Endogenous Network Dynamics
economic造句
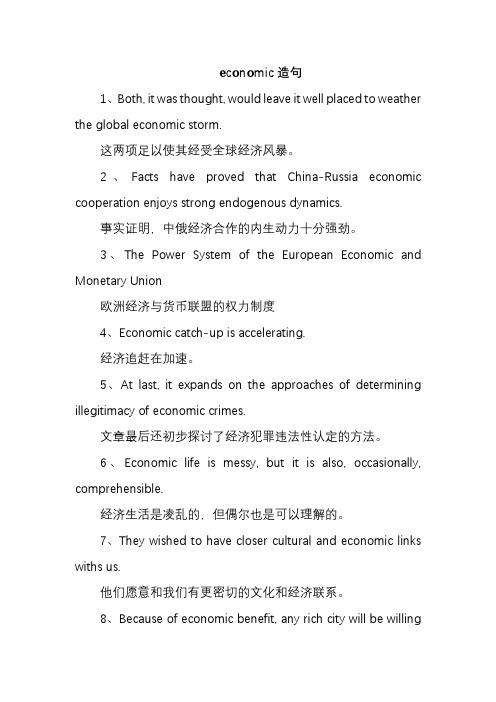
economic造句1、Both, it was thought, would leave it well placed to weather the global economic storm.这两项足以使其经受全球经济风暴。
2、Facts have proved that China-Russia economic cooperation enjoys strong endogenous dynamics.事实证明,中俄经济合作的内生动力十分强劲。
3、The Power System of the European Economic and Monetary Union欧洲经济与货币联盟的权力制度4、Economic catch-up is accelerating.经济追赶在加速。
5、At last, it expands on the approaches of determining illegitimacy of economic crimes.文章最后还初步探讨了经济犯罪违法性认定的方法。
6、Economic life is messy, but it is also, occasionally, comprehensible.经济生活是凌乱的,但偶尔也是可以理解的。
7、They wished to have closer cultural and economic links withs us.他们愿意和我们有更密切的文化和经济联系。
8、Because of economic benefit, any rich city will be willingto export resource to any poor one.经济利益上原因是一些富裕的城市将要出口资源到贫穷的城市。
9、Governmental Man is not an Economic Man but a Public Man政府人是公共人而非经济人10、Italy's poor economic performance is sufficient evidence of his failure to sweep away that machine.意大利糟糕的经济表现足以说明他未能扫除那个核心。
环境工程专业英语第二版重点课文翻译及复习
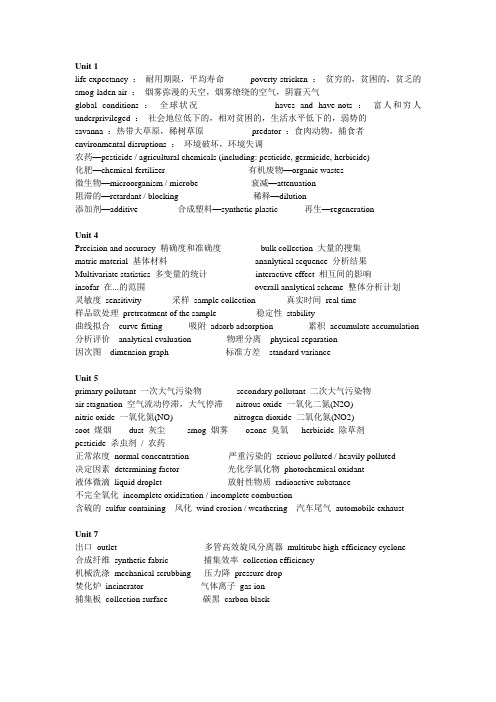
life expectancy :耐用期限,平均寿命poverty-stricken :贫穷的,贫困的,贫乏的smog-laden air :烟雾弥漫的天空,烟雾缭绕的空气,阴霾天气global conditions :全球状况haves and have-nots :富人和穷人underprivileged :社会地位低下的,相对贫困的,生活水平低下的,弱势的savanna :热带大草原,稀树草原predator :食肉动物,捕食者environmental disruptions :环境破坏,环境失调农药—pesticide / agricultural chemicals (including: pesticide, germicide, herbicide)化肥—chemical fertilizer 有机废物—organic wastes微生物—microorganism / microbe 衰减—attenuation阻滞的—retardant / blocking 稀释—dilution添加剂—additive 合成塑料—synthetic plastic 再生—regenerationUnit 4Precision and accuracy 精确度和准确度bulk collection 大量的搜集matric material 基体材料ananlytical sequence 分析结果Multivariate statistics 多变量的统计interactive effect 相互间的影响insofar 在...的范围overall analytical scheme 整体分析计划灵敏度sensitivity 采样sample collection 真实时间real time样品欲处理pretreatment of the sample 稳定性stability曲线拟合curve-fitting 吸附adsorb adsorption 累积accumulate accumulation 分析评价analytical evaluation 物理分离physical separation因次图dimension graph 标准方差standard varianceUnit 5primary pollutant 一次大气污染物secondary pollutant 二次大气污染物air stagnation 空气流动停滞,大气停滞nitrous oxide 一氧化二氮(N2O)nitric oxide 一氧化氮(NO) nitrogen dioxide 二氧化氮(NO2)soot 煤烟dust 灰尘smog 烟雾ozone 臭氧herbicide 除草剂pesticide 杀虫剂/ 农药正常浓度normal concentration 严重污染的serious polluted / heavily polluted决定因素determining factor 光化学氧化物photochemical oxidant液体微滴liquid droplet 放射性物质radioactive substance不完全氧化incomplete oxidization / incomplete combustion含硫的sulfur-containing 风化wind erosion / weathering 汽车尾气automobile exhaustUnit 7出口outlet 多管高效旋风分离器multitube high-efficiency cyclone合成纤维synthetic fabric 捕集效率collection efficiency机械洗涤mechanical scrubbing 压力降pressure drop焚化炉incinerator 气体离子gas ion捕集板collection surface 碳黑carbon black尾气off-gas 可应用性applicability 工业规模full-scale 土壤床soil bed生物过滤器biofilter 固定资本fixed capital 易生物降解的easily biodegraded VOC 挥发性有机化合物APC 大气污染控制Regulatory program 调整项目Financial support 财政支持Operating cost 操作成本Biodegradation capacity 生物降解能力Environmental media 环境介质Biological 生物学的Technologies 技术、工艺Inorganic air pollutants 无机大气污染物Unit 10treatment facilities 处理设备municipality 市政当局, 自治市population equivalent 人口当量basement flooding 地下室浸水per capita per day 每人每天runoff 排水domestic sewage 生活污水type of terrain 地形种类Unit 12land disposal 掩埋处置fecal coliform 粪大肠菌群stringent effluent requirement 严格的废水排放要求assimilation capacity 同化能力practical outlets 可行的排出途径,现实出路aquatic life 水生生物detrimental to human health 对人体健康有害的endogenous phase 内源〔生长〕期Unit 13flow monitoring 流量监测equipment age and reliability 装备老化及其可靠性mechanistic facilities 机械设备microbial activity 微生物活性activated sludge 活性污泥controlling respiration 控制呼吸oxidation ditches 氧化沟on-line automation 在线自动〔监测〕手动控制operator control/ manual control 最优化minimize the effects微处理器microprocessor 统计分析statistical analysis质量衡算mass balance 动力学dynamics氧化还原oxidation and reduction /redox 停留时间residence time模拟simulation 参数parameter 水解hydrolysis 积分integralUnit 1 环境工程本书的内容:本书的目的是使工科和理科学生对环境问题的跨学科的研究有所了解:环境问题的起因,环境问题受关注的原因,如何控制环境问题。
衰老生理

地上部分衰老,多年生草本植物,地上部每年死亡,而根系和其 他地下系统仍然继续生存多年。 渐进衰老,比如多年生常绿木本植物的茎和根能生活多年,而叶片 和繁殖器官则渐次衰老脱落。 落叶衰老,多年生落叶木本植物,发生季节性的叶片同步衰老脱落。
多年生木本植物落叶衰老
落叶衰老
落叶衰老
◆
3.植物衰老的调控
基因
植物生长物质 环境
3.1、衰老的基因调控
植物衰老过程伴随着基因表达的变化。 如植物叶片衰老过程中可能有一些基因受 到抑制而低水平表达,甚至完全不表达; 而另一些基因则在衰老期间被激活,其表 达增强。在衰老的早期叶片中部分mRNA水 平显著下降,如与光合有关的蛋白质、电 子传递体、光系统Ⅱ的基因表达量随叶片 衰老而急剧下降;而另一些如编码水解酶、 催化乙烯生物合成的ACC合成酶和ACC氧 化酶却增加
4.2.1超高产玉米--叶片
超高产春玉米花粒期不同层位叶片 POD活性表现为下位叶> 穗位叶 > 上位叶;CAT、SOD 活性表现为上位叶 > 穗位叶 > 下位叶。灌浆期 玉米不同层位叶片净光合速率、气孔导度、蒸腾速率变化趋势表现为 穗位叶 > 上位叶 > 下位叶;乳熟期不同层位叶片净光合速率、气孔导 度、蒸腾速率表现为上位叶 > 穗位叶 > 下位叶。表明在玉米花粒期, 行间覆膜处理可以提高玉米上位叶及穗位叶的净光合速率、POD 活 性、CAT 活性及 SOD 活性,减小气孔导度,降低叶片蒸腾速率,延 缓叶片衰老,维持花粒期生理功能,保证子粒充分灌浆,提高千粒重, 进而提高产量,实现超高产。(桑丹丹,高聚林等不同覆膜方式下超 高产春玉米花粒期叶片衰老特性研究 玉 米 科 学2009,17(5):77~ 81) 国内外许多学者认为,延缓玉米叶片衰老,保持叶片绿色,延长叶片 光合作用时间,能够提高并延长玉米抽丝后群体光合速率,显著增加 产量;而花后叶片早衰,绿叶面积减小,光合时间缩短,则会明显降 低籽粒产量[1-2]。Gentinetta[3]和 Huffaker[4]等研究的一种具有保绿 习性的玉米自交系变种,在大田试验中取得了显著的增产效果,这也 间接证明了叶片衰老快慢与产量有着密切关系。(活杆成熟)Osikca M.研究指出,在玉米生育后期,叶片维持较高的 CO2同化能力及较 长的功能期是玉米实现高产的前提条件之一[5]。此外,Thomas 等研 究表明,在玉米叶片延衰的同时其产量也会相应提高。
生态学专业英语词汇
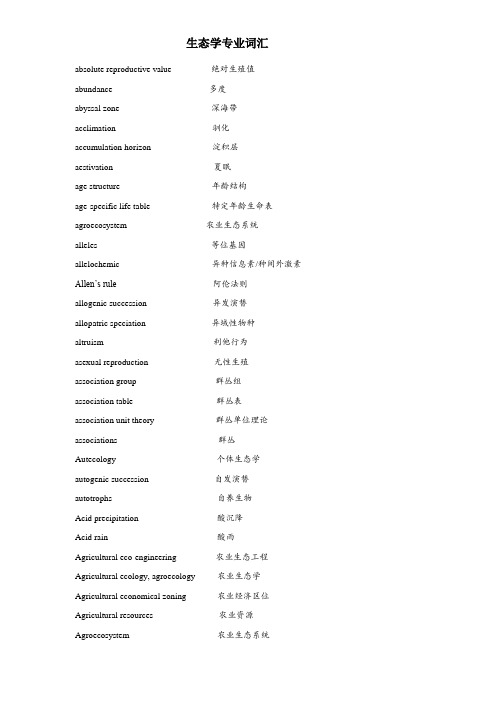
absolute reproductive value 绝对生殖值abundance 多度abyssal zone 深海带acclimation 驯化accumulation horizon 淀积层aestivation 夏眠age structure 年龄结构age-specific life table 特定年龄生命表agroecosystem 农业生态系统alleles 等位基因allelochemic 异种信息素/种间外激素Allen’s rule 阿伦法则allogenic succession 异发演替allopatric speciation 异域性物种altruism 利他行为asexual reproduction 无性生殖association group 群丛组association table 群丛表association unit theory 群丛单位理论associations 群丛Autecology 个体生态学autogenic succession 自发演替autotrophs 自养生物Acid precipitation 酸沉降Acid rain 酸雨Agricultural eco-engineering 农业生态工程Agricultural ecology, agroecology 农业生态学Agricultural economical zoning 农业经济区位Agricultural resources 农业资源Agroecosystem 农业生态系统Agroforestry 农林业系统Allee’s principle 阿利氏群聚原则Allelochemicals 化感物质Allelopathy 化感作用Artificial auxiliary energy 人工辅助能Artificial direct regulation 人工直接调控Artificial information flow 人工信息流Auxiliary energy 辅助能balancing selection hypothesize 平衡说basal area 底面积Bergman’s rule 贝格曼规律bethal zone 半深海带biocoenosis 生物群落bioconcentration 生物浓缩biological enrichment 生物富集biomagnification 生物放大biomass 生物量biome 生物带bionomic strategy 生态对策biosphere 生物圈Biodynamic agriculture 生物动力农业Biogecochemical cycle 生物地球化学循环Biological agriculture 生物农业Biological control 生物防治Biological energy subsidies 生物辅助能Biological oxygen demand BOD 生化需氧量生化耗氧量Biomass 生物量Bionomic strategies 生态对策Biological micro-cycle 生物小循环bottle neck 瓶颈C3 plant C3植物C4 plant C4植物CAM plant CAM植物Capital flow 资金流Carrying capacity 环境容纳量Chemical ecology 化学生态学Chemical oxygen demand COD 化学耗氧量Chinese Ecological Agriculture 中国生态农业Climax 顶极群落Coase’s theory 高斯理论Coevolution 协同进化Commensalisms 偏利作用Community 群落Competition 竞争Competition exclusion principle 竞争排斥原理Components structure 组分结构Controlled availability fertilizers, CAFs 控效肥料Crop productivity potential 作物生产潜力cannibalism 同种相食carnivores 食肉动物carring capacity 负荷量catastrophic 灾难性因素chamaephytesCh 地上芽植物character displacement 特征替代cheliophytes 阴性植物climate climax 气候顶级climate school 气候学派climax community 顶级群落climax 顶级群落cline 渐变群closed community 封闭群落clumped 集群分布coadapted system 协同适应系统coarse grained 粗粒性分布co-dynamics 相互动态co-evolution 协同进化cohort 同生群cold desert 冷荒漠colonization 定居, 建群coloration 色泽communities in littoral zone 沿岸生物群落communities in the limnetic zone 湖沼带生物群落communities in the profundal zone 深水带生物群落Community ecology 群落生态学community matrix 群落矩阵community organization 群落组织community 群落companion species 伴生种compen-satory predation 补偿性捕食competition coefficient 竞争系数competition hypothesis 竞争假说competitive exclusion 竞争排除competive lottery 抽彩式竞争conservation 保守主义者conspicuousness 显著度constancy 恒有度contest competition 干扰竞争contest type of competition 争夺型竞争continental rise 大陆隆continental shelf 大陆架continental slope 大陆坡convergent adaptation 趋同适应convergent oscillation 趋同波动cost of gene recombination 基因重组价cost of mating 交配价cost of meiosis 减数分裂价courtship behavior 求偶行为coverage 盖度crude density 原始密度cryptophytesCr 隐芽植物Decomposer 分解者Desertification 土地沙漠化Detritus food chain 腐食食物链decomposition 分解作用delayed density dependence 延后密度制约density effect 密度效应density ratio 密度比density-dependent 密度制约density-independent 非密度制约deterministic model 决定模型detrial food chain 碎食物链detritus feeder 食碎生物development 发育differential species 区别种diffuse competition 分散竞争diominant-submissive 支配—从属directional selection 定向选择discrete generation 离散世代disruptive selection 分裂选择disturbance climax/disclimax 偏途顶级divergent oscillation 趋异波动dominance 优势度dominant species 建群种dominant species 优势种dominant-submissive 支配—从属关系dry desert 干荒漠dynamic classification 动态分类系统dynamic life table 动态生命表dynamic-composite life table 动态混合生命表earth nucleus 地幔earth’s crust 地核Ecological amplitude 生态幅ecological density 生态密度ecological dominance 生态优势ecological environment 生态环境ecological equivalent 生态等值种ecological factor 生态因子ecological force 生态力ecological invasion 生态入侵ecological natality 生态出生率ecological release 生态释放Ecology 生态学ecosystem development 生态系统的发育Ecosystem ecology 生态系统生态学ecosystem 生态系统acetone hypothesis 生态交错带假说ecotype 生态型ectoderm 外温动物edge effect 边缘效应etiolating phenomenon 黄化现象emergy 能值emigration 迁出endogenous migration 内因性迁移endoderm 内温动物environment hormone 环境激素environment 环境equilibrium theory 平衡理论euphonic zone 透光带euryecious 广栖的euryhaline 广盐性的euryhydric 广水性的euryphagic 广食性的eurythermal 广温性的eurytopic species 广适种eutrophication 富营养化evergreen hardwood forest 常绿硬材林Evolution ecology 进化生态学evolution force 进化力exogenous migration 外因性迁移exploitive competition 利用竞争exponetial growth 指数增长Eco-economical zoning 生态经济区位Ecological agriculture 生态农业Ecological agriculture model pattern 生态农业模式Ecological density 生态密度Ecological effect 生态效益Ecological efficiency 生态效率Ecological factor 生态因子Ecological engineering 生态工程Ecological pyramid 生态金字塔生态锥体Ecological succession 演替Ecological sustainability 生态持续性Ecology 生态学Economic effect 经济效益Economic externality 经济外部性Ecosystem 生态系统Ecotone 群落交错区生态交错区Ecotope 景观元素生态点Ecotype 生态型Edge effect 边缘效应Embodied energy 内含能Emergent property of system 系统的整合特性Energy analysis 能流分析Energy flow chart 能流图Energy flow path 能流路径Energy flow structure 能流结构Energy flowing 能量流Entropy 熵Eutrophication 富营养化Exponential growth form 种群指数增长模式External benefit 收益外泄External cost 成本外摊Feed back 反馈作用First law of thermodynamics 热力学第一定律Fish pond-dike system 基塘系统Flow 流Food chain 食物链Food chain structure 食物链结构Food web 食物网Free energy 自由能First law of resources economic 资源经济学第一定律Functional component adundence 功能组分冗余facultative parthenogenesis 孤雌生殖facultative parthenogenesis 兼性孤雌生殖facultative 兼性因素family selection 家庭选择farmland ecosystem 农田生态系统fecundity schedule 生育力表fecundity 生育力feedback 反馈feeding niches 取食生态位filter food 滤食性生物fine grained 细粒性分布finite rate of increase 周限增长率fitness 合适度floristic-structural classification 植物区系—结构分类系统fluctuation 波动food chain 食物链formation group 群系组formation 群系formation 植物群系foundational