Computational Models of First Language
计算语言学
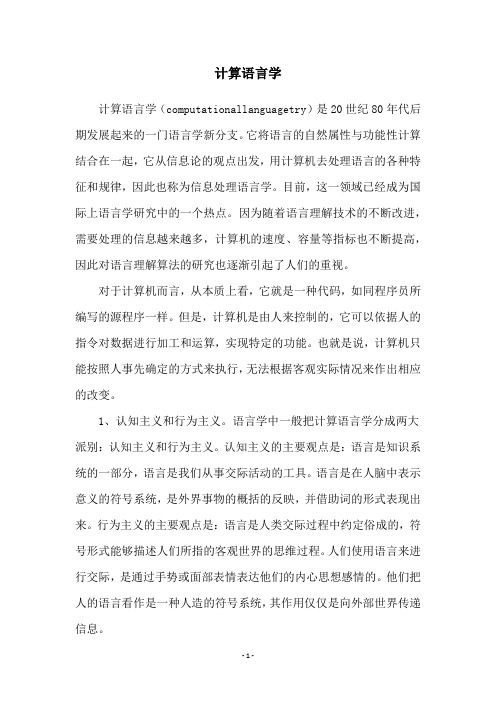
计算语言学计算语言学(computationallanguagetry)是20世纪80年代后期发展起来的一门语言学新分支。
它将语言的自然属性与功能性计算结合在一起,它从信息论的观点出发,用计算机去处理语言的各种特征和规律,因此也称为信息处理语言学。
目前,这一领域已经成为国际上语言学研究中的一个热点。
因为随着语言理解技术的不断改进,需要处理的信息越来越多,计算机的速度、容量等指标也不断提高,因此对语言理解算法的研究也逐渐引起了人们的重视。
对于计算机而言,从本质上看,它就是一种代码,如同程序员所编写的源程序一样。
但是,计算机是由人来控制的,它可以依据人的指令对数据进行加工和运算,实现特定的功能。
也就是说,计算机只能按照人事先确定的方式来执行,无法根据客观实际情况来作出相应的改变。
1、认知主义和行为主义。
语言学中一般把计算语言学分成两大派别:认知主义和行为主义。
认知主义的主要观点是:语言是知识系统的一部分,语言是我们从事交际活动的工具。
语言是在人脑中表示意义的符号系统,是外界事物的概括的反映,并借助词的形式表现出来。
行为主义的主要观点是:语言是人类交际过程中约定俗成的,符号形式能够描述人们所指的客观世界的思维过程。
人们使用语言来进行交际,是通过手势或面部表情表达他们的内心思想感情的。
他们把人的语言看作是一种人造的符号系统,其作用仅仅是向外部世界传递信息。
2、神经科学和心理语言学。
20世纪70年代以后,计算机和信息论的研究蓬勃兴起,并与人类语言学的研究产生了紧密的联系。
人们逐步发现,计算机的行为模式直接来自人的行为模式,即直接来自于大脑的某些脑区。
人脑的某些脑区被称之为高级认知中心,具有推理、解决问题、记忆和逻辑判断等功能,其主要功能是对外界事物的知觉、学习、记忆、存贮和对事物的归类,并做出适当的行为反应。
计算机是电子设备,电子设备在很大程度上都是按照人们事先制定的程序设计的,这样就保证了整个计算机的操作必须严格按照人们事先确定的规则来执行。
数学技术用于英语学习的英文作文
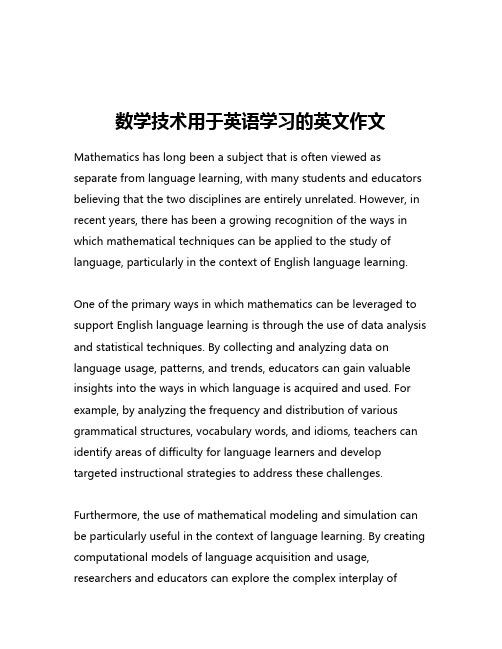
数学技术用于英语学习的英文作文Mathematics has long been a subject that is often viewed as separate from language learning, with many students and educators believing that the two disciplines are entirely unrelated. However, in recent years, there has been a growing recognition of the ways in which mathematical techniques can be applied to the study of language, particularly in the context of English language learning.One of the primary ways in which mathematics can be leveraged to support English language learning is through the use of data analysis and statistical techniques. By collecting and analyzing data on language usage, patterns, and trends, educators can gain valuable insights into the ways in which language is acquired and used. For example, by analyzing the frequency and distribution of various grammatical structures, vocabulary words, and idioms, teachers can identify areas of difficulty for language learners and develop targeted instructional strategies to address these challenges.Furthermore, the use of mathematical modeling and simulation can be particularly useful in the context of language learning. By creating computational models of language acquisition and usage, researchers and educators can explore the complex interplay ofvarious factors that influence language learning, such as age, cognitive abilities, cultural background, and exposure to the target language. These models can then be used to inform the development of more effective language learning materials and teaching methods.Another way in which mathematics can be applied to English language learning is through the use of data visualization techniques. By representing language data in visual formats, such as graphs, charts, and diagrams, educators can help learners to better understand and internalize the patterns and structures of the language. This can be particularly useful in the teaching of grammar, where the visual representation of sentence structures and grammatical rules can make complex concepts more accessible and engaging for students.In addition to these more technical applications of mathematics, the discipline can also be used to enhance the overall learning experience for English language learners. For example, by incorporating mathematical problem-solving strategies into language learning activities, teachers can help students to develop critical thinking and analytical skills that are essential for success in both academic and professional settings. Furthermore, the use of numerical data and quantitative reasoning can help to make language learning more concrete and tangible, providing learnerswith a sense of progress and accomplishment as they master new linguistic concepts and skills.Overall, the integration of mathematical techniques and approaches into the field of English language learning represents a promising and exciting area of educational research and practice. By leveraging the power of data analysis, computational modeling, and visual representation, educators can develop more effective and engaging language learning materials and teaching methods, ultimately helping students to achieve greater proficiency and success in their pursuit of English language mastery.。
