Connectivity reflects coding a model of voltage-based STDP with homeostasis
人工智能导论考核试卷

3. CNN通过卷积和池化操作提取图像特征,降低参数数量,提高模型泛化能力,从而提高图像识别准确性。
4.伦理问题:隐私保护、算法偏见、责任归属。解决策略:制定伦理准则、透明度提升、多样化团队、责任追溯机制。
1.人工智能包括以下哪些技术领域?()
A.机器学习
B.语音识别
C.量子计算
D.数据挖掘
E.虚拟现实
2.以下哪些属于监督学习算法?()
A.支持向量机
B.决策树
C. K-均值聚类
D.线性回归
E.随机森林
3.深度学习中的卷积神经网络(CNN)主要用于哪些任务?()
A.图像分类
B.语音识别
C.自然语言处理
D.视频分析
人工智能导论考核试卷
考生姓名:__________答题日期:__________得分:__________判卷人:__________
一、单项选择题(本题共20小题,每小题1分,共20分,在每小题给出的四个选项中,只有一项是符合题目要求的)
1.以下哪个不是人工智能的研究领域?()
A.机器学习
B.深度学习
D.随机森林
E.支持向量回归
9.以下哪些是推荐系统中的冷启动问题?()
A.用户冷启动
B.项目冷启动
C.模型冷启动
D.数据冷启动
E.系统冷启动
10.以下哪些是迁移学习的主要挑战?()
A.数据分布差异
B.标签空间不匹配
C.模型泛化能力不足
D.源域数据不足
E.目标域数据过拟合
人工智能领域中英文专有名词汇总
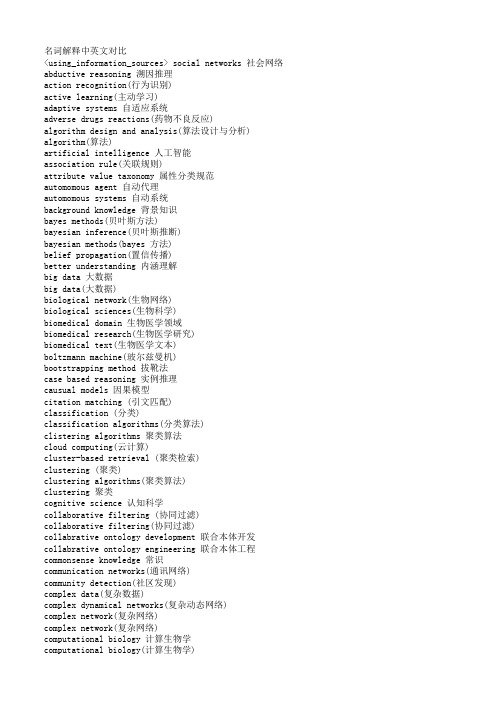
名词解释中英文对比<using_information_sources> social networks 社会网络abductive reasoning 溯因推理action recognition(行为识别)active learning(主动学习)adaptive systems 自适应系统adverse drugs reactions(药物不良反应)algorithm design and analysis(算法设计与分析) algorithm(算法)artificial intelligence 人工智能association rule(关联规则)attribute value taxonomy 属性分类规范automomous agent 自动代理automomous systems 自动系统background knowledge 背景知识bayes methods(贝叶斯方法)bayesian inference(贝叶斯推断)bayesian methods(bayes 方法)belief propagation(置信传播)better understanding 内涵理解big data 大数据big data(大数据)biological network(生物网络)biological sciences(生物科学)biomedical domain 生物医学领域biomedical research(生物医学研究)biomedical text(生物医学文本)boltzmann machine(玻尔兹曼机)bootstrapping method 拔靴法case based reasoning 实例推理causual models 因果模型citation matching (引文匹配)classification (分类)classification algorithms(分类算法)clistering algorithms 聚类算法cloud computing(云计算)cluster-based retrieval (聚类检索)clustering (聚类)clustering algorithms(聚类算法)clustering 聚类cognitive science 认知科学collaborative filtering (协同过滤)collaborative filtering(协同过滤)collabrative ontology development 联合本体开发collabrative ontology engineering 联合本体工程commonsense knowledge 常识communication networks(通讯网络)community detection(社区发现)complex data(复杂数据)complex dynamical networks(复杂动态网络)complex network(复杂网络)complex network(复杂网络)computational biology 计算生物学computational biology(计算生物学)computational complexity(计算复杂性) computational intelligence 智能计算computational modeling(计算模型)computer animation(计算机动画)computer networks(计算机网络)computer science 计算机科学concept clustering 概念聚类concept formation 概念形成concept learning 概念学习concept map 概念图concept model 概念模型concept modelling 概念模型conceptual model 概念模型conditional random field(条件随机场模型) conjunctive quries 合取查询constrained least squares (约束最小二乘) convex programming(凸规划)convolutional neural networks(卷积神经网络) customer relationship management(客户关系管理) data analysis(数据分析)data analysis(数据分析)data center(数据中心)data clustering (数据聚类)data compression(数据压缩)data envelopment analysis (数据包络分析)data fusion 数据融合data generation(数据生成)data handling(数据处理)data hierarchy (数据层次)data integration(数据整合)data integrity 数据完整性data intensive computing(数据密集型计算)data management 数据管理data management(数据管理)data management(数据管理)data miningdata mining 数据挖掘data model 数据模型data models(数据模型)data partitioning 数据划分data point(数据点)data privacy(数据隐私)data security(数据安全)data stream(数据流)data streams(数据流)data structure( 数据结构)data structure(数据结构)data visualisation(数据可视化)data visualization 数据可视化data visualization(数据可视化)data warehouse(数据仓库)data warehouses(数据仓库)data warehousing(数据仓库)database management systems(数据库管理系统)database management(数据库管理)date interlinking 日期互联date linking 日期链接Decision analysis(决策分析)decision maker 决策者decision making (决策)decision models 决策模型decision models 决策模型decision rule 决策规则decision support system 决策支持系统decision support systems (决策支持系统) decision tree(决策树)decission tree 决策树deep