niche 基础生态龛freshwater ecology 淡水生态学freshwater ecosystem 淡水生态系统function response 功能反应gamete selection 配子选择gaps 缺口gaseous cycle 气体循环geng pool 基因库geographic variation 地理变异geographical theory of speciation 物种形成geometric growth 几何级数增长geophytesG 地下芽植物global ecology 全球生态gradient hypothesis 梯度假说grain 粒性grazer 牧食生物grazing food chain 捕食食物链greenhouse effect 温室效应gregarization pheromone 聚集信息素gross primary production 总初级生产力group selection 群体选择growth form 生长型guild 同资源团habal zone 深渊带habitat 生境heath 石楠群丛height 高度hemicryptophytesHe 地面芽植物herbivore 植食herbivores 植食动物heterotrophic succession 异养演替heterotrophs 异养生物hibernation 冬眠homeostasis 内稳态homeostasis 自调节稳态homeostatic organism 内稳态生物homeostatic process 内稳定过程human demography 人口统计学Human ecology 人类生态学humus 腐殖质hydrarch succession 水生演替hydrosere 水生演替系列immigration 迁入importance value 重要值individual converse rate 个体转化率industrial melanism 工业黑化现象inner capacity increase 内禀增长力inner-environment 内环境instantaneous rate of increase 瞬间增长率intensity 强度interference competition 干扰竞争interference competition 干扰竞争intermediate disturbance hypothesis 中度干扰假说internal distribution pattern 内分布型interspecfic relationship 种间关系intertidal zone 潮间带intraspecific relationship 种内关系inversely density dependent 反密度制约Island ecology 岛屿生态学iteroparity 多次繁殖生物iteroparous 多次生殖Jordan’s rule 约丹定律keystone species 关键种kin selection 亲属选择kin selection 亲属选择k-strategists k-策略者land cover 土地覆盖land use 土地利用Landscape ecology 景观生态学law of constant final yield 最后产量衡值法则law of the minimum 最小因子法则law of tolerance 耐受性法则layer 层leached layer 淋溶层leaf area index 叶面积指数leaf area indexLAS 叶面积指数lentic ecosystem 静水生态系统life cycle 生活周期life expectancy 生命期望life form spectrum 生活型谱life form 生活型life history strategy 生活史对策life history 生活史lifespan 生活年限lifetime 寿命limit of tolerance 忍受性限度littoral zone 沿岸带lotic ecosystem 流水生态系统macroclimate 大气候macrofauna 大型生物Marine ecology 海洋生态学marine ecosystem 海洋生态系统maximum natality 最大出生率maximum sustained yield 最大持续生产量megafauna 巨型生物mexofauna 中型生物microclimate 小气候microcolony 微菌落microcommunities 小群落micro-ecosystem 微生态系统micro-environment 微环境microfauna 小型生物microplankton 小型浮游生物migration 迁徙modular organism 构件生物modules 构件Molecular ecology 分子生态学monoclimax theory 单元演替顶级monocultute 单种养殖monogamy 单配偶制monpohagous 单食性monsoon forest 季风林mortality curve 死亡曲线mortality 死亡率mosaic 镶嵌性natality 出生率n-dimensional niche n 维生态龛negative feedback 负反馈net primary productionGP 净初级生产力neutrality controversy 中性说论战niche compression 生态位压缩niche separation 生态位分离niche shift 生态位分离niche 生态位non-equilibrium theory 非平衡理论non-homeostatic organism 非内稳态生物obiotic component 非生物成分ocean-current 洋流ohort life table 同群生命表omnivores 杂食动物open community 开放群落open shrublands 稀疏灌丛opportunist 机会主义者ordination 排序ornamentation 修饰panclimax 泛顶级pantropical 泛热带区parabiosphere 副生物圈parasites 寄生生物parasitoidism 拟寄生parent material 母质层parental care 亲代关怀parental investment 亲本投资patchiness 斑块性per capita growth rate 每员增长率perclimax 前顶级peritrophic mycorrhizae 周边营养性菌根phanerophytesPh 厄尔尼诺El Nino photoperiodism 光周期现象phyplankton 浮游植物physiognomy 外貌Physiological ecology 生理生态学physiological natality 生理出生率phytochrom 色素pioneer community 先锋群落pjarapatric speciation 邻域性物种plankon 浮游生物Poission distribution 泊松分布polyandry 一雌多雄制polyclimax theory 多元顶级理论polygamy 多配偶制polygyny 一雄多雌制polymorphism 多型现象polyphagous 多食性的Population ecology 种群生态学population 种群porosity 粒间空隙positive feedback 正反馈postclimax 超顶级predation 捕食者predator 捕食者present reproductive value 当年繁殖价值prevail climax 优势顶级prey 猎物prezygotic mechanism 合子前隔离primary production 初级生产primary succession 初级演替primary succession 原生演替principle of allocation 分配原理principle of competitive exclusion 竞争互斥原理production rate 生产率production 生产量productivity 生产力progressive succession 进展演替protogynous hermaphriodism 雌雄同体pyramid of energy 能量金字塔radiation adaptation 趋异适应random 随机分布rare species 偶见种reaction time lag 反应滞时reactive species 反映性物种realized natality 实际出生率realized nick 实际生态龛regressive succession 逆行演替relative frequency 相对频度relative reproductive value 相对生殖值reproduction effect 生殖成效reproductive cost 繁殖成本reproductive pattern 生殖格局reproductive time lag 生殖滞时reproductive value 生殖值residual reproductive value 剩余繁殖价值resilient stability 恢复稳定性resistant stability 抵抗稳定性Restoration ecology 恢复生态学richness 丰度r-k continuum of strategies r-k略连续系统r-strategists r-策略者saprovores 食腐者savanna woodland 热带稀树草原林地scavenger 食腐者sciophytes 阳性植物scramble competition 利用竞争scramble type of competition 分摊型竞争secondary metabolites 次生代谢物质secondary production 次级生产力secondary succession 次生演替secondary 次级演替sedimentary cycle 沉积循环selective fertilization 选择受精self-destructive 自我破坏semelparity 一次繁殖生物semelparous 一次生殖sex ratio 性比sexual dimorphism 雌雄二形现象sexual reproduction 有性生殖sexual selection 性选择shade plants 耐阴性植物Shannon-Weiner index 香农—威纳指数sieve selection hypothesis 筛选说similarity 相似度Simpson’s diversity index 辛普森多样性指数social group 社群social hierarchy 社会等级social-economic-natural complex ecosystem 社会--经济--自然复合系统solar emergy 太阳能值solar emjoules 太阳能焦耳solar transformity 太阳能值转换率spatial pattern 空间格局special heterogeneity 空间异质性speciation 物种形成species area curve 物种面积曲线species diversity 物种多样性species evenness or equitability 种的均匀度species heterogeneity 种的不齐性species turnover rate 种的周转率spore reproduction 孢子生殖stability-resilience 稳定恢复力stabilizing selection 稳定选择standing crop 现存量static life table 静态生命表stenoecious 窄栖性的stenohaline 窄盐性的stenohydric 窄水性的stenophagic 窄食性的stenothermal 窄温性的steppe and semideserty 干草原和半荒漠stochastic model 随机模型stratification 成层现象subassociation 亚群丛组subdominant 亚优势种subformation 亚群系succession 演替succulent 肉质植物succulent 肉质植物summed dominance ratio SDR综合优势比summer-green deciduous forest 夏绿落叶林survivorship curve 存活曲线sympatric speciation 同域性物种Synecology 群落生态学synusia 层片Terrestrial ecology 陆地生态学territorial behavior 领域行为territoriality 领域性the –2/3 thinning law –2/3自疏法则the effect of neighbours 邻接效应therophytes Th 一年生植物thertnoperiodism 温周期现象thorn forest and scrub 多刺森林和密灌丛time-specific life table 特定时间生命表torpor 蛰伏total neutrality hypothesis 中性说trophic level 营养级trophic relationship 营养的联系trophic relationship 营养联系tundra and cold forest 苔原和冷森林uniform 均匀分布unitary organism 单体生物univoltine insects 一化性的昆虫upper horizon 覆盖层Urban ecology 城市生态学vegetation subtype 植被亚型vegetation type group 植被型组vegetation type 植被型vegetative propagation 营养生殖volume 体积water cycle 水循环weight 重量xerarch succession 旱生演替xerophytes 旱生植物zero net growth isoline ZNGI零增长线zone of emergent vegetation 挺水植物带zooplankton 浮游动物El Nino 厄尔尼诺feedback 反馈reflex 反射generalist 泛化种defennce behavior 防卫行为flower visitor 访花昆虫non-hierarchical 非等级的non-spatial model 非空间模型non-homeostatic organism 非内稳态生物nonequilibrium metapopulation 非平衡态复合种群nonequilibrium habitat-tracking metapopulation 非平衡态跟踪生境复合种群nonequilibrium declining metapopulation 非平衡态下降复合种群non—niche 非生态位physical environment 非生物环境nonlinear 非线性关系dispersion 分布decomposer 分解者branching process 分支过程molecular taxonomy 分子分类学the neutral theory of molecular evolution 分子进化的中性理论molecular ecology 分子生态学molecular systematics 分子系统学plankton 浮游动物negative feedback 负反馈carrying capacity 负荷量negative interaction 负相互作用negative selection 负选择epifauna 附底动物metapopulation 复合种群eutrohication 富营养化现象relamation 改良coverage 盖度cover ratio 盖度比disturbance 干扰disturbance patch 干扰斑块disturbance corridor 干扰廊道interference 干扰作用height 高度Coarse's hypothesis 高斯假说Coarse's theory 高斯理论phanerophytes 高位芽植物Grenville Orogenesis 格林威尔造山运动individual 个体individualistic concept 个体论概念renewal 更新functional niche 功能生态位aggressive behavior 攻击行为modules 构件modular organism 构件生物keystone species 关键种association coefficients 关联系数light saturation point 光饱和点light compensation point 光补偿点photoperiod 光周期filter 过滤器Hardy-Weinberg principle 哈德-温伯格原理Ocean ecosytem 海洋生态系统Cambrian period 寒武纪siccocolous 旱生植物river corridor 河流廊道contancy 恒有度mangrove 红树林respiration 呼吸量mutualism 互利synomone 互利素synomonal 互利作用allelopathy 化感作用chemical defence 化学防御chemical ecology 化学生态学allelochemicals 化学物质chemocryptic 化学隐藏divisive 划分的environment 环境environmental ethics 环境伦理学environmental carryin capacity 环境容纳量environmental resource patch 环境资源斑块environmental resource corridor 环境资源廊道desert 荒漠desertification 荒漠化desert ecosystem 荒漠生态系统eitiolation phenomenon 黄化现象restoration ecology 恢复生态学chaos 混沌学mixed type 混合型exchange pool 活动库acquired behavior 获得性行为organismic school 机体论学派Fundamental niche 基础生态位Ecology is the scientific study of interactions among organisms and their environment, such as the interactions organisms have with each other and with their abiotic environment. Topics of interest to ecologists include thediversity, distribution, amount biomass, number population of organisms, as well as competition between them within and amongecosystems. Ecosystems are composed of dynamically interacting parts including organisms, the communities they make up, and the non-living components of their environment. Ecosystem processes, such as primary production, pedogenesis, nutrient cycling, and various niche construction activities, regulate the flux of energy and matter through an environment. These processes are sustained by organisms with specific life history traits, and the variety of organisms is calledbiodiversity. Biodiversity, which refers to the varieties of species,genes, and ecosystems, enhances certain ecosystem services.生态是生物和环境之间的相互作用,如生物相互之间以及与他们的非生物环境的相互作用的科学的研究;生态学家感兴趣的话题包括的thediversity,分布量生物量,数量人口的生物,以及他们之间的竞争和amongecosystems内;包括生物体的动态相互作用的部分组成的生态系统,他们的社区做起来,他们的环境和非生物成分;生态过程,如初级生产,成土作用,养分循环,各种小生建设活动的环境,通过调节能量和物质通量;这些过程持续生物体特定的生活史,各种生物calledbiodiversity;生物多样性是指物种,基因和生态系统的品种,提高一定的生态系统服务;Ecology is an interdisciplinary field that includes biology and Earth science. The word "ecology" "Ökologie" was coined in 1866 by the German scientist Ernst Haeckel 1834–1919. Ancient Greek philosophers such as Hippocrates and Aristotle laid the foundations of ecology in their studies on natural history. Modern ecology transformed into a more rigorous science in the late 19th century. Evolutionary concepts on adaptation and natural selection became cornerstones of modernecological theory. Ecology is not synonymous with environment,environmentalism, natural history, or environmental science. It is closely related to evolutionary biology, genetics, and ethology. An understanding of how biodiversity affects ecological function is an important focus area in ecological studies. Ecologists seek to explain:生态学是一个跨学科领域,包括生物学和地球科学; “生态学”的“Ökologie”,是由德国科学家恩斯特·海克尔1834-1919于1866年创造的;如古希腊哲学家希波克拉底和亚里士多德在他们的研究奠定了基础,生态,自然历史;现代生态转化为更严格的科学在19世纪后期;适应和自然选择的进化概念成为了基石modernecological理论;生态环境,环保,自然历史,或环境科学的代名词;它是密切相关,进化生物学,遗传学和行为学;理解生物多样性如何影响生态功能是生态研究的一个重点领域;生态学家寻求解释:Life processes, interactions and adaptationsThe movement of materials and energy through living communitiesThe successional development of ecosystems, andThe abundance and distribution of organisms and biodiversity in the context of the environment.