介绍一位语言学家英语作文

介绍一位语言学家英语作文English Response:A linguist is an individual who has a deepunderstanding of language and its intricate workings. They typically possess expertise in specific aspects of language, such as grammar, syntax, phonetics, and pragmatics, among others. Linguists approach language with a scientific perspective, seeking to unravel its systematic nature and identify patterns that govern its structure and usage.Linguists engage in various research activities, including analyzing linguistic data, studying language change, and exploring the relationships between different languages. They employ a combination of qualitative and quantitative methods, utilizing both descriptive and analytical approaches to advance our understanding of language. Their research contributes significantly to awide range of disciplines, including social sciences, humanities, computer science, and even healthcare.The field of linguistics encompasses a broad spectrumof subfields, each with its unique focus. For instance, sociolinguistics examines the relationship between language and society, while psycholinguistics investigates the psychological processes involved in language production and comprehension. Computational linguistics, on the other hand, explores the intersection of language and technology, developing computational models and tools for language processing.Linguists play a crucial role in various professions, including education, research, and language policy. Theyare often employed as teachers, researchers, or language consultants, using their expertise to educate students, conduct research, and inform language-related policies and practices. Their work has a significant impact on our understanding of language, communication, and culture, shaping how we interact with the world around us.中文回答:语言学家是对语言及其复杂运作有深刻理解的人。
大语言模型的训练流程

大语言模型的训练流程Training a large language model is a complex and time-consuming process that involves multiple steps and considerations. The first step in training a large language model is to gather and pre-process a massive amount of text data. This data is essential for training the model to understand and generate human-like language. In the case of just an English-speaking language model, this would likely involve compiling a diverse range of text from books, articles, websites, and other sources. The more varied and extensive the data, the better the model can learn to generate natural and coherent language.训练一个大型语言模型是一个复杂而耗时的过程,涉及多个步骤和考虑因素。
训练大语言模型的第一步是收集和预处理大量的文本数据。
这些数据对于训练模型理解和生成类似人类语言至关重要。
对于一个只有英语的语言模型来说,这可能涉及从书籍、文章、网站和其他来源编制多样化的文本。
数据越多样化和广泛,模型学习生成自然和连贯语言的能力就越好。
Once the text data is gathered, it needs to be pre-processed to remove any irrelevant or problematic content and to format it in a way that is suitable for training the language model. This may involvetasks such as tokenization, where the text is broken down into smaller units like words or characters, and filtering out any rare or non-standard terms that could negatively impact the model's learning process. Additionally, the data may need to be split into training, validation, and testing sets to evaluate the model's performance.一旦文本数据被收集,就需要对其进行预处理,以删除任何不相关或有问题的内容,并以适合训练语言模型的方式进行格式化。
语言学第六章Part One
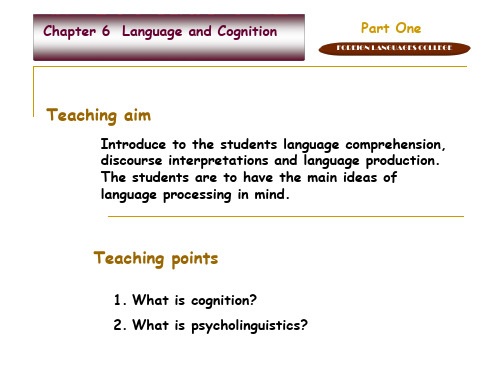
What that? Andrew want that. Not sit here.
