Empirical Semantics of Agent Communication in Open Systems
心理学名词——精选推荐
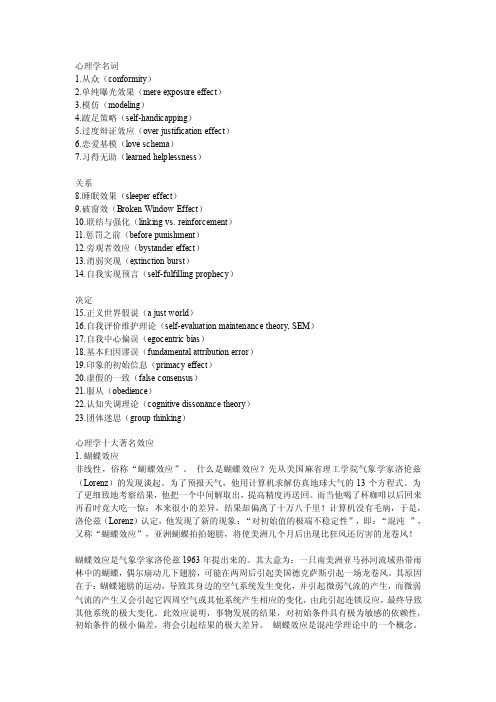
心理学名词1.从众(conformity)2.单纯曝光效果(mere exposure effect)3.模仿(modeling)4.跛足策略(self-handicapping)5.过度辩证效应(over justification effect)6.恋爱基模(love schema)7.习得无助(learned helplessness)关系8.睡眠效果(sleeper effect)9.破窗效(Broken Window Effect)10.联结与强化(linking vs. reinforcement)11.惩罚之前(before punishment)12.旁观者效应(bystander effect)13.消弱突现(extinction burst)14.自我实现预言(self-fulfilling prophecy)决定15.正义世界假说(a just world)16.自我评价维护理论(self-evaluation maintenance theory, SEM)17.自我中心偏误(egocentric bias)18.基本归因谬误(fundamental attribution error)19.印象的初始信息(primacy effect)20.虚假的一致(false consensus)21.服从(obedience)22.认知失调理论(cognitive dissonance theory)23.团体迷思(group thinking)心理学十大著名效应1.蝴蝶效应非线性,俗称“蝴蝶效应”。
什么是蝴蝶效应?先从美国麻省理工学院气象学家洛伦兹(Lorenz)的发现谈起。
为了预报天气,他用计算机求解仿真地球大气的13个方程式。
为了更细致地考察结果,他把一个中间解取出,提高精度再送回。
而当他喝了杯咖啡以后回来再看时竟大吃一惊:本来很小的差异,结果却偏离了十万八千里!计算机没有毛病,于是,洛伦兹(Lorenz)认定,他发现了新的现象:“对初始值的极端不稳定性”,即:“混沌”,又称“蝴蝶效应”,亚洲蝴蝶拍拍翅膀,将使美洲几个月后出现比狂风还厉害的龙卷风!蝴蝶效应是气象学家洛伦兹1963年提出来的。
社会研究方法名解(艾尔巴比)
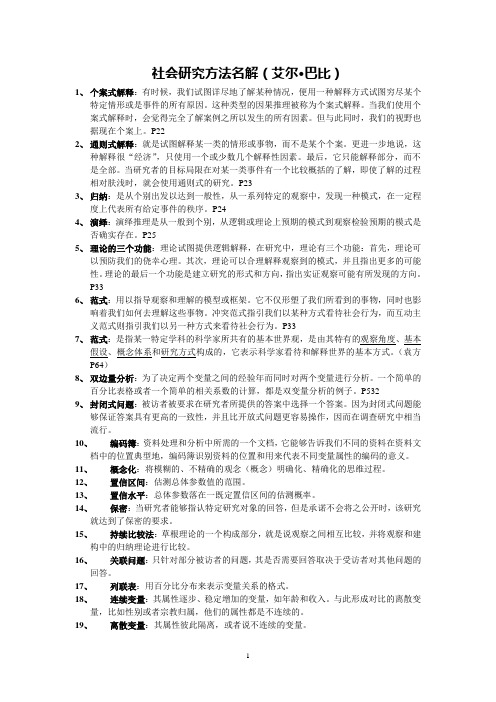
社会研究方法名解(艾尔·巴比)1、个案式解释:有时候,我们试图详尽地了解某种情况,便用一种解释方式试图穷尽某个特定情形或是事件的所有原因。
这种类型的因果推理被称为个案式解释。
当我们使用个案式解释时,会觉得完全了解案例之所以发生的所有因素。
但与此同时,我们的视野也据现在个案上。
P222、通则式解释:就是试图解释某一类的情形或事物,而不是某个个案。
更进一步地说,这种解释很“经济”,只使用一个或少数几个解释性因素。
最后,它只能解释部分,而不是全部。
当研究者的目标局限在对某一类事件有一个比较概括的了解,即使了解的过程相对肤浅时,就会使用通则式的研究。
P233、归纳:是从个别出发以达到一般性,从一系列特定的观察中,发现一种模式,在一定程度上代表所有给定事件的秩序。
P244、演绎:演绎推理是从一般到个别,从逻辑或理论上预期的模式到观察检验预期的模式是否确实存在。
P255、理论的三个功能:理论试图提供逻辑解释,在研究中,理论有三个功能:首先,理论可以预防我们的侥幸心理。
其次,理论可以合理解释观察到的模式,并且指出更多的可能性。
理论的最后一个功能是建立研究的形式和方向,指出实证观察可能有所发现的方向。
P336、范式:用以指导观察和理解的模型或框架。
它不仅形塑了我们所看到的事物,同时也影响着我们如何去理解这些事物。
冲突范式指引我们以某种方式看待社会行为,而互动主义范式则指引我们以另一种方式来看待社会行为。
P337、范式:是指某一特定学科的科学家所共有的基本世界观,是由其特有的观察角度、基本假设、概念体系和研究方式构成的,它表示科学家看待和解释世界的基本方式。
(袁方P64)8、双边量分析:为了决定两个变量之间的经验年而同时对两个变量进行分析。
一个简单的百分比表格或者一个简单的相关系数的计算,都是双变量分析的例子。
P5329、封闭式问题:被访者被要求在研究者所提供的答案中选择一个答案。
因为封闭式问题能够保证答案具有更高的一致性,并且比开放式问题更容易操作,因而在调查研究中相当流行。
Example-based metonymy recognition for proper nouns
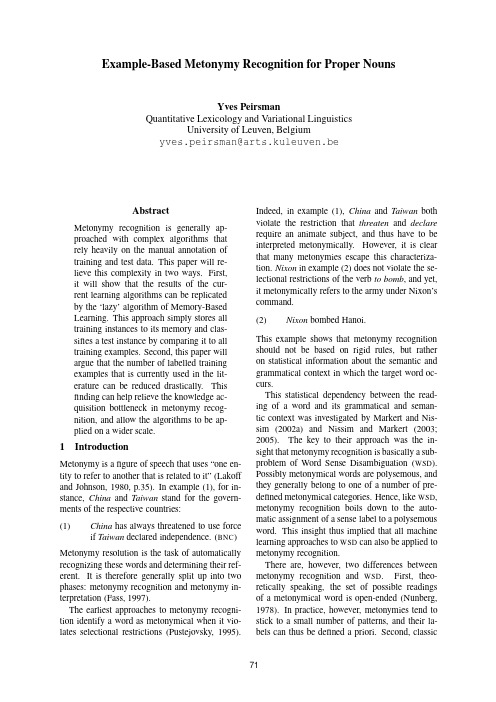
Example-Based Metonymy Recognition for Proper NounsYves PeirsmanQuantitative Lexicology and Variational LinguisticsUniversity of Leuven,Belgiumyves.peirsman@arts.kuleuven.beAbstractMetonymy recognition is generally ap-proached with complex algorithms thatrely heavily on the manual annotation oftraining and test data.This paper will re-lieve this complexity in two ways.First,it will show that the results of the cur-rent learning algorithms can be replicatedby the‘lazy’algorithm of Memory-BasedLearning.This approach simply stores alltraining instances to its memory and clas-sifies a test instance by comparing it to alltraining examples.Second,this paper willargue that the number of labelled trainingexamples that is currently used in the lit-erature can be reduced drastically.Thisfinding can help relieve the knowledge ac-quisition bottleneck in metonymy recog-nition,and allow the algorithms to be ap-plied on a wider scale.1IntroductionMetonymy is afigure of speech that uses“one en-tity to refer to another that is related to it”(Lakoff and Johnson,1980,p.35).In example(1),for in-stance,China and Taiwan stand for the govern-ments of the respective countries:(1)China has always threatened to use forceif Taiwan declared independence.(BNC) Metonymy resolution is the task of automatically recognizing these words and determining their ref-erent.It is therefore generally split up into two phases:metonymy recognition and metonymy in-terpretation(Fass,1997).The earliest approaches to metonymy recogni-tion identify a word as metonymical when it vio-lates selectional restrictions(Pustejovsky,1995).Indeed,in example(1),China and Taiwan both violate the restriction that threaten and declare require an animate subject,and thus have to be interpreted metonymically.However,it is clear that many metonymies escape this characteriza-tion.Nixon in example(2)does not violate the se-lectional restrictions of the verb to bomb,and yet, it metonymically refers to the army under Nixon’s command.(2)Nixon bombed Hanoi.This example shows that metonymy recognition should not be based on rigid rules,but rather on statistical information about the semantic and grammatical context in which the target word oc-curs.This statistical dependency between the read-ing of a word and its grammatical and seman-tic context was investigated by Markert and Nis-sim(2002a)and Nissim and Markert(2003; 2005).The key to their approach was the in-sight that metonymy recognition is basically a sub-problem of Word Sense Disambiguation(WSD). Possibly metonymical words are polysemous,and they generally belong to one of a number of pre-defined metonymical categories.Hence,like WSD, metonymy recognition boils down to the auto-matic assignment of a sense label to a polysemous word.This insight thus implied that all machine learning approaches to WSD can also be applied to metonymy recognition.There are,however,two differences between metonymy recognition and WSD.First,theo-retically speaking,the set of possible readings of a metonymical word is open-ended(Nunberg, 1978).In practice,however,metonymies tend to stick to a small number of patterns,and their la-bels can thus be defined a priori.Second,classic 71WSD algorithms take training instances of one par-ticular word as their input and then disambiguate test instances of the same word.By contrast,since all words of the same semantic class may undergo the same metonymical shifts,metonymy recogni-tion systems can be built for an entire semantic class instead of one particular word(Markert and Nissim,2002a).To this goal,Markert and Nissim extracted from the BNC a corpus of possibly metonymical words from two categories:country names (Markert and Nissim,2002b)and organization names(Nissim and Markert,2005).All these words were annotated with a semantic label —either literal or the metonymical cate-gory they belonged to.For the country names, Markert and Nissim distinguished between place-for-people,place-for-event and place-for-product.For the organi-zation names,the most frequent metonymies are organization-for-members and organization-for-product.In addition, Markert and Nissim used a label mixed for examples that had two readings,and othermet for examples that did not belong to any of the pre-defined metonymical patterns.For both categories,the results were promis-ing.The best algorithms returned an accuracy of 87%for the countries and of76%for the orga-nizations.Grammatical features,which gave the function of a possibly metonymical word and its head,proved indispensable for the accurate recog-nition of metonymies,but led to extremely low recall values,due to data sparseness.Therefore Nissim and Markert(2003)developed an algo-rithm that also relied on semantic information,and tested it on the mixed country data.This algo-rithm used Dekang Lin’s(1998)thesaurus of se-mantically similar words in order to search the training data for instances whose head was sim-ilar,and not just identical,to the test instances. Nissim and Markert(2003)showed that a combi-nation of semantic and grammatical information gave the most promising results(87%). However,Nissim and Markert’s(2003)ap-proach has two major disadvantages.Thefirst of these is its complexity:the best-performing al-gorithm requires smoothing,backing-off to gram-matical roles,iterative searches through clusters of semantically similar words,etc.In section2,I will therefore investigate if a metonymy recognition al-gorithm needs to be that computationally demand-ing.In particular,I will try and replicate Nissim and Markert’s results with the‘lazy’algorithm of Memory-Based Learning.The second disadvantage of Nissim and Mark-ert’s(2003)algorithms is their supervised nature. Because they rely so heavily on the manual an-notation of training and test data,an extension of the classifiers to more metonymical patterns is ex-tremely problematic.Yet,such an extension is es-sential for many tasks throughout