Recursive algorithm
算法分析算法复习题中英文
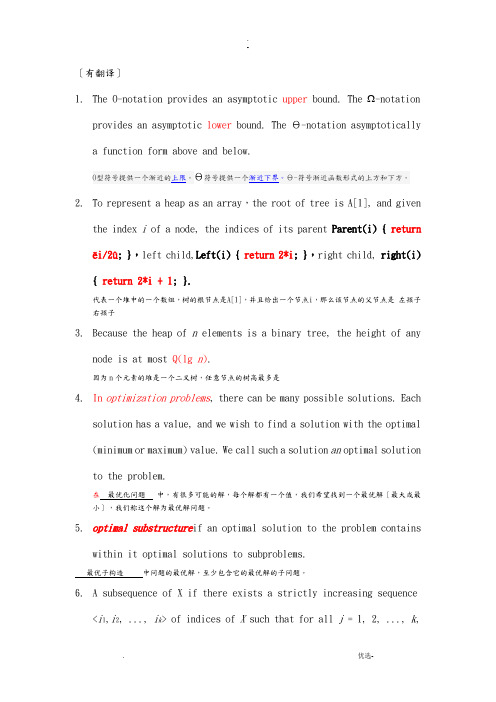
〔有翻译〕1.The O-notation provides an asymptotic upper bound. The -notationprovides an asymptotic lower bound. The Θ-notation asymptoticallya function form above and below.2.To represent a heap as an array,the root of tree is A[1], and giventhe index i of a node, the indices of its parent Parent(i) { return ëi/2û; },left child,Left(i) { return 2*i; },right child, right(i) { return 2*i + 1; }.代表一个堆中的一个数组,树的根节点是A[1],并且给出一个节点i,那么该节点的父节点是左孩子右孩子3.Because the heap of n elements is a binary tree, the height of anynode is at most Q(lg n).因为n个元素的堆是一个二叉树,任意节点的树高最多是4.In optimization problems, there can be many possible solutions. Eachsolution has a value, and we wish to find a solution with the optimal (minimum or maximum) value. We call such a solution an optimal solution to the problem.在最优化问题中,有很多可能的解,每个解都有一个值,我们希望找到一个最优解〔最大或最小〕,我们称这个解为最优解问题。
算法分析与设计基础答案11章
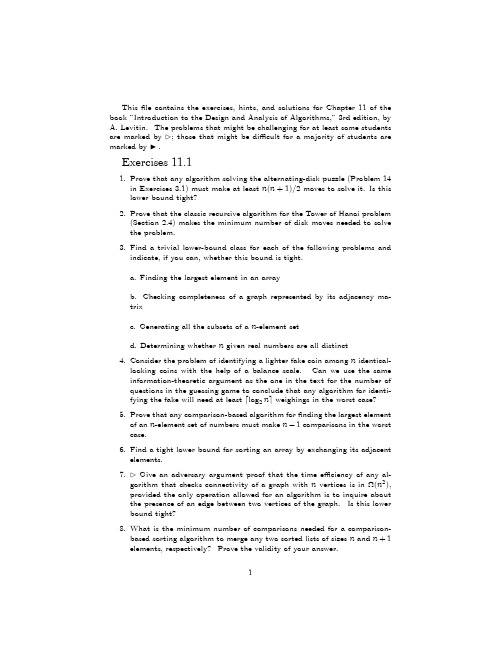
This file contains the exercises, hints, and solutions for Chapter 11 of the book ”Introduction to the Design and Analysis of Algorithms,” 3rd edition, by A. Levitin. The problems that might be challenging for at least some students are marked by B; those that might be difficult for a majority of students are marked by I
[2016最新精品]表面粗糙度测量中的高斯递归滤波算法
![[2016最新精品]表面粗糙度测量中的高斯递归滤波算法](https://img.taocdn.com/s3/m/1660cad4db38376baf1ffc4ffe4733687e21fc49.png)
表面粗糙度测量中的高斯递归滤波算法摘要基于中心极限定理,提出了一个高斯滤波器逼近模型,并利用冲激响应不变法设计了一个数字高斯滤波器。
通过级联方法,该滤波器的幅度传输特性偏差可控制在(-0.28% +1.14%)以内。
该滤波器采用递归实现算法,算法简便,易于实现。
在递归算法的基础上,给出了一种预测边缘初始值的方法,有效抑制了递归算法的边缘效应。
实验结果表明,该滤波器能够稳定可靠地提取工程表面中线,在普通计算机上提取11200点数据表面中线仅耗时170ms,效率很高。
关键词计量学表面粗糙度高斯滤波器边缘效应中图分类号TP2文献标识码A国家标准学科分类与代码520.604Gaussian Recursive Filtering algorithm to Surface Roughness MeasurementAbstract Based on the central limit theorem, an approximation model of Gaussian filter is put forward. Then, a digital Gaussian filter is designed by combination with impulse invariant transformation and this model. Through the cascade use, the amplitude transmission deviation of this filter can be controlled within(-0.28%~+1.14%). Its recursive algorithm is so simple that it is implemented easily. A method of forecasting the initial value of the edge is proposed to restrain the end effect caused by this recursive algorithm. Experimental results show that this filter can extract the mean line of engineering surface stably and reliably, once extraction of 11, 200 point data on a general computer only consumes 170ms, efficiency is demonstrated.Key words Metrology Surface roughness Gaussian filter End effect1 引言在表面粗糙度测量中,表面中线的确定是一个关键问题,它对评定表面特征参数有着重要的作用[1]。
计算机算法设计五大常用算法的分析及实例
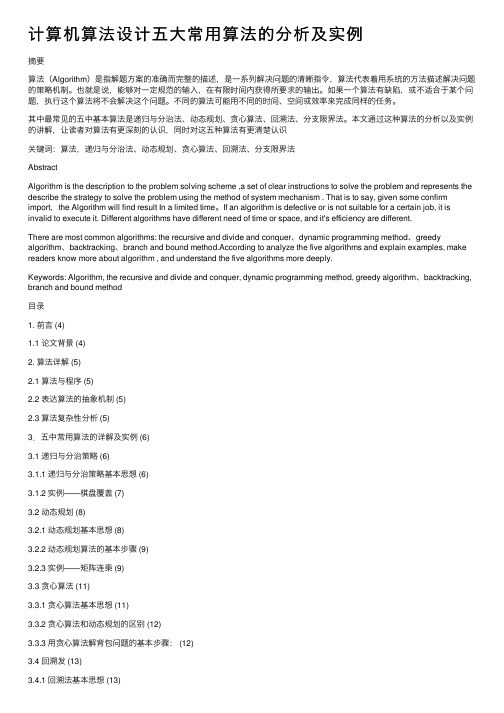
计算机算法设计五⼤常⽤算法的分析及实例摘要算法(Algorithm)是指解题⽅案的准确⽽完整的描述,是⼀系列解决问题的清晰指令,算法代表着⽤系统的⽅法描述解决问题的策略机制。
也就是说,能够对⼀定规范的输⼊,在有限时间内获得所要求的输出。
如果⼀个算法有缺陷,或不适合于某个问题,执⾏这个算法将不会解决这个问题。
不同的算法可能⽤不同的时间、空间或效率来完成同样的任务。
其中最常见的五中基本算法是递归与分治法、动态规划、贪⼼算法、回溯法、分⽀限界法。
本⽂通过这种算法的分析以及实例的讲解,让读者对算法有更深刻的认识,同时对这五种算法有更清楚认识关键词:算法,递归与分治法、动态规划、贪⼼算法、回溯法、分⽀限界法AbstractAlgorithm is the description to the problem solving scheme ,a set of clear instructions to solve the problem and represents the describe the strategy to solve the problem using the method of system mechanism . That is to say, given some confirm import,the Algorithm will find result In a limited time。
If an algorithm is defective or is not suitable for a certain job, it is invalid to execute it. Different algorithms have different need of time or space, and it's efficiency are different.There are most common algorithms: the recursive and divide and conquer、dynamic programming method、greedy algorithm、backtracking、branch and bound method.According to analyze the five algorithms and explain examples, make readers know more about algorithm , and understand the five algorithms more deeply.Keywords: Algorithm, the recursive and divide and conquer, dynamic programming method, greedy algorithm、backtracking, branch and bound method⽬录1. 前⾔ (4)1.1 论⽂背景 (4)2. 算法详解 (5)2.1 算法与程序 (5)2.2 表达算法的抽象机制 (5)2.3 算法复杂性分析 (5)3.五中常⽤算法的详解及实例 (6)3.1 递归与分治策略 (6)3.1.1 递归与分治策略基本思想 (6)3.1.2 实例——棋盘覆盖 (7)3.2 动态规划 (8)3.2.1 动态规划基本思想 (8)3.2.2 动态规划算法的基本步骤 (9)3.2.3 实例——矩阵连乘 (9)3.3 贪⼼算法 (11)3.3.1 贪⼼算法基本思想 (11)3.3.2 贪⼼算法和动态规划的区别 (12)3.3.3 ⽤贪⼼算法解背包问题的基本步骤: (12)3.4 回溯发 (13)3.4.1 回溯法基本思想 (13)3.3.2 回溯发解题基本步骤 (13)3.3.3 实例——0-1背包问题 (14)3.5 分⽀限界法 (15)3.5.1 分⽀限界法思想 (15)3.5.2 实例——装载问题 (16)总结 (18)参考⽂献 (18)1. 前⾔1.1 论⽂背景算法(Algorithm)是指解题⽅案的准确⽽完整的描述,是⼀系列解决问题的清晰指令,算法代表着⽤系统的⽅法描述解决问题的策略机制。
山东建筑大学计算机学院算法分析第三部分复习
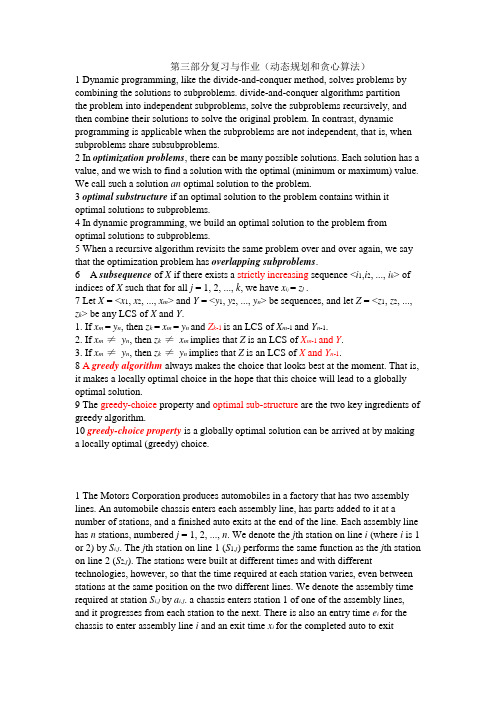
