3D Body Reconstruction from Photos Based on Range Scan
人脸识别中的姿态问题研究
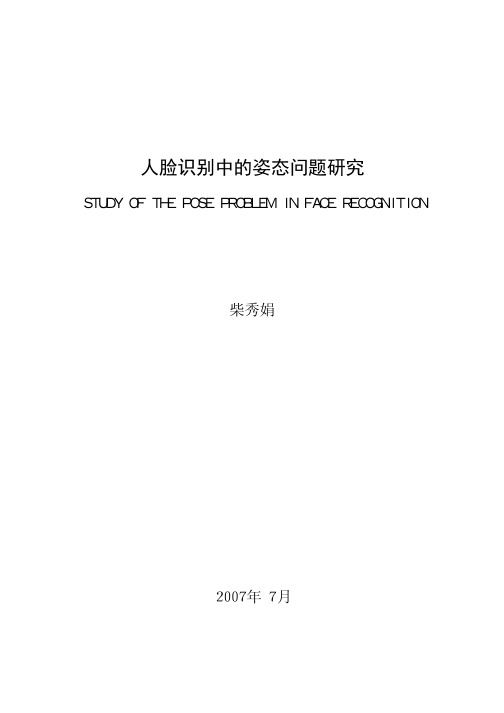
哈尔滨工业大学工学博士学位论文
果更加精确。 (3) 提出了一种基于局部线性回归(LLR)的姿态校正方法。通过将姿态校正
形式化为从非正面人脸估计其虚拟正面视图的预测问题,本文提出了一种基于 线性回归的正面视图预测方法,该方法可以在仅标定输入非正面人脸双眼中心 位置的条件下,通过线性回归算法预测出其虚拟正面视图。考虑到非正面人脸 视图与正面人脸视图之间的映射对于不同人而言实际上并不相同,我们进一步 改进上述算法,提出了一种基于分块模式的姿态校正方法,即所谓的局部线性 回归(LLR)姿态校正方法。该方法基于 3D 人脸结构的先验知识,将人脸区域分 成若干小的面片,在小的面片上进行上述线性回归操作以获得更为精确的预测 结果。实验结果表明,无论从视图校正的视觉效果,还是从人脸识别的精度来 看,此方法都具有很好的性能。
(4) 提出了一种基于 3D 稀疏变形模型(3D SDM)的 3D 人脸重建及姿态校正 方法。本方法利用人脸类的 3D 形状先验知识,根据单幅任意姿态下的人脸图像, 重构其特定的 3D 形状信息。在 3D 人脸重构的基础上,通过图形绘制的方法得 到正面姿态下的人脸视图,用于解决人脸识别中的姿态问题。该方法假设人脸 的 3D 形状分布为高斯分布,将所有人脸的 3D 形状分布空间用主成分分析(PCA) 模型表示。按照预先定义的 2D 人脸图像上的关键特征点,从稠密的 PCA 模型 中得到稀疏的版本,即稀疏变形模型。基于此,在输入图像 2D 特征点的驱动下, 恢复得到该特定人的 3D 形状,进而重构其 3D 人脸,实现姿态校正及任意姿态 下的虚拟视图生成。实验表明:通过 3D 人脸重建得到的虚拟视图结果,从视觉 上来看更加接近于真实人脸图像。同时,以 Gabor PCA+LDA 方法作为识别策 略,在 CMU PIE 数据库中 45 度以内的图像上测试,将姿态校正后的视图作为 输入,平均识别率达到了 97.5%左右,极大的改善了识别系统对于人脸姿态图 像的适应能力。
SingleImage3DReconstruction
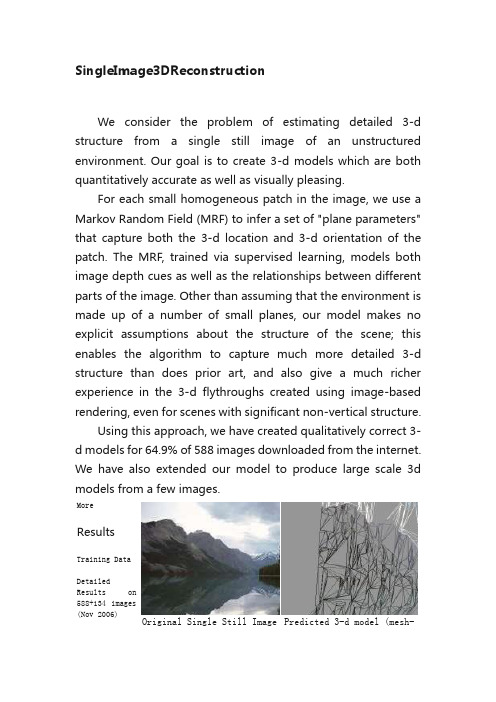
SingleImage3DReconstructionWe consider the problem of estimating detailed 3-d structure from a single still image of an unstructured environment. Our goal is to create 3-d models which are both quantitatively accurate as well as visually pleasing.For each small homogeneous patch in the image, we use a Markov Random Field (MRF) to infer a set of "plane parameters" that capture both the 3-d location and 3-d orientation of the patch. The MRF, trained via supervised learning, models both image depth cues as well as the relationships between different parts of the image. Other than assuming that the environment is made up of a number of small planes, our model makes no explicit assumptions about the structure of the scene; this enables the algorithm to capture much more detailed 3-d structure than does prior art, and also give a much richer experience in the 3-d flythroughs created using image-based rendering, even for scenes with significant non-vertical structure.Using this approach, we have created qualitatively correct 3-d models for 64.9% of 588 images downloaded from the internet. We have also extended our model to produce large scale 3d models from a few images.MoreResultsTraining DataDetailedResults on588+134 images(Nov 2006)Original Single Still Image Predicted 3-d model (mesh-Multi-view results (Jun 2007)Make 3D model from your imagehttp://make3d. (In two simple steps: upload and browse-in-3d !)Publicatio nsLearning 3-D SceneStructure from a Single Still Image,Ashutosh Saxena, Min Sun, Andrew Y. Ng, In ICCV workshop on 3D Representation for Recognition (3dRR-07), 2007. (best paper) [ps, pdf, ppt]3-D Reconstruction from Sparse Views using Monocular Vision,Ashutosh Saxena, Minview).Snapshot of the predicted 3-d flythrough.3-d flythrough (requiresshockwave).Sun, Andrew Y. Ng, In ICCV workshop on Virtual Representation s and Modeling of Large-scale environments (VRML), 2007. [ps, pdf]Also see related publications:Learning depth from single monocular images,Ashutosh Saxena, Sung H. Chung, Andrew Y. Ng. In NIPS 18, 2005.3-D Depth Reconstruction from a Single Still Image,Ashutosh Saxena, Sung H. Chung, Andrew Y. Ng. IJCV, Aug 2007.LinksPeople: Ashutosh Saxena, Min Sun, Andrew Y. NgReconstruction 3d group Wiki Monocular Depth Estimation Improving Stereo-vision Autonomous driving using monocular visionIndoor single image 3-d reconstruction (More)Outdoor single image "popups"。
3D人体姿态数据集TNT15

3D人体姿态数据集TNT15目前,已知的 3D 人体姿态的公开数据集有 Human3.6M、HumanEva、MPII、COCO等,这些数据集由 RBG 图像和人体关节点的空间坐标组成。
根据拍摄人数的不同,数据集可分为单人数据集和多人数据集;根据图像是否连续,数据集可分为单帧图像数据集和连续帧视频数据集。
单人连续帧视频数据集的制作,基本上均依靠动捕设备来捕捉真实人体关节点的空间坐标,同时也有数据集消耗大量人力进行手工标注,以下对几种公开数据集进行简要介绍。
(1)Human3.6M 数据集:简称 H36M 数据集,是目前 3D 人体姿态估计领域中数据集样本数量最多、使用最为广泛的数据集。
H36M 数据集共包含约 360 万张图像,共 11 个演员进行数据集录制,其中只有 S1、S5、S6、S7、S8、S9、S11 这 7 个人带有 3D 人体姿态标签。
H36M 数据集中,分别从 4 个角度对演员进行拍摄录制,视频录制帧数为 50Hz,每位演员表演 17 种日常动作,例如:抽烟、照相、打招呼等。
在3D 人体姿态估计领域的研究中,学者们通常会将S1、S5、S6、S7、S8 这 5 个人的动作样本作为训练集,并将 S9、S11 两人的动作样本作为测试集对算法性能进行评测。
在使用 H36M 数据集时需要注意的是,部分视频的帧数与标注的 3D 人体关节点标签帧数不一致,需要对视频末尾帧数进行剪切后与 3D 人体关节点标签一一对应。
(2)HumanEva 数据集:该数据集由动作捕捉设备制作而成,数据集共录制 4 个演员的 6 种日常动作,例如行走、慢跑、拳击、打招呼等,该数据集共包含 20610 张标注图像,学者们通常会将 S1、S2、S3 这 3 个人的动作作为训练集,共 10041 张图像,剩下的样本则作为测试集,共包含 10569 张图像。
基于图像的大规模三维重建
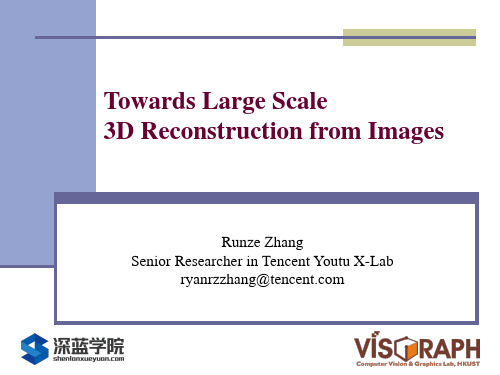
Publication: Z. Luo, T. Shen, L. Zhou, S. Zhu, R. Zhang (Corresponding author), Y. Yao, T. Fang, and L. Quan, “GeoDesc: Learning Local Descriptors by Integrating Geometry Constraints,” in ECCV 2018
Relative Poses