Embed one constituent inside another:
Give doggie paper. Give big doggie paper.
Teaching points
1. What is cognition? 2. What is psycholinguistics?
Commonalities between language and cognition:
childhood cognitive development (Piaget):
[haj]: hi [s]: spray [sr]: shirt, sweater [sæ:]: what’s that?/ hey, look! [ma]: mommy [dæ ]: daddy
Fromkin,V., Rodman, R., & Hymans, N. (2007)An Introduction to Language (8th Ed.). Singapore/Beijing: Thompson/PUP
Two-word stage: around 18m
Child utterance Want cookie More milk Joe see My cup Mature speaker I want a cookie I want some more milk I (Joe) see you This is my cup Purpose Request Request Informing Warning
1. Diaries-Charles Darwin; 2. Tape recorders; 3. Videos and computers. Eg. Dr. Deb Roy (MIT)
The-Role-of-The-First-Language
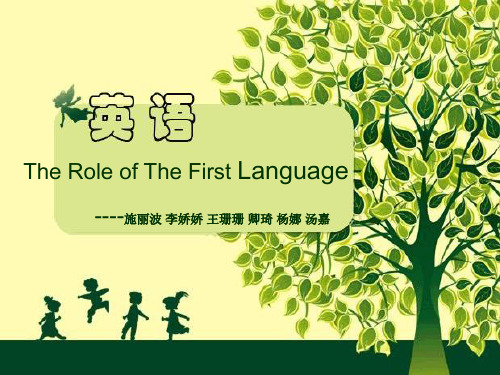
2、regarding the feasibility of comparing languages and the methodology of Contrastive Analysis.
3、reservations about whether Contrastive Analysis had anything relevant to offer to language teaching
Theoretical criticisms
➢The issues will be considered
(1) The attack on “Verbal Behaviors”
(2) The nature of the relationship between “difficulty” and “error”
目录
A multi-factor approach
L1 interference as a learner strategy
Contrastive Analysis
Contrastive pragmatics
Summary and conclusion
1 Introduction
Two popular belief
Empirical research and the predictability of error
➢Four causes of learner error:
1、the learner does not know the structural pattern and so makes a random response 2、 the correct model has been insufficiently practiced 3、distortion may be induced by the first language 4、the student may follow a general rule which is not applicable in particular instance
大语言模型核心技术介绍

大语言模型核心技术介绍Language models are a type of artificial intelligence system that has gained significant traction in recent years. These models are designed to process and generate human language, with the goal of improving natural language processing tasks such as translation, text generation, and question answering. Language models have become increasingly sophisticated, with the most recent advancements in large language models being some of the most significant in the field.语言模型是一种人工智能系统,近年来取得了显著的进展。
这些模型旨在处理和生成人类语言,以改进自然语言处理任务,如翻译、文本生成和问答。
语言模型变得越来越复杂,最近大语言模型的最新进展是该领域最重要的之一。
One of the key components of large language models is the use of neural networks. These networks are designed to mimic the way the human brain processes information, allowing them to learn from large amounts of data and improve their performance over time. By using neural networks, language models are able to understand thecomplex patterns and structures within human language, leading to more accurate and natural language generation.大型语言模型的关键组成部分之一是使用神经网络。
(完整版)胡壮麟语言学教程笔记、重点全解
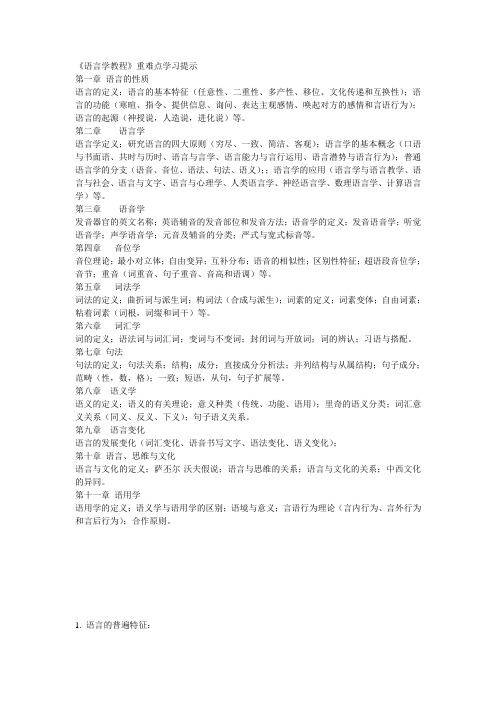
《语言学教程》重难点学习提示第一章语言的性质语言的定义:语言的基本特征(任意性、二重性、多产性、移位、文化传递和互换性);语言的功能(寒暄、指令、提供信息、询问、表达主观感情、唤起对方的感情和言语行为);语言的起源(神授说,人造说,进化说)等。
第二章语言学语言学定义;研究语言的四大原则(穷尽、一致、简洁、客观);语言学的基本概念(口语与书面语、共时与历时、语言与言学、语言能力与言行运用、语言潜势与语言行为);普通语言学的分支(语音、音位、语法、句法、语义);;语言学的应用(语言学与语言教学、语言与社会、语言与文字、语言与心理学、人类语言学、神经语言学、数理语言学、计算语言学)等。
第三章语音学发音器官的英文名称;英语辅音的发音部位和发音方法;语音学的定义;发音语音学;听觉语音学;声学语音学;元音及辅音的分类;严式与宽式标音等。
第四章音位学音位理论;最小对立体;自由变异;互补分布;语音的相似性;区别性特征;超语段音位学;音节;重音(词重音、句子重音、音高和语调)等。
第五章词法学词法的定义;曲折词与派生词;构词法(合成与派生);词素的定义;词素变体;自由词素;粘着词素(词根,词缀和词干)等。
第六章词汇学词的定义;语法词与词汇词;变词与不变词;封闭词与开放词;词的辨认;习语与搭配。
第七章句法句法的定义;句法关系;结构;成分;直接成分分析法;并列结构与从属结构;句子成分;范畴(性,数,格);一致;短语,从句,句子扩展等。
第八章语义学语义的定义;语义的有关理论;意义种类(传统、功能、语用);里奇的语义分类;词汇意义关系(同义、反义、下义);句子语义关系。
第九章语言变化语言的发展变化(词汇变化、语音书写文字、语法变化、语义变化);第十章语言、思维与文化语言与文化的定义;萨丕尔-沃夫假说;语言与思维的关系;语言与文化的关系;中西文化的异同。
第十一章语用学语用学的定义;语义学与语用学的区别;语境与意义;言语行为理论(言内行为、言外行为和言后行为);合作原则。
- 1、下载文档前请自行甄别文档内容的完整性,平台不提供额外的编辑、内容补充、找答案等附加服务。
- 2、"仅部分预览"的文档,不可在线预览部分如存在完整性等问题,可反馈申请退款(可完整预览的文档不适用该条件!)。
- 3、如文档侵犯您的权益,请联系客服反馈,我们会尽快为您处理(人工客服工作时间:9:00-18:30)。
GraSp: Grammar learning from unlabelled speech corporaPeter Juel HenrichsenCMOLCenter for Computational Modelling of Language c/o Dept. of Computational LinguisticsCopenhagen Business SchoolFrederiksberg, Denmarkpjuel@id.cbs.dkAbstractThis paper presents the ongoing projectComputational Models of First LanguageAcquisition, together with its currentproduct, the learning algorithm GraSp.GraSp is designed specifically forinducing grammars from large, unlabelledcorpora of spontaneous (i.e. unscripted)speech. The learning algorithm does notassume a predefined grammaticaltaxonomy; rather the determination ofcategories and their relations is consideredas part of the learning task. While GraSplearning can