A Simple Long Memory Model of Realized Volatility
北大考博辅导:北京大学理论经济学(国家发展)考博难度解析及经验分享
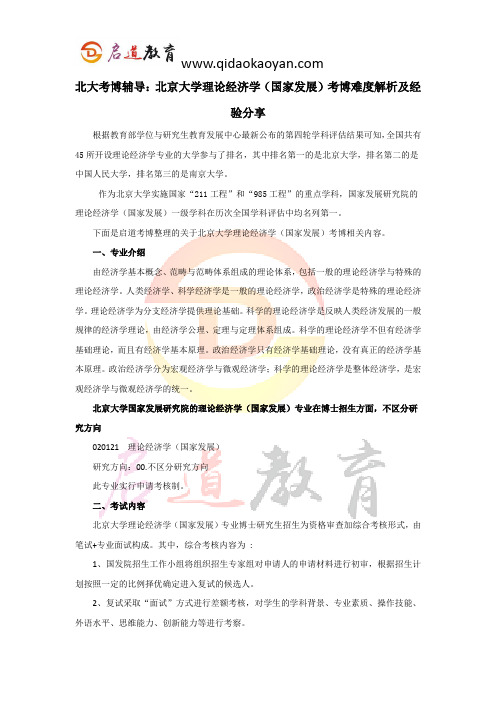
北大考博辅导:北京大学理论经济学(国家发展)考博难度解析及经验分享根据教育部学位与研究生教育发展中心最新公布的第四轮学科评估结果可知,全国共有45所开设理论经济学专业的大学参与了排名,其中排名第一的是北京大学,排名第二的是中国人民大学,排名第三的是南京大学。
作为北京大学实施国家“211工程”和“985工程”的重点学科,国家发展研究院的理论经济学(国家发展)一级学科在历次全国学科评估中均名列第一。
下面是启道考博整理的关于北京大学理论经济学(国家发展)考博相关内容。
一、专业介绍由经济学基本概念、范畴与范畴体系组成的理论体系,包括一般的理论经济学与特殊的理论经济学。
人类经济学、科学经济学是一般的理论经济学,政治经济学是特殊的理论经济学。
理论经济学为分支经济学提供理论基础。
科学的理论经济学是反映人类经济发展的一般规律的经济学理论,由经济学公理、定理与定理体系组成。
科学的理论经济学不但有经济学基础理论,而且有经济学基本原理。
政治经济学只有经济学基础理论,没有真正的经济学基本原理。
政治经济学分为宏观经济学与微观经济学;科学的理论经济学是整体经济学,是宏观经济学与微观经济学的统一。
北京大学国家发展研究院的理论经济学(国家发展)专业在博士招生方面,不区分研究方向020121 理论经济学(国家发展)研究方向:00.不区分研究方向此专业实行申请考核制。
二、考试内容北京大学理论经济学(国家发展)专业博士研究生招生为资格审查加综合考核形式,由笔试+专业面试构成。
其中,综合考核内容为:1、国发院招生工作小组将组织招生专家组对申请人的申请材料进行初审,根据招生计划按照一定的比例择优确定进入复试的候选人。
2、复试采取“面试”方式进行差额考核,对学生的学科背景、专业素质、操作技能、外语水平、思维能力、创新能力等进行考察。
3、考核时间一般安排在3月中下旬。
申请人须向复试专家小组作报告,内容包括个人科研经历和成果介绍、对拟从事研究的领域的了解和看法、本人拟进行的研究工作设想及理由等。
通过模仿或者重复学习英语的英语作文
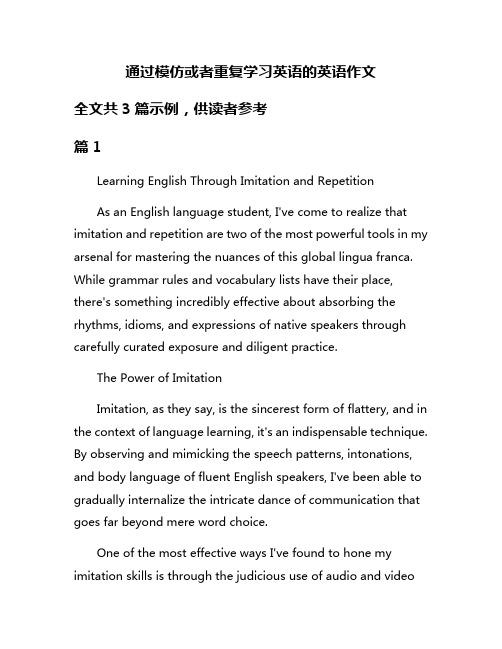
通过模仿或者重复学习英语的英语作文全文共3篇示例,供读者参考篇1Learning English Through Imitation and RepetitionAs an English language student, I've come to realize that imitation and repetition are two of the most powerful tools in my arsenal for mastering the nuances of this global lingua franca. While grammar rules and vocabulary lists have their place, there's something incredibly effective about absorbing the rhythms, idioms, and expressions of native speakers through carefully curated exposure and diligent practice.The Power of ImitationImitation, as they say, is the sincerest form of flattery, and in the context of language learning, it's an indispensable technique. By observing and mimicking the speech patterns, intonations, and body language of fluent English speakers, I've been able to gradually internalize the intricate dance of communication that goes far beyond mere word choice.One of the most effective ways I've found to hone my imitation skills is through the judicious use of audio and videoresources. From podcasts and audiobooks to movies and TV shows, immersing myself in authentic English content has allowed me to pick up on the subtle nuances that textbooks often fail to capture. I've learned to pay attention not just to the words themselves, but also to the rhythms, pauses, and emphases that lend authenticity to native speech.There's a certain thrill in catching myself unconsciously mimicking the cadence or inflection of a character I've grown fond of, or in seamlessly weaving an idiom I've picked up into a conversation. It's a tangible sign that the language is seeping into my bones, becoming not just a set of rules, but a living, breathing entity that I'm slowly but surely making my own.The Role of RepetitionWhile imitation allows me to absorb the melodic and expressive aspects of English, repetition is the hammer that drives those lessons home, forging them into permanent neural pathways. Whether it's through exercises, drills, or goodold-fashioned practice, repetition is the key to transforming tentative utterances into fluid, natural speech.One of the most effective repetition techniques I've employed is the good old-fashioned flashcard method. Creating personalized flashcards with vocabulary words, idioms, or evenentire sentences and phrases, and systematically reviewing them, has been instrumental in cementing new linguistic concepts in my mind. The act of repeatedly recalling and artic篇2Repetition, Repetition, Repetition: My Journey to English Fluency"Repetitio est mater studiorum" - the old Latin saying that repetition is the mother of all learning. As a non-native English speaker, I have found this adage to ring true in my quest to gain fluency in the English language. From my early days of repeating basic vocabulary words to my current practice of imitating native speakers, repetition and imitation have been the keystones to my English language acquisition.I still vividly remember the colorful flashcards my first English tutor used to drill simple words into my young mind - apple, book, cat, dog. At the time, the strange sounds seemed unfamiliar and awkward as they tumbled from my mouth. But through adamant repetition, those foreign utterances slowly became cemented into my vocabulary. I can now rattle off those elementary words without a second thought, their meanings ingrained into my consciousness through years of replication.As my English skills progressed, so did the complexity of the imitation required. Memorizing lengthy dialogues from television shows and movies became one of my favorite pastimes. I would obsessively rewind and rewatch the same clips over and over, keenly observing the mouth movements and vocal inflections of the actors. Attempting to precisely imitate their speech patterns, I would repeat each line until I had it down pat. People likely thought I was mad, manically mouthing words in an empty room like a deranged loner. But I didn't care - I was determined to capture the subtle nuances and natural cadence of native English speakers.This fixation on imitation extended into other facets of my life. I became hyper-aware of how people spoke, mentally filing away new words, idioms, and phrases to incorporate into my own speech. Riding the bus, I'd eavesdrop shamelessly on conversations, covertly mouthing unfamiliar expressions under my breath. At restaurants, I'd attentively study how servers interacted with customers. English became a collection of vocal patterns to be relentlessly catalogued and reproduced. Truthfully, this habit of linguistic voyeurism occasionally bordered on creepy. But it was all in service of the larger aim of sounding more natural and fluent.As tedious as it may sound, this dogmatic cycle of listening, imitating, and repeating was absolutely vital for building up my English proficiency from those initial stumbling blocks of vocabulary. Gradually, I went from merely memorizing words to aping the flow and articulation of complete sentences. Verb tenses, figures of speech, common idioms, and colloquialisms all started clicking into place. My interactions evolved from basic transactional exchanges into more nuanced conversations spanning abstract topics and contexts. The unnaturalstart-and-stop pattern of my early English slowly smoothed out into more fluid, naturalistic speech.Of course, imitation alone wasn't enough - I also had to take risks and ultimately put my skills into practice through real conversations. But first nailing down proper pronunciation and naturalistic patterns of speech was crucial scaffolding. The imitation laid the groundwork, providing a solid model to follow. From there, I could progressively add layers of spontaneity, injecting my own personal flair and stylistic divergences. Without first building that replicable framework through repetition and imitation, my progression into more extemporaneous types of verbal exchange would have been much more halting and uneven.Even today, with a level of English proficiency that allows me to effortlessly converse on complex topics, I still find myself relying on imitation as a tool. Anytime I'm exposed to a particularly eloquent speaker or writer, I'll make a concerted effort to analyze and deconstruct their language patterns. How are they structuring their sentences? What rhetorical devices are they employing? I'll spend time imitating their cadence and aping their turns of phrase, almost as an vocal exercise to stay nimble. It's helped me develop a much richer idiomatic repertoire and expanded my capacity for more vivid methods of expression.The process of imitation may seem like cheating, a way of dodging true language acquisition by simply copying those around you. And to some extent, that criticism is fair - imitation alone is not enough. Comprehensive language learning involves hardwork, formal study of rules and structures, and hopefully some level of immersion. But in my experience, repeated imitation, especially at the earlier stages of the learning process, is an indispensable part of getting conversational rhythms and natural pronunciation patterns deeply ingrained. It provides a solid foundation to build more substantive skills upon. Just as a child innately learns their first language simply through listeningand replicating the speech around them, imitation was a critical first step for me in acquiring English as a secondary language.So if you find yourself struggling to pick up the nuances and proper cadences of English, I cannot emphasize enough the importance of immersing yourself in imitation and repetition. Don't just repeat words and phrases in a vacuum - listen attentively to how native speakers actually employ the language. Pay close attention to stress, rhythm, intonation, and phrasing. Repeat entire expressions, sentences, or dialogues out loud until you can perfectly mirror the original speaker. It may seem monotonous at times, but stick with it. Ingrain those speech patterns into your muscle memory. Once you have those solid models firmly embedded, you'll find it infinitely easier to launch into more spontaneous and open-ended conversations. You'll be able to call upon those imitative foundations to keep your spoken English sounding smooth and natural, even as you freely riff with your own personal linguistic flair.English acquisition through imitation and repetition is, by its nature, an exercise in patience and diligence. It requires conscientious observation, relentless drilling, and persistent practice. But keeping up that cycle of listening, replicating, repeating is absolutely vital, especially early on. It provides therhythm, the melody, the cadences - the very backbone upon which more nuanced and spontaneous English skills can be built. So embrace your inner parrot and prepare to spend lots of time aping and repeating. It may not be the most glamorous path to fluency, but for many like myself, it's an indispensable one.