第三部分复习与作业(动态规划和贪心算法)1 Dynamic programming, like the divide-and-conquer method, solves problems by combining the solutions to subproblems. divide-and-conquer algorithms partitionthe problem into independent subproblems, solve the subproblems recursively, and then combine their solutions to solve the original problem. In contrast, dynamic programming is applicable when the subproblems are not independent, that is, when subproblems share subsubproblems.2 In optimization problems, there can be many possible solutions. Each solution has a value, and we wish to find a solution with the optimal (minimum or maximum) value. We call such a solution an optimal solution to the problem.3 optimal substructure if an optimal solution to the problem contains within it optimal solutions to subproblems.4 In dynamic programming, we build an optimal solution to the problem from optimal solutions to subproblems.5 When a recursive algorithm revisits the same problem over and over again, we say that the optimization problem has overlapping subproblems.6 A subsequence of X if there exists a strictly increasing sequence <i1,i2, ..., i k> of indices of X such that for all j = 1, 2, ..., k, we have x i j= z j .7 Let X = <x1, x2, ..., x m> and Y = <y1, y2, ..., y n> be sequences, and let Z = <z1, z2, ..., z k> be any LCS of X and Y.1. If x m = y n, then z k = x m = y n and Z k-1is an LCS of X m-1 and Y n-1.2. If x m ≠y n, then z k ≠x m implies that Z is an LCS of X m-1 and Y.3. If x m ≠y n, then z k ≠y n implies that Z is an LCS of X and Y n-1.8 A greedy algorithm always makes the choice that looks best at the moment. That is, it makes a locally optimal choice in the hope that this choice will lead to a globally optimal solution.9 The greedy-choice property and optimal sub-structure are the two key ingredients of greedy algorithm.10 greedy-choice property is a globally optimal solution can be arrived at by makinga locally optimal (greedy) choice.1 The Motors Corporation produces automobiles in a factory that has two assembly lines. An automobile chassis enters each assembly line, has parts added to it at a number of stations, and a finished auto exits at the end of the line. Each assembly line has n stations, numbered j = 1, 2, ..., n. We denote the j th station on line i (where i is 1 or 2) by S i,j. The j th station on line 1 (S1,j) performs the same function as the j th station on line2 (S2,j). The stations were built at different times and with different technologies, however, so that the time required at each station varies, even between stations at the same position on the two different lines. We denote the assembly time required at station S i,j by a i,j. a chassis enters station 1 of one of the assembly lines, and it progresses from each station to the next. There is also an entry time e i for the chassis to enter assembly line i and an exit time x i for the completed auto to exitassembly line i. The time to transfer a chassis away from assembly line i after having gone through station S i,j is t i,j, where i = 1, 2 and j = 1, 2, ..., n – 1. A manufacturing problem is to find the fastest way through a factory. Let f i[j] denote the fastest possible time to get a chassis from the starting point through station S i,j. Our ultimate goal is to determine the fastest time to get a chassis all the way through the factory, which we denote by f*. Please write its recursive formula and compute the fastest time and construct the fastest way through the factory of the instance.2 The matrix-chain multiplication problem can be stated as follows: given a chain<A1,A2,…,An>of matrices, where for i=1,2…,n, matrix A i has dimensionP i-1 P i, fully parenthesize the product A1,A2,…,A n in a way that minimizes the number of scalar multiplication. We pick as our subproblems the problems of determining the minimum cost of a parenthesization of A i A i+1 A j for 1 ≤i ≤j ≤n. Let m[i, j] be the minimum number of scalar multiplications needed to compute the matrix A i..j; for the full problem, the cost of a cheapest way to compute A1..n would thus be m[1, n]. Can you define m[i, j] recursively? Find an optimal parenthesization of a matrix-chain product whose sequence of dimensions is <2,1,3,2,4>3 In the longest-common-subsequence (LCS) problem, we are given two sequences X = <x1, x2, ...,x m> and Y = <y1, y2, ..., y n> and wish to find a maximum-length common subsequence of X and Y. Please write its recursive formula and determine an LSC of <1,0,0,1,0,1,0,1> and <0,1,0,1,1,0,1,1,0>.4 The 0–1 knapsack problem is posed as follows. A thief robbing a store finds n items; the i th item is worth v i dollars and weighs w i pounds, where v i and w i are integers. He wants to take as valuable a load as possible, but he can carry at most W pounds in his knapsack for some integer W. Which items should he take? This is called the 0–1 knapsack problem because each item must either be taken or left behind; the thief cannot take a fractional amount of an item or take an item more than once. In the fractional knapsack problem, the setup is the same, but the thief can take fractions of items, rather than having to make a binary (0–1) choice for each item. Can the above two problems is solved by greedy strategy? Why?(P382)5 What is an optimal Huffman code for the following set of frequencies, based on the6 numbers? a:45 b:13 c:12 d:16 e:9 f:5。