belief network(深度信念网络)deep learning(深度学习)defult reasoning 默认推理density estimation(密度估计)design methodology 设计方法论dimension reduction(降维) dimensionality reduction(降维)directed graph(有向图)disaster management 灾害管理disastrous event(灾难性事件)discovery(知识发现)dissimilarity (相异性)distributed databases 分布式数据库distributed databases(分布式数据库) distributed query 分布式查询document clustering (文档聚类)domain experts 领域专家domain knowledge 领域知识domain specific language 领域专用语言dynamic databases(动态数据库)dynamic logic 动态逻辑dynamic network(动态网络)dynamic system(动态系统)earth mover's distance(EMD 距离) education 教育efficient algorithm(有效算法)electric commerce 电子商务electronic health records(电子健康档案) entity disambiguation 实体消歧entity recognition 实体识别entity recognition(实体识别)entity resolution 实体解析event detection 事件检测event detection(事件检测)event extraction 事件抽取event identificaton 事件识别exhaustive indexing 完整索引expert system 专家系统expert systems(专家系统)explanation based learning 解释学习factor graph(因子图)feature extraction 特征提取feature extraction(特征提取)feature extraction(特征提取)feature selection (特征选择)feature selection 特征选择feature selection(特征选择)feature space 特征空间first order logic 一阶逻辑formal logic 形式逻辑formal meaning prepresentation 形式意义表示formal semantics 形式语义formal specification 形式描述frame based system 框为本的系统frequent itemsets(频繁项目集)frequent pattern(频繁模式)fuzzy clustering (模糊聚类)fuzzy clustering (模糊聚类)fuzzy clustering (模糊聚类)fuzzy data mining(模糊数据挖掘)fuzzy logic 模糊逻辑fuzzy set theory(模糊集合论)fuzzy set(模糊集)fuzzy sets 模糊集合fuzzy systems 模糊系统gaussian processes(高斯过程)gene expression data 基因表达数据gene expression(基因表达)generative model(生成模型)generative model(生成模型)genetic algorithm 遗传算法genome wide association study(全基因组关联分析) graph classification(图分类)graph classification(图分类)graph clustering(图聚类)graph data(图数据)graph data(图形数据)graph database 图数据库graph database(图数据库)graph mining(图挖掘)graph mining(图挖掘)graph partitioning 图划分graph query 图查询graph structure(图结构)graph theory(图论)graph theory(图论)graph theory(图论)graph theroy 图论graph visualization(图形可视化)graphical user interface 图形用户界面graphical user interfaces(图形用户界面)health care 卫生保健health care(卫生保健)heterogeneous data source 异构数据源heterogeneous data(异构数据)heterogeneous database 异构数据库heterogeneous information network(异构信息网络) heterogeneous network(异构网络)heterogenous ontology 异构本体heuristic rule 启发式规则hidden markov model(隐马尔可夫模型)hidden markov model(隐马尔可夫模型)hidden markov models(隐马尔可夫模型) hierarchical clustering (层次聚类) homogeneous network(同构网络)human centered computing 人机交互技术human computer interaction 人机交互human interaction 人机交互human robot interaction 人机交互image classification(图像分类)image clustering (图像聚类)image mining( 图像挖掘)image reconstruction(图像重建)image retrieval (图像检索)image segmentation(图像分割)inconsistent ontology 本体不一致incremental learning(增量学习)inductive learning (归纳学习)inference mechanisms 推理机制inference mechanisms(推理机制)inference rule 推理规则information cascades(信息追随)information diffusion(信息扩散)information extraction 信息提取information filtering(信息过滤)information filtering(信息过滤)information integration(信息集成)information network analysis(信息网络分析) information network mining(信息网络挖掘) information network(信息网络)information processing 信息处理information processing 信息处理information resource management (信息资源管理) information retrieval models(信息检索模型) information retrieval 信息检索information retrieval(信息检索)information retrieval(信息检索)information science 情报科学information sources 信息源information system( 信息系统)information system(信息系统)information technology(信息技术)information visualization(信息可视化)instance matching 实例匹配intelligent assistant 智能辅助intelligent systems 智能系统interaction network(交互网络)interactive visualization(交互式可视化)kernel