Towards Large Scale 3D Reconstruction from Images
Camera Registration
Register all cameras into a global system Incremental methods
Select next view by the number of points (Snavely et al., 2006)
Overview
Introduction Large scale 3D reconstruction pipeline Large scale Structure-from-Motion Large scale Multiple View Stereo Conclusion
Towards Large Scale 3D Reconstruction from Images
unity3D学习之视锥体
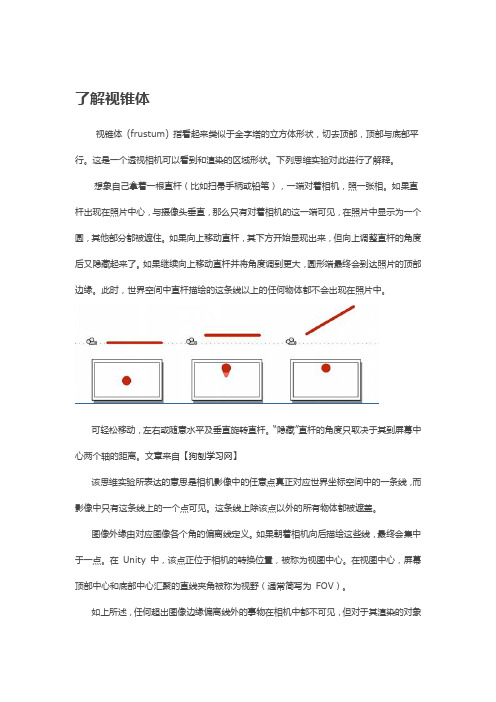
了解视锥体视锥体(frustum) 指看起来类似于金字塔的立方体形状,切去顶部,顶部与底部平行。
这是一个透视相机可以看到和渲染的区域形状。
下列思维实验对此进行了解释。
想象自己拿着一根直杆(比如扫帚手柄或铅笔),一端对着相机,照一张相。
如果直杆出现在照片中心,与摄像头垂直,那么只有对着相机的这一端可见,在照片中显示为一个圆,其他部分都被遮住。
如果向上移动直杆,其下方开始显现出来,但向上调整直杆的角度后又隐藏起来了。
如果继续向上移动直杆并将角度调到更大,圆形端最终会到达照片的顶部边缘。
此时,世界空间中直杆描绘的这条线以上的任何物体都不会出现在照片中。
可轻松移动,左右或随意水平及垂直旋转直杆。
“隐藏”直杆的角度只取决于其到屏幕中心两个轴的距离。
文章来自【狗刨学习网】该思维实验所表达的意思是相机影像中的任意点真正对应世界坐标空间中的一条线,而影像中只有这条线上的一个点可见。
这条线上除该点以外的所有物体都被遮盖。
图像外缘由对应图像各个角的偏离线定义。
如果朝着相机向后描绘这些线,最终会集中于一点。
在Unity 中,该点正位于相机的转换位置,被称为视图中心。
在视图中心,屏幕顶部中心和底部中心汇聚的直线夹角被称为视野(通常简写为FOV)。
如上所述,任何超出图像边缘偏离线外的事物在相机中都不可见,但对于其渲染的对象也有两个其他限制条件。
近裁剪面和远裁剪面与相机的XY 平面平行,都与中心线有一定距离。
比近裁剪面更靠近相机的任何物体和比远裁剪面更远的物体都不会被渲染。
图像偏离线夹角与两个裁剪面定义一个截棱锥,即视锥体。
纹理物体缺陷的视觉检测算法研究--优秀毕业论文
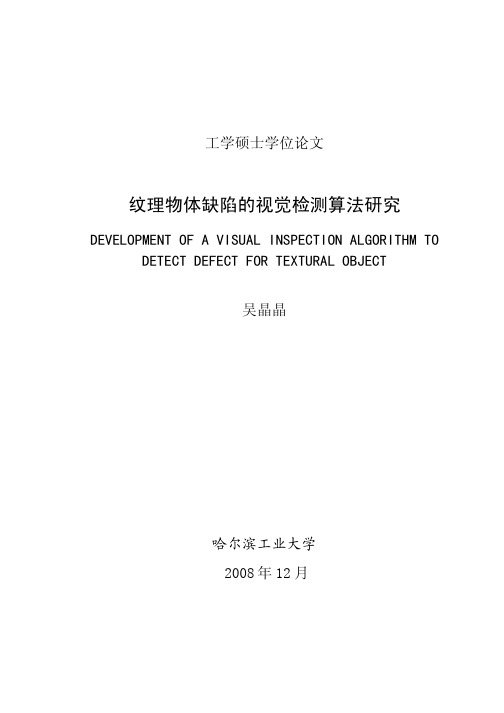
摘 要
在竞争激烈的工业自动化生产过程中,机器视觉对产品质量的把关起着举足 轻重的作用,机器视觉在缺陷检测技术方面的应用也逐渐普遍起来。与常规的检 测技术相比,自动化的视觉检测系统更加经济、快捷、高效与 安全。纹理物体在 工业生产中广泛存在,像用于半导体装配和封装底板和发光二极管,现代 化电子 系统中的印制电路板,以及纺织行业中的布匹和织物等都可认为是含有纹理特征 的物体。本论文主要致力于纹理物体的缺陷检测技术研究,为纹理物体的自动化 检测提供高效而可靠的检测算法。 纹理是描述图像内容的重要特征,纹理分析也已经被成功的应用与纹理分割 和纹理分类当中。本研究提出了一种基于纹理分析技术和参考比较方式的缺陷检 测算法。这种算法能容忍物体变形引起的图像配准误差,对纹理的影响也具有鲁 棒性。本算法旨在为检测出的缺陷区域提供丰富而重要的物理意义,如缺陷区域 的大小、形状、亮度对比度及空间分布等。同时,在参考图像可行的情况下,本 算法可用于同质纹理物体和非同质纹理物体的检测,对非纹理物体 的检测也可取 得不错的效果。 在整个检测过程中,我们采用了可调控金字塔的纹理分析和重构技术。与传 统的小波纹理分析技术不同,我们在小波域中加入处理物体变形和纹理影响的容 忍度控制算法,来实现容忍物体变形和对纹理影响鲁棒的目的。最后可调控金字 塔的重构保证了缺陷区域物理意义恢复的准确性。实验阶段,我们检测了一系列 具有实际应用价值的图像。实验结果表明 本文提出的纹理物体缺陷检测算法具有 高效性和易于实现性。 关键字: 缺陷检测;纹理;物体变形;可调控金字塔;重构
Keywords: defect detection, texture, object distortion, steerable pyramid, reconstruction
II
颠覆传统建模!3D阿尔茨海默病体外模型诞生了!可巧妙模拟人脑,为痴呆治疗带来重大进步

颠覆传统建模!3D阿尔茨海默病体外模型诞生了!可巧妙模拟人脑,为痴呆治疗带来重大进步原创2018-06-28 订阅号APExBIO阿尔茨海默病(Alzheimer’s disease,简称AD) 是一种神经系统退行性疾病,临床上的表现特征为痴呆,主要表现为渐进性记忆障碍、认知功能障碍、人格改变及语言障碍等神经精神症状,以65岁为界分为早发性和晚发性。
阿尔茨海默病(AD)的特征在于β-淀粉样蛋白(beta-amyloid,Aβ)的积累,磷酸化tau的形成,神经胶质细胞的超活化和神经元的丢失。
然而人们对AD的病因及发病机制知之甚少,很大程度上是因为缺乏一种理想的AD模型。
对于体外模型,理想的情况是能够重演AD病理过程的三大点:Aβ的累积,磷酸化tau的聚集和神经炎性,即重演AD患者大脑中多级细胞间的相互作用。
然而,目前的AD神经元模型不包括由小神经胶质细胞介导的神经炎症变化。
近日,来自美国北卡罗来纳大学夏洛特分校的Park等人建立了一个3D的AD体外模型,这是一个微流体装置,里面装载了含人类神经元(neurons)、星形胶质细胞(astrocytes)和小胶质细胞(microglia)的3D培养物。
3D微流体模型呈现出与生理相关的大脑环境,可以重演AD的病理过程,揭示了神经退行性病变的潜在重要炎症机制。
该论文题目为“A 3D human triculture system modeling neurodegeneration and neu roinflammation in Alzheimer’s disease”,在线发表于《nature Neuroscience》杂志。
这个三重培养装置看起来像一个“圆盆”(见下图),神经元和星形胶质细胞在3D凝胶状培养物中生长,来模拟一个“微型大脑”。
淀粉样前体蛋白(amyloid precursor protein, APP)的突变会导致AD。
在这里,瑞典突变(K670/M671L)和伦敦突变(V717I)APP通过慢病毒感染在神经元和星形胶质细胞中表达,导致β-淀粉样蛋白(Aβ)的累积和tau的磷酸化——这是AD的两个病理标志。
利用单目图像重建人体三维模型

算倣语咅信is与电ifiChina Computer&Communication2021年第5期利用单目图像重建人体三维模型钱融王勇王瑛(广东工业大学计算机学院,广东广州510006)摘要:人体三维模型在科幻电影、网上购物的模拟试衣等方面有广泛的应用场景,但是在单目图像重建中存在三维信息缺失、重建模型不具有贴合的三维表面等问题-为了解决上述的问题,笔者提出基于SMPL模型的人体三维模型重建算法。
该算法先预估人物的二维关节点,使用SMPL模型关节与预估的二维关节相匹配,最后利用人体三维模型数据库的姿势信息对重建的人体模型进行姿势先验,使得重建模型具有合理的姿态与形状.实验结果表明,该算法能有效预估人体关节的三维位置,且能重建与图像人物姿势、形态相似的人体三维模型.关键词:人体姿势估计;三维人体重建;单目图像重建;人体形状姿势;SMPL模型中图分类号:TP391.41文献标识码:A文章编号:1003-9767(2021)05-060-05Reconstruction of a Three-dimensional Human Body Model Using Monocular ImagesQIAN Rong,WANG Yong,WANG Ying(School of Computer,Guangdong University of Technology,Guangzhou Guangdong510006,China) Abstract:The human body3D model are widely used in science fiction movies,online shopping simulation fittings,etc,but there is a lack of3D information in monocular image reconstruction,and the reconstructed model does not have problems such as a fit 3D surface.In order to solve the above mentioned problems,a human body3D model reconstruction algorithm based on SMPL model is proposed.The algorithm first estimates the two-dimensional joint points of the character,and uses the SMPL model joints to match the estimated two-dimensional joints;finally,the posture information of the three-dimensional human body model database is used to perform posture prior to the reconstructed human body model,making the reconstructed model reasonable Posture and shape.The algorithm was tested on the PI-INF-3DHP data set.The experimental results show that the algorithm can effectively predict the3D position of human joints,and can reconstruct a3D model of the human body similar to the pose and shape of the image.Keywords:human pose estimation;3D human reconstruction;monocular image reconstruction;human shape and pose;SMPL0引言人体三维模型所承载的信息量远远大于人体二维图像,能满足高层的视觉任务需求,例如在网购中提供线上试衣体验,为科幻电影提供大量的人体三维数据。
