driving blocks,and it is helpful for realizing the adaptive energy man-agement for real-time driving cycles.Third,to improve the real-time and robust performance of the energy management,the previous 100s duration of historical information has been determined to iden-tify a real-time driving pattern.Finally,an adaptive energy management strategy has been proposed.The simulation results indicate that the proposed energy management strategy has better fuel efficiency than the original and conventional dynamic programming-based control strategies.Ó2015Elsevier Ltd.All rights reserved.1.IntroductionEnergy shortages,environmental concerns over air pollution,and the prospect of the global warming support the need for fur-ther development of plug-in hybrid electric vehicles (HEVs).Plug-in HEVs have become more and more popular due to their excellent fuel economy and relatively low cost [1,2].However,pos-sessing both a highly specific energy density for long driving ranges and a highly specific power for deep and shallow dis-charge/charge cycles is difficult for current batteries [3].Because an ultracapacitor has a high power density and can be used as a power buffer during climbing,braking or acceleration,the combi-nation of lithium-ion batteries and ultracapacitors is an efficient solution to prolong the battery service life by optimizing its oper-ating range [4–7].1.1.Literature reviewA few topologies of a hybrid battery/ultracapacitor energy-storage system (HESS)have been proposed and can be roughly classified into four types from the control perspective./10.1016/j.apenergy.2015.06.0030306-2619/Ó2015Elsevier Ltd.All rights reserved.⇑Corresponding author at:National Engineering Laboratory for Electric Vehicles,School of Mechanical Engineering,Beijing Institute of Technology,No.5South Zhongguancun Street,Haidian District,Beijing 100081,China.Tel.:+86(10)68914070;fax:+86(10)68940589.E-mail address:rxiong@ (R.Xiong).They include directly connecting the battery and ultracapacitor in parallel[4],connecting the battery with a DC/DC converter in series before connecting with the ultracapacitor in parallel[5],connecting the ultracapacitor with a DC/DC converter in series before connect-ing with the battery in parallel[7À9],and connecting the battery and the ultracapacitor each with a DC/DC converter in series before they are connected in parallel[7].In addition to these types,Ali Emadi has proposed a new HESS topology in which the battery does not provide power unless its terminal voltage is higher than that of the ultracapacitor[8].However,the required voltage level of the ultracapacitor is twice as much as that of the battery,which is unsup-portable in some applications.Based on our previous research expe-rience on the systematical evaluation results for the four HESS topologies in Ref.[9],the second topology that the battery pack con-nects with a DC/DC converter in series before it is connected with the ultracapacitor pack in parallel has been selected for this study.This type has the potential to fully exert the dynamic performance of the ultracapacitor by avoiding the current/power impact of the bat-tery and to extend the calendar life of the HESS.To achieve optimal energy/power management for HEVs and plug-in HEVs,a number of strategies have been developed[10–12].The rule based strategy is the most direct and widely used method due to its easy implementation and high calculation effi-ciency[13–15].Jalil N et al.have proposed a rule-based strategy to determine the power split between the battery and engine for a ser-ies hybrid electric vehicle[15].To further improve the performance of the energy management system for hybrid electric vehicles,sev-eral optimal energy/power management methods have been pro-posed,such as methods based on a fuzzy logic approach[16]and an equivalent consumption minimization strategy[17].However, with the development of intelligent algorithms,multiple advanced algorithms such as neural network[18],particle swarm optimiza-tion[19],simulated annealing[20],model predictive control[21], and dynamic programming(DP)[22,23]have been widely employed to develop various advanced adaptive/online energy management systems and optimal strategies.With a prior knowledge of the driv-ing cycle,DP-based methods have the ability to locate the global optimal control strategy.However,the actual future driving cycles can hardly be known in advance.In this case,the DP-based strategies cannot be used for an online energy management.Few energy management methods have been conducted for more than two energy