一种组网雷达误差配准和目标跟踪递推算法
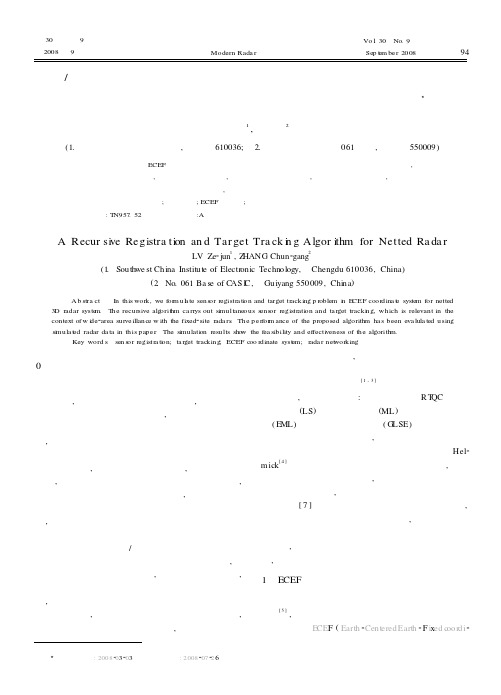
信号/数据处理一种组网雷达误差配准和目标跟踪递推算法3吕泽均1,张春刚2(1.中国西南电子技术研究所, 成都610036; 2.中国航天科工集团061基地, 贵阳550009)【摘要】 提出了一种在ECEF坐标系中对组网三维雷达的系统误差进行估计和目标跟踪联合递推算法,该算法能对系统误差进行在线估计和补偿,适应系统误差的变化,并同时对目标进行跟踪,提高了航迹融合精度,扩大了雷达的监视范围。
用模拟的雷达数据对算法的性能进行了评估,计算机仿真试验验证了该算法的可行性和有效性。
【关键词】 传感器误差配准;目标跟踪;ECEF坐标系;雷达组网中图分类号:T N957.52 文献标识码:AA Recur si ve Registra ti on an d Tar get Tra ck i n g Algor i thm for Netted Ra da rLV Ze2jun1,Z HANG Chun2gang2(1.Southwe st China Institute of Electr onic Technology, Chengdu610036,China)(2.No.061Ba se of C AS I C, Guiyang550009,China)【Ab stra ct】 In this work,we for m ul a te sens or registra ti on and target tracking p roblem i n E CEF coordina t e system for netted 3D radar syste m.T he recursive alg orit hm ca rrys out si multaneous s ens or registrati on and ta rget tracki ng,which is relevant in the context of w i de2area surve illance w ith the fixed2site rada rs.The pe rfor m ance of the proposed algorith m ha s been eva lula ted using si mula t ed radar da ta in this pape r.The si mulati on results sh o w the fea sibility and effecti veness of the algorith m.【Key word s】sen s or registra ti on;ta rget tracking;ECEF coo rdinate syst em;rada r net working0 引 言雷达及其对抗始终是现代战争中争夺制电磁权的重要内容,随着综合电子干扰技术的发展,单部雷达已经很难与电子对抗系统全面抗衡,传统雷达所面临的电子干扰和隐身目标攻击促使雷达向网络化方向发展,如今的雷达及其对抗已经发展到体系对体系的作战模式。
二维矩形条带装箱问题的底部左齐择优匹配算法
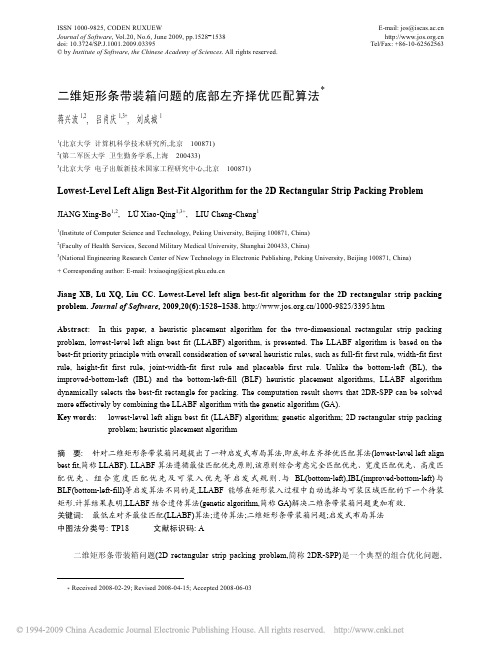
ISSN 1000-9825, CODEN RUXUEW E-mail: jos@Journal of Software, Vol.20, No.6, June 2009, pp.1528−1538 doi: 10.3724/SP.J.1001.2009.03395 Tel/Fax: +86-10-62562563© by Institute of Software, the Chinese Academy of Sciences. All rights reserved.∗二维矩形条带装箱问题的底部左齐择优匹配算法蒋兴波1,2, 吕肖庆1,3+, 刘成城11(北京大学计算机科学技术研究所,北京 100871)2(第二军医大学卫生勤务学系,上海 200433)3(北京大学电子出版新技术国家工程研究中心,北京 100871)Lowest-Level Left Align Best-Fit Algorithm for the 2D Rectangular Strip Packing ProblemJIANG Xing-Bo1,2, LÜ Xiao-Qing1,3+, LIU Cheng-Cheng11(Institute of Computer Science and Technology, Peking University, Beijing 100871, China)2(Faculty of Health Services, Second Military Medical University, Shanghai 200433, China)3(National Engineering Research Center of New Technology in Electronic Publishing, Peking University, Beijing 100871, China)+ Corresponding author: E-mail: lvxiaoqing@Jiang XB, Lü XQ, Liu CC. Lowest-Level left align best-fit algorithm for the 2D rectangular strip packingproblem. Journal of Software, 2009,20(6):1528−1538. /1000-9825/3395.htmAbstract: In this paper, a heuristic placement algorithm for the two-dimensional rectangular strip packingproblem, lowest-level left align best fit (LLABF) algorithm, is presented. The LLABF algorithm is based on thebest-fit priority principle with overall consideration of several heuristic rules, such as full-fit first rule, width-fit firstrule, height-fit first rule, joint-width-fit first rule and placeable first rule. Unlike the bottom-left (BL), theimproved-bottom-left (IBL) and the bottom-left-fill (BLF) heuristic placement algorithms, LLABF algorithmdynamically selects the best-fit rectangle for packing. The computation result shows that 2DR-SPP can be solvedmore effectively by combining the LLABF algorithm with the genetic algorithm (GA).Key words: lowest-level left align best fit (LLABF) algorithm; genetic algorithm; 2D rectangular strip packingproblem; heuristic placement algorithm摘要: 针对二维矩形条带装箱问题提出了一种启发式布局算法,即底部左齐择优匹配算法(lowest-level left alignbest fit,简称LLABF). LLABF算法遵循最佳匹配优先原则,该原则综合考虑完全匹配优先、宽度匹配优先、高度匹配优先、组合宽度匹配优先及可装入优先等启发式规则.与BL(bottom-left),IBL(improved-bottom-left)与BLF(bottom-left-fill)等启发算法不同的是,LLABF能够在矩形装入过程中自动选择与可装区域匹配的下一个待装矩形.计算结果表明,LLABF结合遗传算法(genetic algorithm,简称GA)解决二维条带装箱问题更加有效.关键词: 最低左对齐最佳匹配(LLABF)算法;遗传算法;二维矩形条带装箱问题;启发式布局算法中图法分类号: TP18文献标识码: A二维矩形条带装箱问题(2D rectangular strip packing problem,简称2DR-SPP)是一个典型的组合优化问题,∗ Received 2008-02-29; Revised 2008-04-15; Accepted 2008-06-03蒋兴波等:二维矩形条带装箱问题的底部左齐择优匹配算法1529其应用相当广泛,如工业领域中的新闻组版、布料切割、金属下料等.2DR-SPP通常是指将若干个不同规格的矩形{π1,π2,…,πn}装入宽度W固定、长度L不限的矩形容器C中,要求装完所有矩形后占用高度H packing最小,从而达到节省材料的目的.