生命过程,相互作用和适应通过物质和能量的运动生活社区生态系统的演替发展,上下文环境中的生物和生物多样性的丰度和分布;Ecology is a human science as well. There are many practical applications of ecology in conservation biology, wetland management,natural resource management agroecology, agriculture, forestry,agroforestry, fisheries, city planning urban ecology, community health, economics, basic and applied science, and human social interaction human ecology. Organisms and resources compose ecosystems which, in turn, maintain biophysical feedback mechanisms that moderate processes acting on living biotic and nonliving abiotic components of the planet. Ecosystems sustain life-supporting functions and produce natural capital like biomass production food, fuel, fiber and medicine, the regulationof climate, global biogeochemical cycles, water filtration, soil formation, erosion control, flood protection and many other natural features of scientific, historical, economic, or intrinsic value.生态是人类科学;有很多实际应用中的生态保护生物学,湿地管理,自然资源管理农业生态学,农业,林业,农林业,渔业,城市规划城市生态,社区卫生,经济,基础科学和应用科学,人类社会的互动人类生态学;生物和资源组成的生态系统,反过来,保持生物物理反馈机制,适度的过程,作用于生活生物和无生命的星球生物的组件;生态系统维持生命支持功能和生产生物质生产食品,燃料,纤维和医药,调节气候等自然资本,全球生物地球化学循环,过滤水,土壤的形成,控制水土流失,防洪和许多其他科学的自然特征,历史,经济,或内在价值;Hierarchical ecologyThe scale of ecological dynamics can operate like a closed system, such as aphids migrating on a single tree, while at the same time remain open with regard to broader scale influences, such as atmosphere or climate. Hence, ecologists classify ecosystems hierarchically by analyzing data collected from finer scale units, such as vegetation associations, climate, and soil types, and integrate this information to identify emergent patterns of uniform organization and processes that operate on local to regional, landscape, and chronological scales.生态动力学的规模可以象一个封闭的系统,如在一个单一的树进行迁移的蚜虫操作,而在同一时间保持打开状态,关于更广泛的范围的影响,如大气或气候;因此,生态学家生态系统分类较细规模的单位,如植被协会,气候和土壤类型,通过分析收集的数据分层,整合此信息来确定突发模式统一组织和流程上操作的地方到区域,景观,实足鳞片;To structure the study of ecology into a conceptually manageable framework, the biological world is organized into a nested hierarchy, ranging in scale from genes, to cells, to tissues, to organs, to organisms, to species, topopulations, to communities, to ecosystems, to biomes, and up to the level of the biosphere.6 This framework forms a panarchy7 and exhibits non-linearbehaviors; this means that "effect and cause are disproportionate, so that small changes to critical variables, such as the number of nitrogen fixers, can lead to disproportionate, perhaps irreversible, changes in the system properties.组织生态学研究的一个概念管理的框架,组织成一个嵌套层次的生物世界,规模不等,从基因,细胞,组织,器官,生物体,以品种,群体,社区,生态系统,生物群落,最多对生物圈的水平;6该框架形成一panarchy7和展品的非线性行为,这意味着“效应而引起不成比例,从而使小的变化的关键变量,如固氮细菌的数量,可导致不相称的,或许是不可逆转的,在系统属性的变化;BiodiversityBiodiversity an abbreviation of "biological diversity" describes the diversity of life from genes to ecosystems and spans every level of biological organization. The term has several interpretations, and there are many ways to index, measure, characterize, and represent its complex organization.101112 Biodiversity includes species diversity, ecosystem diversity, and genetic diversity and scientists are interested in the way that this diversity affects the complex ecological processes operating at and among these respective levels.111314 Biodiversity plays an important role inecosystem services which by definition maintain and improve human quality of life.151612 Preventing species extinctions is one way to preserve biodiversity and that goal rests on techniques that preserve genetic diversity, habitat and the ability for species to migrate.citation needed Conservation priorities and management techniques require different approaches and considerations to address the full ecological scope of biodiversity.Natural capital that supports populations is critical for maintaining ecosystem services1718 and speciesmigration e.g., riverine fish runs and avian insect control has been implicated as one mechanism by which those service losses are experienced.19 An understanding of biodiversity has practical applications for species and ecosystem-level conservation planners as they make management recommendations to consulting firms, governments, and industry从基因到生态系统的生物多样性“生物多样性”的缩写描述生命的多样性,跨越各个层次的生物组织;这个词有几种解释,指数,衡量的方法有很多,检定和代表其复杂的组织;10 1112生物多样性包括物种多样性,生态系统多样性,遗传多样性和科学家感兴趣的是这种多样性影响的复杂的生态过程,在各自水平和经营方式;111314生物多样性生态系统服务的定义保持和提高人类的生活质量起着重要的作用;1516 12防止物种灭绝,是保护生物多样性的方式之一,目标在于技术,保持遗传多样性,栖息地和物种迁移的能力;引证需要优先保护和管理技术需要不同的方法和注意事项,以解决全生态生物多样性的范围;支持人口的自然资本是至关重要的维持生态系统服务17 18和物种迁移例如,运行河流鱼类和昆虫控制禽流感已牵连作为一个机制,这些服务亏损经历;19理解生物多样性,物种和生态系统一级保护规划具有实际应用,因为他们提出管理建议,咨询公司,政府和行业•。
Collective dynamics of 'small-world' networks
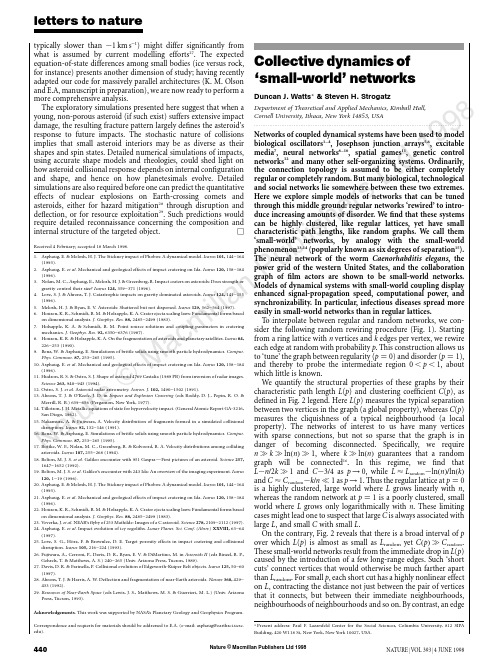
Nature © Macmillan Publishers Ltd 19988typically slower than ϳ1km s −1)might differ significantly from what is assumed by current modelling efforts 27.The expected equation-of-state differences among small bodies (ice versus rock,for instance)presents another dimension of study;having recently adapted our code for massively parallel architectures (K.M.Olson and E.A,manuscript in preparation),we are now ready to perform a more comprehensive analysis.The exploratory simulations presented here suggest that when a young,non-porous asteroid (if such exist)suffers extensive impact damage,the resulting fracture pattern largely defines the asteroid’s response to future impacts.The stochastic nature of collisions implies that small asteroid interiors may be as diverse as their shapes and spin states.Detailed numerical simulations of impacts,using accurate shape models and rheologies,could shed light on how asteroid collisional response depends on internal configuration and shape,and hence on how planetesimals evolve.Detailed simulations are also required before one can predict the quantitative effects of nuclear explosions on Earth-crossing comets and asteroids,either for hazard mitigation 28through disruption and deflection,or for resource exploitation 29.Such predictions would require detailed reconnaissance concerning the composition andinternal structure of the targeted object.ⅪReceived 4February;accepted 18March 1998.1.Asphaug,E.&Melosh,H.J.The Stickney impact of Phobos:A dynamical model.Icarus 101,144–164(1993).2.Asphaug,E.et al .Mechanical and geological effects of impact cratering on Ida.Icarus 120,158–184(1996).3.Nolan,M.C.,Asphaug,E.,Melosh,H.J.&Greenberg,R.Impact craters on asteroids:Does strength orgravity control their size?Icarus 124,359–371(1996).4.Love,S.J.&Ahrens,T.J.Catastrophic impacts on gravity dominated asteroids.Icarus 124,141–155(1996).5.Melosh,H.J.&Ryan,E.V.Asteroids:Shattered but not dispersed.Icarus 129,562–564(1997).6.Housen,K.R.,Schmidt,R.M.&Holsapple,K.A.Crater ejecta scaling laws:Fundamental forms basedon dimensional analysis.J.Geophys.Res.88,2485–2499(1983).7.Holsapple,K.A.&Schmidt,R.M.Point source solutions and coupling parameters in crateringmechanics.J.Geophys.Res.92,6350–6376(1987).8.Housen,K.R.&Holsapple,K.A.On the fragmentation of asteroids and planetary satellites.Icarus 84,226–253(1990).9.Benz,W.&Asphaug,E.Simulations of brittle solids using smooth particle put.mun.87,253–265(1995).10.Asphaug,E.et al .Mechanical and geological effects of impact cratering on Ida.Icarus 120,158–184(1996).11.Hudson,R.S.&Ostro,S.J.Shape of asteroid 4769Castalia (1989PB)from inversion of radar images.Science 263,940–943(1994).12.Ostro,S.J.et al .Asteroid radar astrometry.Astron.J.102,1490–1502(1991).13.Ahrens,T.J.&O’Keefe,J.D.in Impact and Explosion Cratering (eds Roddy,D.J.,Pepin,R.O.&Merrill,R.B.)639–656(Pergamon,New York,1977).14.Tillotson,J.H.Metallic equations of state for hypervelocity impact.(General Atomic Report GA-3216,San Diego,1962).15.Nakamura,A.&Fujiwara,A.Velocity distribution of fragments formed in a simulated collisionaldisruption.Icarus 92,132–146(1991).16.Benz,W.&Asphaug,E.Simulations of brittle solids using smooth particle put.mun.87,253–265(1995).17.Bottke,W.F.,Nolan,M.C.,Greenberg,R.&Kolvoord,R.A.Velocity distributions among collidingasteroids.Icarus 107,255–268(1994).18.Belton,M.J.S.et al .Galileo encounter with 951Gaspra—First pictures of an asteroid.Science 257,1647–1652(1992).19.Belton,M.J.S.et al .Galileo’s encounter with 243Ida:An overview of the imaging experiment.Icarus120,1–19(1996).20.Asphaug,E.&Melosh,H.J.The Stickney impact of Phobos:A dynamical model.Icarus 101,144–164(1993).21.Asphaug,E.et al .Mechanical and geological effects of impact cratering on Ida.Icarus 120,158–184(1996).22.Housen,K.R.,Schmidt,R.M.&Holsapple,K.A.Crater ejecta scaling laws:Fundamental forms basedon dimensional analysis.J.Geophys.Res.88,2485–2499(1983).23.Veverka,J.et al .NEAR’s flyby of 253Mathilde:Images of a C asteroid.Science 278,2109–2112(1997).24.Asphaug,E.et al .Impact evolution of icy regoliths.Lunar Planet.Sci.Conf.(Abstr.)XXVIII,63–64(1997).25.Love,S.G.,Ho¨rz,F.&Brownlee,D.E.Target porosity effects in impact cratering and collisional disruption.Icarus 105,216–224(1993).26.Fujiwara,A.,Cerroni,P .,Davis,D.R.,Ryan,E.V.&DiMartino,M.in Asteroids II (eds Binzel,R.P .,Gehrels,T.&Matthews,A.S.)240–265(Univ.Arizona Press,Tucson,1989).27.Davis,D.R.&Farinella,P.Collisional evolution of Edgeworth-Kuiper Belt objects.Icarus 125,50–60(1997).28.Ahrens,T.J.&Harris,A.W.Deflection and fragmentation of near-Earth asteroids.Nature 360,429–433(1992).29.Resources of Near-Earth Space (eds Lewis,J.S.,Matthews,M.S.&Guerrieri,M.L.)(Univ.ArizonaPress,Tucson,1993).Acknowledgements.This work was supported by NASA’s Planetary Geology and Geophysics Program.Correspondence and requests for materials should be addressed to E.A.