- 1、下载文档前请自行甄别文档内容的完整性,平台不提供额外的编辑、内容补充、找答案等附加服务。
- 2、"仅部分预览"的文档,不可在线预览部分如存在完整性等问题,可反馈申请退款(可完整预览的文档不适用该条件!)。
- 3、如文档侵犯您的权益,请联系客服反馈,我们会尽快为您处理(人工客服工作时间:9:00-18:30)。
3D Body Reconstruction from Photos Based onRange ScanHyewon Seo1,Young In Yeo2and Kwangyun Wohn21CGAL,Chungnam National University220Gung-dong,Yuseong-gu,Daejeon,Koreahseo@cnu.ac.kru.ac.kr/∼hseo2VRLab.,KAIST373-1Guseong-dong,Yuseong-gu Daejeon,Koreayyiguy,wohn@vr.kaist.ac.krhttp://vr.kaist.ac.kr/Abstract.We present a data-driven shape model for reconstructing hu-man body models from one or more2D photos.One of the key tasks inreconstructing the3D model from image data is shape recovery,a taskdone until now in utterly geometric way,in the domain of human bodymodeling.In contrast,we adopt a data-driven,parameterized deformablemodel that is acquired from a collection of range scans of real humanbody.The key idea is to complement the image-based reconstructionmethod by leveraging the quality shape and statistic information accu-mulated from multiple shapes of range-scanned people.In the presenceof ambiguity either from the noise or missing views,our technique hasa bias towards representing as much as possible the previously acquired‘knowledge’on the shape geometry.Texture coordinates are then gen-erated by projecting the modified deformable model onto the front andback images.Our technique has shown to reconstruct successfully humanbody models from minimum number images,even from a single imageinput.1IntroductionOne of the oldest goals in computer graphics has been the problem of reconstruct-ing human body to convincingly depict people in digital worlds.Indeed,digitally modeling human bodies from measurement is being actively and successfully addressed by image-based and hybrid techniques.During its formative years, researchers have focused on developing methods for modeling appearance and movements of real people observed from2D photos or video sequences[6][7][9]. These efforts use silhouette information from multi-view images for determining the shape and optionally the texture of the model to be reconstructed.To sim-plify the problem of general reconstruction,a template or generic model of the class to be modeled–human body–isfit to observations of a particular subject.Today,whole body range scanners are becoming more and more available and hence much of the focus of graphics research has been shifted to the acquisitionof human body models from3D range scans[2][10].The measurements acquired from such scanning devices provide rich set of shape information which otherwise requires considerable amount of time and effort by experienced CG software users.Range scanners however remain by far more expensive,difficult to use, and provide limited accessibility,compared to2D imaging devices.Moreover, many whole body scanners today provide only geometry data without color or texture[5][13][14].We note that combining2D images and the range scanned measurement can lead to successful reconstruction results.The quality shape and collective knowledge from scanned dataset can efficiently be used to complement the shape recovery from image inputs.In the domain of human face reconstruction,for example,one of the most impressive3D reconstructions of human faces was presented by Blanz and Vetter[3].They described a face modeler in which a prior knowledge collected from a set of head scan data is exploited tofind the optimizing surface and texture parameters that bestfit the given image data. While their method suggest a powerful approach to the image-based human modeling,it has not been applied to model human body.Indeed,it would be difficult to extend that method to modeling of entire human body,due to large-scale occlusions and articulations.In this paper,we propose a system for reconstructing an entire human body model from minimum number of multi-view images,exploiting the quality shape captured by range scans.Our specific goal is an on-line clothing store[4]where users can try on garment items on their3D avatar models,hence we limit our focus to the reconstruction of lightly clothed subjects.The distinguishing aspect of our modeler in comparison to existing image-based body modeler is that it employs a data-driven shape model that has been constructed from range scans of real