【US20200099569A1】DATALINEAGESUMMARIZATION【专利】
根据数据分析的英语作文

根据数据分析的英语作文英文回答:Data analysis is the process of inspecting, cleaning, transforming, and modeling data with the goal ofdiscovering useful information, informing conclusions, and supporting decision-making. It involves various techniques and tools to extract valuable insights from raw data.Data analysis plays a crucial role in variousindustries and domains, including business, healthcare, finance, scientific research, and marketing. It enables organizations to make data-driven decisions, identifytrends and patterns, predict future outcomes, optimize processes, and improve overall performance.One of the key benefits of data analysis is its ability to uncover hidden patterns and trends in data. By analyzing large amounts of data, organizations can identify anomalies, correlations, and relationships that may not be evidentfrom a cursory examination. This information can provide valuable insights into customer behavior, market dynamics, or scientific phenomena.Another advantage of data analysis is its predictive power. By leveraging machine learning and statistical models, organizations can use historical data to predict future trends and outcomes. This capability allows businesses to make informed decisions, anticipate market shifts, and develop strategies to maximize their impact.Furthermore, data analysis helps organizations optimize their operations and processes. By identifying inefficiencies, bottlenecks, and areas for improvement, organizations can streamline their workflows, reduce costs, and enhance customer satisfaction.In addition, data analysis is essential for evidence-based decision-making. By providing concrete data and insights, organizations can support their decisions with objective evidence, rather than relying on intuition or subjective assumptions. This approach leads to moreinformed and rational decision-making processes.中文回答:数据分析是指对数据进行检验、清洗、转换和建模,以发现有价值的信息、得出结论并支持决策的流程。
数据分析 英语作文
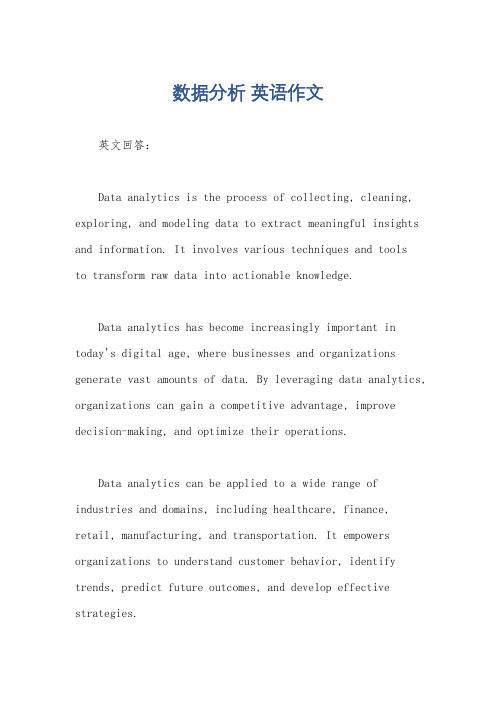
数据分析英语作文英文回答:Data analytics is the process of collecting, cleaning, exploring, and modeling data to extract meaningful insights and information. It involves various techniques and toolsto transform raw data into actionable knowledge.Data analytics has become increasingly important in today's digital age, where businesses and organizations generate vast amounts of data. By leveraging data analytics, organizations can gain a competitive advantage, improve decision-making, and optimize their operations.Data analytics can be applied to a wide range of industries and domains, including healthcare, finance, retail, manufacturing, and transportation. It empowers organizations to understand customer behavior, identify trends, predict future outcomes, and develop effective strategies.There are two main types of data analytics: descriptive and predictive. Descriptive analytics provides insightsinto historical data, while predictive analytics uses statistical models and machine learning algorithms to forecast future events and trends.Data analytics is a multidisciplinary field that draws on statistics, mathematics, computer science, and business knowledge. Data analysts use a variety of tools and techniques, including data visualization, statistical modeling, machine learning, and data mining.The process of data analytics typically involves the following steps:1. Data collection: Gathering data from various sources, such as databases, surveys, and sensors.2. Data cleaning: Removing errors, inconsistencies, and duplicate data.3. Data exploration: Analyzing the data to identify patterns, trends, and outliers.4. Data modeling: Developing statistical or machine learning models to predict future outcomes.5. Data visualization: Presenting the results of data analysis in a clear and understandable way.Data analytics has numerous benefits for organizations, including:Improved decision-making: Data-driven insights enable organizations to make more informed decisions.Increased efficiency: Data analytics can help organizations identify inefficiencies and optimize their processes.Enhanced customer satisfaction: By understanding customer behavior, organizations can improve their products and services.Competitive advantage: Data analytics can provide organizations with a competitive edge by identifying new opportunities and threats.中文回答:数据分析是一项收集、清洗、探索和建模数据的过程,以提取有意义的见解和信息。
summarize函数的用法

summarize函数的用法
summarize()是R语言中用于汇总统计数据的函数。
它通常用于对数据框(data frame)或列表(list)中的元素进行概括性统计。
以下是summarize()函数的基本语法:
summarize(x, ...)
