October 2, 2003 1923 WSPCBook Trim Size for 9in x 6in Recommendation Chapter 5 Recommendati
DECHEN

REV. DE CIÊNCIA & TECNOLOGIA, Piracicaba, v. 11, n. 21, p. 1-73, jan./jun. 2003.R EVISTA DE C IÊNCIA& T ECNOLOGIA • 211COMISSÃO EDITORIALN IVALDO L EMOS C OPPINI – presidente (Engenharia de Produção) K LAUS S CHÜTZER (Engenharia Mecânica)N ELSON C ARVALHO M AESTRELLI (Gestão da Produção)N IVALDI C ALONEGO JÚNIOR (Ciência da Computação)SÔNIA M ARIA M ALMONGE (Engenharia Química)COMITÊ CIENTÍFICOB ERT L AUWERS (Katholieke Universiteit Leuven –Bélgica)C ARLOS A LBERTO G ASPARETTO (Facens/Unicamp –ESTEVAL project partners.– Feature Based Integrated Design Environment.Identification of interdependencies between manufacturing features.Unsuitable finishing quality (Schützer et al., 1999).After considering several possibilities, which could result in this poor surface quality cutting tool geometry , clamping and balancing of the tool system, technological parameters and machining set-ups, it was realized, that the problem came from the CNC of the machine tool, which was incapable of processing the NC program as fast as the feed rate defined in the NC program. So the machine reaches the point refereed in one line of the program and the information to move the tool to the next point was not processed yet, then the machine had to wait a couple of milliseconds to start moving again.This incompatibility between the feed rate defined in the NC program and the processing time of the CNC results in two different situations according to the CNC used:poor surface quality – the CNC tries to move the machine at the programmed feed rate, but it can-Improper surface finishingThe workpiece used and the finishing tool path.The roughing and semi-finishing operations were accomplished using exactly the same technological parameters and cutting strategies for all workpieces.The comparison analysis was done only during the finishing operation and the same technological para-meters, cutting strategies and tools were used in both cases. The finishing operation was distinguished by the methodology of interpolation. The trajectory of the finishing tool path is shown in figure 3. For this operation it was used a 10 mm ball end mill tool at 10000 rpm and the programmed feed rate was 2000 mm/min.YSISThe results of the machining experiments considering the NC program size, the time required to exe-cute the program and the surface quality in terms of roughness and superficial texture were compared and the conclusions are presented below.NC Program SizesThe table 2 presents the finishing programs sizes calculated for both methods. It proves that less infor-mation are required to describe tool paths by the circular/linear method, thus reducing the program size byMETHOD P ROGRAM SIZE N UMBER586 kbCircular/linear83 kb• V. 11, Nº 21 – pp. 29-36Regions where the feed rate as reduced.2000 mm/min660 mm/min 1500 mm/min700 mm/min2000 mm/minRoughness AnalysisIt was used a digital Surftest Equipment to obtain the Ra and Rz parameters. It was analyzed the same areas for all workpieces. Practically, there are not differences between the roughness parameters for both interpolation methods.Surface T exture AnalysisIt was possible to visually verify the differences between the surfaces of both methods. The circular/ linear workpieces are smoother than the linear ones. The figure 5 shows this texture.Irregular surface texture Regular surface textureBesides those transversal marks at the workpiece machined by the linear interpolation, this method also gives a deficient quality in the longitudinal direction, as it is seen in the next photos taken by a CCD camera connected in an microscopy.Figure 6 was taken using a microscopy with magnification 10x of the linear part. The vertical marks are the cusp heights left by the ball end mill tool. The steps-over of the tool path is on the horizontal direc-Fig. 6. Uneven cusps from a linear workpiece.Fig. 7. Even cusps from a circular/linear workpieceIn linear interpolation method is possible to see uneven cusp heights, what can difficult drastically the hand finishing afterwards. This problem is not seen at the workpieces milled by the circular/linear method, as shown in figure 7. It happens due to the more constant cutting movements.In this method, the cusps height are much more uniform, what can help the manual finishing afterwards, by decreasing this process time, and improving the accuracyCONCLUSIONThe High Speed Cutting T echnology can be attractively applied in die and mould manufacturing, among others applications. However, there are several other technologies in the process chain that must be considered to support an efficient HSC process.R EVISTA DE C IÊNCIA & T ECNOLOGIA • V. 11, Nº 21 – pp. 29-36。
国外民粹主义研究前沿 精品
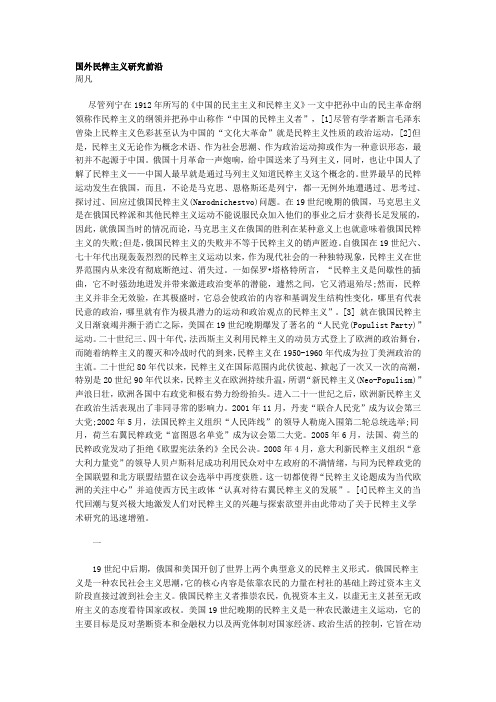
国外民粹主义研究前沿周凡尽管列宁在1912年所写的《中国的民主主义和民粹主义》一文中把孙中山的民主革命纲领称作民粹主义的纲领并把孙中山称作“中国的民粹主义者”,[1]尽管有学者断言毛泽东曾染上民粹主义色彩甚至认为中国的“文化大革命”就是民粹主义性质的政治运动,[2]但是,民粹主义无论作为概念术语、作为社会思潮、作为政治运动抑或作为一种意识形态,最初并不起源于中国。
俄国十月革命一声炮响,给中国送来了马列主义,同时,也让中国人了解了民粹主义——中国人最早就是通过马列主义知道民粹主义这个概念的。
世界最早的民粹运动发生在俄国,而且,不论是马克思、恩格斯还是列宁,都一无例外地遭遇过、思考过、探讨过、回应过俄国民粹主义(Narodnichestvo)问题。
在19世纪晚期的俄国,马克思主义是在俄国民粹派和其他民粹主义运动不能说服民众加入他们的事业之后才获得长足发展的,因此,就俄国当时的情况而论,马克思主义在俄国的胜利在某种意义上也就意味着俄国民粹主义的失败;但是,俄国民粹主义的失败并不等于民粹主义的销声匿迹。
