The International Technology Roadmap for Semiconductors, 2000 Update, Lithography Module. h
集成电路封装基板工艺
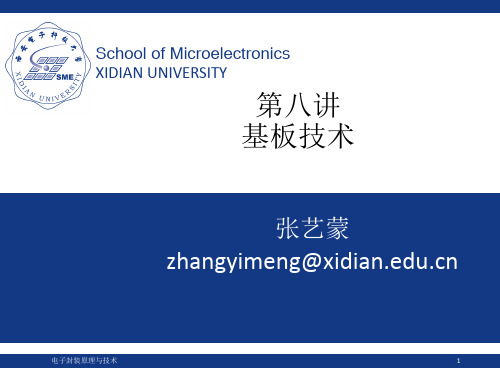
ITRS的描述
• The invention of build-up technology introduced redistribution layers on top of cores. While the build-up layers employed fine line technology and blind vias, the cores essentially continued to use printed wiring board technology with shrinking hole diameters. • The next step in the evolution of substrates was to develop high density cores where via diameters were shrunk to the same scale as the blind vias i.e. 50 μm. • The full advantage of the dense core technology is realized when lines and spaces are reduced to 25 μm or less. Thin photo resists (<15 μm) and high adhesion, low profile copper foils are essential to achieve such resolution. • In parallel coreless substrate technologies are being developed. One of the more common approaches is to form vias in a sheet of dielectric material and to fill the vias with a metal paste to form the basic building block.The dielectric materials have little or no reinforcing material. Control of dimensional stability during processing will be essential.
以前瞻性技术预见等战略分析工具支撑关键核心技术的战略突破集成电路领域案例
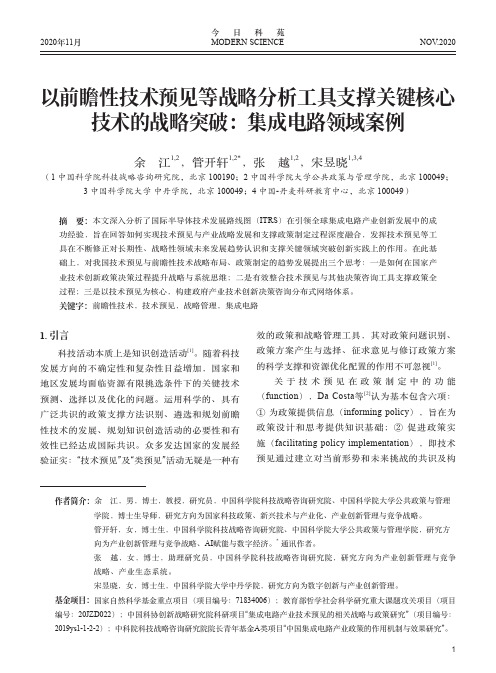
12020年11月NOV .2020今日科苑MODERN SCIENCE1. 引言科技活动本质上是知识创造活动[1]。
随着科技发展方向的不确定性和复杂性日益增加,国家和地区发展均面临资源有限挑选条件下的关键技术预测、选择以及优化的问题。
运用科学的、具有广泛共识的政策支撑方法识别、遴选和规划前瞻性技术的发展、规划知识创造活动的必要性和有效性已经达成国际共识。
众多发达国家的发展经验证实:“技术预见”及“类预见”活动无疑是一种有效的政策和战略管理工具,其对政策问题识别、政策方案产生与选择、征求意见与修订政策方案的科学支撑和资源优化配置的作用不可忽视[1]。
关于技术预见在政策制定中的功能(function ),Da Costa 等[2]认为基本包含六项:① 为政策提供信息(informing policy ),旨在为政策设计和思考提供知识基础;② 促进政策实施(facilitating policy implementation ),即技术预见通过建立对当前形势和未来挑战的共识及构摘 要:本文深入分析了国际半导体技术发展路线图(ITRS )在引领全球集成电路产业创新发展中的成功经验,旨在回答如何实现技术预见与产业战略发展和支撑政策制定过程深度融合,发挥技术预见等工具在不断修正对长期性、战略性领域未来发展趋势认识和支撑关键领域突破创新实践上的作用。
在此基础上,对我国技术预见与前瞻性技术战略布局、政策制定的趋势发展提出三个思考:一是如何在国家产业技术创新政策决策过程提升战略与系统思维;二是有效整合技术预见与其他决策咨询工具支撑政策全过程;三是以技术预见为核心,构建政府产业技术创新决策咨询分布式网络体系。
关键字:前瞻性技术,技术预见,战略管理,集成电路以前瞻性技术预见等战略分析工具支撑关键核心技术的战略突破:集成电路领域案例余 江1,2,管开轩1,2*,张 越1,2,宋昱晓1,3,4(1 中国科学院科技战略咨询研究院,北京 100190;2 中国科学院大学公共政策与管理学院,北京 100049;3 中国科学院大学 中丹学院,北京 100049;4 中国-丹麦科研教育中心,北京 100049)作者简介:余 江,男,博士,教授,研究员,中国科学院科技战略咨询研究院、中国科学院大学公共政策与管理 学院,博士生导师,研究方向为国家科技政策、新兴技术与产业化、产业创新管理与竞争战略。
PARTICLEADHESIONANDREMOVALINPOST-CMPAPPLICATIONS

dry 55%RH wet 55%RH wet 100%RH
70
60
50
40
30
20
10
0
0
0.2
0.4
0.6
0.8
1
1.2
1.4
1.6
1.8
2
Moment Ratio
Microcontamination Research Lab
RESULTS
Aging Effect on Glass Particle Removal from FPD
Removal Efficiency
u To understand and determine the onset of large adhesion forces after polishing such as the development of covalent bonds.
u Study the removal and adhesion forces for alumina and silica slurry particles from silicon wafers (with different films, TOX, W, Cu, TaN, BPSG, etc.).
u The contaminant particle touches the wafer at one point of contact
u Short range van der Waals force dominates near the surface and the contact area increases.