在矩形的装入过程中,要求满足:①πi,πj互不重叠,i≠j,i,j=1,2,…,n;②πi必须装入在矩形容器C内,即矩形在装入过程中不能超出容器C的宽度W;③πi的边必须与矩形容器C的边平行,πi可以90°旋转.2DR-SPP是一个NP完全问题,其计算复杂度随着问题规模的增大呈指数增长.针对该类问题,国内外相关学者作了大量的研究.Hifi[1]给出了一种基于分支定界方法(branch-and-bound procedure)的精确算法,适用于解决中小规模的2D装箱问题;Lesh等人[2]针对2D矩形完美装箱问题,提出了基于分支定界的穷举搜索算法,该算法已证明对于低于30个矩形的装箱是有效的.为了解决大规模的矩形装箱问题,一些启发式算法也相继提出来.Zhang等人[3]提出了一种启发式递归算法HR(heuristic recursive algorithm),该方法基于启发式策略和递归结构,实验结果表明,该算法能够在较短时间内获得较为理想的装箱高度,但其平均运行时间却达到了O(n3).陈端兵等人[4]根据先占角后占边的原则,提出了一种针对矩形装箱的贪心算法.Chen等人[5]给出了一种two-level搜索算法来求解2DR-SPP.Cui[6]给出了一种启发式递归分支定界算法HRBB(heuristic recursive branch-and-bound algorithm),该算法将递归结构和分支定界技术结合在一起来求解2DR-SPP,取得了较好的实验结果.Beltrán等人[7]按照随机搜索原则设计的GRASP(greedy randomized adaptive search procedure)算法与VNS(variable neighbourhood search)结合在一起来求解2DR-SPP.而Alvarez-Valdes等人[8]提出了Reactive GRASP算法,该算法分为构建和改进两个阶段,其测试结果优于文献[7].张德富等人[9]则提出了一种有效的砌墙式启发式算法PH,实验结果表明,该算法对规模较大的2DR-SPP能够获得较好的装箱效果.针对2DR-SPP,目前广泛采用的是遗传算法GA(genetic algorithm)、模拟退火算法SAA(simulated annealing algorithm)等搜索算法与BL(bottom-left)、IBL(improved BL)、BLF(bottom-left-fill)等启发式布局算法相结合的方式.Bortfeldt[10]采用了无任何编码的遗传算法来解决矩形装箱问题.Jackobs[11]提出了一种混合算法,通过GA 与BL启发式布局算法相结合,从而将矩形的装箱问题转换成相对简单的装入序列问题.由于BL算法容易出现即使穷举所有情况仍不能得到最优解的现象,因此,Liu[12]提出了一种IBL算法,并与GA相结合,取得了优于文献[11]的装箱效果.Yeung等人[13]针对布料切割问题提出了一种布局算法LFLA,该布局算法的计算复杂度为O(n),相对于BL算法(其复杂度为O(n2)),效率上有了较大的提高.Zhang等人[14]针对矩形装箱问题给出了meta-heuristic算法,该算法主要基于启发式递归策略和SAA.Dereli等人[15]则采用SAA与递归布局方法相结合来解决2DR-SPP.针对不同数量及不同类型的矩形排样,Hopper[16]分别使用了BL,BLF启发式布局算法和GA,NE(naïve evolution),SA,HC(hill-climbing),RS(random search)启发式搜索算法相结合的方式进行求解.迄今为止,虽然对2DR-SPP进行了大量的研究,但从相关文献给出的测试结果来看,该问题仍然有进一步研究的必要.例如,BL,IBL,BLF,LFLA以及贾志欣等人[17]提出的最低水平线LHL(lowest-horizontal-line)等常见的启发式布局算法,它们在对矩形装入的过程中严格按照矩形的某个排列序列进行,容易产生较大的空洞,浪费空间,因此装箱效果不够理想.相对于BL而言,BLF算法可以取得较好的装箱效果,但其算法的时间复杂度却达到了O(n3)[18],不适宜解决规模较大的2DR-SPP.本文针对上述问题设计出一种启发式布局算法,即底部左齐择优匹配算法(lowest-level left align best fit,简称LLABF).本文第1节重点介绍LLABF算法设计.第2节介绍GA+LLABF算法的实现.第3节主要对标准数据集进行模拟测试,并与相关文献结果进行对比分析.第4节给出结论.1 LLABF算法设计1.1 定义设容器宽度所在方向为横坐标X,长度方向为纵坐标Y,容器的左下角为坐标原点(0,0).容器的底部在y=0处,容器可以在Y轴正方向无限延伸.1530 Journal of Software软件学报 V ol.20, No.6, June 2009队列I={π1,π2,…,πn}表示n个矩形的某个装入序列,其中,πi是矩形编号,πi∈[1,n],对任意i,j∈[1,n],当i≠j时, πi≠πj.设I(i)表示队列I中第i个位置上对应的矩形,它由五元组构成,即I(i)={x,y,w,h,θ},其中,I(i).x,I(i).y分别表示该矩形装入后,其左下角的横、纵坐标;I(i).w,I(i).h,I(i).θ分别表示该矩形的宽度、高度和旋转角度.设队列E={e1,e2,…,e m}表示装入过程中产生的轮廓线集合,元素e k为水平线线段(与X坐标轴平行),它由三元组构成,即e k={x,y,w},其中,e k.x,e k.y表示第k个水平线线段的左端点坐标(起点坐标);e k.w表示第k个水平线线段的宽度;并且对任意0<k<m,有e k.x<e k+1.x,即按轮廓线起点的x坐标从小到大排列.队列E具有以下特征:其y坐标具备唯一性,如果相邻线段具有相同的高度y,则进行合并;所有线段在X坐标上的投影不重叠;所有线段的宽度之和刚好等于矩形容器宽度W.最低水平线定义为队列E中其y坐标最小的水平线.最优高度H opt表示穷举所有可能情况得到的最小装箱高度,也称为最优解.装箱高度H packing表示根据当前装入序列,按照布局算法将所有矩形装入矩形容器C内所得到的最小高度.空洞是指在装箱过程中,由矩形或者矩形与容器边界(如x=0,y=0,x=W或者y=H packing)围成的空白区.空洞越多,材料浪费的程度就越严重.1.2 LLABF启发规则设计1.2.1 基本思想一般情况下,对NP难问题很难直接构造出一个最优解或满意解,所以只能通过搜索的方法在整个解空间中寻找最优解或者满意解.当问题规模较大时,这种盲目搜索或者遍历就变得十分困难,甚至根本不可行.因此,相关学者常利用问题解本身的某些结构特征来构造出一些启发式规则,并按照该规则设计出启发式算法,由该算法求得问题的一个满意解.对于矩形条带装箱问题,它有以下几个可以利用的结构特征:(1) 面积较大的矩形装入后产生的空洞较大,面积较小的矩形装入后产生的空洞较小;(2) 面积较大的矩形装入后产生的空洞常常可以装入面积较小的矩形;(3) 装入过程中产生的轮廓线越规整,即组成轮廓线的水平线数量越少,就越有利于后期矩形的装入.本文中,LLABF启发式算法利用了矩形条带装箱问题的3个结构特征,采用了动态选择方法.该方法遵照最佳匹配优先原则来动态选择下一个待装矩形,使得在矩形装入的每一步都尽可能地获得一个较优的装箱结果.重复执行,直至最终获得整体较优解.最佳匹配优先原则(best-fit priority,简称BFP)即在矩形的装入过程中,优先考虑与当前可装入区域宽度或高度相匹配以及可装入的未排矩形,它包括完全匹配优先、宽度匹配优先、高度匹配优先、组合宽度匹配优先以及可装入优先这5种启发式规则.5种启发式规则中完全匹配优先、宽度匹配优先以及组合宽度匹配优先可以减少空洞的产生,特别是在装入初期,由于用来与可装区域进行比较的矩形较多,因此,其匹配的概率越高,就越不容易产生空洞;完全匹配优先以及高度匹配优先使得装箱轮廓相对规整;高度匹配优先以及可装入优先可以将较小矩形延后装入.5种启发式规则结合起来不但可以使矩形装入后产生的空洞数量较少,而且所有的空洞总面积相对也较小.1.2.2 启发规则设计完全匹配优先(full-fit first,简称FFF). 在可装入的轮廓线中选取最低的水平线e k,如果有多个线段,则优先选取最左边的一段.从待装矩形中按照装入序列依次将矩形与e k进行比较,如果存在宽度或者高度与该线段宽度e k.w相等且装入后刚好左填平或者右填平的矩形则优先装入.完全匹配优先能够减少装入后产生的轮廓线数量,使得装入轮廓朝着顶部平齐的方向发展.宽度匹配优先(width-fit first,简称WFF). 在装入过程中,优先装入宽度或者高度与最低水平线e k等宽的矩形,如果存在多个匹配矩形,则优先装入面积最大的.与完全匹配优先规则不同的是,宽度匹配优先并不要求装入后能够实现左填平或者右填平;同时,该规则使得较小矩形有推迟装入的趋势.另外,WFF不会增加装入轮廓线数量.蒋兴波 等:二维矩形条带装箱问题的底部左齐择优匹配算法1531高度匹配优先(height -fit first ,简称HFF ). 在待装矩形中,按照装入序列查询宽度或高度不大于最低水平线e k 宽度且装入后能够实现左填平的矩形,若存在则装入查询到的首个矩形.与FFF 和WFF 不同,HFF 可能会在最低水平线上产生新的、更小的可装入区域,但却增加了轮廓线e k −1的宽度.组合宽度匹配优先(joint -width -fit first ,简称JWFF ). 按装入序列对两个矩形进行组合,如果组合后的宽度与最低水平线宽度e k 相等,则优先装入组合序列中的首个矩形.例如,存在两种组合I (i 1).w +I (j 1).w =e k .w , I (i 2).w +I (j 2).w =e k .w ,如果I (i 1)的面积大于I (i 2),则首先装入I (i 1),否则装入I (i 2).JWFF,FFF 与WFF 规则避免了在最低水平线e k 上产生新的、更小的可装入区域,从而减少了整个装箱过程产生空洞的可能性.为了保证时效性,算法中设置了查询范围searchNum ,即从当前位置开始,最多可以进行searchNum ×searchNum 次连续矩形的两两组合查询.可装入优先(placeable first ,简称PF ). 在一定范围内,从待装矩形件中按照装入序列依次查找宽度或高度不大于最低水平线e k 宽度的矩形,若存在,则将其装入;若存在多个,则装入面积最大的矩形.PF 可能在最低水平线上产生新的、更小的可装入区域,同时使得较小矩形延迟装入.在矩形的装入过程中,满足FFF,WFF,HFF 以及JWFF 规则的矩形可能并不存在,此时就必须考虑PF.如果满足PF 规则的矩形仍不存在,那么必然会在最低水平线上产生空洞.与BL,IBL,LFLA 以及LHL 算法不同,该空洞是在一定范围内由于不存在可装入的矩形而产生的,因此其面积更小.与BLF 算法不同,LLABF 算法并不需要保存新产生的空洞.