自俄国在19世纪六、七十年代出现轰轰烈烈的民粹主义运动以来,作为现代社会的一种独特现象,民粹主义在世界范围内从来没有彻底断绝过、消失过。
一如保罗•塔格特所言,“民粹主义是间歇性的插曲,它不时强劲地迸发并带来激进政治变革的潜能,遽然之间,它又消退殆尽;然而,民粹主义并非全无效验,在其极盛时,它总会使政治的内容和基调发生结构性变化,哪里有代表民意的政治,哪里就有作为极具潜力的运动和政治观点的民粹主义”。
[3] 就在俄国民粹主义日渐衰竭并濒于消亡之际,美国在19世纪晚期爆发了著名的“人民党(Populist Party)”运动。
二十世纪三、四十年代,法西斯主义利用民粹主义的动员方式登上了欧洲的政治舞台,而随着纳粹主义的覆灭和冷战时代的到来,民粹主义在1950-1960年代成为拉丁美洲政治的主流。
二十世纪80年代以来,民粹主义在国际范围内此伏彼起、掀起了一次又一次的高潮,特别是20世纪90年代以来,民粹主义在欧洲持续升温,所谓“新民粹主义(Neo-Populism)”声浪日壮,欧洲各国中右政党和极右势力纷纷抬头。
2003年美国ISI收录的我国科技期刊
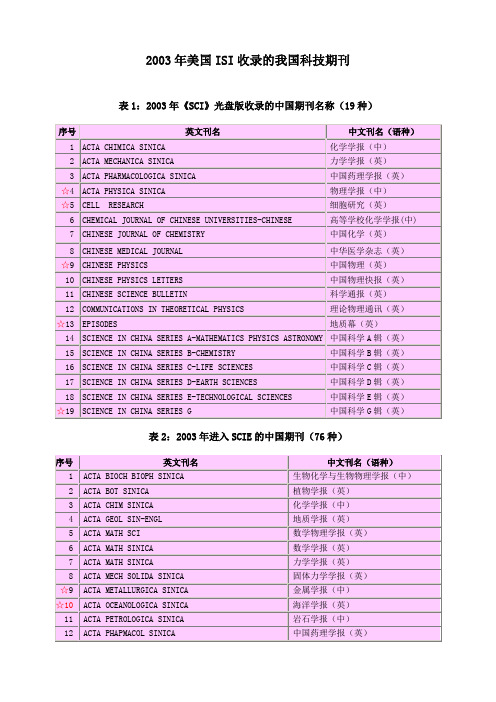
J OF ENVIRONMENTAL SCIENCES
5
半导体学报
6
爆炸与冲击
7
北京航空航天大学学报
8
北京科技大学学报(英文版)
9
北京理工大学学报
10
北京理工大学学报(英文版)
11
北京林业大学学报
12
北京邮电大学学报
13
材料科学技术(英文版)
14
材料科学与工艺
15
材料研究学报
16
船舶力学
17
大连理工大学学报
34
光电工程
35
光电子·激光
36
光学技术
37
光学学报
38
硅酸盐学报
39
国际造纸
40
哈尔滨工业大学学报
41
哈尔滨工业大学学报(英文版)
42
哈尔滨建筑大学学报
43
焊接学报
44
航空材料学报
45
航空学报
46
合成橡胶工业
47
核动力工程
48
红外与毫米波学报
49
化工学报
50
机械工程学报
51
机械工程学报(英文版)
52
21
BIOMED ENVIRON SCI
生物医学与环境科学(英)
22
CELL RESEARCH
细胞研究(英)
23
CHEM J CHINESE U
高等学校化学学报(中)
24
CHEM RES CHINESE U
高等学校化学研究(英)
25
CHINA OCEAN ENG
中国海洋工程(英)
26
CHINESE ANN MATH B
03年的初中英语书
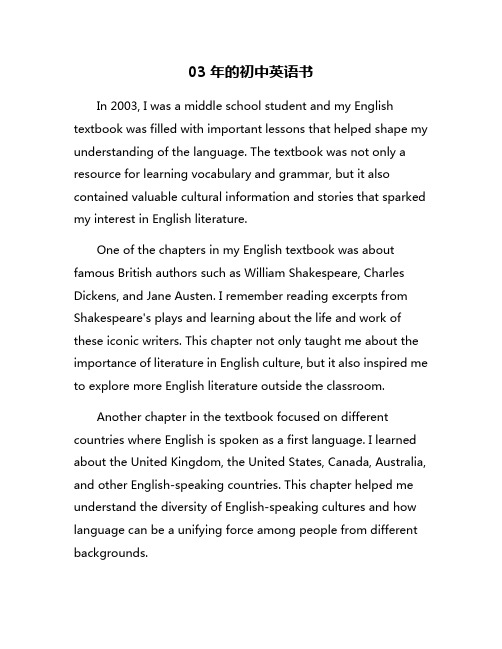
03年的初中英语书In 2003, I was a middle school student and my English textbook was filled with important lessons that helped shape my understanding of the language. The textbook was not only a resource for learning vocabulary and grammar, but it also contained valuable cultural information and stories that sparked my interest in English literature.One of the chapters in my English textbook was about famous British authors such as William Shakespeare, Charles Dickens, and Jane Austen. I remember reading excerpts from Shakespeare's plays and learning about the life and work of these iconic writers. This chapter not only taught me about the importance of literature in English culture, but it also inspired me to explore more English literature outside the classroom.Another chapter in the textbook focused on different countries where English is spoken as a first language. I learned about the United Kingdom, the United States, Canada, Australia, and other English-speaking countries. This chapter helped me understand the diversity of English-speaking cultures and how language can be a unifying force among people from different backgrounds.One of my favorite parts of the textbook was the section on grammar and vocabulary. I learned about verb tenses, adjectives, adverbs, and other important parts of speech. I also studied new vocabulary words and practiced using them in sentences. This section helped me improve my English language skills and become more confident in communicating with others in English.Overall, my experience with the 2003 middle school English textbook was a positive one. It not only taught me the basics of the English language but also introduced me to the richness of English literature and culture. I am grateful for the knowledge and skills I gained from that textbook, as they have laid the foundation for my continued learning and appreciation of the English language.。
历史上的今天 模板
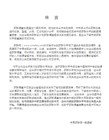
1966年12月15日美国动画家迪斯尼逝世
2008年12月15日两岸直接“三通”实现
0705年12月16日唐朝女皇武则天去世
1770年12月16日德国音乐家贝多芬诞辰
1989年12月16日美国入侵巴拿马
1996年12月16日董建华任香港首任特首
1918年3月9日 协约国开成立伪“满洲国”
1945年3月9日 美军对日本东京进行大轰炸
1876年3月10日 英国发明家贝尔发明电话
1912年3月10日 袁世凯就任民国临时大总统
1933年3月10日 南京政府废两改元统一币制
1954年3月1日 美国试验第一枚氢弹
1955年3月1日 我国发行新版人民币
历史上的今天0302-0308 英中历史组
1919年3月2日第三共产国际在莫斯科成立
1969年3月2日苏联入侵珍宝岛引发中苏边界冲突
0581年3月3日 杨坚代周自立,建立隋朝
1861年3月3日 俄国宣布废除农奴制
1949年2月20日 国民党将故宫文物迁往台湾
1832年2月21日 英国探险队发现了格陵兰岛。
1848年2月21日《共产党宣言》发表
1895年2月21日 孙中山成立兴中会
1972年2月21日 美国总统尼克松正式访华
1799年2月22日 清朝重臣和珅因贪污被赐死
1732年2月22日 美国国父华盛顿出生
1947年12月23日美国发明晶体管
1948年12月23日日本战犯东条英机被处绞刑
2005年12月23日四人帮之一的姚文元去世
1524年12月24日葡萄牙航海家达·伽马去世
年历

|《国家人文历史》|3年|第期|总第期11843年10月英国发行量最大的全国性报纸之一《世界新闻报》,开始发行。
该报以报道名人以及涉及名人丑闻的文章而出名,也因被揭发卷入多宗窃听丑闻而在2011年7月10日停刊。
21803年10月▲美国独立战争时期革命家、政治家塞缪尔亚当斯逝世。
他是激进民主主义的“自由之子”协会创建者之一和领导人,策动了波士顿倾茶事件。
/塞缪尔亚当斯31973年10月中共中央决定撤销军委办事组,成立由军委副主席叶剑英主持的军委办公会议,负责军委日常工作。
同日,成立中央专案组,审查林彪、陈伯达反党集团的问题。
41883年10月第一列东方快车从巴黎东站开出,最终目的地是伊斯坦布尔。
东方快车最初是指通往东方的国际列车,但后来在各种通俗文学中多用以指代激情的异国旅行如阿嘉莎克莉斯蒂的《东方快车谋杀案》。
51903年10月孙中山到达兴中会的发源地檀香山。
他向华侨散发了数千册《革命军》,并连续发表演说,提出只有革命,才能拯救中国。
随后,着手恢复和发展革命组织,取名“中华革命军”。
6公元23年篡汉称帝、建立“新朝”的外戚王莽在绿林军攻入长安时,被刺杀身亡。
新朝开创了中国历史上通过篡位取得政权的先例。
王莽称帝后曾参照《周礼》进行多项改革,史称“王莽改制”。
71913年10月▲亨利福特的汽车制造厂建立了第一条活动装配线,使一台汽车在不到3小时内就制造出来,大大提高了生产效率。
福特汽车81993年10月▲“朦胧诗”代表人物顾城,在新西兰自缢身亡。
顾城“文革”前即开始诗歌创作,曾辞职隐居激流岛,被称为当代仅有的唯灵浪漫主义诗人。
他的“黑夜给了我黑色的眼睛/我却用它寻找光明”成为中国新诗的经典名句。
/顾城91623年10月▲比利时籍官员南怀仁出生。
在中国传教期间,南怀仁受召前往协助神父汤若望,并在康熙年间接替他成为钦天监监副。
他利用所学铸造红夷炮,发明三轮蒸汽伍德罗威尔逊亲自启动大坝闸门,宣布巴拿马运河竣工。
REGULATIONS OF THE HONG KONG SPECIAL ADMINISTRATIVE REGION 2003
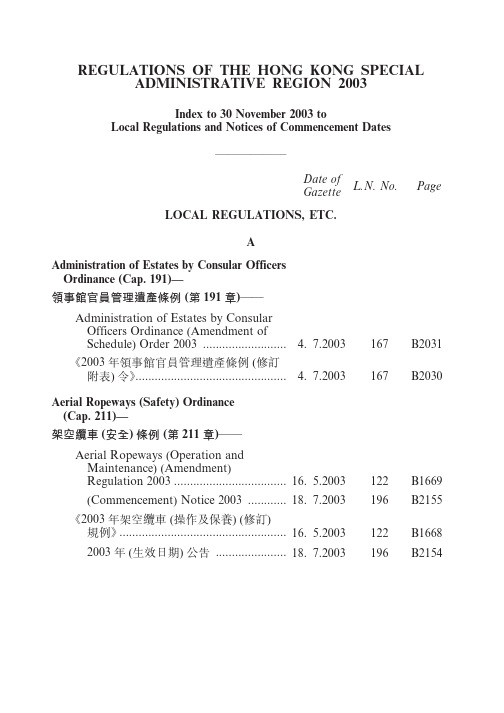
Index to 30 November 2003 to Local Regulations and Notices of Commencement Dates —————— Date of Gazette L.N. No. LOCAL REGULATIONS, ETC. A Administration of Estates by Consular Officers Ordinance (Cap. 191)— ( 191 ) Administration of Estates by Consular Officers Ordinance (Amendment of Schedule) Order 2003 .......................... 4. 7.2003 2003 ) ( ............................................... 4. 7.2003 Page
B2153 B2152
Births and Deaths Registration Ordinance (Cap. 174)— ( 174 ) Births and Deaths Registration Ordinance (Amendment of First Schedule) Order 2003 .......................... 2. 5.2003 2003 ( 1) .. 2. 5.2003
4
INDEX TO LOCAL REGULATIONS, ETC.