其中,x是一个数据框或列表,...表示可选的参数。
在使用summarize()函数时,可以指定一个或多个变量来进行汇总统计。
以下是一个示例,展示如何使用summarize()函数对数据框中的变量进行汇总统计:
library(dplyr)
df <- data.frame(x = rnorm(100), y = rnorm(100))
df %>%
summarize(mean_x = mean(x), mean_y = mean(y), sd_x = sd(x), sd_y = sd(y))
在这个示例中,我们首先加载了dplyr库,然后创建了一个包含两个变量(x和y)的数据框。
接下来,我们使用dplyr库中的%>%操作符将数据框传递给summarize()函数。
在函数中,我们对x和y变量分别计算了均值和标准差,并将结果保存为新的数据框。
注意,在使用summarize()函数时,我们可以通过指定不同的变量来计算不同的统计量。
这使得我们可以轻松地对数据进行概括性统计。
统计结果的英语作文
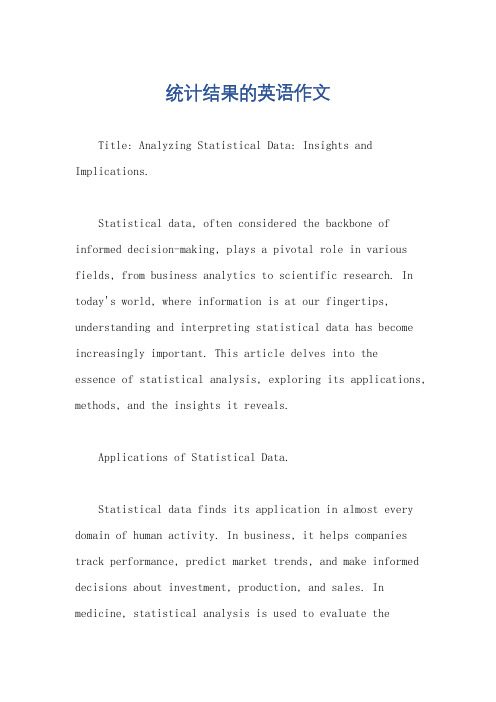
统计结果的英语作文Title: Analyzing Statistical Data: Insights and Implications.Statistical data, often considered the backbone of informed decision-making, plays a pivotal role in various fields, from business analytics to scientific research. In today's world, where information is at our fingertips, understanding and interpreting statistical data has become increasingly important. This article delves into the essence of statistical analysis, exploring its applications, methods, and the insights it reveals.Applications of Statistical Data.Statistical data finds its application in almost every domain of human activity. In business, it helps companies track performance, predict market trends, and make informed decisions about investment, production, and sales. In medicine, statistical analysis is used to evaluate theeffectiveness of treatments, understand disease patterns, and predict health outcomes. In social sciences, it aids in understanding population behaviors, trends, and patterns.Methods of Statistical Analysis.Statistical analysis involves a range of techniques and methods to process and interpret data. Descriptive statistics, for instance, summarize data through measures like mean, median, mode, and standard deviation, providing a snapshot of the data's central tendency and dispersion. Inferential statistics, on the other hand, allows researchers to draw conclusions about a population based on a sample. Techniques such as regression analysis, ANOVA, and chi-square tests are commonly used to test hypotheses and identify relationships between variables.Insights from Statistical Data.Statistical data, when analyzed correctly, can yield profound insights. For instance, in business, it can reveal patterns in customer behavior, market trends, and productperformance. This information can be used to refine marketing strategies, improve product quality, and increase customer satisfaction. In the medical field, statistical analysis can help identify risk factors for diseases, predict patient outcomes, and evaluate the effectiveness of new treatments.Challenges and Limitations.While statistical data can provide valuable insights,it also has its limitations. One of the main challenges is ensuring data quality. Inaccuracies, biases, and incomplete data can lead to misleading conclusions. Additionally, statistical analysis often assumes a certain level of randomness and normality in data, which may not always be the case. Furthermore, statistical significance does not always translate to practical significance, meaning a finding may be statistically significant but may not have a meaningful impact in real-world scenarios.Conclusion.Statistical data, when interpreted correctly, can provide valuable insights across various fields. It helps us understand patterns, trends, and relationships in data, enabling us to make informed decisions and predictions. However, it is important to remember that statistical analysis has its limitations, and it should always be used in conjunction with other forms of evidence and expertise. By harnessing the power of statistical data, we can gain a deeper understanding of the world and make more informed and effective decisions.。