RESULTS
Imprints on the glass surface ( megasonic cleaned after aging)
技术路线图(TechnologyRoadmap)
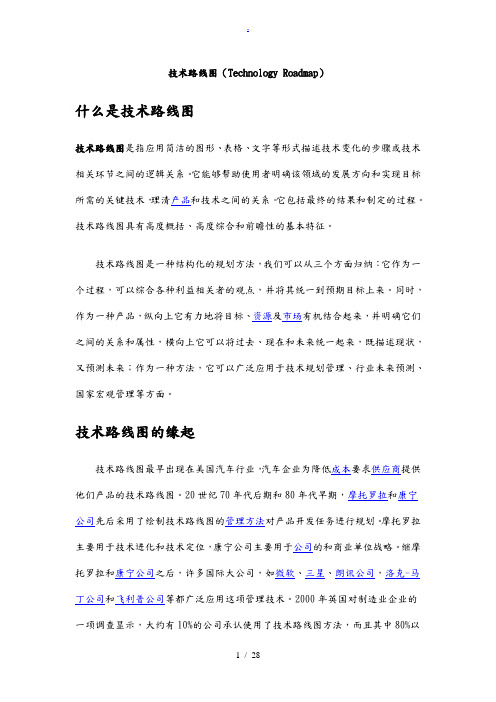
技术路线图(Technology Roadmap)什么是技术路线图技术路线图是指应用简洁的图形、表格、文字等形式描述技术变化的步骤或技术相关环节之间的逻辑关系。
它能够帮助使用者明确该领域的发展方向和实现目标所需的关键技术,理清产品和技术之间的关系。
它包括最终的结果和制定的过程。
技术路线图具有高度概括、高度综合和前瞻性的基本特征。
技术路线图是一种结构化的规划方法,我们可以从三个方面归纳:它作为一个过程,可以综合各种利益相关者的观点,并将其统一到预期目标上来。
同时,作为一种产品,纵向上它有力地将目标、资源及市场有机结合起来,并明确它们之间的关系和属性,横向上它可以将过去、现在和未来统一起来,既描述现状,又预测未来;作为一种方法,它可以广泛应用于技术规划管理、行业未来预测、国家宏观管理等方面。
技术路线图的缘起技术路线图最早出现在美国汽车行业,汽车企业为降低成本要求供应商提供他们产品的技术路线图。
20世纪70年代后期和80年代早期,摩托罗拉和康宁公司先后采用了绘制技术路线图的管理方法对产品开发任务进行规划。
摩托罗拉主要用于技术进化和技术定位,康宁公司主要用于公司的和商业单位战略。
继摩托罗拉和康宁公司之后,许多国际大公司,如微软、三星、朗讯公司,洛克-马丁公司和飞利普公司等都广泛应用这项管理技术。
2000年英国对制造业企业的一项调查显示,大约有10%的公司承认使用了技术路线图方法,而且其中80%以上用了不止一次(C.J.Farrukh, R.Phaal, 2001)[1]。
不仅如此,许多国家政府、产业团体和科研单位也开始利用这种方法来对其所属部门的技术进行规划和管理。
技术路线图真正的奠基人是摩托罗拉公司当时的CEO—Robert Galvin。
当时,Robert Galvin在全公司围发动了一场绘制技术路线图的行动,主要目的是鼓励业务经理适当地关注技术未来并为他们提供一个预测未来过程的工具。
这个工具为设计和研发工程师与做市场调研和营销的同事之间提供了交流的渠道,建立了各部门之间识别重要技术、传达重要技术的机制,使得技术为未来的产品开发和应用服务。
在如今的科技界,摩尔定律不管用了吗?
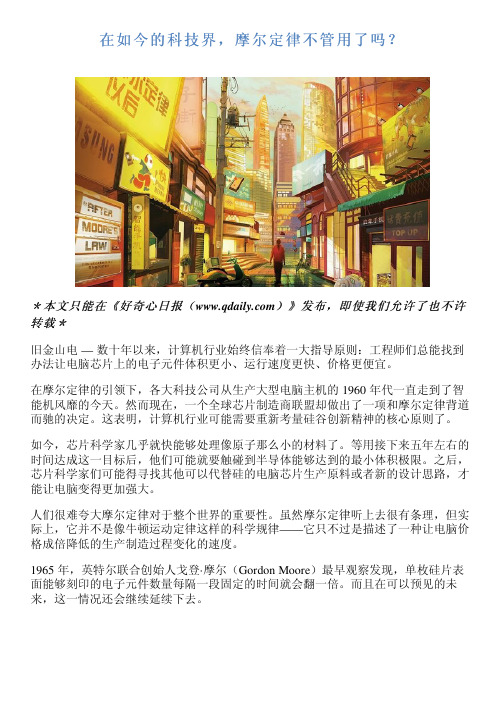
在如今的科技界,摩尔定律不管用了吗?本文只能在《好奇心日报(只能在《好奇心日报()》发布,即使我们允许了也不许*本文转载*旧金山电 — 数十年以来,计算机行业始终信奉着一大指导原则:工程师们总能找到办法让电脑芯片上的电子元件体积更小、运行速度更快、价格更便宜。
在摩尔定律的引领下,各大科技公司从生产大型电脑主机的 1960 年代一直走到了智能机风靡的今天。
然而现在,一个全球芯片制造商联盟却做出了一项和摩尔定律背道而驰的决定。
这表明,计算机行业可能需要重新考量硅谷创新精神的核心原则了。
如今,芯片科学家几乎就快能够处理像原子那么小的材料了。
等用接下来五年左右的时间达成这一目标后,他们可能就要触碰到半导体能够达到的最小体积极限。
之后,芯片科学家们可能得寻找其他可以代替硅的电脑芯片生产原料或者新的设计思路,才能让电脑变得更加强大。
人们很难夸大摩尔定律对于整个世界的重要性。
虽然摩尔定律听上去很有条理,但实际上,它并不是像牛顿运动定律这样的科学规律——它只不过是描述了一种让电脑价格成倍降低的生产制造过程变化的速度。
1965 年,英特尔联合创始人戈登·摩尔(Gordon Moore)最早观察发现,单枚硅片表面能够刻印的电子元件数量每隔一段固定的时间就会翻一倍。
而且在可以预见的未来,这一情况还会继续延续下去。
图片版权:Paul Sakuma / 美联社摩尔观察到这一现象的时候,电子元件最密集的存储芯片还只能容纳约 1000 比特的信息。
换言之:1980 年代,世界上最强大的超级计算机 Cray 2 的体积相当于一台工业用洗衣机,如果放在今天,这台设备造价将会超过 1500 万美元;与之形成鲜明对比的是,2011 年上市的 iPad 2 仅 400 美元,可以轻松放在膝头使用,但它却拥有比Cray2 更强的计算能力。
请注意,和更新的 iPad 设备相比,那款 iPad 2 运行速度已经算是慢的了。
如果没有过去非凡的进步,就不会有如今的计算机行业,Google、亚马逊等公司运营的云计算数据中心造价也将会贵得不可思议;你将无法使用智能机下载使用应用软件,利用应用软件打车回家、叫外卖;破译人类基因组、教会机器倾听等科学突破也不会出现。
MEMS微系统 复习红宝书(北理)

20.BGA : Ball Grid Array 球状矩阵排列
21.SHM:Structural Health Monitoring 结构健康监测
22.ICT:Information and Communications Technologies 信息与通信技术
23.MtM More than Moore 超越摩尔定律
24.FEA:Finite Element Analysis 有限元分析
25.SEM:Scanning Electron Microscope 扫描电子显微镜
12.ITRS International technology Roadmap for Semiconductor 国际半导体技术规
划
.
27.DARPA :Defence Advanced Research Projects Agency of theDepartment of
成,它们各具不同的能带隙。这些材料可以是 GaAs 之类的化合物,也可以是 Si-Ge 之类的半导体合金。按异质结中两种材料导带和价带的对准情况可以把异 质结分为Ⅰ型异质结和Ⅱ型异质结两种。 12.微加工:以微小切除量获得很高精度的尺寸和形状的加工。 13.引线键合:引线键合(Wire Bonding)是一种使用细金属线,利用热、压力、 超声波能量为使金属引线与基板焊盘紧密焊合,实现芯片与基板间的电气互连和 芯片间的信息互通。 14. 倒装芯片:倒装芯片(Flip chip)是一种无引脚结构,一般含有电路单元。 设 计用于通过适当数量的位于其面上的锡球(导电性粘合剂所覆盖),在电气上和 机械上连接于电路。 15.热声焊:热声焊是一种固态键合技术,为热压结合与超音波结合的混合方法。 它可完成电路片与芯片、腔体之间的电连接。 16.各向异性粘接:用各向异性导电胶(主要使用单一或双重成分的环氧树脂)完 成对电路基板与倒装芯片之间的互连。 17.柔性印刷电路:即 FPC,是以聚脂薄膜或聚酰亚胺为基材制成的一种具有高度 可靠性,绝佳曲挠性的印刷电路。通过在可弯曲的轻薄塑料片上,嵌入电路设计, 使在窄小和有限空间中堆嵌大量精密元件,从而形成可弯曲的挠性电路。 18.高深宽比:垂直于加工表面的高度与其加工表面上所具有的特征尺寸的比值 大。 19. 盲孔: 定义 1.位于印刷线路板的顶层和底层表面,具有一定深度,用于表层线路和下面 的内层线路的连接,孔的深度通常不超过一定的比率(孔径)。
一种Sigma-DeltaADC中抽取滤波器的研究
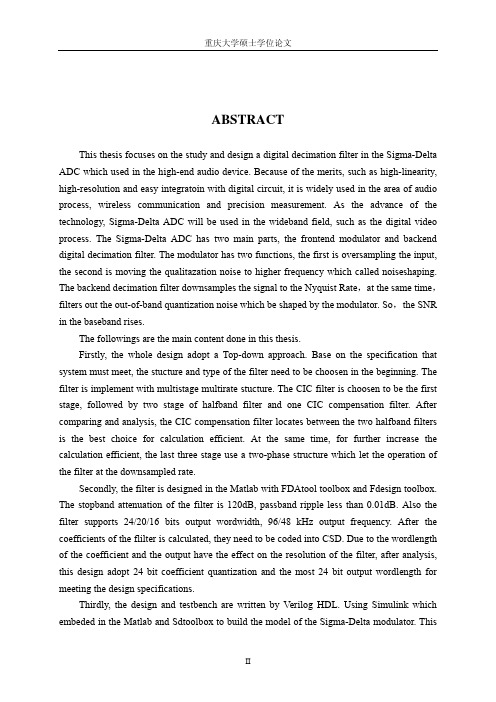
重庆大学硕士学位论文ABSTRACTThis thesis focuses on the study and design a digital decimation filter in the Sigma-Delta ADC which used in the high-end audio device. Because of the merits, such as high-linearity, high-resolution and easy integratoin with digital circuit, it is widely used in the area of audio process, wireless communication and precision measurement. As the advance of the technology, Sigma-Delta ADC will be used in the wideband field, such as the digital video process. The Sigma-Delta ADC has two main parts, the frontend modulator and backend digital decimation filter. The modulator has two functions, the first is oversampling the input, the second is moving the qualitazation noise to higher frequency which called noiseshaping. The backend decimation filter downsamples the signal to the Nyquist Rate,at the same time,filters out the out-of-band quantization noise which be shaped by the modulator. So,the SNR in the baseband rises.The followings are the main content done in this thesis.Firstly, the whole design adopt a Top-down approach. Base on the specification that system must meet, the stucture and type of the filter need to be choosen in the beginning. The filter is implement with multistage multirate stucture. The CIC filter is choosen to be the first stage, followed by two stage of halfband filter and one CIC compensation filter. After comparing and analysis, the CIC compensation filter locates between the two halfband filters is the best choice for calculation efficient. At the same time, for further increase the calculation efficient, the last three stage use a two-phase structure which let the operation of the filter at the downsampled rate.Secondly, the filter is designed in the Matlab with FDAtool toolbox and Fdesign toolbox. The stopband attenuation of the filter is 120dB, passband ripple less than 0.01dB. Also the filter supports 24/20/16 bits output wordwidth, 96/48 kHz output frequency. After the coefficients of the flilter is calculated, they need to be coded into CSD. Due to the wordlength of the coefficient and the output have the effect on the resolution of the filter, after analysis, this design adopt 24 bit coefficient quantization and the most 24 bit output wordlength for meeting the design specifications.Thirdly, the design