(e-mail:asphaug@).letters to nature440NATURE |VOL 393|4JUNE 1998Collective dynamics of ‘small-world’networksDuncan J.Watts *&Steven H.StrogatzDepartment of Theoretical and Applied Mechanics,Kimball Hall,Cornell University,Ithaca,New York 14853,USA.........................................................................................................................Networks of coupled dynamical systems have been used to model biological oscillators 1–4,Josephson junction arrays 5,6,excitable media 7,neural networks 8–10,spatial games 11,genetic control networks 12and many other self-organizing systems.Ordinarily,the connection topology is assumed to be either completely regular or completely random.But many biological,technological and social networks lie somewhere between these two extremes.Here we explore simple models of networks that can be tuned through this middle ground:regular networks ‘rewired’to intro-duce increasing amounts of disorder.We find that these systems can be highly clustered,like regular lattices,yet have small characteristic path lengths,like random graphs.We call them ‘small-world’networks,by analogy with the small-world phenomenon 13,14(popularly known as six degrees of separation 15).The neural network of the worm Caenorhabditis elegans ,the power grid of the western United States,and the collaboration graph of film actors are shown to be small-world networks.Models of dynamical systems with small-world coupling display enhanced signal-propagation speed,computational power,and synchronizability.In particular,infectious diseases spread more easily in small-world networks than in regular lattices.To interpolate between regular and random networks,we con-sider the following random rewiring procedure (Fig.1).Starting from a ring lattice with n vertices and k edges per vertex,we rewire each edge at random with probability p .This construction allows us to ‘tune’the graph between regularity (p ¼0)and disorder (p ¼1),and thereby to probe the intermediate region 0Ͻp Ͻ1,about which little is known.We quantify the structural properties of these graphs by their characteristic path length L (p )and clustering coefficient C (p ),as defined in Fig.2legend.Here L (p )measures the typical separation between two vertices in the graph (a global property),whereas C (p )measures the cliquishness of a typical neighbourhood (a local property).The networks of interest to us have many vertices with sparse connections,but not so sparse that the graph is in danger of becoming disconnected.Specifically,we require n q k q ln ðn Þq 1,where k q ln ðn Þguarantees that a random graph will be connected 16.In this regime,we find that L ϳn =2k q 1and C ϳ3=4as p →0,while L ϷL random ϳln ðn Þ=ln ðk Þand C ϷC random ϳk =n p 1as p →1.Thus the regular lattice at p ¼0is a highly clustered,large world where L grows linearly with n ,whereas the random network at p ¼1is a poorly clustered,small world where L grows only logarithmically with n .These limiting cases might lead one to suspect that large C is always associated with large L ,and small C with small L .On the contrary,Fig.2reveals that there is a broad interval of p over which L (p )is almost as small as L random yet C ðp Þq C random .These small-world networks result from the immediate drop in L (p )caused by the introduction of a few long-range edges.Such ‘short cuts’connect vertices that would otherwise be much farther apart than L random .For small p ,each short cut has a highly nonlinear effect on L ,contracting the distance not just between the pair of vertices that it connects,but between their immediate neighbourhoods,neighbourhoods of neighbourhoods and so on.By contrast,an edge*Present address:Paul zarsfeld Center for the Social Sciences,Columbia University,812SIPA Building,420W118St,New York,New York 10027,USA.Nature © Macmillan Publishers Ltd 19988letters to natureNATURE |VOL 393|4JUNE 1998441removed from a clustered neighbourhood to make a short cut has,at most,a linear effect on C ;hence C (p )remains practically unchanged for small p even though L (p )drops rapidly.The important implica-tion here is that at the local level (as reflected by C (p )),the transition to a small world is almost undetectable.To check the robustness of these results,we have tested many different types of initial regular graphs,as well as different algorithms for random rewiring,and all give qualitatively similar results.The only requirement is that the rewired edges must typically connect vertices that would otherwise be much farther apart than L random .The idealized construction above reveals the key role of short cuts.It suggests that the small-world phenomenon might be common in sparse networks with many vertices,as even a tiny fraction of short cuts would suffice.To test this idea,we have computed L and C for the collaboration graph of actors in feature films (generated from data available at ),the electrical power grid of the western United States,and the neural network of the nematode worm C.elegans 17.All three graphs are of scientific interest.The graph of film actors is a surrogate for a social network 18,with the advantage of being much more easily specified.It is also akin to the graph of mathematical collaborations centred,traditionally,on P.Erdo¨s (partial data available at /ϳgrossman/erdoshp.html).The graph of the power grid is relevant to the efficiency and robustness of power networks 19.And C.elegans is the sole example of a completely mapped neural network.Table 1shows that all three graphs are small-world networks.These examples were not hand-picked;they were chosen because of their inherent interest and because complete wiring diagrams were available.Thus the small-world phenomenon is not merely a curiosity of social networks 13,14nor an artefact of an idealizedmodel—it is probably generic for many large,sparse networks found in nature.We now investigate the functional significance of small-world connectivity for dynamical systems.Our test case is a deliberately simplified model for the spread of an infectious disease.The population structure is modelled by the family of graphs described in Fig.1.At time t ¼0,a single infective individual is introduced into an otherwise healthy population.Infective individuals are removed permanently (by immunity or death)after a period of sickness that lasts one unit of dimensionless time.During this time,each infective individual can infect each of its healthy neighbours with probability r .On subsequent time steps,the disease spreads along the edges of the graph until it either infects the entire population,or it dies out,having infected some fraction of the population in theprocess.p = 0p = 1Regular Small-worldRandomFigure 1Random rewiring procedure for interpolating between a regular ring lattice and a random network,without altering the number of vertices or edges in the graph.We start with a ring of n vertices,each connected to its k nearest neighbours by undirected edges.(For clarity,n ¼20and k ¼4in the schematic examples shown here,but much larger n and k are used in the rest of this Letter.)We choose a vertex and the edge that connects it to its nearest neighbour in a clockwise sense.With probability p ,we reconnect this edge to a vertex chosen uniformly at random over the entire ring,with duplicate edges forbidden;other-wise we leave the edge in place.We repeat this process by moving clockwise around the ring,considering each vertex in turn until one lap is completed.Next,we consider the edges that connect vertices to their second-nearest neighbours clockwise.As before,we randomly rewire each of these edges with probability p ,and continue this process,circulating around the ring and proceeding outward to more distant neighbours after each lap,until each edge in the original lattice has been considered once.(As there are nk /2edges in the entire graph,the rewiring process stops after k /2laps.)Three realizations of this process are shown,for different values of p .For p ¼0,the original ring is unchanged;as p increases,the graph becomes increasingly disordered until for p ¼1,all edges are rewired randomly.One of our main results is that for intermediate values of p ,the graph is a small-world network:highly clustered like a regular graph,yet with small characteristic path length,like a random graph.(See Fig.2.)T able 1Empirical examples of small-world networksL actual L random C actual C random.............................................................................................................................................................................Film actors 3.65 2.990.790.00027Power grid 18.712.40.0800.005C.elegans 2.65 2.250.280.05.............................................................................................................................................................................Characteristic path length L and clustering coefficient C for three real networks,compared to random graphs with the same number of vertices (n )and average number of edges per vertex (k ).(Actors:n ¼225;226,k ¼61.Power grid:n ¼4;941,k ¼2:67.C.elegans :n ¼282,k ¼14.)The graphs are defined as follows.Two actors are joined by an edge if they have acted in a film together.We restrict attention to the giant connected component 16of this graph,which includes ϳ90%of all actors listed in the Internet Movie Database (available at ),as of April 1997.For the power grid,vertices represent generators,transformers and substations,and edges represent high-voltage transmission lines between them.For C.elegans ,an edge joins two neurons if they are connected by either a synapse or a gap junction.We treat all edges as undirected and unweighted,and all vertices as identical,recognizing that these are crude approximations.All three networks show the small-world phenomenon:L ՌL random but C q C random.00.20.40.60.810.00010.0010.010.11pFigure 2Characteristic path length L (p )and clustering coefficient C (p )for the family of randomly rewired graphs described in Fig.1.Here L is defined as the number of edges in the shortest path between two vertices,averaged over all pairs of vertices.The clustering coefficient C (p )is defined as follows.Suppose that a vertex v has k v neighbours;then at most k v ðk v Ϫ1Þ=2edges can exist between them (this occurs when every neighbour of v is connected to every other neighbour of v ).Let C v denote the fraction of these allowable edges that actually exist.Define C as the average of C v over all v .For friendship networks,these statistics have intuitive meanings:L is the average number of friendships in the shortest chain connecting two people;C v reflects the extent to which friends of v are also friends of each other;and thus C measures the cliquishness of a typical friendship circle.The data shown in the figure are averages over 20random realizations of the rewiring process described in Fig.1,and have been normalized by the values L (0),C (0)for a regular lattice.All the graphs have n ¼1;000vertices and an average degree of k ¼10edges per vertex.We note that a logarithmic horizontal scale has been used to resolve the rapid drop in L (p ),corresponding to the onset of the small-world phenomenon.During this drop,C (p )remains almost constant at its value for the regular lattice,indicating that the transition to a small world is almost undetectable at the local level.Nature © Macmillan Publishers Ltd 19988letters to nature442NATURE |VOL 393|4JUNE 1998Two results emerge.First,the critical infectiousness r half ,at which the disease infects half the population,decreases rapidly for small p (Fig.3a).Second,for a disease that is sufficiently infectious to infect the entire population regardless of its structure,the time T (p )required for global infection resembles the L (p )curve (Fig.3b).Thus,infectious diseases are predicted to spread much more easily and quickly in a small world;the alarming and less obvious point is how few short cuts are needed to make the world small.Our model differs in some significant ways from other network models of disease spreading 20–24.All the models indicate that net-work structure influences the speed and extent of disease transmis-sion,but our model illuminates the dynamics as an explicit function of structure (Fig.3),rather than for a few particular topologies,such as random graphs,stars and chains 20–23.In the work closest to ours,Kretschmar and Morris 24have shown that increases in the number of concurrent partnerships can significantly accelerate the propaga-tion of a sexually-transmitted disease that spreads along the edges of a graph.All their graphs are disconnected because they fix the average number of partners per person at k ¼1.An increase in the number of concurrent partnerships causes faster spreading by increasing the number of vertices in the graph’s largest connected component.In contrast,all our graphs are connected;hence the predicted changes in the spreading dynamics are due to more subtle structural features than changes in connectedness.Moreover,changes in the number of concurrent partners are obvious to an individual,whereas transitions leading to a smaller world are not.We have also examined the effect of small-world connectivity on three other dynamical systems.In each case,the elements were coupled according to the family of graphs described in Fig.1.