data analysis例句
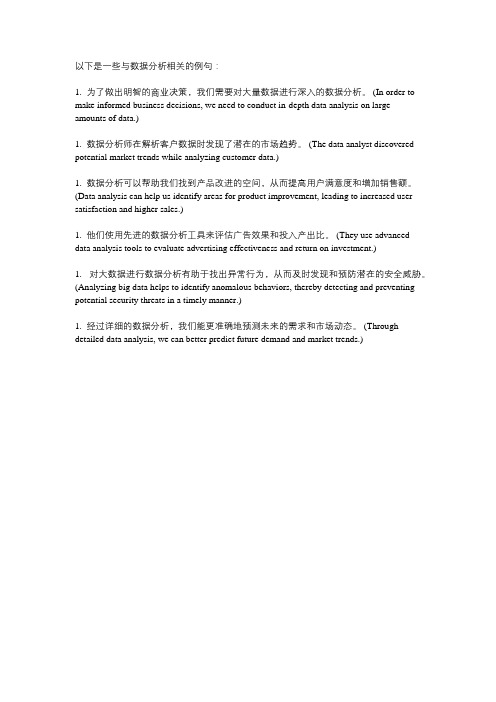
以下是一些与数据分析相关的例句:1. 为了做出明智的商业决策,我们需要对大量数据进行深入的数据分析。
(In order to make informed business decisions, we need to conduct in-depth data analysis on large amounts of data.)1. 数据分析师在解析客户数据时发现了潜在的市场趋势。
(The data analyst discovered potential market trends while analyzing customer data.)1. 数据分析可以帮助我们找到产品改进的空间,从而提高用户满意度和增加销售额。
(Data analysis can help us identify areas for product improvement, leading to increased user satisfaction and higher sales.)1. 他们使用先进的数据分析工具来评估广告效果和投入产出比。
(They use advanced data analysis tools to evaluate advertising effectiveness and return on investment.)1. 对大数据进行数据分析有助于找出异常行为,从而及时发现和预防潜在的安全威胁。
(Analyzing big data helps to identify anomalous behaviors, thereby detecting and preventing potential security threats in a timely manner.)1. 经过详细的数据分析,我们能更准确地预测未来的需求和市场动态。
(Through detailed data analysis, we can better predict future demand and market trends.)。
summary measures 翻 译

Summary measures,也称为总结性统计量,是描述数据分布和集中趋势的一组统计量。
它们是一组能够帮助人们了解数据中心位置和变异程度的指标。
summary measures包括均值、中位数、众数、标准差、方差等。
这些统计量能够帮助人们更好地理解和解释数据的特征,从而更好地进行数据分析和决策。
1. 均值均值是一组数据的所有数值之和除以数据的个数。
均值是描述数据集中趋势的一种方法,它能够代表一组数据的中心位置。
均值的计算公式为:均值 = 总和 / 数据个数2. 中位数中位数是一组数据按照大小顺序排列后位于中间位置的数值。
如果数据个数为偶数,中位数是中间两个数的平均值。
中位数能够更准确地表示数据的中心位置,尤其对于存在特殊值的数据集来说,中位数的稳健性更好。
3. 众数众数是一组数据中出现次数最多的数值。
众数是描述数据中变化趋势的一种统计量,它能够表示数据中的集中现象和典型值。
4. 标准差标准差是一组数据偏离均值的平均距离。
标准差是描述数据变异程度的一种指标,它越大表示数据越分散,标准差越小表示数据越集中。
5. 方差方差是一组数据离均值的偏差平方的平均数。
方差也是描述数据变异程度的一种指标,它是标准差的平方,能够衡量数据的离散程度。
summary measures在数据分析中起着非常重要的作用,它们能够帮助人们更好地理解和描述数据的特征,从而更准确地进行数据分析和预测。
在实际应用中,人们常常根据summary measures对数据进行可视化和摘要,从而更好地从数据中发现规律和提取信息,为决策提供依据和支持。
对summary measures的理解和应用是统计学和数据分析的基础,也是各行各业工作者必备的技能之一。
summary measures 续写为了更好地理解和应用summary measures,在实际数据分析中,我们需要深入了解每个summary measures的特点、适用范围以及计算方法。
summarizecolumns函数后对某列求和
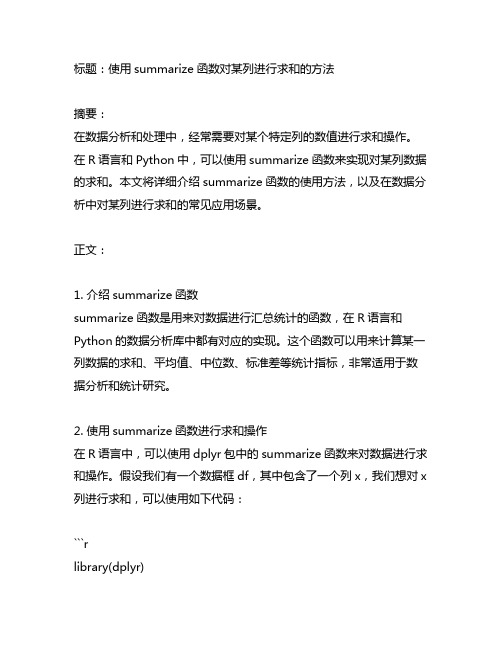
标题:使用summarize函数对某列进行求和的方法摘要:在数据分析和处理中,经常需要对某个特定列的数值进行求和操作。
在R语言和Python中,可以使用summarize函数来实现对某列数据的求和。
本文将详细介绍summarize函数的使用方法,以及在数据分析中对某列进行求和的常见应用场景。
正文:1. 介绍summarize函数summarize函数是用来对数据进行汇总统计的函数,在R语言和Python的数据分析库中都有对应的实现。
这个函数可以用来计算某一列数据的求和、平均值、中位数、标准差等统计指标,非常适用于数据分析和统计研究。
2. 使用summarize函数进行求和操作在R语言中,可以使用dplyr包中的summarize函数来对数据进行求和操作。
假设我们有一个数据框df,其中包含了一个列x,我们想对x 列进行求和,可以使用如下代码:```rlibrary(dplyr)summarize(df, sum_x = sum(x))```上面的代码将对数据框df中的x列进行求和,并将结果存储在sum_x 中。
通过这种方式,我们可以方便地对数据进行求和操作。
在Python的pandas库中,也有类似的功能可以实现。
可以使用groupby函数将数据按照某一列进行分组,然后使用sum函数对分组后的数据进行求和。
具体代码如下:```pythonimport pandas as pddf.groupby('col_name').agg({'target_col': 'sum'})```上面的代码将数据按照col_name列进行分组,并对target_col列进行求和。
通过这种方式,我们可以实现对某列数据的求和操作。
3. 汇总统计的应用场景对某一列数据进行求和是数据分析中很常见的操作。
比如在财务报表分析中,我们需要对某个指标的总和进行统计;在销售数据分析中,我们需要计算某个产品的总销售额;在生产管理中,我们需要对某个产品的总产量进行统计等等。
Data-Purpose Algebra Modeling Data Usage Policies
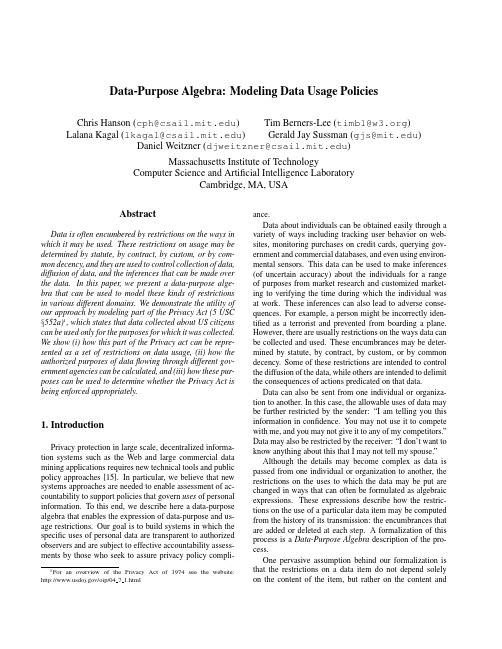
Data-Purpose Algebra:Modeling Data Usage Policies Chris Hanson(cph@)Tim Berners-Lee(timbl@) Lalana Kagal(lkagal@)Gerald Jay Sussman(gjs@) Daniel Weitzner(djweitzner@)Massachusetts Institute of TechnologyComputer Science and Artificial Intelligence LaboratoryCambridge,MA,USAAbstractData is often encumbered by restrictions on the ways in which it may be used.These restrictions on usage may be determined by statute,by contract,by custom,or by com-mon decency,and they are used to control collection of data, diffusion of data,and the inferences that can be made over the data.In this paper,we present a data-purpose alge-bra that can be used to model these kinds of restrictions in various different domains.We demonstrate the utility of our approach by modeling part of the Privacy Act(5USC §552a)1,which states that