be used for a range ofpractical tasks, the long-term goal of theproject is to contribute to the debate ofinnate linguistic knowledge – under thehypothesis that there is no such.IntroductionMost current models of grammar learning assume a set of primitive linguistic categories and constraints, the learning process being modelled as category filling and rule instantiation – rather than category formation and rule creation. Arguably, distributing linguistic data over predefined categories and templates does not qualify as grammar 'learning' in the strictest sense, but is better described as 'adjustment' or 'adaptation'. Indeed, Chomsky, the prime advocate of the hypothesis of innate linguistic principles, has claimed that "in certain fundamental respects we do not really learn language" (Chomsky 1980: 134). As Chomsky points out, the complexity of the learning task is greatly reduced given a structure of primitive linguistic constraints ("a highly restrictive schematism", ibid.). It has however been very hard to establish independently the psychological reality of such a structure, and the question of innateness is still far from settled.While a decisive experiment may never be conceived, the issue could be addressed indirectly, e.g. by asking: Are innate principles and parameters necessary preconditions for grammar acquisition? Or rephrased in the spirit of constructive logic: Can a learning algorithm be devised that learns what the infant learns without incorporating specific linguistic axioms? The presentation of such an algorithm would certainly undermine arguments referring to the 'poverty of the stimulus', showing the innateness hypothesis to be dispensable.This paper presents our first try.1The essential algorithm1.1Psycho-linguistic preconditions Typical spontaneous speech is anything but syntactically 'well-formed' in the Chomskyan sense of the word.right well let's er --= let's look at the applications - erm - let me just ask initially this -- I discussed it with er Reith er but we'll = have to go into it a bit further - is it is it within our erm er = are we free er to er draw up a rather = exiguous list - of people to interview(sample from the London-Lund corpus) Yet informal speech is not perceived as being disorderly (certainly not by the language learning infant), suggesting that its organizingprinciples differ from those of the written language. So, arguably, a speech grammar inducing algorithm should avoid referring to the usual categories of text based linguistics –'sentence', 'determiner phrase', etc.1Instead we allow a large, indefinite number of (indistinguishable) basic categories – and then leave it to the learner to shape them, fill them up, and combine them. For this task, the learner needs a built-in concept of constituency. This kind of innateness is not in conflict with our main hypothesis, we believe, since constituency as such is not specific to linguistic structure.1.2Logical preliminariesFor the reasons explained, we want the learning algorithm to be strictly data-driven. This puts special demands on our parser which must be robust enough to accept input strings with little or no hints of syntactic structure (for the early stages of a learning session), while at the same time retaining the discriminating powers of a standard context free parser (for the later stages).Our solution is a sequent calculus, a variant of the Gentzen-Lambek categorial grammar formalism (L ) enhanced with non-classical rules for isolating a residue of uninterpretable sequent elements. The classical part is identical to L (except that antecedents may be empty).1 Hoekstra (2000) and Nivre (2001) discuss theannotation of spoken corpora with traditional tags.These seven rules capture the input parts that can be interpreted as syntactic constituents (examples below). For the remaining parts, we include two non-classical rules (σL and σR ).2By way of an example, consider the input stringright well let's er let's look at the applicationsas analyzed in an early stage of a learning session. Since no lexical structure has developed yet, the input is mapped onto a sequent of basic (dummy) categories:3c 29 c 22 c 81 c 5 c 81 c 215 c 10 c 1 c 891 ⇒ c 0Using σL recursively, each category of the antecedent (the part to the left of ⇒) is removed from the main sequent. As the procedure is fairly simple, we just show a fragment of the proof.Notice that proofs read most easily bottom-up.c 0–––––– σR c 81+ c 10+ c 1+ c 891+⇒ c 0–––––––––––––––––––––––– σL...