Trust and Epistemic Communities in Biodiversity Data Sharing
人工智能英文参考文献(最新120个)
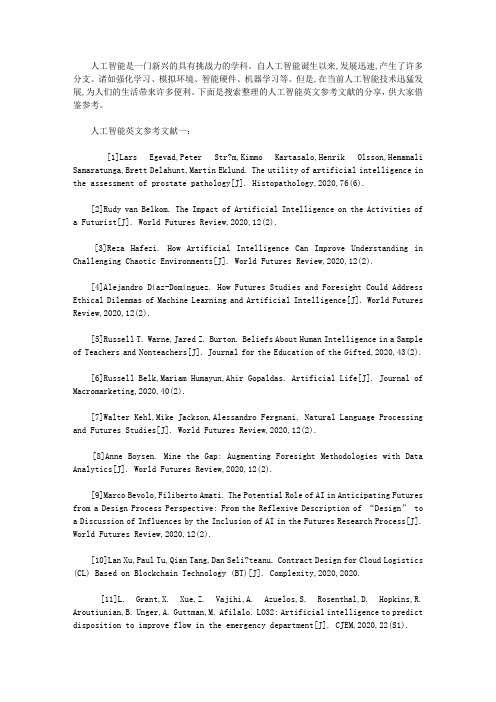
人工智能是一门新兴的具有挑战力的学科。
自人工智能诞生以来,发展迅速,产生了许多分支。
诸如强化学习、模拟环境、智能硬件、机器学习等。
但是,在当前人工智能技术迅猛发展,为人们的生活带来许多便利。
下面是搜索整理的人工智能英文参考文献的分享,供大家借鉴参考。
人工智能英文参考文献一:[1]Lars Egevad,Peter Str?m,Kimmo Kartasalo,Henrik Olsson,Hemamali Samaratunga,Brett Delahunt,Martin Eklund. The utility of artificial intelligence in the assessment of prostate pathology[J]. Histopathology,2020,76(6).[2]Rudy van Belkom. The Impact of Artificial Intelligence on the Activities ofa Futurist[J]. World Futures Review,2020,12(2).[3]Reza Hafezi. How Artificial Intelligence Can Improve Understanding in Challenging Chaotic Environments[J]. World Futures Review,2020,12(2).[4]Alejandro Díaz-Domínguez. How Futures Studies and Foresight Could Address Ethical Dilemmas of Machine Learning and Artificial Intelligence[J]. World Futures Review,2020,12(2).[5]Russell T. Warne,Jared Z. Burton. Beliefs About Human Intelligence in a Sample of Teachers and Nonteachers[J]. Journal for the Education of the Gifted,2020,43(2).[6]Russell Belk,Mariam Humayun,Ahir Gopaldas. Artificial Life[J]. Journal of Macromarketing,2020,40(2).[7]Walter Kehl,Mike Jackson,Alessandro Fergnani. Natural Language Processing and Futures Studies[J]. World Futures Review,2020,12(2).[8]Anne Boysen. Mine the Gap: Augmenting Foresight Methodologies with Data Analytics[J]. World Futures Review,2020,12(2).[9]Marco Bevolo,Filiberto Amati. The Potential Role of AI in Anticipating Futures from a Design Process Perspective: From the Reflexive Description of “Design” to a Discussion of Influences by the Inclusion of AI in the Futures Research Process[J]. World Futures Review,2020,12(2).[10]Lan Xu,Paul Tu,Qian Tang,Dan Seli?teanu. Contract Design for Cloud Logistics (CL) Based on Blockchain Technology (BT)[J]. Complexity,2020,2020.[11]L. Grant,X. Xue,Z. Vajihi,A. Azuelos,S. Rosenthal,D. Hopkins,R. Aroutiunian,B. Unger,A. Guttman,M. Afilalo. LO32: Artificial intelligence to predict disposition to improve flow in the emergency department[J]. CJEM,2020,22(S1).[12]A. Kirubarajan,A. Taher,S. Khan,S. Masood. P071: Artificial intelligence in emergency medicine: A scoping review[J]. CJEM,2020,22(S1).[13]L. Grant,P. Joo,B. Eng,A. Carrington,M. Nemnom,V. Thiruganasambandamoorthy. LO22: Risk-stratification of emergency department syncope by artificial intelligence using machine learning: human, statistics or machine[J]. CJEM,2020,22(S1).[14]Riva Giuseppe,Riva Eleonora. OS for Ind Robots: Manufacturing Robots Get Smarter Thanks to Artificial Intelligence.[J]. Cyberpsychology, behavior and social networking,2020,23(5).[15]Markus M. Obmann,Aurelio Cosentino,Joshy Cyriac,Verena Hofmann,Bram Stieltjes,Daniel T. Boll,Benjamin M. Yeh,Matthias R. Benz. Quantitative enhancement thresholds and machine learning algorithms for the evaluation of renal lesions using single-phase split-filter dual-energy CT[J]. Abdominal Radiology,2020,45(1).[16]Haytham H. Elmousalami,Mahmoud Elaskary. Drilling stuck pipe classification and mitigation in the Gulf of Suez oil fields using artificial intelligence[J]. Journal of Petroleum Exploration and Production Technology,2020,10(10).[17]Rüdiger Schulz-Wendtland,Karin Bock. Bildgebung in der Mammadiagnostik –Ein Ausblick <trans-title xml:lang="en">Imaging in breast diagnostics—an outlook [J]. Der Gyn?kologe,2020,53(6).</trans-title>[18]Nowakowski Piotr,Szwarc Krzysztof,Boryczka Urszula. Combining an artificial intelligence algorithm and a novel vehicle for sustainable e-waste collection[J]. Science of the Total Environment,2020,730.[19]Wang Huaizhi,Liu Yangyang,Zhou Bin,Li Canbing,Cao Guangzhong,Voropai Nikolai,Barakhtenko Evgeny. Taxonomy research of artificial intelligence for deterministic solar power forecasting[J]. Energy Conversion and Management,2020,214.[20]Kagemoto Hiroshi. Forecasting a water-surface wave train with artificial intelligence- A case study[J]. Ocean Engineering,2020,207.[21]Tomonori Aoki,Atsuo Yamada,Kazuharu Aoyama,Hiroaki Saito,Gota Fujisawa,Nariaki Odawara,Ryo Kondo,Akiyoshi Tsuboi,Rei Ishibashi,Ayako Nakada,Ryota Niikura,Mitsuhiro Fujishiro,Shiro Oka,Soichiro Ishihara,Tomoki Matsuda,Masato Nakahori,Shinji Tanaka,Kazuhiko Koike,Tomohiro Tada. Clinical usefulness of a deep learning‐based system as the first screening on small‐bowel capsule endoscopy reading[J]. Digestive Endoscopy,2020,32(4).[22]Masashi Fujii,Hajime Isomoto. Next generation of endoscopy: Harmony with artificial intelligence and robotic‐assisted devices[J]. Digestive Endoscopy,2020,32(4).[23]Roberto Verganti,Luca Vendraminelli,Marco Iansiti. Innovation and Design in the Age of Artificial Intelligence[J]. Journal of Product Innovation Management,2020,37(3).[24]Yuval Elbaz,David Furman,Maytal Caspary Toroker. Modeling Diffusion in Functional Materials: From Density Functional Theory to Artificial Intelligence[J]. Advanced Functional Materials,2020,30(18).[25]Dinesh Visva Gunasekeran,Tien Yin Wong. Artificial Intelligence in Ophthalmology in 2020: A Technology on the Cusp for Translation and Implementation[J]. Asia-Pacific Journal of Ophthalmology,2020,9(2).[26]Fu-Neng Jiang,Li-Jun Dai,Yong-Ding Wu,Sheng-Bang Yang,Yu-Xiang Liang,Xin Zhang,Cui-Yun Zou,Ren-Qiang He,Xiao-Ming Xu,Wei-De Zhong. The study of multiple diagnosis models of human prostate cancer based on Taylor database by artificial neural networks[J]. Journal of the Chinese Medical Association,2020,83(5).