data collected about US citizens can be used only for the purposes for which it was collected. We show(i)how this part of the Privacy act can be repre-sented as a set of restrictions on data usage,(ii)how the authorized purposes of dataflowing through different gov-ernment agencies can be calculated,and(iii)how these pur-poses can be used to determine whether the Privacy Act is being enforced appropriately.1.IntroductionPrivacy protection in large scale,decentralized informa-tion systems such as the Web and large commercial data mining applications requires new technical tools and public policy approaches[15].In particular,we believe that new systems approaches are needed to enable assessment of ac-countability to support policies that govern uses of personal information.To this end,we describe here a data-purpose algebra that enables the expression of data-purpose and us-age restrictions.Our goal is to build systems in which the specific uses of personal data are transparent to authorized observers and are subject to effective accountability assess-ments by those who seek to assure privacy policy compli-1For an overview of the Privacy Act of1974see the website: /oip/0471.html ance.Data about individuals can be obtained easily through a variety of ways including tracking user behavior on web-sites,monitoring purchases on credit cards,querying gov-ernment and commercial databases,and even using environ-mental sensors.This data can be used to make inferences (of uncertain accuracy)about the individuals for a range of purposes from market research and customized market-ing to verifying the time during which the individual was at work.These inferences can also lead to adverse conse-quences.For example,a person might be incorrectly iden-tified as a terrorist and prevented from boarding a plane. However,there are usually restrictions on the ways data can be collected and used.These encumbrances may be deter-mined by statute,by contract,by custom,or by common decency.Some of these restrictions are intended to control the diffusion of the data,while others are intended to delimit the consequences of actions predicated on that data.Data can also be sent from one individual or organiza-tion to another.In this case,the allowable uses of data may be further restricted by the sender:“I am telling you this information in confidence.You may not use it to compete with me,and you may not give it to any of my competitors.”Data may also be restricted by the receiver:“I don’t want to know anything about this that I may not tell my spouse.”Although the details may become complex as data is passed from one individual or organization to another,the restrictions on the uses to which the data may be put are changed in ways that can often be formulated as algebraic expressions.These expressions describe how the restric-tions on the use of a particular data item may be computed from the history of its transmission:the encumbrances that are added or deleted at each step.A formalization of this process is a Data-Purpose Algebra description of the pro-cess.One pervasive assumption behind our formalization is that the restrictions on a data item do not depend solely on the content of the item,but rather on the content andits annotated provenance.For example,a law-enforcement official may not act on improperly obtained evidence,but if the same information were redundantly obtained through lawful channels the official may act,assuming that the in-dependence meets certain constitutional requirements.2.Data-Purpose AlgebraTo formally describe the ways that the use of data may be restricted and the way in which the restrictions