篇3Learning English Through Imitation and RepetitionAs an English learner, I have found that imitation and repetition are two of the most powerful tools in my language acquisition arsenal. By mimicking native speakers and drilling vocabulary, grammar, and pronunciation patterns over and over again, I have been able to make remarkable progress in a relatively short period of time.At first, the idea of imitation and repetition seemed rather dull and tedious to me. I naively thought that simply studying textbooks and completing worksheets would allow the English language to slowly seep into my brain through osmosis. However, after struggling to achieve any measurable results with that approach, I realized I needed to get much more active and hands-on with my English studies.The imitation component kicked in when I started regularly watching movies, television shows, and online videos in English. At the beginning, I could barely understand a word that was being said. The rapid-fire delivery of native English speakers was just a jumbled mess of unfamiliar sounds. But instead of getting discouraged, I kept rewatching the same videos repeatedly while maniacally repeating every word, phrase, and sentence out loud.It felt ridiculous at first, sitting alone in my room parroting lines of dialogue from a movie I had already seen a dozen times. My mouth would contort into unnatural shapes as I tried to wrap my tongue around the strange vowel sounds and consonant clusters of English. My facial muscles would ache from the strain of over-exaggerating my mouth movements in an attempt to match those of the actors on the screen. Sometimes, the repetition would drive me mad, and I would have to take a break after spending hours on just a single scene.However, as bizarre as it seemed at the time, that obsessive level of imitation and repetition truly did work wonders. Gradually, I could sense the language patterns clicking into place within my brain. Words that had once felt like indecipherable gibberish started to sound more like real vocabulary with recognizable meanings and uses. The rhythms and cadences ofEnglish began to feel less foreign and started shaping the natural inclinations of my speech.I supplemented the imitation work with intense drills and repetition of key grammar rules, challenging idioms, irregular verb conjugations, and vocabulary lists. Breaking skills down into their component parts and then reconstructing them through repetition enabled me to attain a level of active mastery that simply would not have been possible through passive studying alone.While repeating the same word or phrase hundreds of times in a row may seem like an extraordinarily tedious exercise, it allowed me to overcome my mental blocks and push past the initial discomfort of producing unfamiliar sounds. With enough repetition, even the most unwieldy words and phrases became reflexive. Proper pronunciation, word stress, and intonation patterns were burned into my muscle memory until I no longer had to consciously think about them.Of course, simply being able to mimic and regurgitate words, phrases, and patterns is not the end goal of language learning. True proficiency requires productive skills that allow one to spontaneously generate original speech and writing. However,imitation and repetition provide the foundational building blocks that make those higher-level abilities possible.By amassing a large repository of memorized linguistic chunks through intense repetition, I could quickly retrieve and re-combine those pre-fabricated pieces into novel constructions during real-time communication. The more linguistic data I was able to stockpile through repetitive drilling, the more tools I had available in my mental toolbox to improvise and create when called upon.One extremely beneficial side effect of theimitation/repetition methodology was that it helped attune my ear to the authentic rhythms and musicality of native English speech. After repeatedly chanting along to countless hours of recorded audio, I could discern even the subtlest nuances of pronunciation, stress, and cadence. Not only did this improve my listening comprehension abilities, but it also enabled me to speak more fluidly and naturally myself rather than sounding rigid or overly accented.Of course, drilling words and re-speaking scripted dialogues is just one component of the language acquisition process. Imitation and repetition activities needed to be balanced withmeaningful input, conversational practice with native speakers, and ample opportunities for creative output.Still, I firmly believe that those core techniques of imitation and repetition provided the crucial foundation that allowed me to progress so rapidly with my English skills. They enabled me to chunk massive amounts of linguistic data into my long-term memory and compile an extensive database of prefabricated phrases to draw upon.By repeatedly forcing my mouth to contort into the proper shapes and my vocal cords to vibrate with the correct stresses and rhythms, I developed automatic muscle memories for producing natural-sounding English speech. All of those memorized patterns and ingrained habits could then be fluidly recombined and repurposed on the fly during extemporaneous communication.While imitation and repetition may seem like rather rudimentary techniques, they absolutely should not be overlooked or underestimated by language learners. Our brains are incredibly efficient pattern recognition and chunking machines, especially when it comes to acquiring languages during childhood through mimicry and repetitive drilling.By tapping into those same neurological pathways as an older learner, and applying imitation and repetition with the same vigor and single-minded intensity as a child, I was able to comprehensively rewire my brain for English in a way that would have never been possible through simply studying rules and definitions alone.So for any fellow English students out there feeling frustrated with their lack of progress, I cannot emphasize enough the importance of shedding your self-consciousness and fully embracing imitation and repetition. Listen and mimic relentlessly. Repeat everything until it becomes mindless habit. It may feel awkward at first, but powering through that initial discomfort by sheer force of stubborn, disciplined repetition is what will ultimately engrain the language deeply into both your muscle memory and cognitive memory.Imitation and repetition may seem like primitive brute-force techniques, but there's an unmistakable brilliance to their straightforward simplicity. They cut through all the complexities and go straight to the core mechanisms of how language acquisition truly works in the human brain. Master those two skills, and you'll be astonished at how rapidly you can progress in English or any other language you attempt to learn.。
逐渐消失的英语短语
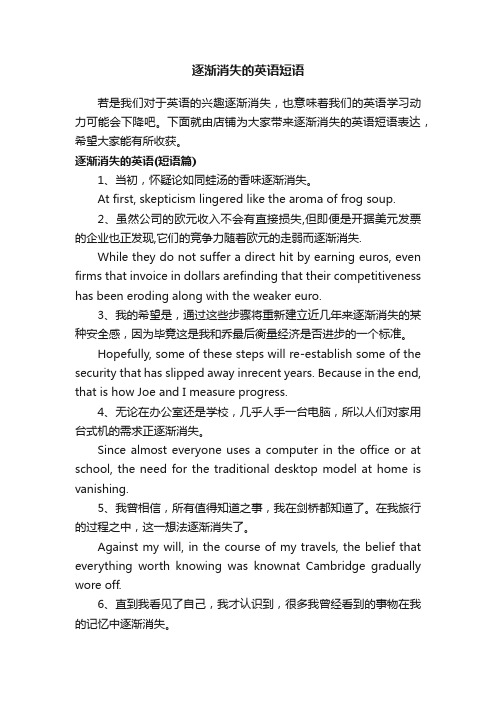
逐渐消失的英语短语若是我们对于英语的兴趣逐渐消失,也意味着我们的英语学习动力可能会下降吧。
下面就由店铺为大家带来逐渐消失的英语短语表达,希望大家能有所收获。
逐渐消失的英语(短语篇)1、当初,怀疑论如同蛙汤的香味逐渐消失。
At first, skepticism lingered like the aroma of frog soup.2、虽然公司的欧元收入不会有直接损失,但即便是开据美元发票的企业也正发现,它们的竞争力随着欧元的走弱而逐渐消失.While they do not suffer a direct hit by earning euros, even firms that invoice in dollars arefinding that their competitiveness has been eroding along with the weaker euro.3、我的希望是,通过这些步骤将重新建立近几年来逐渐消失的某种安全感,因为毕竟这是我和乔最后衡量经济是否进步的一个标准。
Hopefully, some of these steps will re-establish some of the security that has slipped away inrecent years. Because in the end, that is how Joe and I measure progress.4、无论在办公室还是学校,几乎人手一台电脑,所以人们对家用台式机的需求正逐渐消失。
Since almost everyone uses a computer in the office or at school, the need for the traditional desktop model at home is vanishing.5、我曾相信,所有值得知道之事,我在剑桥都知道了。
你是我的榜样英语作文600字写送水工

你是我的榜样英语作文600字写送水工全文共3篇示例,供读者参考篇1You Are My Role Model: An Essay About the Water Delivery WorkerWater, water everywhere, but not a drop to drink! At least that's how it feels living in the desert climate of my city. The scorching sun beats down relentlessly, sapping every last bit of moisture from the air and leaving us all parched and desperate for relief. But just when I think I can't take another sip of that metallic-tasting tap water, I hear the blessed sound of the water delivery truck rumbling down the street.And there he is - the water delivery worker, my real-life superhero, bringing us all the refreshing hydration we so desperately crave. With his bulging muscles glistening with sweat and a bright smile on his tan, rugged face, he leaps from the truck cab like a gazelle. The empty water bottles scattered across our neighborhood don't stand a chance against his Herculean strength as he swiftly loads them into the truck.I can't help but watch in awe as he effortlessly hoists those heavy, five-gallon water jugs up onto his broad shoulders, one after another, like they weigh no more than feathers. How does he do it? His incredible stamina and work ethic are truly inspiring.I don't think I could last five minutes under that scorching desert sun before collapsing in a sweaty, dehydrated heap.But not the water delivery man. No, he powers through the heat with a determination and grit that can only be admired. With each step, sweat glistens off his biceps, forming little rivulets that I imagine are actually my thirst being quenched with each refreshing drop of water he delivers. Is it getting hot in here, or is it just me?What I respect most, though, is his friendly, outgoing personality that brightens everyone's day. No matter how miserable the heat, he always greets us with a warm smile and cheerful wave. "Hot enough for ya?" he'll joke with a wink, his pearly whites glinting like freshly polished diamonds. A simple interaction, but it never fails to lift my spirits.I can't even count how many times I've rushed out to buy water from him, my parched throat feeling like sandpaper, only to be greeted by that signature sunny disposition and sparkling eyes. Suddenly, my discomfort melts away and the only thing I'mthirsty for is his...water. Yeah, his delicious, thirst-quenching water. What did you think I was going to say?Sometimes, if I'm lucky, a thin sheen of sweat will plaster his classic white tank top against his chiseled abdominal muscles, leaving very little to the imagination. And when he bends over to heave those water jugs into place, well...a girl can dream, can't she? I have to resist the urge to run my fingers along the deep crevices and grooves of his strapping shoulders and rippling back muscles as they shift beneath his taut skin.But enough about his physical, uh, attributes. What really makes the water delivery man my role model is his incredible work ethic and selfless dedication to keeping our community healthy and hydrated. Rain or shine (or blazing 115 degree heat), he's out there on the front lines, battling dehydration and making sure no one goes thirsty on his watch.His relentless reliability is something we can all aspire to. Like a finely-tuned machine, he follows his water delivery route with metronomic precision, never missing a drop-off or arriving even a minute behind schedule. He takes immense pride in his work, handling each water bottle with the same gentle care as a mother cupping her newborn baby's face. Those sturdy hands, calloused from years of hard labor, are actually soft and tender...Ahem. Where was I? Oh yes, his dependable work ethic! No matter how grueling the job or how heavy the load, he never complains or cuts corners. He'saidedicated professional to his core, a true paragon of commitment that we should all strive to emulate. If we all approached our lives and responsibilities with even half as much passion and diligence as the water delivery stud - I mean, worker - the world would be a much better place.So you can call me thirsty. You can call me desperate. But I am not ashamed to admit that the water delivery worker is, unequivocally, my role model. In a world that often feels utterly parched of real inspiration, he is a rejuvenating oasis. A cold rush of motivation and admiration that quenches my thirst for something - or someone - to look up to. He's the total package: hardworking, selfless, heroic...and let's not forget that bodacious bod and disarming smile.I dream of the day when I can work up the courage to actually talk to him instead of just ogling from my bedroom window like a creepy stalker. To finally express how much his service to the community has inspired me to become a better, more diligent person. Or maybe I'll just ask him out for a protein shake or something. Hey, a girl can dream! Either way, that waterdelivery stud will always be my one and only role model. Thanks to him, I'll never go thirsty again.