thefield of Nat-ural Language Processing,particularly Machine Translation.This knowledge acquisition bottle-neck is a well-known problem in NLP,and many approaches have been developed to address it.One of these is active learning,or sample selection,a strategy that makes it possible to selectively an-notate those examples that are most helpful to the classifier.It has previously been applied to NLP tasks such as parsing(Hwa,2002;Osborne and Baldridge,2004)and Word Sense Disambiguation (Fujii et al.,1998).In section3,I will introduce active learning into thefield of metonymy recog-nition.2Example-based metonymy recognition As I have argued,Nissim and Markert’s(2003) approach to metonymy recognition is quite com-plex.I therefore wanted to see if this complexity can be dispensed with,and if it can be replaced with the much more simple algorithm of Memory-Based Learning.The advantages of Memory-Based Learning(MBL),which is implemented in the T i MBL classifier(Daelemans et al.,2004)1,are twofold.First,it is based on a plausible psycho-logical hypothesis of human learning.It holds that people interpret new examples of a phenom-enon by comparing them to“stored representa-tions of earlier experiences”(Daelemans et al., 2004,p.19).This contrasts to many other classi-fication algorithms,such as Naive Bayes,whose psychological validity is an object of heavy de-bate.Second,as a result of this learning hypothe-sis,an MBL classifier such as T i MBL eschews the formulation of complex rules or the computation of probabilities during its training phase.Instead it stores all training vectors to its memory,together with their labels.In the test phase,it computes the distance between the test vector and all these train-ing vectors,and simply returns the most frequentlabel of the most similar training examples.One of the most important challenges inMemory-Based Learning is adapting the algorithmto one’s data.This includesfinding a represen-tative seed set as well as determining the rightdistance measures.For my purposes,however, T i MBL’s default settings proved more than satis-factory.T i MBL implements the IB1and IB2algo-rithms that were presented in Aha et al.(1991),butadds a broad choice of distance measures.Its de-fault implementation of the IB1algorithm,whichis called IB1-IG in full(Daelemans and Van denBosch,1992),proved most successful in my ex-periments.It computes the distance between twovectors X and Y by adding up the weighted dis-tancesδbetween their corresponding feature val-ues x i and y i:∆(X,Y)=ni=1w iδ(x i,y i)(3)The most important element in this equation is theweight that is given to each feature.In IB1-IG,features are weighted by their Gain Ratio(equa-tion4),the division of the feature’s InformationGain by its split rmation Gain,the nu-merator in equation(4),“measures how much in-formation it[feature i]contributes to our knowl-edge of the correct class label[...]by comput-ing the difference in uncertainty(i.e.entropy)be-tween the situations without and with knowledgeof the value of that feature”(Daelemans et al.,2004,p.20).In order not“to overestimate the rel-evance of features with large numbers of values”(Daelemans et al.,2004,p.21),this InformationGain is then divided by the split info,the entropyof the feature values(equation5).In the followingequations,C is the set of class labels,H(C)is theentropy of that set,and V i is the set of values forfeature i.w i=H(C)− v∈V i P(v)×H(C|v)2This data is publicly available and can be downloadedfrom /mnissim/mascara.73P F86.6%49.5%N&M81.4%62.7%Table1:Results for the mixed country data.T i MBL:my T i MBL resultsN&M:Nissim and Markert’s(2003)results simple learning phase,T i MBL is able to replicate the results from Nissim and Markert(2003;2005). As table1shows,accuracy for the mixed coun-try data is almost identical to Nissim and Mark-ert’sfigure,and precision,recall and F-score for the metonymical class lie only slightly lower.3 T i MBL’s results for the Hungary data were simi-lar,and equally comparable to Markert and Nis-sim’s(Katja Markert,personal communication). Note,moreover,that these results were reached with grammatical information only,whereas Nis-sim and Markert’s(2003)algorithm relied on se-mantics as well.Next,table2indicates that T i MBL’s accuracy for the mixed organization data lies about1.5%be-low Nissim and Markert’s(2005)figure.This re-sult should be treated with caution,however.First, Nissim and Markert’s available organization data had not yet been annotated for grammatical fea-tures,and my annotation may slightly differ from theirs.Second,Nissim and Markert used several feature vectors for instances with more than one grammatical role andfiltered all mixed instances from the training set.A test instance was treated as mixed only when its several feature vectors were classified differently.My experiments,in contrast, were similar to those for the location data,in that each instance corresponded to one vector.Hence, the slightly lower performance of T i MBL is prob-ably due to differences between the two experi-ments.Thesefirst experiments thus demonstrate that Memory-Based Learning can give state-of-the-art performance in metonymy recognition.In this re-spect,it is important to stress that the results for the country data were reached without any se-mantic information,whereas Nissim and Mark-ert’s(2003)algorithm used Dekang Lin’s(1998) clusters of semantically similar words in order to deal with data sparseness.This fact,togetherAcc RT i MBL78.65%65.10%76.0%—Figure1:Accuracy learning curves for the mixed country data with and without semantic informa-tion.in more detail.4Asfigure1indicates,with re-spect to overall accuracy,semantic features have a negative influence:the learning curve with both features climbs much more slowly than that with only grammatical features.Hence,contrary to my expectations,grammatical features seem to allow a better generalization from a limited number of training instances.With respect to the F-score on the metonymical category infigure2,the differ-ences are much less outspoken.Both features give similar learning curves,but semantic features lead to a higherfinal F-score.In particular,the use of semantic features results in a lower precisionfig-ure,but a higher recall score.Semantic features thus cause the classifier to slightly overgeneralize from the metonymic training examples.There are two possible reasons for this inabil-ity of semantic information to improve the clas-sifier’s performance.First,WordNet’s synsets do not always map well to one of our semantic la-bels:many are rather broad and allow for several readings of the target word,while others are too specific to make generalization possible.Second, there is the predominance of prepositional phrases in our data.With their closed set of heads,the number of examples that benefits from semantic information about its head is actually rather small. Nevertheless,myfirst round of experiments has indicated that Memory-Based Learning is a sim-ple but robust approach to metonymy recogni-tion.It is able to replace current approaches that need smoothing or iterative searches through a the-saurus,with a simple,distance-based algorithm.Figure3:Accuracy learning curves for the coun-try data with random and maximum-distance se-lection of training examples.over all possible labels.The algorithm then picks those instances with the lowest confidence,since these will contain valuable information about the training set(and hopefully also the test set)that is still unknown to the system.One problem with Memory-Based Learning al-gorithms is that they do not directly output prob-abilities.Since they are example-based,they can only give the distances between the unlabelled in-stance and all labelled training instances.Never-theless,these distances can be used as a measure of certainty,too:we can assume that the system is most certain about the classification of test in-stances that lie very close to one or more