are trans-formed as the data is processed and passed from one agent to another,we annotate each data item with extra informa-tion.Each data item i,in addition to its content q=QD(i),is annotated with its agent a=AD(i),a category k=KD (i),and a set of purposes p=PD(i)for which it canbe used.An item is constructed from its content,agent, category,and purposes i=I(q,a,k,p).The agent is the producer of this data.The category is a set of data items containing this particular item.This set may be named but is not likely to be enumerated;for example a typical legal category is“US citizen.”The set of purposes is explicit;a typical purpose in the set of purposes is“criminal law en-forcement.”A data item i may be processed by some agent a′to pro-duce a new data item.(Seefigure1.)The new data item has the same kinds of annotations as the original one,but the process generates new content and new annotations as functions of the original.The functions are specific to the kind of process performed by the agent.For example,medical data about an identified person may be severely restricted as to its allowable uses.But it may be anonymized for use in the education of medical doc-tors.In such a case,the allowed purposes of the anonymized data may be wider than those of the original data,and the category of the data will be different.On the other hand, when a person enters a medical establishment for treatment a record is made of the patient’s name,address,and date of birth.This data itself typically has few usage restrictions, but the fact of it appearing in a medical record adds restric-tions required by the HIPAA law.An agent may combine data from multiple agents to pro-duce new data.(Seefigure2.)In this case the functions may be considerably more complex.For example,a person at the medical office may use a public source,such as a telephone directory,to verify the recorded telephone number of a pa-tient.This process combines highly restricted information from a medical record with unrestricted public information, but the result remains restricted.3.An Example Formalization:Privacy ActIn the illustration that follows we consider a simplified formulation of the rules for data passed among Systems of Records(SORs),specified by the associated Systems of Records Notices(SORNs),as defined by the Privacy Act(5 USC§552a).Let r be a data repository;if the repository is a SOR, it has an associated SORN n=N(r),which gives infor-mation about the permissible uses of the data in the SOR. The SORN specifies input conditions:the allowed sources OS(n)from which data may be collected,the data cate-gories KS(n)that may be collected,and the purposes PS(n) for which data that is collected may be used.It also speci-fies a set of routine uses U(n)for data extracted from that SOR.Each routine use u∈U(n)specifies a set of possible re-cipient organizations OR(u),categories of data KR(u)that may be transferred to those organizations,and the set of au-thorized purposes PR(u)for which the specified recipient organizations may use the given data.Any particular re-cipient r1may be a sub-organization of a possible recipient organization r2specified in a SORN.This(non-strict)rela-tion is notated r1 r2.Likewise,any particular category k1may be a subset of a category k2specified in a SORN. This relation is notated k1⊆k2.The purposes allowed for data i that has been transferred from a SOR s to a SOR r depend on the purposes that came with the data and the input conditions on the SORN for r. So,if s is not one of the allowed sources or the category of the data is not one of the allowed categories the data may not be used for any