篇2You Are My Role Model - An Essay About the Water Delivery WorkerAs I wake up each morning and get ready for school, one of the first sights I see out my bedroom window is Mr. Rahul arriving in his bright blue truck. He's our local water delivery worker, bringing those big blue bottles of drinking water to homes and businesses around the neighborhood. While my friends may idolize football players, movie stars or YouTube celebrities, Mr. Rahul is the person I most look up to and admire. Let me tell you why this humble yet hardworking man is my role model.Every day, no matter if it's blazing hot summer or the depths of the monsoon rains, Mr. Rahul shows up with a smile on his face, ready to do his job. He doesn't complain or make excuses. He just gets out of the truck and gets to work. I've seen him hauling those heavy water bottles up apartment stairs, loading and unloading his truck, even helping others by carrying theirgroceries. No task is too big or too small for him. His work ethic and positive attitude are inspirations to me.What's even more impressive is that Mr. Rahul maintains this level of effort and enthusiasm despite the nature of his job. Delivering water bottles doesn't seem like the most glamorous profession. It's hot, physically demanding labor. He could easily feel that this work is beneath him and put in minimal effort. But not Mr. Rahul. He takes pride in his work and giving his customers good service. He wants to do his absolute best, not just get by. That's the kind of dedicated worker and high-quality person I hope to become.I'll never forget the time I was running late for school and rushing out the door. I wasn't paying attention and tripped over one of the water bottles, dropping all my books and papers everywhere. Within seconds, Mr. Rahul was there, helping me collect my scattered belongings. He even gave me a few rewarding words of encouragement about staying focused on my studies. His kindness and the way he looks out for others, especially us neighborhood kids, makes him a role model of awesome character.Mr. Rahul hasn't had an easy life from what I understand. He came to this city as an immigrant, not knowing the language orhaving many resources. Through sheer perseverance and diligence, he found work and built a stable life for himself and his family. Whenever I start feeling sorry for myself or lacking motivation, I remember Mr. Rahul's story of overcoming hardship. I think about how he couldn't have achieved his goals without commitment and pushing himself daily. His example inspires me to work harder, aim higher, and never give up when faced with obstacles.In a world increasingly obsessed with fame, fortune and status, Mr. Rahul is a refreshing reminder that a truly admirable role model doesn't need those things. He's just an ordinary man doing an ordinary job. But he does it with extraordinary effort, integrity and care for others. Those are the qualities I want to embody as I get older. So yes, actors and athletes may get the glory. But for exemplifying the values of hard work, humility, resilience and basic human decency, Mr. Rahul will always be the real superstar in my eyes - a role model for life.篇3You Are My Role Model: An Ode to the English Water Delivery WorkerIt was just another sweltering summer day when I first encountered Mr. Johnson, our trusty water delivery man. Little did I know that this seemingly ordinary individual would soon become an extraordinary source of inspiration, forever etched in my memory as a paragon of perseverance and resilience.As the relentless sun beat down upon the parched streets, Mr. Johnson's battered truck navigated the winding roads, its cargo of life-sustaining liquid sloshing rhythmically with each turn. Drenched in perspiration, his weathered face bore the marks of a lifetime spent laboring under the scorching rays, a testament to his unwavering dedication.With each stop, I marveled at the ease with which he hoisted the cumbersome water bottles onto his broad shoulders, carrying them as if they weighed no more than feathers. It was a remarkable display of strength, one that belied the inherent grace with which he moved, his footsteps leaving barely a trace on the sun-baked pavement.Yet, it was not merely his physical prowess that captured my admiration; it was the warmth of his smile and the kindness that radiated from his eyes that truly captivated me. Despite the arduous nature of his work, Mr. Johnson greeted each customer with a genuine enthusiasm that seemed to transcend theoppressive heat, his cheerful demeanor a refreshing oasis amidst the arid landscape.As I watched him interact with the diverse array of individuals who awaited his arrival, I couldn't help but marvel at the ease with which he communicated. With a command of the English language that belied his humble occupation, he effortlessly bridged the gaps between cultures and backgrounds, forging connections that extended far beyond the simple exchange of goods and currency.It was in those moments that I realized the true depth of Mr. Johnson's character, for he was not merely a water delivery man; he was a ambassador of goodwill, spreading joy and compassion with every stop he made.But perhaps the most profound lesson I learned from Mr. Johnson was his unwavering resilience in the face of adversity. On days when the mercury soared to unbearable heights, when the very act of breathing seemed like a Herculean feat, he soldiered on, his footsteps never faltering, his resolve never wavering.I recall one particularly brutal afternoon when the air itself seemed to shimmer with heat, and the prospect of venturing outside was akin to stepping into the very fires of Hades itself.Yet, there was Mr. Johnson, trudging resolutely from door to door, his face etched with determination, his eyes fixed on the horizon.It was then that I understood the true meaning of perseverance, for in that moment, Mr. Johnson embodied the very essence of resilience, a living testament to the indomitable human spirit.As the years have passed, and my own path has taken me far from the streets where I first encountered this remarkable man, his legacy remains indelibly etched upon my heart. Whenever I find myself faced with seemingly insurmountable challenges, I think back to Mr. Johnson and the quiet strength he embodied, and I am reminded that no obstacle is too great, no burden too heavy, for those who possess an unwavering resolve.In a world that often celebrates the extraordinary and the grandiose, it is all too easy to overlook the unsung heroes who walk among us, their deeds as humble as their demeanors. Yet, it is individuals like Mr. Johnson who truly embody the essence of human greatness, their lives a tapestry woven from threads of perseverance, kindness, and an indomitable spirit.So, to Mr. Johnson, and to all those who labor tirelessly in the shadows, I offer this heartfelt tribute: You are my role model,a beacon of hope in a world that often seems shrouded in darkness. Your example has taught me that true greatness lies not in the accolades we receive or the riches we amass, but in the quiet dignity with which we carry out our duties, and the indelible impact we leave upon the lives we touch.Thank you, Mr. Johnson, for being the embodiment of resilience, for reminding me that even in the face of adversity, there is always。
2023年高考英语模拟卷

【赢在高考·黄金8卷】备战2023年高考英语模拟卷(浙江卷)07(考试时间:120分钟试卷满分:150分)注意事项:1.答卷前,考生务必将自己的姓名、考生号等填写在答题卡和试卷指定位置上。
2.回答选择题时,选出每小题答案后,用铅笔把答题卡对应题目的答案标号涂黑。
如需改动,用橡皮擦干净后,再选涂其他答案标号。
回答非选择题时,将答案写在答题卡上。
写在本试卷上无效。
3.考试结束后,将本试卷和答题卡一并交回。
第一部分听力(共两节,满分30 分)第一节(共5小题;每小题1分,满分5分)听下面5段对话。
每段对话后有一个小题,从题中所给的A、B、C三个选项中选出最佳选项。
听完每段对话后,你都有10秒钟的时间来回答有关小题和阅读下一小题。
每段对话仅读一遍。
1. How would Laura most probably go to work?A. By car.B. By bus.C. By bike.2. What are the speakers mainly talking about?A. A stranger.B. An artwork.C. A suspect.3. Where does the conversation take place?A. In a restaurant.B. In a shop.C. In a cinema.4. What does the woman want to do?A. Get a receipt.B. Deposit her luggage.C. Purchase some products.5. Why does the woman approach the man?A. To get a job.B. To walk with him.C. To give him notice.第二节(共15小题;每小题1分,满分15分)听下面5段对话或独白。
每段对话或独白后有几个小题,从题中所给的A、B、C 三个选项中选出最佳选项。
Modeling and Forecasting realized volatility

MODELING AND FORECASTING REALIZED VOLATILITY *by Torben G. Andersen a , Tim Bollerslev b , Francis X. Diebold c and Paul Labys dFirst Draft: January 1999Revised: January 2001, January 2002We provide a general framework for integration of high-frequency intraday data into the measurement,modeling, and forecasting of daily and lower frequency return volatilities and return distributions. Most procedures for modeling and forecasting financial asset return volatilities, correlations, and distributions rely on potentially restrictive and complicated parametric multivariate ARCH or stochastic volatilitymodels. Use of realized volatility constructed from high-frequency intraday returns, in contrast, permits the use of traditional time-series methods for modeling and forecasting. Building on the theory ofcontinuous-time arbitrage-free price processes and the theory of quadratic variation, we develop formal links between realized volatility and the conditional covariance matrix. Next, using continuouslyrecorded observations for the Deutschemark / Dollar and Yen / Dollar spot exchange rates covering more than a decade, we find that forecasts from a simple long-memory Gaussian vector autoregression for the logarithmic daily realized volatilities perform admirably compared to a variety of popular daily ARCH and more complicated high-frequency models. Moreover, the vector autoregressive volatility forecast,coupled with a parametric lognormal-normal mixture distribution implied by the theoretically andempirically grounded assumption of normally distributed standardized returns, produces well-calibrated density forecasts of future returns, and correspondingly accurate quantile predictions. Our results hold promise for practical modeling and forecasting of the large covariance matrices relevant in asset pricing,asset allocation and financial risk management applications.K EYWORDS : Continuous-time methods, quadratic variation, realized volatility, realized correlation, high-frequency data, exchange rates, vector autoregression, long memory, volatility forecasting, correlation forecasting, density forecasting, risk management, value at risk._________________* This research was supported by the National Science Foundation. We are grateful to Olsen and Associates, who generously made available their intraday exchange rate data. For insightful suggestions and comments we thank three anonymous referees and the Co-Editor, as well as Kobi Bodoukh, Sean Campbell, Rob Engle, Eric Ghysels, Atsushi Inoue, Eric Renault, Jeff Russell, Neil Shephard, Til Schuermann, Clara Vega, Ken West, and seminar participants at BIS (Basel), Chicago, CIRANO/Montreal, Emory,Iowa, Michigan, Minnesota, NYU, Penn, Rice, UCLA, UCSB, the June 2000 Meeting of the Western Finance Association, the July 2001 NSF/NBER Conference on Forecasting and Empirical Methods in Macroeconomics and Finance, the November 2001 NBER Meeting on Financial Risk Management, and the January 2002 North American Meeting of the Econometric Society.a Department of Finance, Kellogg School of Management, Northwestern University, Evanston, IL 60208, and NBER,phone: 847-467-1285, e-mail: t-andersen@bDepartment of Economics, Duke University, Durham, NC 27708, and NBER,phone: 919-660-1846, e-mail: boller@ c Department of Economics, University of Pennsylvania, Philadelphia, PA 