Date of Gazette L.N. No. B Banking (Amendment) Ordinance 2002 (6 of 2002)— 2002 6 ) 16 16 ( ) (2002
# 2003. The American Astronomical Society. All rights reserved. Printed in U.S.A. NICMOS CO
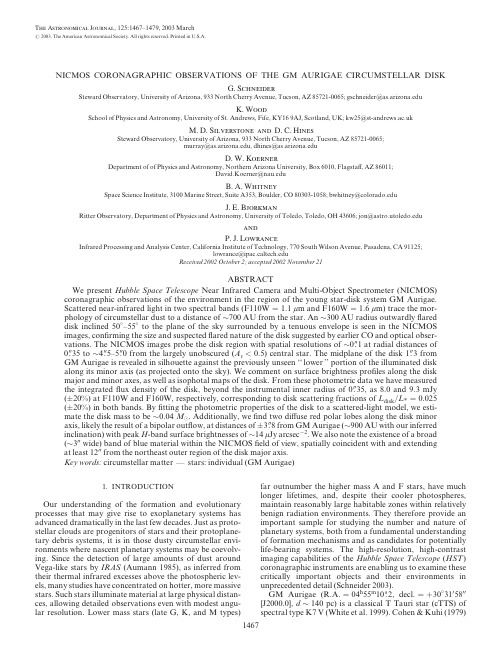
NICMOS CORONAGRAPHIC OBSERVATIONS OF THE GM AURIGAE CIRCUMSTELLAR DISKG.SchneiderSteward Observatory,University of Arizona,933North Cherry Avenue,Tucson,AZ85721-0065;gschneider@K.WoodSchool of Physics and Astronomy,University of St.Andrews,Fife,KY169AJ,Scotland,UK;kw25@M.D.Silverstone and D.C.HinesSteward Observatory,University of Arizona,933North Cherry Avenue,Tucson,AZ85721-0065;murray@,dhines@D.W.KoernerDepartment of of Physics and Astronomy,Northern Arizona University,Box6010,Flagstaff,AZ86011;David.Koerner@B.A.WhitneySpace Science Institute,3100Marine Street,Suite A353,Boulder,CO80303-1058;bwhitney@J.E.BjorkmanRitter Observatory,Department of Physics and Astronomy,University of Toledo,Toledo,OH43606;jon@andP.J.LowranceInfrared Processing and Analysis Center,California Institute of Technology,770South Wilson Avenue,Pasadena,CA91125;lowrance@Received2002October2;accepted2002November21ABSTRACTWe present Hubble Space Telescope Near Infrared Camera and Multi-Object Spectrometer(NICMOS) coronagraphic observations of the environment in the region of the young star-disk system GM Aurigae.Scattered near-infrared light in two spectral bands(F110W=1.1l m and F160W=1.6l m)trace the mor-phology of circumstellar dust to a distance of$700AU from the star.An$300AU radius outwardlyflared disk inclined50 –55 to the plane of the sky surrounded by a tenuous envelope is seen in the NICMOS images,confirming the size and suspectedflared nature of the disk suggested by earlier CO and optical obser-vations.The NICMOS images probe the disk region with spatial resolutions of$0>1at radial distances of0>35to$4>5–5>0from the largely unobscured(A v<0.5)central star.The midplane of the disk1>3fromGM Aurigae is revealed in silhouette against the previously unseen‘‘lower’’portion of the illuminated disk along its minor axis(as projected onto the sky).We comment on surface brightness profiles along the disk major and minor axes,as well as isophotal maps of the disk.From these photometric data we have measured the integratedflux density of the disk,beyond the instrumental inner radius of0>35,as8.0and9.3mJy (Æ20%)at F110W and F160W,respectively,corresponding to disk scattering fractions of L disk/L*=0.025 (Æ20%)in both bands.Byfitting the photometric properties of the disk to a scattered-light model,we esti-mate the disk mass to be$0.04M .Additionally,wefind two diffuse red polar lobes along the disk minor axis,likely the result of a bipolar outflow,at distances ofÆ3>8from GM Aurigae($900AU with our inferred inclination)with peak H-band surface brightnesses of$14l Jy arcsecÀ2.We also note the existence of a broad ($300wide)band of blue material within the NICMOSfield of view,spatially coincident with and extending at least1200from the northeast outer region of the disk major axis.Key words:circumstellar matter—stars:individual(GM Aurigae)1.INTRODUCTIONOur understanding of the formation and evolutionary processes that may give rise to exoplanetary systems has advanced dramatically in the last few decades.Just as proto-stellar clouds are progenitors of stars and their protoplane-tary debris systems,it is in those dusty circumstellar envi-ronments where nascent planetary systems may be coevolv-ing.Since the detection of large amounts of dust around Vega-like stars by IRAS(Aumann1985),as inferred from their thermal infrared excesses above the photospheric lev-els,many studies have concentrated on hotter,more massive stars.Such stars illuminate material at large physical distan-ces,allowing detailed observations even with modest angu-lar resolution.Lower mass stars(late G,K,and M types)far outnumber the higher mass A and F stars,have much longer lifetimes,and,despite their cooler photospheres, maintain reasonably large habitable zones within relatively benign radiation environments.They therefore provide an important sample for studying the number and nature of planetary systems,both from a fundamental understanding of formation mechanisms and as candidates for potentially life-bearing systems.The high-resolution,high-contrast imaging capabilities of the Hubble Space Telescope(HST) coronagraphic instruments are enabling us to examine these critically important objects and their environments in unprecedented detail(Schneider2003).GM Aurigae(R.A.=04h55m1092,decl.=+30 3105800 [J2000.0],d$140pc)is a classical T Tauri star(cTTS)of spectral type K7V(White et al.1999).Cohen&Kuhi(1979)The Astronomical Journal,125:1467–1479,2003March#2003.The American Astronomical Society.All rights reserved.Printed in U.S.A.1467found GM Aurigae’s effective temperature to be3970K, and its age was estimated by Siess,Forestini,&Bertout (1999)as1.3Æ0.3Myr.Duplicity studies(e.g.,Konig, Neuhauser,&Stelzer(2001)indicate GM Aurigae is a single star,and its mass has been determined dynamically by Simon,Dutrey,&Guilloteau(2000)as0.84Æ0.05M . GM Aurigae exhibits strong H (White&Ghez2001),as well as Paschen- and Bracket- emission,as is common for young stars(Folha&Emerson2001).Its near-IR apparent magnitudes as found from the Two Micron All Sky Survey (2MASS)were reported1as J=9.315Æ0.036and H=8.578Æ0.026though,as is typical for cTT stars,GM Aurigae exhibits intrinsic brightness variations of a few tenths of a magnitude in the near-IR(e.g.,Bouvier et al. 1993,1995).The presence of a large amount of dust around GM Auri-gae was established by Beckwith et al.(1990)in a1.3mm continuum survey of86pre–main-sequence(PMS)stars in the Taurus-Aurigae region.Koerner,Sargent,&Beckwith (1993)reported13CO(2!1)and1.4mm continuum emis-sion,which they interpreted as originating from the core of a950Æ530AU disk of gas and dust coincident with the position of GM Aurigae.From their CO observations they found differential rotation in the velocityfield about an axis at PA=50 ,consistent with Keplerian rotation.Dutrey et al.(1998)resolved the dust disk with1.3and2.7mm contin-uum emission by using the IRAM interferometer and con-firmed the kinematic results of Koerner et al.(1993).They inferred a minimum radius for the disk of$200AU(1>4) and constrained the inclination of the rotation axis to 57 Æ5 from the line of sight.The disk was detected but unresolved with a totalflux density of22mJy in a2.7mm BIMA survey conducted by Looney&Mundy(2000), though they suggested a slight extension perpendicular to the large-scale CO structure.Polarized850l m emission(at the3%level)was reported by Tamura et al.(1999)and interpreted as arising from ther-mal reradiation by magnetically aligned dust grains in the disk.Skrutskie et al.(1990)found significant mid-IR emis-sion over the stellar photospheric level,indicative of a reradiating circumstellar dust disk.The relativelyflat mid-IR excess and millimeter emission(Koerner et al.1993)were fitted to a model spectral energy distribution(SED)by Chiang&Goldreich(1997)assuming a passive face-on disk. More recent work by Chiang&Goldreich(1999)suggested a polar inclination of$60 to the line of sight and a visual extinction of GM Aurigae by its circumstellar disk of0.5 mag,larger than the A v of0.14suggested by Cohen&Kuhi (1979)or more recently A v=0.31by Gullbring et al.(1998). Therein Chiang&Goldreich(1999)described GM Aurigae as a‘‘class II source,only slightly obscured by its circum-stellar disk’’which,given its inferred angular extent of a few arcseconds,has made its direct imaging in scattered light observationally challenging.Stapelfeldt et al.(1997)reported imaging the GM Auri-gae disk in the visible with the HST Wide Field and Plane-tary Camera2(WFPC2).Their imaging observations demonstrated the existence of an upwardlyflared disk with a morphology implicating an inclination to the line of sight of$65 ,similar to the inclination deduced by Dutrey et al.(1998).They estimated a large disk-scattering fraction with $8%of the visible light from the GM Aurigae system pro-vided by the circumstellar material.Koerner et al.(1998)confirmed both theflared nature of the disk and the suggested inclination with the HST Near Infrared Camera and Multi-Object Spectrometer (NICMOS),utilizing its coronagraphic imaging mode at1.1 and1.6l m in a preliminary analysis of those data before the coronagraphic imaging mode of NICMOS was fully charac-terized and calibrated.Here,with those instrumental char-acteristics now well understood,we discuss in greater detail these spatially resolved NICMOS imaging observations, which elucidate the morphology and geometrical character-istics of the scattered light disk.We present thefirst imaging of the lower portion of the disk,partially shadowed by opti-cally thick material in the midplane.With these newly revealed features,the GM Aurigae disk appears to have an intrinsic morphology similar to HH30(Cotera et al.2001), Haro6-5B(Padgett et al.1999),and the companion to HK Tau(Stapelfeldt et al.1998).With these images we also present evidence for the existence of a bipolar outflow at an early stage of collimation.Such outflows,though unde-tected in the less sensitive optical GM Aurigae observations of Stapelfeldt et al.