overview
Overview of the World Maps

Slovenia Portugal
Andorra
Croatia Bosnia and Herzegovina
Sarajevo Belgrade
Lisbon
Madrid
Italy
Rome
Serbia and Montenegro
Skopje Tirana
Bulgaria
Sofia
Spain
MaceAlba- donia nia Greece
San Francisco Los Angeles
U.S.A.
Dallas Atlanta New Orleans
Houston
Miami
Mexico
Monterrey Guadalajara Mexico
United States
Alaska
Juneau Olympia
Washington Montana
Salem
Helena
New Hampshire North Dakota
Bismarck Saint Paul
Maine
Minnesota
Vermont
Montpelier Albany
Augusta Concord
Oregon Idaho
Boise Pierre
Massachusetts Wisconsin
Pennsylvania Iowa
Des Moines
Trenton
Illinois
Ohio
Indianapolis Columbus
Harrisburg Annapolis
Dover
Nebraska
Denver Lincoln Topeka Jefferson City
SAP OverviewPPT课件
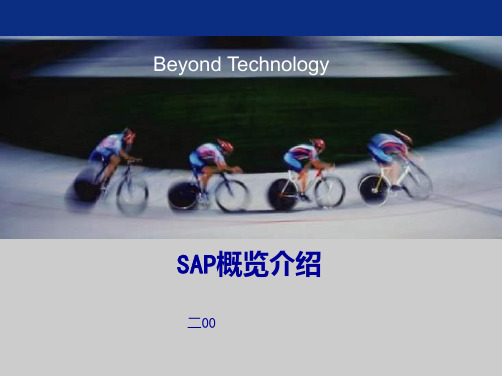
SAP 公司
• SAP -- 36 Years in the Software Business
• Headquartered in Waldorf, Germany
• Founded by five former IBM systems engineers
财务分析
自助服务 劳动力分析
运作分析
管理会计
公司治理
财务供应链管理
员工生命周期管理
员工事务管理
生产
项目管理
维护
质量管理
分销
销售订单管理
环境、健康和安全
激励和佣金管理
不动产
信息集成
流程集成
应用平台
mySAP ERP 比较 SAP R/3 Enterprise的部分功能差异
关键要点:
相对 SAP R/3 Enterprise, mySAP ERP 包括大量新增功能
mySAP Business Suite
Cross-Industry Solutions: mySAP CRM (CRM) mySAP SCM (APO) mySAP BI (SEM ,BW)
Users:
Customers Employees Vendors Stakeholders
mySAP PLM (PLM) mySAP SRM(SRM) Industry Solutions:
MySAP ERP
Financials (FI, CO, AA, PS) Logistics (MM, SD, PP, QM, PM, CS)
•
SAP系统的基本概念
• 7月6日 13:30—16:00: SAP产品功能介绍(一)
Overview
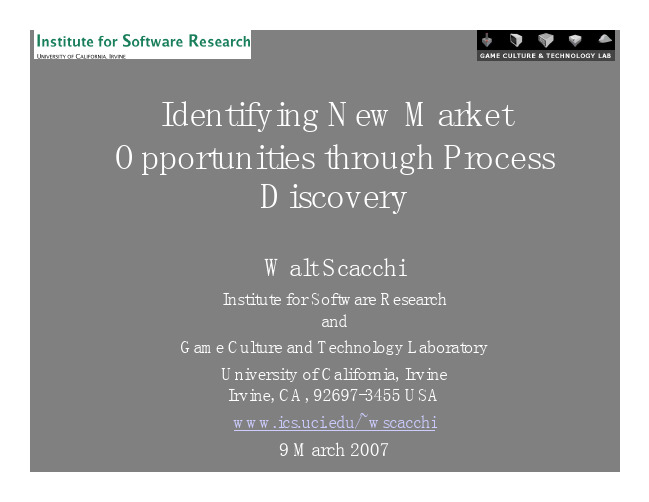
5 W. Scacchi, CMAG Presentation, 9 March 2007
Other Motivating Applications
• Game-based virtual worlds
– Most MMOG companies don’t know their own processes, nor those active/emerging within game community (e.g., external/gray markets for in-game resources)
Discovering socio-technical and cultural evolution processes
• New OSSD processes under study