bodies.Subsequently,we exploit the quality shape as well as statistical information collected from a database of laser-scanned bodies,in the presence of ambiguity,noise,or even underconstraints caused by missing views.1.1Related workWhile image-based model reconstruction has been at the center of digital human modeling across several research groups,the majority of research progress in this avenue falls into the category of facial modeling.This is perhaps primarily due to the complex articulated structure and high degree of self-occlusion exhibited in our bodies.One approach that has been extensively investigated is model-based tech-niques.Hilton et al[6]and Lee et al[7]have gathered silhouette observations from multiview images such that they are used to transform a template humanoid model.Affine transformation has been followed by geometric deformation of the prior surface model.They use feature point locations along the silhouette tofind the correspondence among different views and to generate consistent texture co-ordinates.More recently,Sand et al[9]use multi-view recordings to derive the skeleton configuration of a moving subject,which subsequently derives the skin surface shape.These works show how a prior knowledge can be used to avoid dif-Fig.1.Overview Of Our Approachficulties of general reconstruction.However,they do not accumulate observations which can efficiently be used to handle uncertainties.The strength of gathering information from collective observation has been illustrated in face model acquisition by Blanz and Vetter[3].In their modeler, a highly detail3D face shape and texture spaces have been obtained,by trans-forming some two hundred laser-scanned faces into vector representation.Given a single photograph of a face,its3D shape,orientation in space,and the il-lumination conditions are estimated.Starting from a rough initial estimate of shape,surface color,and lighting parameters,an optimization algorithm itera-tivelyfinds the best matching parameters to the input image.Shape and texture constraints derived from the statistics of our example faces are used to guide au-tomated matching.While these methods are quite powerful,they have not been applied to image-based reconstruction of an entire human body.These consider-ations lead us to look for a more robust approach to image-based human body modeling.Based on our previous work[11],we adopt a model parameterization scheme based on a collection of observations of real bodies.Also adopted is asurface optimization framework,in order to match multiple camera images.As aresult,our technique handles complex articulated structure of the entire human body,and still runs at an arguably interactive speed.1.2OrganizationAn overview of our approach is illustrated in Figure1.In Section2,we describe the way we take photographs of a subject,and extract silhouettes and feature points on the images.We then use a deformable model that is based on the previous work of Seo and Magnenat-Thalmann[10]for the shape recovery,as detailed in ing the silhouette data extracted from the input images, we explore the body space(a range of coefficient parameters that have been spanned by the database of the deformable model)andfind the bestfitting deformation parameters on a template model.Finally,Section4describes the method we use to generate texture coordinate data by projecting the deformed template model onto the input images.After demonstrating the result models in Section5,we conclude the paper in Section6.2Acquiring and processing input images2.1Taking photographsWefirst take a minimum number of photographs of a subject,to acquire ob-servations on a subject.Our system in principle does not require any special camera arrangements,nor does it require specific number of views.In actuality, however,at least two views–one from the front and the other from the back –are preferred,as we want to generate complete texture on the entire body. This is because our deformable model does not contain color data(See Section 5.1).In our experiments,we also found that adding side view makes considerable improvement on the characteristics of the body shape.Hence,we mostly take three photographs using a single camera,each from the front,the side,and the back sides of the subject,unless otherwise specified.Throughout this paper,we assume that the subjects are lightly clothed.To simplify the combinatorial complexity of the human shape and posture,we re-quire the subject to stand in the specific posture;the