function(核函数)kernel operator (核算子)keyword search(关键字检索)knowledege reuse 知识再利用knowledgeknowledgeknowledge acquisitionknowledge base 知识库knowledge based system 知识系统knowledge building 知识建构knowledge capture 知识获取knowledge construction 知识建构knowledge discovery(知识发现)knowledge extraction 知识提取knowledge fusion 知识融合knowledge integrationknowledge management systems 知识管理系统knowledge management 知识管理knowledge management(知识管理)knowledge model 知识模型knowledge reasoningknowledge representationknowledge representation(知识表达) knowledge sharing 知识共享knowledge storageknowledge technology 知识技术knowledge verification 知识验证language model(语言模型)language modeling approach(语言模型方法) large graph(大图)large graph(大图)learning(无监督学习)life science 生命科学linear programming(线性规划)link analysis (链接分析)link prediction(链接预测)link prediction(链接预测)link prediction(链接预测)linked data(关联数据)location based service(基于位置的服务) loclation based services(基于位置的服务) logic programming 逻辑编程logical implication 逻辑蕴涵logistic regression(logistic 回归)machine learning 机器学习machine translation(机器翻译)management system(管理系统)management( 知识管理)manifold learning(流形学习)markov chains 马尔可夫链markov processes(马尔可夫过程)matching function 匹配函数matrix decomposition(矩阵分解)matrix decomposition(矩阵分解)maximum likelihood estimation(最大似然估计)medical research(医学研究)mixture of gaussians(混合高斯模型)mobile computing(移动计算)multi agnet systems 多智能体系统multiagent systems 多智能体系统multimedia 多媒体natural language processing 自然语言处理natural language processing(自然语言处理) nearest neighbor (近邻)network analysis( 网络分析)network analysis(网络分析)network analysis(网络分析)network formation(组网)network structure(网络结构)network theory(网络理论)network topology(网络拓扑)network visualization(网络可视化)neural network(神经网络)neural networks (神经网络)neural networks(神经网络)nonlinear dynamics(非线性动力学)nonmonotonic reasoning 非单调推理nonnegative matrix factorization (非负矩阵分解) nonnegative matrix factorization(非负矩阵分解) object detection(目标检测)object oriented 面向对象object recognition(目标识别)object recognition(目标识别)online community(网络社区)online social network(在线社交网络)online social networks(在线社交网络)ontology alignment 本体映射ontology development 本体开发ontology engineering 本体工程ontology evolution 本体演化ontology extraction 本体抽取ontology interoperablity 互用性本体ontology language 本体语言ontology mapping 本体映射ontology matching 本体匹配ontology versioning 本体版本ontology 本体论open government data 政府公开数据opinion analysis(舆情分析)opinion mining(意见挖掘)opinion mining(意见挖掘)outlier detection(孤立点检测)parallel processing(并行处理)patient care(病人医疗护理)pattern classification(模式分类)pattern matching(模式匹配)pattern mining(模式挖掘)pattern recognition 模式识别pattern recognition(模式识别)pattern recognition(模式识别)personal data(个人数据)prediction algorithms(预测算法)predictive model 预测模型predictive models(预测模型)privacy preservation(隐私保护)probabilistic logic(概率逻辑)probabilistic logic(概率逻辑)probabilistic model(概率模型)probabilistic model(概率模型)probability distribution(概率分布)probability distribution(概率分布)project management(项目管理)pruning technique(修剪技术)quality management 质量管理query expansion(查询扩展)query language 查询语言query language(查询语言)query processing(查询处理)query rewrite 查询重写question answering system 问答系统random forest(随机森林)random graph(随机图)random processes(随机过程)random walk(随机游走)range query(范围查询)RDF database 资源描述框架数据库RDF query 资源描述框架查询RDF repository 资源描述框架存储库RDF storge 资源描述框架存储real time(实时)recommender system(推荐系统)recommender system(推荐系统)recommender systems 推荐系统recommender systems(推荐系统)record linkage 记录链接recurrent neural network(递归神经网络) regression(回归)reinforcement learning 强化学习reinforcement learning(强化学习)relation extraction 关系抽取relational database 关系数据库relational learning 关系学习relevance feedback (相关反馈)resource description