图1给出了按照最佳匹配优先原则的部分启发规则实现矩形装入的示意图,其中,矩形的装入序列是4 2 7 6 5 1 8 3.图1(a)表示已经装入了4个矩形,产生了由4个线段e 1,e 2,e 3,e 4组成的装入轮廓,其中,e 2为最低水平线,其对应的区域是接下来首先要考虑的区域.图1(b)采用完全匹配优先:与最低水平线e 2等宽的矩形有1号和3号,但由于只有3号矩形装入后能够实现左填平,因此首先选择该矩形装入.图1(c)采用宽度匹配优先:满足该条件的矩形有1号和3号,但由于1号矩形面积较大,所以优先装入.图1(d)采用高度优先:1,8,3这3个矩形都满足其宽度不大于e 2.w ,但只有8号和3号矩形装入后能够实现左填平,且8号矩形位置在3号之前,所以优先装入.(a) (b)(c) (d)Fig.1 Packing result according to BFP principle图1 按BFP 原则的装箱结果图1.3 LLABF 算法的实现LLABF 算法是按照BFP 原则进行装箱的启发式布局算法,其算法实现如下所示.1532 Journal of Software软件学报 V ol.20, No.6, June 2009LLABF (input: I; output: H packing)beginInitContours(E); InitCoordinate(I); H packing=0; // 初始化;H packing:装箱高度① for (ii=1; ii≤n; ii++)begin②if (I(ii).x>−1) goto ①; // 当前矩形件已被装入在E中取最低水平线e k;j=−1; //记录用于装入的矩形件所在序列号FullFitFirst(ii,I,e k,j); if (j>−1) goto ④;//完全匹配FFFWidthFitFirst(ii,I,e k,j); if (j>−1) goto ④; //宽度匹配WFFHeightFitFirst(ii,I,e k,j); if (j>−1) goto ④; //高度匹配HFFJointWidthFitFirst(ii,I,e k,7,j); if (j>−1) goto ④; // 组合宽度匹配JWFFPlaceableFirst(ii,I,e k,n/6,j); if (j>−1) goto ④; //可装入优先PF③合并E中的边,转②;④将I集合中第j位元素对应的矩形件I(j)装入在E中的最低水平线e k上;如果未旋转,则H packing=max(H packing,I(j).x+I(j).h),否则,H packing=max(H packing,I(j).x+I(j).w);更新集合I和E;如果j>ii,则转②;endend其中,InitCoordinate(I)是对所有矩形块的坐标x,y进行初始化.在初始阶段,由于所有的矩形都未进行装入, InitCoordinate(I)将其坐标x,y全部初始化为−1,即对任意i∈[1,n],I(i).x=I(i).y=−1.InitContours(E)对装箱轮廓线进行初始化.在初始阶段,由于容器C未被装入,因此,其轮廓线仅由一个水平线线段构成,即E={e1},其中,e1={0,0,W},W为容器C的宽度.FullFitFirst,WidthFitFirst,HeightFitFirst,JointWidthFitFirst,PlaceableFirst分别表示按照FFF,WFF,HFF, JWFF以及PF等启发式规则在队列I中第ii位开始向后依次查找与最低水平线e k相匹配矩形的查询过程,其返回值为j.其中,JointWidthFitFirst,PlaceableFirst中的查询范围分别设置为7,n/6,为多次实验后取得的经验值.1.4 LLABF算法举例图2(a)是5个矩形构成的一个完美装箱图,其装箱高度H packing等于最优高度H opt,空间的利用率为100%.对于BL,IBL,LFLA以及LHL算法,要实现图2(a)的完美装箱(不考虑旋转),其装入序列就必须为1 2 3 4 5.对于序列为1 3 4 5 2和1 3 2 4 5,其装箱结果分别如图2 (b)和图2(c)所示,这两种装入序列都会产生较大的空洞.(a)(b) (c)Fig.2 Packing result according to BL, IBL, LFLA or LHL algorithm图2 BL,IBL,LFLA或LHL算法的装箱结果图对于同样的装入序列,采用LLABF算法则能得到如图2(a)所示的完美装箱.图3显示了装入序列为1 3 4 5蒋兴波 等:二维矩形条带装箱问题的底部左齐择优匹配算法15332、采用LLABF 算法得到的装箱结果(其中,*表示该位置上的矩形已经装入容器中).初始化:E ={e 1},e 1={0,0,W };对任意i ∈[1,n ],I (i ).x =I (i ).y =−1; 开始阶段虽然所有矩形都不满足完全匹配FFF 、宽度匹配WFF 以及高度匹配,但I (1).w +I (5).w =e 1.w ,满足两两组合宽度匹配JWFF,所以将编号为1的矩形装入在矩形容器C 的左下角;装入结果如图3(c)所示,此时,E ={e 1,e 2};取最低水平线e 2,按照LLABF 启发式规则依次查询匹配矩形,其中I (5),即编号为2的矩形满足WFF,故将其装入在e 2上;装入结果如图3(d)所示,此时,E ={e 1,e 2};以此类推,其装入过程如图3(e)~图3(g)所示.根据LLABF 算法,如果装入序列符合1 2 3 * *或者1 3 * * * 模式(其中,*代表未列出的矩形编号),其结果都与1 2 3 4 5序列的装箱结果相同,即都能得到如图3(a)所示的完美装箱.在图3中,满足第1种模式的装入序列包括1 2 3 4 5,1 2 3 5 4两种,满足第2种模式的装入序列包括1 3 2 4 5,1 3 4 2 5,1 3 2 5 4,1 3 5 2 4,1 3 5 4 2,1 3 4 5 2这6种.针对图2中的5个矩形的装箱问题,LLABF 找到最优解的概率是BL,IBL,LFLA 以及LHL 的8倍之多.因此,相对于传统布局算法,LLABF 算法更容易找到最优解.Fig.3 Packing result according to LLABF algorithm图3 LLABF 算法的装箱结果图2 GA +LLABF 算法实现2.1 算法的总体流程 本文采用遗传算法GA 与LLABF 相结合的方式来解决二维矩形条带装箱问题.其中,GA 用来实现矩形装入序列的初步确定,LLABF 则根据自身的启发式规则将矩形装入到矩形容器中.GA+LLABF 的算法流程如下所示.(1) 个体染色体编码.(2) k =0;随机产生m 个个体组成初始群体pop (k )={I 1,I 2,…,I m }.(3) 对单个个体I i 执行LLABF(Ii ,H packing );记录个体适应度f (I i )(f (I i )=1/H packing ).(4) 判断是否满足停止条件.若是,则停止计算,输出最佳结果;否则,继续.(5) 利用排序选择操作选择m 个个体形成新的群体selpop (k +1).(6) 根据交叉概率P c 进行交叉操作,产生群体crosspop (k +1).(a) (b) 1 3 4 5 2(c) * 3 4 5 2 (d) * 3 4 5 * (e) * * 4 5 * (f) * * * 5 * (g) * * * * *1534 Journal of Software软件学报 V ol.20, No.6, June 2009(7) 根据变异概率P m进行变异操作,产生群体mutpop(k+1).(8) pop(k+1)=mutpop(k+1);k=k+1;转(3),循环.2.2 编码及适应度函数矩形装箱采用整数编码(encoding),即n个矩形分别用整数1,2,…,n编号,矩形的一个装箱方案对应于一个染色体编码,I j=(π1,π2,…,πn),其中,|πi|表示矩形编号(1≤|πi|≤n),当πi<0时,矩形旋转90°,否则不旋转;I j表示第j个个体.例如:个体I j的染色体编码为(−5 3 −7 6 1 10 8 −2 4 −9),表示装入序列为5 3 7 6 1 10 8 2 4 9.在装入过程中,编号为5,7,2,9的矩形进行90°旋转.适应度函数(fitness function)可以由装箱高度的倒数表示,即f(I j)=1/H packing(I j).2.3 选择为了避免丢失上一代产生的最佳个体,本文在采用排序选择方法(rank-based select model)的同时,还混合使用了最优保存策略(elitist model).排序选择方法主要依据个体适应度值之间的大小关系,对个体适应度是否取正值或负值以及个体适应度之间的数值差异程度并无特别要求.其步骤如下:(1) 对群体中所有个体按照适应度从大到小排序.(2) 根据下列公式计算每个个体的选择概率:()iP=α×(1−α/m)I,sP表示排列在第i个位置的个体的选择概率,m表示群体大小,α为调节参数.其中,()is(3) 根据步骤(2)计算得出的个体概率值作为其能够被遗传到下一代的概率,基于该值应用比例选择(赌盘选择)的方法产生下一代群体.2.4 交叉(crossover)交叉是产生新个体的主要方法.本文主要采用环形部分交叉(circular-based part crossover):①随机生成起始交叉点P cr∈[1,n];②随机生成交叉长度L cr∈[1,n];③将P cr后的L cr位基因进行交换,如果P cr+L cr>n,则将染色体前P cr+L cr−n位基因进行互换;④然后依次将未出现的基因填入空白基因座.2.5 变异(mutation)变异是产生新个体的辅助方法,它决定了遗传算法的局部搜索能力,保持群体的多样性.本文中的变异包括两部分:一是旋转变异,二是装入序列变异.(1) 旋转变异旋转变异采用了单点取反和环形部分取反两种方式.单点取反(single point reversion)变异:①随机生成变异点P mu∈[1,n];②取反.例如,对于个体I j=(π1,π2,…, πi,…,πn),P mu=i,则变异后产生的新个体为I′=(π1,π2,…,−πi,…,πn).j环形部分取反(circular-based part reversion)变异:①随机生成变异点P mu∈[1,n];②随机生成变异长度L mu∈[1,n];③将P mu后的L mu位取反,如果P mu+L mu>n,则将染色体首部的P mu+L mu−n位基因取反.(2) 装入序列变异装入序列变异采用了两点位置互换与环形部分逆转两种方式.两点位置互换(two point exchange)变异:①随机生成两个变异点i,j∈[1,n];②将两个基因互换.