(1997),have been observed in the cir-cumstellar environments of similar stars(such as HH30),as well as stars of higher mass and somewhat older ages(e.g., the Herbig Ae/Be stars SU Aurigae;Grady et al.2001)and HD163296(Grady et al.2000).2.OBSERVATIONSObservations of the GM Aurigae circumstellar disk were obtained with the HST NICMOS coronagraph on UT1998 March25.Two spectralfilters were employed:F110W(cen-tral wavelength=1.0998l m,FWHM=0.5915l m; roughly similar to J band)and F160W(central wave-length=1.5940l m,FWHM=0.4000l m;a close analog to H band).The spacecraft was rolled to a single NICMOS camera2field orientation angle(position angle of the image +Y-axis=214=51east of north)for all images.With each filter two STEP128NSAMP=14MULTIACCUM imag-ing sequences(Schultz et al.2001)were executed,yielding total integration times of1279.86s after combining the reduced images in each passband.The NICMOS coronagraphic optics are in camera2, which provides a256Â256pixelfield of view at a scale of $76mas pixelÀ1.A star of F110W and F160W magnitude 20.4and19.4appeared in thefield of view,12>7to the northeast of GM Aurigae,from which the FWHM of the coronagraphic point-spread functions(PSFs)were contem-poraneously determined as0>098and0>132,respectively.21See /2mass/releases/second/doc/ explsup.html.2NICMOS coronagraphy is performed with the reimaged focal plane at the detector moved to a marginally different position than used for direct imaging to maximize coronagraphic contrast.In addition,the HST focus interface to the science instruments,including NICMOS,changes by very small amounts with the spacecraft orbit and attitude,which affects thefine structure of the PSF(Schneider et al.2001).The instantaneous spatial scale of a resolution element may be determined(for a given spectral band)from unocculted target acquisition images,closely spaced in time preceding the coronagraphic images.Here,we have determined this contemporaneously for each passband(expressed as the FWHM of the PSF from an unresolved point source)by using the well-exposed star that appeared serendipitously in thefield of view.1468SCHNEIDER ET AL.Vol.125These images were acquired as part of the NICMOS IDT Environments of Nearby Stars(EONS)program(GTO/ 7233;B.A.Smith,principal investigator)and were thefirst NICMOS coronagraphic science images obtained following the installation of the instrument in HST.The target was placed into the0>3($4pixel)radius coronagraphic‘‘hole’’by an autonomous on-board acquisition process.A posteri-ori,GM Aurigae was found to have been decentered by (D X=0.49,D Y=À0.01)pixels from the low scatter point of the coronagraphic system,the offset resulting from a bug in the target acquisitionflight software that wasfixed soon after this observation.3.DATA CALIBRATION,REDUCTION,AND PROCESSING3.1.Photometric CalibrationCount rate images corrected for the wavelength-dependent instrumentalflat-field response,pixel-dependent dark currents,and detector nonlinearities were created from the raw MULTIACCUM frames by using an IDL-based analog3to the IRAF STSDAS NICMOS CALNICA image reduction software(Stobie et al.1998), and calibration referencefiles,both created by the NICMOS IDT.Known underresponsive pixels and those with excessive dark currents were replaced using two-dimensional Gaussian-weighted interpolation of neigh-boring pixels.In doing so we used weighting radii of3 and5pixels(appropriate for the F110W and F160W res-olution elements,respectively,as sampled with the cam-era2pixels).The two count rate images for eachfilter were averaged and image artifacts not compensated for in the CALNICA processing4were quantitatively charac-terized and removed.These electronically induced arti-facts include zero-level offsets between the four detector quadrants,elevated count rates along a strip orthogonal to the readout direction encompassing deeply exposed pixels,low-amplitude electronic‘‘echoes’’of deeply exposed targets at the same locations in the other detec-tor quadrants,and residual imprints of the detectorflat field from uncompensated pedestal effects.The corrected count rate images were converted to photo-metric units based upon the absolute on-orbit photometric calibration determined by the NICMOS IDT of2.031l JyÀ1 ADUÀ1sÀ1pixelÀ1for F110W and2.190l JyÀ1ADUÀ1sÀ1 pixelÀ1for F160W(M.Rieke2002,private communica-tion).The photometric zero points to convert to in-band magnitudes,ascertained from the same calibrations,yield 1775Jy(F110W)and1083Jy(F160W)for the total energy for a zero-magnitude(Vega system)star.3.2.Reference Point-Spread FunctionsThe NICMOS coronagraph provides gains in image con-trast of factors of a few to about10within a few arcseconds of an occulted star by reducing the intensity of the diffracted energy in the wings of the stellar point-spread function and the‘‘downstream’’instrumental scatter from the masked PSF core(Schneider et al.1998).Further improvements,of up to factors of$50,are obtained by subtracting well-matched,high S/N(signal-to-noise ratio),template PSFs of reference stars.Ideally,a reference coronagraphic PSF to be subtracted from a target image should be observed at the same subpixel location within the coronagraphic hole.Nor-mally,this is achieved to a very high degree of precision by the on-board autonomous target acquisition process.How-ever,this process wasflawed at the time of the GM Aurigae observation.During the HST NICMOS SMOV-2calibration pro-gram we obtained deeply exposed reference PSFs in the F160Wfilter rastered in a subpixel grid in the corona-graph(SMOV/7052;G.Schneider,principal investiga-tor).Fortuitously,in that grid,three MULTIACCUM images of a bright reference star(BD+032964,H=5.0) were positioned at(D X=+0.50,D Y=0.00)pixels with respect to the low scatter point of the coronagraphic sys-tem.To the achievable pointing precision,this is differen-tially identical to the mispointed GM Aurigae observation.Hence,for our F160W observations we cre-ated a reference PSF from these observations of BD +032964for subsequent subtraction from the GM Auri-gae data set.Unfortunately,an equivalent raster was not carried out under the F110Wfilter.The sensitivity to decentering,however,is far less severe at1.1l m than at 1.6l m primarily because of the smaller spatial scale (68%)of the shorter wavelength PSF(Schneider et al. 2001).For our F110W observation we created a hole-centered reference PSF obtained in the same calibration program(from10raw images)with the recognition that the residual pointing misalignment would be the principal source of systematic error limiting the background sub-traction at small radii near GM Aurigae.Reference PSF images were reduced and calibrated from this data set in the same manner as were our target images.3.3.Geometrical Correction,Rectification,and Scale The NICMOS camera2X=Y image plane pixel scales differ by$0.9%.Additionally,the absolute scales varied by small amounts during the early part of the HST Cycle 7mission.5As a result the image scales for the BD +032964reference PSFs and the target(GM Aurigae) images differ and required geometrical rectification and rescaling before the reference PSFs could be subtracted. For the epochs of these observations the NICMOS cam-era2pixel scales were for1997July22(BD+032964) (76.11,75.43)mas pixelÀ1and for1998March25(GM Aurigae)(75.99,75.31)mas pixelÀ1.The F110W and F160W photometrically calibrated images were resampled on a common grid to square up the pixels on the sky(adjusting the Y-scale to match the X-scale) and the target images were resampled to match their refer-ence PSF image scale.Image resampling was done by sinc function apodized bicubic interpolation with the NICMOS IDT IDP3software(Schneider&Stobie2002),so all images were geometrically rectified to have76.11Â76.11mas2 pixels,with the total(area integrated)flux conserved in the resampling process.3IDL is a registered trademark of Research Systems Incorporated,aKodak company.4See /hst/nicmos/performance/anomalies.5See /hst/nicmos/performance/platescale/ rel_platescale.html.No.3,2003GM AURIGAE CIRCUMSTELLAR DISK14693.4.Target/PSF Image Registration,Scaling,and SubtractionAfter correcting for the camera 2geometrical distortions,the F110W and F160W reference PSF images were regis-tered,flux-scaled,and subtracted from the corresponding target images (also using IDP3).PSF subtraction residuals in the difference images are spatially correlated over mult-iple pixel scales in the radial direction along (and immedi-ately adjacent to)the bright diffraction artifacts from the HST secondary mirror supports (Schneider &Silverstone 2002).The reference PSF subtractions were constrained to minimize the energy in the diffraction spike residuals by simultaneously adjusting the reference PSF star brightness and position.Because of small anisotropic secular instabil-ities in the HST plus NICMOS instrumental system,a glob-ally optimized solution over the entire field cannot be obtained and is the dominant source of uncertainty in ascer-taining the correct flux density scaling for the reference PSF (Schneider et al.2001).Here we found the ratio of the GM Aurigae photospheric flux density to that of BD +032964was 0.0380Æ0.0008at F110W and 0.0325Æ0.0006at F160W.BD +032964,with spectral type G2V,has a spec-tral energy distribution under the F110W and F160W filter passbands that is very similar to GM Aurigae.Hence,color-dependent differential PSF structures (arising from the small mismatch in spectral types)are very small and do not significantly contribute to the imperfections in nulling the underlying stellar flux (e.g.,Weinberger et al.1999,2002).Following Schneider et al.