sources[13–28].Most publications have investigated control strategies for EVs and HEVs powered by the battery and engine or the battery and ultracapacitor[24–28]. Specifically,for a series plug-in HEV with HESS,the optimization allocation problem for electricity energy among the battery,ultra-capacitor and engine-generator has not been solved effectively.1.2.Motivation and innovationThe purpose of this study is to propose an adaptive energy man-agement approach via driving pattern recognition and dynamic pro-gramming and to improve the energy management efficiency for a plug-in HEV with a HESS.To avoid the adverse effects of the optimal result against unknown cycles,the driving pattern recognition(DPR) method has been employed to classify and train the typical driving patterns.With the DP algorithm,the micro-control strategies for dif-ferent classified driving patterns can be developed in a systematic ing the fuzzy control algorithm-based predictive approach, the current driving pattern can be recognized with a period of histor-ical driving information.To realize the optimal energy allocation between the engine-generator and HESS with less computational cost,a hierarchical control strategy has been proposed for three energy sources from a multi-scale view.The proposed energy man-agement strategy has been verified and evaluated by a combined driving cycle and Japanese10–15mode driving anization of the paperThis paper is organized as follows.Section2describes the con-figuration of the plug-in HEV and the original control strategy. Then,the classification of driving blocks,construction of DPR,sub-system modeling,DP formulation and energy management system are illustrated in Section3.The verification and evaluation of the proposed method are reported in Section4,and conclusions are drawn in Section5.2.Plug-in hybrid electric vehicle configuration2.1.Vehicle configurationThe structure of the researched target is illustrated in Fig.1. The electricity power of the plug-in HEV comes from two parts: the HESS and assistance power unit(APU).The APU consists of an80kW permanent magnetic generator and a 1.9L gasoline engine,and the rated power of the APU is75kW.Detailed mod-eling of the APU and HESS are introduced in Section3.2.The tar-get vehicle is an electric bus and its essential parameters are presented in Table1.2.2.Hierarchical energy management for the plug-in HEVThe energy management system of the plug-in HEV can be divided into two layers.The upper level is for controlling the energy between the APU and HESS,and the lower level is for con-trolling the energy between the battery and ultracapacitor.2.2.1.Upper level control strategyThe main objective of the energy management is to minimize the operation cost of the plug-in HEV.For optimizing the alloca-tion of energy/power between the HESS and APU,a systematic energy management strategy is necessary.The original control strategy of the researched plug-in HEV is a typical charge deplet-ing(CD)and charge sustaining(CS)method.Itfirst operates the plug-in HEV with the CD mode,which is similar to a pure electric vehicle,and then operates the plug-in HEV with the CS mode once its state-of-charge(SoC)level hit the lower threshold,which is similar to a traditional hybrid electric vehicle.The detailed original control rules in the CS model are identified by the required power of the plug-in HEV-P n and they are described by the following conditions.Condition I:P n<0.The HESS absorbs as much energy as possible,and the excess energy is consumed by the traditional mechanical braking system. It is noted that the APU is turned off in condition I.Condition II:P n P75kW.The output power of APUÀP APU will maintain to its rated power (75kW),and the insufficient power will be supported by the HESS.Condition III:06P n675kWIf the SoC of the battery pack(z b)is bigger than its lower thresh-old(z b,min),the HESS will provide the total required power and the APU will be in the off stage.If the SoC of the battery pack(z b)is smaller than its lower threshold(z b,min),the APU will work in the rated power condi-tion and the redundant power will be used to charge the HESS to its predetermined level.S.Zhang,R.Xiong/Applied Energy155(2015)68–78692.2.2.Lower level control strategyThe power management between the battery pack and ultraca-pacitor pack is implemented via a rule based control strategy with the following rules[28].Condition I:P HESS<0When the required power of HESS(P HESS)is negative,the ultra-capacitor pack will absorb as much braking energy as possible until its SoC is bigger than its upper