[27]Matheus Calil Faleiros,Marcello Henrique Nogueira-Barbosa,Vitor Faeda Dalto,JoséRaniery Ferreira Júnior,Ariane Priscilla Magalh?es Tenório,Rodrigo Luppino-Assad,Paulo Louzada-Junior,Rangaraj Mandayam Rangayyan,Paulo Mazzoncini de Azevedo-Marques. Machine learning techniques for computer-aided classification of active inflammatory sacroiliitis in magnetic resonance imaging[J]. Advances in Rheumatology,2020,60(1078).[28]Balamurugan Balakreshnan,Grant Richards,Gaurav Nanda,Huachao Mao,Ragu Athinarayanan,Joseph Zaccaria. PPE Compliance Detection using Artificial Intelligence in Learning Factories[J]. Procedia Manufacturing,2020,45.[29]M. Stévenin,V. Avisse,N. Ducarme,A. de Broca. Qui est responsable si un robot autonome vient à entra?ner un dommage ?[J]. Ethique et Santé,2020.[30]Fatemeh Barzegari Banadkooki,Mohammad Ehteram,Fatemeh Panahi,Saad Sh. Sammen,Faridah Binti Othman,Ahmed EL-Shafie. Estimation of Total Dissolved Solids (TDS) using New Hybrid Machine Learning Models[J]. Journal of Hydrology,2020.[31]Adam J. Schwartz,Henry D. Clarke,Mark J. Spangehl,Joshua S. Bingham,DavidA. Etzioni,Matthew R. Neville. Can a Convolutional Neural Network Classify Knee Osteoarthritis on Plain Radiographs as Accurately as Fellowship-Trained Knee Arthroplasty Surgeons?[J]. The Journal of Arthroplasty,2020.[32]Ivana Nizetic Kosovic,Toni Mastelic,Damir Ivankovic. Using Artificial Intelligence on environmental data from Internet of Things for estimating solar radiation: Comprehensive analysis[J]. Journal of Cleaner Production,2020.[33]Lauren Fried,Andrea Tan,Shirin Bajaj,Tracey N. Liebman,David Polsky,Jennifer A. Stein. Technological advances for the detection of melanoma: Part I. Advances in diagnostic techniques[J]. Journal of the American Academy of Dermatology,2020.[34]Mohammed Amoon,Torki Altameem,Ayman Altameem. Internet of things Sensor Assisted Security and Quality Analysis for Health Care Data Sets Using Artificial Intelligent Based Heuristic Health Management System[J]. Measurement,2020.[35]E. Lotan,C. Tschider,D.K. Sodickson,A. Caplan,M. Bruno,B. Zhang,Yvonne W. Lui. Medical Imaging and Privacy in the Era of Artificial Intelligence: Myth, Fallacy, and the Future[J]. Journal of the American College of Radiology,2020.[36]Fabien Lareyre,Cédric Adam,Marion Carrier,Juliette Raffort. Artificial Intelligence in Vascular Surgery: moving from Big Data to Smart Data[J]. Annals of Vascular Surgery,2020.[37]Ilesanmi Daniyan,Khumbulani Mpofu,Moses Oyesola,Boitumelo Ramatsetse,Adefemi Adeodu. Artificial intelligence for predictive maintenance in the railcar learning factories[J]. Procedia Manufacturing,2020,45.[38]Janet L. McCauley,Anthony E. Swartz. Reframing Telehealth[J]. Obstetrics and Gynecology Clinics of North America,2020.[39]Jean-Emmanuel Bibault,Lei Xing. Screening for chronic obstructive pulmonary disease with artificial intelligence[J]. The Lancet Digital Health,2020,2(5).[40]Andrea Laghi. Cautions about radiologic diagnosis of COVID-19 infection driven by artificial intelligence[J]. The Lancet Digital Health,2020,2(5).人工智能英文参考文献二:[41]K. Orhan,I. S. Bayrakdar,M. Ezhov,A. Kravtsov,T. ?zyürek. Evaluation of artificial intelligence for detecting periapical pathosis on cone‐beam computed tomography scans[J]. International Endodontic Journal,2020,53(5).[42]Avila A M,Mezi? I. Data-driven analysis and forecasting of highway traffic dynamics.[J]. Nature communications,2020,11(1).[43]Neri Emanuele,Miele Vittorio,Coppola Francesca,Grassi Roberto. Use of CT andartificial intelligence in suspected or COVID-19 positive patients: statement of the Italian Society of Medical and Interventional Radiology.[J]. La Radiologia medica,2020.[44]Tau Noam,Stundzia Audrius,Yasufuku Kazuhiro,Hussey Douglas,Metser Ur. Convolutional Neural Networks in Predicting Nodal and Distant Metastatic Potential of Newly Diagnosed Non-Small Cell Lung Cancer on FDG PET Images.[J]. AJR. American journal of roentgenology,2020.[45]Coppola Francesca,Faggioni Lorenzo,Regge Daniele,Giovagnoni Andrea,Golfieri Rita,Bibbolino Corrado,Miele Vittorio,Neri Emanuele,Grassi Roberto. Artificial intelligence: radiologists' expectations and opinions gleaned from a nationwide online survey.[J]. La Radiologia medica,2020.[46]?. ? ? ? ? [J]. ,2020,25(4).[47]Savage Rock H,van Assen Marly,Martin Simon S,Sahbaee Pooyan,Griffith Lewis P,Giovagnoli Dante,Sperl Jonathan I,Hopfgartner Christian,K?rgel Rainer,Schoepf U Joseph. Utilizing Artificial Intelligence to Determine Bone Mineral Density Via Chest Computed Tomography.[J]. Journal of thoracic imaging,2020,35 Suppl 1.[48]Brzezicki Maksymilian A,Bridger Nicholas E,Kobeti? Matthew D,Ostrowski Maciej,Grabowski Waldemar,Gill Simran S,Neumann Sandra. Artificial intelligence outperforms human students in conducting neurosurgical audits.[J]. Clinical neurology and neurosurgery,2020,192.[49]Lockhart Mark E,Smith Andrew D. Fatty Liver Disease: Artificial Intelligence Takes on the Challenge.[J]. Radiology,2020,295(2).[50]Wood Edward H,Korot Edward,Storey Philip P,Muscat Stephanie,Williams George A,Drenser Kimberly A. The retina revolution: signaling pathway therapies, genetic therapies, mitochondrial therapies, artificial intelligence.[J]. Current opinion in ophthalmology,2020,31(3).[51]Ho Dean,Quake Stephen R,McCabe Edward R B,Chng Wee Joo,Chow Edward K,Ding Xianting,Gelb Bruce D,Ginsburg Geoffrey S,Hassenstab Jason,Ho Chih-Ming,Mobley William C,Nolan Garry P,Rosen Steven T,Tan Patrick,Yen Yun,Zarrinpar Ali. Enabling Technologies for Personalized and Precision Medicine.[J]. Trends in biotechnology,2020,38(5).