(2001)we estimated the uncer-tainty in the flux density of the disk arising from the uncertainty in the flux scaling of the target and referencestars and in the presence of imperfectly nulled artifacts from the diffraction spikes.6We find this uncertainty is d 20%of the total flux density integrated outward from any inner radius beyond 0>4in both spectral bands.4.GM AURIGAE SCATTERED LIGHT DISKThe morphology of the light scattered in the circumstellar region of GM Aurigae displays the characteristic pattern of a flared disk (Whitney &Hartmann 1992)and is shown in a series of four images presented in increasing levels of sensi-tivity in Figure 1a –1d .GM Aurigae itself is occulted in the 0>3radius coronagraphic hole (black circle ).The upper por-tion of an outwardly flared disk,inclined to the line of sight,is readily seen in both the F110W and F160W spectral bands (Fig.1a ).The disk is bilaterally symmetric,brighter ‘‘forward ’’(to the north)of the major axis,and mirrored in its surface brightness distribution about the minor axis.The forward scattering edge of the lower portion of the disk,‘‘below ’’the higher opacity midplane in the region of the minor axis to the north,becomes visible at lower flux den-sities (Fig.1b ,where the central portion of the disk is satu-rated).At lower surface brightness (Fig.1c –1d )a kinked broad ‘‘ribbon ’’of blue material extends to thenortheastFig.1.—NICMOS Camera 2PSF-subtracted coronagraphic images of the GM Aurigae scattered light disk.To accommodate the very large dynamic range over the spatial regions of interest,the paired F110W (top )and F160W (bottom )images are shown in several different linear display stretches:(a )À1to +10l Jy per pixel;(b )À0.5to +2l Jy per pixel;(c )À0.2to +0.5l Jy per pixel;(d )À0.05to +0.1l Jy per pixel.6Unlike other stars in our disk survey,the GM Aurigae images were acquired with only a single orientation of the NICMOS camera 2corona-graphic field.As a result,the regions of the disk coincident with the diffraction spike residuals,after PSF subtraction,cannot be replaced by data obtained at a second field orientation,which would have sampled the affected regions of the disk.1470SCHNEIDER ET AL.Vol.125for a distance of at least1200,declining in brightness in both F110W and F160W with increasing distance from GMAurigae.Finally,diffuse red polar lobes(likely of outflowmaterial)are seen symmetrically along the minor axis at dis-tances of$3>5–400,with peak H-band surface brightnessess$0.14l Jy per camera2pixel.Figure2,a two-color combi-nation of the F110W and F160W images,shows these fea-tures and their‘‘colors,’’in two logarithmic stretches.The morphology of the circumstellar light from the innerportions of the disk(to$100along the minor axis in the for-ward-scattering direction and$1>6along the major axis) traced by earlier highest spatial resolution(F555W)WFPC2 observations of Stapelfeldt et al.(1997)is in general agree-ment with the NICMOS images.Material in the circumstel-lar environment beyond these distances from GM Auriage remains undetected in the less sensitive WFPC2observa-tions.Those noncoronagraphic observations and those in the other WFPC2bands(F675W and F814W)are of signifi-cantly lower signal-to-noise ratio,have more limited dynamic range(due to the sampling mode of the instru-ment),and are adversely affected by larger relative ampli-tude PSF-subtraction residuals than the NICMOS data. For these reasons we confine our discussions of the disk fea-tures and base our scattered light models only on the NIC-MOS observations,which are of higher photometric fidelity.We note the disparity by a factor of2in the disk-scattering fraction of$8%reported by Stapelfeldt et al. (1997)relative to our NICMOS measure of$4%(see x4.1). Rather than a strong intrinsic color difference under the two spatially adjacent passbands of WFPC2(F814W)and NICMOS(F110W)filters,we suggest this is likely caused by systematic instrumental effects in the WFPC2data.Fig.2.—Wavelength-dependent morphology of the GM Aurigae circumstellar environment,illustrated in a two-color composite image derived from F110W(blue)and F160W(red)PSF-subtracted frames.The logarithmic display stretch of the full19>4Â19>3field shows the polar lobes(red)along the disk minor axis,the ribbon of material extending$1300to the northeast(blue),and thefield star used(in each band)to measure the FWHM of the coronagraphic focus PSF.The midplane of the disk and the upper edge of the lower(forward)scattering surface are readily seen at a lower sensitivity logarithmic stretch (inset).No.3,2003GM AURIGAE CIRCUMSTELLAR DISK14714.1.Photometry of the DiskThe photometric efficacy of the GM Aurigae minus BD +032964PSF subtractions permits measurement of the flux density of the circumstellar disk to radial distances closely approaching the geometrical edge of the coronagraphic hole.We estimate the total flux density of the disk from 0>35to 5>0to be 8.0mJy at F110W and 9.3mJy at F160W,both with uncertainties d 20%.We obtained two short (1.22s)but high S/N (each $320)F160W noncoronagraphic tar-get acquisition images of GM Aurigae immediately prior to our coronagraphic exposures.After calibration we mea-sured the F160W flux density of GM Aurigae as 0.344Æ0.007Jy,or H =8.74Æ0.02,which is in agreement with the H =8.73Æ0.16mag in Kenyon &Hartmann (1995).We do not have a contemporaneous measure of the brightness of the star in the NICMOS F110W bands but adopt the J magnitude of 9.37from Kenyon &Hartmann (1995)as a very close surrogate given the reproducibility of the F160W (H band)measure at the time of our observa-tions.From this we find the disk-scattering fraction (ratio of light scattered by the disk to starlight),L disk /L *,is 0.025(Æ20%)in both spectral bands.Thus,in the near-IR,L disk /L *is very similar to that found for the optically thick face-on disk around the K7Ve cTTS TW Hydrae by Weinberger et al.(2002;with L disk /L *=0.024and 0.021for F110W and F160W,respectively).To facilitate flux density meas-urements of the GM Aurigae disk with other instrumental systems that might not probe as effectively to small radii,in Figure 3we provide the integrated flux densities in both bands as a function of the inner radius sampled.The southwest side of the disk beyond 4>5from GM Aurigae along the major axis fell outside the NICMOS field of view.At that distance the disk major axis surface bright-ness declines to 23l Jy arcsec À2in F110W and to 17l Jy arcsec À2in F160W.The disk isophotes,shown in contour maps for both the F110W and F160W images in Figure 4a –4b ,respectively,exhibit a high degree of bilateral symmetry,and the northeast side of the disk extends to approximately 500.The outer edge of the detectable disk adjoining the northeast major axis is spatially coincident with a broad and diffuse ‘‘ribbon ’’of material,which makes the determi-nation of the full extent of the disk difficult,but it would likely be $500in the absence of this material.The position angle of the disk minor axis in the direction of forward scattering was found to be 328=5Æ2=5(see x 5.1).The radial surface brightness profile of the disk,mea-sured along a sector 45 in azimuthal extent centered on the minor axis (toward the northwest),is shown in Figure 5for both filters.These brightness profiles are globally well fitted to power laws with brightness falloffs of r À2.9and r À3.5for F110W and F160W,respectively.Some statistically signifi-cant deviations from these simple power laws are apparent and are understood through an examination of the images themselves.The surface brightness of the northwest minor axis sector of the disk is depressed from the power-law fit in a radial zone $1>3from GM Aurigae,most notably in the F160W filter.This decrement in surface brightness is likely due to higher opacity material in the midplane of the disk that,because of the viewing geometry,partially obscures the lower portion of the disk.The surface brightness in F160W then rises significantly above the r À3.5power-law fit with a maximum deviation at $1>85.The F160W flux densities in this region of $150l Jy arcsec À2likely arise from scattering offthe upper surface of the lower portion of the disk unob-scured by material in the midplane.4.2.Axial Flux DensitiesIn Figure 6we show the F110W and F160W flux densities along both the disk minor and major axes,in both directions outward from GM Aurigae,as functions of distance from the star.The flux densities shown are measured in 1pixel–wide radial increments in regions 100in extent centered on and orthogonal to the axes.In the direction of forward scat-tering (along the minor axis),the flux density decrement at about À1>3,likely due to shadowing by the midplane,and the relative enhancement from the forward-scattering sur-face of the lower part of the disk at about À1>85are readily apparent.The flux density in the ‘‘positive ’’direction along the minor axis (in Fig.6),where the line of sight is to the ‘‘back ’’of the upper portion of the disk,falls offmore rap-idly.In both directions localized brightness enhancements are seen in F160W at about Æ3>5–400,which we interpret as weakly collimated material from a bipolar outflow (see x 7).As one might expect,none of these features are seen along the disk major axis (though the major axis toward the south-west is truncated by the edge of the field of view).Unlike the overall ‘‘front-to-back ’’brightness asymmetry along the minor axis,we find the radial brightness along the major axis reflectively symmetric about GM Aurigae.5.SCATTERED LIGHT MODELWe modeled the NICMOS scattered light images with passively heated disks to determine the geometry (orienta-tion,inclination,and size)and physical characteristics (disk mass,scale height,and envelope infall rate)of the GM Auri-gae circumstellar disk.The properties of the disk grains gov-ern the wavelength-dependent width of the dust lane,the spatial variation of the scattered light,and the slope oftheFig.3.—Area-integrated F110W and F160W flux densities of the GM Aurigae circumstellar disk measured radially outward from the inner radii indicated to a distance of 500.Photometric uncertainties are d 20%of the measured flux densities.1472SCHNEIDER ET AL.Vol.125。