threshold(z uc,max),and then the bat-tery is allowed to absorb the remaining energy.Condition II:P HESS P0If06P HESS<30kW,z b>0.201and z uc<0.85,then P b=30kW and P uc=P HESSÀP b.If06P HESS<30kW,z b>0.201and z uc P0.85,then P b=P HESS and P uc=0.If P HESS P30kW,z b>0.201and z uc P0.515,then P b=30kW and P uc=P HESSÀP b.If P HPESS P30kW,z b>0.201and z uc<0.515,then P b=P HESS and P uc=0.If P HESS P0kW,z b60.201and z uc P0.515,then P b=0and P uc=P HESS.If P HESS P0kW,z b60.201and z uc<0.515,then P b=0and P uc=0.where z b denotes the SoC of the battery pack,z uc denotes the SoC of the ultracapacitor pack,P b denotes the output power of the battery pack,and P uc denotes the output power of the ultracapac-itor pack.3.Decomposition of the drive cycles3.1.Driving blocks classificationThe traditional DPR method based power management approaches tend to use the existing unbroken driving cycles to classify the driving blocks and then develop the control strategies by recognizing a whole driving cycle[29–32].However,for a given driving cycle,these methods usually contain several types of driv-ing blocks that have been neglected in these methods.Fig.2illus-trates that different types of drive patterns may have similar driving blocks and that the same driving cycle may have different driving blocks.Thus,the control strategy developed by a whole driving cycle can hardly ensure the optimal vehicle performance. To overcome the drawback,a novel classification method that has the ability to classify the driving block into several groups has been proposed.The driving blocks from three typical drive cycles are plotted in Fig.2,which includes the Chinese Bus Driving Cycle(CBDC), ECEÀEUDC drive cycle and MANHATTAN drive cycle.For a deter-minate driving cycle,the number of description parameters may be as high as62[32].Too many parameters may unnecessarily bias the calculation.The average speed has been reported as the unique parameter to use in Ref.[33].This study considers the average and maximum speed of each block as its classification parameters.The calculation method for the average speed and maximum speed is displayed below:V ai¼Zv dt=tð1ÞV max i¼maxðv j;j¼1;2...kÞð2Þwhere V ai denotes the average speed of each driving block,i denotes the index of the driving blocks,and V maxi denotes the maximum speed of each driving block.The fuzzy logic controller is employed to classify the drive blocks and identify the driving types for the DPR process.The con-troller consists of four parts(as displayed in Fig.3),including fuzzi-fication,rule base,fuzzy reasoning and defuzzification.The fuzzification part is used to fuzzify the input values and these fuzzified values will be converted into the output fuzzy values through the fuzzy reasoning block based on the rule base part. Then,the output fuzzy variable will be defuzzified by theTable1Basic parameters of the plug-in HEV.Name Value UnitVehicle loaded mass M16,500kgEfficiency of the transmission system g00.9/Rolling resistance coefficient f0.011/Windward area A ar 6.6m2Air resistance coefficient C ar0.55/Gravitational acceleration g9.81m/s2Correction coefficient of rotating mass d 1.03/70S.Zhang,R.Xiong/Applied Energy155(2015)68–78defuzzification block.The details of the fuzzy logic controller oper-ation process are displayed below:(1)FuzzificationFuzzy sets represent the linguistic terms,and the linguistic terms of the input variables and output variable are set to Low ÀLevel (low speed driving pattern),Middle ÀLevel (medium speed driving pat-tern)and High ÀLevel (high speed driving pattern).It is worth noting that,for the low speed driving pattern,the maximum velocity is less than 25km/h,and the average velocity less than 15km/h.For the medium speed driving pattern,the maximum velocity is between 25km/h and 45km/h,and the average velocity is between 15km/h and 25km/h.For the high speed driving pattern,the maxi-mum velocity is greater than 45km/h,and the average velocity is greater than 25km/h.The input variables are fuzzified by member-ship functions as shown in Fig.4.Once the average speed and max-imum speed of a driving block are known,we can determine the memberships (Lower Àlevel l L (v ),Middle Àlevel l M (v )and High Àlevel l H (v ),where v denotes the input variables)through membership functions.After comparing these values,we can finally obtain the fuzzification results,which are the fuzzy sets.Rule baseFuzzificationOutput driving patternDrive block12i 8128 V ...V ...V max(,...)mn mn mn imn mn R R R R R R R R R R =⎧⎨=⎩S.Zhang,R.Xiong /Applied Energy 155(2015)68–7871(2)Rule