[52]Fischer Andreas M,Varga-Szemes Akos,van Assen Marly,Griffith L Parkwood,Sahbaee Pooyan,Sperl Jonathan I,Nance John W,Schoepf U Joseph. Comparison of Artificial Intelligence-Based Fully Automatic Chest CT Emphysema Quantification to Pulmonary Function Testing.[J]. AJR. American journal ofroentgenology,2020,214(5).[53]Moore William,Ko Jane,Gozansky Elliott. Artificial Intelligence Pertaining to Cardiothoracic Imaging and Patient Care: Beyond Image Interpretation.[J]. Journal of thoracic imaging,2020,35(3).[54]Hwang Eui Jin,Park Chang Min. Clinical Implementation of Deep Learning in Thoracic Radiology: Potential Applications and Challenges.[J]. Korean journal of radiology,2020,21(5).[55]Mateen Bilal A,David Anna L,Denaxas Spiros. Electronic Health Records to Predict Gestational Diabetes Risk.[J]. Trends in pharmacological sciences,2020,41(5).[56]Yao Xiang,Mao Ling,Lv Shunli,Ren Zhenghong,Li Wentao,Ren Ke. CT radiomics features as a diagnostic tool for classifying basal ganglia infarction onset time.[J]. Journal of the neurological sciences,2020,412.[57]van Assen Marly,Banerjee Imon,De Cecco Carlo N. Beyond the Artificial Intelligence Hype: What Lies Behind the Algorithms and What We Can Achieve.[J]. Journal of thoracic imaging,2020,35 Suppl 1.[58]Guzik Tomasz J,Fuster Valentin. Leaders in Cardiovascular Research: Valentin Fuster.[J]. Cardiovascular research,2020,116(6).[59]Fischer Andreas M,Eid Marwen,De Cecco Carlo N,Gulsun Mehmet A,van Assen Marly,Nance John W,Sahbaee Pooyan,De Santis Domenico,Bauer Maximilian J,Jacobs Brian E,Varga-Szemes Akos,Kabakus Ismail M,Sharma Puneet,Jackson Logan J,Schoepf U Joseph. Accuracy of an Artificial Intelligence Deep Learning Algorithm Implementing a Recurrent Neural Network With Long Short-term Memory for the Automated Detection of Calcified Plaques From Coronary Computed Tomography Angiography.[J]. Journal of thoracic imaging,2020,35 Suppl 1.[60]Ghosh Adarsh,Kandasamy Devasenathipathy. Interpretable Artificial Intelligence: Why and When.[J]. AJR. American journal of roentgenology,2020,214(5).[61]M.Rosario González-Rodríguez,M.Carmen Díaz-Fernández,Carmen Pacheco Gómez. Facial-expression recognition: An emergent approach to the measurement of tourist satisfaction through emotions[J]. Telematics and Informatics,2020,51.[62]Ru-Xi Ding,Iván Palomares,Xueqing Wang,Guo-Rui Yang,Bingsheng Liu,Yucheng Dong,Enrique Herrera-Viedma,Francisco Herrera. Large-Scale decision-making: Characterization, taxonomy, challenges and future directions from an Artificial Intelligence and applications perspective[J]. Information Fusion,2020,59.[63]Abdulrhman H. Al-Jebrni,Brendan Chwyl,Xiao Yu Wang,Alexander Wong,Bechara J. Saab. AI-enabled remote and objective quantification of stress at scale[J]. Biomedical Signal Processing and Control,2020,59.[64]Gillian Thomas,Elizabeth Eisenhauer,Robert G. Bristow,Cai Grau,Coen Hurkmans,Piet Ost,Matthias Guckenberger,Eric Deutsch,Denis Lacombe,Damien C. Weber. The European Organisation for Research and Treatment of Cancer, State of Science in radiation oncology and priorities for clinical trials meeting report[J]. European Journal of Cancer,2020,131.[65]Muhammad Asif. Are QM models aligned with Industry 4.0? A perspective on current practices[J]. Journal of Cleaner Production,2020,258.[66]Siva Teja Kakileti,Himanshu J. Madhu,Geetha Manjunath,Leonard Wee,Andre Dekker,Sudhakar Sampangi. Personalized risk prediction for breast cancer pre-screening using artificial intelligence and thermal radiomics[J]. Artificial Intelligence In Medicine,2020,105.[67]. Evaluation of Payer Budget Impact Associated with the Use of Artificial Intelligence in Vitro Diagnostic, Kidneyintelx, to Modify DKD Progression:[J]. American Journal of Kidney Diseases,2020,75(5).[68]Rohit Nishant,Mike Kennedy,Jacqueline Corbett. Artificial intelligence for sustainability: Challenges, opportunities, and a research agenda[J]. International Journal of Information Management,2020,53.[69]Hoang Nguyen,Xuan-Nam Bui. Soft computing models for predicting blast-induced air over-pressure: A novel artificial intelligence approach[J]. Applied Soft Computing Journal,2020,92.[70]Benjamin S. Hopkins,Aditya Mazmudar,Conor Driscoll,Mark Svet,Jack Goergen,Max Kelsten,Nathan A. Shlobin,Kartik Kesavabhotla,Zachary A Smith,Nader S Dahdaleh. Using artificial intelligence (AI) to predict postoperative surgical site infection: A retrospective cohort of 4046 posterior spinal fusions[J]. Clinical Neurology and Neurosurgery,2020,192.[71]Mei Yang,Runze Zhou,Xiangjun Qiu,Xiangfei Feng,Jian Sun,Qunshan Wang,Qiufen Lu,Pengpai Zhang,Bo Liu,Wei Li,Mu Chen,Yan Zhao,Binfeng Mo,Xin Zhou,Xi Zhang,Yingxue Hua,Jin Guo,Fangfang Bi,Yajun Cao,Feng Ling,Shengming Shi,Yi-Gang Li. Artificial intelligence-assisted analysis on the association between exposure to ambient fine particulate matter and incidence of arrhythmias in outpatients of Shanghai community hospitals[J]. Environment International,2020,139.[72]Fatemehalsadat Madaeni,Rachid Lhissou,Karem Chokmani,Sebastien Raymond,Yves Gauthier. Ice jam formation, breakup and prediction methods based on hydroclimatic data using artificial intelligence: A review[J]. Cold Regions Science and Technology,2020,174.[73]Steve Chukwuebuka Arum,David Grace,Paul Daniel Mitchell. A review of wireless communication using high-altitude platforms for extended coverage and capacity[J]. Computer Communications,2020,157.[74]Yong-Hong Kuo,Nicholas B. Chan,Janny M.Y. Leung,Helen Meng,Anthony Man-Cho So,Kelvin K.F. Tsoi,Colin A. Graham. An Integrated Approach of Machine Learning and Systems Thinking for Waiting Time Prediction in an Emergency Department[J]. International Journal of Medical Informatics,2020,139.