and testbench are written by Verilog HDL. Using Simulink which embeded in the Matlab and Sdtoolbox to build the model of the Sigma-Delta modulator. Thismodel is used to generate the dataflow of output of the modulator which is used to simulate and validate the function of the filter in the Modelsim.Finally, after validation the code, the next step of the design is synthesis the Verilog HDL by Design Compiler to get the netlist. Then the layout of the design can be achieved by the Auto-Place-and-Route tool, Astro. The technology library in my design is 0.18 um standard cell library. The area of the chip is 1.7mm*1.7mm. As such design adopts the top-down design method, it has good capability of duplication and transplantation. The operation of digital filter is a pure DSP process, so it is suitable for the use of FPGA to implement the filter. At last, Quartus, a FPGA software, is used to simulate the implement of the filter in the FPGA.Keywords: Sigma-Delta ADC, CSD, Decimation filter, CIC filter1 绪论1.1 引言根据“国际半导体技术路线”(International Technology Roadmap for Semiconductor, ITRS)的报告,CMOS工艺的特征尺寸会在未来至少十年当中继续降低,到2013年将会达到32nm。
半导体发展史

前言自从有人类以来,已经过了上百万年的岁月。
社会的进步可以用当时人类使用的器物来代表,从远古的石器时代、到铜器,再进步到铁器时代。
现今,以硅为原料的电子元件产值,则超过了以钢为原料的产值,人类的历史因而正式进入了一个新的时代,也就是硅的时代。
硅所代表的正是半导体元件,包括记忆元件、微处理机、逻辑元件、光电元件与侦测器等等在内,举凡电视、电话、电脑、电冰箱、汽车,这些半导体元件无时无刻都在为我们服务。
硅是地壳中最常见的元素,许多石头的主要成分都是二氧化硅,然而,经过数百道制程做出的积体电路,其价值可达上万美金;把石头变成硅晶片的过程是一项点石成金的成就,也是近代科学的奇蹟!在日本,有人把半导体比喻为工业社会的稻米,是近代社会一日不可或缺的。
在国防上,惟有扎实的电子工业基础,才有强大的国防能力,1991年的波斯湾战争中,美国已经把新一代电子武器发挥得淋漓尽致。
从1970年代以来,美国与日本间发生多次贸易摩擦,但最后在许多项目美国都妥协了,但是为了半导体,双方均不肯轻易让步,最后两国政府慎重其事地签订了协议,足证对此事的重视程度,这是因为半导体工业发展的成败,关系着国家的命脉,不可不慎。
在台湾,半导体工业是新竹科学园区的主要支柱,半导体公司也是最赚钱的企业,台湾如果要成为明日的科技硅岛,半导体工业是我们必经的途径.半导体的起源在二十世纪的近代科学,特别是量子力学发展知道金属材料拥有良好的导电与导热特性,而陶瓷材料则否,性质出来之前,人们对于四周物体的认识仍然属于较为巨观的瞭解,那时已经介于这两者之间的,就是半导体材料。
英国科学家法拉第(MIChael Faraday,1791~1867),在电磁学方面拥有许多贡献,但较不为人所知的,则是他在1833年发现的其中一种半导体材料:硫化银,因为它的电阻随着温度上升而降低,当时只觉得这件事有些奇特,并没有激起太大的火花;然而,今天我们已经知道,随着温度的提升,晶格震动越厉害,使得电阻增加,但对半导体而言,温度上升使自由载子的浓度增加,反而有助于导电,这也是半导体一个非常重要的物理性质。
- 1、下载文档前请自行甄别文档内容的完整性,平台不提供额外的编辑、内容补充、找答案等附加服务。
- 2、"仅部分预览"的文档,不可在线预览部分如存在完整性等问题,可反馈申请退款(可完整预览的文档不适用该条件!)。
- 3、如文档侵犯您的权益,请联系客服反馈,我们会尽快为您处理(人工客服工作时间:9:00-18:30)。
COMPARISON OF SUPER VISED LEARNING METHODS FOR SPIKE TIME CODING IN SPIKING NEURAL NETWORKSANDRZEJ KASIŃSKI,FILIP PONULAKAbstract.In this review we focus our attention on the supervised learning methods for spiketime coding in Spiking Neural Networks(SNN).This study is motivated by the recent experi-mental results on information coding in the biological neural systems which suggest that precisetiming of individual spikes may be essential for efficient computation in the brain.We pose a fundamental question,what paradigms of neural temporal coding can be imple-mented with the recent learning methods.In order to answer this question we discuss various approaches to the considered learning task.We shortly describe the particular learning algorithms and report the results of experiments.Finally we discuss properties,assumptions and limitations of each method.We complete thisreview with a comprehensive list of pointers to the literature.1.IntroductionFor many years a common belief was that the essential information in neurons is encoded in theirfiring rates.However,recent neurophysiological results suggest that efficient processing of information in neural systems can be founded also on the precise timing of action potentials (spikes)([1,2,3]).In the barn owl auditory system,neurons detecting coincidence receive volleys of precisely timed spikes from both ears([4,5]).Under the influence of a common oscillatory drive in the rat hippocampus,the strength of a constant stimulus is coded in the relative timing of neuronal action potentials([6]).In humans precise timing offirst spikes in tactile afferents encodes touch signals at thefinger tips([1]).Time codes have also been suggested for rapid visual processing([1]).Precise temporal coding paradigm is required in some artificial control systems.Examples are neuroprosthetic systems which aim at producing a functionally useful movements of the paralysed limbs by exciting muscles or nerves with the sequences of short electrical impulses([7]).Precise relative timing of impulses is critical for generating the desired,smooth movement trajectories.In addition to aforementioned examples,it has been theoretically demonstrated that the tem-poral neural code is very efficient whenever the fast processing of information is required([8]).All these arguments provide strong motivation for investigating the computational properties of the systems that compute with precisely timed spikes.It is generally agreed that artificial Spiking Neural Networks(SNN)([4,9,10])are capable of exploiting time as a resource for coding and computation in a much more sophisticated manner than typical neural computational models([11,12]).SNNs appear to be an interesting tool for investigating the temporal neural coding and for exploiting its computational potential.Although significant progress has already been made to recognize information codes that can be beneficial for computation in SNN([4,9,11,13]),it is still an open problem to determine efficient neural learning mechanisms that enable implementation of these particular time-coding schemes.Unsupervised spike-based learning methods,such as LTP,LTD and STDP have already been widely investigated and described in the