of its training instances,and less certain about those that are further away.Therefore the selection function that minimizes the probability of the most likely label can intuitively be replaced by one that max-imizes the distance from the labelled training in-stances.However,figure3shows that for the mixed country instances,this function is not an option. Both learning curves give the results of an algo-rithm that starts withfifty random instances,and then iteratively adds ten new training instances to this initial seed set.The algorithm behind the solid curve chooses these instances randomly,whereas the one behind the dotted line selects those that are most distant from the labelled training exam-ples.In thefirst half of the learning process,both functions are equally successful;in the second the distance-based function performs better,but only slightly so.There are two reasons for this bad initial per-formance of the active learning function.First,it is not able to distinguish between informativeandFigure4:Accuracy learning curves for the coun-try data with random and maximum/minimum-distance selection of training examples. unusual training instances.This is because a large distance from the seed set simply means that the particular instance’s feature values are relatively unknown.This does not necessarily imply that the instance is informative to the classifier,how-ever.After all,it may be so unusual and so badly representative of the training(and test)set that the algorithm had better exclude it—something that is impossible on the basis of distances only.This bias towards outliers is a well-known disadvantage of many simple active learning algorithms.A sec-ond type of bias is due to the fact that the data has been annotated with a few features only.More par-ticularly,the present algorithm will keep adding instances whose head is not yet represented in the training set.This entails that it will put off adding instances whose function is pp,simply because other functions(subj,gen,...)have a wider variety in heads.Again,the result is a labelled set that is not very representative of the entire training set.There are,however,a few easy ways to increase the number of prototypical examples in the train-ing set.In a second run of experiments,I used an active learning function that added not only those instances that were most distant from the labelled training set,but also those that were closest to it. After a few test runs,I decided to add six distant and four close instances on each iteration.Figure4 shows that such a function is indeed fairly success-ful.Because it builds a labelled training set that is more representative of the test set,this algorithm clearly reduces the number of annotated instances that is needed to reach a given performance.Despite its success,this function is obviously not yet a sophisticated way of selecting good train-76Figure5:Accuracy learning curves for the organi-zation data with random and distance-based(AL) selection of training examples with a random seed set.ing examples.The selection of the initial seed set in particular can be improved upon:ideally,this seed set should take into account the overall dis-tribution of the training examples.Currently,the seeds are chosen randomly.Thisflaw in the al-gorithm becomes clear if it is applied to another data set:figure5shows that it does not outper-form random selection on the organization data, for instance.As I suggested,the selection of prototypical or representative instances as seeds can be used to make the present algorithm more robust.Again,it is possible to use distance measures to do this:be-fore the selection of seed instances,the algorithm can calculate for each unlabelled instance its dis-tance from each of the other unlabelled instances. In this way,it can build a prototypical seed set by selecting those instances with the smallest dis-tance on average.Figure6indicates that such an algorithm indeed outperforms random sample se-lection on the mixed organization data.For the calculation of the initial distances,each feature re-ceived the same weight.The algorithm then se-lected50random samples from the‘most proto-typical’half of the training set.5The other settings were the same as above.With the present small number of features,how-ever,such a prototypical seed set is not yet always as advantageous as it could be.A few experiments indicated that it did not lead to better performance on the mixed country data,for instance.However, as soon as a wider variety of features is taken into account(as with the organization data),the advan-pling can help choose those instances that are most helpful to the classifier.A few distance-based al-gorithms were able to drastically reduce the num-ber of training instances that is needed for a given accuracy,both for the country and the organization names.If current metonymy recognition algorithms are to be used in a system that can recognize all pos-sible metonymical patterns across a broad variety of semantic classes,it is crucial that the required number of labelled training examples be reduced. This paper has taken thefirst steps along this path and has set out some interesting questions for fu-ture research.This research should include the investigation of new features that can make clas-sifiers more robust and allow us to measure their confidence more reliably.This confidence mea-surement can then also be used in semi-supervised learning algorithms,for instance,where the clas-sifier itself labels the majority of training exam-ples.Only with techniques such as selective sam-pling and semi-supervised learning can the knowl-edge acquisition bottleneck in metonymy recogni-tion be addressed.AcknowledgementsI would like to thank Mirella Lapata,Dirk Geer-aerts and Dirk Speelman for their feedback on this project.I am also very grateful to Katja Markert and Malvina Nissim for their helpful information about their research.ReferencesD.W.Aha, D.Kibler,and M.K.Albert.1991.Instance-based learning algorithms.Machine Learning,6:37–66.W.Daelemans and A.Van den Bosch.1992.Generali-sation performance of backpropagation learning on a syllabification task.In M.F.J.Drossaers and A.Ni-jholt,editors,Proceedings of TWLT3:Connection-ism and Natural Language Processing,pages27–37, Enschede,The Netherlands.W.Daelemans,J.Zavrel,K.Van der Sloot,andA.Van den Bosch.2004.TiMBL:Tilburg Memory-Based Learner.Technical report,Induction of Linguistic Knowledge,Computational Linguistics, Tilburg University.D.Fass.1997.Processing Metaphor and Metonymy.Stanford,CA:Ablex.A.Fujii,K.Inui,T.Tokunaga,and H.Tanaka.1998.Selective sampling for example-based wordsense putational Linguistics, 24(4):573–597.R.Hwa.2002.Sample selection for statistical parsing.Computational Linguistics,30(3):253–276.koff and M.Johnson.1980.Metaphors We LiveBy.London:The University of Chicago Press.D.Lin.1998.An information-theoretic definition ofsimilarity.In Proceedings of the International Con-ference on Machine Learning,Madison,USA.K.Markert and M.Nissim.2002a.Metonymy res-olution as a classification task.In Proceedings of the Conference on Empirical Methods in Natural Language Processing(EMNLP2002),Philadelphia, USA.K.Markert and M.Nissim.2002b.Towards a cor-pus annotated for metonymies:the case of location names.In Proceedings of the Third International Conference on Language Resources and Evaluation (LREC2002),Las Palmas,Spain.M.Nissim and K.Markert.2003.Syntactic features and word similarity for supervised metonymy res-olution.In Proceedings of the41st Annual Meet-ing of the Association for Computational Linguistics (ACL-03),Sapporo,Japan.M.Nissim and K.Markert.2005.Learning to buy a Renault and talk to BMW:A supervised approach to conventional metonymy.In H.Bunt,editor,Pro-ceedings of the6th International Workshop on Com-putational Semantics,Tilburg,The Netherlands. G.Nunberg.1978.The Pragmatics of Reference.Ph.D.thesis,City University of New York.M.Osborne and J.Baldridge.2004.Ensemble-based active learning for parse selection.In Proceedings of the Human Language Technology Conference of the North American Chapter of the Association for Computational Linguistics(HLT-NAACL).Boston, USA.J.Pustejovsky.1995.The Generative Lexicon.Cam-bridge,MA:MIT Press.78。