limbs are straight and apart from the torso as shown in Figure1.We also require that photos are taken in front of a blue backdrop,to facilitate automatic silhouette extraction.In this paper,images have been captured by the Nikon coolpix5000camera and stored in1920by2560resolutions for the texture mapping.They have been reduced to 480by640resolutions for speedup during the silhouette comparison.2.2Virtual camera setup for the template model projectionWe now describe the camera arrangements and projection matrix we use for projecting the template model onto the image space.The main idea is to simulate virtual camera as closely as possible to the physical setup.This allows us to useinput images directly for the silhouette comparison without additional process such as image size normalization.In this paper,we adopted Tsai’s Pinhole camera model[12],which basically is a pinhole model taking the1st order radial lens distortion into account.A camera has5intrinsic parameters(focal length f,1st order radial lens distortion value Kappa,center of lens distortion C x,C y,scale factor S x)and6extrinsic parameters6(R x,R y,R z,T x,T y,R z).To calculate these intrinsic and extrin-sic parameters,we have taken an image of a calibration frame,similarly to the approach presented by Ahn et al[1].The intrinsic parameters we found are illus-trated in Table1.The intrinsic parameters like Kappa,C x,and C y are used to calculate the degree of distortion for each pixel.In our experiments,the degrees of distortion were less than one pixel,and thus were simply discarded from the projection matrix.Table1.Intrinsic parameters calculated for our cameraf Kappa C x C y S x21.7mm0.0000314247.0308.80.99Next,we setup our virtual camera from the measured parameters of the physical camera;the camera is approximately5meters distant from the template model and1.2meters from the ground.From the basic trigonometry we have obtained the FOVy(Field of View y)angle of25.4degrees.FOVy determines the perspective projection matrix we use for projecting the template model onto the image space.2.3Silhouette extraction and feature point identificationWe take photos in front of a uniformly colored screen so that simple methods such as using color key can be used for the automatic silhouette detection.The method we use is a standard background subtraction to isolate silhouettes from images using a color key.Among several color models,we use the Hue-Saturation-Value(HSV)color model.HSV model is commonly used in interactive color selection tools,be-cause of its similarity to the way how humans perceive colors. Wefirst map each pixel in the image to the color space defined by the HSV hexagonal cone.Pixels in the background region form a cluster in the HSV space, as illustrated in Figure2.In our experiments,wefirst project an image of the background drape to obtain the range of background pixel clusters.The cluster is defined by the H value of180o∼240o,and S value larger than a threshold, say,‘0.3’.As a subject stands in front of the background,shadows appear and they contribute to the background clouds elongated downwards along the V axis of the hexagon.Thus,we use color keys in H and S to determine the backgroundpixel cluster.As illustrated in Figure2,shadows have been successfully labeled as background.Fig.2.(a)Projection of images onto the HSV color space:Empty background(left), front(middle)and side(right)views.(b)Silhouette extraction results of two subjects with white(left)and black(right)underwearsNext,we label12∼15feature points on the silhouettes.In addition to the silhouette data as described earlier,we make use of a number of feature points when matching the template model to the target subject in the ing features points allows to deform the template not only to match the silhouette but also to ensure the correspondence.Unfortunately,only a limited set of feature points can be found automatically,such as those on the top of the head,the bottom of the feet and the tip of hands.For the rest of the feature points,the user manually places them on the images.Feature points on the template model are identified in a similar way on the3D mesh(see Figure3).3Shape recovery by searching deformation space3.1Parameterized body space constructionFor the shape recovery we use a parameterized shape model that is based on our previous work[10].In that modeler,each of the scanned body shape is represented by combining two shape vectors that are used to deform the template.These vectors