例如:I j=(π1, π2,…,πi1,…,πi2,…,πn),i1,i2∈[1,n],则变异后产生的新个体为I′=(π1,π2,…,πi2,…,πi1,…,πn).j环形部分逆转(circular-based part inversion)变异:①随机生成变异点P mu∈[1,n];②随机生成变异长度L mu∈[1,n];③将P mu后的L mu位逆转,即将(P mu+i) mod n(即P mu+i对n取模)与(P mu+L mu−i) mod n互换,i∈[0,L mu/2].蒋兴波等:二维矩形条带装箱问题的底部左齐择优匹配算法15353 实验结果实验平台:Pentium 4 3.0GHz,512M,Windows Server 2003.运行参数:交叉概率P c=0.95,变异概率P m=0.85.本文的实验针对两组标准测试问题来进行,第1组来自文献[11],第2组来自文献[16].3.1 实验1文献[11]给出了两组标准测试实例,均由40×15的大矩形切割而成.其中,第1组实例由25个矩形组成,第2组实例由50个矩形组成.本文实验中,GA的群体大小和运行代数参照文献[12],分别设为20和100.对每组实例皆重复运行100次.实验结果对比见表1.Table 1Computational results of four algorithms表14种算法的计算结果Jakobs S[11] GA+BL Liu DQ[12]GA+IBLBortfeldt A[10]SPGALGA+LLABFW×H nH min H avg H min H avg H min H avg H min H avg H max40×15 25 17 17.481616.9716 16.001515.001540×15 50 17 17.281617.0115 15.001515.0015 表1中,H min,H max,H avg分别指多次运行中得到的最小H packing、最大H packing及平均H packing.由表1可以看出,GA+LLABF算法在对2组标准实例进行的100次测试中均找到了最优解15,而其他3种算法中,除了SPGAL找到了第2组数据集的最优解之外,其余算法求得的H packing均大于H opt.因此,实验结果表明,本文的GA+LLABF算法更加有效.3.2 实验2在文献[16]中,Hopper等人给出了21组不同尺寸的测试实例,这些实例分成7个大类,每个大类由3组实例组成,其矩形个数从16个~197个不等.每个实例的最优高度H opt已经给出,其相关数据见文献[16].在本文的算法中,初始种群大小参照文献[16],设为50,终止条件为进化代数等于 2 500代,或者装箱高度H packin等于最优解H opt.对每组测试实例重复运行10次,记录每个大类30次(每个大类3个实例,每个实例进行10次测试)测试的平均装箱高度到最优解的相对距离RDBSOH,RDBSOH(%)=100×(30次平均H packing−H opt)/H opt.实验结果对比见表2.Table 2Computational results of 12 algorithms (relative distance of best solution to optimum height (%))表2 12种算法的计算结果(RDBSOH(%))Algorithm C1 C2 C3 C4 C5 C6 C7 AverageGA+BLF[16] 4 7 5 3 4 4 5 4.57 SA+BLF[16] 4 6 5 3 3 3 4 4.00 GRASP+VNS[7]14.40 17.33 12.93 6.80 4.51 3.55 2.89 8.92 HR[3]8.33 4.45 6.67 2.22 1.85 2.5 1.8 3.97 Hybrid SA[15] 1.66 4.88 4.00 3.94 3.18 3.30 3.38 3.48SA+HR[14] 5.00 4.47 2.23 2.22 1.86 2.5 3.24 3.07 PH[9] 5.00 4.44 4.44 3.33 1.11 1.11 1.25 2.95HRBB[6] 1.70 0.00 1.10 2.20 1.90 1.40 1.30 1.40GRASP[8]0.00 0.00 1.08 1.64 1.10 1.56 1.36 1.33SPGAL[10] 1.70 0.90 2.20 1.40 0.00 0.70 0.50 1.00 A1[5]0.00 0.00 1.11 1.67 1.11 0.83 0.42 0.73 GA+LLABF 0.00 0.00 0.00 0.00 0.00 0.00 0.40 0.06 从表2可以看出,在现有的算法中,按照Averag e即平均RDBSOH,位居前4位的较优算法依次为A1, SPGAL,GRASP与HRBB,它们对7大类实例计算得出的Average分别为0.73,1.0,1.33和1.4.而本文给出的GA+LLABF算法计算得出的Average为0.06,其中6大类实例的RDBSOH为0,即对应的所有实例其10次实验结果皆取得了最优解.因此,实验结果进一步表明,针对2DR-SPP,本文提出的GA+LLABF算法优于其他算法.1536Journal of Software 软件学报 V ol.20, No.6, June 2009表3给出了GRASP+VNS,GRASP,HRBB 以及本文的GA+LLABF 对21组实例求得的实际测试结果H packing .在GA+LLABF 算法对21组实例的测试中,10次实验皆能获得最优解H opt 的有18组(21组中,除C 71,C 72,C 73外),占全部实例的86%(18/21),而GRASP 为38%(8/21)、HRBB 为33%(7/21);10次实验中至少获得1次最优解的实例有19组(即最小高度H min =最优高度H opt ),占全部实例的91%(19/21),而GRASP 为38%(8/21)、HRBB 为43%(9/21);10次实验获得的最大高度H max 与最优解H opt 之间的距离最多为1个基本单位.因此,无论是最优解所占比例还是最小高度H min 、平均高度H avg 以及最大高度H max ,GA+LLABF 求得的结果均具有明显的优势.Table 3 Computational results (H packing ) of 21 instances表3 21组实例计算结果 Beltrán DJ GRASP+VNS [7] (20 runs) Alvarez-Valdes R GRASP [8] (10 runs) Cui YD HRBB [6] (6 runs) GA+LLABF(20 runs) CategoryH opt n H min H avg H max H min H avg H min H avg H max H min H avg H max C 1120 16 21 22.30 23 20 20.00 2020.0020 20 20.00 20 C 1220 17 21 23.20 25 20 20.00 2121.0021 20 20.00 20 C 1320 16 22 23.15 24 20 20.00 2020.0020 20 20.00 20 C 2115 25 16 17.20 19 15 15.00 1515.0015 15 15.00 15 C 2215 25 16 18.00 20 15 15.00 1515.0015 15 15.00 15 C 2315 25 16 17.60 20 15 15.00 1515.0015 15 15.00 15 C 3130 28 32 32.55 34 30 30.00 3030.0030 30 30.00 30 C 3230 29 32 34.25 37 31 31.00 3131.0031 30 30.00 30 C 3330 28 33 34.85 37 30 30.00 3030.0030 30 30.00 30 C 4160 49 63 63.55 66 61 61.00 6060.5061 60 60.00 60 C 4260 49 61 64.60 68 61 61.00 6161.3362 60 60.00 60 C 4360 49 62 64.10 66 61 61.00 6161.0061 60 60.00 60 C 5190 73 92 93.45 95 91 91.00 9090.6791 90 90.00 90 C 5290 73 93 94.75 97 91 91.00 9292.0092 90 90.00 90 C 5390 73 92 94.00 98 91 91.00 9191.1792 90 90.00 90 C 61120 97 123 124.40 130121121.90121121.00121120 120.00 120C 62120 97 123 124.65 128121121.90121121.83122120 120.00 120C 63120 97 122 123.75 126121121.90121121.33122120 120.00 120C 71240 196 244 246.45 248244244.00242242.17243241 241.00 241C 72240 197 244 247.05 255242242.90245245.00245241 241.00 241C 73 240 196 245 247.30 258243243.00241241.33242240 240.90 241图4(H packing =H opt =120)、图5(H packing =H opt =240)显示了由GA+LLABF 算法求得的部分实例装箱图,其装箱高度皆为最优高度.Fig.4 Packing result of C 63 Fig.5 Packing result of C 73图4 C 63的装箱结果图 图5 C 73的装箱结果图 LLABF 算法在对矩形的装入过程中,每一步均按照FFF,WFF,HFF,JWFF 以及PF 规则依次查询与最低水平蒋兴波等:二维矩形条带装箱问题的底部左齐择优匹配算法1537线相匹配的矩形.对于FFF,如果某个阶段存在i个待装矩形,那么最坏情况下将比较i次;其余4个启发式规则除了JWFF的比较次数为常数K以外,皆与完全匹配优先类似,因此,单步比较次数最坏情况下为4i+K.将所有n 个矩形全部装入容器中,总的比较次数最坏情况下为4×(n+n−1+n−2+…+2+1)+n×K,共2n×(n+1)+n×K,因此其复杂度为O(n2).对于矩形在容器中的装入,主要的比较次数在于查找最低水平线,而最低水平线的数量不会超过n,所以,其复杂度最坏情况下为O(n2).整体上,LLABF的时间复杂度为O(n2).同时,对于GA,由于群体规模和进化代数皆为常数,其本身的比较次数也为常数,所以GA+LLABF算法的复杂度主要由LLABF决定,即最坏情况下为O(n2).