[75]Matteo Terzi,Gian Antonio Susto,Pratik Chaudhari. Directional adversarial training for cost sensitive deep learning classification applications[J]. Engineering Applications of Artificial Intelligence,2020,91.[76]Arman Kilic. Artificial Intelligence and Machine Learning in Cardiovascular Health Care[J]. The Annals of Thoracic Surgery,2020,109(5).[77]Hossein Azarmdel,Ahmad Jahanbakhshi,Seyed Saeid Mohtasebi,Alfredo Rosado Mu?oz. Evaluation of image processing technique as an expert system in mulberry fruit grading based on ripeness level using artificial neural networks (ANNs) and support vector machine (SVM)[J]. Postharvest Biology and Technology,2020,166.[78]Wafaa Wardah,Abdollah Dehzangi,Ghazaleh Taherzadeh,Mahmood A. Rashid,M.G.M. Khan,Tatsuhiko Tsunoda,Alok Sharma. Predicting protein-peptide binding sites with a deep convolutional neural network[J]. Journal of Theoretical Biology,2020,496.[79]Francisco F.X. Vasconcelos,Róger M. Sarmento,Pedro P. Rebou?as Filho,Victor Hugo C. de Albuquerque. Artificial intelligence techniques empowered edge-cloud architecture for brain CT image analysis[J]. Engineering Applications of Artificial Intelligence,2020,91.[80]Masaaki Konishi. Bioethanol production estimated from volatile compositions in hydrolysates of lignocellulosic biomass by deep learning[J]. Journal of Bioscience and Bioengineering,2020,129(6).人工智能英文参考文献三:[81]J. Kwon,K. Kim. Artificial Intelligence for Early Prediction of Pulmonary Hypertension Using Electrocardiography[J]. Journal of Heart and Lung Transplantation,2020,39(4).[82]C. Maathuis,W. Pieters,J. van den Berg. Decision support model for effects estimation and proportionality assessment for targeting in cyber operations[J]. Defence Technology,2020.[83]Samer Ellahham. Artificial Intelligence in Diabetes Care[J]. The American Journal of Medicine,2020.[84]Yi-Ting Hsieh,Lee-Ming Chuang,Yi-Der Jiang,Tien-Jyun Chang,Chung-May Yang,Chang-Hao Yang,Li-Wei Chan,Tzu-Yun Kao,Ta-Ching Chen,Hsuan-Chieh Lin,Chin-Han Tsai,Mingke Chen. Application of deep learning image assessment software VeriSee? for diabetic retinopathy screening[J]. Journal of the Formosan Medical Association,2020.[85]Emre ARTUN,Burak KULGA. Selection of candidate wells for re-fracturing in tight gas sand reservoirs using fuzzy inference[J]. Petroleum Exploration and Development Online,2020,47(2).[86]Alberto Arenal,Cristina Armu?a,Claudio Feijoo,Sergio Ramos,Zimu Xu,Ana Moreno. Innovation ecosystems theory revisited: The case of artificial intelligence in China[J]. Telecommunications Policy,2020.[87]T. Som,M. Dwivedi,C. Dubey,A. Sharma. Parametric Studies on Artificial Intelligence Techniques for Battery SOC Management and Optimization of Renewable Power[J]. Procedia Computer Science,2020,167.[88]Bushra Kidwai,Nadesh RK. Design and Development of Diagnostic Chabot for supporting Primary Health Care Systems[J]. Procedia Computer Science,2020,167.[89]Asl? Bozda?,Ye?im Dokuz,?znur Begüm G?k?ek. Spatial prediction of PM 10 concentration using machine learning algorithms in Ankara, Turkey[J]. Environmental Pollution,2020.[90]K.P. Smith,J.E. Kirby. Image analysis and artificial intelligence in infectious disease diagnostics[J]. Clinical Microbiology and Infection,2020.[91]Alklih Mohamad YOUSEF,Ghahfarokhi Payam KAVOUSI,Marwan ALNUAIMI,Yara ALATRACH. Predictive data analytics application for enhanced oil recovery in a mature field in the Middle East[J]. Petroleum Exploration and Development Online,2020,47(2).[92]Omer F. Ahmad,Danail Stoyanov,Laurence B. Lovat. Barriers and pitfalls for artificial intelligence in gastroenterology: Ethical and regulatory issues[J]. Techniques and Innovations in Gastrointestinal Endoscopy,2020,22(2).[93]Sanne A. Hoogenboom,Ulas Bagci,Michael B. Wallace. Artificial intelligence in gastroenterology. The current state of play and the potential. How will it affect our practice and when?[J]. Techniques and Innovations in Gastrointestinal Endoscopy,2020,22(2).[94]Douglas K. Rex. Can we do resect and discard with artificial intelligence-assisted colon polyp “optical biopsy?”[J]. Techniques and Innovations in Gastrointestinal Endoscopy,2020,22(2).[95]Neal Shahidi,Michael J. Bourke. Can artificial intelligence accurately diagnose endoscopically curable gastrointestinal cancers?[J]. Techniques and Innovations in Gastrointestinal Endoscopy,2020,22(2).[96]Michael Byrne. Artificial intelligence in gastroenterology[J]. Techniques and Innovations in Gastrointestinal Endoscopy,2020,22(2).[97]Piet C. de Groen. Using artificial intelligence to improve adequacy of inspection in gastrointestinal endoscopy[J]. Techniques and Innovations in Gastrointestinal Endoscopy,2020,22(2).[98]Robin Zachariah,Andrew Ninh,William Karnes. Artificial intelligence for colon polyp detection: Why should we embrace this?[J]. Techniques and Innovations in Gastrointestinal Endoscopy,2020,22(2).[99]Alexandra T. Greenhill,Bethany R. Edmunds. A primer of artificial intelligence in medicine[J]. Techniques and Innovations in Gastrointestinal Endoscopy,2020,22(2).[100]Tomohiro Tada,Toshiaki Hirasawa,Toshiyuki Yoshio. The role for artificial intelligence in evaluation of upper GI cancer[J]. Techniques and Innovations in Gastrointestinal Endoscopy,2020,22(2).[101]Yahui Jiang,Meng Yang,Shuhao Wang,Xiangchun Li,Yan Sun. Emerging role of deep learning‐based artificial intelligence in tumor pathology[J]. Cancer Communications,2020,40(4).