(1)For cellular automata charged with the computational task of density classification 25,we find that a simple ‘majority-rule’running on a small-world graph can outperform all known human and genetic algorithm-generated rules running on a ring lattice.(2)For the iterated,multi-player ‘Prisoner’s dilemma’11played on a graph,we find that as the fraction of short cuts increases,cooperation is less likely to emerge in a population of players using a generalized ‘tit-for-tat’26strategy.The likelihood of cooperative strategies evolving out of an initial cooperative/non-cooperative mix also decreases with increasing p .(3)Small-world networks of coupled phase oscillators synchronize almost as readily as in the mean-field model 2,despite having orders of magnitude fewer edges.This result may be relevant to the observed synchronization of widely separated neurons in the visual cortex 27if,as seems plausible,the brain has a small-world architecture.We hope that our work will stimulate further studies of small-world networks.Their distinctive combination of high clustering with short characteristic path length cannot be captured by traditional approximations such as those based on regular lattices or random graphs.Although small-world architecture has not received much attention,we suggest that it will probably turn out to be widespread in biological,social and man-made systems,oftenwith important dynamical consequences.ⅪReceived 27November 1997;accepted 6April 1998.1.Winfree,A.T.The Geometry of Biological Time (Springer,New Y ork,1980).2.Kuramoto,Y.Chemical Oscillations,Waves,and Turbulence (Springer,Berlin,1984).3.Strogatz,S.H.&Stewart,I.Coupled oscillators and biological synchronization.Sci.Am.269(6),102–109(1993).4.Bressloff,P .C.,Coombes,S.&De Souza,B.Dynamics of a ring of pulse-coupled oscillators:a group theoretic approach.Phys.Rev.Lett.79,2791–2794(1997).5.Braiman,Y.,Lindner,J.F.&Ditto,W.L.Taming spatiotemporal chaos with disorder.Nature 378,465–467(1995).6.Wiesenfeld,K.New results on frequency-locking dynamics of disordered Josephson arrays.Physica B 222,315–319(1996).7.Gerhardt,M.,Schuster,H.&Tyson,J.J.A cellular automaton model of excitable media including curvature and dispersion.Science 247,1563–1566(1990).8.Collins,J.J.,Chow,C.C.&Imhoff,T.T.Stochastic resonance without tuning.Nature 376,236–238(1995).9.Hopfield,J.J.&Herz,A.V.M.Rapid local synchronization of action potentials:Toward computation with coupled integrate-and-fire neurons.Proc.Natl A 92,6655–6662(1995).10.Abbott,L.F.&van Vreeswijk,C.Asynchronous states in neural networks of pulse-coupled oscillators.Phys.Rev.E 48(2),1483–1490(1993).11.Nowak,M.A.&May,R.M.Evolutionary games and spatial chaos.Nature 359,826–829(1992).12.Kauffman,S.A.Metabolic stability and epigenesis in randomly constructed genetic nets.J.Theor.Biol.22,437–467(1969).gram,S.The small world problem.Psychol.Today 2,60–67(1967).14.Kochen,M.(ed.)The Small World (Ablex,Norwood,NJ,1989).15.Guare,J.Six Degrees of Separation:A Play (Vintage Books,New Y ork,1990).16.Bollaba´s,B.Random Graphs (Academic,London,1985).17.Achacoso,T.B.&Yamamoto,W.S.AY’s Neuroanatomy of C.elegans for Computation (CRC Press,BocaRaton,FL,1992).18.Wasserman,S.&Faust,K.Social Network Analysis:Methods and Applications (Cambridge Univ.Press,1994).19.Phadke,A.G.&Thorp,puter Relaying for Power Systems (Wiley,New Y ork,1988).20.Sattenspiel,L.&Simon,C.P .The spread and persistence of infectious diseases in structured populations.Math.Biosci.90,341–366(1988).21.Longini,I.M.Jr A mathematical model for predicting the geographic spread of new infectious agents.Math.Biosci.90,367–383(1988).22.Hess,G.Disease in metapopulation models:implications for conservation.Ecology 77,1617–1632(1996).23.Blythe,S.P .,Castillo-Chavez,C.&Palmer,J.S.T oward a unified theory of sexual mixing and pair formation.Math.Biosci.107,379–405(1991).24.Kretschmar,M.&Morris,M.Measures of concurrency in networks and the spread of infectious disease.Math.Biosci.133,165–195(1996).25.Das,R.,Mitchell,M.&Crutchfield,J.P .in Parallel Problem Solving from Nature (eds Davido,Y.,Schwefel,H.-P.&Ma¨nner,R.)344–353(Lecture Notes in Computer Science 866,Springer,Berlin,1994).26.Axelrod,R.The Evolution of Cooperation (Basic Books,New Y ork,1984).27.Gray,C.M.,Ko¨nig,P .,Engel,A.K.&Singer,W.Oscillatory responses in cat visual cortex exhibit inter-columnar synchronization which reflects global stimulus properties.Nature 338,334–337(1989).Acknowledgements.We thank B.Tjaden for providing the film actor data,and J.Thorp and K.Bae for the Western States Power Grid data.This work was supported by the US National Science Foundation (Division of Mathematical Sciences).Correspondence and requests for materials should be addressed to D.J.W.(e-mail:djw24@).0.150.20.250.30.350.00010.0010.010.11rhalfpaFigure 3Simulation results for a simple model of disease spreading.The community structure is given by one realization of the family of randomly rewired graphs used in Fig.1.a ,Critical infectiousness r half ,at which the disease infects half the population,decreases with p .b ,The time T (p )required for a maximally infectious disease (r ¼1)to spread throughout the entire population has essen-tially the same functional form as the characteristic path length L (p ).Even if only a few per cent of the edges in the original lattice are randomly rewired,the time to global infection is nearly as short as for a random graph.0.20.40.60.810.00010.0010.010.11pb。
增强会展业发展的内生动力
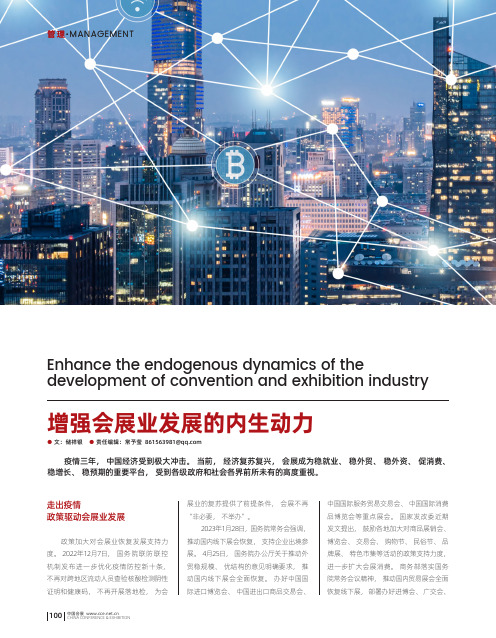
管理增强会展业发展的内生动力● 文:储祥银 ●责任编辑:常予莹****************疫情三年,中国经济受到极大冲击。
当前,经济复苏复兴,会展成为稳就业、稳外贸、稳外资、促消费、稳增长、稳预期的重要平台,受到各级政府和社会各界前所未有的高度重视。
Enhance the endogenous dynamics of thedevelopment of convention and exhibition industry走出疫情政策驱动会展业发展政策加大对会展业恢复发展支持力度。
2022年12月7日,国务院联防联控机制发布进一步优化疫情防控新十条,不再对跨地区流动人员查验核酸检测阴性证明和健康码,不再开展落地检,为会展业的复苏提供了前提条件,会展不再“非必要,不举办”。
2023年1月28日,国务院常务会强调,推动国内线下展会恢复,支持企业出境参展。
4月25日,国务院办公厅关于推动外贸稳规模、优结构的意见明确要求,推动国内线下展会全面恢复。
办好中国国际进口博览会、中国进出口商品交易会、中国国际服务贸易交易会、中国国际消费品博览会等重点展会。
国家发改委近期发文提出,鼓励各地加大对商品展销会、博览会、交易会、购物节、民俗节、品牌展、特色市集等活动的政策支持力度,进一步扩大会展消费。
商务部落实国务院常务会议精神,推动国内贸易展会全面恢复线下展,部署办好进博会、广交会、·MANAGEMENT中国国际高新技术成果交易会等一系列重点展会;全力支持外贸企业参加境外各类专业展,创造更多的贸易机会。
各地政府纷纷出台政策,加大对会展业恢复发展支持力度。
北京政府工作报告提出“抓紧推进新国展二期、第四使馆区等重大项目建设,增强雁栖湖国际会都、国家会议中心二期服务功能保障”;“高水平办好中国服务贸易博览会、中关村论坛、金融界论坛”。
上海市为服务办好中国国际进口博览会专门出台保障条例;《上海市提振信心扩需求稳增长促发展行动方案》提出,支持会展行业恢复重振,对2023年在上海举办的对外经济技术展览会给予补贴;支持举办中国国际工业博览会、中国品牌博览会、上海国际汽车工业展览会和上海碳中和技术、产品与成果博览会等重大会展活动;近期,又推出了《上海市推动会展经济高质量发展三年行动方案》。
系统动力学(名词解释)

名词解释:System Dynamics:A powerful methodology for framing, understanding, and discussing complex policy issues and problems,is a methodology for studying and managing complex feedback systems, such as one finds in business and other social systems.Mental models:are the images (attitudes and assumptions) we carry in our minds about ourselves, other people, institutions, and every aspect of the world which guide our interpretations and behavior.System:The behavior of a system depends not only on the behavior of the components of a system but also on how these components interact.System dynamics:is the application of systems theory to the behavior of complex systems. complex systems:are systems in which there are multiple interactions between many different components (or agents).dynamic hypothesis:Once the problem has been identified and characterized over an appropriate time, modeler must develop a theory called “dynamic hypothesis”that accounts for the problematic behavior.Chaos:When the present determines the future, but the approximate present does not approximately determine the futurelimit cycles:If an oscillatory system with a locally unstable equilibrium is given a slight nudge off its equilibrium point, its swings grow larger and larger until they are constrained by various nonlinearities. Such oscillations are known as limit cycles.dynamic complexity:The complexity generated by the interactive-feedback causalitydirected graph:It is a graph, or set of nodes connected by edges, where the edges have a direction associated with them.Cause and effect :is a simple idea in which actions and decisions have consequences, Feedback: is the process in which an action taken by a person or thing will eventually affect that person or thing.Feedback loop: is a closed sequence of causes and effects简答:1.The problem of system dynamics has the following four characteristics:Complexity: On one hand many factors are included in the system; on the other hand these factors in the given system are connected with each other.Dynamics: Dynamic characteristics reflect the developing and changing laws of nature.Nonlinearity. Nonlinear characteristics are important relationships among many factors in system dynamics.Time lag: It describes a situation where the system or a certain part of the system only change after a period of time under the input.2.Steps of the modeling processStep 1 Problem Articulation (Boundary Selection):Theme selection, Key variables, Time horizon, Dynamic problem definition (reference modes).Step 2 Formulation of Dynamic Hypothesis:Initial hypothesis generation, Endogenous focus,Mapping.Step 3 Formulation of a Simulation Model:Specification, Estimation, Tests.Step 4 Testing:Comparison to reference modes, Robustness under extreme conditions, Sensitivity. Step 5 Policy Design and Evaluation:Scenario specification, Policy design, “What if. . .”analysis, Sensitivity analysis, Interactions of policies.3.Characteristics of Complex SystemsTightly Coupled (“Everything influences everything else”,“You can’t just do one thing”) Dynamic (Change occurs at many time scales)Policy Resistant (Many obvious solutions to problems fail or actually worsen the situation.) Counterintuitive (Cause and effect are distant in time and space)Exhibit Tradeoffs (Long term behavior is often different from short term behavior)4.Why are models useful?1)Creating a model forces one to better understand the real system. Models can help explain observations and help guide the development of future experiments.2)Models can be extremely useful in explaining how a system works to yourself and to others.3)Experiments can be performed to help understand the response of a system to changes without harming the real system.4)A model is the only method that one can use to estimate the future behavior of a system to past, present, and future processes that may influence a system.5)Models can be extremely helpful in policy development.5.4 types of cause contribute explain a phenomenon1) Material cause: the substance that made it so2)Efficient cause: the direct efforts that made it so3)Formal cause: the plan or agent that made it so4)Final cause: the ultimate purpose for being so。
