Occam's Razor in Metacomputation the Notion of a Perfect Process Tree
4. Sentence Structure
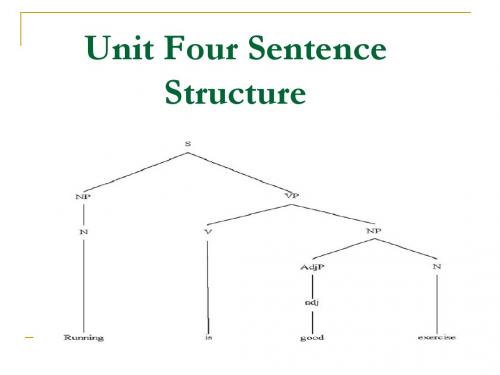
Different attitudes toward this issue
Under the bed is all dusty. Near the fire is quite a bit warmer.
In England some speakers are perfectly happy with these sentences. Some speakers are not. In America, it’s about fifty-fifty, half and half. In Australia, almost everyone likes these sentences. In New Zealand they won’t commit themselves.
√
AdvPs and PPs as subject
Slowly is exactly how he speaks.
They said that they were relieved that China was fighting
the global recession with an enormous fiscal stimulus program to spur domestic growth, and added that now was not the time to antagonize Beijing. ((/2009/11/15/world/asia/15china. html?_r=1&hp) )
The indirect object has the following characteristics:
Found with ditransitive verbs only; Characteristically a NP, but occasionally be a finite nominal clause, e.g.
范数规则化(L0,核范数等)

机器学习中的范数规则化之(一)L0、L1与L2范数zouxy09@ /zouxy09今天我们聊聊机器学习中出现的非常频繁的问题:过拟合与规则化。
我们先简单的来理解下常用的L0、L1、L2和核范数规则化。
最后聊下规则化项参数的选择问题。
这里因为篇幅比较庞大,为了不吓到大家,我将这个五个部分分成两篇博文。
知识有限,以下都是我一些浅显的看法,如果理解存在错误,希望大家不吝指正。
谢谢。
监督机器学习问题无非就是“minimize your error while regularizing your parameters”,也就是在规则化参数的同时最小化误差。
最小化误差是为了让我们的模型拟合我们的训练数据,而规则化参数是防止我们的模型过分拟合我们的训练数据。
多么简约的哲学啊!因为参数太多,会导致我们的模型复杂度上升,容易过拟合,也就是我们的训练误差会很小。
但训练误差小并不是我们的最终目标,我们的目标是希望模型的测试误差小,也就是能准确的预测新的样本。
所以,我们需要保证模型“简单”的基础上最小化训练误差,这样得到的参数才具有好的泛化性能(也就是测试误差也小),而模型“简单”就是通过规则函数来实现的。
另外,规则项的使用还可以约束我们的模型的特性。
这样就可以将人对这个模型的先验知识融入到模型的学习当中,强行地让学习到的模型具有人想要的特性,例如稀疏、低秩、平滑等等。
要知道,有时候人的先验是非常重要的。
前人的经验会让你少走很多弯路,这就是为什么我们平时学习最好找个大牛带带的原因。
一句点拨可以为我们拨开眼前乌云,还我们一片晴空万里,醍醐灌顶。
对机器学习也是一样,如果被我们人稍微点拨一下,它肯定能更快的学习相应的任务。
只是由于人和机器的交流目前还没有那么直接的方法,目前这个媒介只能由规则项来担当了。
还有几种角度来看待规则化的。
规则化符合奥卡姆剃刀(Occam's razor)原理。
这名字好霸气,razor!不过它的思想很平易近人:在所有可能选择的模型中,我们应该选择能够很好地解释已知数据并且十分简单的模型。
辐射3 中文版控制台代码
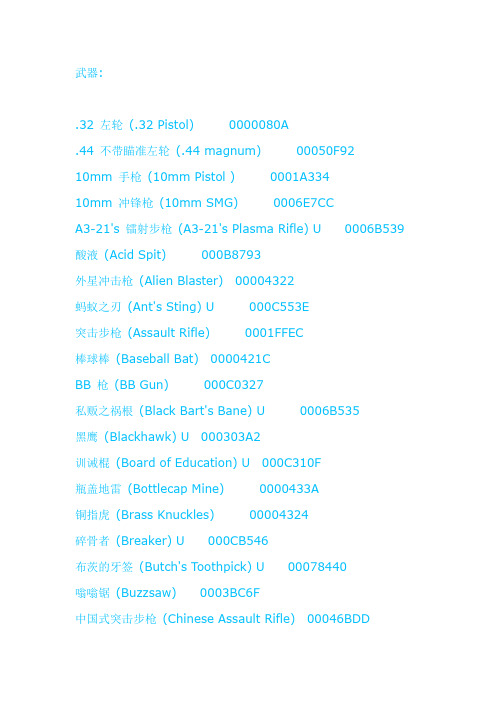
武器:.32 左轮(.32 Pistol) 0000080A.44 不带瞄准左轮(.44 magnum) 00050F9210mm 手枪(10mm Pistol ) 0001A33410mm 冲锋枪(10mm SMG) 0006E7CCA3-21's 镭射步枪(A3-21's Plasma Rifle) U 0006B539 酸液(Acid Spit) 000B8793外星冲击枪(Alien Blaster) 00004322蚂蚁之刃(Ant's Sting) U 000C553E突击步枪(Assault Rifle) 0001FFEC棒球棒(Baseball Bat) 0000421CBB 枪(BB Gun) 000C0327私贩之祸根(Black Bart's Bane) U 0006B535黑鹰(Blackhawk) U 000303A2训诫棍(Board of Education) U 000C310F瓶盖地雷(Bottlecap Mine) 0000433A铜指虎(Brass Knuckles) 00004324碎骨者(Breaker) U 000CB546布茨的牙签(Butch's Toothpick) U 00078440嗡嗡锯(Buzzsaw) 0003BC6F中国式突击步枪(Chinese Assault Rifle) 00046BDD中国式军官宝剑(Chinese Officer's Sword) 0006415D驳壳枪(Chinese Pistol) 00004325祝融V418驳壳枪(Chinese Pistol (Zhu-Rong v418 ))U 00060C2C克劳尔的贴肉刀(Clover's Cleaver) U 000C80B8考尔.奥特姆的10mm手枪(Col. Autumn's 10mm Pistol)0006B531考尔.奥特姆的镭射手枪(Col. Autumn's Laser Pistol)U 000ABBE4战斗匕首(Combat Knife) 00004326半自动散弹枪(Combat Shotgun) 0003713D天谴撕碎者(Curse Breaker) U 000C80BB镖枪(Dart Gun) 0000432A死亡拳爪(Deathclaw Gauntlet) 0000432B电晕(Electrical Zap) 00022FF1尤金(多管机枪)(Eugene) U 0006B538王者之棍(Excalibat) U 000C80BCMIRV 核弹群发器(Experimental MIRV) U 0003422B胖子核弹(Fat Man) 0000432C福克斯的超级动力锤(Fawkes' Super Sledge) U 0007843F 消防栓(Fire Hydrant) 00021367火焰刀(Firelance) 000C80BA拳霸(Fisto!) U 000CB601火焰喷射器(Flamer) 00078C60手雷(Frag Grenade) 00004330地雷(Frag Mine) 0000433C加特林镭射枪(Gatling Laser) 0000432E臂部镭射Hand Laser 00018B9E劫道棍(Highwayman's Friend) U 00078442狩猎步枪(Hunting Rifle) 00004333杰克(Jack) U 000C6E5B刀(Knife) 00004334镭射(Laser) 00050ED0镭射手枪(Laser Pistol) 000B4178镭射步枪(Laser Rifle ) 00074795执法者手枪(Law Dog) U 0006B532铅管(Lead Pipe) 00004337自由镭射枪(Liberty Laser ) 00033FE2自由能量炸弹(LibertyPrimeWeapBomb ) 0005932F 林肯拉杆枪(Lincoln's Repeater ) 0003C07A爱痕指虎(Love Tap ) U 000C80B9迷魂枪(Mesmeron) 00004339多管机枪(Minigun ) 0000433F诱饵手雷(Mirelurk Bait Grenade) 00030664捣蛋发射器(Miss Launcher ) U 000B2644导弹发射器(Missile Launcher) 00057E8F射钉枪(Nail Board) 000A01DD可乐手雷(Nuka-Grenade ) 00004342奥'格雷迪的和平缔造者(O'Grady's Peacemaker) U 0007843D奥卡姆剃刀(Occam's Razor) U 000CB602无痛杀手(Ol' Painless ) U 00066C76等离子手雷(Plasma Grenade) 00004332等离子枪(Plasma Gun) 0007C10C等离子地雷(Plasma Mine) 0000433D等离子手枪(Plasma Pistol) 00004343等离子步枪(Plasma Rifle) 00004344布朗克的说服(Plunkett's Valid Points ) U 000CAFA9警棍(Police Batton) 00004345台球杆(Pool Cue) 00004346动力拳套(Power Fist ) 00004347守护者的凝视光线(Protectron's Gaze) U 000C553F脉冲手雷(Pulse Grenade) 00004331脉冲地雷(Pulse Mine) 0000433E放射性喷雾(Radioactive Spit) 00058717轨道射钉枪(Railway Rifle) 00004348驱逐棍(Repellent Stick ) 0002D3B7预备役步枪(Reservist's Rifle) U 00092966开膛手(Ripper ) U 00004349垃圾发射器(Rock-it launcher ) 0000434B面粉杆(Rolling Pin) 00029769短管散弹枪(Sawed-Off Shotgun ) 0000434C带瞄准镜的.44手枪(Scoped .44 Magnum) 0000434D火焰刀(Shishkebab) 0000434E尖叫(Shriek) 0007F598消音10mm 手枪(Silenced 10mm Pistol) 00004350浆纱刀(Slasher Knife) 0002869C大锤(Sledge Hammer) 00004351电子充能包(Smuglers End (Laser Pistol)) 0006B536狙击步枪(Sniper Rifle ) 00004353刺指虎(Spiked Knuckels) 00004354刀刀乐(Stabhappy ) U 000C80BE超级动力锤(Super Sledge ) 000B0E7C折叠刀(Switchblade) 0006407F希尼的10mm冲锋枪(Sydney's 10mm "Ultra" SMG) U 0005DEEE连击球杆(The Break) U 00066C77锁膝枪(The Kneecapper) U 0006B53A震慑拳套(The Shocker) U 000BFF62软骨锤(The Tenderizer) U 000A874B恐怖散弹枪(The T errible Shotgun) U 0006B534卸胎棒(Tire Iron) 00004328吸血鬼之刺(Vampire's Edge) U 00078441飞鸟轰击炮(Vertibird Bomb Gun) 0003E5E2飞鸟机炮(Vertibird Gun) 00089C51胜利狙击步枪(Victory Rifle ) U 000CB548接着弹药:弹药:.308 (.308 Caliber Round) 0006B53C 不多说了.32 (.32 Caliber Round) 000207F7 不多说了.44 (.44 Round Magnum) 0002937E 不多说了10毫米(10mm Round) 00004241 不多说了5.56毫米(5.56mm Round) 00078CC4 不多说了5毫米(5mm Round) 0006B53D 不多说了外星能量电池(Alien Powercell) 00029364 外星枪BB弹(BB - Ammo) 0002935B 不多说了飞镖(Dart) 00047419 毒镖枪电子充能包(Electron Charge Pack) 000615AF 镭射加特林能量电池(Energy Cell) 00020772 镭射手枪燃料(Flamer Fuel) 00029371 火焰喷射器迷魂枪能量电池(Mesmetron Power Cell ) 0006A80D 迷魂枪聚合电池(Microfusion Cell) 00078CC3 镭射步枪迷你核弹(Mini Nuke ) 00020799 不多说了导弹(Missile) 000B8791 不多说了钉子(Railway Spikes) 00029384 不多说了散弹(Shotgun Shell) 00028EEA 不多说了索尼克能量包(Sonic Energy) 00056634 这个不知道装甲来了:装甲:动力头盔00014C08动力装甲00014E13牛皮外套00018DE5英克雷科学家外套0001B5BD荒地流浪者外套0001BA00彩色眼镜0001C295避难所实验室外套0001CBDC101避难所保安装甲0001CBDD僵尸面具0001DC1C战前休闲服0001EA6D战斗装甲0001EA6D皮革装甲00020423战斗头盔00020426执法者风衣00020429隧道蛇外套0002042E掠夺者带刺装甲0002042F掠夺者变态头盔00020432游骑兵战役装甲00023030柳钉城保安头盔00023030柳钉城保安装甲000239CC被放逐者动力装甲00023B62 屁屁男孩手套00025B83侦查装甲头盔00028EAD童帽00028FF8战前棒球帽00028FF9眼镜帽00028FFA粗糙商人外套0002B385麦克格雷迪的头盔0002D11B 奴隶帽0002F563侦查装甲0003064D放辐射外衣00033078高级放辐射外衣0003307A掠夺者虐待装甲0003307C掠夺者荒地装甲0003307D掠夺者爆破装甲0003307E金属盔甲0003307F金属头盔00033080曲棍球面具00033598棒球童帽000340C1战前童装000340C3战前童装(脏)000340C4战前帽子000340C8战前软帽000340CD战前休闲服(脏)000340CF战前春装000340D0战前春装(脏)000340D1中国伞兵战斗服000340D2中国伞兵战斗服(脏)000340D3 实验室技师外套000340D7科学家外套000340D9李博士的外套000340DA英克雷军官统一外套000340DE儿童警察帽子000340E1儿童地下老鼠外套000340E4性感睡衣000340E6麦克格雷迪的外套000340E7绿洲长袍000340E8绿洲村民长袍000340E9红色紧身衣000340EA红色头巾000340EB87避难所紧身衣000340ED112避难所紧身衣000340EF101避难所紧身儿童衣000340F2破烂外套000340F8下阶军官外套000340F9荒地侦查者外套000340FA荒地运动外套000340FB一品脱大小的猛砍面具000340FC 眼镜000340FD荒地医生外套000340FE荒地外科医生外套000340FF守护者战役头盔00034105十便士统一保安服00034119若比科紧身衣0003411A红色赛车手紧身衣0003411B101避难所保安头盔0003411C 蚂蚁女装甲0003411D机器人装甲0003411E蚂蚁女头盔0003411F机器人头盔00034120101避难所装甲紧身衣00034121 