respectively encode the skeleton-driven deformation(joint vector)and vertex displacement of a template model(displacement vector)that is necessaryto reproduce its shape.By collecting these shape vectors from multiple subjects, we obtain what we call the body space.Given a set of example body shapes represented as vectors,we apply principal component analysis(PCA)to both joint and displacement vectors.The result is two linear models for the two components:j=¯j+P j b jd=¯d+P d b dwhere¯j and¯d are the mean vector,P j and P d are sets of orthogonal modes of variation,and b j and b d are the sets of parameters.The appearance of any body models can thus be represented by the set of PC coefficients of joint vector b j and that of displacement vector b d.A new model can be synthesized for a given pair b j,b d by deforming the template from vector j and adding the vertex displacement using the map described by d.Note that the PCA has the additional benefit that the dimension of the vectors can drastically be reduced without losing the quality of shape.Upon finding the orthogonal basis,the original data vector v of dimension n can be represented by the projection of itself onto thefirst M( n)eigen vectors that correspond to the M largest eigen values.In this paper,we have used30bases both for the b d and b j.Thus,each body is represented as a set of parameter vector consisting of30PC’s for the joints and30for displacement,giving a total of60parameters for the body shape space.3.2Search-based deformationBuilding such parameterization of the body space as described in the previous section permits us to easily and efficiently explore the coefficients,thus simpli-fying the complex process of acquiring geometry of full body.Given a set of images,we use the extracted silhouette information to reconstruct the geom-etry by searching the body space(a range of coefficient parameters that have been spanned by the database of the deformable model)andfinding an optimum parameter set.A set of coefficient parameters comprises an optimum solution if,when collectively applied to the template model,it produces silhouettes that bestfit the given image silhouettes.The key point is that instances of the models are deformed in ways that are found in the example set,guaranteeing a realistic, robust shape acquisition.Wefind the solution in a coarse-to-fine manner.Since the deformation is pa-rameterized with PCA space for each of the vector components,wefirstfind the optimizing joint parameter b j,followed by the subsequent search for the bd in the displacement vector space.Our optimization technique is based on a direc-tion set method[8].The algorithm repeats‘search-deform-compare’loop until we obtain sufficient degree of matching between the silhouette of the deformed model and that of the input image–It generates a body shape from the current coefficients,projects the body model onto2D space,and updates the coefficients according to the silhouette difference.Thefirst set of iterations is performed byoptimizing only thefirst coefficients controlling thefirst few PCs.In subsequence iterations,more PCs are added to further deform the template.3.3Error metricOne important step in our modeler is to measure the silhouette matching error between the segmented images to projections of the template under deformation. We consider two error terms:Thefirst one is the distance between corresponding feature points.The second one is the silhouette error.Distance between corresponding feature points Thefirst criterion of a good match is the distance between corresponding feature points in the image space.We define the distance error term E d as the sum of the squared distances between each corresponding feature point’s location in the data image and its location on the projected image of the template mesh:E d=dist2(P(F T,i),F D,i),(i=1...n),where n is the number of feature points and dist is the Euclidean distance among two pixels in the image,F D,i is the i-th feature pixel in the image,F T,i the corresponding i-th feature point on the template model,and P:R3→R2 describes the perspective projection of the template mesh to the2D images.We consider a sparse set of feature points that are important for calculating joint configurations(scale,and rotation of each joint except for the root that has translation)of the template model.We have found that feature points around the neck,arm-pits,wrists,crotch and ankles are particularly important,as they undergo relatively high degree of