Dynamics
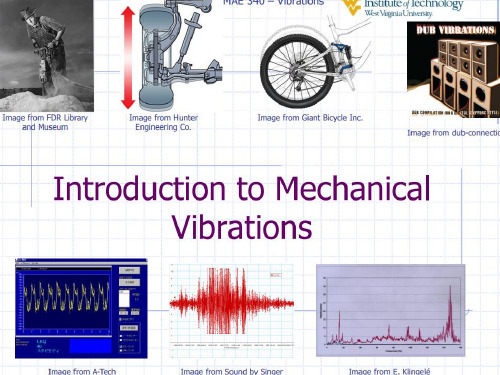
Henry Allen Gleason (1882-1975)
Key terms associated with his continuum concept: individualistic reductionist random contingent non-equilibrial disorganized
Succession
• As taught in most high school and lower level biology classes:
– Two types: primary and secondary – Sequence or stages from pioneer to mature
Outline: Early concepts of vegetation dynamics
1. Organismal views (Clements) 2. Individualistic views (Gleason, Whittaker, Watt, Egler) 3. Ecosystem ecology and resurgence of organismal views (Odum) 4. Next sets of slides will cover more recent ideas in disturbance ecology and in biocomplexity
John Muir
• Founder of the Sierra Club, one of the first environmental organizations • Saw nature as a cathedral, holy and timeless, without change, a reflection of religious handiwork, God was telos
- 1、下载文档前请自行甄别文档内容的完整性,平台不提供额外的编辑、内容补充、找答案等附加服务。
- 2、"仅部分预览"的文档,不可在线预览部分如存在完整性等问题,可反馈申请退款(可完整预览的文档不适用该条件!)。
- 3、如文档侵犯您的权益,请联系客服反馈,我们会尽快为您处理(人工客服工作时间:9:00-18:30)。
SUSTAINABLE DEVELOPMENT SeriesEditor: Carlo CarraroEndogenous Network DynamicsBy Frank H. Page Jr, Department of Economics, Indiana University and Centre d’Economie de la Sorbonne, Université Paris 1Myrna H. Wooders, Department of Economics, Vanderbilt University and Department of Economics, University of WarwickSummaryIn all social and economic interactions, individuals or coalitions choose not only with whom to interact but how to interact, and over time both the structure (the “with whom”) and the strategy (“the how”) of interactions change. Our objectives here are to model the structure and strategy of interactions prevailing at any point in time as a directed network and to address the following open question in the theory of social and economic network formation: given the rules of network and coalition formation, the preferences of individuals over networks, the strategic behavior of coalitions in forming networks, and the trembles of nature, what network and coalitional dynamics are likely to emerge and persist. Our main contributions are (i) to formulate the problem of network and coalition formation as a dynamic, stochastic game, (ii) to show that this game possesses a stationary correlated equilibrium (in network and coalition formation strategies), (iii) to show that, together with the trembles of nature, this stationary correlated equilibrium determines an equilibrium Markov process of network and coalition formation, and (iv) to show that this endogenous process possesses a finite, nonempty set of ergodic measures, and generates a finite, disjoint collection of nonempty subsets of networks and coalitions, each constituting a basin of attraction. We also extend to the setting of endogenous Markov dynamics the notions of pairwise stability (J ackson-Wolinsky, 1996), strong stability (J acksonvan den Nouweland, 2005), and Nash stability (Bala-Goyal, 2000), and we show that in order for any network-coalition pair to persist and be stable (pairwise, strong, or Nash) it is necessary and sufficient that the pair reside in one of finitely many basins of attraction. The results we obtain here for endogenous network dynamics and stochastic basins of attraction are the dynamic analogs of our earlier results on endogenous network formation and strategic basins of attraction in static, abstract games of network formation (Page and Wooders, 2008), and build on the seminal contributions of Jackson and Watts (2002), Konishi and Ray (2003), and Dutta, Ghosal, and Ray (2005).Keywords: Endogenous Network Dynamics, Dynamic Stochastic Games of Network Formation, Equilibrium Markov Process of Network Formation, Basins of Attraction, Harris Decomposition, Ergodic Probability Measures, Dynamic Path Dominance Core, Dynamic Pairwise StabilityJEL Classification: A14, C71, C72This paper was begun while Page andWooders were visiting CES-CERMSEM at the University of Paris 1 in June 2006. The authors thank CES-CERMSEM and Paris 1 for their hospitality. The authors thank Matt Jackson, J.-J. Herings, Luca Merlino, and the participants in the 2009 CTN Workshop in Maastricht for many insightful comments. END_84m.tex. URLs: /~fpage/, . This paper has been presented at the 14th Coalition Theory Network Workshop held in Maastricht, The Netherlands, on 23-24 January 2009 and organised by the Maastricht University CTN group (Department of Economics, http://www.feem-web.it/ctn/12d_maa.php).Address for correspondence:Frank H. PageDepartment of EconomicsIndiana UniversityBloomington, IN 47405E-mail: fpage@The opinions expressed in this paper do not necessarily reflect the position ofFondazione Eni Enrico MatteiCorso Magenta, 63, 20123 Milano (I), web site: www.feem.it, e-mail: working.papers@feem.itEndogenous Network DynamicsFrank H.Page,Jr.1 Department of Economics Indiana University Bloomington,IN47405fpage@Myrna H.Wooders2 Department of Economics Vanderbilt UniversityNashville,TN37235 m.wooders@June,2006Current Version,May200931Also,Centre d’Economie de la Sorbonne,Universite Paris1,Pantheon-Sorbonne.2Also,Department of Economics,University of Warwick,Coventry CV47AL,UK.3This paper was begun while Page and Wooders were visiting CES-CERMSEM at the Uni-versity of Paris1in June2006.The authors thank CES-CERMSEM and Paris1for their hos-pitality.The authors thank Matt Jackson,J.-J.Herings,Luca Merlino,and the participants in the2009CTN Workshop in Maastricht for many insightful comments.END_84m.tex. URLs:/~fpage/,.AbstractIn all social and economic interactions,individuals or coalitions choose not only with whom to interact but how to interact,and over time both the structure(the“with whom”)and the strategy(“the how”)of interactions change.Our objectives here are to model the structure and strategy of interactions prevailing at any point in time as a directed network and to address the following open question in the theory of social and economic network formation:given the rules of network and coalition formation, the preferences of individuals over networks,the strategic behavior of coalitions in forming networks,and the trembles of nature,what network and coalitional dynamics are likely to emergence and persist.Our main contributions are(i)to formulate the problem of network and coalition formation as a dynamic,stochastic game,(ii) to show that this game possesses a stationary correlated equilibrium(in network and coalition formation strategies),(iii)to show that,together with the trembles of nature,this stationary correlated equilibrium determines an equilibrium Markov process of network and coalition formation,and(iv)to show that this endogenous process possesses afinite,nonempty set of ergodic measures,and generates afinite, disjoint collection of nonempty subsets of networks and coalitions,each constituting a basin of attraction.We also extend to the setting of endogenous Markov dynamics the notions of pairwise stability(Jackson-Wolinsky,1996),strong stability(Jackson-van den Nouweland,2005),and Nash stability(Bala-Goyal,2000),and we show that in order for any network-coalition pair to persist and be stable(pairwise,strong, or Nash)it is necessary and sufficient that the pair reside in one offinitely many basins of attraction.The results we obtain here for endogenous network dynamics and stochastic basins of attraction are the dynamic analogs of our earlier results on endogenous network formation and strategic basins of attraction in static,abstract games of network formation(Page and Wooders,2008),and build on the seminal contributions of Jackson and Watts(2002),Konishi and Ray(2003),and Dutta, Ghosal,and Ray(2005).KEYWORDS:endogenous network dynamics,dynamic stochastic games of net-work formation,equilibrium Markov process of network formation,basins of attrac-tion,Harris decomposition,ergodic probability measures,dynamic path dominance core,dynamic pairwise stability.JEL Classifications:A14,C71,C721Introduction1.1OverviewIn all social and economic interactions,individuals or coalitions choose not only with whom to interact but how to interact,and over time both the structure(the“with whom”)and the strategy(“the how”)of interactions change.Our objectives here are to model the structure and strategy of interactions prevailing at any point in time as a directed network and to address the following open question in the theory of social and economic network formation:given the rules of network formation,the preferences of individuals over networks,the strategic behavior of coalitions in forming networks, and the trembles of nature,what network and coalitional dynamics are likely to emergence and persist.Thus,we propose to study the emergence of endogenous network and coalitional dynamics from strategic behavior and the randomness in nature.Our main contributions are(i)to formulate the problem of network formation as a dynamic,stochastic game,(ii)to show that this game possesses an equilibrium in stationary correlated network and coalition formation strategies,(iii)to show that, together with the trembles of nature,these equilibrium strategies determine an equi-librium Markov process of network and coalition formation which respects the rules of network formation and the preferences of individuals,and(iv)to show that,although uncountably many networks may form,this equilibrium Markov