AI术语

人工智能专业重要词汇表1、A开头的词汇:Artificial General Intelligence/AGI通用人工智能Artificial Intelligence/AI人工智能Association analysis关联分析Attention mechanism注意力机制Attribute conditional independence assumption属性条件独立性假设Attribute space属性空间Attribute value属性值Autoencoder自编码器Automatic speech recognition自动语音识别Automatic summarization自动摘要Average gradient平均梯度Average-Pooling平均池化Accumulated error backpropagation累积误差逆传播Activation Function激活函数Adaptive Resonance Theory/ART自适应谐振理论Addictive model加性学习Adversarial Networks对抗网络Affine Layer仿射层Affinity matrix亲和矩阵Agent代理/ 智能体Algorithm算法Alpha-beta pruningα-β剪枝Anomaly detection异常检测Approximation近似Area Under ROC Curve/AUC R oc 曲线下面积2、B开头的词汇Backpropagation Through Time通过时间的反向传播Backpropagation/BP反向传播Base learner基学习器Base learning algorithm基学习算法Batch Normalization/BN批量归一化Bayes decision rule贝叶斯判定准则Bayes Model Averaging/BMA贝叶斯模型平均Bayes optimal classifier贝叶斯最优分类器Bayesian decision theory贝叶斯决策论Bayesian network贝叶斯网络Between-class scatter matrix类间散度矩阵Bias偏置/ 偏差Bias-variance decomposition偏差-方差分解Bias-Variance Dilemma偏差–方差困境Bi-directional Long-Short Term Memory/Bi-LSTM双向长短期记忆Binary classification二分类Binomial test二项检验Bi-partition二分法Boltzmann machine玻尔兹曼机Bootstrap sampling自助采样法/可重复采样/有放回采样Bootstrapping自助法Break-Event Point/BEP平衡点3、C开头的词汇Calibration校准Cascade-Correlation级联相关Categorical attribute离散属性Class-conditional probability类条件概率Classification and regression tree/CART分类与回归树Classifier分类器Class-imbalance类别不平衡Closed -form闭式Cluster簇/类/集群Cluster analysis聚类分析Clustering聚类Clustering ensemble聚类集成Co-adapting共适应Coding matrix编码矩阵COLT国际学习理论会议Committee-based learning基于委员会的学习Competitive learning竞争型学习Component learner组件学习器Comprehensibility可解释性Computation Cost计算成本Computational Linguistics计算语言学Computer vision计算机视觉Concept drift概念漂移Concept Learning System /CLS概念学习系统Conditional entropy条件熵Conditional mutual information条件互信息Conditional Probability Table/CPT条件概率表Conditional random field/CRF条件随机场Conditional risk条件风险Confidence置信度Confusion matrix混淆矩阵Connection weight连接权Connectionism连结主义Consistency一致性/相合性Contingency table列联表Continuous attribute连续属性Convergence收敛Conversational agent会话智能体Convex quadratic programming凸二次规划Convexity凸性Convolutional neural network/CNN卷积神经网络Co-occurrence同现Correlation coefficient相关系数Cosine similarity余弦相似度Cost curve成本曲线Cost Function成本函数Cost matrix成本矩阵Cost-sensitive成本敏感Cross entropy交叉熵Cross validation交叉验证Crowdsourcing众包Curse of dimensionality维数灾难Cut point截断点Cutting plane algorithm割平面法4、D开头的词汇Data mining数据挖掘Data set数据集Decision Boundary决策边界Decision stump决策树桩Decision tree决策树/判定树Deduction演绎Deep Belief Network深度信念网络Deep Convolutional Generative Adversarial Network/DCGAN深度卷积生成对抗网络Deep learning深度学习Deep neural network/DNN深度神经网络Deep Q-Learning深度Q 学习Deep Q-Network深度Q 网络Density estimation密度估计Density-based clustering密度聚类Differentiable neural computer可微分神经计算机Dimensionality reduction algorithm降维算法Directed edge有向边Disagreement measure不合度量Discriminative model判别模型Discriminator判别器Distance measure距离度量Distance metric learning距离度量学习Distribution分布Divergence散度Diversity measure多样性度量/差异性度量Domain adaption领域自适应Downsampling下采样D-separation (Directed separation)有向分离Dual problem对偶问题Dummy node哑结点Dynamic Fusion动态融合Dynamic programming动态规划5、E开头的词汇Eigenvalue decomposition特征值分解Embedding嵌入Emotional analysis情绪分析Empirical conditional entropy经验条件熵Empirical entropy经验熵Empirical error经验误差Empirical risk经验风险End-to-End端到端Energy-based model基于能量的模型Ensemble learning集成学习Ensemble pruning集成修剪Error Correcting Output Codes/ECOC纠错输出码Error rate错误率Error-ambiguity decomposition误差-分歧分解Euclidean distance欧氏距离Evolutionary computation演化计算Expectation-Maximization期望最大化Expected loss期望损失Exploding Gradient Problem梯度爆炸问题Exponential loss function指数损失函数Extreme Learning Machine/ELM超限学习机6、F开头的词汇Factorization因子分解False negative假负类False positive假正类False Positive Rate/FPR假正例率Feature engineering特征工程Feature selection特征选择Feature vector特征向量Featured Learning特征学习Feedforward Neural Networks/FNN前馈神经网络Fine-tuning微调Flipping output翻转法Fluctuation震荡Forward stagewise algorithm前向分步算法Frequentist频率主义学派Full-rank matrix满秩矩阵Functional neuron功能神经元7、G开头的词汇Gain ratio增益率Game theory博弈论Gaussian kernel function高斯核函数Gaussian Mixture Model高斯混合模型General Problem Solving通用问题求解Generalization泛化Generalization error泛化误差Generalization error bound泛化误差上界Generalized Lagrange function广义拉格朗日函数Generalized linear model广义线性模型Generalized Rayleigh quotient广义瑞利商Generative Adversarial Networks/GAN生成对抗网络Generative Model生成模型Generator生成器Genetic Algorithm/GA遗传算法Gibbs sampling吉布斯采样Gini index基尼指数Global minimum全局最小Global Optimization全局优化Gradient boosting梯度提升Gradient Descent梯度下降Graph theory图论Ground-truth真相/真实8、H开头的词汇Hard margin硬间隔Hard voting硬投票Harmonic mean调和平均Hesse matrix海塞矩阵Hidden dynamic model隐动态模型Hidden layer隐藏层Hidden Markov Model/HMM隐马尔可夫模型Hierarchical clustering层次聚类Hilbert space希尔伯特空间Hinge loss function合页损失函数Hold-out留出法Homogeneous同质Hybrid computing混合计算Hyperparameter超参数Hypothesis假设Hypothesis test假设验证9、I开头的词汇ICML国际机器学习会议Improved iterative scaling/IIS改进的迭代尺度法Incremental learning增量学习Independent and identically distributed/i.i.d.独立同分布Independent Component Analysis/ICA独立成分分析Indicator function指示函数Individual learner个体学习器Induction归纳Inductive bias归纳偏好Inductive learning归纳学习Inductive Logic Programming/ILP归纳逻辑程序设计Information entropy信息熵Information gain信息增益Input layer输入层Insensitive loss不敏感损失Inter-cluster similarity簇间相似度International Conference for Machine Learning/ICML国际机器学习大会Intra-cluster similarity簇内相似度Intrinsic value固有值Isometric Mapping/Isomap等度量映射Isotonic regression等分回归Iterative Dichotomiser迭代二分器10、K开头的词汇Kernel method核方法Kernel trick核技巧Kernelized Linear Discriminant Analysis/KLDA核线性判别分析K-fold cross validation k 折交叉验证/k 倍交叉验证K-Means Clustering K –均值聚类K-Nearest Neighbours Algorithm/KNN K近邻算法Knowledge base知识库Knowledge Representation知识表征11、L开头的词汇Label space标记空间Lagrange duality拉格朗日对偶性Lagrange multiplier拉格朗日乘子Laplace smoothing拉普拉斯平滑Laplacian correction拉普拉斯修正Latent Dirichlet Allocation隐狄利克雷分布Latent semantic analysis潜在语义分析Latent variable隐变量Lazy learning懒惰学习Learner学习器Learning by analogy类比学习Learning rate学习率Learning Vector Quantization/LVQ学习向量量化Least squares regression tree最小二乘回归树Leave-One-Out/LOO留一法linear chain conditional random field线性链条件随机场Linear Discriminant Analysis/LDA线性判别分析Linear model线性模型Linear Regression线性回归Link function联系函数Local Markov property局部马尔可夫性Local minimum局部最小Log likelihood对数似然Log odds/logit对数几率Logistic Regression Logistic 回归Log-likelihood对数似然Log-linear regression对数线性回归Long-Short Term Memory/LSTM长短期记忆Loss function损失函数12、M开头的词汇Machine translation/MT机器翻译Macron-P宏查准率Macron-R宏查全率Majority voting绝对多数投票法Manifold assumption流形假设Manifold learning流形学习Margin theory间隔理论Marginal distribution边际分布Marginal independence边际独立性Marginalization边际化Markov Chain Monte Carlo/MCMC马尔可夫链蒙特卡罗方法Markov Random Field马尔可夫随机场Maximal clique最大团Maximum Likelihood Estimation/MLE极大似然估计/极大似然法Maximum margin最大间隔Maximum weighted spanning tree最大带权生成树Max-Pooling最大池化Mean squared error均方误差Meta-learner元学习器Metric learning度量学习Micro-P微查准率Micro-R微查全率Minimal Description Length/MDL最小描述长度Minimax game极小极大博弈Misclassification cost误分类成本Mixture of experts混合专家Momentum动量Moral graph道德图/端正图Multi-class classification多分类Multi-document summarization多文档摘要Multi-layer feedforward neural networks多层前馈神经网络Multilayer Perceptron/MLP多层感知器Multimodal learning多模态学习Multiple Dimensional Scaling多维缩放Multiple linear regression多元线性回归Multi-response Linear Regression /MLR多响应线性回归Mutual information互信息13、N开头的词汇Naive bayes朴素贝叶斯Naive Bayes Classifier朴素贝叶斯分类器Named entity recognition命名实体识别Nash equilibrium纳什均衡Natural language generation/NLG自然语言生成Natural language processing自然语言处理Negative class负类Negative correlation负相关法Negative Log Likelihood负对数似然Neighbourhood Component Analysis/NCA近邻成分分析Neural Machine Translation神经机器翻译Neural Turing Machine神经图灵机Newton method牛顿法NIPS国际神经信息处理系统会议No Free Lunch Theorem/NFL没有免费的午餐定理Noise-contrastive