baseThe rule base displayed in Table 2shows that the fuzzy logic is a typical type of the A +B ?C (if A and B,then C)mode,where A denotes the fuzzy sets of average speed,B denotes the fuzzy sets of maximum speed and C denotes the fuzzy sets of driving block pattern.The reasoning process is based on the Mamdani fuzzy theory [34].From each rule shown in Table 2,we can obtain a correspond-ing fuzzy relation matrix R i by the cross-product of A i and B i .The fuzzy relation matrix R can be obtained by the combination of the fuzzy relation matrix R i using the following equation:R ¼R 1V R 2...V R i ...V R 8R mn ¼max ðR 1mn ;R 2mn ...R imn :R 8mn Þð3Þwhere m and n (m =1,2,3and n =1,2,3)denote the index of matrix elements for R i and R .(3)Fuzzy reasoningWhen we obtain the fuzzification results of the input variables (A 1and B 1)and the fuzzy relation matrix R ,we can locate the driv-ing types using the following equation:C 1¼ðA 1ÂB 1Þ Rð4Þwhere C 1denotes the fuzzy set for the output variable.(4)DefuzzificationThe results obtained by Eq.(4)from a fuzzy set,which is not applicable under real conditions.Thus,we should convert the fuzzy set into a known driving pattern.The biggest subordinate principle is employed to locate the driving block pattern.According to this principle,the driving block pattern is located to the value whose membership is the biggest one in the domain of discourse.Fig.5shows the classification results.From Fig.5we can observe that most of the driving blocks have similar profiles in the rearranged drive cycles.Three drive cycles will be used for the power manage-ment design during the following DP process.3.2.Modeling for subsystemsThe correspondingly optimal control strategy can be developed through analyzing the DP optimization results under different types of driving conditions.By combining these strategies together with the proposed DPR process,a suboptimal solution for the energy management of the plug-in HEV can be achieved.Before the implementation of the DP formulation process,we should first build the subsystem models.Considering that the DP process relies on the state equations of the plug-in HEV powertrain system,if the total order number of the system is too high,the computational cost is usually unacceptable.In this way,simplified backward sim-ulation models for the HESS,APU and vehicle are developed.It is noting that the parameters of the battery pack,ultracapacitor pack and DC/DC converter in the HESS were determined by the Ref.[9].The models are described as follows.(1)APU modelAs an independent power unit in the series powertrain,the engine and generator are mechanically decoupled from the drive-line.The APU operates according to the maximum efficiency line.The optimal fuel rate line of the APU is derived from the APU effi-ciency map from a combination of the efficiency map between the engine and generator.The optimal fuel rate line of the APU system is shown in Fig.6(a).In this study,we neglect the APU transience influence and calculate the fuel consumption of the engine by its static operating points.Once the output power of APU is deter-mined,we can obtain the fuel consumption using the following equation:_m f ¼f ðP APU Þð5Þwhere _mf denotes fuel consumption and f ðP APU Þdescribes the map-ping function between the output power of APU and engine fuel rate according to the combined optimal fuel rate line presented in Fig.6(a).Fig.6(b)shows the fuel consumption when the APU output is 1kJ of energy under different output power conditions.From Fig.6(b)we can observe that the efficiency of the APU will increase with increasing output power,and when the output power reaches 75kW,the efficiency is at its highest.Thus,as the rated power and maximum power of APU,75kW is the best operation point of the APU.(2)Ultracapacitor model The dynamic property of the ultracapacitor is recognized as a series connection of a resistance R c (0.0756X )and an idealTable 2Rule base for the driving pattern recognition.V a ÀLowV a ÀMiddle V a ÀHigh V max ÀLow Low Middle –V max ÀMiddle Low Middle High V max ÀHighMiddleMiddleHigh72S.Zhang,R.Xiong /Applied Energy 155(2015)68–78capacitor (maximum voltage is 576V).The operation behavior of the ultracapacitor is illustrated by the following equation:U ct ¼U co ÀR c i c ð6Þwhere U ct is terminal voltage of ultracapacitor,U co denotes the ideal capacitor voltage and i c denotes the output current.