[102]Kristopher D. Knott,Andreas Seraphim,Joao B. Augusto,Hui Xue,Liza Chacko,Nay Aung,Steffen E. Petersen,Jackie A. Cooper,Charlotte Manisty,Anish N. Bhuva,Tushar Kotecha,Christos V. Bourantas,Rhodri H. Davies,Louise A.E. Brown,Sven Plein,Marianna Fontana,Peter Kellman,James C. Moon. The Prognostic Significance of Quantitative Myocardial Perfusion: An Artificial Intelligence–Based Approach Using Perfusion Mapping[J]. Circulation,2020,141(16).[103]Muhammad Asad,Ahmed Moustafa,Takayuki Ito. FedOpt: Towards Communication Efficiency and Privacy Preservation in Federated Learning[J]. Applied Sciences,2020,10(8).[104]Wu Wenzhi,Zhang Yan,Wang Pu,Zhang Li,Wang Guixiang,Lei Guanghui,Xiao Qiang,Cao Xiaochen,Bian Yueran,Xie Simiao,Huang Fei,Luo Na,Zhang Jingyuan,Luo Mingyan. Psychological stress of medical staffs during outbreak of COVID-19 and adjustment strategy.[J]. Journal of medical virology,2020.[105]. Eyenuk Fulfills Contract for Artificial Intelligence Grading of Retinal Images[J]. Telecomworldwire,2020.[106]Kim Tae Woo,Duhachek Adam. Artificial Intelligence and Persuasion: A Construal-Level Account.[J]. Psychological science,2020,31(4).[107]McCall Becky. COVID-19 and artificial intelligence: protecting health-care workers and curbing the spread.[J]. The Lancet. Digital health,2020,2(4).[108]Alca?iz Mariano,Chicchi Giglioli Irene A,Sirera Marian,Minissi Eleonora,Abad Luis. [Autism spectrum disorder biomarkers based on biosignals, virtual reality and artificial intelligence].[J]. Medicina,2020,80 Suppl 2.[109]Cong Lei,Feng Wanbing,Yao Zhigang,Zhou Xiaoming,Xiao Wei. Deep Learning Model as a New Trend in Computer-aided Diagnosis of Tumor Pathology for Lung Cancer.[J]. Journal of Cancer,2020,11(12).[110]Wang Fengdan,Gu Xiao,Chen Shi,Liu Yongliang,Shen Qing,Pan Hui,Shi Lei,Jin Zhengyu. Artificial intelligence system can achieve comparable results to experts for bone age assessment of Chinese children with abnormal growth and development.[J]. PeerJ,2020,8.[111]Hu Wenmo,Yang Huayu,Xu Haifeng,Mao Yilei. Radiomics based on artificial intelligence in liver diseases: where we are?[J]. Gastroenterology report,2020,8(2).[112]Batayneh Wafa,Abdulhay Enas,Alothman Mohammad. Prediction of the performance of artificial neural networks in mapping sEMG to finger joint angles via signal pre-investigation techniques.[J]. Heliyon,2020,6(4).[113]Aydin Emrah,Türkmen ?nan Utku,Namli G?zde,?ztürk ?i?dem,Esen Ay?e B,Eray Y Nur,Ero?lu Egemen,Akova Fatih. A novel and simple machine learning algorithm for preoperative diagnosis of acute appendicitis in children.[J]. Pediatric surgery international,2020.[114]Ellahham Samer. Artificial Intelligence in Diabetes Care.[J]. The Americanjournal of medicine,2020.[115]David J. Winkel,Thomas J. Weikert,Hanns-Christian Breit,Guillaume Chabin,Eli Gibson,Tobias J. Heye,Dorin Comaniciu,Daniel T. Boll. Validation of a fully automated liver segmentation algorithm using multi-scale deep reinforcement learning and comparison versus manual segmentation[J]. European Journal of Radiology,2020,126.[116]Binjie Fu,Guoshu Wang,Mingyue Wu,Wangjia Li,Yineng Zheng,Zhigang Chu,Fajin Lv. Influence of CT effective dose and convolution kernel on the detection of pulmonary nodules in different artificial intelligence software systems: A phantom study[J]. European Journal of Radiology,2020,126.[117]Georgios N. Kouziokas. A new W-SVM kernel combining PSO-neural network transformed vector and Bayesian optimized SVM in GDP forecasting[J]. Engineering Applications of Artificial Intelligence,2020,92.[118]Qingsong Ruan,Zilin Wang,Yaping Zhou,Dayong Lv. A new investor sentiment indicator ( ISI ) based on artificial intelligence: A powerful return predictor in China[J]. Economic Modelling,2020,88.[119]Mohamed Abdel-Basset,Weiping Ding,Laila Abdel-Fatah. The fusion of Internet of Intelligent Things (IoIT) in remote diagnosis of obstructive Sleep Apnea: A survey and a new model[J]. Information Fusion,2020,61.[120]Federico Caobelli. Artificial intelligence in medical imaging: Game over for radiologists?[J]. European Journal of Radiology,2020,126.以上就是关于人工智能参考文献的分享,希望对你有所帮助。
翻译研究与认知范式交叉融合的途径
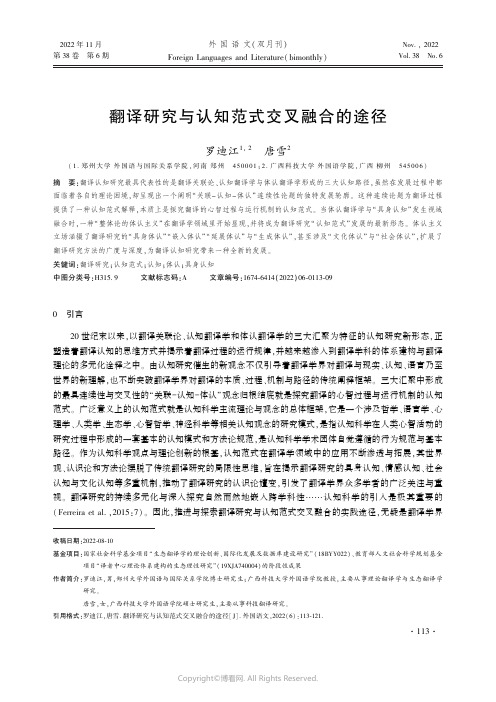
2022年11月第38卷㊀第6期外国语文(双月刊)Foreign Languages and Literature(bimonthly)Nov.,2022Vol.38㊀No.6收稿日期:2022-08-10基金项目:国家社会科学基金项目 生态翻译学的理论创新㊁国际化发展及数据库建设研究 (18BYY022)㊁教育部人文社会科学规划基金项目 译者中心理论体系建构的生态理性研究 (19XJA740004)的阶段性成果作者简介:罗迪江,男,郑州大学外国语与国际关系学院博士研究生;广西科技大学外国语学院教授,主要从事理论翻译学与生态翻译学研究㊂唐雪,女,广西科技大学外国语学院硕士研究生,主要从事科技翻译研究㊂引用格式:罗迪江,唐雪.翻译研究与认知范式交叉融合的途径[J].外国语文,2022(6):113-121.翻译研究与认知范式交叉融合的途径罗迪江1,2㊀唐雪2(1.郑州大学外国语与国际关系学院,河南郑州㊀450001;2.广西科技大学外国语学院,广西柳州㊀545006)摘㊀要:翻译认知研究最具代表性的是翻译关联论㊁认知翻译学与体认翻译学形成的三大认知路径,虽然在发展过程中都面临着各自的理论困境,却呈现出一个阐明 关联-认知-体认 连续性论题的独特发展轮廓㊂这种连续论题为翻译过程提供了一种认知范式解释,本质上是探究翻译的心智过程与运行机制的认知范式㊂当体认翻译学与 具身认知 发生视域融合时,一种 整体论的体认主义 在翻译学领域里开始显现,并将成为翻译研究 认知范式 发展的最新形态㊂体认主义立场涵摄了翻译研究的 具身体认 嵌入体认 延展体认 与 生成体认 ,甚至涉及 文化体认 与 社会体认 ,扩展了翻译研究方法的广度与深度,为翻译认知研究带来一种全新的发展㊂关键词:翻译研究;认知范式;认知;体认;具身认知中图分类号:H315.9㊀㊀㊀文献标志码:A㊀㊀㊀文章编号:1674-6414(2022)06-0113-090㊀引言20世纪末以来,以翻译关联论㊁认知翻译学和体认翻译学的三大汇聚为特征的认知研究新形态,正塑造着翻译认知的思维方式并揭示着翻译过程的运行规律,并越来越渗入到翻译学科的体系建构与翻译理论的多元化诠释之中㊂由认知研究催生的新观念不仅引导着翻译学界对翻译与现实㊁认知㊁语言乃至世界的新理解,也不断突破翻译学界对翻译的本质㊁过程㊁机制与路径的传统阐释框架㊂三大汇聚中形成的最具连续性与交叉性的 关联-认知-体认 观念归根结底就是探究翻译的心智过程与运行机制的认知范式㊂广泛意义上的认知范式就是认知科学主流理论与观念的总体框架,它是一个涉及哲学㊁语言学㊁心理学㊁人类学㊁生态学㊁心智哲学㊁神经科学等相关认知观念的研究模式,是指认知科学在人类心智活动的研究过程中形成的一套基本的认知模式和方法论规范,是认知科学学术团体自觉遵循的行为规范与基本路径㊂作为认知科学观点与理论创新的根基,认知范式在翻译学领域中的应用不断渗透与拓展,其世界观㊁认识论和方法论摆脱了传统翻译研究的局限性思维,旨在揭示翻译研究的具身认知㊁情感认知㊁社会认知与文化认知等多重机制,推动了翻译研究的认识论嬗变,引发了翻译学界众多学者的广泛关注与重视㊂翻译研究的持续多元化与深入探究自然而然地嵌入跨学科性 