purpose:RIN(i,s,r)=PS(r)if∃o((o∈OS(N(r)))∧(s o))∧∃k((k∈KS(N(r)))∧(KD(i)⊆k))={}otherwiseThe set of applicable routine uses A(i,s,r)for transfer of data item i from a SOR s to a recipient r is just the set of those entries for which the recipient is allowed by theSORN for s and for which the category of the data KD(i)isin the allowed categories KR(u)for that routine use u:A(i,s,r)={u∈U(N(s))|∃o((o∈OR(u))∧(r o))∧∃k((k∈KR(u))∧(KD(i)⊆k))}The restriction on authorized purposes of a transfer from a source to a recipient is that the purposes must be autho-rized by one or more of the applicable routine uses.ROUT(i,s,r)= u∈A(i,s,r)P R(u)The authorized purposes Z(i,s,r)to which a recipient r may put a data item i extracted from a source s is then restricted to be those purposes particular to that data itemFigure1.Unary Process:A data item i may be processed by some agent a′to produce a new data item.The new content is some function Q(QD(i))of the given content.The agent of the new dataitem is a′,the new category is a function K(KD(i))of the given category,and the allowed purposes ofthe new data item is a more complex function P(PD (i),AD(i),a′,KD(i))that may depend on the originalpurposes,the agents,and the category of the original data. that are also allowed by the authorized purposes specifiedin the SORN:Z(i,s,r)=PD (i)∩RIN(i,s,r)∩ROUT(i,s,r)So Z(i,s,r)is the set of purposes of the new item held by the recipient r with the content of the old item i held by the source s.The result of a transfer process AXFERof an item i from a source s to a recipient r is a new item:I(QD (i),AXFER,KD(i),Z(i,s,r))4.ImplementationWe are constructing a system[15]that uses the data-purpose algebra to derive usage purposes for data,and con-sequently analyze usage of the data by government agen-cies.Our system expects as input the historical log of data collection,analysis,and transfer between individuals and government agencies.Based upon current government ef-forts,we presume that this log as well as case activities will exist in XML[2].We plan to automatically convert the XML transactional data into the Resource Description Framework(RDF)[9];annotate the transactional data with agent,category,and purposes as described in this paper;and then encode the derivation steps in the Proof Markup Lan-guage[5],thereby providing an interoperable justification representation for explanation and accountability.We are also defining notices(in RDF)for the Systems of Records that appear in the transaction log.In our hypothetical sce-nario,a traveler named John Doe from New York boards a flight in New York and sets in motion a chain of inferences (some of which are factually incorrect and some of which are reached in violation of the Privacy Act)that generates a series of adverse consequences for him.A transaction log that leads up to an adverse consequence—such as John Doe being arrested—is passed through the data-purpose algebra of the Privacy Act.This causes each data item in the log to be annotated with its au-thorized purposes,as described in the earlier section.A data item that has an empty list of purposes shows the point at which a policy violation occurred.All further uses of these data items without purposes,including inferences made,in the log are also invalid.We have built an implementation of the data-purpose al-gebra in Scheme[10].To illustrate how closely the program corresponds to the mathematics,figure3shows an imple-mentation of ROUT(i,s,r)and A(i,s,r)in Scheme.The overall correspondence between code and mathematics is quite close.In order to enable users to understand where and how the Privacy Act has been violated in a