estimation噪音对比估计Nominal attribute列名属性Non-convex optimization非凸优化Nonlinear model非线性模型Non-metric distance非度量距离Non-negative matrix factorization非负矩阵分解Non-ordinal attribute无序属性Non-Saturating Game非饱和博弈Norm范数Normalization归一化Nuclear norm核范数Numerical attribute数值属性14、O开头的词汇Objective function目标函数Oblique decision tree斜决策树Occam’s razor奥卡姆剃刀Odds几率Off-Policy离策略One shot learning一次性学习One-Dependent Estimator/ODE独依赖估计On-Policy在策略Ordinal attribute有序属性Out-of-bag estimate包外估计Output layer输出层Output smearing输出调制法Overfitting过拟合/过配Oversampling过采样15、P开头的词汇Paired t-test成对t 检验Pairwise成对型Pairwise Markov property成对马尔可夫性Parameter参数Parameter estimation参数估计Parameter tuning调参Parse tree解析树Particle Swarm Optimization/PSO粒子群优化算法Part-of-speech tagging词性标注Perceptron感知机Performance measure性能度量Plug and Play Generative Network即插即用生成网络Plurality voting相对多数投票法Polarity detection极性检测Polynomial kernel function多项式核函数Pooling池化Positive class正类Positive definite matrix正定矩阵Post-hoc test后续检验Post-pruning后剪枝potential function势函数Precision查准率/准确率Prepruning预剪枝Principal component analysis/PCA主成分分析Principle of multiple explanations多释原则Prior先验Probability Graphical Model概率图模型Proximal Gradient Descent/PGD近端梯度下降Pruning剪枝Pseudo-label伪标记16、Q开头的词汇Quantized Neural Network量子化神经网络Quantum computer量子计算机Quantum Computing量子计算Quasi Newton method拟牛顿法17、R开头的词汇Radial Basis Function/RBF径向基函数Random Forest Algorithm随机森林算法Random walk随机漫步Recall查全率/召回率Receiver Operating Characteristic/ROC受试者工作特征Rectified Linear Unit/ReLU线性修正单元Recurrent Neural Network循环神经网络Recursive neural network递归神经网络Reference model参考模型Regression回归Regularization正则化Reinforcement learning/RL强化学习Representation learning表征学习Representer theorem表示定理reproducing kernel Hilbert space/RKHS再生核希尔伯特空间Re-sampling重采样法Rescaling再缩放Residual Mapping残差映射Residual Network残差网络Restricted Boltzmann Machine/RBM受限玻尔兹曼机Restricted Isometry Property/RIP限定等距性Re-weighting重赋权法Robustness稳健性/鲁棒性Root node根结点Rule Engine规则引擎Rule learning规则学习18、S开头的词汇Saddle point鞍点Sample space样本空间Sampling采样Score function评分函数Self-Driving自动驾驶Self-Organizing Map/SOM自组织映射Semi-naive Bayes classifiers半朴素贝叶斯分类器Semi-Supervised Learning半监督学习semi-Supervised Support Vector Machine半监督支持向量机Sentiment analysis情感分析Separating hyperplane分离超平面Sigmoid function Sigmoid 函数Similarity measure相似度度量Simulated annealing模拟退火Simultaneous localization and mapping同步定位与地图构建Singular Value Decomposition奇异值分解Slack variables松弛变量Smoothing平滑Soft margin软间隔Soft margin maximization软间隔最大化Soft voting软投票Sparse representation稀疏表征Sparsity稀疏性Specialization特化Spectral Clustering谱聚类Speech Recognition语音识别Splitting variable切分变量Squashing function挤压函数Stability-plasticity dilemma可塑性-稳定性困境Statistical learning统计学习Status feature function状态特征函Stochastic gradient descent随机梯度下降Stratified sampling分层采样Structural risk结构风险Structural risk minimization/SRM结构风险最小化Subspace子空间Supervised learning监督学习/有导师学习support vector expansion支持向量展式Support Vector Machine/SVM支持向量机Surrogat loss替代损失Surrogate function替代函数Symbolic learning符号学习Symbolism符号主义Synset同义词集19、T开头的词汇T-Distribution Stochastic Neighbour Embedding/t-SNE T–分布随机近邻嵌入Tensor张量Tensor Processing Units/TPU张量处理单元The least square method最小二乘法Threshold阈值Threshold logic unit阈值逻辑单元Threshold-moving阈值移动Time Step时间步骤Tokenization标记化Training error训练误差Training instance训练示例/训练例Transductive learning直推学习Transfer learning迁移学习Treebank树库Tria-by-error试错法True negative真负类True positive真正类True Positive Rate/TPR真正例率Turing Machine图灵机Twice-learning二次学习20、U开头的词汇Underfitting欠拟合/欠配Undersampling欠采样Understandability可理解性Unequal cost非均等代价Unit-step function单位阶跃函数Univariate decision tree单变量决策树Unsupervised learning无监督学习/无导师学习Unsupervised layer-wise training无监督逐层训练Upsampling上采样21、V开头的词汇Vanishing Gradient Problem梯度消失问题Variational inference变分推断VC Theory VC维理论Version space版本空间Viterbi algorithm维特比算法Von Neumann architecture冯·诺伊曼架构22、W开头的词汇Wasserstein GAN/WGAN Wasserstein生成对抗网络Weak learner弱学习器Weight权重Weight sharing权共享Weighted voting加权投票法Within-class scatter matrix类内散度矩阵Word embedding词嵌入Word sense disambiguation词义消歧23、Z开头的词汇Zero-data learning零数据学习Zero-shot learning零次学习。
大语言模型增强因果推断
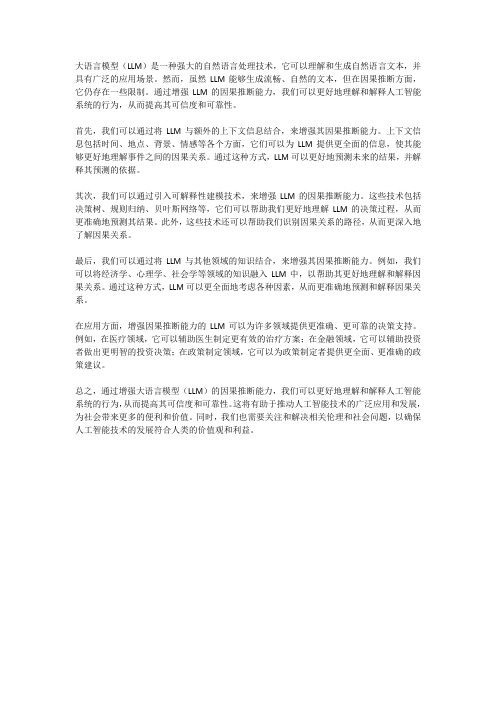
大语言模型(LLM)是一种强大的自然语言处理技术,它可以理解和生成自然语言文本,并具有广泛的应用场景。
然而,虽然LLM能够生成流畅、自然的文本,但在因果推断方面,它仍存在一些限制。
通过增强LLM的因果推断能力,我们可以更好地理解和解释人工智能系统的行为,从而提高其可信度和可靠性。
首先,我们可以通过将LLM与额外的上下文信息结合,来增强其因果推断能力。
上下文信息包括时间、地点、背景、情感等各个方面,它们可以为LLM提供更全面的信息,使其能够更好地理解事件之间的因果关系。
通过这种方式,LLM可以更好地预测未来的结果,并解释其预测的依据。
其次,我们可以通过引入可解释性建模技术,来增强LLM的因果推断能力。
这些技术包括决策树、规则归纳、贝叶斯网络等,它们可以帮助我们更好地理解LLM的决策过程,从而更准确地预测其结果。
此外,这些技术还可以帮助我们识别因果关系的路径,从而更深入地了解因果关系。
最后,我们可以通过将LLM与其他领域的知识结合,来增强其因果推断能力。
例如,我们可以将经济学、心理学、社会学等领域的知识融入LLM中,以帮助其更好地理解和解释因果关系。
通过这种方式,LLM可以更全面地考虑各种因素,从而更准确地预测和解释因果关系。
在应用方面,增强因果推断能力的LLM可以为许多领域提供更准确、更可靠的决策支持。
例如,在医疗领域,它可以辅助医生制定更有效的治疗方案;在金融领域,它可以辅助投资者做出更明智的投资决策;在政策制定领域,它可以为政策制定者提供更全面、更准确的政策建议。
总之,通过增强大语言模型(LLM)的因果推断能力,我们可以更好地理解和解释人工智能系统的行为,从而提高其可信度和可靠性。
这将有助于推动人工智能技术的广泛应用和发展,为社会带来更多的便利和价值。
同时,我们也需要关注和解决相关伦理和社会问题,以确保人工智能技术的发展符合人类的价值观和利益。
英汉仿拟的关联阐释
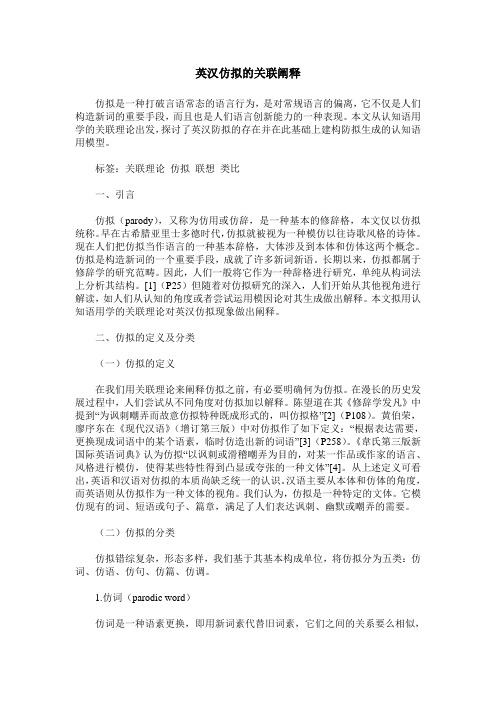
英汉仿拟的关联阐释仿拟是一种打破言语常态的语言行为,是对常规语言的偏离,它不仅是人们构造新词的重要手段,而且也是人们语言创新能力的一种表现。
本文从认知语用学的关联理论出发,探讨了英汉防拟的存在并在此基础上建构防拟生成的认知语用模型。
标签:关联理论仿拟联想类比一、引言仿拟(parody),又称为仿用或仿辞,是一种基本的修辞格,本文仅以仿拟统称。
早在古希腊亚里士多德时代,仿拟就被视为一种模仿以往诗歌风格的诗体。
现在人们把仿拟当作语言的一种基本辞格,大体涉及到本体和仿体这两个概念。
仿拟是构造新词的一个重要手段,成就了许多新词新语。
长期以来,仿拟都属于修辞学的研究范畴。
因此,人们一般将它作为一种辞格进行研究,单纯从构词法上分析其结构。
[1](P25)但随着对仿拟研究的深入,人们开始从其他视角进行解读,如人们从认知的角度或者尝试运用模因论对其生成做出解释。
本文拟用认知语用学的关联理论对英汉仿拟现象做出阐释。
二、仿拟的定义及分类(一)仿拟的定义在我们用关联理论来阐释仿拟之前,有必要明确何为仿拟。
在漫长的历史发展过程中,人们尝试从不同角度对仿拟加以解释。
陈望道在其《修辞学发凡》中提到“为讽刺嘲弄而故意仿拟特种既成形式的,叫仿拟格”[2](P108)。
黄伯荣,廖序东在《现代汉语》(增订第三版)中对仿拟作了如下定义:“根据表达需要,更换现成词语中的某个语素,临时仿造出新的词语”[3](P258)。
《韋氏第三版新国际英语词典》认为仿拟“以讽刺或滑稽嘲弄为目的,对某一作品或作家的语言、风格进行模仿,使得某些特性得到凸显或夸张的一种文体”[4]。
从上述定义可看出,英语和汉语对仿拟的本质尚缺乏统一的认识。
汉语主要从本体和仿体的角度,而英语则从仿拟作为一种文体的视角。
我们认为,仿拟是一种特定的文体。
它模仿现有的词、短语或句子、篇章,满足了人们表达讽刺、幽默或嘲弄的需要。
(二)仿拟的分类仿拟错综复杂,形态多样,我们基于其基本构成单位,将仿拟分为五类:仿词、仿语、仿句、仿篇、仿调。
人工智能主要研究方法
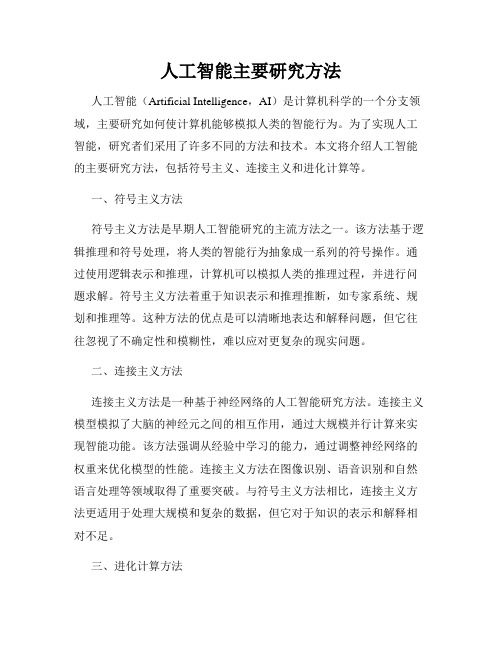
人工智能主要研究方法人工智能(Artificial Intelligence,AI)是计算机科学的一个分支领域,主要研究如何使计算机能够模拟人类的智能行为。
为了实现人工智能,研究者们采用了许多不同的方法和技术。
本文将介绍人工智能的主要研究方法,包括符号主义、连接主义和进化计算等。
一、符号主义方法符号主义方法是早期人工智能研究的主流方法之一。
该方法基于逻辑推理和符号处理,将人类的智能行为抽象成一系列的符号操作。
通过使用逻辑表示和推理,计算机可以模拟人类的推理过程,并进行问题求解。
符号主义方法着重于知识表示和推理推断,如专家系统、规划和推理等。
这种方法的优点是可以清晰地表达和解释问题,但它往往忽视了不确定性和模糊性,难以应对更复杂的现实问题。
二、连接主义方法连接主义方法是一种基于神经网络的人工智能研究方法。
连接主义模型模拟了大脑的神经元之间的相互作用,通过大规模并行计算来实现智能功能。
该方法强调从经验中学习的能力,通过调整神经网络的权重来优化模型的性能。
连接主义方法在图像识别、语音识别和自然语言处理等领域取得了重要突破。
与符号主义方法相比,连接主义方法更适用于处理大规模和复杂的数据,但它对于知识的表示和解释相对不足。
三、进化计算方法进化计算方法是一种基于生物进化理论的人工智能研究方法。
通过模拟遗传算法、进化策略和遗传规划等算法,进化计算方法通过迭代的方式来搜索最优解。
该方法模拟了进化的过程,通过适应度评估和进化操作来不断改进解的质量。
进化计算方法在优化问题、机器学习和数据挖掘等领域具有广泛的应用。
相对于前两种方法,进化计算方法更加灵活和自适应,但其效率较低,需要大量计算资源。
四、混合方法除了以上三种主要的研究方法外,还有一种被广泛采用的混合方法。
混合方法结合了符号主义、连接主义和进化计算方法的优点,以解决更复杂的问题。
例如,在人工智能的自动驾驶领域,研究者们同时采用了符号主义方法对规则进行建模,以及连接主义方法对感知和决策进行学习。
拉斯韦尔与他对传播学的贡献
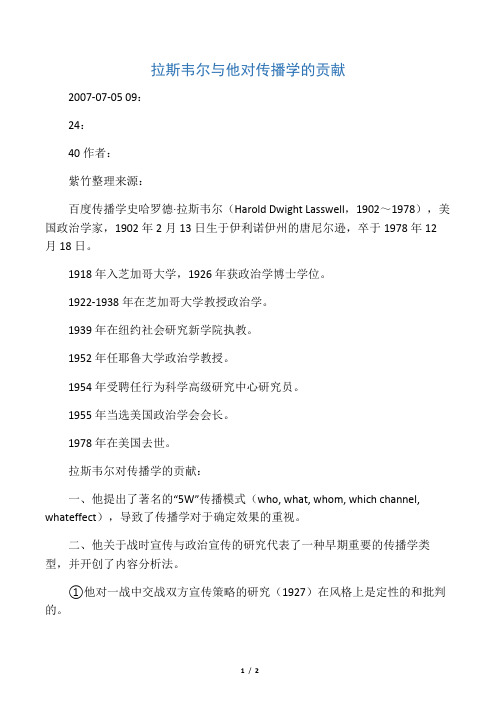
拉斯韦尔与他对传播学的贡献2007-07-05 09:24:40作者:紫竹整理来源:百度传播学史哈罗德·拉斯韦尔(Harold Dwight Lasswell,1902~1978),美国政治学家,1902年2月13日生于伊利诺伊州的唐尼尔逊,卒于1978年12月18日。
1918年入芝加哥大学,1926年获政治学博士学位。
1922-1938年在芝加哥大学教授政治学。
1939年在纽约社会研究新学院执教。
1952年任耶鲁大学政治学教授。
1954年受聘任行为科学高级研究中心研究员。
1955年当选美国政治学会会长。
1978年在美国去世。
拉斯韦尔对传播学的贡献:一、他提出了著名的“5W”传播模式(who, what, whom, which channel, whateffect),导致了传播学对于确定效果的重视。
二、他关于战时宣传与政治宣传的研究代表了一种早期重要的传播学类型,并开创了内容分析法。
①他对一战中交战双方宣传策略的研究(1927)在风格上是定性的和批判的。
他分析了协约国通过气球、飞机、炮弹向敌方战线散发的宣传传单所使用的说服策略,包括分裂敌人(诸如协约国努力使奥地利裔匈牙利人疏远德国),摧垮敌人的士气(诸如强调有成千上万的美国军队正如何抵达法国),控诉野蛮暴行的敌人(诸如德国士兵对于比利时儿童的虐待)等。
②他对二战期间宣传的的研究(1949)是定量的和统计学的。
三、他在二战时期承担了洛克菲勒基金会的“战时传播项目”,提出了传播在社会中的3个功能:①监督社会环境;②协调社会关系;③传递文化遗产(后来传播学者增加了传播的第4种功能:娱乐)。
四、他将弗洛伊德的精神分析理论引入美国社会科学。
他通过内容分析的途径将弗洛伊德的本我-自我-超我运用到政治学问题之中,在社会层面上运用了个体内部的弗洛伊德理论。
拉斯韦尔的主要论著:《世界大战中的宣传技巧》(1927)《精神病理学与政治学》(“Psychopathology and Politics”,1930年)《政治学:谁得到什么?什么时候和如何得到?》(“Politics:WhoGetsWhat,When,How”,1936年)《传播的结构和功能》(“The Structure and Function of Communication”,1948年)《政治的语言:语义的定量研究》(“The Language of Politics:Studies inQuantitative Semantics”,1965年)《世界历史上的宣传性传播》(“PropagandaCommunicatoninWorldHistory”,1979年,与人合著)。
- 1、下载文档前请自行甄别文档内容的完整性,平台不提供额外的编辑、内容补充、找答案等附加服务。
- 2、"仅部分预览"的文档,不可在线预览部分如存在完整性等问题,可反馈申请退款(可完整预览的文档不适用该条件!)。