– Joining and contributing to a project in progress – Role-task migration: from project periphery to center – Alliance formation and community development
Process discovery
• Participant observation (online, Web-based ethnography) to tailor process meta-model • Collection, annotation, and tracking of participant created/modified artifacts
– Objects of interaction marking events and event flow – How objects are situated in facilitating collaboration, conflict, or conflict mitigation – Requires scalable, concurrent content crawling and indexing – Guided by meta-model and multi-mode process models
英文教材食品安全Overview_food_safety
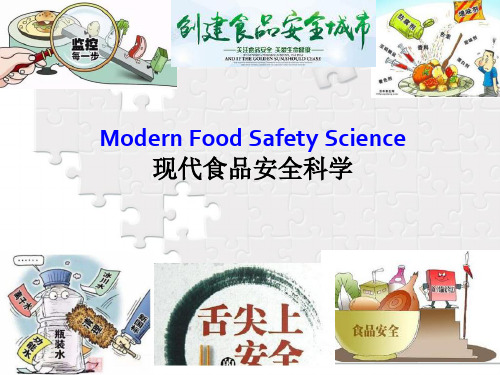
Safety or quality issue?
Unusual kidney stone 三鹿结石宝宝
September 11, 2008 Exposed in News - Stone kidney baby in Gansu To 12 October, Sufferred baby 52,857 12800 Hospitalized
How will study this course?
Lecture references
Nollet, L. M. L and Toldra, F. (2007). Advances in Food Diagnostics. Paster, T. (2007). The HACCP Training Food Safety. Motarjemi, Y. & Adams, M. (2006). Emerging Food Borne Pathogens. Rasco, B. A. (2005). Bioterrorism & Food Safety. Lees, M. (2003). Food Authenticity & Traceability. D`Mello, J. P. F. (2003). Food Safety. Riviere, J. (2002). Chemical Food Safety.
1. "Access by all people at all times to enough food for an
active, healthy life." (World Bank) 2. "All people at all times have both physical and economic
overview用法 -回复
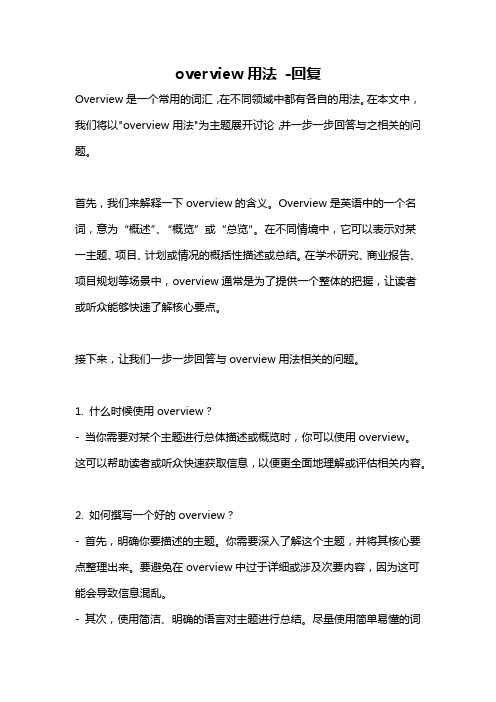
overview用法-回复Overview是一个常用的词汇,在不同领域中都有各自的用法。
在本文中,我们将以"overview用法"为主题展开讨论,并一步一步回答与之相关的问题。
首先,我们来解释一下overview的含义。
Overview是英语中的一个名词,意为“概述”、“概览”或“总览”。
在不同情境中,它可以表示对某一主题、项目、计划或情况的概括性描述或总结。
在学术研究、商业报告、项目规划等场景中,overview通常是为了提供一个整体的把握,让读者或听众能够快速了解核心要点。
接下来,让我们一步一步回答与overview用法相关的问题。
1. 什么时候使用overview?- 当你需要对某个主题进行总体描述或概览时,你可以使用overview。
这可以帮助读者或听众快速获取信息,以便更全面地理解或评估相关内容。
2. 如何撰写一个好的overview?- 首先,明确你要描述的主题。
你需要深入了解这个主题,并将其核心要点整理出来。
要避免在overview中过于详细或涉及次要内容,因为这可能会导致信息混乱。