迷茫者魔术外套00034122迷茫者多事外套00034123迷茫者冒险外套00034124赞茨琼斯的帽子00034126赏金猎人风衣0003E54A口罩0003E591101避难所使用紧身衣000425BA 英克雷动力装甲0004443E林肯的帽子0004445B眼球头盔0004E6A0联盟帽子00050E44奥托姆上校制服0005157E玛帕尔的树衣0005A6CA婆佩拉的兜帽0005A6CB星星睡衣0005B6EA十便士安全头盔0005B6EB战前秋装0005BB63战前公园散步装0005BB66战前秋装(脏)0005BB6F战前公园散步装(脏)0005BB70战前商务套装(脏)0005C682太阳眼镜0005C99F李博士的眼镜0005C9A0皲裂眼镜0005C9A1大框眼镜0005DC82放逐者动力装甲00060C70放逐者动力头盔00060C72军用动力装甲00061A72里昂的装甲00061A73放逐者--头盔000645ED神秘陌生人外套0006696D战前春装(脏)00066C71特斯拉动力装甲0006B464特斯拉动力头盔0006B465神秘陌生人帽子0006B467101避难所改装装甲0006C587林登的被放逐者动力装甲00070877大头巾00073D57三狗的外套00073FEC雷德克斯的曲棍球面具0007401C 头巾00074296星星头盔0007494C破烂的帽子00074950荒地侦查帽子00074952兄弟会动力装甲00075201钢铁兄弟会动力头盔00075201 钢铁兄弟会动力装甲00075203 兄弟会动力头盔00075203医疗原型动力装甲0007836E地鼠莫瑞帽00078643掠夺者电焊头盔00078644掠夺者野狗头盔00078645中国军帽00078646英克雷军官帽子00078647粗糙商人帽子00078648爸爸的外套00079F09儿童太空帽0007C109改装使用紧身衣0007C17S遮阳帽0007C17D甏日聚会帽0007CFF0战前外套与手表0008198C万斯的长外套00083C5C兄弟会抄写者长袍000854CF里昂的长袍00087274内衣00089B52巴顿的假发0008A6DD迷茫者专家外套0008C83C特斯拉装甲0008F571恐怖头巾0008F775十便士先生的套装00096CB7儿童眼镜0009B185摩托车头盔0009B186摩托车护目镜0009B188风暴猎人帽子0009B189绿洲德鲁伊教团头巾0009B18A 迷茫者噪音外套000A3045鹰爪公司装甲000A6F76鹰爪战斗装甲000A6F76T-51B动力装甲000A6F77T-51B动力头盔000A6F78警帽000AB491三狗的眼镜000ACDA4鹰爪战斗头盔000B0278战前商务套装(脏破)000B1056 乌鸦的眼球头盔000B17A0 108避难所紧身衣000B73F1 106避难所紧身衣000B73F292避难所紧身衣000B73F2聚会三角帽000B75E2杂工紧身衣000BF6FD全面防护服000C09D4简易防毒面具000C111A里斯科的实验室外套000C24F9 迷茫者巡逻外套000C5D34实验室外科医生外套000C71E1 塔克玛公园联盟帽子000C72FB 消防员面具000C7C4E流浪者皮甲000C7C54顽皮睡衣000C8E07绿洲放逐者头巾000C942D77避难所紧身衣000CAFBE辩护者装甲000CB543民兵装甲000CB544冒险家外套000CB549幸运太阳镜000CB549陈旧的战斗盔甲000CB5EF 陈旧的战斗头盔000CB5F0 英克雷骑兵装甲000CB5F3 英克雷骑兵头盔000CB5F4 流氓皮革装甲000CB5F5启示者装甲000CB5F5启示者头盔000CB5F7罗比动力头盔000CB5F8复合侦查装甲000CB5FA复合侦查头盔000CB5FB掠夺者时髦装甲000CB5FC 竖辫头盔000CB5FD穿过的掠夺者装甲000CB5FE 公路匪徒装甲000CB5FF帕罗头盔000CB600中国伞兵战斗服(脏)000CB603 普通帽子000CB604通用研究服000CB605荒地传说外套000CB609商务套装000CB60C书籍:科技全书0003403C中国陆军训练手册00034045D.C内科医学杂志00034043迪恩的电子学0003403D卧倒和掩护0006A80C格罗尼野蛮人00034040枪炮和子弹0003403E说谎,国会风格00034044星体运动的秘密0002D3A3诺克拉.特斯拉和你00034041失落的天堂000BACFE拳击技巧0003403F废土生存手稿0002D3B2垃圾镇商人传奇00034042今天为生存打滚的人00034046美国陆军:30个便利火焰喷射器食谱00034048 废土生存手册000C5634你是特别的000AB2EF其他:发夹0000000A 瓶盖0000000F 手指000604DE。
乔姆斯基的生成语法
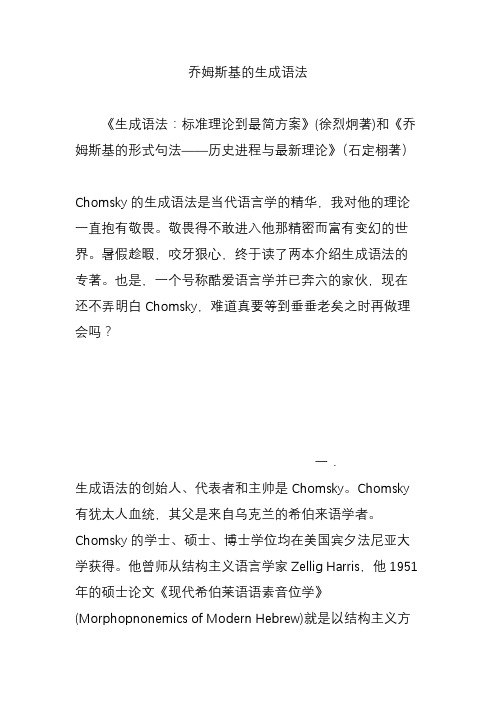
乔姆斯基的生成语法《生成语法:标准理论到最简方案》(徐烈炯著)和《乔姆斯基的形式句法——历史进程与最新理论》(石定栩著)Chomsky的生成语法是当代语言学的精华,我对他的理论一直抱有敬畏。
敬畏得不敢进入他那精密而富有变幻的世界。
暑假趁暇,咬牙狠心,终于读了两本介绍生成语法的专著。
也是,一个号称酷爱语言学并已奔六的家伙,现在还不弄明白Chomsky,难道真要等到垂垂老矣之时再做理会吗?一.生成语法的创始人、代表者和主帅是Chomsky。
Chomsky 有犹太人血统,其父是来自乌克兰的希伯来语学者。
Chomsky的学士、硕士、博士学位均在美国宾夕法尼亚大学获得。
他曾师从结构主义语言学家Zellig Harris,他1951年的硕士论文《现代希伯莱语语素音位学》(Morphopnonemics of Modern Hebrew)就是以结构主义方法进行研究的。
1955年,27岁的他完成博士论文《转换分析》,获得博士学位。
他在博士论文基础上,写成了著名的《句法结构》一书于1957年出版。
Chomsky后来一直在麻省理工学院的现代语言学系任教,麻省理工学院成了生成语法的大本营。
《句法结构》的出版打破了结构主义在语言学的统治,标志着生成语法的问世。
Chomsky的生成语法被认为是20世纪现代语言学研究上最伟大的贡献,在语言研究领域引发了堪称“乔姆斯基革命”的突破。
Chomsky对语言的看法大致可以归纳为以下几点:一.语言反映了心理。
Chomsky一再强调他研究的语言学是心理学,最终是研究大脑的生物学,语言过程实为某种“心智表现”或“心智运算”。
心智(mind)宛如计算机内的程序。
他进而提出语言学应纳入生物语言学(Biolinguistics)即语言的生物学(biology of linguistics)。
Chomsky 等多数生成语言学家认为语言学是经验科学,他们有的甚至认为语言学属于自然科学,而不是通常认为的属于社会科学。
100个最流行的营销词汇
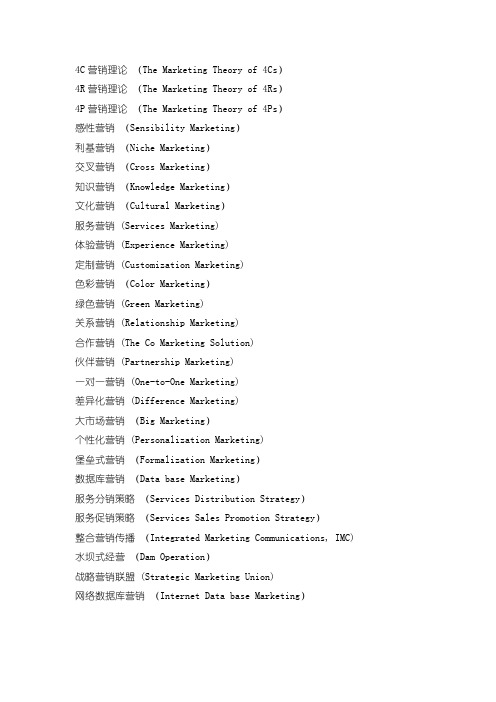
4C营销理论(The Marketing Theory of 4Cs)4R营销理论(The Marketing Theory of 4Rs)4P营销理论(The Marketing Theory of 4Ps)感性营销(Sensibility Marketing)利基营销(Niche Marketing)交叉营销(Cross Marketing)知识营销(Knowledge Marketing)文化营销(Cultural Marketing)服务营销 (Services Marketing)体验营销 (Experience Marketing)定制营销 (Customization Marketing)色彩营销(Color Marketing)绿色营销 (Green Marketing)关系营销 (Relationship Marketing)合作营销 (The Co Marketing Solution)伙伴营销 (Partnership Marketing)一对一营销 (One-to-One Marketing)差异化营销 (Difference Marketing)大市场营销(Big Marketing)个性化营销 (Personalization Marketing)堡垒式营销(Formalization Marketing)数据库营销(Data base Marketing)服务分销策略(Services Distribution Strategy)服务促销策略(Services Sales Promotion Strategy)整合营销传播(Integrated Marketing Communications, IMC)水坝式经营(Dam Operation)战略营销联盟 (Strategic Marketing Union)网络数据库营销(Internet Data base Marketing)“整时营销”与“晚盈利”(Profit by Timing Marketing and Lag Profit Marketing)管理篇目标管理(Management by Objectives, MBO),现在这个缩写也常用于代称“管理层收购”(Management Buy Out)标杆瞄准(Benchmarking)开明管理(Open Management)宽容管理(Allowance Management)危机管理(Crisis Management)标杆管理(Benchmarking Management)人格管理(Character Management)品牌管理(Brand Management)变革管理(Change Management)沟通管理(Communication Management)走动管理(Management by Walking Around,MBWA)价值管理(Value Management)钩稽管理(Innovation and Practice Management)能本管理 (Capacity Core Management)绩效管理(Managing For Performance)赋权管理(Delegation Management)灵捷管理(Celerity Management)物流管理 (Logistics Management/Physical Distribution ) (Physical Distribution为传统意义上的物流)知识管理(Knowledge Management)时间管理(Time-Management)互动管理(Interactive Management)T型管理 (T Management)预算管理(Budget Management)末日管理(End Management)柔性管理 (Soft Management)例外管理(Exception Management)K型管理 (K Management)EVA管理 (Economic Value Added, EVA)5S管理法(5S :Seiri、Seiten、Seigo、Seiketsu、Shitsuke)零缺陷管理(Zero Defects)一分钟管理(One Minute Management)供应链管理(Supply Chain Management, SCM)客户关系管理(Customer Relationship Management,CRM)产品数据管理 (Product Data Management, PDM)过程质量管理法(Process of Quality Management)管理驾驶舱(Cockpit of Management)OEC管理法 (Over All Every Control and Clear)数字化管理 (Digital Management)海豚式管理 (Management as Porpoise)丰田式管理(Toyota- Management)跨文化管理(Span-Culture Management)蚂蚁式管理(Style of Ant Management)购销比价管理(Purchase by Grade Management)企业内容管理(Enterprise Content Management)企业健康管理(Health of Enterprise Management)薪酬外包管理(Salary Episodic Management)戴明的质量管理 (William Edwards Dem’s Quality Management)六西格玛管理法 (Six Sigma)倒金字塔管理(Handstand Pyramidal Management)变形虫式管理 (Amoeba Management)</P< p>定律篇木桶定律(Cannikin Law)墨菲定律 (Moffe’s Law)羊群效应(Sheep-Flock Effect)帕金森定律(Parkinson’s Law)华盛顿合作定律 (Washington Company Law)手表定律(Watch Law)蘑菇定律(Mushroom Law)鲇鱼效应(Weever Effect)飞轮效应(Flywheel Effect)光环效应(Halo Effect)马太效应(Matthew Effect)蝴蝶效应(Butterfly Effect)多米诺效应(Domicile Effect)皮格马利翁效应(Pygmalion Effect)彼德原理 (The Peter Principle)破窗理论(Break Pane Law)路径依赖(Path Dependence)奥卡姆剃刀(Occam’s Razor)博弈论 (Game Theory)定位法则(Orientation Law)80/20原理(80/20 Law)X理论-Y理论(Theory X- Theory Y)超Y理论(Exceed theory Y)综合篇7S模型(Principle of 7S)ABC分析法(ABC-Analysis)SWOT分析 (SWOT Analysis)波士顿矩阵法(Boston Matrix Analysis)新7S原则 (Principle of New 7S)PDCA循环(PDCA Cyc)平衡记分卡 (Balanced Score Card)品管圈(Quality Control Circle,QCC)零库存(In-Time Inventory)顾客份额(Constituency Share)业务流程重组 (Business Process Reengineer)动态薪酬(Dynamic Salary)管理审计(Managed Audit)管理层收购(Management Buy-out)逆向供应链 (Reverse Supply Chain)宽带薪酬设计(Broad Band Salary Design)员工持股计划(Employee Stock Ownership Plan,ESOP)人力资源外包(Epiboly HR)360度绩效反馈 (360-Degree Performance Feedback)人力资源价值链(Human Resource Value Chain柯氏模式(Kirkpatrick Model)归因模型(Attribution Model)期望模型(Expectancy Model)五力模型(The Five-force Model)安东尼模型(Anthony Model)CS经营战略(Customer Satisfaction)532绩效考核模型(532 Performance Appraisal Model)101℃理论(101℃Theory)双因素激励理论(Dual Stimulant Theory)注意力经济(The Economy of Attention)灵捷竞争(Adroitly Compete)德尔菲法(Delphi Technique)执行力(Execution)领导力(Leadership)学习力(Learning Capacity)企业教练(Corporate Coach)首席知识官(Chief Knowledge Officer)第五级领导者(Fifth Rank Leader)智力资本(Intellect Capital)智能资本(Intellectual Capital)高情商团队(High EQ Team)学习型组织(Learning Organization)知识型企业(Knowledge Enterprise)高智商企业(Knowledge-Intensive Enterprise)灵捷组织(Adroitly Organization)虚拟企业(Virtual Enterprise,VE)</P< p>。