–––––––––––––––––––– σLc 215+c 81 c 10 c 1 c 891 ⇒ c 0––––––––––––––––––––––––– σL c 5+c 81 c 215 c 10 c 1 c 891 ⇒ c 0––––––––––––––––––––––––––––––– σL ... c 5 c 81 c 215 c 10 c 1 c 891 ⇒ c 0In this proof there are no link s, meaning that no grammatical structure was found. Later, when the lexicon has developed, the parser may2 The calculus presented here is slightly simplified.Two rules are missing, and so is the reserved category T ('noise') used e.g. for consequents (in place of c 0 of the example). Cf. Henrichsen (2000).3 By convention the indexing of category names reflects the frequency distribution: If word W has rank n in the training corpus, it is initialized as W :c n .recognize more structure in the same input:–––––––l––––––––lc10⇒c10c891⇒c891–––––––––––––––––*Rc10 c891⇒ c10*c891c81 c215⇒ c0––––––l–––––––––––––––––––––––––––––/L c1⇒ c1c81 c215/(c10*c891) c10 c891⇒ c0–––––––––––––––––––––––––––––––––––––\L ... c81c215/(c10*c891)c10 c1c1\c891⇒ c0 ... let's look at the applicationsThis proof tree has three link s, meaning that the disorder of the input string (wrt. the new lexicon) has dropped by three degrees. More on disorder shortly.1.3The algorithm in outlineHaving presented the sequent parser, we now show its embedding in the learning algorithm GraSp (Gra mmar of Sp eech).For reasons mentioned earlier, the common inventory of categories (S, NP, CN, etc) is avoided. Instead each lexeme initially inhabits its own proto-category. If a training corpus has, say, 12,345 word types the initial lexicon maps them onto as many different categories. A learning session, then, is a sequence of lexical changes, introducing, removing, and manipulating the operators /, \, and * as guided by a well-defined measure of structural disorder.We prefer formal terms without a linguistic bias ("no innate linguistic constraints"). Suggestive linguistic interpretations are provided in square brackets.A-F summarize the learning algorithm.A) There are categories. Complex categories are built from basic categories using /, \, and *: Basic categoriesc1, c2, c3, ... , c12345 , ...Complex categoriesc1\c12345, c2/c3, c4*c5, c2/(c3\(c4*c5))B) A lexicon is a mapping of lexemes [word types represented in phonetic or enriched-orthographic encoding] onto categories.C) An input segment is an instance of a lexeme [an input word]. A solo is a string of segments [an utterance delimited by e.g. turntakes and pauses]. A corpus is a bag of soli [a transcript of a conversation].D) Applying an update L:C1→C2 in lexicon Lex means changing the mapping of L in Lex from C1 to C2. Valid changes are minimal, i.e. C2 is construed from C1 by adding or removing 1 basic category (using \, /, or *).E) The learning process is guided by a measure of disorder. The disorder function Dis takes a sequent Σ [the lexical mapping of an utterance] returning the number of uninterpretable atoms in Σ, i.e. σ+s and σ–s in a (maximally linked) proof. Dis(Σ)=0 iff Σ is Lambek valid. Examples: Dis( c a/c b c b⇒ c a )= 0Dis( c a/c b c b⇒ c c )= 2Dis( c b c a/c b⇒ c c )= 4Dis( c a/c b c c c b ⇒ c a )= 1Dis( c a/c c c b c a\c c ⇒ c a )= 2DIS(Lex,K) is the total amount of disorder in training corpus K wrt. lexicon Lex, i.e. the sum of Dis-values for all soli in K as mapped by Lex.F) A learning session is an iterative process. In each iteration i a suitable update U i is applied in the lexicon Lex i–1 producing Lex i . Quantifying over all possible updates, U i is picked so as to maximize the drop in disorder (DisDrop):DisDrop = DIS(Lex i–1,K) – DIS(Lex i,K)The session terminates when no suitable update remains.It is possible to GraSp efficiently and yet preserve logical completeness. See Henrichsen (2000) for discussion and demonstrations.1.4A staged learning sessionGiven this tiny corpus of four soli ('utterances')if you must you canif you must you must and if we must we mustif you must you can and if you can you mustif we must you must and if you must you must, GraSp produces the lexicon below.As shown, training corpora can be manufactured so as to produce lexical structure fairly similar to what is found in CG textbooks. Such close similarity is however not typical of 'naturalistic' learning sessions – as will be clear in section 2.1.5 Why categorial grammar?In CG, all structural information is located in the lexicon. Grammar rules (e.g. VP→V t N) and parts of speech (e.g. 