- 3、如文档侵犯您的权益,请联系客服反馈,我们会尽快为您处理(人工客服工作时间:9:00-18:30)。
Empirical Semantics of Agent Communication in OpenSystemsMatthias NicklesAI/Cognition Group Department of Informatics T echnical University of Munich nickles@Gerhard WeissAI/Cognition Group Department of Informatics Technical University of Munich weissg@ABSTRACTThe paper proposes a novel approach to the semantics of communication of self-interested and autonomous agents in open systems.It defines the semantics of communicative acts primarily as the observable effect of their actual use in social encounters,and differs thus fundamentally from men-talistic and current objectivist approaches to the semantics of agent communication languages.Empirical communica-tion semantics enables the designer of agent-oriented soft-ware applications as well as agents to reason about social structures on the level of dynamically formed expectations, which we consider to be a crucial capability especially for social reasoning within and about open systems with truly autonomous black-or gray-box agents.KeywordsAgent Communication Languages,Open Systems1.INTRODUCTIONAlthough many approaches to the semantics of agent com-munication languages(ACL)have already been proposed, it is widely realized in distributed artificial intelligence that a comprehensive understanding of agent communication is still outstanding.Due to agent autonomy,which is certainly the crucial property of artificial agents,agents need to com-municate to achieve their goals interactively,where com-munication has to be seen in contrast to the exchange of information among ordinary objects.While atfirst glance it seems to be relatively easy to define a proper formal se-mantics for the so-called“content level”of agent languages (in contrast to the speech act illocution encoded by means of performatives),as it has been done using,e.g.,first order predicate calculus for KIF[8],there is still no general model of the actual usage and effects of utterances in social en-counters,afield which is traditionally studied in linguistical pragmatics and sociology.Currently,two major approaches to the meaning of agent communication in a broader sense, covering both traditional sentence semantics and pragmat-ics,exist,if we do not count plain interaction protocols(in some sense very simple social semantics.Interaction proto-cols can for example be used to provide a partial semantics for FIPA-ACL[5,13])and low-level formalisms like mes-sage passing.The older mentalistic approach(e.g.[6,3]) specifies the meaning of utterances by means of a descrip-tion of the mental states of the respective agents(i.e.,their beliefs and intentions,and thus indirectly their behaviour),while the more recent approaches(e.g.[1,4])try to de-termine communication from an objectivistic point of view, focussing on public rules.The former approach has two well-known shortcomings,which eventually led to the devel-opment of the latter:At least in open multiagent systems, agents appear more or less as black boxes,which makes it in general impossible to impose and verify a semantic described in terms of cognition.Furthermore,they make simplifying but unrealistic assumptions to ensure mental homogeneity among the agents,for example that the interacting agents were benevolent and sincere.Objectivist semantics in con-trast is fully verifiable,it achieves a big deal of complexity reduction through limiting itself to a small set of norma-tive rules,and has therefore been a significant step ahead. But it oversimplifies social processes in favor of traditional sentence-level semantics,and it doesn’t have a concept of meaning dynamics and generalization(cf.below).In gen-eral,we doubt that the predominately normative,static and definite concepts of current approaches to ACL semantics, borrowed from the study of programming languages and in-teraction protocols,are adequate to cope with concepts cru-cial for the successful deployment of agents to heterogenous, open environments with changing populations like the inter-net.Of course,this issue is less problematic for particular environments where agent benevolence and sincerity can be presumed and agent behavior is relatively restricted,but for upcoming information-rich environments like the Seman-tic Web,three particular communication-related properties, which are traditionally associated with human sociality,de-serve increased attention:1)meaning is usually the result of multiple heterogeneous,possibly indefinite and conflict-ing communications,2)benevolence and sincerity can not be assumed,and3)homogenous mental architectures and thus the uniform processing of communicated information cannot be assumed also.The reminder of this paper is organized as follows:The next section provides a more detailed descriptions of the difficulties of normative and non-normative meaning assign-ment.Section3outlines our semantical approach in re-sponse to these issues.Finally,section4draws some con-clusions regarding future work.2.ISSUES IN ACL SEMANTICS FOR OPENENVIRONMENTSThe meaning of utterances has two dimensions that need to be covered by a comprehensive approach to the semantics of agent communication:First,the sentence level,which isthe aspect of meaning that is traditionally subject of lin-guistical semantics.This aspect of meaning is contextual-ized with an application domain description in the form of a(assumably)consented ontology.In addition,a calculus to describe objects and events within the environment the respective utterance refers to has to be provided,for ex-ample predicate logic and temporal modalities.The second dimension of meaning,its pragmatics(i.e.,the actual use and effect of utterances in social encounters),contributes by far the most difficulties in ACL research.This is mainly due to agent autonomy,which makes it difficult to obtain deterministic descriptions of agent behavior.Thus,current objectivist approaches either deliberatively avoid pragmat-ics at all,or try to impose pragmatical rules in a normative manner(leaving beside mentalistic approaches,which are not suitable for black-or gray-box agents in open system for obvious reasons).In open systems,this is very prob-lematic for reasons pointed out in the following,although of course,not all of the described issues do deserve attention in all application domains.2.1Dynamics and Indefiniteness of Meaning The meaning of an utterance can most generally be char-acterized as its consequences,i.e.,the course of events caused by it[16,15,17].The currently most advanced approaches to ACL semantics determine this meaning as the result of a functional application of the respective speech act to the current discourse context,which has been derived as the outcome of the previous speech acts(e.g.,[4]).The dis-course context is thereby seen as a set of facts(e.g.,about anaphora,roles and other social structures),which serve as parameters for the application of the speech acts,while the speech acts themselves function as afixed mapping rule be-ing statically assigned to the ACL words.Such approaches work if the set of speech act types is known a-priori,each al-lowed utterance denotes afixed and known illocutionary act, and the constraining a certain discourse context imposes on the course of future speech acts is sufficient regarding the design goals,normative and known from the beginning on. They do not work,if these conditions are not known a-priori to either the system designer or the agents,or if they vary in a manner during run-time of the MAS which is not fore-seeable at design-time,as it can occur in heterogenous,open systems.2.2Speech Act TaxonomyThe usual set of performative types current ACLs provide represents an important,but nevertheless incomplete part of the set proposed by speech act theory[9,10].A perfor-mative has primarily the purpose to make the illocutionary act an agent performs explicit,and thus the set of performa-tives available to black-box agents would have to cover every possible communicational