19104, and NBER,phone: 215-898-1507, e-mail: fdiebold@dGraduate Group in Economics, University of Pennsylvania, 3718 Locust Walk, Philadelphia, PA 19104,phone: 801-536-1511, e-mail: labys@ Copyright © 2000-2002 T.G. Andersen, T. Bollerslev, F.X. Diebold and P. LabysAndersen, T., Bollerslev, T., Diebold, F.X. and Labys, P. (2003),"Modeling and Forecasting Realized Volatility,"Econometrica, 71, 529-626.1. INTRODUCTIONThe joint distributional characteristics of asset returns are pivotal for many issues in financial economics. They are the key ingredients for the pricing of financial instruments, and they speak directly to the risk-return tradeoff central to portfolio allocation, performance evaluation, and managerial decision-making. Moreover, they are intimately related to the fractiles of conditional portfolio return distributions, which govern the likelihood of extreme shifts in portfolio value and are therefore central to financial risk management, figuring prominently in both regulatory and private-sector initiatives.The most critical feature of the conditional return distribution is arguably its second moment structure, which is empirically the dominant time-varying characteristic of the distribution. This fact has spurred an enormous literature on the modeling and forecasting of return volatility.1 Over time, the availability of data for increasingly shorter return horizons has allowed the focus to shift from modeling at quarterly and monthly frequencies to the weekly and daily horizons. Forecasting performance has improved with the incorporation of more data, not only because high-frequency volatility turns out to be highly predictable, but also because the information in high-frequency data proves useful for forecasting at longer horizons, such as monthly or quarterly.In some respects, however, progress in volatility modeling has slowed in the last decade. First, the availability of truly high-frequency intraday data has made scant impact on the modeling of, say, daily return volatility. It has become apparent that standard volatility models used for forecasting at the daily level cannot readily accommodate the information in intraday data, and models specified directly for the intraday data generally fail to capture the longer interdaily volatility movements sufficiently well. As a result, standard practice is still to produce forecasts of daily volatility from daily return observations, even when higher-frequency data are available. Second, the focus of volatility modeling continues to be decidedly very low-dimensional, if not universally univariate. Many multivariate ARCH and stochastic volatility models for time-varying return volatilities and conditional distributions have, of course, been proposed (see, for example, the surveys by Bollerslev, Engle and Nelson (1994) and Ghysels, Harvey and Renault (1996)), but those models generally suffer from a curse-of-dimensionality problem that severely constrains their practical application. Consequently, it is rare to see substantive applications of those multivariate models dealing with more than a few assets simultaneously.In view of such difficulties, finance practitioners have largely eschewed formal volatility modeling and forecasting in the higher-dimensional situations of practical relevance, relying instead on1 Here and throughout, we use the generic term “volatilities” in reference both to variances (or standard deviations)ad hoc methods, such as simple exponential smoothing coupled with an assumption of conditionally normally distributed returns.2 Although such methods rely on counterfactual assumptions and are almost surely suboptimal, practitioners have been swayed by considerations of feasibility, simplicity and speed of implementation in high-dimensional environments.Set against this rather discouraging background, we seek to improve matters. We propose a new and rigorous framework for volatility forecasting and conditional return fractile, or value-at-risk (VaR), calculation, with two key properties. First, it efficiently exploits the information in intraday return data, without having to explicitly model the intraday data, producing significant improvements in predictive performance relative to standard procedures that rely on daily data alone. Second, it achieves a simplicity and ease of implementation, which, for example, holds promise for high-dimensional return volatility modeling.We progress by focusing on an empirical measure of daily return variability called realized volatility, which is easily computed from high-frequency intra-period returns. The theory of quadratic variation suggests that, under suitable conditions, realized volatility is an unbiased and highly efficient estimator of return volatility, as discussed in Andersen, Bollerslev, Diebold and Labys (2001) (henceforth ABDL) as well as in concurrent work by Barndorff-Nielsen and Shephard (2002, 2001a).3 Building on the notion of continuous-time arbitrage-free price processes, we advance in several directions, including rigorous theoretical foundations, multivariate emphasis, explicit focus on forecasting, and links to modern risk management via modeling of the entire conditional density.Empirically, by treating volatility as observed rather than latent, our approach facilitates modeling and forecasting using simple methods based directly on observable variables.4 We illustrate the ideas using the highly liquid U.S. dollar ($), Deutschemark (DM), and Japanese yen (¥) spot exchange rate markets. Our full sample consists of nearly thirteen years of continuously recorded spot quotations from 1986 through 1999. During that period, the dollar, Deutschemark and yen constituted2This approach is exemplified by the highly influential “RiskMetrics” of J.P. Morgan (1997).3 Earlier work by Comte and Renault (1998), within the context of estimation of a long-memory stochastic volatility model, helped to elevate the discussion of realized and integrated volatility to a more rigorous theoretical level.4 The direct modeling of observable volatility proxies was pioneered by Taylor (1986), who fit ARMA models to absolute and squared returns. Subsequent empirical work exploiting related univariate approaches based on improved realized volatility measures from a heuristic perspective includes French, Schwert and Stambaugh (1987) and Schwert (1989), who rely on daily returns to estimate models for monthly realized U.S. equity volatility, and Hsieh (1991), who fits an AR(5) model to a time series of daily realized logarithmic volatilities constructed from 15-minute S&P500 returns.the main axes of the international financial system, and thus spanned the majority of the systematic currency risk faced by large institutional investors and international corporations.We break the sample into a ten-year "in-sample" estimation period, and a subsequent two-and-a-half-year "out-of-sample" forecasting period. The basic distributional and dynamic characteristics of the foreign exchange returns and realized volatilities during the in-sample period have been analyzed in detail by ABDL (2000a, 2001).5 Three pieces of their results form the foundation on which the empirical analysis of this paper is built. First, although raw returns are clearly leptokurtic, returns standardized by realized volatilities are approximately Gaussian. Second, although the distributions of realized volatilities are clearly right-skewed, the distributions of the logarithms of realized volatilities are approximately Gaussian. Third, the long-run dynamics of realized logarithmic volatilities are well approximated by a fractionally-integrated long-memory process.Motivated by the three ABDL empirical regularities, we proceed to estimate and evaluate a multivariate model for the logarithmic realized volatilities: a fractionally-integrated Gaussian vector autoregression (VAR) . Importantly, our approach explicitly permits measurement errors in the realized volatilities. Comparing the resulting volatility forecasts to those obtained from currently popular daily volatility models and more complicated high-frequency models, we find that our simple Gaussian VAR forecasts generally produce superior forecasts. Furthermore, we show that, given the theoretically motivated and empirically plausible assumption of normally distributed returns conditional on the realized volatilities, the resulting lognormal-normal mixture forecast distribution provides conditionally well-calibrated density forecasts of returns, from which we obtain accurate estimates of conditional return quantiles.In the remainder of this paper, we proceed as follows. We begin in section 2 by formally developing the relevant quadratic variation theory within a standard frictionless arbitrage-free multivariate pricing environment. In section 3 we discuss the practical construction of realized volatilities from high-frequency foreign exchange returns. Next, in section 4 we summarize the salient distributional features of returns and volatilities, which motivate the long-memory trivariate Gaussian VAR that we estimate in section 5. In section 6 we compare the resulting volatility point forecasts to those obtained from more traditional volatility models. We also evaluate the success of the density forecasts and corresponding VaR estimates generated from the long-memory Gaussian VAR in5 Strikingly similar and hence confirmatory qualitative findings have been obtained from a separate sample consisting of individual U.S. stock returns in Andersen, Bollerslev, Diebold and Ebens (2001).conjunction with a lognormal-normal mixture distribution. In section 7 we conclude with suggestions for future research and discussion of issues related to the practical implementation of our approach for other financial instruments and markets.2. QUADRATIC RETURN VARIATION AND REALIZED VOLATILITYWe consider an n -dimensional price process defined on a complete probability space, (,Û, P), evolvingin continuous time over the interval [0,T], where T denotes a positive integer. We further consider an information filtration, i.e., an increasing family of -fields, (Ût )t 0[0,T] f Û , which satisfies the usual conditions of P -completeness and right continuity. Finally, we assume that the asset prices through time t , including the relevant state variables, are included in the information set Ût .Under the standard assumptions that the return process does not allow for arbitrage and has afinite instantaneous mean the asset price process, as well as smooth transformations thereof, belong to the class of special semi-martingales, as detailed by Back (1991). A fundamental result of stochastic integration theory states that such processes permit a unique canonical decomposition. In particular, we have the following characterization of the logarithmic asset price vector process, p = (p(t))t 0[0,T].PROPOSITION 1: For any n-dimensional arbitrage-free vector price process with finite mean, the logarithmic vector price process, p, may be written uniquely as the sum of a finite variation and predictable mean component, A = (A 1 , ... , A n ), and a local martingale, M = (M 1 , ... , M n ). These may each be decomposed into a continuous sample-path and jump part,p(t) = p(0) + A(t) + M(t) = p(0) + A c (t) + )A(t) + M c (t) + )M(t),(1)where the finite-variation predictable components, A c and )A, are respectively continuous and pure jump processes, while