- 1、下载文档前请自行甄别文档内容的完整性,平台不提供额外的编辑、内容补充、找答案等附加服务。
- 2、"仅部分预览"的文档,不可在线预览部分如存在完整性等问题,可反馈申请退款(可完整预览的文档不适用该条件!)。
- 3、如文档侵犯您的权益,请联系客服反馈,我们会尽快为您处理(人工客服工作时间:9:00-18:30)。
October2,200319:23WSPC/Book Trim Size for9in x6in RecommendationChapter5Recommendation Based on PersonalPreferencePei WangDepartment of Computer and Information SciencesTemple University,Philadelphia,PA19122,USAE-mail:pei.wang@This chapterfirst defines a recommendation process,which helps the user to select products from a large number of candidates according to per-sonal preference.Though both conventional database and fuzzy databasehave been used for this task,none of the two provide a proper solution.Anew approach is introduced,which is easy to design and maintain,and pro-vides well justified results.The central idea of this approach is to interpretuser preference as consisting of multiple criteria,each of which is relative toavailable data.The procedure introduced here forms a membership func-tion at run-time according to user request and available data,then use itto rank the candidates.5.1The problemIn web-based e-commerce and on-line shopping,people often meet the“se-lection problem”,that is,the user is looking for a product of a certaintype,withflexibility or uncertainty in the details of the request.Typically,the user has certain“constraints”that must be satisfied,as well as certain“preference”that are desired,but not specified in absolute terms.For example,if the user is looking for a home computer,then most requests are in the form of preference:almost everyone prefers a fast CPU,a large hard drive,a cheap price,and so on,but few of the requests reallyhas afixed range.If the user is search for aflight,then the departure andarrival airports may be determined,while the other parts of the request1October2,200319:23WSPC/Book Trim Size for9in x6in Recommendation2Computational Web Intelligence:Intelligent Technology for Web Applications(such as departure date and time,arrival time,number of transfers,and soon)may beflexible to different degrees.In this situation,if there are M products satisfying the constraints, and all of them are displayed to the user,then often it is too muchinformation,and the user gets little help in using the preferences toget afinal decision.For this situation,a“recommendation system”(also called“recommender system”)is desired[Jameson et.al.,(2002);Kautz,(1998)].Briefly speaking,the function of a recommendation systemis to reduce the size of the displayed list from M to N(which is a muchsmaller number),under the condition that the user’s preference is respectedin the process.Obviously,we do not want N to be too large,otherwise the recommen-dation system does not help much.On the other side,we do not want N tobe too small—if N is one,then the“recommendation system”is actuallymaking decision for the user.This is not desired,under the assumptionthat not all the relevant factors about thefinal decision can be includedin the constraints and preferences,therefore the user still want to comparethe top candidates,and to make thefinal decision,by taking all relevantfactors into consideration.This is exactly what we usually expect whenasking friends or experts for“recommendations”—we hope them to re-duce the number of candidates to a manageable level,without making thefinal decisions for us.Of course,the desired N may be different from situation to situation, and from user to user,but in general,we can take a default value in therange betweenfive and ten.Such a choice is consistent with the psycholog-ical research on human memory(the well known“7±2”phenomenon),aswell as the common practice of picking the“top ten”in various categories.If we can assume that the user only need one product from the M can-didates(which is usually true),and that given sufficient time for analysis,comparison,and actual evaluation,the user would select product P i,thena“good”recommendation system should almost always provides a top-Nlist containing P i in it.With the help of such a system,the user’s decision-making process is simplified,but still get(usually)the same result.According to the above definition of the problem,there are still several ways of making recommendations,which have been explored to variousdegrees[Jameson et.al.,(2002);Kautz,(1998)].For example,recommen-dation can be made according to a top-N list determined by the votingof domain experts or users for each category.Such votes can be collectedexplicitly or implicitly,such as through a“collaborativefiltering”.Or,rec-ommendations can be made according to the similarity among users andsimilarity among products.For example,according to the selections theusers have made before,they are classified into several groups,and theOctober2,200319:23WSPC/Book Trim Size for9in x6in RecommendationRecommendation Based On Personal Preference3 preference of each group is determined by statistics.When a new user isclassified into a certain group,recommendations are made according to thepreference of the members of the same group.What we study in this chapter is a special situation,where we assume that there is no historical information available(such as votes,comments,reviews,past selections,and so on),and the recommendation is only basedon the constraints and preferences provided by the current user.Sincethese requirements are highly personal and change from case to case,thereis no way to anticipate them,and to determine a top-N list for every casein advance.Instead,the recommendation has to be formed at run-timeaccording to user’s requests.Formally,the problem is defined as the following.DB is a database(or an information system of another type)that contains descriptions aboutproducts of certain category.Each product d i is specified as a vector d i=<d i1,d i2,···,d it>,in which each d ij is the value of the product on anattribute A j.In the simplest form,the user’s request consists of a constraint vector c=<c1,c2,···,c t>and a preference vector p=<p1,p2,···,p t>.Eachelement of these two vectors is a condition for the corresponding attributevalue.A condition in constraint,c j,is a binary expression of the form“X relation v”,where X is a variable representing an attribute value,v is a constant value of the corresponding attribute,and relation is one ofthe following relations:=,=,<,≤,>,≥,where the last four are only de-fined on attributes whose values form a total order(therefore X=Y canalways be further specified as X<Y or X>Y).The value of such anexpression is either1(for“true”)or0(for“false”).A condition in preference has the same form as a constraint,exceptthat the relation is“≈”,for“similar to”,and the expression is not binary,but has a value in a certain range.That is,to what extent a preferenceis satisfied is a matter of degree.Without losing generality,we take therange to be the interval[0,1].As a special case,“X≈v”has value1if and only if“X=v”is true.Therefore,the similarity relation canbe seen as a generalization of the equality relation,from binary to multi-valued.For attributes whose values form a total order,we define two newrelations:“X ”is defined as“X≈v min”,and“X ”is defined as“X≈v max”,where“v min”and“v max”are the minimum and maximumvalue of the corresponding attribute,respectively(they do not need to beafinite value).Intuitively,these preferences mean“has a small value”and“has a large value”,respectively.Therefore,constraints and preferences correspond to different ways to assign a“score”to a value of a product on an attribute.After applyingOctober2,200319:23WSPC/Book Trim Size for9in x6in Recommendation4Computational Web Intelligence:Intelligent Technology for Web Applicationson a value d ij,a constraint c j provides a score in{0,1},a preference p jprovides a score in[0,1].Since the variable X in above requirements is just a place holder,it can be omitted without causing confusion.In the following,therefore,we willuse“relation v”for a constraint,and use“≈v”,“ ”,or“ ”for a pref-erence.There is a special condition“null”for“no constraint/preference”,which should be ignored in the recommendation procedure.For example,if eachflight(from a city to another in a given date)is specified only by departure time,arrival time,and prices,then the pref-erence“leave around9AM,arrive early,with a low price”is representedas“≈9, , ”.Similarly,if each computer is specified only by its CPUspeed,hard-drive size,and price,then the preference“fast and cheap”isrepresented as“ ,null, ”.Given these definitions,we can represent a recommendation procedure for top-N products as the following:(1)Apply the user constraint c=<c1,c2,···,c t>on the database DB.For each product d i=<d i1,d i2,···,d it>,if its value d ij satisfiescondition c j for all j=1···t,then it becomes a candidate for recom-mendation.