literature([14,15,16,17,18,19,20]).However,unsuper-vised approach is not suitable to the learning tasks that require an explicit goal definition.In this article we focus on supervised learning methods for precise spike timing in SNN.The goal of our study is to determine what paradigms of neural information coding can be implemented with the recent approaches.Date:27.10.2005.Key words and phrases.Supervised Learning,Spiking Neural Networks,Time Coding,Temporal Sequences of Spikes.The work was partially supported by the State Committee for Scientific Research,project1445/T11/2004/27.12ANDRZEJ KASIŃSKI,FILIP PONULAKFirst,we present the supervised learning methods for spike timing,which are known from the literature.We classify these methods to more general groups representing particular learning approaches and shortly describe each of the learning algorithms.Finally,we summarize main facts about the learning approaches and discuss their properties.2.Review of Learning MethodsIn this section we present some representative methods for supervised learning in SNN.For all these methods the common goal of learning can be stated as follows:Given a sequence of input spikes trains S in (t )and a sequence of the target output spikes S d (t ),find vector of synaptic weights w ,such that outputs of learning neurons S out (t )are close to S d (t ).2.1.Methods based on gradient evaluation.Learning in the traditional,artificial neural networks (ANN)is usually performed by gradient ascent techniques ([21]).However,explicit evaluation of gradient in SNN is infeasible due to discontinuous-in-time nature of spiking neurons.Indirect approaches or special simplifications must be assumed to deal with this problem.In ([22,23])Bohte and colleagues presented one of such approaches.Their method,called Spike-Prop ,is analogous to the backpropagation algorithm ([24])known from the traditional Artificial Neural Networks.The target of SpikeProp is to learn a set of desired firing times,denoted t d j ,at the postsynapticneurons j ∈J for a given set of input patterns S in (t ).Each neuron in a simulated network isallowed to fire only once during a single simulation cycle.The learning method is based on an explicit evaluation of the gradient of E =1/2 j (t d j −t out j )2with respect to the weights of each synaptic input to j (where t out j is an actual firing time of neuron j ).To overcome the discontinuous nature of spiking neurons,authors approximated the thresholding ly,it was assumed that for a small region around t =t out j ,thefunction V m j (t ),denoting the membrane potential of j ,could be linearly approximated.On thisassumption error-backpropagation equations were derived for a fully connected feedforward network with hidden layers.SpikeProp algorithm has been re-investigated in ([25,26,27,28]).It was found that the weight initialization is a critical factor for a good performance of the learning rule.In ([25])the weights were initialized with the values that led the network to the successful training in a similar number of iterations as in ([22]),but with large learning rates,although Bohte argued that the approxi-mation of the threshold function implies that only small learning rates can be used ([23]).Other experiments of Moore ([25])also provided evidence that negative weights could be allowed and still led to successful convergence,which was in contradiction to the conclusions of Bohte.Xin and Embrechts ([27])proposed a modification of the learning algorithm by including the momentum term in the weight update equation.It has been demonstrated that this modification significantly speeded up the convergence of SpikeP rop .In ([26])additional learning rules were introduced for the synaptic delays,time constants and for the neurons’thresholds.This resulted with smaller network topologies and also with faster algorithm convergence.Finally,Tiňo and Mills ([28])ex-tended SpikeProp to recurrent network topologies,to account for the temporal dependencies in the input stream.Neither the original SpikeProp method nor any of the proposed modifications enable learning of patterns composed of more than one spike per neuron.Properties of the SpikeProp method were demonstrated in a set of classification experiments.These included standard and interpolated XOR problem ([13]).SpikeProp authors encoded the input and output values by time delays,associating the analog values with the corresponding “earlier”or “later”firing times.In the interpolated XOR experiment the network could learn the presented input with an accuracy of the order of the algorithm integration time-step.The classification abilities of SpikeProp were also tested on a number of common benchmark datasets (the Iris dataset,the Wisconsin breast-cancer dataset and the Statlog Landsat dataset).For these problems the accuracy of SNN trained with SpikeProp was comparable to that of sig-moidal neural network.Moreover,in experiments on the real-world datasets,the SpikeProp algo-rithm always converged,whereas the compared ANN algorithms,such as Levenberg–Marquardt algorithm,occasionally failed.The main drawback of the SpikeProp method is that there is no mechanism to “prop-up”the synaptic weights once the postsynaptic neuron no longer fires for any input pattern.LEARNING SPIKE TIMING IN SNN3 Moreover,in the SpikeProp approach only thefirst spike produced by a neuron is relevant and the rest of the time course of the neuron is ignored.Whenever