- 其次,使用简洁、明确的语言对主题进行总结。
尽量使用简单易懂的词汇和短句,避免使用过多的行话或专业术语,以确保广大受众都能理解。
- 最后,在概述中强调主要亮点和关键信息,可以通过使用粗体、强调句子或其他标记方法来突出重点。
3. 在哪些场景下常见到overview的使用?- 在学术研究中,研究论文通常会在摘要部分提供一个overview,即对研究目的、方法、结果和结论的简要总结。
- 在商业报告或市场分析中,overview可以提供公司业绩、市场趋势、竞争对手等方面的综合概述。
- 在项目规划或产品开发中,overview可能包含项目的目标、时间表、预算以及关键的里程碑事件等。
4. 如何在演讲或演示中有效使用overview?- 在演讲或演示中,overview可以用于开场白或导言部分,帮助听众快速掌握主题的要点和结构。
TSM 标准Overview 4-24-7 - 中文-简
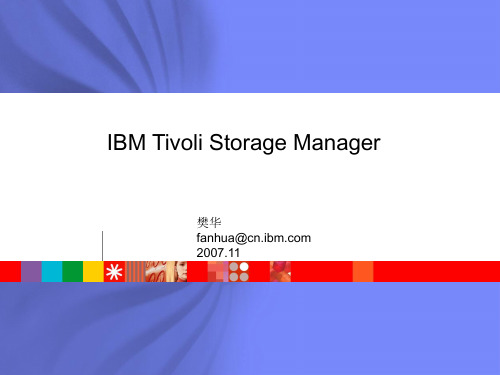
第二周 140 GB
2周 总计备份 460GB
第一周 320 GB
IBM System Storage IBM Software Group | Tivoli software
永久增量备份
全 + 差异备份 第2周 651 GB 202 GB
Day 127 GB 14 Day 107 GB 13
客户端 TSM 客户端 客户端 客户端
生命周期管理
•迁移非活跃数据 •透明地回调数据 •基于策略管理 •与备份集成
12
/software/tivoli/products/storage-mgr-space/
A1 B1 C1 D1
A1 B1 C1 D1 A1 B1 C1 D1 A1 A2 B2 B3 C1 C2 C3 D1
A2 B2 C2
B2 C2 A2 C3 B3
B3 C3
恢复
5 个磁带 9 个文件 2 个磁带 9 个文件 1 个磁带 4 文件
B2 C2
A2 B2 C2
A2 B2 C2 C3
A2 B2 B3 C2 C3
Offsite Tape
© 2007 IBM Corporation
IBM System Storage IBM Software Group | Tivoli software
先进的复制技术 - 基于快照
应用系统 应用数据 本地快照版本 备份系统
快速无损备份
创建快照
备份到TSM服 务器
Disk
执行快照在线备份数据库应用,对 应用几无影响。 在另一台系统上将数据转移到TSM 存储池。
一个TSM可进行多种强大的数据保护功能 可进行多种强大的数据保护功能 一个
被动语态
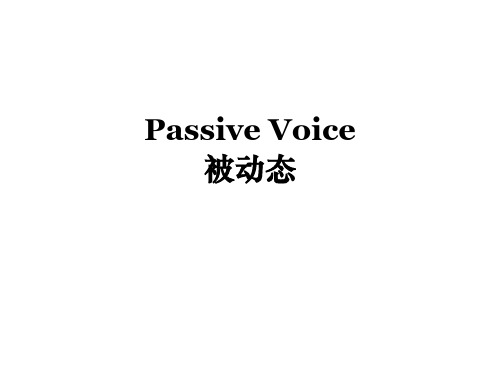
By sustainability we mean the ability to maintain a certain status or process in existing systems. (A-manner S V O)
By sustainability the ability to maintain a certain status or process in existing systems is meant. (A-manner S V) By sustainability is meant the ability to maintain a certain status or process in existing systems. (A-manner V S)
Active clause (主动句):
Jimmy considers Mark Recipient 接受者 gives Mark Patient 受动者 a bottle. Patient 受动者 a bottle a geek. OC宾补 Agent 施动者 Jimmy gives Agent 施动者 Jimmy Agent 施动者 The first floor contains Location 地点 This year Time 时间 Tactics can win you sees
A bottle is given (to Mark) (by Jimmy). The game can be won (for you) (with tactics). She got sacked (by her boss).