'transitive verb', 'common noun') are treated as variants of the same formal kind. This reduces the dimensionality of thelogical learning space, since a CG-based learner needs to induce just a single kind of structure.Besides its formal elegance, the CG basis accomodates a particular kind of cognitive models, viz. those that reject the idea of separate mental modules for lexical and grammatical processing (e.g. Bates 1997). As we see it, our formal approach allows us the luxury of not taking sides in the heated debate of modularity.5 2Learning from spoken languageThe current GraSp implementation completes a learning session in about one hour when fed with our main corpus.6 Such a session spans 2500-4000 iterations and delivers a lexicon rich 4 For perspicuity, two of the GraSped categories –viz. 'can':(c2\c5)*(c5\c1) and 'we':(c2/c6)*c6 – are replaced in the table by functional equivalents.5 A caveat: Even if we do share some tools with other CG-based NL learning programmes, our goals are distinct, and our results do not compare easily with e.g. Kanazawa (1994), Watkinson (2000). In terms of philosophy, GraSp seems closer to connectionist approaches to NLL.6 The Danish corpus BySoc (person interviews). Size: 1.0 mio. words. Duration: 100 hours. Style: Labovian interviews. Transcription: Enriched orthography. Tagging: none. Ref.: http://www.cphling.dk/BySoc in microparadigms and microstructure. Lexical structure develops mainly around content words while most function words retain their initial category. The structure grown is almost fractal in character with lots of inter-connected categories, while the traditional large open classes − nouns, verbs, prepositions, etc. − are absent as such. The following sections present some samples from the main corpus session (Henrichsen 2000 has a detailed description). 2.1Microparadigms{ "Den Franske", "Nyboder","Sølvgades", "Krebses" } These four lexemes – or rather lexeme clusters –chose to co-categorize. The collection does not resemble a traditional syntactic paradigm, yet the connection is quite clear: all four items appeared in the training corpus as names of primary schools.The final categories are superficially different, but are easily seen to be functionally equivalent.The same session delivered several other microparadigms: a collection of family members (in English translation: brother, grandfather, younger-brother, stepfather, sister-in-law, etc.), a class of negative polarity items, a class of mass terms, a class of disjunctive operators, etc. (Henrichsen 2000 6.4.2).GraSp-paradigms are usually small and almost always intuitively 'natural' (not unlike the small categories of L1 learners reported by e.g. Lucariello 1985).2.2MicrogrammarsGraSp'ed grammar rules are generally not of the kind studied within traditional phrase structure grammar. Still PSG-like 'islands' do occur, in the form of isolated networks of connected lexemes.Centred around lexeme 'Pauls', a microgrammar (of street names) has evolved almost directly translatable into rewrite rules:7PP → 'i' N 1 'Gade'PP → 'på' N 1 'Plads'PP → 'på' N 2N 1→ X 'Pauls'N 2→ X 'Annæ'N x → X YX → 'Sankt' | 'Skt.' | 'Sct.'Y→ 'Pauls' | 'Josef' | 'Joseph' | 'Knuds' | ...2.3Idioms and locutionsConsider the five utterances of the main corpus containing the word 'rafle' (cast-dice INF ):8det gør den der er ikke noget at rafle om der der er ikke så meget at rafle om der er ikke noget og rafle omsætte sig ned og rafle lidt med fyrene der at rafle om derOn most of its occurrences, 'rafle' takes part in the idiom "der er ikke noget/meget og/at rafle om", often followed by a resumptive 'der'(literally: there is not anything /much and /to7 Lexemes 'Sankt', 'Sct.', and 'Skt.' have in effectcocategorized, since it holds that (x /y )*y ⇒ x . This cocategorization is quite neat considering that GraSp is blind to the interior of lexemes. c 9 and c 22 are the categories of 'i' (in ) and 'på' (on ).8 In writing, only two out of five would probably qualify as syntactically well-formed sentences.cast-dice INF about (there ), meaning: this is not a subject of negotiations). Lexeme 'ikke' (category c 8) occurs in the left context of 'rafle' more often than not, and this fact is reflected in the final category of 'rafle':rafle: ((c 12\(c 8\(c 5\(c 7\c 5808))))/c 7)/c 42Similarly for the lexemes 'der' (c 7), 'er' (c 5), 'at'(c 12), and 'om' (c 42) which are also present in the argument structure of the category, while the top functor is the initial 'rafle' category (c 5808).The minimal context motivating the full rafle category is:("..." means that any amount and kind of material may intervene). This template is a quite accurate description of an acknowledged Danish idiom.Such idioms have a specific categorial signature in the GraSped lexicon: a rich, but flat argument structure (i.e. analyzed solely by σR )centered around a single low-frequency functor (analyzed by σL ). Further examples with the same signature:... i ...