intention,which might be hardly foreseeable.As a work-around for this problem,contempo-rary agent languages like FIPA-ACL allow for an extension of the basic set of performatives,but not in computational adoption to run-time meaning dynamics.Another observa-tion which has been made in this respect is that the quite strict distinction traditional ACLs draw between performa-tive and content level(as with the duo KQML and KIF),is quite arbitrary[12],as well as the boundary of assertive and non-assertive performatives in speech act theory[11].2.3Rationality in InteractionCommunication has an unique property:It constructs a social situation,which is inherently consistent and reason-able,even if it opposes the“real world”outside communi-cation and the cognitive beliefs of the agents:1)Commu-nicated information is supposed to be consistent with infor-mation previously communicated by the same agent,or this agent at least justifies his change of mind,2)the agent de-fends and asserts his utterances by means of argumentation or other rational means like rewards and sanctions,and3) information not expressed explicitly can be deduced from information communicated before and background knowl-edge.If,for example,in an open auction on the internet some agent a asserts“I will deliver the goods if you win the auction.”,an observer does not need to believe him.But the observer believes that the further communication of a com-plies with this assertion.To make communication work,this belief is to some extent independent from reasoning about the true motives“within the agents mind”.Agent a is sup-posed to act at least for some time in a rational manner in accordance with the social image that he projects for himself by means of communication(e.g.,a sanctions the denial of his proposal,rewards its acceptance etc).The information about such(bounded-)rational attitude is implicitly associ-ated with each communication of a self-interested agent,and is thus part of communication semantics.Some approaches to ACL semantics somehow target this kind of social ratio-nality via the introduction of social commitments,but this term is quite underspecified[12],and often comes along with mental concepts like the“whole-hearted satisfaction”[2]to equip commitments with reliable intentions.2.4Generalization of MeaningCurrent approaches to ACL semantics are intended pri-marily for dyadic situations.Some of them allow for mes-sage broadcasts,but they lack a concept for unification and weighting of multiple messages or,respectively,responses, to reflect a(possibly inconsistent)common point of view of multiple agents,or to enable collaboration in joint commu-nicative action.It is hardly imaginable,how thousands or even millions of agents shall contribute to web semantics,if information agents are unable to generalize upon their com-munications by means of statistical evaluation.Of course, such abilities could be provided through special frameworks like recommender systems,but in fact,generalization is an inevitable part of communication meaning.In human com-munication,generalization of knowledge especially plays an important role for the assignment of meaning to underspeci-fied messages,and the temporal generalization of communi-cation trajectories(i.e.,the prediction of their effects from experience)in our opinion even provides the most appropri-ate semantics for agent communication in open systems,as we will specify next.In response to the described issues, we propose the following aspects a semantical framework should consider for being suitable in open environments.•Expectation-orientation and evolution of semantics.The meaning of utterances lies primarily within their ex-pectable consequences and might evolve during systemoperation.If no a-priori assumptions can be made,em-pirical evaluation of observations of message exchangeamong agents is the only feasible way to capture thismeaning.•Support for generalization.In complex or underspeci-fied social situations,it is required to generalize expec-tations regarding observed utterances to assign thema reasonable(however revisable)default meaning.•Rational attitude.Mentalistic approaches to agent se-mantics are not feasible for black-box agents,but nev-ertheless,even for such agents rational planning andacting can be assumed.Therefore,semantics assign-ment has to consider“social rationality”,i.e.,(bounded-)rational attitude in behavior.•Dynamic,context-driven performative ontology.Toovercome restrictions due to a pre-defined set of per-formatives,we plead for dynamic performative typeswhich gain their illocutionary force from the social con-text of their actual use.•Run-time derivation and propagation of semantics.Since empirical communication semantics are obtained dy-namically at run-time,a technical facility is requiredto derive and,if this facility is not located within theagents themselves,to propagate them similar to dictio-naries and grammars for human languages.Focussingon the design process of open systems,we’ve developedsuch an instance in form of a special middle-agent(theso-called Social System Mirror[15,16,17]).3.EMPIRICAL MODELLING OF COMMU-NICATIONThe communication model we propose is grounded in So-cial Systems Theory[14],as it has been introduced for the empirical derivation of communication structures of artifi-cial agents[15,16].This model is based on the assumption that sociality in truly open systems is basically the observ-able result of communication,and therefore social structures (e.g.,roles,public ontologies,organizational patterns)can be represented by means of probabilistic expectation struc-tures regarding future communication processes,which are dynamically extrapolated from observed message trajecto-ries.This observer is usually an agent which participates in the communication himself,but it could also be the system designer or a human application user.In our model[17],which we can only sketch very briefly and informally in this work for lack of space,a single com-munication attempt can be seen as a declarative request to act in conformance with the information asserted by an utterance(our approach does not follow speech act theory here because in our model an utterance always performs a declaration).In contrast to non-communicative events,an utterance has no(significant)direct impact on the physi-cal environment.Instead,its consequences are achieved so-cially,and,most important,the addressee is free to deny the communicated proposition.Since an utterance is al-ways explicitly produced by a self-interested agent to influ-ence the addressee,communicated content can not be“be-lieved”directly(otherwise the addressee could have derived its truth/usefulness herself and a communication would thus be unnecessary),but needs to be accompanied with social reasons given to the addressee to increase the probability of an acceptance of the communicated content.This can be done either explicitly by previous or subsequent communica-tions(e.g.,“If you comply,I’ll comply too”),or implicitly by means of generalizations from past events(e.g.,trust).The whole of the expectations which are triggered by a commu-nication in the context of the preceding communication pro-cess we call its rational hull.The rational hull specifies the traditional speech act context(anaphora,common ground information like public ontologies etc.),and,more impor-tant,the rational social relationships which steer the accep-tance or denial of communicated content according the ra-tional attitudes the agents exhibit.Typically,rational hulls are initially very indefinite and become increasingly definite in the course of interaction,provided that the agents work towards some(temporary)mutual understanding.Our model is centered around the following terms,which we propose primarily as“empirical replacements and supple-ments”for terms associated with traditional ACL semantics, like“message content”and“commitment”.Expectation structures The empirically