a neuronfires a single spike,it is not allowed tofire again.For this reason the method cannot learn patterns consisting of multiple spikes.Thus it is suitable to implement only on the’time-to-first-spike’coding scheme([1]).2.2.Statistical methods.In([29,30]),authors proposed to derive a supervised spike-based learning algorithm starting with statistical learning criteria.Their method is based on the approach proposed by Barber.However,in([31])the author considered supervised learning for neurons operating on the discrete time scale.Pfister and colleagues extended this study to the continuous case.The fundamental hypothesis in([29])and([30])is to assume that the instantaneousfiring rate of the postsynaptic neuron j is determined by a point process with time dependent stochastic intensityρj(t)=g(V m j(t))that depends nonlinearly upon the membrane potential V m j(t).The firing rateρj(t)is known as escape rate([4]).The goal of the considered learning rule is to optimise the weights w j in order to maximise thelikelihood of getting postsynapticfiring times S outj (t)=S d j(t),given thefiring rateρj(t).Theoptimisation is performed via gradient ascent of the likelihood of the postsynapticfiring for one or several desiredfiring times.The advantage of the discussed probabilistic approach is that it allows to describe explicitly the likelihood P j S out j(t)|S in j(t) of emitting a S out j(t)for a given input S in j(t).Moreover,since this likelihood is a smooth function of its parameters,it is straightforward to differentiate it with respect to the synaptic efficacies w j.On the basis of this remark authors proposed a rule of the synaptic weights modifications that can be described by a two-phase learning window similar to that of Spike-Timing Dependent Plas-ticity(STDP)([19,20]).Authors demonstrated that the shape of the learning window was strongly influenced by the constraints imposed by the different scenarios of the optimization procedure.The described learning rule applies to all synaptic inputs of the learning neuron.It is also assumed that the postsynaptic neuron j receives additional’teaching’input I(t)that could either arise from a second group of neurons or from the intracellular current injection.The role of I(t) is to increase the probability that the neuronfires at or close to the desiredfiring time t d j.In this context the learning mechanism can also be viewed as a probabilistic version of the spike-based Supervised-Hebbian learning(described in section2.6).In([30])authors present a set of experiments which differ in the stimulation mode and the specific tasks of the learning neuron.The algorithm is applied to the spike response model(SRM) with escape noise as a generative model of neuron([4]).Authors consider different scenarios of the experiments:•different sources of’teaching’signal(the signal is given by a supervisor as a train of spikes or as a strong current pulse of short duration);•allowing(or not)for other postsynaptic spikes to be generated spontaneously;•implementing a temporal coding scheme where the postsynaptic neuron responds to one of the presynaptic spike patterns with a desired output spike train containing several spikes while staying inactive for the other presynaptic spike patterns.The experiments demonstrate the ability of the learning method to precisely set the time of the singlefirings at the neuron output.However,since in all experiments a desired postsynaptic spike train consisted of at most2spikes,it is hard to estimate a potential,practical suitability of the proposed method to learn complex spike trains consisting of dozens of spikes.2.3.Linear algebra methods.Carnell and Richardson proposed to apply linear algebra appa-ratus to the task of spike-time learning([32]).Authors begin with the definitions of the inner product,orthogonality and projection operations for the time series of spikes.They also introduce a specific metrics(norm),as a measure of the difference between two given time series.On the basis of these definitions authors formulate some algorithms for the approximation of the target pattern S d(t)given a set of input patterns S in(t)and a set of adjustable synaptic weights w:4ANDRZEJ KASIŃSKI,FILIP PONULAK(1)Gram-Schmidt solution:the Gram-Schmidt process([33,34])is used tofind an orthogonalbasis for the subspace spanned by a set of input time series S in(t).Having the orthogonal basis,the best approximation in the subspace to any given element of S d(t)can be found.(2)Iterative solution:the projection of error E onto direction of times series S in i is evaluated,with i randomly chosen in each iteration.Error is defined as a difference between the target and the actual time series E=S d(t)−S out(t).The algorithm is evaluated until norm(E)is sufficiently small.Authors demonstrated in a set of experiments that the iterative algorithm is able to approximate the target time series of spikes.The experiments were performed with the Liquid State Machine (LSM)network architecture([35,36])and LIF neurons([4]).Only an output neuron was subjected to learning.The approximated spike trains consisted of10spikes(spanned within a1second interval).In the successful training case,an input vector S in(t)was generated by500neurons. Good approximation of S d(t)was obtained after about600iterations.The presented results revealed that the ability of the method to produce the desired target patterns is