认知科学的引入是极其重要的(Ferreira et al.,2015:7)㊂因此,推进与探索翻译研究与认知范式交叉融合的实践途径,无疑是翻译学界㊃311㊃㊀外国语文2022年第6期㊀在探究翻译认知的过程中需要自觉担当的学术责任,也是推动翻译研究 认知范式 创建的根本要求㊂本文正是基于对翻译认知研究中发生变迁的反思,聚焦于翻译研究中最为活跃㊁最为丰富㊁涉足最深的翻译关联论㊁认知翻译学与体认翻译学三大汇聚区域,呈现一个阐明 关联-认知-体认 连续性论题的发展轮廓,同时揭示它们面对的理论困境以及解决问题的可能途径,进而提出一种整体论的体认主义立场㊂1㊀翻译认知研究的三条路径及其面临的理论困境翻译研究最初的理论构想实际上是建立一门科学化㊁学科化㊁区别于现代语言学的独立学科,这种理论构想在霍姆斯(Holmes,1972)的 翻译研究的名与实 中获得较为全面系统的阐释与说明,被普遍认为是 学科的创建宣言 (Gentzler,2001:93)㊂它确立了翻译学科谱系的三大范畴:(1)理论翻译学,建立解释和预测这些现象的普遍规则;(2)描述翻译学,描写经验世界中表现为翻译行为和翻译过程的各种现象;(3)应用翻译学,在翻译实践中,使用由第一㊁二个范畴衍生出翻译研究的信息(Toury,1995/2012:4)㊂其中,描述翻译学的过程导向研究关注的是翻译过程,涉及的是翻译主体的心智活动与认知机制,包括意识突显㊁逻辑推理㊁问题求解㊁行为感知㊁信息加工以及心理表征的综合研究,这种综合研究促使翻译研究形成一种探讨翻译过程涉及的感知㊁认知㊁推理㊁决策等心智活动的心理翻译学㊂目前,心理翻译学在翻译学领域涉及更多的是翻译认知研究,它涉及翻译关联论㊁认知翻译学与体认翻译学,侧重于翻译过程的认知研究,形成独特的 关联-认知-体认 连续性论题,总体上就是对翻译现象与翻译过程提供一种认知范式的解释与说明㊂1.1翻译关联论及其理论困境翻译认知研究的第一条路径是20世纪后期以斯波伯与威尔逊(Sperber et al.,1986)的关联理论为基础而产生的翻译关联论㊂它的基础假设是以关联为核心的心智推理理论,判定翻译行为是以认知关联为基本单元的生成与转换的明示-推理过程,因而翻译研究的范畴是心智官能,而不是纯粹的文本㊂翻译家格特(Gutt,1991/2014)将翻译视为一种寻找认知关联的交际活动㊂认知关联就成为驱动一切翻译行为的内在力量,也是翻译行为沟通现实世界的心理表征㊂格特(Gutt,1991/2014:25)用 语义表征 来指称 心理表征 ,即心智的语言模块输出㊂唯有寻找到心理表征与翻译行为之间的内在关联,才能建构起一种说明翻译的关联机制㊂就此而言,翻译研究最重要的是确定具有关联性的心理表征对翻译行为的决定性作用,而关联性是架起翻译行为与心理表征的桥梁㊂进入21世纪以来,关联理论的翻译观得到进一步深化,强调 它能以关联性抓住翻译问题的本质特征,具有翻译的本体论意义,对指导翻译实践和翻译批评也有着积极的意义 (赵彦春,2003:121)㊂随后,赵彦春(2005:97)以关联理论为基础,进一步完善关联理论的翻译观,提出翻译学归结论,将 关联 提升到本体论的地位进行界定与诠释,其基本主张包含:(1)翻译行为必须具有关联性,否则作为交际的翻译行为必然是失败的,因此 关联 是翻译的第一原则;(2)翻译是一个对源语(语内或语际)进行阐释的明示-推理过程,译者要根据交际者的意图和受体的期待进行取舍,译文的质量取决于相关因素间的趋同度㊂在明示-推理过程中,只有找到原文与译文之间的最佳关联,才能建立起一种解释与说明翻译的普遍原理㊂由此,关联才是真正架起认知世界与现实世界㊁原文与译文之间的沟通桥梁㊂纵观翻译研究的发展过程,从1972年霍姆斯翻译学科基本构想中描述翻译学的过程取向研究,到1991年格特认为管辖翻译的基本原则是关联,可以说,从过程取向到关联原则跨出了关键一步,使之成为翻译研究的认知范式的雏形㊂2005年赵彦春对关联理论与翻译研究进一步深化并建构一个全面㊁系㊃411㊃㊀罗迪江㊀唐雪㊀翻译研究与认知范式交叉融合的途径㊀统的翻译学归结论,将认知关联归结为翻译行为的本体,这是从认知视角考察翻译过程的进一步显现㊂目前,翻译关联论的最大问题就是关于认知关联的规范性问题㊂倘若假定认知关联就是遵从关联原则对心理表征进行审视,表征的内容如何确定?倘若翻译行为都是关联性的,那么规范性又如何在心理表征中获得体现呢?如何体现出心理因果律所支配的翻译行为呢?回归到翻译实践,它也忽略了翻译活动的规范性问题㊂从知识论的角度来看,翻译认知不是基于关联表征的行为,而是基于知识表征的引导而采取理性的行为㊂如此,翻译认知者需要在获得了翻译概念化知识的前提下对翻译认知系统内的信息进行概念整合,对翻译进行理性控制,才可能采取正确的行为㊂如果接受认知关联是翻译行为的根本驱动力,那么它引起的争论的问题就是:能否依赖翻译知识为翻译认知作出清晰的界限呢?这就存在着认知关联的客观性问题㊂1.2认知翻译学及其理论困境翻译认知研究的第二条路径是衍生于认知语言学理论的认知翻译学㊂从问题求解来看,认知翻译学就是为了解决翻译关联论的客观性问题产生的㊂认知翻译学作为一个正式术语率先由西班牙翻译学家穆尼兹(Muñoz,2010:169)提出,它关注的是翻译与认知的融合研究,强调认知是认识与理解翻译行为的思维方式㊂ 认知必然拥有一些东西的聚类,它们共享的基本特征是基于表征的行为㊂ (Pylyshyn,1984: xi-xii)不管是始发语的理解还是目的语的产出,都要受到心理表征的制约,可以说是认知的制约(吴义诚,2010:55)㊂因此,所谓认知翻译学,就是要解释与说明表征翻译行为的主体具备何种翻译知识㊁拥有何种翻译目的以及何种特性的翻译行为,即翻译主体拥有什么样的心理表征㊂在国内翻译学界,认知翻译学最初来源于认知语言学家王寅(2005:17)基于认知语言学的 现实-认知-语言 原则提出的翻译认知观: 翻译是一种认知活动,是以现实体验为背景的认知主体所参与的多重互动为认知基础的,译者在透彻理解源语言语篇所表达出的各类意义的基础上,尽量将其在目标语言中映射转述出来,在译文中应着力勾画出作者所欲描写的现实世界和认知世界㊂ 作为一种认知活动,翻译具有体验性㊁互动性㊁创造性㊁语篇性㊁和谐性与世界间性(现实世界与认知世界的间性)㊂一方面,翻译的体验性是翻译主体基于自身的认知㊁概念㊁意义㊁推理㊁理解和语言对客观世界的感知和经验的结果;另一方面,翻译的世界间性要求译者在翻译过程中充分考虑译出原作所欲表达的现实世界与认知世界,这就从根本上解决翻译关联论的规范性与客观性问题㊂随后,王寅(2012:17)拓展了翻译的认知观,将认知翻译学称为 认知翻译研究 ;后来,王寅(2013:52)将认知语言学中的识解机制引入翻译研究,探讨认知过程与翻译过程的内在关联,以突显㊁辖域㊁背景㊁视角与详略度为识解机制探究翻译过程,进而正式将 认知翻译学 描写为 如何在译入语中识解原作者在原作品中的原意图 ,且运用认知语言学所提出的用以解释语言表达主观性的 识解机制 ,从认知角度来简析翻译中的常见方法,以期能为翻译过程研究提供一个更为具体的新思路㊂从广泛意义上说,认知翻译学是翻译学的一种新范式,是在认知科学的框架下研究翻译理论㊁翻译实践以及翻译现象的相关问题(文旭,2018:112)㊂在认知翻译学的推动下,翻译概念由翻译关联论的 一种关联活动 转变为 一种认知活动 ,而翻译作为一种认知活动的内涵也随着研究的深入发展得到进一步的深化与拓展,例如, 翻译是一个具有原型属性的概念范畴,是一项以范畴转换为基础的认知活动 (文旭等,2020:2)㊂认知翻译学是认知语言学和翻译相结合的全新研究范式㊂ (吴淑琼等,2020:119)它将认知视为研究的本体,强调认知的交互性㊁表征性㊁抽象性等特征,把范畴化理论㊁识解理论㊁隐喻理论㊁概念整合理论等融入翻译研究之中,所涉及的识解㊁注意㊁突显㊁视角㊁图式㊁隐喻㊁转喻㊁参照点等概念成了解释与说明㊃511㊃㊀外国语文2022年第6期㊀翻译过程㊁现象与本质的认知方式,从而揭示翻译的认知机制与心智活动,拓展了翻译研究的边界与视界㊂尽管运用了认知语言学的各种概念㊁方法㊁手段来研究翻译,认知翻译学的主流进路并没有产生出公认的研究方案㊂翻译学界意识到,认知概念与翻译过程联系最为直接的问题变成相对杂乱的难题,认知与翻译的关系似乎变成了认知语言学的附属,对认知的理解和对翻译现象的说明之间的认识论鸿沟仍然难以获得真正解决,这就成了当前认知翻译学研究的理论困境㊂1.3体认翻译学及其理论困境翻译认知研究中最新的路径当属王寅(2021:43)提出具有本土化特征的体认翻译学(Embodied-Cognitive Translatology),它旨在凸显核心词 体认 在翻译研究中的本体地位,实质上就是意欲以体认为认识方式解决认知翻译学研究的理论困境㊂体认分析的出发点不应该是孤立的认知主体,而是一个包含互动体验的 体 与认知加工的 认 构成的复杂系统; 体 与 认 形成了一种认识与理解翻译的结构耦合,它们在结构耦合的驱动中彼此塑造着翻译的生成㊂体认翻译学将翻译描写为: 翻译是一种基于多重互动的体认活动,译者在透彻理解原文语篇所表达的有关现实世界和认知世界中各类意义的基础上,运用多种体认方式(如感觉知觉㊁意象图式㊁范畴化㊁概念化㊁认知模型㊁隐转喻㊁概念整合㊁识解㊁像似性等)将这些意义映射进译入语,基于创造性模仿机制将这些意义建构和转述出来㊂ (王寅,2021:46)从概念来源来看,体认翻译学来源于并又超越于认知翻译学,强调翻译的体认性,认为译文之 同 来源于 体 , 异 归因于 认 ㊂ 前者是后者的基础,没有 体 就没有 认 ;后者是前者的升华,没有 认 而只有 体 也形不成人的心智和语言,人便会无异于动物㊂ (王寅,2014:63)概而言之,体认翻译学强调翻译是一种体认活动,认为翻译之 同 皆因较为客观的 互动体验 所致,翻译之 异 则产生自较为主观的 认知加工 (王寅,2020:750)㊂以此为切入点,翻译概念就由认知翻译学的 一种认知活动 转变为体认翻译学的 一种体认活动 ,其思想内涵获得了进一步的丰富与拓展,而 体认 就是一个比 关联 认知 更具体㊁更综合㊁更普遍的描述㊂通过翻译概念的体认分析,体认翻译学认为意向性㊁目的性㊁认知㊁体认㊁感知㊁隐喻㊁概念㊁范畴化等心智范畴的属性是外在于 体 而内在于 认 的㊂面对翻译关联论与认知翻译学面临的难题,体认翻译学不仅能从 体 的视角解决翻译关联论的规范性㊁客观性问题而且能从 认 的视角去揭示翻译的创造性与能动性,更为重要的是能从多元化的理论层面上解决认知翻译学存在着附属于认知语言学的问题,以及对认知理解和对翻译现象说明之间的认识论鸿沟问题㊂从理论建构来看,无论是将认知语言学修补为体认语言学,还是将认知翻译学修补为体认翻译学,体认翻译学都离不开认知语言学,因为认知语言学是体认翻译学的根源㊂如何认识与理解认知语言学的基础性理论成为体认翻译学反思的根本性问题㊂李德凤教授指出: 认知语言学可以为 翻译过程研究 提供理论支撑 我们去研究它的翻译认知过程,可以借用认知语言学的理论,但它是众多可与 翻译过程研究 结合的理论之一,还有很多理论可以和 翻译过程研究 相结合,包括神经科学㊁认知科学㊁心理语言学的理论,甚至文化学的理论㊂ (徐然等,2020:73)进而言之,如何引入神经科学㊁认知科学㊁心理学等相关理论来探讨翻译过程与翻译认知,成为建基于认知语言学之上的体认翻译学需要考虑的关键问题㊂从认识语言学探讨翻译的心智活动与认知本质的初衷考虑,我们看到,尽管在理论建构方面将马列主义唯物论㊁语言哲学㊁体认语言学㊁体验哲学㊁后现代哲学与认知科学等理论引入翻译研究进而强调体认的本体地位,以及在实践应用方面将映射㊁创仿㊁概念整合等作为体认的具体认知方式等方面都取得很大的成就,但作为 体认 最显著特征的 具身性 等相关概念,如何呈现于体认翻译学,如何超越 只见树木不见森林 的理论话语体系,这都是目前体认翻译学面临的理论困境㊂正如王寅(2021:49)所说, 体认翻㊃611㊃㊀罗迪江㊀唐雪㊀翻译研究与认知范式交叉融合的途径㊀译学犹如一个初生婴儿,有待哺育与关怀 ㊂2㊀翻译研究的体认主义立场如果以关联理论作为翻译认知路径的起点,可以说,翻译研究的认知范式经历了认知翻译学与体认翻译学并走过30年不平凡的历程㊂30年的历程见证了翻译研究与认知范式的交叉融合:其一,揭示翻译的心智活动与认知机制;其二,说明翻译过程及其行为的体认机制㊂这两大观点的交织已经广泛地渗透到翻译研究 认知范式 的理论基础之中,这不仅超出了翻译学科内部问题域和理论解释范围,触及对认知范式做出的整体反思,而且体现为翻译研究 认知范式 的多维度建构㊂从翻译学发展的角度来看,面对日益丰富的研究主题和研究方法的多元化,翻译学界逐渐形成一种认知范式的学术共同体,真正推进翻译研究 认知范式 的整体建构并积极反馈认知范式产生的综合效应㊂这种综合效应一方面体现了 具身认知 