particular transaction log,we are developing a user interface for our system.The UI provides several views into the annotated log,including: (a)a time-line of events;(b)flow of data through the records systems;(c)transaction details;and(d)data-purpose calcu-lations.5.Related WorkOur algebra is closely related to work on privacy policy representation and enforcement.W3C’s P3P framework al-lows websites to publish their privacy policy,which can be matched against users’privacy preferences[16].Comple-mentary languages for defining privacy preferences such as APPEL[4]and XPREF[1]have also been defined.The P3P framework is specifically aimed at web privacy in terms ofFigure2.Binary Process:Two data items,i and j,may be processed by some agent a′′to producea new data item.The new content is some function Q(QD (i),QD(j))of the content of i and j.Theagent of the new data item is a′′and the new category is a function K(KD (i),KD(j))of the categoriesof i and j.The allowed purposes of the new data item is a complex function that may depend on the original purposes,the agents,and the categories of i and j.collecting,storing,and sharing user information and deals solely with cookies and clickstream data.In contrast,our data-purpose algebra has a broader scope and models re-strictions on how data can be used in general.While P3P is concerned with privacy protection issues for web users,EPAL1.1[8]specifies enterprise-level poli-cies for data objects in the enterprise.Like P3P,EPAL has a limited scope and does not provide a general model of data restrictions.Policy languages such as Extensible Access Control Markup Language(XACML)[7]and KAoS[13,14]are used to describe access control ing these lan-guages it is possible to define restrictions on actions that deal with specific data thereby enforcing restrictions on data usage.However,access control policies are enforced at the time of the user request,whereas our work is focused on accountability.Privacy approaches such as ContextBroker[3]and Se-mantic eWallet[6]are being developed for pervasive com-puting environments where the behavior of users may be monitored and used.These approaches enforce users’pri-vacy preferences by preventing their data from being used by or modifying the data available to context aware services.6.Future WorkThe example in this paper shows how to cover many kinds of formalizable requirements,such as those of the Pri-vacy Act.But there are harder rmal and im-plicit restrictions on data usage may result in contradictory conclusions about what purpose restrictions apply.Further work is required to define means by which these conflicting results can be resolved.Anonymization is often used to remove identifying char-acteristics of data.However,it has been shown that sets of“anonymized”data can often be combined to discover the identities of the parties[11].Is the combined data re-stricted?It depends on the laws.If the law requires that medical records are restricted,then that conclusion is in-dependent of how they are derived.On the other hand,it is possible to combine two restricted pieces of information to produce a less restricted deduction.For example,if the same information is available from two different sources, then the restriction on the combination may be relaxed to be the uses allowed by each source separately,or it may not,depending on the details.The data-purpose algebra is designed to compute al-lowed purposes based on how data is derived,but as in the case of re-constituted medical records,this is not al-ways sufficient.We believe that this model can be extended to handle such cases by adding content-dependent global rules,for example by using the category of a data item to indicate when such a rule should be applied.7.SummaryThe algebraic approach is well suited to modeling the allowable uses of information when the restrictions on that(define(restriction-on-output record source recipient) (rdf-set:union*(append-map ts:routine-use.purposes(applicable-routine-uses record source recipient)))) (define(applicable-routine-uses record source recipient) (keep-matching-items(ts:sorn.routine-uses(ts:sor.notice source)) (lambda(use)(and(there-exists?