– all well-known Danish locutions.9There are of course plenty of simpler and faster algorithms available for extracting idioms.Most such algorithms however include specific knowledge about idioms (topological and morphological patterns, concepts of mutual information, heuristic and statistical rules, etc.).Our algorithm has no such inclination: it does not search for idioms, but merely finds them.Observe also that GraSp may induce idiom templates like the ones shown even from corpora without a single verbatim occurrence.9 For entry rafle , Danish-Danish dictionary Politikenhas this paradigmatic example: "Der er ikke noget at rafle om". Also fortænke , blæse , kinamands have examples near-identical with the learned templates.3Learning from exotic corporaIn order to test GraSp as a general purpose learner we have used the algorithm on a range of non-verbal data. We have had GraSp study melodic patterns in musical scores and prosodic patterns in spontaneous speech (and even dna-structure of the banana fly). Results are not yet conclusive, but encouraging (Henrichsen 2002).When fed with HTML-formatted text, GraSp delivers a lexical patchwork of linguistic structure and HTML-structure. GraSp's uncritical appetite for context-free structure makes it a candidate for intelligent web-crawling. We are preparing an experiment with a large number of cloned learners to be let loose in the internet, reporting back on the structure of the documents they see. Since GraSp produces formatting definitions as output (rather than requiring it as input), the algorithm could save the www-programmer the troubles of preparing his web-crawler for this-and-that format.Of course such experiments are side-issues. However, as discussed in the next section, learning from non-verbal sources may serve as an inspiration in the L1 learning domain also.4Towards a model of L1 acquisition4.1Artificial language learningTraining infants in language tasks within artificial (i.e. semantically empty) languages is an established psycho-linguistic method. Infants have been shown able to extract structural information – e.g. rules of phonemic segmentation, prosodic contour, and even abstract grammar (Cutler 1994, Gomez 1999, Ellefson 2000) – from streams of carefully designed nonsense. Such results are an important source of inspiration for us, since the experimental conditions are relatively easy to simulate. We are conducting a series of 'retakes' with the GraSp learner in the subject's role. Below we present an example.In an often-quoted experiment, psychologist Jenny Saffran and her team had eight-months-old infants listening to continuous streams of nonsense syllables: ti, do, pa, bu, la, go, etc. Some streams were organized in three-syllable 'words' like padoti and golabu (repeated in random order) while others consisted of the same syllables in random order. After just two minutes of listening, the subjects were able to distinguish the two kinds of streams. Conclusion: Infants can learn to identify compound words on the basis of structural clues alone, in a semantic vacuum.Presented with similar streams of syllables, the GraSp learner too discovers word-hood.It may be objected that such streams of presegmented syllables do not represent the experimental conditions faithfully, leaping over the difficult task of segmentation. While we do not yet have a definitive answer to this objection, we observe that replacing "pa do ti go la bu (..)" by "p a d o t i g o l a b u (..)" has the GraSp learner discover syllable-hood and word-hood on a par.114.2Naturalistic language learningEven if human learners can demonstrably learn structural rules without access to semantic and pragmatic cues, this is certainly not the typical L1 acquisition scenario. Our current learning model fails to reflect the natural conditions in a number of ways, being a purely syntactic calculus working on symbolic input organized in well-delimited strings. Natural learning, in contrast, draws on far richer input sources:•continuous (unsegmented) input streams •suprasegmental (prosodic) information•sensory data•background knowledge10 As seen, padoti has selected do for its functional head, and golabu, bu. These