process generates a finite,disjoint collection of nonempty subsets of networks and coalitions,each consti-tuting a basin of attraction,and possesses afinite,nonempty set of ergodic measures.In our prior work on static abstract games of network formation(Page and Wood-ers,2007,denoted PW07),we have shown that,given the rules of network formation and the preferences of individuals,these games possess strategic basins of attraction and these contain all networks that are likely to emerge and persist as the game un-folds.Moreover,we have shown that when any one of these strategic basins contains only one network,then the game possesses a network(i.e.,the single network con-tained in the singleton basin)that is stable against all coalitional network deviation strategies-and thus the game has a nonempty path dominance core.Finally,we have shown in PW07that depending on how we specialize the rules of network formation and the dominance relation over networks,any network contained in the path domi-nance core is pairwise stable(Jackson-Wolinsky,1996),strongly stable(Jackson-van den Nouweland,2005),Nash(Bala-Goyal,200),or consistent(Chwe,1994).We show here that there are many parallels between the static abstract game formulation and our prior results for static games and the results we obtain here for our Markov dynamic game formulation.This is suggested already by the seminal paper by Jackson and Watts(2002)on the evolution of networks.Jackson and Watts present a basic theory(and to our knowledge thefirst theory)of stochastic dynamic network formation over afinite set of linking networks governed by a Markov chain generated by the myopic strategic behavior of players(following the Jackson-Wolinsky rules of network formation)and the trembles of nature.Their model builds on the earlier,nonstochastic model of dynamic network formation due to Watts(2001)-as1far as we know,thefirst models of network dynamics(see also Skyrms and Pemantle (2000)).By considering a sequence of perturbed irreducible and aperiodic Markov chains(i.e.,each with a unique invariant measure)converging to the original Markov chain,they show that any pairwise stable network is necessarily contained in the support of an invariant measure-that is,in the support of a probability measure that places all its support on sets of networks likely to form in the long run.We show here that similar conclusions can be reached for directed networks with many arc types governed by arbitrary network formation rules.In a general Markov game setting,with farsighted players,what precisely does it mean for a network to be pairwise stable-or stable in any sense?For example, if the state space of networks is large,then the endogenous Markov process of net-work formation is likely to have many invariant measures-and in fact many ergodic probability measures(i.e.,measures that place all their probability mass on a single absorbing set).Which absorbing set contains networks stable in the sense of pairwise stability,or strong stability,or Nash stability?These are some of the questions we answer here in our study of endogenous network dynamics.We conjecture that in any reasonable dynamic,stochastic model of network forma-tion the endogenously determined Markov process of network and coalition formation will possess ergodic probability measures and generate basins of attraction.We show here that in fact the endogenous Markov process possesses onlyfinitely many ergodic measures and basins of attraction.This endogenousfiniteness property of equilib-rium has serious implications for empirical work on networks.In particular,since nature does not afford the empirical observer multiple observations across states but rather only multiple observations across time,the fact that onlyfinitely many long run equilibrium sets are possible and more importantly,the fact that on these sets (i.e.,on these basins of attraction)state averages are equal to time averages gives meaning and significance to time series observations which seek to infer the long run equilibrium network.Moreover,to the extent that networks can truly represent vari-ous social and economic interactions,our understanding of how and why the network formation process moves toward or away from any particular basin can potentially shed new light on the persistence or transience of many social and economic con-ditions.For example,how and why does a particular path of entrepreneurial and scientific interactions carry an economy beyond a tipping point and onto a path of economic growth driven by a particular industry-and why might it fail to do so? How and why does a particular path of product line-nonlinear pricing schedule con-figurations lead a strategically competitive industry to become more concentrated-or fade?These are some of the applied questions which hopefully can be addressed using a model of endogenous network dynamics1.2Endogenous Network DynamicsOur approach to endogenous dynamics is motivated by the observation that the sto-chastic process governing network and coalition formation through time is determined not only by nature’s randomness(or nature’s trembles)through time-as envisioned in random graph theoretic approaches-but also by the strategic behavior of individu-2als and coalitions through time in attempting to influence the networks and coalitions that emerge under the prevailing rules of network formation and the trembles of na-ture.Thus,here we will develop a theory of endogenous network and coalitional dynamics that brings together elements of random graph theory and game theory in a dynamic stochastic game model of network and coalition formation.While dy-namic stochastic games have been used elsewhere in economics(see,for example, Amir(1991,1996),Amir and Lambson(2003),and Chakrabarti(1999,2008),Duffie, Geanakoplos,Mas-Colell,and McLennan(1994),Mertens and Parthasarathy(1987, 1991),Nowak(2003,2007)),their application to the analysis of the evolution of social and economic networks is relatively new.Our plan of analysis has two parts.In part(1)we will construct our dynamic game model of network and coalition formation,and then show that this game has an equi-librium in stationary correlated stationary strategies.Our model has six primitives consisting of the following:(i)a feasible set of directed networks representing all pos-sible configurations of social or economic interactions,(ii)a feasible set of coalitions allowed to form under the rules of network formation for the purpose of proposing alternative networks,(iii)a state space consisting of feasible network-coalition pairs, (iv)a set of players and player constraint correspondences specifying for each player and in each state the set of feasible alternative networks and coalitions that a player can propose under the rules of network formation as a member of the current or sta-tus quo coalition-and as a nonmember,(v)a set of player discount rates and payofffunctions defined on the graph of players’product constraint correspondence,and(vi) a stochastic law of motion.This stochastic law of motion represents nature and spec-ifies the probability with which each possible new status quo network-coalition(i.e., new state)might emerge as a function of the status quo network-coalition pair(i.e., the current state)and the profile of player-proposed new status quo network-coalition pairs(i.e.,the current action profile).Using these primitives,we will construct a dis-counted stochastic game model of network formation,and then show that this game possesses a stationary correlated equilibrium in network-coalition proposal strategies.Finally,in part(1)we will show that,together with the stochastic law of motion, these stationary correlated equilibrium strategies determine an equilibrium Markov process of network and coalition formation.More importantly,we will be able to con-clude via classical results due to Blackwell(1965)(also,Himmelberg,Parthasarathy, and vanVleck(1976)),Nowak and Raghavan(1992),and Duffie,Geanakoplos,Mas-Colell,and McLennan(1994))that these stationary correlated equilibrium strategies are optimal against player defections to any other history-dependent network-coalition proposal strategies-thus showing that our decision to focus on stationary correlated strategies is well-founded.In part(2),we will analyze the stability properties the endogenous Markov process of network and coalition formation.In particular,using methods of stability analysis essentially due to Nummelin(1984)and Meyn and Tweedie(1993)-and based on the profound work of Doeblin(1937,1940)-we will show that the equilibrium Markov process of network and coalition formation possesses ergodic probability measures and generates basins of attraction.We will then study in some detail the number3and structure of these basins of attraction as well as the structure of set of invariant probability measures.More importantly,we will show that the equilibrium process possesses onlyfinitely many ergodic measures and basins of attraction.Finally,in part(2),we will extend the definitions of pairwise stable,strongly stable,Nash,and consistent networks to the dynamic Markov setting of the model and show that these various types of stable networks can persist only in the basins of attraction generated under the appropriate specification of the rules of network formation and feasible coalitions.1.3Related LiteratureTo our knowledge,thefirst paper to study endogenous dynamics in a related model is the paper by Konishi and Ray(2003)on dynamic coalition formation.The primitives of their model consist of(i)afinite set of outcomes(possibly afinite set of networks), (ii)a set of coalitional constraint correspondences specifying for each coalition and each status quo outcome,the set of new outcomes a coalition might bring about if allowed to do so,and(iii)a discount rate and set of player payofffunctions defined on the set of all outcomes.Konishi and Ray show that their model possesses an equi-librium process of coalition formation,that is,a stochastic law of motion governing movement from one outcome to another such that(a)if a move from one outcome to another takes place with positive probability,then for some coalition this move makes sense in that no coalition member is made worse offby the move and no further move makes all coalition members better off,and(b)if for a given outcome there is another outcome making all members of some coalition better offand no further outcome makes this coalition even better off,then a move to another outcome takes place with probability1(i.e.,the probability of standing still at the given outcome is zero).The notion of a player being better offis reckoned in terms of a player’s valuation