(ts:routine-use.recipients use)(lambda(recipients)(org-elt?recipient recipients)))(there-exists?(ts:data-record.categories record)(lambda(category)(there-exists?(ts:routine-use.categories use)(lambda(category*)(rdf-subset?category category*)))))))))Figure3.Scheme implementation of ROUT(i,s,r)and A(i,s,r).use are determined by the path by which the information is obtained,but it is not so good at dealing with restrictions that are time dependent or inherent in the content of the information,independent of the path.When formalized algebraically,computations are di-rectly representable as purely functional computer pro-grams.This makes it easy to verify that a program that implements the data-purpose algebraic computations is cor-rect.AcknowledgementsHal Abelson,Deborah McGuinness,and K.Krasnow Waterman read drafts of this paper and provided many use-ful and insightful comments.The work reported in this pa-per is supported by the US National Science Foundation Cy-bertrust(05-518)program.References[1]R.Agrawal,J.Kiernan,R.Srikant,and Y.Xu.XPref:Apreference language for works,48(5):809–827,2005.[2]T.Bray,J.Paoli, C.M.Sperberg-McQueen, E.Maler,and F.Yergeau.Extensible markup language(XML)1.0./TR/REC-xml/.[3]H.Chen,T.Finin,and A.Joshi.An intelligent broker forcontext-aware systems.In Adjunct Proceedings of Ubicomp 2003,Seattle,Washington,USA,October12–15,2003,Oct.2003.[4]L.Cranor,ngheinrich,and M.Marchiori.A P3P preference exchange language(APPEL)./TR/P3P-preferences/.[5]P.P.da Silva,D.L.McGuinness,and R.Fikes.A proofmarkup language for semantic web rmationSystems,31(4–5):381–395,June–July2006.[6] F.Gandon and N.Sadeh.Semantic web technologies to rec-oncile privacy and context awareness.Web Semantics Jour-nal,2004.[7]S.Godik and T.Moses.OASIS extensible access controlmarkup language(XACML).OASIS Committee Specifica-tion cs-xacml-specification-1.0,Nov.2002.[8]IBM.EPAL1.1./security/enterprise-privacy/epal/Specification/index.html,2003.[9] F.Manola and ler.RDF primer./TR/rdf-primer/.[10]G.L.Steele,Jr.and G.J.Sussman.The revised report onscheme,a dialect of lisp.MIT AI Memo452,Jan.1978. [11]L.Sweeney.Guaranteeing anonymity when sharing medicaldata,the datafly system.Journal of the American MedicalInformatics Association,1997.[12]U.S.Department of Justice and Department of HomelandSecurity.National information exchange model(NIEM)./.[13] zok,J.Bradshaw,P.Hayes,R.Jeffers,M.Johnson,S.Kulkarni,M.Breedy,J.Lott,and L.Bunch.DAML real-ity check:A case study of KAoS domain and policy ser-vices.In International Semantic Web Conference(ISWC03),Sanibel Island,Florida,2003.[14] zok,J.M.Bradshaw,R.Jeffers,M.Johnson,A.Tate,J.Dalton,and S.Aitken.Policy and contract managementfor semantic web services.In AAAI Spring Symposium,FirstInternational Semantic Web Services Symposium,2004. [15] D.Weitzner,H.Abelson,T.Berners-Lee, C.Hanson,J.Hendler,L.Kagal,D.McGuinness,G.Sussman,andK.K.Waterman.Transparent accountable inferencing forprivacy risk management.In The Semantic Web meets eGov-ernment,AAAI Spring Symposium,2006.[16]World Wide Web Consortium.Platform for privacy prefer-ences(P3P)project./P3P/.。