transformation for a matching.Note that they overlap pretty much with anthropometric landmarks as well.27points were manually placed on the template mesh prior to projection to the images.On the images,15and12feature points were defined on the front and side views, respectively.In addition to approximating distance error,this term also insures the correspondence at the feature locations.In Figure3,corresponding feature points are represented with the same color.Silhouette error Using the distances among each feature point location will not result in a successful matching,because even though corresponding feature points are in the same position,actual body shapes can be different from each other.To acquire detailed match of the template model to the image,we define a silhouette error and denote as E a By silhouette error we refer to the fraction of pixels for which the projected and observed silhouettes do not match,as shown in Figure3.The number of background pixels that lie inside the projected template model is summed up with that of foreground pixels that lie outside of it:E a=(T(i,j)·¯D(i,j))T(i,j)+(¯T(i,j)·D(i,j))D(i,j)Fig.3.Distance between corresponding feature points(left)and non-overlapping area error calculated(right)on the front and side photos of a male subject(right)T(i,j),and¯T(i,j)are the boolean values indicating if the pixel at location (i,j)is inside and outside of the template model,respectively.D(i,j)and¯D(i,j) are1if the pixel located at(i,j)is foreground and background,respectively. This notion of non-overlapping area is effectively equivalent to the silhouette error used by Sand et al[9].Combining the error We use weighted sum of the two error terms,as denoted by E=αE d+(1-α)Eα.At thefirst iterations we need to quickly search for joint parameters,hence we setα=1.Feature points from both the frontal and the side images are measured.Next,we further improve thefitting accuracy by setting α=0.3.The deformable model isfirstfit to the frontal image and then side image error is added.Finally,the displacement map is explored with the same setting.At each iteration,we combine the errors from the frontal image and the side image,so that thefitting of the template to frontal and side images can simultaneously be handled.4Mapping texturesOne of our goals is to generate color data of the model,to maximize visual effects. Photo images are then used to generate texture on the geometry obtained from the above phase.Although we require the subject to keep consistent poses among different views,they may be slightly different from one view to another,as they are temporally distinct views.To handle such inconsistency,we use only the front and the side images for the shape recovery,and we handle the texture coordinate creation process for the front and the back parts separately.Two separate texture coordinates are obtained by projecting the deformed template model onto the front and the back images:If the angle between vertex normal and the view direction is between-π/2andπ/2,we project the vertex on the deformed template surface onto the front image.The other vertices areprojected on to the back image.Prior to the second projection,we must adjustthe posture of the model by matching the template with the silhouette data on the back image.This is due to the slight difference among postures seen from one view to another.5Results5.1DatasetWhole body scans of40male bodies of European adults,recorded with Tecmath T M laser scanners[13]were used in our experiments.The scanner does not provide color data,and thus we have used input photos to generate texture maps.The dataset was originally captured for made-to-measure garment retails.Analogous to those subjects for the image-based reconstruction,these subjects for the three dimensional scan were lightly clothed without accessories,and were in a standing posture with arms and legs slightly apart.Additionally,the face was removed digitally via a vertical cut anterior to forehead,with manual editing on the point cloud.5.2Model reconstructionWe have applied our modeler to a number of example images.Some of the result models are illustrated in our video demo.In all examples,we matched the template model built from thefirst30joint and30displacement principal components that were derived from the whole body scan dataset,as described in the previous section.Once the silhouettes have been extracted,the whole matching procedure was performed in less than1000iterations for each principal component(PC),taking a total of6∼7minutes on a Pentium4processor when 3PCs of the joint vector and3PCs of the displacement vector are optimized. 