各项异性扩散由来及原理
超强自由电子激光的各向异性扩散研究
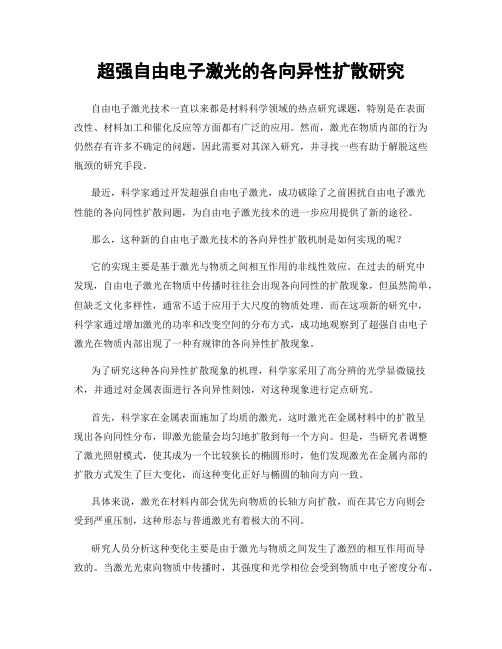
超强自由电子激光的各向异性扩散研究自由电子激光技术一直以来都是材料科学领域的热点研究课题,特别是在表面改性、材料加工和催化反应等方面都有广泛的应用。
然而,激光在物质内部的行为仍然存有许多不确定的问题,因此需要对其深入研究,并寻找一些有助于解脱这些瓶颈的研究手段。
最近,科学家通过开发超强自由电子激光,成功破除了之前困扰自由电子激光性能的各向同性扩散问题,为自由电子激光技术的进一步应用提供了新的途径。
那么,这种新的自由电子激光技术的各向异性扩散机制是如何实现的呢?它的实现主要是基于激光与物质之间相互作用的非线性效应。
在过去的研究中发现,自由电子激光在物质中传播时往往会出现各向同性的扩散现象,但虽然简单,但缺乏文化多样性,通常不适于应用于大尺度的物质处理。
而在这项新的研究中,科学家通过增加激光的功率和改变空间的分布方式,成功地观察到了超强自由电子激光在物质内部出现了一种有规律的各向异性扩散现象。
为了研究这种各向异性扩散现象的机理,科学家采用了高分辨的光学显微镜技术,并通过对金属表面进行各向异性刻蚀,对这种现象进行定点研究。
首先,科学家在金属表面施加了均质的激光,这时激光在金属材料中的扩散呈现出各向同性分布,即激光能量会均匀地扩散到每一个方向。
但是,当研究者调整了激光照射模式,使其成为一个比较狭长的椭圆形时,他们发现激光在金属内部的扩散方式发生了巨大变化,而这种变化正好与椭圆的轴向方向一致。
具体来说,激光在材料内部会优先向物质的长轴方向扩散,而在其它方向则会受到严重压制,这种形态与普通激光有着极大的不同。
研究人员分析这种变化主要是由于激光与物质之间发生了激烈的相互作用而导致的。
当激光光束向物质中传播时,其强度和光学相位会受到物质中电子密度分布、介电函数和激光入射方向等因素的影响而发生变化,这就会引起光束在物质中传播时发生相位差。
而当光束进入高密度电子区域时,则会被电子弹性散射所束缚,并向高密度区域扩散,导致其扩散方向与物质轴向发生了一定的关联。
关于影响金属材料固态扩散的因素与控制

影响金属材料固态扩散的因素与控制摘要:由扩散第一定律可知,在浓度梯度一定时,原子扩散仅取决于扩散系数D。
对于典型的原子扩散过程,D符合Arrhenius公式,。
因此,D仅取决于D0、Q和T,凡是能改变这三个参数的因素都将影响扩散过程。
关键词:温度,因素,扩散,组元,系数一,温度由扩散系数表达式看出,温度越高,原子动能越大,扩散系数呈指数增加。
以C 在γ-Fe中扩散为例,已知D0=2.0×10-5m2/s,Q=140×103J/mol,计算出927℃和1027℃时C的扩散系数分别为1.76×10-11m2/s,5.15×10-11m2/s。
温度升高100℃,扩散系数增加三倍多。
这说明对于在高温下发生的与扩散有关的过程,温度是最重要的影响因素。
应该注意,有些材料在不同温度范围内的扩散机制可能不同,那么每种机制对应的D和Q不同,D便不同。
在这种情况下,~并不是一条直线,而是由若干条直线组成的折线。
例如,许多卤化物和氧化物等离子化合物的扩散系数在某一温度会发生突变,反映了在这一温度以上和以下受到两种不同的机制控制。
图3.15表示出Na+离子在NaCl晶体中扩散系数的实验值。