(2)The above step produces a set of candidates of size M.If M≤N,thenrecommendation is unnecessary,and the procedure ends.Otherwisethe procedure continues.(3)Apply the user preference p=<p1,p2,···,p t>to each product d i inthe candidate set,and get a vector of scores<s i1,s i2,···,s it>,wheres ij is the return value of applying preference p j to value d ij.(4)Apply a summarizing function f(x)to the score vector of each productd i in the candidate set to get a total score s i=f(<s i1,s i2,···,s it>),which is a numerical value.(5)Select the top N products according to their total scores,and returnthem as the recommendation to the user.5.2The existing techniquesDescribed in this way,recommendation can be seen as selective informationretrieval.Can we just use existing techniques,such as relational database,to carry out this process?Obviously,the constraints we defined above are very close to conditions in database queries.Now the question is:can the preferences defined abovebe properly handled as conventional database queries?Obviously,a directmapping does not exist,because database queries are based on binary logic,October2,200319:23WSPC/Book Trim Size for9in x6in RecommendationRecommendation Based On Personal Preference5 that is,whether a data item satisfies a condition is a matter of true or false,not a matter of degree.But how about approximative mapping?Maybe wecan translate a preference into a query condition without losing too muchinformation.Such an approximation is possible—actually people are forced to do so on a daily basis.If a user prefers aflight departing around9AM,she oftenhas to specify her preference as a query condition for a time interval[9-∆,9+∆],where∆is a constant,like30minutes or so.If a user prefers a fastand cheap computer,he has to specify the CPU speed as above a certainthreshold(such as2GHz)and the price as below a certain threshold(suchas$1000).Since the query language of conventional database only uses binary con-ditions,what happened above is that preferences are converted into con-straints,then represented as query conditions.Of course this is an approx-imation,but is it good enough?We say that the very idea of“recommendation”is lost in such an approx-imation,so it is unacceptable as a way to build a recommendation system.Here the problem is:if preference is treated as constraints,whether a prod-uct satisfies the user’s request is a matter of true or false,and there is noway to rank the candidates that satisfying all the conditions.Consequently,the user simply gets all products that returned by the query,or a randomsubset of it as the“top-N”list,which is not really based on user preference.When the user is forced to specify an interval for each attribute value, two extreme cases often happen:the query either returns no product orreturns too many.For example,when the user is looking for a“fast andcheap notebook computer”,if the request is“translated”into query for“faster than3GHz and cheaper than$500”,there may be nothing available,but if it is treated as a query for“faster than1GHz and cheaper than$3000”,then there may befive hundred of them.If we really want a certainnumber(say5to10)of products returned by the query,the conditions init should be tuned properly.For example,a query for“faster than2GHzand cheaper than$1250”returnsfive products,which looks like a goodrecommendation—until we think about the following issues.To translate the preferences into a query which returns data items around a certain number,the user must be either very familiar with thedistributions of the attribute values,or willing to try many different inter-vals/thresholds.In the former case,the user is an expert in the domain,so rarely needs any recommendation at all.In the latter case,the pro-cess is very time-consuming and often frustrating—many readers of thisbook may have such personal experience.Recommendation system cannotassume either of the two cases,because it is designed exactly to help theusers who neither are experts in thefield,nor have the time and patienceOctober2,200319:23WSPC/Book Trim Size for9in x6in Recommendation6Computational Web Intelligence:Intelligent Technology for Web Applicationsto evaluate many possibilities.Furthermore,even when“faster than2GHz and cheaper than$1250”returns5products,the list may exclude some good candidates.For exam-ple,there may be a product which is a little bit slower than2GHz,butmuch cheaper than all thefive products in the list,or a product which isa little bit more expensive than$1250,but much faster than thefive prod-ucts in the list.No matter how the query condition is determined,suchpossibilities always exist.This is the case,because binary query cannot handle trade-offamong multiple preferences.When a user preference contains multiple compo-nents,it is normal,rather than exceptional,for them to conflict with eachother.For example,in almost all shopping situations,users prefer productswhich are cheap and with high quality,even when we all know that thesetwo preferences usually cannot be optimized together.Without a quantita-tive measurement on“degree of satisfying”or something like that,trade-offbecomes arbitrary.What it means is that even though database query is still the most often used technique for the users to request for data items,it does not providemuch help for selective retrieval,because of the binary expressions used inthe query language.If we want to directly process the preference defined before,we need conditions that different values satisfy to different degrees.Under thisconsideration,the most obvious solution is to apply fuzzy logic[Zadeh,(1975)]into database query,which leads to the idea of“fuzzy database”[Yang and Lee-Kwang,(2000);Yang et.al.,(2001)].A recommendation system based on fuzzy logic can be designed ac-cording to the“recommendation procedure”defined previously,where theprocessing of the constraints are just like in conventional database.Thepreferences are specified using“linguistic variables”,such as“≈fast”forspeed,and“≈cheap”for price.Each linguistic value corresponds to afuzzy set,with a membership function to calculate the score for each at-tribute value.Finally,the total score of a product is the minimum of all theindividual scores,because in fuzzy logic the(default)function for“AND”(i.e.,conjunction of conditions)is“min”.In this way,we can indeed get a recommendation system satisfying our previous description,and it no longer suffers from the problem in conven-tional database discussed above.Since the idea of fuzzy logic has been wellknown for many years,and this application is not that difficult,why havenot we seen many such systems?Among all reasons,a major issue is the design and maintenance of the membership functions.According to the common interpretation of mem-bership function,it measures a subjective opinion on the“compatibility”October2,200319:23WSPC/Book Trim Size for9in x6in RecommendationRecommendation Based On Personal Preference7 between the linguistic value and numerical values[Zadeh,(1975)].For ex-ample,it can be said that“The membership of$1000to cheap is0.9”,“Themembership of$1250to cheap is0.85”,and so on.Therefore,the designerof the recommendation system is responsible for specifying membershipfunctions for every linguistic value.Furthermore,it is well known that the same linguistic value,such as “cheap”,corresponds to very different membership functions when used ondifferent categories.Clearly,what can be labeled as“cheap”can have radi-cally different prices for categories like notebook computer,super computer,house,notebook,pencil,and so on.Consequently,a separate membershipfunction is needed for each linguistic value on each category.Even if thatcan be provided,there is still trouble—these functions may need to beadjusted from time to time.For example,what is considered as“a cheapnotebook computer”two years ago is no longer“cheap”according to thecurrent situation in the market.In summery,though it is possible to build a recommendation system us-ing fuzzy logic,the design and maintenance process is complicated(becauseof the relative nature of the membership functions),and the recommenda-tions are hard to justify(because of the subjective nature of the membershipfunctions).All these problem comes from a common root,that is,though fuzzy logic represents and processes fuzziness in various ways,it does not properlyinterpret fuzziness.Since this problem has been analyzed in detail in aprevious publication[Wang,(1996)],we