the local martingales, M c and )M, are respectively continuous sample-path and compensated jump processes, and by definition M(0) / A(0) / 0. Moreover, the predictable jumps are associated with genuine jump risk, in the sense that if )A(t) ú 0, thenP [ sgn( )A(t) ) = - sgn( )A(t)+)M(t) ) ] > 0 ,(2)where sgn(x) / 1 for x $0 and sgn(x) / -1 for x < 0.Equation (1) is standard, see, for example, Protter (1992), chapter 3. Equation (2) is an implication of6 This does not appear particularly restrictive. For example, if an announcement is pending, a natural way to model the arrival time is according to a continuous hazard function. Then the probability of a jump within each (infinitesimal)instant of time is zero - there is no discrete probability mass - and by arbitrage there cannot be a predictable jump.the no-arbitrage condition. Whenever )A(t) ú 0, there is a predictable jump in the price - the timing and size of the jump is perfectly known (just) prior to the jump event - and hence there is a trivial arbitrage (with probability one) unless there is a simultaneous jump in the martingale component, )M(t) ú 0. Moreover, the concurrent martingale jump must be large enough (with strictly positive probability) to overturn the gain associated with a position dictated by sgn()A(t)).Proposition 1 provides a general characterization of the asset return process. We denote the(continuously compounded) return over [t-h,t] by r(t,h) = p(t) - p(t-h). The cumulative return process from t=0 onward, r = (r(t))t 0[0,T] , is then r(t) / r(t,t) = p(t) - p(0) = A(t) + M(t). Clearly, r(t) inherits all the main properties of p(t) and may likewise be decomposed uniquely into the predictable andintegrable mean component, A , and the local martingale, M . The predictability of A still allows for quite general properties in the (instantaneous) mean process, for example it may evolve stochastically and display jumps. Nonetheless, the continuous component of the mean return must have smooth sample paths compared to those of a non-constant continuous martingale - such as a Brownian motion - and any jump in the mean must be accompanied by a corresponding predictable jump (of unknown magnitude) in the compensated jump martingale, )M . Consequently, there are two types of jumps in the return process, namely, predictable jumps where )A(t)ú0 and equation (2) applies, and purely unanticipated jumps where )A(t)=0 but )M(t)ú0. The latter jump event will typically occur when unanticipated news hit the market. In contrast, the former type of predictable jump may be associated with the release of information according to a predetermined schedule, such as macroeconomic news releases or company earnings reports. Nonetheless, it is worth noting that any slight uncertainty about the precise timing of the news (even to within a fraction of a second) invalidates the assumption of predictability and removes the jump in the mean process. If there are no such perfectly anticipated news releases, the predictable,finite variation mean return, A , may still evolve stochastically, but it will have continuous sample paths. This constraint is implicitly invoked in the vast majority of the continuous-time models employed in the literature.6Because the return process is a semi-martingale it has an associated quadratic variation process. Quadratic variation plays a critical role in our theoretical developments. The following proposition7 All of the properties in Proposition 2 follow, for example, from Protter (1992), chapter 2.8 In the general case with predictable jumps the last term in equation (4) is simply replaced by0#s #tr i (s)r j (s),where r i (s) /A i (s) + M i (s) explicitly incorporates both types of jumps. However, as discussed above, this case is arguable of little interest from a practical empirical perspective.enumerates some essential properties of the quadratic return variation process.7PROPOSITION 2: For any n-dimensional arbitrage-free price process with finite mean, the quadratic variation nxn matrix process of the associated return process, [r,r] = { [r,r]t }t 0[0,T] , is well-defined. The i’th diagonal element is called the quadratic variation process of the i’th asset return while the ij’th off-diagonal element, [r i ,r j ], is called the quadratic covariation process between asset returns i and j. The quadratic variation and covariation processes have the following properties:(i)For an increasing sequence of random partitions of [0,T], 0 = J m,0 # J m,1 # ..., such thatsup j $1(J m,j+1 - J m,j )60 and sup j $1 J m,j 6T for m 64 with probability one, we have thatlim m 64 { E j $1 [r(t v J m,j ) - r(t v J m,j-1)] [r(t v J m,j ) - r(t v J m,j-1)]’ } 6 [r,r]t ,(3)where t v J / min(t,J ), t 0 [0,T], and the convergence is uniform on [0,T] in probability.(ii)If the finite variation component, A, in the canonical return decomposition in Proposition 1 iscontinuous, then[r i ,r j ]t = [M i ,M j ]t = [M i c ,M j c ]t + E 0#s #t )M i (s) )M j (s) .(4)The terminology of quadratic variation is justified by property (i) of Proposition 2. Property (ii) reflects the fact that the quadratic variation of continuous finite-variation processes is zero, so the meancomponent becomes irrelevant for the quadratic variation.8 Moreover, jump components only contribute to the quadratic covariation if there are simultaneous jumps in the price path for the i ’th and j ’th asset,whereas the squared jump size contributes one-for-one to the quadratic variation. The quadratic variation process measures the realized sample-path variation of the squared return processes. Under the weak auxiliary condition ensuring property (ii), this variation is exclusively induced by the innovations to the return process. As such, the quadratic covariation constitutes, in theory, a unique and invariant ex-post realized volatility measure that is essentially model free. Notice that property (i) also suggests that we9 This has previously been discussed by Comte and Renault (1998) in the context of estimating the spot volatility for a stochastic volatility model corresponding to the derivative of the quadratic variation (integrated volatility) process. 10 This same intuition underlies the consistent filtering results for continuous sample path diffusions in Merton (1980)and Nelson and Foster (1995).may approximate the quadratic variation by cumulating cross-products of high-frequency returns.9 We refer to such measures, obtained from actual high-frequency data, as realized volatilities .The above results suggest that the quadratic variation is the dominant determinant of the return covariance matrix, especially for shorter horizons. Specifically, the variation induced by the genuine return innovations, represented by the martingale component, locally is an order of magnitude larger than the return variation caused by changes in the conditional mean.10 We have the following theorem which generalizes previous results in ABDL (2001).THEOREM 1: Consider an n-dimensional square-integrable arbitrage-free logarithmic price process with a continuous mean return, as in property (ii) of Proposition 2. The conditional return covariance matrix at time t over [t, t+h], where 0 # t # t+h # T, is then given byCov(r(t+h,h)*Ût ) = E([r,r ]t+h - [r,r ]t *Ût ) + 'A (t+h,h) + 'AM (t+h,h) + 'AM ’(t+h,h),(5)where 'A (t+h,h) = Cov(A(t+h) - A(t) * Ût ) and 'AM (t+h,h) = E(A(t+h) [M(t+h) - M(t)]’ *Ût ).PROOF: From equation (1), r(t+h,h) = [ A(t+h) - A(t) ] + [ M(t+h) - M(t) ]. The martingale property implies E( M(t+h) - M(t) *Ût ) = E( [M(t+h) - M(t)] A(t) *Ût ) = 0, so, for i,j 0 {1, ..., n}, Cov( [A i (t+h)- A i (t)], [M j (t+h) - M j (t)] * Ût ) = E( A i (t+h) [M j (t+h) - M j (t)] * Ût ). It therefore follows that Cov(r(t+h,h) * Ût ) = Cov( M(t+h) - M(t) * Ût ) + 'A (t+h,h) + 'AM (t+h,h) + 'AM ’(t+h,h). Hence, it only remains to show that the conditional covariance of the martingale term equals the expected value of the quadratic variation. We proceed by verifying the equality for an arbitrary element of the covariancematrix. If this is the i ’th diagonal element, we are studying a univariate square-integrable martingale and by Protter (1992), chapter II.6, corollary 3, we have E[M i 2(t+h)] = E( [M i ,M i ]t+h ), so Var(M i (t+h) -M i (t) * Ût ) = E( [M i ,M i ]t+h - [M i ,M i ]t * Ût ) = E( [r i ,r i ]t+h - [r i ,r i ]t * Ût ), where the second equality follows from equation (3) of Proposition 2. This confirms the result for the diagonal elements of the covariance matrix. An identical argument works for the off-diagonal terms by noting that the sum of two square-integrable martingales remains a square-integrable martingale and then applying the reasoning toeach component of the polarization identity, [M i ,M j ]t = ½ ( [M i +M j , M i +M j ]t - [M i ,M i ]t - [M j ,M j ]t ). In particular, it follows as above that E( [M i ,M j ]t+h - [M i ,M j ]t * Ût ) = ½ [ Var( [M i (t+h)+M j (t+h)] -[(M i (t)+M j (t)]* Ût ) - Var( M i (t+h) - M i (t)*Ût ) - Var( M j (t+h) - M j (t)*Ût ) ]= Cov( [M i (t+h) - M i (t)],[M j (t+h) - M j (t)]*Ût ). Equation (3) of Proposition 2 again ensures that this equals E( [r i ,r j ]t+h - [r i ,r j ]t * Ût ). 9Two scenarios highlight the role of the quadratic variation in driving the return volatility process. These important special cases are collected in a corollary which follows immediately from Theorem 1.COROLLARY 1: Consider an n-dimensional square-integrable arbitrage-free logarithmic price process, as described in Theorem 1. If the mean process, {A(s) - A(t)}s 0[t,t+h] , conditional on information at time t is independent of the return innovation process, {M(u)}u 0[t,t+h], then the conditional return covariance matrix reduces to the conditional expectation of the quadratic return variation plus the conditional variance of the mean component, i.e., for 0 # t # t+h # T,Cov( r(t+h,h) * Ût ) = E( [r,r ]t+h - [r,r ]t * Ût ) + 'A (t+h,h).If the mean process, {A(s) - A(t)}s 0[t,t+h], conditional on information at time t is a predetermined function over [t, t+h], then the conditional return covariance matrix equals the conditional expectation of the quadratic return variation process, i.e., for 0 # t # t+h # T,Cov( r(t+h,h) * Ût ) = E( [r,r ]t+h - [r,r ]t * Ût ).