表4给出了表2中所有算法的计算时间.由于算法的实现方式、测试平台与环境的不同,要对算法的快慢进行精确比较是不科学也是不可行的.但是从表4中,我们能够大致看出各种算法的运行快慢程度.由于本文算法采用了装箱高度等于最优解作为终止运行条件之一,因此,虽然C5的规模大于C4,但其获得最优解的速度明显快于C4,所以运行时间相对较小.Table 4Run times of 12 algorithms (s)表412种算法的运行时间(s)Algorithm C1 C2 C3 C4 C5 C6 C7 Averagea[16]a SA+BLF[16]42.00 144.00 240.00 1 980.00 6 900.0022 920.00250 860.00 40 441.86b GRASP+VNS[7] 0.00 0.00 0.00 0.00 0.02 0.07 1.37 0.21c HR[3]0.000.000.03 0.14 0.69 2.21 36.07 5.59dHybrid SA[15] 3.64 6.36 18.78101.20287.27 757.20 1650.6 403.57e SA+HR[14] 23.20 42.80 59.20170.00343.60 444.90 1328.80 344.64f PH[9]0.00 0.00 0.00 1.51 5.69 23.74 707.12 105.45g HRBB[6] 0.27 0.33 0.88 2.19 2.83 2.24 4.26 1.86h GRASP[8]- - -- ---60.00i SPGAL[10]--- ----139.00j A1[5]0.370.611.71 0.15 0.40 3.98 45.02 7.46GA+LLABF0.000.032.233.6 1.3 33.2 328.3 52.67a—Pentium Pro 200MHz and 65MB memory c—Dell GX260 with a 2.4GHz CPUe—Dell GX270 with a 3.0GHz CPU g—Pentium 4 2.8GHZ, 512MB memory i—Pentium PC with 2GHz b—Pentium 166MHz and 96MB RAM d—Pentium Ⅲ 797MHZf—Pentium 4 1.6GHZ, 256MB memory h—Pentium 4 mobile 2.0GHZ, 512MB memory j—2.4GHz PC with 512MB memory4 结论与展望本文分析了传统布局算法的不足,提出了一种启发式布局算法LLABF,并将其与GA相结合.实验结果表明,GA+LLABF算法解决2DR-SPP问题更加有效.但是要评价一种算法的优劣,除了要考虑算法的求解结果以外,还应兼顾算法的运行效率.因此,改进算法以进一步提高运算速度,将是我们下一步的研究重点.References:[1] Hifi M. Exact algorithms for the guillotine strip cutting/packing problem. Computers & Operations Research, 1998,25(11):925−940.[2] Lesh N, Marks J, McMahon A, Mitzenmacher M. Exhaustive approaches to 2D rectanglar perfect packings. Information ProcessingLetters, 2004,90:7−14.[3] Zhang DF, Kang Y, Deng AS. A new heuristic recursive algorithm for the strip rectangular packing problem. Computers &Operations Research, 2006,33(8):2209−2217.[4] Chen DB, Huang WQ. Greedy algorithm for recatngle-packing problem. Computer Engineering, 2007,33(4):160−162 (in Chinesewith English abstract).[5] Chen M, Huang WQ. A two-level search algorithm for 2D rectangular packing problem. Computers & Industrial Engineering, 2007,53(1):123−136.。
中序遍历英文缩写

中序遍历英文缩写Binary trees are a fundamental data structure in computer science, and one of the most important operations performed on them is the traversal of their nodes. The in-order traversal, also known as the "central" or "symmetric" traversal, is a specific method of traversing a binary tree. This traversal technique visits the left subtree, then the root node, and finally the right subtree, resulting in a sequence of nodes that is sorted in ascending order.The in-order traversal of a binary tree is denoted by the abbreviation "InOr" or "I". This abbreviation is commonly used in various algorithms and data structures that involve binary trees, and it is important for developers and computer scientists to understand its significance.One of the key applications of in-order traversal is in the representation and manipulation of mathematical expressions. Binary trees can be used to represent mathematical expressions, with the internal nodes representing operators and the leaf nodes representing operands. By performing an in-order traversal of such a tree, we can recover the original expression in its standard infix notation, where the operators are placed between their operands.For example, consider the expression "(A + B) * (C - D)". This expression can be represented as a binary tree, with the root node representing the multiplication operator, the left subtree representing the addition of A and B, and the right subtree representing the subtraction of C and D. By performing an in-order traversal of this tree, we can recover the original expression in its infix form.In addition to its use in expression representation, in-order traversal is also important in the implementation of various binary tree-based data structures, such as binary search trees (BSTs). In a BST, the in-order traversal of the tree will visit the nodes in ascending order of their values, which can be useful for efficient searching, insertion, and deletion operations.Furthermore, in-order traversal is a fundamental technique used in the design and analysis of algorithms that operate on binary trees. Many algorithms, such as those for finding the minimum or maximum value in a BST, rely on the properties of in-order traversal to ensure their correctness and efficiency.To perform an in-order traversal of a binary tree, the following recursive algorithm can be used:1. Traverse the left subtree (if it exists)2. Visit the root node3. Traverse the right subtree (if it exists)This algorithm can be implemented in various programming languages, and it is a common interview question for aspiring computer scientists and software engineers.In conclusion, the in-order traversal of binary trees is a crucial concept in computer science, with applications in the representation and manipulation of mathematical expressions, the implementation of binary tree-based data structures, and the design and analysis of algorithms that operate on binary trees. Understanding the in-order traversal and its associated abbreviation "InOr" or "I" is essential for any developer or computer scientist working with binary trees and related data structures.。