(3)Battery modelTo execute the optimization and analyze the dynamic features of the battery,we need a ‘‘discrete-time cell dynamic model’’that relates the SoC to its voltage.Based on our research experience in battery control and state estimation [2,35],a classical lumped parameters battery model,the Thevenin model,has been selected for this study.Its electrical behavior can be expressed as the following:_U D ¼À1D D U D þ1D i L U t ¼U oc ÀU D Ài L R i(ð7Þwhere U oc denotes the open circuit voltage (OCV)of a battery,R i denotes the series resistance,and R D and C D denote the diffusion resistance and diffusion capacitance,respectively.The parameter i L denotes the load current (positive indicates discharging and neg-ative indicates charging),U D denotes the diffusion voltage and U t denotes terminal voltage.It is worth noting that the lithium-ion battery cell with graphite anodes and nickel–manganese–cobalt oxide (NMC)cathodes is used in this study,and its upper and lower cutoff voltages are 4.2V and 3.0V,respectively.Each cell has a nominal capacity of 79A h and nominal voltage of 3.7V.It is noted that the battery pack consists of 135lithium-ion battery cells con-nected in series.Thus,the nominal capacity and voltage of the bat-tery pack are 79A h and 499.5V,respectively.The total energy of the battery pack is 39kW h according to the energy requirement of the target vehicle.The parameters of the battery model have been obtained by the parameter identification method based on the recursive least squares filter described in Ref.[35].(4)DC/DC converter modelTo analyze the dynamic behavior of the HESS,we first need a model for the DC/DC converter.Table 3shows an efficiency map n (i DC ,P DC )to describe the operating behavior of the DC/DC con-verter,where i DC and P DC denote the output current and output power of the DC/DC converter,respectively.The current points contain four levels:i DC =10A,i DC =50A,i DC =100A,and i DC P 150A.The power points contain five levels:P DC =10kW;P DC =20kW;P DC =30kW;P DC =40kW;and P DC P 50kW.The rated power of the DC/DC converter is 30kW.(5)Vehicle and transmission modelIn this study we only consider the vehicle’s longitudinal dynam-ics,namely,the vehicle is modeled as a point-mass.The required power (P n )of the plug-in HEV can be calculated from Eq.(8)[36].Its transmission is modeled as a fixed efficiency g as shown in Eq.(8).P n ¼u a g Mgf cos ðb Þ3600þMg sin ðb Þ3600þC ar A ar 76140u 2a þd M 3600d u ad tð8Þwhere u a denotes the vehicle velocity and b represents the grade of the road.3.3.Formulation of the DP algorithmBased on the above models,the DP algorithm can be used to locate the optimal power distribution ratio between the APU and HESS.According to Bellman’s optimization theory,a numerical-based DP approach is adopted and the state equation of the battery model and ultracapacitor model can be generally expressed by the following equation:x ðk þ1Þ¼f ðx ðk Þ;u ðk ÞÞð9Þwhere x (k )denotes the state vector of system and u (k )denotes con-trol variable (power increment P iAPU ).The parameter x (k )can be cal-culated by the following equation:x ðk Þ¼P APU z b z uc U D ½ ð10ÞIt is obvious that we can reduce the state order by removing the output power of the APU from the state equation and selecting the output power as the control variable,but this reduction will lead to drastic fluctuations in the output power of the APU from the lowest working point directly to the highest working point;it is not reasonable.To reduce the dimensions of the optimization problem and improve the control efficiency for the multi-power system of the plug-in HEV,the energy management of the HESS employs the rule-based strategy,which has been illustrated in Section 2.2;this strategy is helpful for reducing the order of the state equation and calculation burden.Then,the control variable has been simplified to the power increment of the APU (P iAPU )and once the output power of the APU is provided then the output power of HESS will be determined according to the required power of the plug-in HEV.The detailed state equations of the APU,battery and ultracapac-itor are described by the following equation:P APU ðk þ1Þ¼P APU ðk ÞþP iAPU ðk Þð11ÞU D ðk þ1Þz b ðk þ1Þ¼exp ðÀD t =R D C D Þ001U D ðk Þz b ðk Þþð1Àexp ðÀD t =R D C D ÞÞR DÀ1=3600Q bi L ðk Þð12Þz uc ðk þ1Þ¼z uc ðk ÞÂQ c Ài cQ cð13Þwhere Q b and Q c denote the nominal capacity of the battery packand ultracapacitor pack,respectively,i L and i C denote the output current of the battery pack and the ultracapacitor pack respectively,and D t denotes the calculation step,which herein is set to1s .The optimization target is to locate the optimal control variable P iAPU to minimize a cost function (minimum usage costs),as