(6)Under the conditions leading to equation (6), the quadratic variation is the critical ingredient in volatility measurement and forecasting. This follows as the quadratic variation represents the actual variability of the return innovations, and the conditional covariance matrix is the conditional expectation of this quantity. Moreover, it implies that the time t+h ex-post realized quadratic variation is an unbiased estimator for the return covariance matrix conditional on information at time t .Although the corollary’s strong implications rely upon specific assumptions, these sufficientconditions are not as restrictive as an initial assessment may suggest, and they are satisfied for a wide set of popular models. For example, a constant mean is frequently invoked in daily or weekly return models. Equation (6) further allows for deterministic intra-period variation in the conditional mean,11 Merton (1982) provides a similar intuitive account of the continuous record h-asymptotics . These limiting results are also closely related to the theory rationalizing the quadratic variation formulas in Proposition 2 and Theorem 1.induced by time-of-day or other calendar effects. Of course, equation (6) also accommodates a stochastic mean process as long as it remains a function, over the interval [t, t+h], of variables in the time tinformation set. Specification (6) does, however, preclude feedback effects from the random intra-period evolution of the system to the instantaneous mean. Although such feedback effects may be present in high-frequency returns, they are likely trivial in magnitude over daily or weekly frequencies, as we argue subsequently. It is also worth stressing that (6) is compatible with the existence of an asymmetric return-volatility relation (sometimes called a leverage effect), which arises from a correlation between the return innovations, measured as deviations from the conditional mean, and the innovations to the volatility process. In other words, the leverage effect is separate from a contemporaneous correlation between the return innovations and the instantaneous mean return. Furthermore, as emphasized above,equation (6) does allow for the return innovations over [t-h, t] to impact the conditional mean over [t,t+h] and onwards, so that the intra-period evolution of the system may still impact the future expected returns. In fact, this is how potential interaction between risk and return is captured in discrete-time stochastic volatility or ARCH models with leverage effects.In contrast to equation (6), the first expression in Corollary 1 involving 'A explicitlyaccommodates continually evolving random variation in the conditional mean process, although the random mean variation must be independent of the return innovations. Even with this feature present,the quadratic variation is likely an order of magnitude larger than the mean variation, and hence the former remains the critical determinant of the return volatility over shorter horizons. This observation follows from the fact that over horizons of length h , with h small, the variance of the mean return is of order h 2, while the quadratic variation is of order h . It is an empirical question whether these results are a good guide for volatility measurement at relevant frequencies.11 To illustrate the implications at a daily horizon, consider an asset return with standard deviation of 1% daily, or 15.8% annually, and a (large)mean return of 0.1%, or about 25% annually. The squared mean return is still only one-hundredth of the variance. The expected daily variation of the mean return is obviously smaller yet, unless the required daily return is assumed to behave truly erratically within the day. In fact, we would generally expect the within-day variance of the expected daily return to be much smaller than the expected daily return itself. Hence, the daily return fluctuations induced by within-day variations in the mean return are almostcertainly trivial. For a weekly horizon, similar calculations suggest that the identical conclusion applies.。
HAR模型包:自动回归模型的估计、模拟和预测说明书
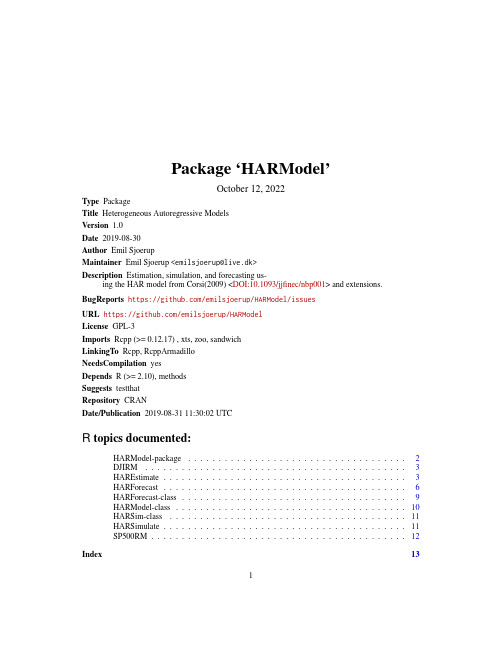
Package‘HARModel’October12,2022Type PackageTitle Heterogeneous Autoregressive ModelsVersion1.0Date2019-08-30Author Emil SjoerupMaintainer Emil Sjoerup<*******************>Description Estimation,simulation,and forecasting us-ing the HAR model from Corsi(2009)<DOI:10.1093/jjfinec/nbp001>and extensions. BugReports https:///emilsjoerup/HARModel/issuesURL https:///emilsjoerup/HARModelLicense GPL-3Imports Rcpp(>=0.12.17),xts,zoo,sandwichLinkingTo Rcpp,RcppArmadilloNeedsCompilation yesDepends R(>=2.10),methodsSuggests testthatRepository CRANDate/Publication2019-08-3111:30:02UTCR topics documented:HARModel-package (2)DJIRM (3)HAREstimate (3)HARForecast (6)HARForecast-class (9)HARModel-class (10)HARSim-class (11)HARSimulate (11)SP500RM (12)Index1312HARModel-package HARModel-package Heterogeneous Autoregressive ModelsDescriptionEstimation,simulation,and forecasting using the HAR model from Corsi(2009)<DOI:10.1093/jjfinec/nbp001>and extensions.DetailsThe DESCRIPTIONfile:Package:HARModelType:PackageTitle:Heterogeneous Autoregressive ModelsVersion: 1.0Date:2019-08-30Author:Emil SjoerupMaintainer:Emil Sjoerup<*******************>Description:Estimation,simulation,and forecasting using the HAR model from Corsi(2009)<DOI:10.1093/jjfinec/n BugReports:https:///emilsjoerup/HARModel/issuesURL:https:///emilsjoerup/HARModelLicense:GPL-3Imports:Rcpp(>=0.12.17),xts,zoo,sandwichLinkingTo:Rcpp,RcppArmadilloNeedsCompilation:YesDepends:R(>=2.10),methodsSuggests:testthatIndex of help topics:DJIRM Dow Jones Realized MeasuresHAREstimate HAR estimationHARForecast HAR forecastingHARForecast-class HARForecastHARModel-class HARModelHARModel-package Heterogeneous Autoregressive ModelsHARSim-class HARSimHARSimulate HAR simulationSP500RM SP500Realized MeasuresAuthor(s)Emil SjoerupMaintainer:Emil Sjoerup<*******************>DJIRM3 ReferencesCorsi,F.2009,A Simple Approximate Long-Memory Model of Realized V olatility,Journal of Fi-nancial Econometrics,174–196.DJIRM Dow Jones Realized MeasuresDescriptionRealized measures for the Dow Jones Industial index from2001to september2018FormatA large xts objectDetailsSee the website of the data set for details.Sourcehttps:///dataReferencesHeber,Gerd,Asger Lunde,Neil Shephard and Kevin Sheppard(2009)"Oxford-Man Institute’s realized library",Oxford-Man Institute,University of Oxford.Library version:0.3HAREstimate HAR estimationDescriptionHAR estimationUsageHAREstimate(RM,BPV=NULL,RQ=NULL,periods=c(1,5,22),periodsJ=NULL,periodsRQ=NULL,type="HAR",insanityFilter=TRUE,h=1)ArgumentsRM A numeric containing a realized measure of the integrated volatility.BPV A numeric containing the estimate of the continuous part of the integrated volatility used for HARJ and HARQ-J types.RQ A numeric containing the realized quarticity used for HARQ and HARQ-J types.periods A numeric denoting which lags should be used in the estimation,standard of c(1,5,22)is in line with Corsi(2009).periodsJ A numeric denoting which lags should be used in Jump estimation,if applica-ble.periodsRQ A numeric denoting which lags should be used in Realized Quarticity estima-tion,if applicable.type A character denoting which type of HAR model to estimate.insanityFilter A logical denoting whether the insanityfilter should be used for thefitted values of the estimation see Bollerslev,Patton&Quaedvlieg(2016)footnote17.h A integer denoting the whether and how much to aggregate the realized vari-ance estimator,if h=5the model is for the weekly volatility and if h=22,themodel is for the monthly volatility,the default of1designates no aggregation. DetailsThe estimates for the HARQ and HARQ-J models differ slightly from the results of BPQ(2016).This is due to a small difference in the demeaning approach for the realized quarticity.Here,the demeaning is done with mean(RQ)over all periods.ValueA HARModel objectAuthor(s)Emil SjoerupReferencesCorsi,F.2009,A Simple Approximate Long-Memory Model of Realized V olatility,Journal of Fi-nancial Econometrics,174–196.Bollerslev,T.,Patton,A.,Quaedvlieg,R.2016,Exploiting the errors:A simple approach for im-proved volatility forecasting,Journal of Econometrics,vol.192,issue1,1-18.Examples#Vanilla HAR from Corsi(2009)#load datadata("SP500RM")SP500rv=SP500RM$RV#Estimate the HAR model:FitHAR=HAREstimate(RM=SP500rv,periods=c(1,5,22))#extract the estimated coefficients:coef(FitHAR)#plot the fitted valuesplot(FitHAR)#calculate the Q-like loss-function:mean(qlike(FitHAR))#HAR-J:#load datadata("SP500RM")SP500rv=SP500RM$RVSP500bpv=SP500RM$BPV#Estimate the HAR-J model:FitHARJ=HAREstimate(RM=SP500rv,BPV=SP500bpv,periods=c(1,5,22),periodsJ=c(1,5,22),type="HARJ") #Calculate the Q-like loss-function:mean(qlike(FitHARJ))#HAR-Q of BPQ(2016)with weekly aggregation#load datadata("SP500RM")SP500rv=SP500RM$RVSP500rq=SP500RM$RQ#Estimate the HAR-Q model:FitHARQ=HAREstimate(RM=SP500rv,RQ=SP500rq,periods=c(1,5,22),periodsRQ=c(1,5,22),type="HARQ",h=5)#Show the model:show(FitHARQ)#Extract the coefficients:HARQcoef=coef(FitHARQ)#HARQ-J of BPQ(2016)with monthly aggregation#load datadata("SP500RM")SP500rv=SP500RM$RVSP500rq=SP500RM$RQSP500bpv=SP500RM$BPV#Estimate the HARQ-J model:FitHARQJ=HAREstimate(RM=SP500rv,BPV=SP500bpv,RQ=SP500rq,periods=c(1,5,22),periodsJ=c(1),periodsRQ=c(1),type="HARQ-J",h=22)#show the model:show(FitHARQJ)HARForecast HAR forecastingDescriptionRolling out of sample forecasting of a HAR model.UsageHARForecast(RM,BPV=NULL,RQ=NULL,periods=c(1,5,22),periodsJ=NULL,periodsRQ=NULL,nRoll=10,nAhead=1,type="HAR",windowType="rolling",insanityFilter=TRUE,h=1)ArgumentsRM An xts object containing a realized measure of the integrated volatility.BPV A numeric containing the jump proportion of the realized measure used for HARJ and HARQ-J types.RQ A numeric containing the realized quarticity used for HARQ and HARQ-J types.periods A vector denoting which lags should be used in the estimation,standard of c(1,5,22)is in line with Corsi(2009).periodsJ A numeric denoting which lags should be used in Jump estimation,if applica-ble.periodsRQ A numeric denoting which lags should be used in Realized Quarticity estima-tion,if applicable.nRoll How many rolling forecasts should be performed.nAhead The length of each rolling forecast.type A character denoting which type of HAR model to estimate.windowType A character denoting which kind of window to use,either"rolling"/"fixed"or "increasing"/"expanding".2-letter abbreviations can be used.insanityFilter A logical denoting whether the insanityfilter should be used for the forecasted values see Bollerslev,Patton&Quaedvlieg(2016)footnote17.h A integer denoting the whether and how much to aggregate the realized vari-ance estimator,if h=5the model is forecasting the weekly volatility and if h=22,the model is forecasting the monthly volatility,the default of1designatesno aggregation..DetailsNot all models in this package are’complete’,which means some models use AR(1)processes to forecast e.g.realized quarticity in order to construct more than one step ahead forecasts.The maximumm lag of the continuous or quarticity data must be lower than the maximum of the realized measure lag vector,the other cases are not implemented.The estimates for the HARQ and HARQ-J models differ slightly from the results of BPQ(2016).This is due to a small difference in the demeaning approach for the realized quarticity.Here,the demeaning is done with mean(RQ)over all periods.If h is greater than1,then nAhead must be one,as multi-period ahead forecasts have not been implemented.ValueA HARForecast objectAuthor(s)Emil SjoerupReferencesCorsi,F.2009,A Simple Approximate Long-Memory Model of Realized V olatility,Journal of Fi-nancial Econometrics,174–196.Bollerslev,T.,Patton,A.,Quaedvlieg,R.2016,Exploiting the errors:A simple approach for im-proved volatility forecasting,Journal of Econometrics,vol.192,issue1,1-18.See AlsoSee Also HAREstimateExamples#HAR of Corsi(2009)#load data:data("SP500RM")SP500rv=SP500RM$RVForecastHAR=HARForecast(SP500rv,periods=c(1,5,22),nRoll=50,nAhead=50,type="HAR")#plot the forecasted series along with the actual realizations:plot(ForecastHAR)#Calculate the MSE:mean(forecastRes(ForecastHAR)^2)#Calculate the Q-like loss function:mean(qlike(ForecastHAR))#HARJ#load data:data("SP500RM")SP500rv=SP500RM$RVSP500bpv=SP500RM$BPVForecastHARJ=HARForecast(SP500rv,BPV=SP500bpv,periods=c(1,5,22),periodsJ=c(1,5,22),nRoll=50,nAhead=50,type="HARJ")#Show the model:show(ForecastHARJ)#Extract the forecasted series:forc=getForc(ForecastHARJ)#HARQ BPQ(2016)#load datadata("SP500RM")SP500rv=SP500RM$RVSP500rq=SP500RM$RQForecastHARQ=HARForecast(SP500rv,RQ=SP500rq,periods=c(1,5,22),periodsRQ=c(1,5,22),nRoll=50,nAhead=50,type="HARQ")#HARQ-J BPQ(2016)with weekly aggregation.