营销管理的常用模型和理论

营销管理的常用模型和理论多点竞争战略杜邦分析法(DuPont Analysis)GE矩阵(GE Matrix/Mckinsey Matrix)盖洛普路径(The Gallup Path)竞争资源四层次模型价值链信息化管理竞争优势因果关系模式竞争对手分析工具完整价值链分析(VCA)的基本原理脚本法(Scenarios,Scenario Analysis)KT决策法(KT Matrix)扩张方法矩阵利益相关者分析(StakeholderAnalysis)雷达图分析法卢因的力场分析法六顶思考帽(Six Thinking Hats)华信惠悦人力资本指数(HCI,Human Capital Index)横向价值链分析行业内战略集团分析基本竞争战略(Generic Competitive Strategies)竞争战略三角模型(Triangle Model)价值网模型(value net)绩效棱柱模型(Performance Prism)利润库分析法核心竞争力分析模型(Core competence analysis)麦肯锡7S模型(Mckinsey 7S Model)麦肯锡三层面理论(Three aspect theories)麦肯锡逻辑树分析法诺兰的阶段模型PEST分析模型(PEST Analysis)PESTEL分析模型PAEI管理角色模型PIMS分析佩罗的技术分类企业素质与活力分析QFD法ECR系统(Efficient Consumer Response,有效消费者反应系统) SECI模型(SECI Model)过程决策程序图法(PDPC法,Process Decision Program Chart)树状图(Tree Diagram or Dedrogram)关联图法(Inter-relationship diagraph)KJ法又称A型图解法、亲和图法(Affinity Diagram信息孤岛管理信息系统(Management Information System,简称MIS)SWOT分析模型SCOR模型SFO模型SCP分析模型安索夫矩阵三维商业定义ADL矩阵安迪•格鲁夫的六力分析模型标杆分析SERVQUAL模型鱼骨图头脑风暴法PDCA循环帕累托法则(Pareto Principle,80/20法则)SMART原则ABC分类法(Activity Based Classification)KPI(Key Performance Indicator,关键绩效指标)波特钻石理论模型(Michael Porter diamond Model)波特竞争战略轮盘模型定向政策矩阵(Directional Policy Matrix,指导性政策矩阵,简称DPM或DP矩阵) 二元核心模式(dual-core approach)服务金三角(Service Triangle)福克纳和鲍曼的顾客矩阵服务质量(Quality of Service,QoF)全面质量管理朱兰的质量三元论DMAIC模型六西格玛(Six Sigma)差距分析(Gap Analysis,又称缺口分析、差异分析)CSP模型QSPM矩阵战略地位与行动评价矩阵(SPACE矩阵)波士顿矩阵(BCG Matrix)BCG三四规则矩阵波士顿经验曲线(BCG Experience Curve)内部因素评价矩阵(Internal Factor Evaluation Matrix,IFE矩阵)外部因素评价矩阵(EFE矩阵)内部-外部矩阵(Internal-External Matrix,IE矩阵)大战略矩阵(Grand Strategy Matrix)变革五因素策略方格模型(Strategic Grid Model)波特行业竞争结构分析模型价值链管理(Value Chain Management,VCM)学习型组织(Learning Organization)企业价值关联分析模型企业竞争力九力分析模型企业战略五要素分析法人力资源成熟度模型(People Capability Maturity Model,PCMM)人力资源经济分析RA TER指数RFM模型瑞定的学习模型GREP模型3C战略三角模型汤姆森和斯特克兰方法V矩阵陀螺理论(Gyroscope Theory)SIPOC模型战略钟模型战略地图(Strategy Map)组织成长阶段模型(Growth_Phases Model)战略选择矩阵管理要素分析模型360度薪酬目标管理(mbo)鱼缸会议情形分析图TDC矩阵(Time Distance Complexity Matrix)QQTC模型PARTS战略(Participators、Added values、Rules、Tactics、Scope)布莱克的管理方格理论(Management Grid Theory)布雷德福的敏感性训练理论(Sensitivity Training)情绪ABC理论期望理论(Expectancy Theory)“复杂人”假设赫兹伯格的双因素激励理论霍桑效应奥卡姆剃刀定律(Occam's Razor, Ockham's Razor)符号互动论(symbolic interactionism)戈夫曼拟剧论(Goffman's Dramaturgical Theory)常人方法学(Ethnomethodology)皮格马利翁效应(Pygmalion Effect)利克特的支持关系理论(Support Relation Theory)马斯洛人类需求五层次理论(Maslow's Hierarchy of Needs) X理论和Y理论(Theory X and Theory Y)理性选择理论(Rational Choice Theory)心理定格(Frames)团体力学理论社会惰化(social loafing)领导行为连续体理论(Leadership Continuum)“自我实现人”假设(Self-Actualizing Man)战略群模型综合战略理论重要性-迫切性模型(PQM)知识链模型(Knowledge Chain)知识价值链模型(Knowledge value chain, KVC)知识螺旋(Knowledge Spiral)平衡计分卡(The Balanced ScoreCard,简称BSC)组织结构模型供应链管理(Supply Chain Management ,简称SCM)波特价值链分析模型(Michael Porter's Value Chain Model) 客户关系管理(Customer Relationship Management,CRM) PIMS分析(Profit Impact of Market Strategies)企业核心能力(Core Capability of Enterprise)BPR(Business Process Reengineering/Business Process Re-engineering,业务流程重)。
人工智能英汉
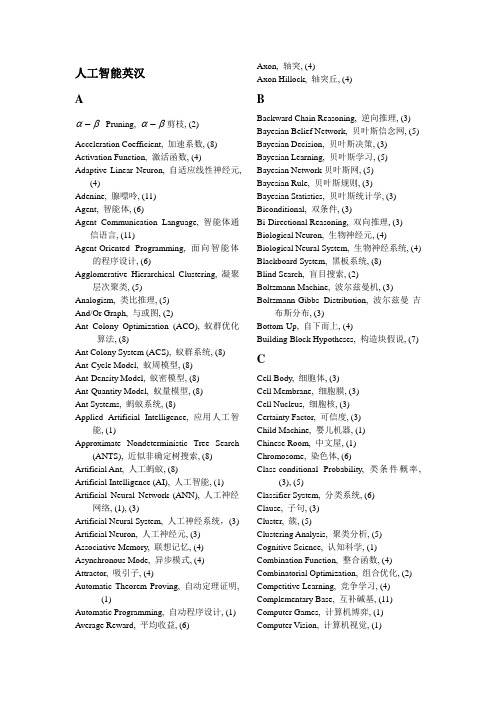
人工智能英汉Aβα-Pruning, βα-剪枝, (2) Acceleration Coefficient, 加速系数, (8) Activation Function, 激活函数, (4) Adaptive Linear Neuron, 自适应线性神经元,(4)Adenine, 腺嘌呤, (11)Agent, 智能体, (6)Agent Communication Language, 智能体通信语言, (11)Agent-Oriented Programming, 面向智能体的程序设计, (6)Agglomerative Hierarchical Clustering, 凝聚层次聚类, (5)Analogism, 类比推理, (5)And/Or Graph, 与或图, (2)Ant Colony Optimization (ACO), 蚁群优化算法, (8)Ant Colony System (ACS), 蚁群系统, (8) Ant-Cycle Model, 蚁周模型, (8)Ant-Density Model, 蚁密模型, (8)Ant-Quantity Model, 蚁量模型, (8)Ant Systems, 蚂蚁系统, (8)Applied Artificial Intelligence, 应用人工智能, (1)Approximate Nondeterministic Tree Search (ANTS), 近似非确定树搜索, (8) Artificial Ant, 人工蚂蚁, (8)Artificial Intelligence (AI), 人工智能, (1) Artificial Neural Network (ANN), 人工神经网络, (1), (3)Artificial Neural System, 人工神经系统,(3) Artificial Neuron, 人工神经元, (3) Associative Memory, 联想记忆, (4) Asynchronous Mode, 异步模式, (4) Attractor, 吸引子, (4)Automatic Theorem Proving, 自动定理证明,(1)Automatic Programming, 自动程序设计, (1) Average Reward, 平均收益, (6) Axon, 轴突, (4)Axon Hillock, 轴突丘, (4)BBackward Chain Reasoning, 逆向推理, (3) Bayesian Belief Network, 贝叶斯信念网, (5) Bayesian Decision, 贝叶斯决策, (3) Bayesian Learning, 贝叶斯学习, (5) Bayesian Network贝叶斯网, (5)Bayesian Rule, 贝叶斯规则, (3)Bayesian Statistics, 贝叶斯统计学, (3) Biconditional, 双条件, (3)Bi-Directional Reasoning, 双向推理, (3) Biological Neuron, 生物神经元, (4) Biological Neural System, 生物神经系统, (4) Blackboard System, 黑板系统, (8)Blind Search, 盲目搜索, (2)Boltzmann Machine, 波尔兹曼机, (3) Boltzmann-Gibbs Distribution, 波尔兹曼-吉布斯分布, (3)Bottom-Up, 自下而上, (4)Building Block Hypotheses, 构造块假说, (7) CCell Body, 细胞体, (3)Cell Membrane, 细胞膜, (3)Cell Nucleus, 细胞核, (3)Certainty Factor, 可信度, (3)Child Machine, 婴儿机器, (1)Chinese Room, 中文屋, (1) Chromosome, 染色体, (6)Class-conditional Probability, 类条件概率,(3), (5)Classifier System, 分类系统, (6)Clause, 子句, (3)Cluster, 簇, (5)Clustering Analysis, 聚类分析, (5) Cognitive Science, 认知科学, (1) Combination Function, 整合函数, (4) Combinatorial Optimization, 组合优化, (2) Competitive Learning, 竞争学习, (4) Complementary Base, 互补碱基, (11) Computer Games, 计算机博弈, (1) Computer Vision, 计算机视觉, (1)Conflict Resolution, 冲突消解, (3) Conjunction, 合取, (3)Conjunctive Normal Form (CNF), 合取范式,(3)Collapse, 坍缩, (11)Connectionism, 连接主义, (3) Connective, 连接词, (3)Content Addressable Memory, 联想记忆, (4) Control Policy, 控制策略, (6)Crossover, 交叉, (7)Cytosine, 胞嘧啶, (11)DData Mining, 数据挖掘, (1)Decision Tree, 决策树, (5) Decoherence, 消相干, (11)Deduction, 演绎, (3)Default Reasoning, 默认推理(缺省推理),(3)Defining Length, 定义长度, (7)Rule (Delta Rule), 德尔塔规则, 18(3) Deliberative Agent, 慎思型智能体, (6) Dempster-Shafer Theory, 证据理论, (3) Dendrites, 树突, (4)Deoxyribonucleic Acid (DNA), 脱氧核糖核酸, (6), (11)Disjunction, 析取, (3)Distributed Artificial Intelligence (DAI), 分布式人工智能, (1)Distributed Expert Systems, 分布式专家系统,(9)Divisive Hierarchical Clustering, 分裂层次聚类, (5)DNA Computer, DNA计算机, (11)DNA Computing, DNA计算, (11) Discounted Cumulative Reward, 累计折扣收益, (6)Domain Expert, 领域专家, (10) Dominance Operation, 显性操作, (7) Double Helix, 双螺旋结构, (11)Dynamical Network, 动态网络, (3)E8-Puzzle Problem, 八数码问题, (2) Eletro-Optical Hybrid Computer, 光电混合机, (11)Elitist strategy for ant systems (EAS), 精化蚂蚁系统, (8)Energy Function, 能量函数, (3) Entailment, 永真蕴含, (3) Entanglement, 纠缠, (11)Entropy, 熵, (5)Equivalence, 等价式, (3)Error Back-Propagation, 误差反向传播, (4) Evaluation Function, 评估函数, (6) Evidence Theory, 证据理论, (3) Evolution, 进化, (7)Evolution Strategies (ES), 进化策略, (7) Evolutionary Algorithms (EA), 进化算法, (7) Evolutionary Computation (EC), 进化计算,(7)Evolutionary Programming (EP), 进化规划,(7)Existential Quantification, 存在量词, (3) Expert System, 专家系统, (1)Expert System Shell, 专家系统外壳, (9) Explanation-Based Learning, 解释学习, (5) Explanation Facility, 解释机构, (9)FFactoring, 因子分解, (11)Feedback Network, 反馈型网络, (4) Feedforward Network, 前馈型网络, (1) Feasible Solution, 可行解, (2)Finite Horizon Reward, 