choices are arbitrary.11 The very influential Eimas (1971) showed one-month-old infants to be able to distinguish /p/ and /b/. Many follow-ups have established that phonemic segmentation develops very early and may be innate.Any model of first language acquisition must be prepared to integrate such information sources. Among these, the extra-linguistic sources are perhaps the most challenging, since they introduce a syntactic-semantic interface in the model. As it seems, the formal simplicity of one-dimensional learning (cf. sect. 1.5) is at stake.If, however, semantic information (such as sensory data) could be 'syntactified' and included in the lexical structure in a principled way, single stratum learning could be regained. We are currently working on a formal upgrading of the calculus using a framework of constructive type theory (Coquant 1988, Ranta 1994). In CTT, the radical lexicalism of categorial grammar is taken even a step further, representing semantic information in the same data structure as grammatical and lexical information. This formal upgrading takes a substantial refinement of the Dis function (cf. sect. 1.3 E) as the determination of 'structural disorder' must now include contextual reasoning (cf. Henrichsen 1998). We are pursuing a design with σ+ and σ– as instructions to respectively insert and search for information in a CTT-style context.These formal considerations are reflections of our cognitive hypotheses. Our aim is to study learning as a radically data-driven process drawing on linguistic and extra-linguistic information sources on a par – and we should like our formal system to fit like a glove.5Concluding remarksAs far as we know, GraSp is the first published algorithm for extracting grammatical taxonomy out of untagged corpora of spoken language.12 This in an uneasy situation, since if our findings are not comparable to those of other approaches to grammar learning, how could our results be judged − or falsified? Important issues wide open to discussion are: validation of results, psycho-linguistic relevance of the experimental setup, principled ways of surpassing the context-free limitations of Lambek grammar (inherited in GraSp), just to mention a few.On the other hand, already the spin-offs of our project (the collection of non-linguistic learners) do inspire confidence in our tenets, we 12 The learning experiment sketched in Moortgat (2001) shares some of GraSp's features.think – even if the big issue of psychological realism has so far only just been touched.The GraSp implementation referred to in this paper is available for test runs athttp://www.id.cbs.dk/~pjuel/GraSpReferencesBates, E.; J.C. Goodman (1997) On the Inseparability of Grammar and the Lexicon: Evidence From Acquisition, Aphasia, and Real-time Processing; Language and Cognitive Processes 12, 507-584 Chomsky, N. (1980) Rules and Representations; Columbia Univ. PressCoquant, T.; G. Huet (1988) The Calculus of Constructions; Info. & Computation 76, 95-120 Cutler, A. (1994) Segmentation Problems, Rhythmic Solutions; Lingua 92, 81-104Eimas, P.D.; E.D. Siqueland; P.W. Jusczyk (1971) Speech Perception in Infants; Science 171 303-306 Ellefson, M.R.; M.H.Christiansen (2000) Subjacency Constraints Without Universal Grammar: Evidence from Artificial Language Learning and Connectionist Modelling;22nd Ann. Conference of the Cognitive Science Society, Erlbaum, 645-650 Gomez, R.L.; L.A. Gerken (1999) Artificial Gram-mar Learning by 1-year-olds Leads to Specific and Abstract Knowledge; Cognition 70 109-135 Henrichsen, P.J. (1998) Does the Sentence Exist? Do We Need It?; in K. Korta et al. (eds) Discourse, Interaction, and Communication; Kluwer Acad. Henrichsen, P.J. (2000) Learning Within Grasp −Interactive Investigations into the Grammar of Speech; Ph.D., http://www.id.cbs.dk/~pjuel/GraSp Henrichsen, P.J. (2002) GraSp: Grammar Learning With a Healthy Appetite (in prep.)Hoekstra, H. et al. (2000) Syntactic Annotation for the Spoken Dutch Corpus Project; CLIN2000 Kanazawa (1994) Learnable Classes of CG; Ph.D. Moortgat, M. (2001) Structural Equations in Language Learning; 4th LACL2001 1-16Nivre, J.; L. Grönqvist (2001) Tagging a Corpus of Spoken Swedish: Int. Jn. of Corpus Ling. 6:1 47-78 Ranta, A. (1994) Type-Theoretical Grammar; Oxford Saffran, J.R. et al. (1996) Statistical Learning By 8-Months-Old Infants; Science 274 1926-1928 Watkinson S.; S. Manandhar (2000) Unsupervised Lexical Learning with CG; in Cussens J. et al. (eds) Learning Language in Logic; Springer。