obtained prob-ability distribution of all future event sequences re-sulting from the observance of the agents and theirenvironment.It needs to be adopted for each newlyobserved event and subsumes both communicative ac-tions and ordinary“physical”events.Expectation struc-tures can be modelled in multiple levels of generaliza-tion to enable the description of underspecified utter-ances,communication patterns,collaborative meaning(e.g.,opinions of agent groups)and agent roles(whichare basically generalizations of single-agent behavior).Expectation structures also provide for the commonground which contextualizes communication acts. Social expectation structures The part of the expecta-tion structures consisting of social expectations thatresult from communication processes and constrain fu-ture communications.The effect a certain utterancebrings about in terms of social expectation structuresis the semantics of this utterance.In contrast,physicalexpectation structures are domain-dependent expecta-tion structures(i.e.,ontological information about theagents environment).Physical expectation structuresinclude purely normative interaction structures but nosocial expectations.Utterances An agent action event with the following prop-erties:1)it is occurring under mutual observation,2)without considering social expectation structures,theevent would have a very low probability,3)its expectedconsequences in terms of physical expectation struc-tures only are of low relevance(think of the generationof sound waves through human voice),and4)consid-ering social expectation structures,the event needs tobe informative,i.e.,its probability must be lower1andmust result in a change of expectations.For utterancesusing a formal language and reliable technical messagetransmission,criteria1)to3)are clearly met. Projections Our equivalent to the terms“performative”and“content”.A projection is a part of the expecta-tion structures the uttering agent selects as an asser-tion regarding the future of the communication systemto make the addressee act in conformance with it(e.g.,fulfill a request).The observer needs to compare theutterance with both social and physical expectationstructures tofind the most probable match.The mostbasic kind of projection is obtained through demon-strative acting,where the uttering agent encodes itsmessage by means of“playing-act”.Rational hulls The rational behavior an agent is expected to perform to propagate,support and defend a certainuttered projection(e.g.,sanctions or argumentation).The rational hull is defined as the set of social expec-tations arising from the assumption that the utteringagent tries(at least for some time)to maximize theprobability that subsequent events are consistent withthe respective projection.A commitment can be seenas one possible means to such maximization:An agentcommits herself to perform certain actions to bringabout a certain behavior from another agent. Communication processes A set of probabilistically cor-related utterances with the following properties:1)each agent acts in consistence with the rational hullsinduced from his own utterances(e.g.,he does not con-tradict himself),and2)each projection is consistentwith1),i.e.it does not deny that property1)is met.Criterium2)is somehow an empirical kind of mentalunderstanding:With each communication,an agentacknowledges implicitly that the other agent tries toget accepted his projections,even if he does not agreewith these projections.Communication spheres A communication process together with the expectation structures arising from this pro-cess.Whereas a communication sphere is basically thesame as the interaction system known from social sys-tems theory[14],and resembles some of the propertiesof spheres of commitment[7],in our model it has dy-namic,empirically discovered boundaries in the sensethat communications which do not fulfill the consis-tency criteria for communication processes are not partof the respective sphere(but they can be modelledusing higher-order communication structures).Ex-amples for communication spheres are open marketsand auctions(cf.[16]for a case study on empiricalexpectation-oriented modelling of a trading platform)and(agent-supported)forums on the internet.Higher-order communication structures Social expec-tations which govern multiple communication spheresat the same time.4.CONCLUSIONWe’ve proposed a novel approach to the semantics of agent communication,based on the empirical evaluation of ob-served messages.We believe that this approach adopts bet-ter in comparison to traditional mentalistic or objectivist semantics to the peculiarities of large,heterogenous open system,as it enables both the software designer and the agents themselves to analyze the meaning of messages on the level of empirical expectations without the need to know about mental agent properties or architectural details.The biggest challenge for future research efforts in this respect is the development of an expectation-oriented ACL,respec-tively the assessment of an empirical(or semi-empirical, semi-normative)semantics to traditional ACLs.For this purpose,we are currently investigating the use offlexible behavioral patterns assigned to performatives of FIPA-ACL [5]to serve as a default semantics for empirical meaning as-signment.Acknowledgements:This work has been supported by the German National Science Foundation(DFG)under grant BR609/11-2.Thanks to Michael Rovatsos for useful com-ments on the topic of this work.5.REFERENCES[1]M.P.Singh.A social semantics for agentcommunication languages.In Proceedings of theIJCAI Workshop on Agent CommunicationLanguages,2000.[2]M.P.Singh.Multiagent Systems-A TheoreticalFramework for Intentions,Know-How,andCommunications.LNCS799,Springer,New York,1994.[3]brou,T.Finin.Semantics and conversations foran agent communication language.In Proceedings ofthe Fifteenth International Joint Conference onArtificial Intelligence(IJCAI-97),1997.[4]F.Guerin and J.Pitt.Denotational Semantics forAgent Communication Languages.In Proceedings ofthe5th International Conference on AutonomousAgents(Agents’01),ACM Press,2001.[5]FIPA,Foundation for Intelligent Agents,http://www.fi.[6]P.R.Cohen,municative actionsfor artificial agents.In Proceedings of the FirstInternational Conference on Multiagent Systems(ICMAS-95),1995.[7]M.Singh.Multiagent Systems as Spheres ofCommitment.In Proceedings of the ICMAS Workshop on Norms,Obligations,and Conventions,1996[8]M.R.Genesereth,R.E.Fikes.Knowledge InterchangeFormat,Version3.0Reference Manual.TechnicalReport Logic-92-1,Stanford University,Stanford,1992.[9]J.L.Austin.How to do things with words.ClarendonPress,Oxford,1962.[10]J.R.Searle.A taxonomy of illocutionary acts.In K.Gunderson(Ed.),Language,mind,and knowledge,pages344–369.University of Minnesota Press,1975. [11]K.Bach,R.Harnish.Linguistic Communication andSpeech Acts.MIT Press,Cambridge,Massachusetts,1979.[12]M.Colombetti,M.Verdicchio.An analysis of agentspeech acts as institutional actions.In Proceedings of the First International Joint Conference onAutonomous Agents and Multiagent Systems(AAMAS2002),2002.[13]J.Pitt,F.Bellifemine.A Protocol-Based Semanticsfor FIPA97ACL and its Implementation in JADE.Technical report,CSELT S.p.A.,1999.[14]N.Luhmann.Social Systems.Stanford UniversityPress,Palo Alto,CA,1995.[15]K.F.Lorentzen,M.Nickles.Ordnung aus Chaos–Prolegomena zu einer Luhmann’schen Modellierungdeentropisierender Strukturbildung inMultiagentensystemen.In T.Kron(Ed.),Luhmannmodelliert.Ans¨a tze zur Simulation vonKommunikationssystemen,Leske&Budrich,2002. [16]W.Brauer,M.Nickles,M.Rovatsos,G.Weiß,K.F.Lorentzen.Expectation-Oriented Analysis and Design.In Proceedings of The Second International Workshop on Agent-Oriented Software Engineering(AOSE-2001),LNCS2222,Springer,Berlin,2001. [17]M.Nickles.An Observation-based Approach to theSemantics of Agent Communication.Research Report FKI-24x-03,AI/Cognition Group,TechnicalUniversity of Munich,2003.To appear.。