横向有限收益, (6) First-order Logic, 一阶谓词逻辑, (3) Fitness, 适应度, (7)Forward Chain Reasoning, 正向推理, (3) Frame Problem, 框架问题, (1)Framework Theory, 框架理论, (3)Free-Space Optical Interconnect, 自由空间光互连, (11)Fuzziness, 模糊性, (3)Fuzzy Logic, 模糊逻辑, (3)Fuzzy Reasoning, 模糊推理, (3)Fuzzy Relation, 模糊关系, (3)Fuzzy Set, 模糊集, (3)GGame Theory, 博弈论, (8)Gene, 基因, (7)Generation, 代, (6)Genetic Algorithms, 遗传算法, (7)Genetic Programming, 遗传规划(遗传编程),(7)Global Search, 全局搜索, (2)Gradient Descent, 梯度下降, (4)Graph Search, 图搜索, (2)Group Rationality, 群体理性, (8) Guanine, 鸟嘌呤, (11)HHanoi Problem, 梵塔问题, (2)Hebbrian Learning, 赫伯学习, (4)Heuristic Information, 启发式信息, (2) Heuristic Search, 启发式搜索, (2)Hidden Layer, 隐含层, (4)Hierarchical Clustering, 层次聚类, (5) Holographic Memory, 全息存储, (11) Hopfield Network, 霍普菲尔德网络, (4) Hybrid Agent, 混合型智能体, (6)Hype-Cube Framework, 超立方体框架, (8)IImplication, 蕴含, (3)Implicit Parallelism, 隐并行性, (7) Individual, 个体, (6)Individual Rationality, 个体理性, (8) Induction, 归纳, (3)Inductive Learning, 归纳学习, (5) Inference Engine, 推理机, (9)Information Gain, 信息增益, (3)Input Layer, 输入层, (4)Interpolation, 插值, (4)Intelligence, 智能, (1)Intelligent Control, 智能控制, (1) Intelligent Decision Supporting System (IDSS), 智能决策支持系统,(1) Inversion Operation, 倒位操作, (7)JJoint Probability Distribution, 联合概率分布,(5) KK-means, K-均值, (5)K-medoids, K-中心点, (3)Knowledge, 知识, (3)Knowledge Acquisition, 知识获取, (9) Knowledge Base, 知识库, (9)Knowledge Discovery, 知识发现, (1) Knowledge Engineering, 知识工程, (1) Knowledge Engineer, 知识工程师, (9) Knowledge Engineering Language, 知识工程语言, (9)Knowledge Interchange Format (KIF), 知识交换格式, (8)Knowledge Query and ManipulationLanguage (KQML), 知识查询与操纵语言,(8)Knowledge Representation, 知识表示, (3)LLearning, 学习, (3)Learning by Analog, 类比学习, (5) Learning Factor, 学习因子, (8)Learning from Instruction, 指导式学习, (5) Learning Rate, 学习率, (6)Least Mean Squared (LSM), 最小均方误差,(4)Linear Function, 线性函数, (3)List Processing Language (LISP), 表处理语言, (10)Literal, 文字, (3)Local Search, 局部搜索, (2)Logic, 逻辑, (3)Lyapunov Theorem, 李亚普罗夫定理, (4) Lyapunov Function, 李亚普罗夫函数, (4)MMachine Learning, 机器学习, (1), (5) Markov Decision Process (MDP), 马尔科夫决策过程, (6)Markov Chain Model, 马尔科夫链模型, (7) Maximum A Posteriori (MAP), 极大后验概率估计, (5)Maxmin Search, 极大极小搜索, (2)MAX-MIN Ant Systems (MMAS), 最大最小蚂蚁系统, (8)Membership, 隶属度, (3)Membership Function, 隶属函数, (3) Metaheuristic Search, 元启发式搜索, (2) Metagame Theory, 元博弈理论, (8) Mexican Hat Function, 墨西哥草帽函数, (4) Migration Operation, 迁移操作, (7) Minimum Description Length (MDL), 最小描述长度, (5)Minimum Squared Error (MSE), 最小二乘法,(4)Mobile Agent, 移动智能体, (6)Model-based Methods, 基于模型的方法, (6) Model-free Methods, 模型无关方法, (6) Modern Heuristic Search, 现代启发式搜索,(2)Monotonic Reasoning, 单调推理, (3)Most General Unification (MGU), 最一般合一, (3)Multi-Agent Systems, 多智能体系统, (8) Multi-Layer Perceptron, 多层感知器, (4) Mutation, 突变, (6)Myelin Sheath, 髓鞘, (4)(μ+1)-ES, (μ+1) -进化规划, (7)(μ+λ)-ES, (μ+λ) -进化规划, (7) (μ,λ)-ES, (μ,λ) -进化规划, (7)NNaïve Bayesian Classifiers, 朴素贝叶斯分类器, (5)Natural Deduction, 自然演绎推理, (3) Natural Language Processing, 自然语言处理,(1)Negation, 否定, (3)Network Architecture, 网络结构, (6)Neural Cell, 神经细胞, (4)Neural Optimization, 神经优化, (4) Neuron, 神经元, (4)Neuron Computing, 神经计算, (4)Neuron Computation, 神经计算, (4)Neuron Computer, 神经计算机, (4) Niche Operation, 生态操作, (7) Nitrogenous base, 碱基, (11)Non-Linear Dynamical System, 非线性动力系统, (4)Non-Monotonic Reasoning, 非单调推理, (3) Nouvelle Artificial Intelligence, 行为智能,(6)OOccam’s Razor, 奥坎姆剃刀, (5)(1+1)-ES, (1+1) -进化规划, (7)Optical Computation, 光计算, (11)Optical Computing, 光计算, (11)Optical Computer, 光计算机, (11)Optical Fiber, 光纤, (11)Optical Waveguide, 光波导, (11)Optical Interconnect, 光互连, (11) Optimization, 优化, (2)Optimal Solution, 最优解, (2)Orthogonal Sum, 正交和, (3)Output Layer, 输出层, (4)Outer Product, 外积法, 23(4)PPanmictic Recombination, 混杂重组, (7) Particle, 粒子, (8)Particle Swarm, 粒子群, (8)Particle Swarm Optimization (PSO), 粒子群优化算法, (8)Partition Clustering, 划分聚类, (5) Partitioning Around Medoids, K-中心点, (3) Pattern Recognition, 模式识别, (1) Perceptron, 感知器, (4)Pheromone, 信息素, (8)Physical Symbol System Hypothesis, 物理符号系统假设, (1)Plausibility Function, 不可驳斥函数(似然函数), (3)Population, 物种群体, (6)Posterior Probability, 后验概率, (3)Priori Probability, 先验概率, (3), (5) Probability, 随机性, (3)Probabilistic Reasoning, 概率推理, (3) Probability Assignment Function, 概率分配函数, (3)Problem Solving, 问题求解, (2)Problem Reduction, 问题归约, (2)Problem Decomposition, 问题分解, (2) Problem Transformation, 问题变换, (2) Product Rule, 产生式规则, (3)Product System, 产生式系统, (3) Programming in Logic (PROLOG), 逻辑编程, (10)Proposition, 命题, (3)Propositional Logic, 命题逻辑, (3)Pure Optical Computer, 全光计算机, (11)QQ-Function, Q-函数, (6)Q-learning, Q-学习, (6)Quantifier, 量词, (3)Quantum Circuit, 量子电路, (11)Quantum Fourier Transform, 量子傅立叶变换, (11)Quantum Gate, 量子门, (11)Quantum Mechanics, 量子力学, (11) Quantum Parallelism, 量子并行性, (11) Qubit, 量子比特, (11)RRadial Basis Function (RBF), 径向基函数,(4)Rank based ant systems (ASrank), 基于排列的蚂蚁系统, (8)Reactive Agent, 反应型智能体, (6) Recombination, 重组, (6)Recurrent Network, 循环网络, (3) Reinforcement Learning, 强化学习, (3) Resolution, 归结, (3)Resolution Proof, 归结反演, (3) Resolution Strategy, 归结策略, (3) Reasoning, 推理, (3)Reward Function, 奖励函数, (6) Robotics, 机器人学, (1)Rote Learning, 机械式学习, (5)SSchema Theorem, 模板定理, (6) Search, 搜索, (2)Selection, 选择, (7)Self-organizing Maps, 自组织特征映射, (4) Semantic Network, 语义网络, (3)Sexual Differentiation, 性别区分, (7) Shor’s algorithm, 绍尔算法, (11)Sigmoid Function, Sigmoid 函数(S型函数),(4)Signal Function, 信号函数, (3)Situated Artificial Intelligence, 现场式人工智能, (1)Spatial Light Modulator (SLM), 空间光调制器, (11)Speech Act Theory, 言语行为理论, (8) Stable State, 稳定状态, (4)Stability Analysis, 稳定性分析, (4)State Space, 状态空间, (2)State Transfer Function, 状态转移函数,(6)Substitution, 置换, (3)Stochastic Learning, 随机型学习, (4) Strong Artificial Intelligence (AI), 强人工智能, (1)Subsumption Architecture, 包容结构, (6) Superposition, 叠加, (11)Supervised Learning, 监督学习, (4), (5) Swarm Intelligence, 群智能, (8)Symbolic Artificial Intelligence (AI), 符号式人工智能(符号主义), (3) Synapse, 突触, (4)Synaptic Terminals, 突触末梢, (4) Synchronous Mode, 同步模式, (4)TThreshold, 阈值, (4)Threshold Function, 阈值函数, (4) Thymine, 胸腺嘧啶, (11)Topological Structure, 拓扑结构, (4)Top-Down, 自上而下, (4)Transfer Function, 转移函数, (4)Travel Salesman Problem, 旅行商问题, (4) Turing Test, 图灵测试, (1)UUncertain Reasoning, 不确定性推理, (3)Uncertainty, 不确定性, (3)Unification, 合一, (3)Universal Quantification, 全称量词, (4) Unsupervised Learning, 非监督学习, (4), (5)WWeak Artificial Intelligence (Weak AI), 弱人工智能, (1)Weight, 权值, (4)Widrow-Hoff Rule, 维德诺-霍夫规则, (4)。
超级思维高效解决问题的20个思维模型

超级思维高效解决问题的20个思维模型很多时候,你可能会因为思绪混乱而无从说起,或者因为事情繁多而无比焦虑。
在遇到难以解决的问题时,绝大多数情况下都是因为思维不清、逻辑混乱所致。
在应对复杂问题、困难决策以及影响人生的障碍时,世界上最伟大的思想家、问题解决者以及决策者都会借助于一系列原则、捷径、框架以及聪明的思考工具。
本文介绍了20个高效解决问题的思维模型,希望能帮助你成为解决问题的高手。
编者按作者 | Thomas Oppong原题| Super Thinking: 20 Ideas to Add to Your Thinking Toolbox题源 | Devika Arora展开剩余92%译者 | 俊一转自 | 36氪在这篇文章中,我将跟大家分享一些自己惯用的思考原则。
我主要借助于这些思考原则,来改善并提高自身的理性思维。
这20个思维模型,有助于你更好地思考。
我希望它们能激发你的思维好奇心。
每一个思维模型都能为你提供一个不同的思考框架,让你从不同的角度来看待和思考生活。
1. 能力圈(Circle of competence)如果你希望提高自己在生活和事业方面的成功几率,那你就需要界定自身的知识界限。