#load datadata("SP500RM")SP500rv=SP500RM$RVSP500rq=SP500RM$RQSP500bpv=SP500RM$BPVForecastHARQJ=HARForecast(SP500rv,RQ=SP500rq,BPV=SP500bpv,periods=c(1,5,22),periodsJ=c(1,5,22),periodsRQ=c(1,5,22),nRoll=50,nAhead=1,type="HARQ-J",h=5)HARForecast-class9 HARForecast-class HARForecastDescriptionClass for HARForecast objectObjects from the ClassA virtual Class:No objects may be created from itSlotsmodel:Object of class HARModel.see HARModelforecast:Object of class matrix containing the forecasted seriesinfo:Object of class list cointaining:•elapsedTime:Object of class difftime containing the time elapsed in seconds•rolls:Integer containing the amount of rolls done in the forecasting routine•horizon:Integer containing the length of the horizon used for forecasting during eachof the rollsdata:Object of class list containing:•dates:Object of type Integer or Date containing the indices of the forecasted serieseither in integer or date format•observations:Object of type numeric or xts containing the in-sample observations•forecastComparison:Object of type numeric or xts containing the observations keptout of sample for thefirst rollMethodsshow:signature(object="HARForecast"):Shows summaryplot:signature(x="HARForecast",y="missing"):Plot the out of sample observed series with the forecasts overlayeduncmean:signature(object="HARForecast"):Extracts the unconditional mean from the Model coef:signature(object="HARForecast"):Extracts the coefficients from thefirst estimated Modelqlike:signature(object="HARForecast"):Calculate the out of sample’qlike’loss function for a HARForecast objectforecastres:signature(object="HARForecast"):Retrieve the forecast residuals from HAR-Forecast objectforc:signature(object="HARForecast"):Retrieve the forecasted series.Author(s)Emil Sjoerup10HARModel-class HARModel-class HARModelDescriptionClass for HARModel objectsObjects from the ClassA virtual Class:No objects may be created from it.Slotsmodel:Object of class lm.Contains the linear modelfitted.info:Object of class list cointaining:•periods:numeric containing the lags used to create the model.If the type isn’t"HAR",then the related periods-(RQ)and/or(J)will also be included.•dates:Date object containing the dates for which the estimation was done,only appli-cable if the Model was estimated using an"xts"object.Methodsshow:signature(object="HARModel")Shows summaryplot:signature(x="HARModel",y="missing"):Plots the observed values withfitted values overlayeduncmean:signature(object="HARModel"):Extracts the unconditional mean from the Model, only available when type="HAR"coef:signature(object="HARModel"):Extracts the coefficients from the ModelsandwichNeweyWest:signature(object="HARModel"):Utilize the sandwich package to cre-ate newey west standard errorsqlike:signature(object="HARModel"):Calculate the in sample’qlike’loss function for a HARModel objectlogLik:A wrapper for the"lm"subclass of the HARModel objectconfint:A wrapper for the"lm"subclass of the HARModel objectresiduals:A wrapper for the"lm"subclass of the HARModel objectsummary:A wrapper for the"lm"subclass of the HARModel objectAuthor(s)Emil SjoerupHARSim-class11 HARSim-class HARSimDescriptionClass for HARSim objectObjects from the ClassA virtual Class:No objects may be created from itSlotssimulation:Object of class numeric containing the simulated seriesinfo:Object of class list cointaining:•len:Object of class numeric containing the length of the simulated series•periods:Object of class numeric containing the lag-vector used for simulation•coefficients:Object of class numeric containing the coefficients used for simulation•errorTermSD:Object of class numeric containing the standard error of the error term•elapsedTime:Object of class difftime containing the time elapsed in secondsMethodsshow:signature(object="HARSim"):Shows summaryplot:signature(x="HARSim",y="missing"):Plot the forecasted series and observed series as well as the residualsuncmean:signature(object="HARSim"):Extracts the unconditional mean from the simulation coef:signature(object="HARSim"):Extracts the coefficients from the simulationAuthor(s)Emil SjoerupHARSimulate HAR simulationDescriptionSimulates a HAR model.From using the AR representation of the HAR model.UsageHARSimulate(len=1500,periods=c(1,5,22),coef=c(0.01,0.36,0.28,0.28),errorTermSD=0.001)12SP500RMArgumentslen An integer determining the length of the simulated process.periods A numeric of lags for constructing the model,standard is c(1,5,22).coef A numeric of coefficients which will be used to simulate the process.errorTermSD A numeric determining the standard deviation of the error term.ValueA HARSim objectAuthor(s)Emil SjoerupReferencesCorsi,F.2009,A Simple Approximate Long-Memory Model of Realized V olatility,Journal of Fi-nancial Econometrics,174–196.Examplesset.seed(123)#Simulate the process of size10000HARSim=HARSimulate(len=10000,periods=c(1,5,22),coef=c(0.01,0.36,0.28,0.28),errorTermSD=0.001) HARFit=HAREstimate(HARSim@simulation,periods=c(1,5,22))SP500RM SP500Realized MeasuresDescriptionRealized measures from the SP500index from April1997to August2013.FormatA large xts object.Source/~ap172/code.htmlReferencesBollerslev,T.,A.J.Patton,and R.Quaedvlieg,2016,Exploiting the Errors:A Simple Approach for Improved V olatility Forecasting,Journal of Econometrics,192,1-18.Index∗HARHARForecast,6HARSimulate,11∗Heterogeneous Autoregressive model HARModel-package,2∗classesHARForecast-class,9HARModel-class,10HARSim-class,11∗datasetsDJIRM,3SP500RM,12∗forecastHARForecast,6∗simulationHARSimulate,11coef,HARForecast-method(HARForecast-class),9coef,HARModel-method(HARModel-class), 10coef,HARSim-method(HARSim-class),11 confint,HARModel-method(HARModel-class),10DJIRM,3fitted.values,HARModel-method(HARModel-class),10 forecastRes(HARForecast-class),9 forecastRes,ANY-method(HARForecast-class),9 forecastRes,HARForecast-method(HARForecast-class),9getForc(HARForecast-class),9 getForc,ANY-method(HARForecast-class), 9getForc,HARForecast-method(HARForecast-class),9HAREstimate,3,7HARForecast,6,7HARForecast-class,9HARModel,4,9HARModel(HARModel-package),2HARModel-class,10HARModel-package,2HARSim,12HARSim-class,11HARSimulate,11logLik,HARModel-method(HARModel-class),10plot,HARForecast,missing-method(HARForecast-class),9plot,HARModel,missing-method(HARModel-class),10plot,HARSim,missing-method(HARSim-class),11qlike(HARModel-class),10qlike,ANY-method(HARModel-class),10qlike,HARForecast-method(HARForecast-class),9qlike,HARModel-method(HARModel-class),10residuals,HARModel-method(HARModel-class),10sandwichNeweyWest(HARModel-class),10sandwichNeweyWest,ANY-method(HARModel-class),10sandwichNeweyWest,HARModel-method(HARModel-class),10show,HARForecast-method(HARForecast-class),9show,HARModel-method(HARModel-class),10show,HARSim-method(HARSim-class),11 1314INDEX SP500RM,12summary,HARModel-method(HARModel-class),10uncmean(HARModel-class),10uncmean,ANY-method(HARModel-class),10uncmean,HARForecast-method(HARForecast-class),9uncmean,HARModel-method(HARModel-class),10uncmean,HARSim-method(HARSim-class),11。
Long short-term memory

Long short-termmemoryA simple LSTM gate with only input,output,and forget gates. LSTM gates may have more gates.[1]Long short-term memory(LSTM)is a recurrent neural network(RNN)architecture(an artificial neural network) published[2]in1997by Sepp Hochreiter and Jürgen Schmidhuber.Like most RNNs,an LSTM network is universal in the sense that given enough network units it can compute anything a conventional computer can com-pute,provided it has the proper weight matrix,which may be viewed as its program.Unlike traditional RNNs,an LSTM network is well-suited to learn from experience to classify,process and predict time series when there are very long time lags of unknown size between important events.This is one of the main reasons why LSTM out-performs alternative RNNs and Hidden Markov Models and other sequence learning methods in numerous appli-cations.For example,LSTM achieved the best known results in unsegmented connected handwriting recogni-tion,[3]and in2009won the ICDAR handwriting compe-tition.LSTM networks have also been used for automatic speech recognition,and were a major component of a net-work that in2013achieved a record17.7%phoneme er-ror rate on the classic TIMIT natural speech dataset.[4] 1ArchitectureAn LSTM network is an artificial neural network that contains LSTM blocks instead of,or in addition to,regu-lar network units.An LSTM block may be described as a“smart”network unit that can remember a value for an arbitrary length of time.An LSTM block contains gates that determine when the input is significant enough to re-member,when it should continue to remember or forget the value,and when it should output the value.A typical implementation of an LSTM block is shown to the right.The four units shown at the bottom of thefig-A typical implementation of an LSTM block.ure are sigmoid units y=s(∑w i x i),where s is some squashing function,such as the logistic function.The left-most of these units computes a value which is condition-ally fed as an input value to the block’s memory.The other three units serve as gates to determine when values are allowed toflow into or out of the block’s memory.The second unit from the left(on the bottom row)is the“in-put gate”.When it outputs a value close to zero,it zeros out the value from the left-most unit,effectively blocking that value from entering into the next layer.The third unit from the left is the“forget gate”.When it outputs a value close to zero,the block will effectively forget whatever value it was remembering.The right-most unit(on the bottom row)is the“output gate”.It determines when the unit should output the value in its memory.The units con-taining theΠsymbol compute the product of their inputs (y=Πx i).These units have no weights.The unit with theΣsymbol computes a linear function of its inputs( y=∑w i x i).The output of this unit is not squashed so that it can remember the same value for many time-steps without the value decaying.This value is fed back in so that the block can“remember”it(as long as the forget gate allows).Typically,this value is also fed into the3 gating units to help them make gating decisions.125REFERENCES2TrainingTo minimize LSTM’s total error on a set of train-ing sequences,iterative gradient descent such as backpropagation through time can be used to change each weight in proportion to its derivative with respect to the error.A major problem with gradient descent for stan-dard RNNs is that error gradients vanish exponentially quickly with the size of the time lag between important events,asfirst realized in1991.[5][6]With LSTM blocks, however,when error values are back-propagated from the output,the error becomes trapped in the memory portion of the block.This is referred to as an“error carousel”, which continuously feeds error back to each of the gates until they become trained to cut offthe value.Thus,reg-ular backpropagation is effective at training an LSTM block to remember values for very long durations. LSTM can also be trained by a combination of artificial evolution for weights to the hidden units,and pseudo-inverse or support vector machines for weights to the out-put units.