strongly influenced by the number and variability of spikes in S in(t)and that the quality of approximation increased for longer sequences of spikes.This is a common conclusion for all LSM systems.As afinal remark,we state that the presented algorithm([32])is one out of only few algorithms that enable learning the patterns consisting of multiple spikes.However the algorithm updates weights in a batch mode and for this reason it is not suitable for the online learning.In some applications this can be considered as a drawback.2.4.Evolutionary methods.In([37]),authors investigate the viability of evolutionary strategies (ES)for supervised learning in spiking neural networks.The use of an evolutionary strategy is motivated by emphasising the ability of ES to work on real numbers without complex binary encoding schemes.ES proved to be well suited for solving continuous optimisation problems([38]).Unlike genetic algorithms,the primary search operator in ES is the mutation.A number of different mutation operators have been proposed.The traditional mutation operator adds to the alleles of the genes in the population some random value generated according to Gaussian distribution.Other mutation operators include the use of Cauchy distribution.The use of Cauchy distribution allows exploration of the search space by making large mutations and helping to prevent premature convergence.On the other hand the use of Gaussian mutation allows to exploit the best solutions found in a local search.In this algorithm,not only the synaptic strengths,but also the synaptic delays are the adjustable parameters.The spiking network is mapped to a vector of real values,which consists of the weights and delays of the synapses.A set of such vectors(individuals)will form the population evolving according to the ES.The population is expected to converge to a globally optimal network,tuned to the particular input patterns.The learning properties of the algorithm were tested in a set of classification tasks with XOR and Iris benchmark dataset.The SRM neuron models and the feed-forward fully connected spiking networks have been used.Similarly to([22])the analog values have been mapped here intofiring delays.Authors reported results comparable to those obtained with known classification algorithms (BP,LM,SpikeProp).Some limitation of the algorithm arises from the fact that each neuron is allowed to generate at most a single spike during the simulation time.Therefore the method is not suitable to learn pat-terns consisting of multiple spikes.Another disadvantage,common to all evolutionary algorithms, is that the computation with this approach is very time consuming.2.5.Learning in Synfire Chains.Human learning often involves relating two signals separated in time,or linking a signal,an action and a subsequent effect into a causal relationship.These events are often separated in time,but nonetheless,humans can link them,thereby allowing them to accurately predict the right moment for a particular action.Synfire chains(SFC)are considered as a possible mechanism for representing such relations between delayed events.SFC is a feedforward multi-layered architecture(a chain),in which spiking activity can propagate in a synchronous wave of neuronalfiring(a pulse packet)from one layer of the chain to the successive ones([39]). Each step in the SFC requires a pool of neurons whosefirings simultaneously raise the potentialLEARNING SPIKE TIMING IN SNN5 of the next pool of neurons to thefiring level.In this mechanism each cell of the chainfires only once.In([40]),a specific neural architecture-INFERNET is introduced.The architecture is an instance of the SFC.Its structure is organized into clusters of nodes called subnets.Each subnet is fully connected.Some subnet nodes have connections to external subnet nodes.The nodes are represented here by a simple model similar to SRM([4]).The learning task is to reproduce the temporal relation between two successive inputs(thefirst one-presented to thefirst layer of SFC and the latter one,considered as the’teaching’signal, given to the last layer).Thus the task is tofind a link between thefiring input nodes and the firing target nodes with a target time delay.Two successive inputs can be separated by several tenths of a second and a single connection cannot alone be responsible for such long delays.Therefore a long chain of successive pools of node firings might be required.In the reported approach the particular synaptic connections are modified by the rule similar to STDP,however with the additional non-Hebbian term.In our opinion this implies that the synaptic weights between the particular neurons must be strong enough to ensure that the wave of excitation will eventually reach the output subnet.This is the necessary condition which guaranties that the Hebbian rules would be activated.In([40]),author discussed experiments in which two inputs are presented,one(the probe) at time0ms and one(the target)some time later.The task for the network was to correctly reproduce the temporal association between these two inputs and therefore build an SFC between them.While trained,the network was able to trigger this synfire chain whenever thefirst input was presented.In this task author reported some difficulties.The algorithm could correctly reinforce a connection that led to the probe nodefiring at the right time,but could not in general prevent the target