一定要清楚地认识自己,自己知道的是什么,不知道的又是什么。
要始终坚持在能力圈内行事,并学会抵挡为了追逐更多利益而要跨出能力圈的诱惑。
与此同时,努力扩大自己的能力圈。
在确定能力圈界限后,当我们在相关领域的专业知识、分析、理念等方面的能力提高时,我们的能力圈自然就会扩大。
不过,在这个过程中,千万不要自欺欺人,一定要清晰地认识到自己所处的位置。
图片来源:hackernoon2. 纯粹接触效应(Mere-exposure effect)我们对某个方面的偏好,仅仅是由于熟悉它而形成的一种倾向。
就某个事物而言,你看到或听到的越多,那你就会越喜欢它,这也被称作熟悉定律(familiarity principle)。
- 1、下载文档前请自行甄别文档内容的完整性,平台不提供额外的编辑、内容补充、找答案等附加服务。
- 2、"仅部分预览"的文档,不可在线预览部分如存在完整性等问题,可反馈申请退款(可完整预览的文档不适用该条件!)。
- 3、如文档侵犯您的权益,请联系客服反馈,我们会尽快为您处理(人工客服工作时间:9:00-18:30)。
Occam’s Razor in Metacomputation:the Notion of a Perfect Process TreeRobert Glück1Andrei V. Klimov2Institut für Computersprachen Keldysh Institute of Applied Mathematics University of Technology Vienna Russian Academy of SciencesA-1040 Vienna, Austria125047 Moscow, RussiaAbstract. We introduce the notion of a perfect process tree as a model for thefull propagation of information in metacomputation. Starting with constantpropagation we construct step-by-step the driving mechanism used in super-compilation which ensures the perfect propagation of information. The conceptof a simple supercompiler based on perfect driving coupled with a simplefolding strategy is explained. As an example we demonstrate that specializing anaive pattern matcher with respect to a fixed pattern obtains the efficiency of amatcher generated by the Knuth, Morris & Pratt algorithm.1IntroductionResearch in the field of program specialization extends the state-of-the-art in two directions: extending existing methods to new languages and improving the techniques for the specialization of programs. While the first goal can be stated clearly, the second goal is often expressed in rather vague terms such as ‘strength’ and ‘transformation depth’. Often new methods are introduced rather ad hoc and it is hard to see how much has been achieved and what the limitations are.How should one assess the quality of a program specialization method? Various criteria are conceivable. In this paper we propose the notion of a perfect process tree. The goal is to propagate ‘enough’ information to be able to prune all infeasible program branches at specialization time. Many existing methods, such as partial evaluation, develop imperfect process trees. This should not be taken as a negative statement, but — on the contrary — as a motivation for improving specialization methods further. We present different methods for spe-cializing a simple programming language with tree-structured data, called S-Graph. Starting from constant propagation we develop step-by-step a driving mechanism which ensures the perfect propagation of information along the specialized program branches. The use of perfect driving is shown by introducing a supercompiler with a simple folding strategy. As an example we demonstrate that specializing a naive pattern matcher with respect to a fixed pattern obtains the efficiency of a matcher generated by the Knuth, Morris & Pratt algorithm.2Background: Process-Based TransformationIn this section we review Turchin’s concept of process-based program transformation. A program p∈Pgm, p : Data→Data, given data d∈Data, defines the behavior of a machine: a computation process. A computation process is a potentially infinite sequence of states and transitions. A state may contain a program point and a store. Each state and transition in a deterministic computation process is fully defined: process(p,d) = s1→s2→s3→… (This may be defined by some well-understood semantics such as operational or denotational semantics, omit-1Supported by the Austrian Science Foundation (FWF) under grant number J0780-PHY.Current address: DIKU, Dept. of Computer Science, University of Copenhagen, Universitetsparken 1,DK-2100 Copenhagen Ø, Denmark. Email: glueck@diku.dk.2Supported by the Russian Foundation for Fundamental Research under grant number 93-12-628 and inpart by the ‘Österreichische Forschungsgemeinschaft’ under grant number 06/1789.Email: And.Klimov@refal.msk.su.ted here). The set of processes, P = {process(p,d) | d∈Data}, captures the semantics of a pro-gram as a whole. But how can one describe and manipulate the set of processes constructively? Process Graph. For process-based program transformation one instead uses a process graph. A process graph is used to describe and manipulate the set of computation processes. In the following this will be done assuming given a subset of p’s initial states (this is the connection to program specialization).Each node in a process graph represents a set of states and is called a configuration. Any program graph has a single root node, called initial configuration, representing a subset of program p’s initial states. A configuration c which branches to two or more configurations in a process graph represents a conditional transition from one set of program states to two or more sets of program states. An edge originating from a branching configuration c is labeled with a test on c. Abstractly this could be thought of as selecting the set of states causing control to fol-low this edge (a configuration which branches usually corresponds to a test in the program p). Definition. A process graph g is correct for a program p with respect to an initial configuration ci iff(i) the process graph is complete: if program p takes state s into s' in a computation originating from an initial state si ∈ci, and if s is represented by configuration c, then there is a transition from c to c' in the process graph of p such that c' includes s'.(ii) the nodes branched to from a branching configuration c are uniquely determined by the tests on c (the configuration c is divided into disjoint sets of states following the corresponding branches).In general, a program p may have many different correct process graphs. A specific computation process follows a unique walk — a sequence of nodes and edges — in the process graph for program p. Because of the two requirements above, any computation process of p corresponds to exactly one walk in a correct process graph for program p (the requirement (i) says there is at least one walk, and (ii) says there is at most one walk). A process graph g for a program p is a model of p’s computational behavior for a given set of initial states ci. In the following we refer to process graphs when we mean correct process graphs.Graph Developers. How can one construct a process graph for a program and an initial config-uration? Supercompilation [22,24] uses two methods for graph development: driving and folding.Driving. This is a general method for constructing a (potentially infinite) process tree (a process graph that happens to be a tree) by step-wise exploring all possible computations of a program p starting from an initial configuration ci [20,22,24]. At any point during driving one has a perhaps incomplete process tree and a way to extend the process tree by adding a new node. Driving follows all possible computation processes starting from an initial configuration ci and continues until every leaf of the