framework 资源描述框架restricted boltzmann machines(受限玻尔兹曼机) retrieval models(检索模型)rough set theroy 粗糙集理论rough set 粗糙集rule based system 基于规则系统rule based 基于规则rule induction (规则归纳)rule learning (规则学习)rule learning 规则学习schema mapping 模式映射schema matching 模式匹配scientific domain 科学域search problems(搜索问题)semantic (web) technology 语义技术semantic analysis 语义分析semantic annotation 语义标注semantic computing 语义计算semantic integration 语义集成semantic interpretation 语义解释semantic model 语义模型semantic network 语义网络semantic relatedness 语义相关性semantic relation learning 语义关系学习semantic search 语义检索semantic similarity 语义相似度semantic similarity(语义相似度)semantic web rule language 语义网规则语言semantic web 语义网semantic web(语义网)semantic workflow 语义工作流semi supervised learning(半监督学习)sensor data(传感器数据)sensor networks(传感器网络)sentiment analysis(情感分析)sentiment analysis(情感分析)sequential pattern(序列模式)service oriented architecture 面向服务的体系结构shortest path(最短路径)similar kernel function(相似核函数)similarity measure(相似性度量)similarity relationship (相似关系)similarity search(相似搜索)similarity(相似性)situation aware 情境感知social behavior(社交行为)social influence(社会影响)social interaction(社交互动)social interaction(社交互动)social learning(社会学习)social life networks(社交生活网络)social machine 社交机器social media(社交媒体)social media(社交媒体)social media(社交媒体)social network analysis 社会网络分析social network analysis(社交网络分析)social network(社交网络)social network(社交网络)social science(社会科学)social tagging system(社交标签系统)social tagging(社交标签)social web(社交网页)sparse coding(稀疏编码)sparse matrices(稀疏矩阵)sparse representation(稀疏表示)spatial database(空间数据库)spatial reasoning 空间推理statistical analysis(统计分析)statistical model 统计模型string matching(串匹配)structural risk minimization (结构风险最小化) structured data 结构化数据subgraph matching 子图匹配subspace clustering(子空间聚类)supervised learning( 有support vector machine 支持向量机support vector machines(支持向量机)system dynamics(系统动力学)tag recommendation(标签推荐)taxonmy induction 感应规范temporal logic 时态逻辑temporal reasoning 时序推理text analysis(文本分析)text anaylsis 文本分析text classification (文本分类)text data(文本数据)text mining technique(文本挖掘技术)text mining 文本挖掘text mining(文本挖掘)text summarization(文本摘要)thesaurus alignment 同义对齐time frequency analysis(时频分析)time series analysis( 时time series data(时间序列数据)time series data(时间序列数据)time series(时间序列)topic model(主题模型)topic modeling(主题模型)transfer learning 迁移学习triple store 三元组存储uncertainty reasoning 不精确推理undirected graph(无向图)unified modeling language 统一建模语言unsupervisedupper bound(上界)user behavior(用户行为)user generated content(用户生成内容)utility mining(效用挖掘)visual analytics(可视化分析)visual content(视觉内容)visual representation(视觉表征)visualisation(可视化)visualization technique(可视化技术) visualization tool(可视化工具)web 2.0(网络2.0)web forum(web 论坛)web mining(网络挖掘)web of data 数据网web ontology lanuage 网络本体语言web pages(web 页面)web resource 网络资源web science 万维科学web search (网络检索)web usage mining(web 使用挖掘)wireless networks 无线网络world knowledge 世界知识world wide web 万维网world wide web(万维网)xml database 可扩展标志语言数据库附录 2 Data Mining 知识图谱(共包含二级节点15 个,三级节点93 个)间序列分析)监督学习)领域 二级分类 三级分类。
超高速基带通信算法英文
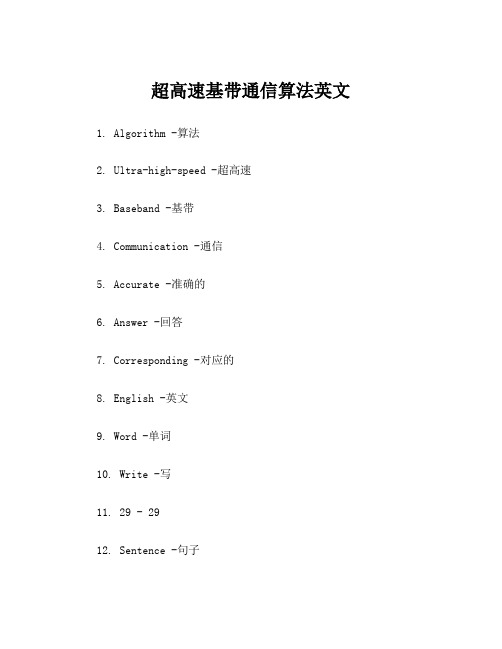
超高速基带通信算法英文1. Algorithm -算法2. Ultra-high-speed -超高速3. Baseband -基带4. Communication -通信5. Accurate -准确的6. Answer -回答7. Corresponding -对应的8. English -英文9. Word -单词10. Write -写11. 29 - 2912. Sentence -句子13. Bilingual -双语的14. The algorithm for ultra-high-speed baseband communication is highly efficient.超高速基带通信算法非常高效。
15. Can you accurately answer the corresponding English words?你能准确回答对应的英文单词吗?16. Please write 29 sentences in bilingual.请用双语写29个句子。
17. The development of ultra-high-speed baseband communication algorithms has revolutionized the field of telecommunications.超高速基带通信算法的发展彻底改变了电信领域。