- 1、下载文档前请自行甄别文档内容的完整性,平台不提供额外的编辑、内容补充、找答案等附加服务。
- 2、"仅部分预览"的文档,不可在线预览部分如存在完整性等问题,可反馈申请退款(可完整预览的文档不适用该条件!)。
- 3、如文档侵犯您的权益,请联系客服反馈,我们会尽快为您处理(人工客服工作时间:9:00-18:30)。
just did this
int N = Integer.parseInt(args[0]); int width = 512; int height = (int) (2 * width / Math.sqrt(3)); double size = width / Math.pow(3.0, N); StdDraw.create(width, height); StdDraw.go(0, width * Math.sqrt(3) / 2); StdDraw.penDown(); koch(N, size); StdDraw.rotate(-120); koch(N, size); StdDraw.rotate(-120); koch(N, size); draw it StdDraw.show(); } }
•
http://w w w .cs.Pri /IntroCS
2
Greatest Common Divisor
Find largest integer d that evenly divides into p and q.
"p if q = 0 gcd( p, q) = # $ gcd(q, p % q) otherwise
! ! ! ! ! ! !
n=0
n=1
!
n=2
n=3
Java implementation.
public static int gcd(int p, int q) { if (q == 0) return p; else return gcd(q, p % q); }
base case reduction step
base case reduction step, converges to base case
Greatest Common Divisor
Find largest integer d that evenly divides into p and q.
"p if q = 0 gcd( p, q) = # $ gcd(q, p % q) otherwise
public static void koch(int n, double size) { if (n == 0) StdDraw.goForward(size); else { koch(n-1, size); StdDraw.rotate(60); koch(n-1, size); StdDraw.rotate(-120); koch(n-1, size); StdDraw.rotate(60); koch(n-1, size); } }
p q q
p % q
Applications.
! !
x
x
x
x
x
x
x gcd
x
!
Simplify fractions: 1272/4032 = 53/168. RSA cryptosystem (stay tuned). History of algorithms.
Euclid, 300 BCE
3
p = 8x q = 3x gcd(p, q) = x
Toweon
public class Hanoi { public static void hanoi(int n, String from, String temp, String to) { if (n == 0) return; hanoi(n-1, from, to, temp); System.out.println("Move disc " + n + " from " + from + " to " + to); hanoi(n-1, temp, from, to); } public static void main(String[] args) { int N = Integer.parseInt(args[0]); hanoi(N, "A", "B", "C"); } }
5 6
Koch Curve: Recursion Tree
n 2
Koch Snowflake
Koch snowflake of order n. Draw Koch curve of order n. Turn -120º. Draw Koch curve of order n. Turn -120º. Draw Koch curve of order n.
compute parameters
Start
Finish
Towers of Hanoi demo
Edouard Lucas (1883)
11 12
Towers of Hanoi: Recursive Solution A B C
Towers of Hanoi Legend
Is world going to end (according to legend)? 40 golden discs on 3 diamond pegs. World ends when certain group of monks accomplish task.
! ! !
Closely related to mathematical induction.
Finish
Drawing Hands M. C. Escher, 1948
Introducti on to Computer Sci ence
•
Robert Sedgew i ck and Kevi n Wayne
Koch Snowflake
Koch curve of order n. Draw curve of order n-1. Turn 60º. Draw curve of order n-1. Turn -120º. Draw curve of order n-1. Turn 60º. Draw curve of order n-1.
% java Hanoi 3 Move disc 1 from Move disc 2 from Move disc 1 from Move disc 3 from Move disc 1 from Move disc 2 from Move disc 1 from
A A C A B B A
to to to to to to to
C B B C A C C
% java Hanoi 4 Move disc 1 from Move disc 2 from Move disc 1 from Move disc 3 from Move disc 1 from Move disc 2 from Move disc 1 from Move disc 4 from Move disc 1 from Move disc 2 from Move disc 1 from Move disc 3 from Move disc 1 from Move disc 2 from Move disc 1 from
! !
Q. Will computer algorithms help?
Move N-1 smallest discs to pole B.
Move largest disc to pole C.
Move N-1 smallest discs to pole C.
14 15
Towers of Hanoi: Recursive Solution
A A B A C C A A B B C B A A B
to to to to to to to to to to to to to to to
B C C B A B B C C A A C B C C
subdivisions of ruler
16
17
Towers of Hanoi: Recursion Tree
1, ABC
move 1 from A to C
Recursive algorithm yields non-recursive solution! Alternate between two moves: – move smallest disc to right (left) if N is even (odd) – make only legal move not involving smallest disc
!
0, ACB
Overview
2.7: Recursion
What is recursion? When one function calls itself directly or indirectly. Why learn recursion? New mode of thinking. Powerful programming tool. Divide-and-conquer paradigm.
! ! ! ! !
L 1 1
R 1
L 1
L 0 0
R 0
L 0 0
L 0
R 0
L 0 0
L 0
R 0
L 0 0
L 0
R 0
L 0
L
R
L
L
L
R
L
R
60º
L
R
L
L
L
R
L
L: Rotate
R: Rotate -120º
7 10
Koch Snowflake in Java
Towers of Hanoi
Move all the discs from the leftmost peg to the rightmost one. Only one disc may be moved at a time. A disc can be placed either on empty peg or on top of a larger disc.