process tree represents only terminal states. Driving covers the activities of specializing and unfolding in partial evaluation.Folding. The ultimate goal is to construct a finite process graph for a program p and an initial configuration ci. At any point during driving one may, instead of extending the process graph by driving, try to fold new transitions back into old graph configurations. Folding may include adding a new edge back from a non-terminal configuration N to another configuration M in the process graph, or merging two ‘close enough’ configurations N and M: given a configuration M with a path to N, one may replace the edges originating from M and create a new edge instead to a generalized configuration M' representing a superset of the states represented by M and N. Given a finite process graph g that is correct for program p and the set of initial states ci, one may then construct a new program q from g (this is easy to achieve in practice). We will require that g will be correct for q, and q will be functionally equivalent to p with respect to the set of initial states ci. Our aim, of course, is to make q more efficient than the original program p.3Perfect Process GraphHow can one assess the ‘quality’ of a process graph? Clearly, if there is some edge in a process graph which is not used by any computation process, the process graph can’t be considered as an ‘optimal’ model of the program’s computational behavior. That is, the process graph contains at least one edge for which no initial state exists to follow it. We say that the more infeasible walks exist in a process tree, the worse is the process graph.Definition. A walk w in a process graph g is feasible if at least one initial state exists which follows w.Definition. A node n in a process graph g is feasible if it belongs at least to one feasible walk w in g.Definition. A process graph is perfect if all its walks are feasible.Infeasible walks not only increases the size of a process graph, but also reduces the efficiency of feasible walks. Consider the last feasible node in an otherwise infeasible walk. Since the node is feasible, at least one feasible walk goes through it. Since the infeasible walk goes through it as well, the node is a branching node: one branch is feasible, another is infeasible. Each branching has several conditions which have to be tested. This is extra work. Thus infeasible branches in-troduce additional tests and thereby degrade the efficiency of feasible walks. The more interpre-tive an algorithm is, the less perfect its process graph [23].Example. Consider the following fragment of a graph (or the program represented by it — we will not distinguish here). The branches 'B and 'C are in feasible, and the tests EQA?2 and EQA?3 are redundant. There exists no initial state which follows the branches 'B and 'C.(IF (EQA?1 x '5)(IF (EQA?2 x '5) 'A 'B)(IF (EQA?3 x '5) 'C 'D))Perfect Graph Developers. How can one construct a perfect process graph for a program and a given initial configuration? Unfortunately, no algorithm exists that could transform any program p into an equivalent finite, perfect process graph (formally proven in [22]). That is, one can not build a perfect graph developer. However, perfect tree developers for ‘well-formed’ languages exist which develop perfect process trees for an arbitrary program p and an initial configuration ci. This is the case with the programming language presented in this paper. While the problem of perfect graph development cannot be solved in general, perfect tree development may be achieved. This is the main motivation for studying it.Construction guideline. (1) Start by devising a perfect tree developer; (2) make the correspond-ing graph developer as ‘perfect’ as possible, without sacrificing computability and termination. Why do we consider this as essential? Because the first goal may be achieved constructively for ‘well-formed’ languages, while the second goal can not be achieved in general. Another aspect: one can not expect to make ‘clever’ folding decisions based on insufficient information ob-tained by driving. Once a perfect driving mechanism is constructed, it is a solid ground for the further refinement of a graph developer. As a result, the problem of approximation in the devel-opment of process graphs is driven into one corner: folding.4The Language S-GraphThe choice of the subject language is crucial for writing concise and clear algorithms for program specialization. In order to concentrate on the essence of driving, we limit ourselves to a pure symbol manipulation language, called S-Graph. As the name implies, one can think of S-Graph programs as being textual representations of graphs.S-Graph is a first-order, functional programming language restricted to tail-recursion. The only data type are well-founded, i.e. non-circular, S-expressions (as known from Lisp). Despite its simplicity the language is complete and universal. The semantics of the language is straightforward. A program is a list of function definitions where each function body is anProg::=[Def*]Def::=(DEFINE Fname [Var*] Tree)Tree::=(LET Var Exp Tree) | (CALL Fname [Arg*])(IF Cntr Tree Tree) | ExpCntr::=(CONS? Arg Var Var) | (EQA? Arg Arg)Exp::=Arg | (CONS Exp Exp)Arg::=Val | VarVal::=(ATOM Atom)Var::=(VAR Name)Fig. 1. Syntax of flat S-Graph.expression built from a few elements: conditionals IF, local bindings LET, function calls CALL, constructors CONS and atomic constants (drawn from an infinite set of symbols).Note the conditional in S-Graph: the test Cntr may update the environment. As in su per-compilation, we refer to such tests as contractions [24]. Two elementary contractions are sufficient for S-expressions:(EQA?x y)tests the equality of two atoms: x’s value and y’s value; if the arguments are non-atomic then the test is undefined.(CONS?x h t)if the value of x is a pair (CONS a b), then the test succeeds and the vari-able h is bound to a and the variable t to b; otherwise, the test is false. The arguments of function calls and contractions are restricted to variables and atomic constants in order to limit the number of places where values may be constructed. Because there are no nested function calls, we call this variant of the language flat (i.e. it corresponds to a flow-chart language). This is generalizable to nested function calls at the cost of more complex driving algorithms. In the following we will refer to the flat variant of the language simply as S-Graph. Example. String pattern matching is a typical problem to which various specialization methods have been applied. The subject program is a naive pattern matcher which checks whether a string p (the pattern) occurs within another string s. The matcher is fair ly simple: it returns 'SUCCESS if p occurs in s, 'FAILURE otherwise. The function LOOP compares the pattern with the beginning of the string. If the comparison fails the first element of the string is cut off and the function tries to match the remaining string against the pattern. This strategy is not optimal because the same elements in the string may be tested several times. In case of a mismatch the string is shifted by one and no further information is used for advancing in the string.Syntactic sugar: we write 'Atom as shorthand for (ATOM Atom), and lowercase identifiers as shorthand for (VAR Name).