function implied by the maximization of the expected discounted stream of payoffs with respect to the stochastic law of motion.Stated loosely,then,Konishi and Ray show that for their model there is a law of motion which generates coalitionally Pareto improving moves from one outcome to another(i.e.,in our case it would be from one network to another).Our model differs from the model of Konishi and Ray in several respects.First,in our model movements from one network(outcome)to another are largely determined by the strategic behavior of individuals within feasible coalitions.In Konishi and Ray,coalitions are passive and strategic behavior plays no part in determining the movement from one outcome to another.They simply show that there model is consistent with there being a law of motion which moves the outcome along in a coalitionally Pareto improving way.In this sense-i.e.,in the sense that movement is nonstrategic-their model is more closely related to random graph theoretic models of network dynamics.In our model,equilibrium strategic behavior,together with natures trembles,are central to determining equilibrium network dynamics.Second,whereas Konishi and Ray,for technical reasons,restrict attention to a finite set of outcomes(in our model,afinite set of networks),we allow for uncount-ably many networks-this to allow for consideration of networks with a large number4of nodes or networks with uncountably many arc types.This generalization is more than a technical nicety.In order to capture the myriad and potentially complex nature of interactions between players(say for example in a stock market or in a contracting game with multiple principals and multiple agents)we must allow there to be uncountably many possible types of interactions.In our model the set of po-tential interactions are represented by a set of arc types with each arc type(or arc label)representing a particular type of interaction(or connection)between nodes in a directed network.Thus,because we allow for uncountably many arc types in de-scribing the possiblyfinite number of interactions between nodes,in our model there are uncountably many possible networks(or outcomes in the language of Konishi and Ray).Moreover,in order to model large networks(i.e.,networks with many nodes),in our model we can allow there to be infinitely many nodes-although here we focus exclusively on thefinite nodes case.Third,while Konishi and Ray restrict attention at the outset to Markov laws of motion,we will show that our strategi-cally determined equilibrium Markov process of network and coalition formation is robust against all possible alternative dynamics induced by history-dependent types of strategic behavior.Thus,at least for the class of Konishi-Ray types of models,we will show that Markov laws of motion are stable and robust with respect to other forms of history-dependent laws of motion.1Finally,whereas Konishi and Ray focus on the existence of an equilibrium process of coalition formation,here we will not only establish the existence of a strategically determined equilibrium process of network and coalition formation,but also we will show that this process possesses a nonempty set of ergodic measures and generates basins of attraction.Dutta,Ghosal,and Ray(2005)extend the Konishi-Ray type model to consider a particular form of strategic behavior(i.e.,strategic behavior governed by a particular set of network formation rules)in a dynamic game of network formation over afinite set of undirected linking networks(rather than directed networks).They show that their model has a Nash equilibrium and identify conditions under which efficiency can be sustained in equilibrium-thus,continuing in a dynamic setting the seminal work of Jackson and Wolinsky(1996)and Dutta and Mutuswami(1997)on equilibrium and efficiency.Here our focus is on equilibrium and stability rather than equilibrium and efficiency and our analysis is carried out in a dynamic,stochastic game model of network and coalition formation,admitting all forms of network formation rules, over an uncountable set of directed networks.While Dutta,Ghosal,and Ray restrict attention to Markov strategies and show that there is an equilibrium in this class of network formation strategies,here,we show that there is an equilibrium in the class of all stationary correlated network and coalition proposal strategies and that this type of equilibrium is optimal relative to the class of all history-dependent net-work formation strategies.Moreover,as mentioned above,we show that in general, the resulting equilibrium Markov network and coalitional dynamics possess ergodic 1By a Markov law of motion we mean a stochastic law of motion where probabilistic movements from one outcome or network to another depend only on the current outcome rather than on some history of outcomes.5measures and generate network and coalitional basin of attraction.We view the starting point of our research to be the pioneering work of Jackson and Watts(2002)already discussed briefly above.Our model of endogenous net-work and coalitional dynamics extends their work on stochastic network dynamics in several respects.First,in our model players behave farsightedly in attempting to influence the path of network and coalition formation-farsighted in the sense of dynamic programing(e.g.,Dutta,Ghosal,and Ray(2005))2.Moreover,in our model the game is played over a(possibly)uncountable collection of directed networks un-der general rules of network formation which include not only the Jackson-Wolinsky rules,but also other more complex rules.In our model the law of motion is such that the trembles of nature are Markovian rather than i.i.d.as in Jackson and Watt, and are functions of the current state and the current profile of network and coali-tion proposals by players.Extending the notion of pairwise stability to a dynamic setting,one of the benchmarks for our research is to show that in a Markov model of network and coalition formation,if a network is dynamically pairwise stable,then in order to persist,it must be contained in one offinitely many basins of attraction, and therefore,contained in the support of an ergodic probability measure.2See Chwe(1994),Page,Wooders,and Kamat(2005),and Page and Wooders(2005)for notions of farsighted behavior in static,abstract games.62Primitives2.1The Space of Directed NetworksWe begin by giving the formal definition of a directed network.Let N be afinite set of nodes with typical element denoted by i and let A be a compact metric space of arcs with typical element denoted by a.Denote by d A the metric on A and by d N the discrete metric on N.3Arcs represent potential connections between nodes, and depending on the application,nodes can represent economic agents or economic objects such as markets orfirms.Definition1(Directed Networks)Given node set N and arc set A,a directed network,G,is a nonempty,closed subset of A×(N×N).The collection of all directed networks is denoted by P f(A×(N×N)).A directed network G∈P f(A×(N×N))thus consists of a closed set of ordered pairs of the form(a,(i,i ))where a is an arc type or an arc label and(i,i )is an ordered pair of nodes.We shall refer to any pair(a,(i,i ))∈G as a connection in network G.Thus,a network G is a closed set of connections specifying how the nodes in N are connected by the arcs in A.In a directed network order matters.In particular,(a,(i,i ))∈G means that nodes i and i are connected by a type a arc from node i to node i .Note that under our definition of a directed network,loops are allowed-that is, we allow an arc to go from a given node back to that given node.4Finally,note that under our definition an arc can be used multiple times in a given network and multiple arcs can go from one node to another.However,our definition does not allow an arc a to go from a node i to a node i multiple times.The following notation is useful in describing networks.Given directed network G∈P f(A×(N×N)),letG(a):= (i,i )∈N×N:(a,(i,i ))∈G ,G+(i):= a∈A:(a,(i,i ))∈G for some i ∈N ,andG−(i ):= a∈A:(a,(i,i ))∈G for some i∈N .Thus,in network G,3The discrete metric d N is given byd N(i,i )= 1if i=i0otherwise.4By allowing loops we are able to represent a network having no connections between distinct nodes as a network consisting entirely of loops at each node.7G(a)is the set of node pairs connected by arc a,G+(i)is the set of arcs leaving node i,andG−(i )is the set of arcs entering node i .If for some arc a∈A,G(a)is empty,then arc a is not used in network G.Also, if for some node i∈N,G+(i)∪G−(i)is empty,then node i is said to be isolated.Because A×(N×N)is a compact metric space,the set of networks P f(A×(N×N)) equipped with the Hausdorffmetric h is a compact metric space(see Aliprantis and Border(1999),sections3.14-3.16).Formally,the Hausdorffmetric is defined as follows:First,let the distance between connection(a,(i0,i1))∈A×(N×N)and network G∈P f(A×(N×N))be given byd((a,(i0,i1)),G):=infd (a,(i0,i1)),(a ,(i 0,i 1)) ,(a ,(i 0,i 1))∈Gwhered (a,(i0,i1)),(a ,(i 0,i 1)) :=d A(a,a )+d N(i0,i 0)+d N(i1,i 1)is the metric on A×(N×N).Given this distance measure between connections and networks,the Hausdorffmetric h is then defined ash(G,G ):=max sup(a,(i0,i1))∈G d((a,(i0,i1)),G ),sup(a ,(i 0,i 1))∈G d((a ,(i 0,i 1)),G) ,for G and G in P f(A×(N×N)).5Given the nature of the discrete metric on the set of nodes,it is easy to see that if the Hausdorffdistance between networks G and G is less thanε∈(0,1),that is, if networks G and G are withinεdistance forε<1,then the same set of nodes are involved in connections in both networks and the networks differ only in the way these nodes are connected(i.e.,in the types of arcs used in making the connections). Thus if h(G,G )<ε<1,then(a,(i,i ))∈G if and only if(a ,(i,i ))∈Gfor arcs a and a with d A(a,a )<ε.Convergence in the space of directed networks(P f(A×(N×N)),h)can be charac-terized via the notions of limit inferior and limit superior.Let{G n}n be a sequence of directed networks.The limit inferior of this sequence,denoted by Li(G n),is defined as follows:connection(a,(i,i ))∈Li(G n)if and only if there is a sequence of connec-tions{(a n,(i n,i n))}n converging to(a,(i,i ))(i.e.,(a n,(i n,i n))d→(a,(i,i )))where for each n connection(a n,(i n,i n))is contained in network G n.The limit superior, denoted by Ls(G n),is defined as follows:connection(a,(i,i ))∈Ls(G n)if and only if there is a subsequence of connections{(a n k,(i n k,i n k))}k converging to(a,(i,i )) 5It is important to note that because A×(N×N)is compact,all metrics compatible with the product topology on A×(N×N),generate the same Hausdorffmetric topology on P f(A×(N×N)) (see Theorem3.77in Aliprantis and Border(1999)).8。