18. We need an accurate algorithm to optimize the baseband communication system.我们需要一个准确的算法来优化基带通信系统。
19. Do you have the answer to the corresponding English word for "communication"?你对于"communication"这个对应的英文单词有答案吗?20. The English word for "algorithm" is the same in both languages."algorithm"这个英文单词在两种语言中都一样。
dtnl练习题(打印版)
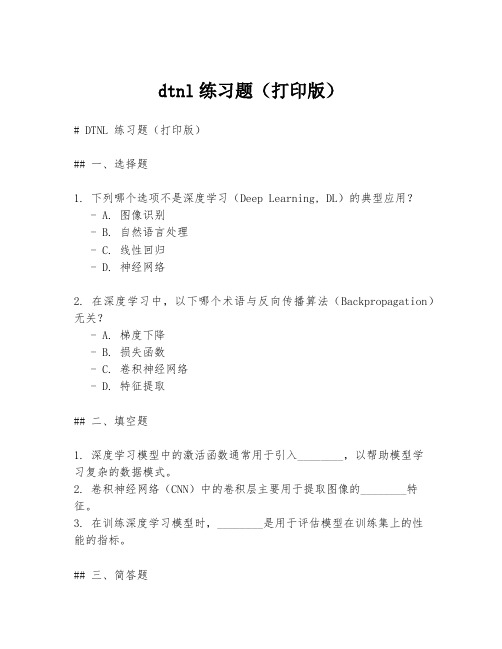
dtnl练习题(打印版)# DTNL 练习题(打印版)## 一、选择题1. 下列哪个选项不是深度学习(Deep Learning, DL)的典型应用?- A. 图像识别- B. 自然语言处理- C. 线性回归- D. 神经网络2. 在深度学习中,以下哪个术语与反向传播算法(Backpropagation)无关?- A. 梯度下降- B. 损失函数- C. 卷积神经网络- D. 特征提取## 二、填空题1. 深度学习模型中的激活函数通常用于引入________,以帮助模型学习复杂的数据模式。
2. 卷积神经网络(CNN)中的卷积层主要用于提取图像的________特征。
3. 在训练深度学习模型时,________是用于评估模型在训练集上的性能的指标。
## 三、简答题1. 简要描述什么是深度学习,并说明它与传统机器学习方法的主要区别。
2. 解释什么是过拟合(Overfitting),并给出避免过拟合的几种策略。
## 四、计算题给定一个简单的神经网络,包含一个输入层,两个隐藏层和一个输出层。
假设输入层有4个神经元,第一个隐藏层有8个神经元,第二个隐藏层有6个神经元,输出层有3个神经元。
如果输入层的激活值为[0.2, 0.5, 0.8, 1.0],第一个隐藏层的权重矩阵为:\[W_1 =\begin{bmatrix}0.1 & 0.2 & 0.3 & 0.4 \\0.5 & 0.6 & 0.7 & 0.8 \\0.9 & 1.0 & 1.1 & 1.2 \\\end{bmatrix}\]第一个隐藏层的偏置向量为 \( b_1 = [0.1, 0.2, 0.3, 0.4] \),激活函数为 ReLU。
请计算第一个隐藏层的输出激活值。
## 五、编程题编写一个简单的 Python 函数,该函数接受一个列表作为输入,返回列表中所有元素的和。
```pythondef sum_elements(input_list):# 你的代码pass```## 六、案例分析题考虑一个实际问题,例如图像识别、语音识别或自然语言处理等,描述如何使用深度学习技术来解决这个问题,并简要说明所选择的模型架构和训练过程。
《2024年基于上下文感知及边界引导的伪装物体检测研究》范文
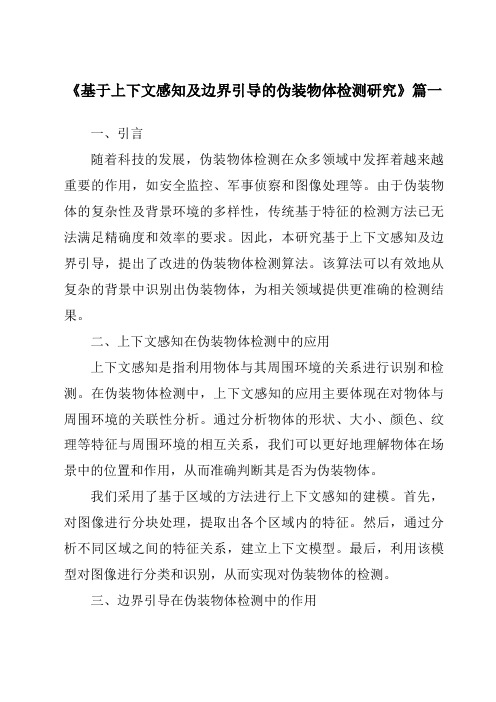
《基于上下文感知及边界引导的伪装物体检测研究》篇一一、引言随着科技的发展,伪装物体检测在众多领域中发挥着越来越重要的作用,如安全监控、军事侦察和图像处理等。
由于伪装物体的复杂性及背景环境的多样性,传统基于特征的检测方法已无法满足精确度和效率的要求。
因此,本研究基于上下文感知及边界引导,提出了改进的伪装物体检测算法。
该算法可以有效地从复杂的背景中识别出伪装物体,为相关领域提供更准确的检测结果。
二、上下文感知在伪装物体检测中的应用上下文感知是指利用物体与其周围环境的关系进行识别和检测。
在伪装物体检测中,上下文感知的应用主要体现在对物体与周围环境的关联性分析。
通过分析物体的形状、大小、颜色、纹理等特征与周围环境的相互关系,我们可以更好地理解物体在场景中的位置和作用,从而准确判断其是否为伪装物体。
我们采用了基于区域的方法进行上下文感知的建模。
首先,对图像进行分块处理,提取出各个区域内的特征。
然后,通过分析不同区域之间的特征关系,建立上下文模型。
最后,利用该模型对图像进行分类和识别,从而实现对伪装物体的检测。
三、边界引导在伪装物体检测中的作用边界引导是指利用图像中的边缘信息对物体进行定位和识别。
在伪装物体检测中,边界引导的作用主要体现在对物体边缘的精确提取和识别。
通过分析物体的边缘特征,我们可以更准确地判断其形状和位置,从而实现对伪装物体的精确检测。
我们采用了基于边缘检测的方法进行边界引导的实现。
首先,对图像进行预处理,增强边缘信息。
然后,利用边缘检测算法提取出图像中的边缘特征。
最后,结合上下文感知的结果,对边缘特征进行进一步的分析和处理,从而实现对伪装物体的精确检测。
四、实验与分析为了验证本算法的有效性,我们进行了大量的实验。
实验结果表明,基于上下文感知及边界引导的伪装物体检测算法具有较高的准确性和稳定性。
与传统的基于特征的检测方法相比,本算法在处理复杂背景和多变环境下的伪装物体检测问题时表现出更强的鲁棒性。
2023年人工智能现代科技知识考试题与答案
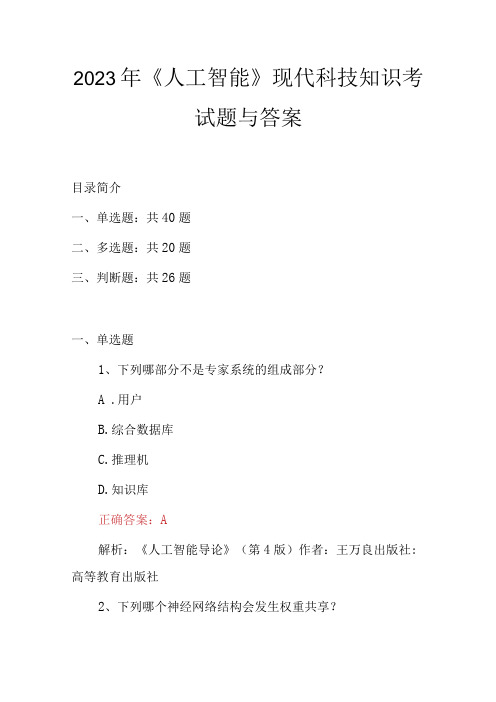
2023年《人工智能》现代科技知识考试题与答案目录简介一、单选题:共40题二、多选题:共20题三、判断题:共26题一、单选题1、下列哪部分不是专家系统的组成部分?A .用户B.综合数据库C.推理机D.知识库正确答案:A解析:《人工智能导论》(第4版)作者:王万良出版社: 高等教育出版社2、下列哪个神经网络结构会发生权重共享?A.卷积神经网络B.循环神经网络C.全连接神经网络D. A 和B正确答案:D解析:《深度学习、优化与识别》作者:焦李成出版社: 清华大学出版社3、下列哪个不属于常用的文本分类的特征选择算法?A.卡方检验值B.互信息C .信息增益D.主成分分析正确答案:D解析:《自然语言处理》作者:刘挺出版社:高等教育出版社4、下列哪个不是人工智能的技术应用领域?A.搜索技术B.数据挖掘C.智能控制D .编译原理解析:《走进人工智能》作者:周旺出版社:高等教育出版社5、Q(s,a)是指在给定状态s的情况下,采取行动a之后,后续的各个状态所能得到的回报()。
A.总和B.最大值C.最小值D.期望值正确答案:D解析:《深度学习、优化与识别》作者:焦李成出版社: 清华大学出版社6、数据科学家可能会同时使用多个算法(模型)进行预测,并且最后把这些算法的结果集成起来进行最后的预测(集成学习),以下对集成学习说法正确的是()。
A.单个模型之间有高相关性B.单个模型之间有低相关性C,在集成学习中使用“平均权重”而不是“投票”会比较好D.单个模型都是用的一个算法解析:《机器学习方法》作者:李航出版社:清华大学出版社7、以下哪种技术对于减少数据集的维度会更好?A.删除缺少值太多的列B.删除数据差异较大的列C.删除不同数据趋势的列D.都不是正确答案:A解析:《机器学习》作者:周志华出版社:清华大学出版社8、在强化学习过程中,学习率越大,表示采用新的尝试得到的结果比例越(),保持旧的结果的比例越()。
A .大,小B.大,大C.小,大D.小,小正确答案:A解析:《深度学习、优化与识别》作者:焦李成出版社: 清华大学出版社9、以下哪种方法不属于特征选择的标准方法?A.嵌入B.过滤C ,包装D.抽样正确答案:D解析:《深度学习、优化与识别》作者:焦李成出版社: 清华大学出版社10、要想让机器具有智能,必须让机器具有知识。
人工智能中级认证考试

一、选择题1.在机器学习中,哪种算法常用于分类问题,通过构建决策树来进行预测?A.线性回归B.决策树算法(答案)C.K-means聚类D.主成分分析2.下列哪一项不是深度学习的常用框架?A.TensorFlowB.PyTorchC.Scikit-learn(答案)D.Keras3.在神经网络中,权重和偏置的初始值设置对训练过程有很大影响。