5.3Single image inputBecause our modeler is based on optimization-based searching and not on an entirely geometric technique,we can handle some ambiguous situations robustly. To demonstrate such robust behavior of our modeler,we have reconstructed a 3D geometry using only a single image input.In Figure4(a),we used only the front image of the subject to reconstruct the shape of the template model. Analogously,only the side image of the subject was used to reconstruct the shape shown in Figure4(b).In both cases,a back view image was used to complete the texture map.6ConclusionWe have presented a technique for reconstructing human body model from a limited number of2D ing the three-dimensional body space that has been generated from processing range scans,we propose reconstructing the3DFig.4.A reconstructed model by using a single image input.(a)front image(b)side imagesurface in a different manner than existing modelers.For the shape recovery we start with a deformable template model whose deformation is parameterized with PCA of the scanned body shapes.Given a set of images,the optimizing shape is found by searching the shape space,such that it minimizes the matching error measured between silhouettes.The idea is to start from a space consisting of a few PCs and to increase its size by progressively adding new PCs.This provides us powerful means of matching the template model to the image in a coarse-to-fine manner.In addition,a high level of detail and accuracy is acquired,since our modeler essentially blends multiple shapes of the human body acquired from 3D laser scanners.This constitutes a good complement to geometric methods, which cannot capture detailed shape solely from image input.Our system is intended for off-line reconstruction of geometry,but it is rea-sonably efficient and can also be adopted for on-line applications.When we reduce the number of PCs to be optimized during searching,say4∼5for each of the shape vector,we can obtain fairly good results without losing much of the accuracy.Additionally,we have successfully integrated our models in animation systems.We are currently exploring an extension of this technique with which we can reconstruct the body shape even when given images of casually dressedsubjects.AcknowledgementsThe authors are grateful to Gun-Woo Kim,who has helped us with the format-ting of the paper.This work has been supported in part by Chungnam National Univeristy,BK21project of EECS Department at KAIST,and MMRC project funded by SK Telecom.References1.Ahn J.H.,Sul C.H.,Park E.K.,Wohn K.W.,”Lan-based Optical Motion CaptureSystem”,pp.429-432,Proc.Korea Conference on Human Computer Interaction 2000.2.Allen B.,Curless B.,Popovic Z,”The space of human body shapes:reconstruc-tion and parameterization from range scans”,Proc.SIGGRAPH’03,pp.587-594, Addison-Wesley,2003.3.Blanz B.and Vetter T.,”A morphable model for the synthesis of3D faces”,Proc.SIGGRAPH’99,Addison-Wesley,pp.187-194,1999.4.Cordier F.,Seo H.,Magnenat-Thalmann N.,”Made-to-Measure Technologies forOnline Clothing Store”,pp.38-48,IEEE CG&A special issue on Web Graphics, January2003.5.Hamamatsu BL scanner,http://www.hpk.co.jp6.Hilton A.,Beresford D.,Gentils T.,Smith R.J.,Sun W.and Illingworth J.,”Whole-body modelling of people from multi-view images to populate virtual worlds”, Visual Computer:International Journal of Computer Graphics,16(7),pp.411-436,2000.7.Lee W.,Gu J.,and Magnenat-Thalmann N.,”Generating Animatable3D Vir-tual Humans from Photographs”,Computer Graphics Forum,vol.19,no.3,Proc.Eurographics2000In-terlaken,Switzerland,August,pp.1-10,2000.8.Press W.H.,Flannery B.P.,Teukolsky S.A.,and Vetterling W.T.,”NumericalRecipes in C”,The art of scientific computing.Cambridge University Press,1988.9.Sand P.,McMillan L.,Popovic J.,”Continuous Capture of Skin Deformation”,Proc.ACM SIGGRAPH2003,pp.578-586.10.Seo H.and Magnenat-Thalmann N.,”An Automatic Modeling of Human Bodiesfrom Sizing Parameters”,ACM SIGGRAPH Symposium on Interactive3D Graph-ics,ACM Press,pp.19-26,2003.11.Seo H.,”Parameterized Human Body Modeling”,PhD thesis,Departementd’informatique,University of Geneva,2004.12.Tsai R.Y.,”An Efficient and Accurate Camera Calibration Technique for3D Ma-chine Vision”,Proceedings of IEEE Conference on Computer Vision and Pattern Recognition,Miami Beach,FL,1986,pp.364-374.13.Tecmath AG,.14.Telmat Industrie SA,.。