(DEFINE MATCH [p s](CALL LOOP [p s p s])) ; initialize loop(DEFINE LOOP [p s pp ss](IF (CONS? p phead ptail)(IF (CONS? s shead stail)(IF (EQA? phead shead)(CALL LOOP [ptail stail pp ss]) ; continue(CALL NEXT [pp ss])) ; shift string'FAILURE)'SUCCESS))(DEFINE NEXT [p s](IF (CONS? s shead stail)(CALL LOOP [p stail p stail]) ; restart loop'FAILURE))Fig. 2. Naive string matcher in S-Graph.Interpretive Definition. The semantics of S-Graph is defined by an interpretive definition (this will be the starting point for defining driving). In order to write the interpreter in a concise way we use some shorthand notations (the syntax is Haskell-like, the semantics is call-by-value): [v1a c1,…,v n a c n]an environment consisting of a list of variables bindings,e&[…]the function & updates the environment e with the list of variable bindings […], x/e the function / substitutes all variables in the expression x by the values given by the bindings in the environment e.The parameter containing the text of the interpreted program is omitted. The function mkEnv builds a new environment from a list of variables vs, a list of arguments as and an environment e; the function getDef returns the definition of a function given its name. The evaluation of an expression Exp does not ‘compute’ anything, it can only build up a structure. Note that a is a sugared version of a constructor.State. A computation state in S-Graph is fully defined by the current program point and the cur-rent environment. Variables originating from a program are called program variables (p-vari-ables) and are bound to constants in the environment. Since we consider only tail-recursive S-Graph programs, states include only one program point PPoint (such as IF, CALL, LET).State ::= PPoint EnvEnv ::= [Bind*]Bind ::= Var a ConstConst ::= ATOM Atom | CONS Const ConstFig. 4. State in S-Graph.5Information PropagationThe main hindrance in removing redundant tests is the lack of sufficient information about unknown values during program specialization. Starting from constant propagation we will develop step-by-step a driving mechanism for S-Graph which ensures the full propagation of information along the specialized program branches. Each step represents a different degree of information propagation.5.1Constant PropagationConstants are the most elementary form of information that can be propagated during program specialization. During specialization we do not deal with precise states, but with configurations representing sets of states. If we do not want to define perfect driving, then we may approximate the sets of states using covering configurations that represent larger sets of states. Configuration. A simple method for representing sets of states constructively uses expressions with free variables [24]. We introduce placeholders, called configuration variables (c-variables), which range over arbitrary constants. A p-variable in the environment of a configu-ration may be bound to a constant or to a c-variable (representing a ‘dynamic’ values). This is sufficient for constant propagation.Conf ::= PPoint CenvCenv ::= [Bind*]Bind ::= Var a CvalCval ::= Const | CVAR NameConst ::= ATOM Atom | CONS Const ConstFig. 5. Configuration for constant propagation.Driving. The first version is obtained by extending the S-Graph interpreter (Fig. 3) to propagate constants wherever possible and to produce residual code where the involved constants are ‘dynamic’ (Fig. 7).•If the result of evaluating an expression in a LET is not a constant then the p-variable is bound to a fresh c-variable (generated by the function newcvar).•If a contraction (EQA?, CONS?) can not be decided then both branches have to be driven (function cntr returns BOTH and an environment for each branch).This implements what is known as constant propagation, and corresponds to first-order partial evaluators based on constant propagation (e.g. [12]).Remark. It was noticed [12] that the test const? does not require the values proper and may be approximated in a separate pre-processing phase, called binding-time analysis. This granted the first self-application of a partial evaluator.5.2Partially Static StructuresA simple extension is the propagation of partially static structures in driving. This corresponds to first-order partial evaluators using partially static structures (e.g. [16,10,2,4]). This extension completes the construction of the function dev (in the following we will refine the handling of contractions during driving).Configuration. The description of a configuration is refined by replacing the definition of Cval.Conf ::= PPoint CenvCenv ::= [Bind*]Bind ::= Var a CvalCval ::= ATOM Atom | CVAR Name | CONS Cval CvalFig. 6. Configuration for partially static structures.Driving. The propagation of partially static structures is obtained by replacing the LET clause in function dev (Fig. 7) bydev (LET v x t) e = dev t (e&[v a x/e])Fig. 7. Constant propagation in S-Graph.5.3Propagation of Contraction InformationUsing constant propagation and partially static structures we are able to prune many infeasible branches, but not all (see example in Sect. 3).Configuration. In addition to the propagation of information by substitution (which we refer to as positive information, or assertions), we need to propagate the negation of this information (restrictions). We refine configurations by adding a list of restrictions on c-variables. A restriction of the form Rval#Rval states which values must not be equal. The restriction list may contain zero, one or more restrictions for each c-variable. Otherwise the configuration remains unchanged (Fig. 8). The a and # are sugared versions of constructors.Assertions. Propagating assertions requires updating the bindings of p-variables (corresponding to the well-known concept of unification). To capture the information that two unknown values are equal, we exploit the equality of c-variables. This is done by adding an extra case for equal c-variables to the EQA? clause. This goes beyond constant propagation. For example, the asser-tion x='5 is passed into the then-branch simply by replacing the c-variable cx by '5: ([x a cx],[]) (EQA? x '5)⇒then-branch:([x a'5],[])Conf::= PPoint CenvCenv::= [Bind*] [Restr*]Bind::= Var a CvalCval::= ATOM Atom | CVAR Name | CONS Cval CvalRestr::= Rval # RvalRval::= ATOM Atom | CVAR Name | CONSFig. 