dis-played below:J ¼X N À1k ¼0L ðx ðk Þ;u ðk ÞÞ¼X N À1k ¼0½L fuel ðx ðk Þ;u ðk ÞÞþL e ðx ðk Þ;u ðk ÞÞ L fuel ðx ðk Þ;u ðk ÞÞ¼_m ðk ÞM fuel L e ðx ðk Þ;u ðk ÞÞ¼i L ðk ÞU t ðk ÞM e8>>>><>>>>:ð14Þwhere N denotes the duration of the driving cycle,L denotes the instantaneous cost which represents usage costs,L fuel and L e denote the fuel and electric cost,respectively,and M fuel and M e denote the current fuel and electric price,respectively.It is noted that,M fuel and M e are set to 8.9908RMB per liter and 0.799RMB per KWH,respectively (according to the data on Dec.62014,in Beijing).During the optimization process,the following inequalityTable 3Efficiency map of the DC/DC converter [9].n (i DC ,P DC )10kW 20kW 30kW 40kW P 50kW 10A 92%95%97%95%94%50A 91%93%96%93%92%100A 88%91%95%92%91%P 150A82%89%92%91%90%S.Zhang,R.Xiong /Applied Energy 155(2015)68–7873constraints are necessary to ensure safe and reasonable operation of the APU and HPS:z b ;min 6z b ðk Þ6z b ;max z uc ;min 6z uc ðk Þ6z uc ;max j zuc ;end Àz uc ;start j 60:5%i L ;min 6i L ðk Þ6i L ;max i c ;min 6i c ðk Þ6i c ;max U t ;min 6U t ðk Þ6U t ;max8>>>>>>>><>>>>>>>>:ð15Þwhere z b,min and z b,max denote the lower and upper bounds of thebattery SoC,U t,min and U t,max denote the lower and upper con-strains of the battery terminal voltage,i L,min and i L max denote the lower and upper constrains of the battery current,and i c,min and i c,max denote the bounds of the ultracapacitor current.The param-eters z uc,start and z uc,end denote the start and end values of the SoC of the ultracapacitor pack during the optimization process.It is worth noting that less than 0.5%of the difference between the start and end SoC of the ultracapacitor pack indicates the energy consump-tion of ultracapacitor pack can be approximated to zero.In this study,z b,min and z b,max are set to 0.2and 1,respectively,U t,min and U t,max are set to be 405V and 567V,respectively,i L,min and i L,max are set to À158A (À2C)and 158A (2C),respectively,and i c,min and i c,max are set to À500A and 500A,respectively.According to the dynamic optimization theory of Bellman,we first need to solve the problem from the last stage and then we extend the problem to solve for the last two stages and last three stages until all of the stages are included.Under this condition the optimization problem has been decomposed into a sequence of minimization problems as shown below.Step N À1:J ÃN À1ðx ðN À1ÞÞ¼min u ðN À1Þ½L ðx ðN À1Þ;u ðN À1ÞÞþG ðx ðN ÞÞð16ÞStep k ,for 06k <N À1:J Ãk ðx ðk ÞÞ¼min u ðk Þ½L ðx ðk Þ;u ðk ÞÞþJ Ãk þ1ðx ðk þ1ÞÞð17Þwhere J k *(x (k ))denotes the optimal value function at state x (k )start-ing from k th time stage to the last stage.The operation of the opti-mization process is subject to constraints presented in Eq.(15).Because the APU and HESS are nonlinear systems,the DP optimiza-tion process has been implemented with some approximations.Quantization and interpolation are used to solve Eq.(17)numeri-cally.At each step,the function J k (x (k ))is only calculated at the grid points of the state variables.The values of J k *(x (k ))in Eq.(17)and G (x (N ))in Eq.(16)will be determined through linear interpolation when the next state does not fall on a quantized value.3.4.Power management design frameworkThe detailed development process of the adaptive energy man-agement for the plug-in HEV with the DPR and DP is illustrated in Fig.7.It mainly consists of four parts:driving cycle classification,system modeling,DP processing and DPR process.The detailed operation for each part has been described in the above sections.First,three typical drive cycles are used to train the representa-tive driving blocks with the fuzzy logic algorithm based on the driving cycle classification method.The driving cycle classification process is used to rearrange the driving blocks into several types of new cycles.Its classification is implemented with the average speed and maximum speed.Driving pattern recognitionOriginal driving cycleReal driving cycle Classified driving blocksFuzzy logic based classification module Fuzzy logic based DPR74S.Zhang,R.Xiong /Applied Energy 155(2015)68–78。
新能源汽车专业英语最新版精品课件第2章第1节
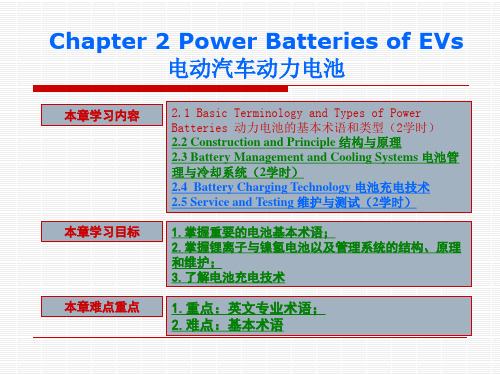
components of a PEV battery. A cell is the smallest possible battery that contains electrodes, a separator, and an electrolyte. The output voltage of a typical cell varies between one to six volts. A module consists of multiple cells that are usually connected in either series or parallel. Similarly, a pack is composed of a few modules located in a single location.