*********************************************************** Put “他给她偷了一朵玫瑰” into active and passive constructions: Active: He stole a rose for her. / He stole her a rose.
SAP HANA功能介绍Overview
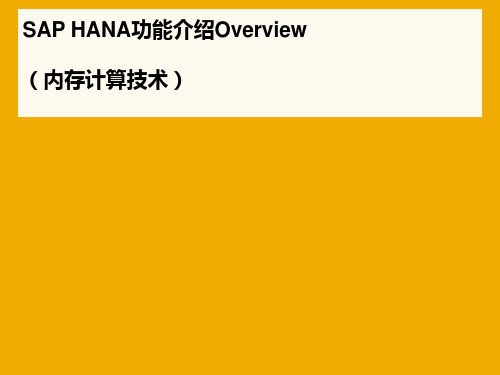
改善库存管理
降低库存成本
能够在销售和计划会议 期间快速创建、分析多 样化假设情境
极大改进时效性,快速 科学决策
更为准确地进行合规性 管理,降低成本、缩短 研发时间
将销售报表的运算时间 由数小时降低至数秒
能够在实时数据基础上 做出实时的决策
找出不同业务单元中客 户的相互依赖关系
SQL (ODBC/JDBC) DBSQL
BICS
Real Time
SAP HANA
(Appliance)
SAP In-Memory Database
Sybase Replication Server
DB
SAP BusinessObjects Data Services
SAP BW
3rd Party
Custom Web Developed UI Sybase Unwired
ERP
SAP BusinessObjects BI 4.0
WebI
Dashboards Crystal
Semantic Layer
Crystal
Explorer A Office
SQL (ODBC/JDBC)
SQL (ODBC/JDBC)
可以与行式数据存储的表关联
映射到内存
行式的数据组织 A 10 € B 35 $ C 2 € D 40 € E 12 $
列式的数据组织 A B C D E 10 35 2 40 12 € $ € € $ memory address
10
内存计算 — 创新带来业务机会
硬件技术创新
多核架构(每个刀片8 x 8 核心 CPU) 多刀片大规模并行扩展 刀片服务器价格低廉
- 1、下载文档前请自行甄别文档内容的完整性,平台不提供额外的编辑、内容补充、找答案等附加服务。
- 2、"仅部分预览"的文档,不可在线预览部分如存在完整性等问题,可反馈申请退款(可完整预览的文档不适用该条件!)。
- 3、如文档侵犯您的权益,请联系客服反馈,我们会尽快为您处理(人工客服工作时间:9:00-18:30)。
Introduction 1
1
Introduction
During recent years there have been advances in data learning using kernel methods. Kernel representation offers an alternative learning to non-linear functions by projecting the data into a high dimensional feature space to increase the computational power of the linear learning machines, though this still leaves open the issue of how best to choose the features or the kernel function in ways that will improve performance. We review some of the methods that have been developed for learning the feature space. • Principal Component Analysis (PCA) is a multivariate data analysis procedure that involves a transformation of a number of possibly correlated variables into a smaller number of uncorrelated variables known as principal components. PCA only makes use of the training inputs while making no use of the labels. • Independent Component Analysis (ICA) in contrast to correlation-based transformations such as PCA not only decorrelates the signals but also reduces higher-order statistical dependencies, attempting to make the signals as independent as possible. In other words, ICA is a way of finding a linear not only orthogonal co-ordinate system in any multivariate data. The directions of the axes of this co-ordinate system are determined by both the second and higher order statistics of the original data. The goal is to perform a linear transform which makes the resulting variables as statistically independent from each other as possible. • Partial Least Squares (PLS) is a method similar to canonical correlation analysis. It selects feature directions that are useful for the task at hand, though PLS only uses one view of an object and the label as the corresponding pair. PLS could be thought of as a method, which looks for directions that are good at distinguishing the different labels. • Canonical Correlation Analysis (CCA) is a method of correlating linear relationships between two multidimensional variables. CCA can be seen as using complex labels as a way of guiding feature selection towards the underling semantics. CCA makes use of two views of the same semantic object to extract the representation of the semantics. The main difference between CCA and the other three methods is that CCA is closely related to mutual information (Borga 1998 [3]). Hence CCA can be easily motivated in information based tasks and is our natural selection. Proposed