哪种初始化方法可以帮助避免梯度消失或爆炸问题?A.随机初始化B.Xavier/Glorot初始化(答案)C.全零初始化D.全一初始化4.以下哪项技术常用于自然语言处理(NLP)中的词嵌入表示?A.词袋模型B.TF-IDFC.Word2Vec(答案)D.One-hot编码5.在强化学习中,智能体(Agent)根据什么来选择动作?A.奖励函数(答案)B.状态转移概率C.动作空间大小D.环境模型6.下列哪一项不是卷积神经网络(CNN)的常用层?A.卷积层B.池化层C.全连接层D.循环层(答案)7.在机器学习的模型评估中,哪种方法可以用于评估分类模型的性能,通过计算真正例、假正例、真反例和假反例的数量?A.混淆矩阵(答案)B.ROC曲线C.交叉验证D.准确率8.以下哪个算法是基于实例的学习算法,通过计算新数据与训练集中每个数据点的相似度来进行分类或回归?A.K-近邻算法(答案)B.支持向量机C.神经网络D.决策树9.在自然语言处理中,哪种技术可以用于将句子或文档转换为固定长度的向量表示?A.词嵌入B.文本分类C.情感分析D.Sentence Embedding(答案)10.以下哪个术语用于描述在训练过程中,模型在训练集上的性能逐渐提高,但在测试集上的性能开始下降的现象?A.过拟合(答案)B.欠拟合C.交叉验证D.泛化能力。
参考文献(人工智能)
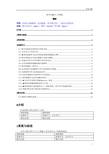
参考文献(人工智能)曹晖目的:对参考文献整理(包括摘要、读书笔记等),方便以后的使用。
分类:粗分为论文(paper)、教程(tutorial)和文摘(digest)。
0介绍 (1)1系统与综述 (1)2神经网络 (2)3机器学习 (2)3.1联合训练的有效性和可用性分析 (2)3.2文本学习工作的引导 (2)3.3★采用机器学习技术来构造受限领域搜索引擎 (3)3.4联合训练来合并标识数据与未标识数据 (5)3.5在超文本学习中应用统计和关系方法 (5)3.6在关系领域发现测试集合规律性 (6)3.7网页挖掘的一阶学习 (6)3.8从多语种文本数据库中学习单语种语言模型 (6)3.9从因特网中学习以构造知识库 (7)3.10未标识数据在有指导学习中的角色 (8)3.11使用增强学习来有效爬行网页 (8)3.12★文本学习和相关智能A GENTS:综述 (9)3.13★新事件检测和跟踪的学习方法 (15)3.14★信息检索中的机器学习——神经网络,符号学习和遗传算法 (15)3.15用NLP来对用户特征进行机器学习 (15)4模式识别 (16)4.1JA VA中的模式处理 (16)0介绍1系统与综述2神经网络3机器学习3.1 联合训练的有效性和可用性分析标题:Analyzing the Effectiveness and Applicability of Co-training链接:Papers 论文集\AI 人工智能\Machine Learning 机器学习\Analyzing the Effectiveness and Applicability of Co-training.ps作者:Kamal Nigam, Rayid Ghani备注:Kamal Nigam (School of Computer Science, Carnegie Mellon University, Pittsburgh, PA 15213, knigam@)Rayid Ghani (School of Computer Science, Carnegie Mellon University, Pittsburgh, PA 15213 rayid@)摘要:Recently there has been significant interest in supervised learning algorithms that combine labeled and unlabeled data for text learning tasks. The co-training setting [1] applies todatasets that have a natural separation of their features into two disjoint sets. We demonstrate that when learning from labeled and unlabeled data, algorithms explicitly leveraging a natural independent split of the features outperform algorithms that do not. When a natural split does not exist, co-training algorithms that manufacture a feature split may out-perform algorithms not using a split. These results help explain why co-training algorithms are both discriminativein nature and robust to the assumptions of their embedded classifiers.3.2 文本学习工作的引导标题:Bootstrapping for Text Learning Tasks链接:Papers 论文集\AI 人工智能\Machine Learning 机器学习\Bootstrap for Text Learning Tasks.ps作者:Rosie Jones, Andrew McCallum, Kamal Nigam, Ellen Riloff备注:Rosie Jones (rosie@, 1 School of Computer Science, Carnegie Mellon University, Pittsburgh, PA 15213)Andrew McCallum (mccallum@, 2 Just Research, 4616 Henry Street, Pittsburgh, PA 15213)Kamal Nigam (knigam@)Ellen Riloff (riloff@, Department of Computer Science, University of Utah, Salt Lake City, UT 84112)摘要:When applying text learning algorithms to complex tasks, it is tedious and expensive to hand-label the large amounts of training data necessary for good performance. This paper presents bootstrapping as an alternative approach to learning from large sets of labeled data. Instead of a large quantity of labeled data, this paper advocates using a small amount of seed information and alarge collection of easily-obtained unlabeled data. Bootstrapping initializes a learner with the seed information; it then iterates, applying the learner to calculate labels for the unlabeled data, and incorporating some of these labels into the training input for the learner. Two case studies of this approach are presented. Bootstrapping for information extraction provides 76% precision for a 250-word dictionary for extracting locations from web pages, when starting with just a few seed locations. Bootstrapping a text