8. Configuration for perfect driving.Restrictions. Propagating restrictions requires updating a list of restrictions on c-variables. For example, the restriction x≠'5 is passed into the else-branch by adding a restriction on the c-variable cx:([x a cx],[]) (EQA? x '5)⇒else-branch:([x a cx],[cx#'5])The mechanism for checking restrictions is separated into the function both which is common for both contractions. If a contraction can not be decided using the list of p-variable bindings, then the list of restrictions is checked whether, possibly, a restriction exists which can be used to decide the contraction. In case such a restriction is found one can cut off the infeasible then-branch. This is done in function both by checking whether a substitution in the list of restrictions leads to a contradiction.Auxiliary functions. To simplify the definition we provide the following three functions for manipulating a configuration environment e: the function & adds new bindings to e, the function \ adds new restrictions to e, and the substitution / is extended to substitute variables in the configuration environment. In our case these functions may be defined as follows (the function ++ is list append, the function b2r converts a Bind into a Restr):(b,r) & bs = ((b++bs), r)(b,r) / bs = ((b/bs), (r/(b2r bs)))(b,r) \ bs = ( b, (r++(b2r bs)))During driving, c-variables are generated and may disappear as result of a substitution, leaving ‘dangling’ restrictions or tautologies, such as 'A#'B. They may be cleared out (e.g. after /), though this does not interfere with driving.Correctness and Perfectness. In order to verify S-Graph driving we have to prove that the mechanism is correct and perfect. The correctness of driving with respect to the interpretive definition of S-Graph ensures that the process tree contain at least all necessary (and maybe some infeasible) branches. The perfectness of driving guarantees that the process tree contains no infeasible branches. The existence of a perfectness theorem guarantees that driving propa-gates all information sufficient for pruning the process tree to its minimal size (omitted due to lack of space). This completes the task of defining perfect driving for S-Graph (Fig. 9). Remark. In order to keep the presented perfect tree developer as simple as possible and at the same time to preserve the termination properties of the subject programs in the residual pro-grams, we require that subject programs do not go ‘wrong’, i.e. atomic equality EQA? is not applied to non-atomic arguments. This may be guaranteed by adding a CONS? test for each non-atomic argument of EQA? in the subject programs.6Perfect Driving of a Naive Pattern MatcherBy specializing the naive pattern matcher (Fig. 2) with respect to a fixed pattern we show that perfect driving coupled with a simple folding strategy obtains the efficiency of a matcher generated by the Knuth, Morris & Pratt (KMP) algorithm [15]. This effect is achieved without the need for an ‘insightful reprogramming’ of the naive matcher as necessary for partial evaluation [5,11]. The complexity of the specialized algorithm is O(n), where n is the length of the string. The naive algorithm has complexity O(m.n), where m is the length of the pattern.Folding. At any point during driving one has a way to examine a non-terminal configuration and to decide to do one of: (i) fold the current configuration into an existing configuration; (ii) drive the configuration further. Two questions are relevant for folding:1) Which program points do we consider for folding?2) What is the criterion for folding?It is sufficient to couple perfect driving with folding of identical configurations for obtaining efficient matchers from a naive pattern matcher and a given pattern. Driving can be coupled with more sophisticated folding strategies, but this is beyond the scope of this paper (and not needed for the example). Folding of identical configurations answers the questions as follows:1) Dynamic conditionals are considered for folding.2) Two configurations represent the same set of states.The method of dynamic conditionals is a well-known technique in program specialization [22,3]: only those program points are considered for folding which introduce a branching (the conditional can not be decided, it is ‘dynamic’).When the descriptions of two configurations are identical (i.e. contain the same bindings and restrictions, modulo variable renaming) they represent the same set of states and one may, instead of continuing driving, add a new transition back from the current configuration into the old configuration.(DEFINE F1 [s](IF (CONS? s shead-1 stail-2)(IF (EQA? shead-1 'A)(IF (CONS? stail-2 shead-3 stail-4)(IF (EQA? shead-3 'A)(CALL F5 [stail-4])(CALL F1 [stail-4]))'FAILURE)(CALL F1 [stail-2]))'FAILURE))(DEFINE F5 [stail-4](IF (CONS? stail-4 shead-5 stail-6)(IF (EQA? shead-5 'B)'SUCCESS(IF (EQA? shead-5 'A)(CALL F5 [stail-6])(CALL F1 [stail-6])))'FAILURE))Fig. 10. KMP-like residual program for the pattern AAB.7Related WorkThe principles of driving were first formulated in the early seventies by Turchin [20,21] and further developed in the eighties [22,24]. From its very inception, supercompilation has been tied to a specific programming language, called Refal [24]. Applications of supercompilation include, among others, program specialization, program inversion and theorem proving. Other related aspects have been investigated in [1,7,8,13,14,17,18,26]. The notion of perfect process graphs and perfect driving were introduced in [22,23].The language S-Graph is closely related to Turchin’s Refal graphs [25]. But due to S-Graph’s simpler data structure, untyped variables and only two elementary contractions, one may build rather clear and concise driving algorithms. In particular, there is only one way to compose and decompose S-expressions (as opposed to Refal data structures). There is a close relation between driving and the neighborhood analysis for S-Graph [1]. Another ‘graph-like’language representing decision trees, was used by Bondorf for the implementation of a self-applicable partial evaluator Treemix [2].Specializing a naive string matcher is a typical problem to which various methods of program manipulation have been applied. A partial evaluator can achieve the same non-trivial result after identifying static components in the naive matcher and reprogramming the subject program [5,11]. Clearly, doing this by using an “automatic insight” frees the user from performing such subtle tasks. Generalized Partial Computation (GPC), another principle for program specialization based on partial evaluation and theorem proving, achieves the same optimal version [6]. In its essence GPC is related to driving, but differs in the propagation of arbitrary predicates, assuming the use of a theorem prover. Disunification in GPC was considered in [19]. It is not surprising, that the same optimal pattern matcher can be achieved by a Refal supercompiler [9]. Note that we used only a small part of the supercompilation method-ology: perfect driving for a language with tree-structured data coupled with a simple folding strategy.。