[7]In reinforcement learning applications LSTM can be trained by policy gradient methods,evolution strategies or genetic algorithms.3ApplicationsApplications of LSTM include:•Robot control[8]•Time series prediction[9]•Speech recognition[10][11][12]•Rhythm learning[13]•Music composition[14]•Grammar learning[15][16][17]•Handwriting recognition[18][19]•Human action recognition[20]•Protein Homology Detection[21]4See also•Artificial neural network•Prefrontal Cortex Basal Ganglia Working Memory (PBWM)•Recurrent neural network•Time series•Long-term potentiation 5References[1]Klaus Greff,Rupesh Kumar Srivastava,Jan Koutník,BasR.Steunebrink,Jürgen Schmidhuber(2015).“LSTM:A Search Space Odyssey”.arXiv:1503.04069.[2]Sepp Hochreiter and Jürgen Schmidhuber(1997).“Longshort-term memory”(PDF).Neural Computation9(8): 1735–1780.doi:10.1162/neco.1997.9.8.1735.PMID 9377276.[3] A.Graves,M.Liwicki,S.Fernandez,R.Bertolami,H.Bunke,J.Schmidhuber.A Novel Connectionist System for Improved Unconstrained Handwriting Recognition.IEEE Transactions on Pattern Analysis and Machine In-telligence,vol.31,no.5,2009.[4]Graves,Alex;Mohamed,Abdel-rahman;Hinton,Geof-frey(2013).“Speech Recognition with Deep Recurrent Neural Networks”.Acoustics,Speech and Signal Pro-cessing(ICASSP),2013IEEE International Conference on: 6645–6649.[5]S.Hochreiter.Untersuchungen zu dynamischen neu-ronalen Netzen.Diploma thesis,Institut rmatik, Technische Univ.Munich,1991.[6]S.Hochreiter,Y.Bengio,P.Frasconi,and J.Schmid-huber.Gradientflow in recurrent nets:the difficulty of learning long-term dependencies.In S.C.Kremer and J.F.Kolen,editors,A Field Guide to Dynamical RecurrentNeural Networks.IEEE Press,2001.[7]Schmidhuber,J.;Wierstra, D.;Gagliolo,M.;Gomez, F.(2007).“Training Recurrent Networks by Evolino”.Neural Computation19(3):757–779.doi:10.1162/neco.2007.19.3.757.[8]H.Mayer,F.Gomez,D.Wierstra,I.Nagy,A.Knoll,andJ.Schmidhuber.A System for Robotic Heart Surgery that Learns to Tie Knots Using Recurrent Neural Networks.Advanced Robotics,22/13–14,pp.1521–1537,2008. [9]J.Schmidhuber and D.Wierstra and F.J.Gomez.Evolino:Hybrid Neuroevolution/Optimal Linear Search for Sequence Learning.Proceedings of the19th Interna-tional Joint Conference on Artificial Intelligence(IJCAI), Edinburgh,pp.853–858,2005.[10]Graves, A.;Schmidhuber,J.(2005).“Framewisephoneme classification with bidirectional LSTM and other neural network architectures”.Neural Networks18(5–6): 602–610.doi:10.1016/j.neunet.2005.06.042.[11]S.Fernandez,A.Graves,J.Schmidhuber.An applica-tion of recurrent neural networks to discriminative key-word spotting.Intl.Conf.on Artificial Neural Networks ICANN'07,2007.[12]Graves,Alex;Mohamed,Abdel-rahman;Hinton,Geof-frey(2013).“Speech Recognition with Deep Recurrent Neural Networks”.Acoustics,Speech and Signal Pro-cessing(ICASSP),2013IEEE International Conference on: 6645–6649.3[13]Gers, F.;Schraudolph,N.;Schmidhuber,J.(2002).“Learning precise timing with LSTM recurrent net-works”.Journal of Machine Learning Research3:115–143.[14] D.Eck and J.Schmidhuber.Learning The Long-TermStructure of the Blues.In J.Dorronsoro,ed.,Proceedings of Int.Conf.on Artificial Neural Networks ICANN'02, Madrid,pages284–289,Springer,Berlin,2002.[15]Schmidhuber,J.;Gers, F.;Eck, D.;Schmidhu-ber,J.;Gers, F.(2002).“Learning nonregular lan-guages:A comparison of simple recurrent networks and LSTM”.Neural Computation14(9):2039–2041.doi:10.1162/089976602320263980.[16]Gers,F.A.;Schmidhuber,J.(2001).“LSTM RecurrentNetworks Learn Simple Context Free and Context Sensi-tive Languages”.IEEE Transactions on Neural Networks 12(6):1333–1340.doi:10.1109/72.963769.[17]Perez-Ortiz,J.A.;Gers, F.A.;Eck, D.;Schmidhu-ber,J.(2003).“Kalmanfilters improve LSTM net-work performance in problems unsolvable by traditional recurrent nets”.Neural Networks16(2):241–250.doi:10.1016/s0893-6080(02)00219-8.[18] A.Graves,J.Schmidhuber.Offline Handwriting Recog-nition with Multidimensional Recurrent Neural Networks.Advances in Neural Information Processing Systems22, NIPS'22,pp545–552,Vancouver,MIT Press,2009. [19] A.Graves,S.Fernandez,M.Liwicki,H.Bunke,J.Schmidhuber.Unconstrained online handwriting recog-nition with recurrent neural networks.Advances in Neu-ral Information Processing Systems21,NIPS'21,pp577–584,2008,MIT Press,Cambridge,MA,2008.[20]M.Baccouche, F.Mamalet,C Wolf, C.Garcia, A.Baskurt.Sequential Deep Learning for Human Action Recognition.2nd International Workshop on Human Be-havior Understanding(HBU),A.A.Salah,B.Lepri ed.Amsterdam,Netherlands.pp.29–39.Lecture Notes in Computer Science7065.Springer.2011[21]Hochreiter,S.;Heusel,M.;Obermayer,K.(2007).“Fast model-based protein homology detection with-out alignment”.Bioinformatics23(14):1728–1736.doi:10.1093/bioinformatics/btm247.PMID17488755. 6External links•Recurrent Neural Networks with over30LSTM pa-pers by Jürgen Schmidhuber's group at IDSIA •Gers PhD thesis on LSTM networks.•Fraud detection paper with two chapters devoted to explaining recurrent neural networks,especially LSTM.•Paper on a high-performing extension of LSTM that has been simplified to a single node type and can train arbitrary architectures.•Tutorial:How to implement LSTM in python with theano47TEXT AND IMAGE SOURCES,CONTRIBUTORS,AND LICENSES 7Text and image sources,contributors,and licenses7.1Text•Long short-term memory Source:https:///wiki/Long_short-term_memory?oldid=720271917Contributors:Fnielsen, Michael Hardy,Glenn,Rich Farmbrough,Denoir,Woohookitty,Rjwilmsi,Tony1,SmackBot,Derek farn,Ninjakannon,Magioladitis, Barkeep,Pwoolf,Headlessplatter,M4gnum0n,Muhandes,Jncraton,Yobot,Dithridge,Richard.decal,Omnipaedista,Valdemus,Olexa Riznyk,Albertzeyer,BiObserver,Silenceisgod,Epsiloner,Ego White Tray,Mister Mormon,Dexbot,Hmainsbot1,Mogism,Velvel2, Mpritham,Thoreyrunars and Anonymous:187.2Images•File:Long_Short_Term_Memory.png Source:https:///wikipedia/commons/d/d5/Long_Short_Term_Memory.png License:CC BY-SA4.0Contributors:Own work Original artist:BiObserver•File:Lstm_block.svg Source:https:///wikipedia/commons/8/8d/Lstm_block.svg License:Public domain Contrib-utors:(Original text:Headlessplatter(talk)(Uploads)-I made this image myself and I gift it to the public domain.)Original artist: Headlessplatter(talk)(Uploads)7.3Content license•Creative Commons Attribution-Share Alike3.0。
- 1、下载文档前请自行甄别文档内容的完整性,平台不提供额外的编辑、内容补充、找答案等附加服务。
- 2、"仅部分预览"的文档,不可在线预览部分如存在完整性等问题,可反馈申请退款(可完整预览的文档不适用该条件!)。
- 3、如文档侵犯您的权益,请联系客服反馈,我们会尽快为您处理(人工客服工作时间:9:00-18:30)。
Fulvio Corsi
1
November 2002 This version: 18th August 2004
Abstract
In the present work we propose a new realized volatility model to directly model and forecast the time series behavior of volatility. The purpose is to obtain a conditional volatility model based on realized volatility which is able to reproduce the memory persistence observed in the data but, at the same time, remains parsimonious and easy to estimate. Inspired by the Heterogeneous Market Hypothesis and the asymmetric propagation of volatility between long and short time horizons, we propose an additive cascade of different volatility components generated by the actions of different types of market participants. This additive volatility cascade leads to a simple AR-type model in the realized volatility with the feature of considering volatilities realized over different time horizons. We term this model, Heterogeneous Autoregressive model of the Realized Volatility (HAR-RV). In spite of the simplicity of its structure, simulation results seem to confirm that the HAR-RV model successfully achieves the purpose of reproducing the main empirical features of financial data (long memory, fat tail, self-similarity) in a very simple and parsimonious way. Preliminary results on the estimation and forecast of the HAR-RV model on USD/CHF data, show remarkably good out of sample forecasting performance which steadily and substantially outperforms those of standard models.
Institute of Finance, University of Lugano, Via Buffi 13, CH-6904 - Lugano, Switzerland. National Centre of Competence in Research ”Financial Valuation and Risk Management” (NCCR - FINRISK) supported by the Swiss National Science Foundation. E-mail: fulvio.corsi@lu.unisi.ch The author would like to gratefully acknowledges Michel Dacorogna, Ulrich M¨ uller, Gilles Zumbach, Paul Lynch, Giovanni Barone-Adesi, Patrick Gagliardini and Loriano Mancini for insightful discussions and the Olsen Group (www.olsen.ch) for providing thespite volatility is one of the prevailing features of financial markets, it is still an ambiguous term for which there is no unique, universally accepted definition. So far most of the studies have considered volatility as an unobservable variable and therefore used a fully specified conditional mean and conditional variance model to estimate and analyze that latent volatility. Modelling the unobserved conditional variance was one of the most prolific topics in the financial literature which led to all ARCH-GARCH developments and stochastic volatility models. In general this kind of models suffer a twofold weakness: first, they are not able to replicate main empirical features of financial data; second, the estimation procedure required are often non trivial (especially in the case of stochastic volatility models). An alternative approach is to construct an observable proxy for the latent volatility by using intraday high frequency data. This proxy has recently been labelled Realized Volatility by Andersen, Bollerslev, Diebold and Labys (2001). In the present work we will employ the high frequency realized volatility estimators developed in Zumbach, Corsi and Trapletti (2002) to directly analyze, model and forecast the time series behavior of FX volatility. The final purpose is to obtain a conditional volatility model based on realized volatility which is able to account for all the main empirical features observed in the data and, at the same time, which remains very parsimonious and easy to estimate. Inspired by the Heterogeneous Market Hypothesis (M¨ uller et al. 1993) which led to the HARCH model of M¨ uller et al. (1997) and Dacorogna et al. (1998) and by the asymmetric propagation of volatility between long and short time horizons, we propose an additive cascade model of different volatility components each of which generated by the actions of different types of market participants. This additive volatility cascade leads to a simple AR-type model in the realized volatility with the feature of considering volatilities realized over different time horizons. We thus term this model, Heterogeneous Autoregressive model of Realized Volatility (HAR-RV). Surprisingly, in spite of its simplicity and the fact that it does not formally belong to the class of long memory models, the HAR-RV model is able to reproduce the same memory persistence observed in volatility as well as many of the other main stylized facts of financial data. The rest of the paper is organized as follows. Section 2 briefly reviews the notion of realized volatility, introducing our notation and discussing the empirical issues related to its practical implementation. Section 3 describes the data set employed in the study and reviews the general stylized facts of FX data. Section 4 describes the motivations and derivation of the HAR-RV model. Section 5 shows the properties of the simulated HAR-RV series while section 6 describes the estimation and forecast results of the model for the twelve years USD/CHF series. Section 7 concludes.