classifier from a few keywords per class and a class hierarchy provides accuracy of 66%, a level close to human agreement, when placing computer science research papers into a topic hierarchy. The success of these two examples argues for the strength of the general bootstrapping approach for text learning tasks.3.3 ★采用机器学习技术来构造受限领域搜索引擎标题:Building Domain-specific Search Engines with Machine Learning Techniques链接:Papers 论文集\AI 人工智能\Machine Learning 机器学习\Building Domain-Specific Search Engines with Machine Learning Techniques.ps作者:Andrew McCallum, Kamal Nigam, Jason Rennie, Kristie Seymore备注:Andrew McCallum (mccallum@ , Just Research, 4616 Henry Street Pittsburgh, PA 15213)Kamal Nigam (knigam@ , School of Computer Science, Carnegie Mellon University Pittsburgh, PA 15213)Jason Rennie (jr6b@)Kristie Seymore (kseymore@)摘要:Domain-specific search engines are growing in popularity because they offer increased accuracy and extra functionality not possible with the general, Web-wide search engines. For example, allows complex queries by age-group, size, location and cost over summer camps. Unfortunately these domain-specific search engines are difficult and time-consuming to maintain. This paper proposes the use of machine learning techniques to greatly automate the creation and maintenance of domain-specific search engines. We describe new research in reinforcement learning, information extraction and text classification that enables efficient spidering, identifying informative text segments, and populating topic hierarchies. Using these techniques, we have built a demonstration system: a search engine forcomputer science research papers. It already contains over 50,000 papers and is publicly available at ....采用多项Naive Bayes 文本分类模型。
- 1、下载文档前请自行甄别文档内容的完整性,平台不提供额外的编辑、内容补充、找答案等附加服务。
- 2、"仅部分预览"的文档,不可在线预览部分如存在完整性等问题,可反馈申请退款(可完整预览的文档不适用该条件!)。
- 3、如文档侵犯您的权益,请联系客服反馈,我们会尽快为您处理(人工客服工作时间:9:00-18:30)。
Connectivity reflects coding: a model of voltage-based STDP with homeostasis
Claudia Clopath1, பைடு நூலகம்ars Büsing1,2, Eleni Vasilaki1,2 & Wulfram Gerstner1
Electrophysiological connectivity patterns in cortex often have a few strong connections, which are sometimes bidirectional, among a lot of weak connections. To explain these connectivity patterns, we created a model of spike timing–dependent plasticity (STDP) in which synaptic changes depend on presynaptic spike arrival and the postsynaptic membrane potential, filtered with two different time constants. Our model describes several nonlinear effects that are observed in STDP experiments, as well as the voltage dependence of plasticity. We found that, in a simulated recurrent network of spiking neurons, our plasticity rule led not only to development of localized receptive fields but also to connectivity patterns that reflect the neural code. For temporal coding procedures with spatio-temporal input correlations, strong connections were predominantly unidirectional, whereas they were bidirectional under rate-coded input with spatial correlations only. Thus, variable connectivity patterns in the brain could reflect different coding principles across brain areas; moreover, our simulations suggested that plasticity is fast. Experience-dependent changes in receptive fields1,2 or in learned behavior relate to changes in synaptic strength. Electrophysiological measurements of functional connectivity patterns in slices of neural tissue3,4 or anatomical connectivity measures can only present a snapshot of the momentary connectivity, which may change over time5. The question then arises of