为体认翻译学提供的思想资源,强调体认性与具身性的本质关联;另一方面预示着一种整体论的体认主义立场的出现㊂2.1具身认知为体认翻译学提供的思想资源进入21世纪后,以翻译关联论为核心的认知范式逐渐显示其理论在解释客观性与规范性方面的局限,成为翻译学界求解的问题以及推进认知范式创建需要面对的难题㊂国内外一批学者创建了以认知语言学为理论基础㊁以认知观念为理论特征的认知翻译学㊂翻译作为一种认知活动的观点,逐渐获得翻译学界的关注与认可㊂同时,翻译学界对翻译认知的理解逐步演变,范围越来越宽泛,这加剧了对认知的理解与对翻译现象的说明之间的认识论鸿沟㊂为解决目前存在的问题,体认翻译学应运而生,它将翻译视为一种体认活动,强调翻译过程的体认机制,关注翻译过程的 体 与 认 的辩证关系,以此诠释翻译之 同 与 异 ,从而揭示翻译的体认性特征㊂事实上,体认翻译学除了吸收认知语言学及衍生而来的认知翻译学外,还继承与融合了辩证唯物论㊁体验哲学与认知科学的相关思想,其理论构想就是试图以认知语言学㊁辩证唯物论㊁体验哲学与认知科学为理论基础,对翻译本质进行体认性的大一统说明,进而打通翻译学与辩证唯物论㊁语言学㊁语言哲学㊁体验哲学㊁后现代哲学㊁认知科学等理论之间的通道㊂因此,对翻译本质的体认性分析是综合性㊁整体性的㊂如果不立足于内在与体验的体认性观点,那么就无法对翻译现象作出特有的认知分析,因为从一个非体认性的立场来看,翻译不过是一系列纯粹的语言转换活动,对于这些活动没有与之相应的体认性观念 没有 体 ,也没有 认 ,因而也就没有翻译的客观性与主观性,没有理性与感性,没有必然性与或然性,没有确定性与创造性㊂没有体认性,翻译就失去了具身认知意义上的生命力㊂这就意味着,翻译的本质预示着体认性的另一种表达:具身性㊂将翻译视为一种特殊的具身性与情景性认知活动是翻译认知维度的新研究趋向(Alves et al.,2017:549)㊂根本而言,体认翻译学中的 体认性 与认知科学中的 具身性 具有内在的关联性契合㊂为了更为深入地理解翻译本质,以体认性观念为理论特征的体认翻译学需要引入具身性观念,并对狭义的具身性(涉身性)与广义的具身性进行深入的探讨,以丰富与拓展体认翻译学的理论体系及其解释力㊂刘晓力(2020:32)曾对狭义的涉身性与广义的涉身性做出了明确的区分: 狭义的涉身性观念着重强调身体对于认知具有的奠基性意义,特别是说明有机体的多模态感知运动对于认知所具有的核心地位;广义的涉身性观念是在此基础上强调阐释认知的基本单元应当是大脑-身体-环境的耦合体,其理论目标是说明认知主体如何参与不同情境并以何种特殊方式与环境进行积极主动的交互㊂ 对于体认翻译学来说,探究体认性就意味着离不开对具身性的研究,对具身性的研究意味着离不开对 具身认知㊁嵌入认知㊁延展认㊃711㊃㊀外国语文2022年第6期㊀知与生成认知 (Gallagher,2005:17)进行探讨㊂目前,具身性观念正引起翻译学界的极大关注,渗透并扩张于当代翻译研究之中,逐渐形成了独具一格的具身认知范式㊂仲伟合等(2015:70)认为, 具身认知强调人类认知的具身性与情境(适应)性以及完型心理之特征,那么具身性翻译认知研究之思维强调译者认知心理的具身性㊁系统层次性与系统融合性特征 ;穆尼兹(Muñoz,2017:560)指出: 认知翻译研究的趋向是计算翻译学与认知翻译学,前者关注认知主义与信息处理方法,后者侧重具身认知㊁嵌入认知㊁延展认知㊁生成认知㊂ 就翻译研究而言,不论是嵌入认知还是延展认知,抑或是生成认知,它们实际上都是衍生于具身性观念对翻译认知不同方面的具体说明㊂如果建立在认知范式的基础上,翻译关联论㊁认知翻译学与体认翻译学都有自身内在的理论困境㊂摆脱这种困境,一方面需要引入具身性观念,将具身认知㊁嵌入认知㊁延展认知与生成认知的新观念融入翻译研究之中,形成更具包容性与开放性的体认性观念;另一方面需要强调 体 与 认 的辩证关系,倡导翻译之 同 与翻译之 异 的视域融合,借鉴广义具身认知提供的思想资源对翻译本质进行更深层次的理解:其一,翻译不仅仅是一种认知活动,更是一种体认活动,而且是分布在整个现实-认知-语言之中的生成过程;其二,翻译不是预先给定的,而是通过翻译体认㊁翻译行为与翻译环境互动而动态建构的;其三,对翻译过程的理解必须重视具身认知对翻译行为产生的影响,不能单纯将其视为一种简单的语言转换活动;其四,复杂的翻译认知功能不仅奠基于感觉㊁知觉㊁识解㊁推理㊁概念化等之间的耦合作用,还依赖于翻译主体的意向性㊁情感㊁自主性与主体间性多方面的协调㊂2.2体认主义立场的显现当体认翻译学与具身认知发生视域融合时,一个更清晰㊁更有力的结果是:体认翻译学需要建构自身内在的体认性观点,它涉及的是具身体认㊁嵌入体认㊁延展体认与生成体认,进而将它们概括为广义体认性研究论域的核心内容㊂换而言之,体认性在翻译研究中成为一种包容性的㊁独特的翻译概念,并预示着一种新的体认主义立场的出现,而具身体认㊁嵌入体认㊁延展体认与生成体认会在翻译研究的体认主义立场中将获得进一步深入的诠释与说明㊂与仅仅关注关联性的翻译关联论与只关注心智活动的认知翻译学的解释不同,这种体认主义更推崇辩证唯物论与认知科学共质下 体 (互动体验)与 认 (认知加工)的辩证关系,以 体 诠释翻译之 同 (模仿性),以 认 来诠释翻译之 异 (创造性),认为只有将 体 与 认 的 连续性整合运作 才能真正揭示翻译过程与翻译认知的体认性本质㊂体认主义立场的出现使得 体 与 认 这个现象在翻译研究中变得异常醒目,这也是翻译主体在翻译过程中始终面临的最大张力与底线;为了揭示翻译的本质,翻译主体必须从 体 与 认 的双重逻辑中 以身作则 为翻译 操心 ,翻译就是一项充满具身性的 操心 行为㊂因此,翻译主体就是一种具身主体,而翻译是一种以体认为导向的具身行为㊂一方面,体认依赖于翻译经验的,这种翻译经验来自身体对翻译的体验;另一方面,翻译所进行的各种体验内含在一个更广泛的心理㊁文化和社会的语境中㊂也就是说,翻译的一切秘密必须到具身认知的体认性中去寻找,翻译本质上是一个具身的动态系统,它不仅是一个体认过程,而且是一个具身认知的建构过程㊂依此而言,认知翻译学的认知性观念不足以使人们充分理解翻译的本质,还需要引入体认语言学中的 体 以突显 现实-认知-语言 的互动体验观,考虑翻译主体的身体过程㊁情感过程以及对与此相关的现实世界之间的体认方式㊂这就必须借鉴体验哲学与认知科学的思想,建立一种更具包容性的体认主义整合方案㊂这种方案将体认源于心智㊁身体与现实世界交互作用的生成过程,将体认主体的翻译活动作为塑造我们的翻译系统以及我们体认这个世界方式的过程,翻译认知就是翻译主体参与主体间的㊁语言的㊁社会的和文化的体认过程㊂可见,理解翻译既需要研究㊃811㊃。
基于Citespace知识图谱分析国际城市生物多样性研究

第41卷第6期生态科学41(6): 211–221 2022年11月Ecological Science Nov. 2022 张思凝,吴然. 基于Citespace知识图谱分析国际城市生物多样性研究[J]. 生态科学, 2022, 41(6): 211–221.ZHANG Sining, WU Ran. Knowledge mapping analysis of urban biodiversity research based on Citespace[J]. Ecological Science, 2022, 41(6): 211–221.基于Citespace知识图谱分析国际城市生物多样性研究张思凝*,吴然西南交通大学建筑学院,成都 611756【摘要】生物多样性保护一直是城市生态学与风景园林学的重要议题。
以Web of Science核心数据库收录的近二十几年(1999—2020)的城市生物多样性文献为研究对象,利用文献计量分析与可视化知识图谱分析方法,将检索并剔除重复后的770篇文献导入Citespace 5.7.R2中。
进一步分析了其时间与期刊分布,学科与研究方向分布,及国家与机构合作分布等基本特征;进行了外文文献中城市生物多样性的文献共被引分析与共现知识图谱分析;并利用关键词共现分析了其研究热点;突现词突发性探测算法分析了其研究前沿,包括四大方向:城市化对生物同质与物种丰富度的影响;城市绿地管理与生物多样性保护;城市生物多样性保护的关键因素;提高公众的保护意识与参与性。
以期为相关科研工作者与我国城市生物多样性相关研究提供参考与借鉴。
关键词:Citespace;城市生物多样性;知识图谱;文献计量分析doi:10.14108/ki.1008-8873.2022.06.025 中图分类号:TU986 文献标识码:A 文章编号:1008-8873(2022)06-211-11Knowledge mapping analysis of urban biodiversity research based on Citespace ZHANG Sining*, WU RanSchool of Architecture, Southwest Jiaotong University, Chengdu 611756, China.Abstract: Biodiversity conservation has always been an important topic in urban ecology and landscape architecture. In this paper, the urban biodiversity literature, from 1999 to 2020, were collected in the core database of Web of Science. 770 publications were retrieved and analyzed after eliminating the duplicates by using the bibliometric analysis and visual knowledge map analysis in CiteSpace 5.7.R2. Further analysis of its basic characteristics was done, such as the distribution by year and journals, the development of disciplines and research areas, and countries and institutions distribution. Besides, the co-citation analysis and co-occurrence knowledge map analysis of urban biodiversity in the English literature was carried out. Keywords co-occurrence analysis reveals the research hot spots. The burst detection algorithm tells the research frontiers, including four areas: the impact of urbanization on biological homogeneity and species richness; urban green space management and biodiversity conservation; key factors for urban biodiversity conservation; the enhancement of public awareness and participation in biodiversity protection. This paper aims to provide a reference for related researchers and research on urban biodiversity in China.Key words: Citespace; urban biodiversity; knowledge mapping; bibliometric analysis收稿日期: 2021-07-14; 修订日期: 2021-09-18基金项目:国家自然科学基金项目(52008345); 中央高校基本科研业务费专项基金项目(2682020CX43)作者简介:张思凝(1989—), 女, 四川成都人, 博士, 助理研究员, 主要从事风景园林规划与设计研究*通信作者: 张思凝212 生态科学41卷0 前言1992年,联合国环境与发展大会颁布了《联合国生物多样性公约》。
欧盟《减排分担条例》NDCs背景下气候目标主义立法规制
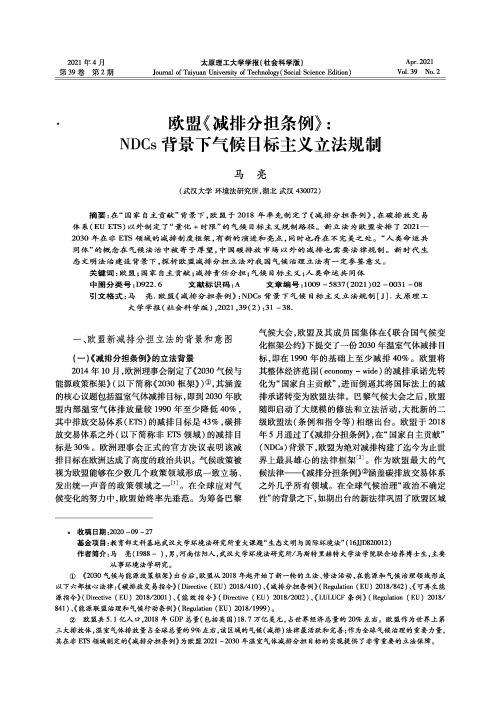
2021年4月第39卷第2期Apr.2021Vol.39No.2太原理工大学学报(社会科学版)Journal of Taiyuan University of Technology(Social Science Edition)欧盟《减排分担条例》:NDCs背景下气候目标主义立法规制马亮(武汉大学环境法研究所,湖北武汉430072)摘要:在“国家自主贡献”背景下,欧盟于2018年率先制定了《减排分担条例》,在碳排放交易体系(EU ETS)以外制定了“量化+时限”的气候目标主义规制路径。