['ɪndɪkeɪtə(r)] n.指示器,指标,指示灯
measure
['meʒə(r)]
n.度量,度量单位;
metric
['metrɪk]
n.度量标准,尺度
2.1 Basic Terminology and Types of Power Batteries 动力电池的基本术语和类型
module moving-off nominal pack packaging parameter quantify
1.掌握重要的电池基本术语; 2.掌握锂离子与镍氢电池以及管理系统的结构、原理 和维护; 3.了解电池充电技术
1.重点:英文专业术语; 2.难点:基本术语
2.1 Basic Terminology and Types of Power Batteries 动力电池的基本术语和类型
Energy Conversion and Management

determine the initial SOC denoted as SOC0 and solve the variation of SOC0 resulting from self-discharge and other factors. Thirdly, the error is larger when the battery works at high and low temperature or when the current fluctuates dramatically. Finally, the method is an open loop SOC estimator and errors in the current detector are accumulated by the estimator. The longer the estimator is operated, the larger the cumulative error becomes. In order to solve these problems, the Ah counting approach is always combined with other approaches. For example, the Ah counting approach is
positive while discharging and negative while charging. g is the coulombic efficiency. Usually, g ¼ 1 for discharge and g < 1 for
Exploration on Teaching Reform of New Energy Vehic

Exploration on Teaching Reform of New Energy Vehicle in Colleges and UniversitiesYajun HanJiangsu University of Technology (Depar tments of automotive and traffic engineering)Abstract: With the development of higher education in recent years, the level and speed of the new energy automotive technology is rising year by year, and now the new energy automotive technology teaching has gradually become the key point of automobile manufacture engineering fields and obligatory. With the continuous development of political and economic society, it is necessary to carry out more comprehensive teaching exploration and research for various teaching plans, contents and models of new energy vehicle courses in practice, so as to promote the teaching of the new energy vehicle course to be effectively deepened and reformed in practice to ensure the scientific research of new energy vehicle course teaching and rapid development of keeping pace with the times. Doing so can greatly improve the teaching quality of this course and promote the majority of students to improve their learning efficiency.Keywords: Universities; Automobile teaching; ReformDOI: 10.47297/wspiedWSP2516-250004.202105061. IntroductionNew energy vehicles are accompanied by the development of global economic and social integration. They can not only represent the development of various world-class automobile manufacturing industries in the world, but also directly promote the healthy development of the global economy. In addition, other regions and countries vigorously promote the wide application of new energy vehicle products in recent years, which also promoted the development of new energy vehicles. This constantly promotes the transformation and upgrading product structure of the whole new energy vehicle manufacturing, making full use of energy and contributing to environmental protection. However, due to theAbout the author:Yajun Han (1988-05), female, Qing dao Shan dong, han nationality, university lecturer, doctor, unmanned ground vehicle ,track tracking. Control BMS. Funded project:Research Fund for the Auto-assisted safe Driving System based on fusion Communication Technology Source: Jiangsu univeristy of Technology Undergraduate Innovation and Entrepreneurship Project No. : KYX20027.Journal of International Education and Development Vol.5 No.6 2021current oil shortage and serious environmental pollution, development of new energy automobile products and industries can effectively save oil resources, but also effectively reduce automobile emissions. Therefore, the construction and development of new energy vehicles is of great value to the development of China and the world. In the face of this grim situation, the new energy vehicle engineer training industry has cooperated with universities to open a series of professional training courses. In order to ensure the teaching quality of these courses, the teaching methods of corresponding courses have been deepened and reformed, so as to promote the sustainable development of new energy vehicle technology majors.2. Significance and Necessity of New Energy V ehicle T eaching Reform in Colleges and UniversitiesWith the continuous deepening and reform of higher education, in order to meet the development needs of the times and the talent needs of society and market, some institutions of higher learning have set up training management mechanisms and pilot reforms. In addition, the reform has been gradually inferred in various places, which promotes the current training path of higher students to be innovated with the progress and effect of the mechanism reform in recent years. Facing the current situation and sustainable development strategy and the application of green environmental protection technology, the new energy vehicle course point has been combined with the New energy Vehicle Research Institute of China Jiaotong University.The first important task of this course is to help college students further understand new energy vehicles in all aspects. The development and change of new energy automobile manufacturing industry has become an inevitable trend. But these conditions should be based on the basis of a wealth of relevant professionals, so the reform of the curriculum system of new energy vehicles is indeed conducive to cultivating more professional talents related to new energy vehicles. But to some extent, it should also be conducive to promoting the progress of new energy automobile related industries, so the reform of new energy automobile curriculum system is still an inevitable trend of the automobile industry.3. Measures for T eaching Reform of New Energy V ehicles in Colleges and Universities(1) Teaching Scheme of Innovative New Energy VehiclesFor the construction and development of modern new energy vehicle course, the modern automobile energy saving and modern new energy technology whichExploration on Teaching Reform of New Energy Vehicle in Colleges and Universities has just started gradually develop, effectively contain the modern automobile energy saving of various natural resources and modern new energy technology. Besides, he construction and development of new energy vehicles have gradually added new technology and modern scientific methods, making the current modern new energy constantly improve.At the same time, the current new energy vehicles increasingly