nodes fromfiring earlier,if some other’inter-nodes’fired several times before.Indeed,a careful analysis of the learning equations confirms that there is no rule for avoiding spuriousfiring.We conclude that the learning method under consideration represents an interesting approach to spike-time learning problem in SNN.In this method it is assumed that the time of postsynaptic neuronfiring depends mostly on the signal propagation delay in the presynatpic neurons.The ’time-weight’dependence is neglected.The author focuses on modifying the topology of the net-work,to obtain the desired delay between the signal delivered to the network input and the signal generated at the network output.However,with this approach the objective function(the desired time delay)is not a continuous function of the parameters(synaptic weights)of the optimization algorithm.For this reason the algorithm can be considered as a discrete optimization technique.This approach enables to attain the precision that takes values not from the continuous domain,but from afinite set of possible solutions(since global delay is a combination of thefixed component delays,constituting afinite set).The quality of approximation depends in general on the number and diversity of connection delays.Another limitation of the method is,again,that it can learn only singlefiring times and thus can be applied only to the’time-to-first-spike’coding scheme.Author claims that the method enables to learn sequentially many synfire chains.This property would be very interesting in the context of the real-life applications.Unfortunately,it is not described in the cited article,how this multi-learning can be achieved.2.6.Spike-based supervised-Hebbian learning.In this subsection we discuss methods that represent,so called,Supervised-Hebbian Learning(SHL)approach.In this approach Hebbian processes[41]are supervised by an additional’teaching’signal that reinforces the postsynaptic neuron tofire at the target times.The’teaching’signal can be transmitted to the neuron in a form of the synaptic currents or as the intracellularly injected currents.Ruf and Schmitt[42]proposed one of thefirst spike-based methods similar to SHL approach. In theirfirst attempt,they have defined the learning rule for the monosynaptic excitation.The learning process was based on three spikes(two presynaptic and one postsynaptic)generated during each learning cycle.Thefirst presynaptic spike at time t in1was considered as an input signal,whereas the second presynatpic spikes at t in2=t d pointed to the targetfiring time for the postsynaptic neuron.The learning rule reads:∆w=η(t out−t d),whereη>0is the learning rate6ANDRZEJ KASIŃSKI,FILIP PONULAKand t out is the actual time of the postsynaptic spike.This learning rule was applied after every learning cycle.It is easy to demonstrate that under certain conditions t out converges to t d.With this method it was possible to train only a single synaptic input,whereas neurons usually receive their inputs from several presynaptic neurons.The corresponding synaptic weights could still be learned in the way described above,if the weights were learned sequentially(a single synapse per learning cycle).This is,however,a very inefficient approach.As a solution to this problem authors proposed a parallel algorithm.Surprisingly,although this algorithm is considered as an extension to the monosynaptic rule,yet it does not aim at achieving the desired timing of the postsynaptic neuron.Instead,the goal is to modify synaptic weights to approach some target weight vector w d given by the difference between pre-and postsynapticfiring times,that is w d i=(t d−t in i)for any presynaptic neuron i.Authors claim that such an approach can be useful in the temporal pattern analysis in SNN,however no details are given to explain it.Thorough analysis of the Supervised-Hebbian learning in the context of spiking neurons was performed by Legenstein,Naeger and Maass([43]).The learning method,considered by authors,implements STDP process with supervision realised by the extra input currents injected to the learning neuron.These currents forced the learning neuron tofire at the target points in time and prevented it fromfiring at other times.Authors investigated the suitability of this approach to learn any given transformation of input to output spiking sequences.It is well-known that the common version of STDP always produces bimodal distribution of weights,where each weight either assumes its minimal or its maximal possible value.Therefore in this article authors considered mostly the target transformations that could be implemented with such bimodal distribution of weights.Authors reported a set of experiments in which they consider different options of uncorrelated and correlated inputs with the pure and noisy teacher signal.The learning algorithm was also tested with a multiplicative variation of STDP([44]).In contrast to standard STDP,this modified rule enabled producing intermediate stable weight values.However,authors reported that learning with this modified version of STDP was highly sensitive to input signal distributions.In all experiments LIF neuron models and the dynamic synapses models were used([45,46]). However,the synaptic plasticity was considered only for the excitatory connections.The results reported in([43])demonstrated that the learning algorithm was able to approximate the given target