whether the connectivity patterns and their changes can be connected to basic forms of synaptic plasticity6 such as long-term potentiation (LTP) and depression (LTD)7. LTP and LTD depend on the exact timing of pre- and postsynaptic action potentials2–8 but also on postsynaptic voltage9,10 and presynaptic stimulation frequency11. STDP has attracted particular interest in recent years, as temporal coding schemes in which information is contained in the exact timing of spikes rather than mean frequency can be learned by a neural system using STDP12,13 (review in ref. 14). However, the question of whether STDP is more fundamental than frequency-dependent plasticity or voltage-dependent plasticity rules has not been resolved despite an intense debate15. Moreover, it is unclear how the interplay of coding and plasticity yields the functional connectivity patterns that are seen in experiments. In particular, the presence or absence of bidirectional connectivity between cortical pyramidal neurons seems to be contradictory across experimental preparations in visual3 or somatosensory cortex4. Recent experiments have shown that STDP is strongly influenced by postsynaptic voltage before action potential firing16 but were unable to answer the question of whether spiketiming dependence is a direct consequence of voltage dependence or the manifestation of an independent process. In addition, STDP depends on stimulation frequency16, suggesting an interaction between timing- and frequency-dependent processes16. We found that a simple Hebbian plasticity rule that pairs presynaptic spike arrival with the postsynaptic membrane potential was sufficient to explain STDP and the dependence of plasticity on presynaptic stimulation frequency. Moreover, the intricate interplay of voltage and spike timing as well as the frequency dependence of STDP can be explained in our model from one single principle. In contrast with earlier attempts towards a unified description of synaptic plasticity17,18, our model is a phenomenological one. It does not give an explicit interpretation in terms of biophysical quantities such a calcium concentration17, CaMKII18, glutamate binding, NMDA receptors, etc. Instead, it aims at a minimal description of the major phenomena observed in electrophysiology experiments. The advantage of such a minimal model is that it allows us to discuss functional consequences in small19–21, and possibly even large22,23, networks. We found that the learning rule led to input spec ificity in small networks of up to ten neurons, which is necessary for receptive field development, similar to earlier models of STDP12,19 or ratebased plasticity rules24,25. We explicitly addressed the question of whether functional connectivity patterns of cortical pyramidal neurons measured in recent electrophysiological studies3,4 could be the result of plasticity during continued stimulation of neuronal model networks, particularly bidirectional connections3 that are incompatible with standard STDP models12,19. The mathematical simplicity of our model enabled us to identify conditions under which it becomes equivalent to the well-known Bienenstock-Cooper-Munro (BCM) model24 used in classical rate-based descriptions of developmental learning and, similar to some earlier models of STDP26,27, and why our model is fundamentally different from classical STDP models12,14,19, is widely used for temporal coding. RESULTS To study the means by which connectivity patterns in cortex can emerge from plasticity, we needed a plasticity rule that was consistent