tend to develop electric vehicles, new energy vehicle training courses in colleges and universities need to guide and cultivate students' professional ethics and basic learning qualities. In addition, there is a more urgent need to cultivate students with good professional skills and specialties, prompting students from other regions to participate in the continuous innovation and development of the courses.For example, it was decided to further modify the course teaching scheme of the major of electric vehicles after discussion between the head of the teaching and research section of the major of new energy vehicles and the professional teachers. They decided to make the teaching content closely related to the daily life of the practitioners, pay attention to the research of the electric vehicle major, and turn the course into a compulsory one. The new teaching plan further increases the number of courses for the major of new energy vehicles, and divides the courses into theoretical and practical cultivation of knowledge.(2) Innovating Teaching Contents of New Energy Vehicles in Colleges and UniversitiesIn the new energy vehicles currently professional teaching reform, compared with other professional courses, this course started slow. But because of the formation and development of new energy vehicles need to have a lot of teaching and scientific research information resources, gradually some of the new energy vehicles related technology and its development of scientific research information and data collated and added to the course teaching according to the actual teaching and research. Thus, this initiative promotes the cultivation and innovation of students' awareness and thinking of new energy vehicles.For example, in the course and teaching of new energy vehicles, the preferential policies for the development of new energy vehicle industry and the basic practice and technical training of related technologies should be added appropriately. According to the practical cases at home and abroad, and integrate them into the teaching, so that students can master more practical foundation and skills in the course and teaching of new energy vehicles.(3) Improving the Teaching Methods of New Energy Vehicles in Colleges and UniversitiesIn the teaching reform of new energy vehicles courses, only appropriateJournal of International Education and Development Vol.5 No.6 2021teaching means and methods can promote the implementation of the professional course of new energy vehicles and improve its teaching quality. Case study method is a useful method. In the development of new energy vehicles, the new energy vehicles of major brands at home and abroad are relatively rapid development, and the network platform is also most of the information about the development of new energy vehicles. Therefore, teachers can reasonably use some successful cases of typical new energy vehicles and pure electric vehicles to carry out teaching in the whole process of classroom teaching. In this way, we can not only effectively improve the classroom practice and learning quality of Rising Sun sheng, but also help our students understand the important difficulties of knowledge more easily.For example, teachers can make full use of the E50 in the Roewe electric vehicle series when teaching in class. They can use the multimedia to directly play the case, while through the explanation and analysis of these cases, to promote people in the study, improve the accumulation of knowledge, solve some learning problems.(4) Strengthening Teaching Staff of New Energy Vehicle Courses in Colleges and UniversitiesIn reforming the curriculum of new energy vehicles, schools also need to continue to strengthen the construction and training of teachers, improve the professional skills and quality of school teachers, give play to the role of teachers as leaders and guide teachers to independent learning. In addition, the school has carried out the teaching mode of open class according to the needs. Through the evaluation of other teachers in the public teaching mode, we improve our own teaching plan and improve the comprehensive learning quality of students. At the same time, the school also needs to consciously change the performance appraisal mechanism in the class teaching. Teachers no longer restrict students to the final and mid-term assessment, but start from the daily learning and participation of all students in activities, and the improvement of the efficiency of all teaching and classroom.For example, teachers also need to pay attention to classroom evaluation and student performance. Using some of the practical teaching, teachers use these courses to get students to come on stage and talk about themselves. Students can also evaluate what they don't know about the teaching content of the teacher, so as to effectively promote the teachers to establish a teaching structure to improve themselves and help them improve their learning efficiency.4. ConclusionTo sum up, the current teaching reform of new energy vehicles in universities will be an inevitable trend to promote the sustainable and healthy developmentExploration on Teaching Reform of New Energy Vehicle in Colleges and Universitiesof the new energy vehicle product industry. But want to do a better job to deepen education reform and new energy vehicles course, new energy vehicles related courses still need the perspective of the traditional teaching concept and technology, innovation of teaching content, improving teaching methods and teaching means, to strengthen professional teachers reserves to the school, and finally to establish an innovative new energy vehicles related curriculum evaluation system. In addition, the evaluation system can promote most students to successfully complete the adaptation to the environment for the new energy vehicle related body in practice, laying a foundation for the sustainable and healthy development of the new energy vehicle product industry in the future.References[1] Duan Min, Shi Jing, Zhang Liping, , et al. “Experimental (Practice) Teaching Sys-tem of New Energy Vehicle Engineering Major Direction” [J]. Journal of Liaoning University of Technology,106-11(2016).[2] Li Bo, Zhou Ting, Bei Shaoyi, et al. “Analysis of Teaching Reform of The Principleand Structure Course of New Energy Vehicles” [J]. Journal of Higher Education, 10(2017).[3] Yang SHAN. “Discussion on Practical Teaching Reform and Exploration of NewEnergy Vehicle Major” [J]. Auto Time, 57-58(2019).。