transformations quite well.These positive results were achieved not only for the case where the synaptic weights were the adjustable parameters,but also for a more realistic inter-pretation suggested by experimental results where STDP modulated the initial release probability of dynamic synapses([46]).Legenstein and colleagues proved that the method has the convergence property in average for arbitrary uncorrelated Poisson input spike trains.On the other hand,authors demonstrated that the convergence cannot be guarantied in a general case.Authors reported the following drawback of the considered algorithm:Since the teacher currents suppress all undesiredfirings during the training,the only correlations of pre-and postsynaptic activities occur around the targetfiring times.At other times there is no correlation and thus no mechanism to weaken these synaptic weights that led the neuron tofire at undesired times during the testing phase.Another reported problem is common to all Supervised-Hebbian approaches:Synapses continue to change their parameters even if the neuronfires already exactly at the desired times.Thus stable solutions can be achieved only by applying some additional constraints or extra learning rules to the original SHL.Despite these problems,the presented approach proves high ability to implement the precise spike timing coding scheme.Moreover this is thefirst method,of so far presented in this article, that enables learning of the target transformations from the input to the output spike trains. 2.7.ReSuMe-Remote Supervision.We have seen in section2.6that the supervised-Hebbian approach demonstrated interesting learning properties.With this approach it was feasible,not only to learn the desired sequences of spikes,but also to reconstruct the target input-output trans-formations.Moreover,this approach inherited interesting properties of the traditional HebbianLEARNING SPIKE TIMING IN SNN 7n k t n j w nt n i w A in d BC Figure 1.Mechanisms underlying ReSuMe learning:(A)Remote supervisionconcept .The target spike train,transmitted via neuron n d j (i ),is not directlydelivered to the learning neuron n out i .However,it determines (illustrated bydotted line)the changes of the synaptic efficacy w ki ,between a presynaptic neuron n in k (i )and n out i .(B,C)Learning windows.Changes of the synaptic efficacy w kiare triggered by target or postsynaptic action potentials.The amplitude of changeis determined by the functions W d (s d )and W out (s out ),called learning windows.paradigm:it is local in time and space,simple and thus suitable for online processing.On the other hand,it was demonstrated that SHL displays several serious disadvantages that may yield problems when more complex learning tasks are considered.Here we discuss ReSuMe -Remote Supervised Method proposed in ([47]).It is argued that the method possesses interesting properties of SHL approach,while avoiding its drawbacks.The goal of the ReSuMe learning is to impose on a neural network the desired input-output properties,i.e.to produce the desired spike trains in response to the given input sequences.ReSuMe takes advantage of the Hebbian (correlation)processes and integrates them with a novel concept of remote supervision.The name ’remote supervision’comes from the fact that the target signals are not directly delivered to the learning neurons (as it is the case in SHL),however they still co-determine the changes of the synaptic efficacies in the connections terminating at the learning neurons.This is schematically illustrated in Fig.1.A.In more details,a synaptic efficacy w ki ,between any given presynaptic neuron n in k (i )and a corresponding postsynaptic neuron n out i ,is modified according to two rules.The first rule depends on the correlation between n in k (i )and n out i firing times.The second rule is determined by the correlation between n in k (i )and n d j (i )firing times.By n d j (i )we denote a ’teacher’neuron deliveringthe target signal for n out i .For the excitatory synapses these two rules have the forms similar to STDP and anti-STDP and are described by the learning windows W d (s d )and W out (s out )(Fig.1.B and 1.C).The parameters s d and s out denote the time delays (t d j −t in k )and (t out i −t in k ),respectively.For the inhibitory synapses the learning windows differ only in signs in regard to W d (s d )and W out (s out ).The balance of the learning rules,defined for each synapse,leads to the optimal weight values required for obtaining the desired timing of spikes at the learning neurons.The ReSuMe method is biologically plausible,since it is based on the Hebbian-like processes.Also the remote supervision concept,applied to ReSuMe ,can be biologically justified on the basis of heterosynaptic plasticity -a phenomenon recently observed in the neurophysiological experiments ([14,17,48]).High learning ability of ReSuMe has been confirmed through the extensive simulation experi-ments ([47,49,50]).Here we present results of an experiment discussed in ([47]),where ReSuMe was used to train the network to produce the desired sequence of spikes S d (t )in response to the given,specified input spike train S in (t )(Fig.2).ReSuMe has been applied to the LSM network consisting of 800LIF neurons.Both S in (t )and S d (t )signals were generated randomly over a time interval of 400ms (Fig.2.A,C).A single learning neuron was trained over 100learning sessions.An。