by H. Hotelling in 1936 [12], CCA can be seen as the problem of finding basis vectors for two sets of variables such that the correlation between the projections of the variables onto these basis vectors are mutually maximised. In an attempt to increase the flexibility of the feature selection, kernelisation of CCA (KCCA) has been applied to map the hypotheses to a higher-dimensional feature space. KCCA has been applied in some preliminary work by Fyfe & Lai [8], Akaho [1] and the recently Vinokourov et al. [19] with improved results.
Canonical correlation analysis; An overview with application to learning methods
David R. Hardoon , Sandor Szedmak and John Shawe-Taylor Department of Computer Science Royal Holloway, University of London {davidh, sandor, john}@
Introduction 2
During recent years there has been a vast increase in the amount of multimedia content available both off-line and online, though we are unable to access or make use of this data unless it is organised in such a way as to allow efficient browsing. To enable content based retrieval with no reference to labeling we attempt to learn the semantic representation of images and their associated text. We present a general approach using KCCA that can be used for content [11] to as well as mate based retrieval [18, 11]. In both cases we compare the KCCA approach to the Generalised Vector Space Model (GVSM), which aims at capturing some term-term correlations by looking at co-occurrence information. This study aims to serve as a tutorial and give additional novel contributions in the following ways: • In this study we follow the work of Borga [4] where we represent the eigenproblem as two eigenvalue equations as this allows us to reduce the computation time and dimensionality of the eigenvectors. • Further to that, we follow the idea of Bach & Jordan [2] to compute a new correlation matrix with reduced dimensionality. Though Bach & Jordan [2] address a very different problem, they use the same underlining technique of Cholesky decomposition to re-represent the kernel matrices. We show that by using partial Gram-Schmidt orthogonolisation [6] is equivalent to incomplete Cholesky decomposition, in the sense that incomplete Cholesky decomposition can be seen as a dual implementation of partial Gram-Schmidt. • We show that the general approach can be adapted to two different types of problems, content and mate retrieval, by only changing the selection of eigenvectors used in the semantic projection. • To simplify the learning of the KCCA we explore a method of selecting the regularization parameter a priori such that it gives a value that performs well in several different tasks. In this study we also present a generalisation of the framework for canonical correlatis based on the works of Gifi (1990) and Ketterling (1971). The purpose of the generalisation is to extend the canonical correlation as an associativity measure between two set of variables to more than two sets, whilst preserving most of its properties. The generalisation starts with the optimisation problem formulation of canonical correlation. By changing the objective function we will arrive at the multi set problem. Applying similar constraint sets in the optimisation problems we find that the feasible solutions are singular vectors of matrices, which are derived the same way for the original and generalised problem. In Section 2 we present the theoretical background of CCA. In Section 3