will not repeat the discussion here.We just want to say that because of this problem,fuzzy logic does not workwell in recommendation systems,except in special situations where only afew membership functions are needed,and they do not change over time.5.3A new approachThe main content of this chapter is to introduce a new approach for rec-ommendation system design.This approach is similar to the fuzzy-logicapproach discussed above,except that the membership functions are auto-matically generated from the available data by an algorithm,therefore theresults are well justified,and the design and maintenance of the system isrelatively easy.This approach of recommendation is a by-product,a practical applica-tion,of the author’s research on general-purpose intelligent system[Wang,(1995)].In the following,we only introduce the aspects of the researchthat is directly related to the recommendation procedure.For how thisprocedure is related to the big picture of artificial intelligence,please visitOctober2,200319:23WSPC/Book Trim Size for9in x6in Recommendation8Computational Web Intelligence:Intelligent Technology for Web Applicationsthe author’s website at /~pwang/for relatedmaterials.Again,here we treat the constraints in the same way as in conventional database.After the M candidates are returned by the query,the recom-mendation task is treated as a task of selecting“good”instances of a givenconcept among given candidates.Concretely,a preference vector p=<p1,p2,···,p t>defines a conceptC p,the instance of which is what the user is looking for.For example,“fastand cheap notebook computers”is such a concept,and“flights(from onegiven city to another)leaving around9AM(on a certain day)and arriveas early as possible”is another.Such a concept is defined by the preferencevector,as a set of properties.That is,“fast and cheap notebook computers”is a subset of notebook computers that have properties as being“fast”andbeing“cheap”.According to the model of categorization used in this approach,in the recommendation process the concept C p is defined collectively by all thegiven properties,each of which contributes to the meaning of the concept,and none of which is sufficient or necessary for the membership by itself.Furthermore,whether an attribute value satisfy a corresponding propertyis a matter of degree.Unlike in fuzzy logic,where degree of membership is a subjective judg-ment that cannot be further analyzed,in our approach the“score”of eachvalue for a given preference is the proportion of positive evidence among allevidence,that is,s=w+/(w++w−),where w+and w−are the amountof positive and negative evidence,respectively,for the preference to be sat-isfied by the value.How is evidence defined and measured?Let us start from a concrete example.If the price of a notebook computer is$1250,then to what extentit belongs to the concept of“cheap notebook computers”?According toour theory[Wang,(1996)],such a question cannot be answered without a“reference class”,that is,it depends on the answer of another question:“Compare to what?”.Adjectives like“cheap”get their meaning from re-lations like“cheaper than”,though the object of the comparison is oftenomitted in the expression.In the recommendation process,we assume thatthe default objects of comparison are the other candidates.Therefore,weinterpret“cheap”as“cheaper than the other candidates”.Since usuallythere are multiple candidates,and some of them may be cheaper,and oth-ers more expensive,than the product under consideration,whether it is“cheaper than the other candidates”is usually a matter of degree.If there are M candidates that satisfy the constraints,then they are used to score one another for the preferences.To decide the score for a$1250computer to be labeled as“cheap”,the other M−1candidates areOctober2,200319:23WSPC/Book Trim Size for9in x6in RecommendationRecommendation Based On Personal Preference9 compared to it one by one in price,where more expensive ones are countedas positive evidence,and cheaper ones as negative evidence,for the labeling(candidates with the same price provide no evidence).The total amountof evidence is the sum of the amount of positive evidence and the amountof negative evidence.Therefore,among the M candidates,if there are m1of them are more expensive than$1250,and m2of them are cheaper than$1250,then the score for a$1250computer to be labeled as“cheap”canbe simply taken as m1/(m1+m2)[Wang,(1996)].Especially,the cheapestcandidate gets a score1.0,and the most expensive one get0.0,for the givenpreference.The above approach can be applied to a preference as far as the values of the corresponding attribute form a total order,even if the values arenot numerical.The following is a general definition of evidence in therecommendation process:•When a value d ij is evaluated according to a preference p j of the form“≈v”(“similar to v”),if another value d kj(of another candidate)isfarther away from v than d ij is,it is positive evidence;if d kj is closerto v than d ij is,it is negative evidence.•When a value d ij is evaluated according to a preference p j of the form“ ”(“has a small value”),if another value d kj(of another candidate)is larger than d ij,it is positive evidence;if d kj is smaller than d ij,it isnegative evidence.•When a value d ij is evaluated according to a preference p j of the form“ ”(“has a large value”),if another value d kj(of another candidate)is smaller than d ij,it is positive evidence;if d kj is larger than d ij,it isnegative evidence.After separating positive and negative evidence from non-evidence amongthe other candidates,their number can be used as the above m1and m2,respectively,then from them the score of the given value can be calculatedaccording to the previous formula.For a given attribute,if its values are not only comparable,but also numerical,sometimes the distance between values should be taken intoaccount when scores are calculated.For example,to evaluate the score for$1250to be labeled as“cheap”,the existence of a$750and a$1200,as theprices of other candidates,are very different.Though both are negativeevidence,the former is clearly a much“stronger”one than the latter.Forthis situation,a more meaningful way to calculate the amount of evidenceis to give each piece of evidence a“weight”,which is the difference of thatvalue and the given value.For the above case,the weights of the two piecesof evidence are1250-750=500and1250-1200=50,respectively.Nowthe amount of(positive and negative)evidence m1and m2are weightedOctober 2,200319:23WSPC/Book Trim Size for 9in x 6in Recommendation10Computational Web Intelligence:Intelligent Technology for Web Applicationssum of pieces of evidence.For a product d i ,after its attribute values get their scores as a vector<s i 1,s i 2,···,s it >according to a given preference p =<p 1,p 2,···,p t >,the next step is to combine them into a total score s i ,which representsthe membership for the product to be an instance of the concept C p .Herewe treat each preference p i as an independent channel to collect (positiveand negative)evidence for the membership relation.Therefore,evidencecollected in each channel should be pooled together.As a default rule(assume all the preferences are equally weighted and each score is obtainedfrom the same number of comparisons),the total scoreis simply the averageof the individual scores,that is,s i =( t j =1s ij )/t .Now let us give a complete and general algorithm for the recommenda-tion procedure described above.The database DB is a collection of data items,each of which is a vectord i =<d i 1,d i 2,···,d it >,in which each d ij is the value of d i on an attributeA j .In other words,DB is a matrix,where each row is a data item,andeach column corresponds to an attribute.A recommendation request r consists of two components,a constraintvector c =<c 1,c 2,···,c t >and a preference vector p =<p 1,p 2,···,p t >.Each c j has the form “relation j v j ”,where v j is a constant value,andrelation j is one of the following relations:=,=,<,≤,>,≥.Theevaluation of c j against a value d ij should return 1(true )or 0(false ).Each p j has the form ‘≈v j ”,“ ”,or “ ”.The evaluation of p j against avalue d ij should return a real number in [0,1].For a given DB and a given r ,the recommendation procedure,for atop-N list of data items satisfying r in DB ,is the following:(1)Translate c into a (conventional)database query on DB ,and get thecandidate set,which includes all data items in DB satisfying c .(2)Assume the size of the candidates set M .If M ≤N ,then recommen-dation is unnecessary,and the procedure may ends here.Otherwise theprocedure continues.(3)Apply the user preference p =<p 1,p 2,···,p t >to each data item d i ,and get a vector of scores <s i 1,s i 2,···,s it >,where s ij is the returnvalue of applying preference p j to value d ij .(4)Let the total score of a candidate d i to be ( tj =1s ij )/t .(5)Select the top N data items according to their total scores,and returnthem as the recommendation to the user.As options,the total scoreof each may be displayed,and they may be sorted according to theirtotal scores.In the above step (3),for each value d ij and preference p j ,the score s ijis the ratio of positive evidence among all evidence,that is,s ij =w +/(w ++。