新立法为欧盟安排了2021—2030年在非ETS领域的减排制度框架,有新的演进和亮点,同时也存在不完美之处。
“人类命运共同体”的概念在气候法治中被寄予厚望,中国碳排放市场以外的减排也需要法律规制。
新时代生态文明法治建设背景下,探析欧盟减排分担立法对我国气候治理立法有一定参鉴意义。
关键词:欧盟;国家自主贡献;减排责任分担;气候目标主义;人类命运共同体中图分类号:D922.6文献标识码:A文章编号:1009-5837(2021)02-0031-08引文格式:马亮.欧盟《减排分担条例》:NDCs背景下气候目标主义立法规制[J].太原理工大学学报(社会科学版),2021,39(2):31-38.一、欧盟新减排分担立法的背景和意图(-)《减排分担条例》的立法背景2014年10月,欧洲理事会制定了《2030气候与能源政策框架》(以下简称《2030框架》)①,其涵盖的核心议题包括温室气体减排目标,即到2030年欧盟内部温室气体排放量较1990年至少降低40%,其中排放交易体系(ETS)的减排目标是43%,碳排放交易体系之外(以下简称非ETS领域)的减排目标是30%。
欧洲理事会正式的官方决议表明该减排目标在欧洲达成了高度的政治共识。
气候政策被视为欧盟能够在少数几个政策领域形成一致立场、发出统一声音的政策领域之一⑴。
在全球应对气候变化的努力中,欧盟始终率先垂范。
- 1、下载文档前请自行甄别文档内容的完整性,平台不提供额外的编辑、内容补充、找答案等附加服务。
- 2、"仅部分预览"的文档,不可在线预览部分如存在完整性等问题,可反馈申请退款(可完整预览的文档不适用该条件!)。
- 3、如文档侵犯您的权益,请联系客服反馈,我们会尽快为您处理(人工客服工作时间:9:00-18:30)。
TRUST AND EPISTEMIC COMMUNITIES IN BIODIVERSITY DATA SHARING Nancy A. Van House School of Information Management and Systems University of California Berkeley, CA 94710-4600 USA +1 510 642 0855 vanhouse@sims.berkeley.edu
ABSTRACT Trust is a key element of knowledge work: what we know depends largely on others. This paper discusses the concepts of communities of practice and epistemic cultures, and their implication for design of digital libraries that support data sharing, with particular reference to practices of trust and credibility. It uses an empirical study of a biodiversity digital library of data from a variety of sources to illustrate implications digital library design and operation. It concludes that diversity and uncomfortable boundary areas typify, not only digital library user groups, but the design and operation of digital libraries.
Categories and Subject Descriptors H3.7 [Information Storage and Retrieval]: Digital libraries -- User issues.
General Terms Management, Design, Human Factors.
Keywords Biodiversity; trust; credibility; communities of practice; epistemic cultures; users.
1. INTRODUCTION Knowledge is a collective good. We rely on others. ....The relations in which we have and hold knowledge has a moral character, and the word I use to indicate that more relation is trust... I argue that the identification of trustworthy agents is necessary to the constitution of any body of knowledge…[W]hat we know of comets, icebergs, and neutrinos irreducibly contains what we know about those people who speak for and about those things, just as what we know about the virtues of people
is informed by their speech about things that exist in the world ([39]; italics in original).
In all knowledge work, we rely on others – present and distant, known and unknown. Digital libraries have the capacity to radically alter practices of collaboration and knowledge work by making it possible to use, not only published work, but unpublished work from a variety of sources. Libraries, including digital libraries, that contain published information participate in the institutional structure of the publishing system that vets and warrants knowledge. Networked information, however, can cross the social and technical boundaries between published and unpublished, private and public, and local and global that used to, practically if not ideologically, limit our possibilities for sharing information knowledge work.
This increased access highlights questions of trust: Whom or what do we believe? How do we decide? And how do we design digital libraries to facilitate these judgments?
The social nature of information and of knowledge work and its implications for the design of computer-based systems are of concern in a number of research areas, including computer-supported collaborative work (CSCW), recommendation and collaborative filtering systems [31], and social navigation [13]. A growing literature investigates the assessment of Web-based resources (e.g., [1, 4, 7, 8, 35]. A new book [2] presents an array of socially-informed investigations of digital library use.
Most of these investigations are rooted in empirical observations of people’s behavior or in system design projects, sometimes with reference to the literature of social psychology and organizational behavior. This paper, in contrast, draws on social epistemology ([10, 14, 52]) and science studies [17], two areas with long histories of investigating the social nature of knowledge. My contention is that their insights could fruitfully inform systems design. The dialogue could potentially go both ways. Digital libraries can make visible previously taken-for-granted practices of knowledge work, questioning and clarifying the understandings of social epistemology.
In this paper, I draw on the literature of social epistemology, situated learning, and science studies to better understand the practices of trust in knowledge work, the role of community in assessments of credibility, and design decisions in a digital library of biodiversity data. The purpose is not to present an ideal solution to problems of assessing credibility of sources, but to examine the practices by which people address this problem on a day-to-day basis by looking at how a specific digital library (DL)
Permission to make digital or hard copies of all or part of this work for personal or classroom use is granted without fee provided that copies are not made or distributed for profit or commercial advantage and that copies bear this notice and the full citation on the first page. To copy otherwise, or republish, to post on servers or to redistribute to lists, requires prior specific permission and/or a fee.