其中,高温区发生的是以点缺陷扩散为主的本征扩散,低温区发生的是以夹杂产生或控制的缺陷扩散为主的非本征扩散。
二,成分1,组元性质原子在晶体结构中跳动时必须要挣脱其周围原子对它的束缚才能实现跃迁,这就要部分地破坏原子结合键,因此扩散激活能Q和扩散系数D必然与表征原子结合键大小的宏观或者微观参量有关。
无论是在纯金属还是在合金中,原子结合键越弱,Q越小,D越大。
合金中的情况也一样。
考虑A、B组成的二元合金,若B组元的加入能使合金的熔点降低,则合金的互扩散系数增加;反之,若能使合金的熔点升高,则合金的互扩散系数减小,在微观参量上,凡是能使固溶体溶解度减小的因素,都会降低溶质原子的扩散激活能,扩散系数增大。
DWI原理和应用
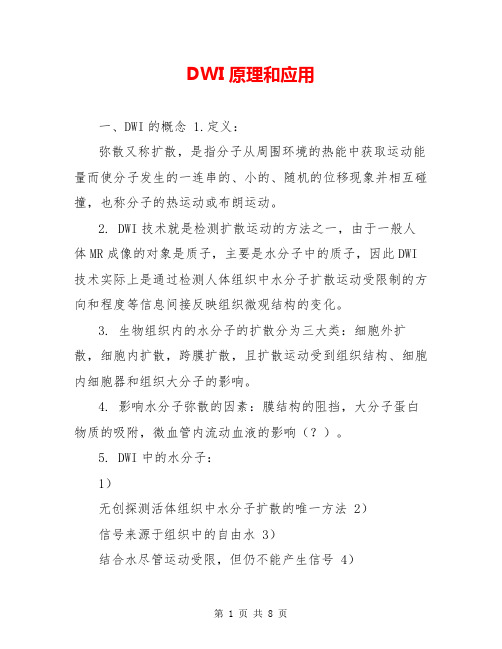
DWI原理和应用一、DWI的概念 1.定义:弥散又称扩散,是指分子从周围环境的热能中获取运动能量而使分子发生的一连串的、小的、随机的位移现象并相互碰撞,也称分子的热运动或布朗运动。
2. DWI技术就是检测扩散运动的方法之一,由于一般人体MR成像的对象是质子,主要是水分子中的质子,因此DWI 技术实际上是通过检测人体组织中水分子扩散运动受限制的方向和程度等信息间接反映组织微观结构的变化。
3. 生物组织内的水分子的扩散分为三大类:细胞外扩散,细胞内扩散,跨膜扩散,且扩散运动受到组织结构、细胞内细胞器和组织大分子的影响。
4. 影响水分子弥散的因素:膜结构的阻挡,大分子蛋白物质的吸附,微血管内流动血液的影响(?)。
5. DWI中的水分子:1)无创探测活体组织中水分子扩散的唯一方法 2)信号来源于组织中的自由水 3)结合水尽管运动受限,但仍不能产生信号 4)不同组织对自由水扩散限制程度不同 5)产生DWI对比 6)检测组织中自由水限制性扩散的程度 6. 常规DWI,主要对细胞外自由水运动敏感 T2WI基础上,施加扩散梯度,组织信号衰减 1)自由水扩散越自由=信号丢失多,DWI信号越低 2)自由水扩散越受限=信号丢失少,DWI信号越高 7. 在均匀介质中,任何方向的弥散系数都相等,这种弥散称为各向同性扩散(eg.脑脊液);在非均匀介质中,各方向的弥散系数不等,这种弥散称为各向异性扩散(eg.脑白质纤维素)。
各向异性扩散在人体组织中是普遍存在的,其中最典型的是脑白质神经纤维束。
水分子在神经纤维长轴方向上扩散运动相对自由,而在垂直于神经纤维长轴的方向上,水分子的扩散运动将明显受到细胞膜和髓鞘的限制。
二、 DWI的原理 1.以SE-EPI序列来介绍DWI的基本原理。
射频脉冲使体素内质子的相位一致,射频脉冲关闭后,由于组织的T2弛豫和主磁场不均匀将造成质子逐渐失相位,从而造成宏观横向磁化矢量的衰减。
除了上述两种因素以外,我们在某个方向上施加一个扩散梯度场,人为在该方向上制造磁场不均匀,造成体素内质子群失相位,然后在施加一个强度与持续时间完全相同的反向扩散梯度场,则会出现两种情况:在该方向上没有位移的质子不会受两次梯度场强的影响而失相位,而移动的质子因两次梯度场引起的相位变化不能相互抵消,而失相位信号衰减。
扩散原理PPT课件

扩散系数热力学 因子
对于理想混合体系,活度系数
D
* i
自扩散系数
i 1 D i D i*RT i B
;
Di组分i的分扩散系数,或本征扩散系数
.
16
讨论:
(1)扩散 外界条件:u/ x的存在
Di 代表了质点的性质,如 半径 、电荷数、极化性能等
基质结构:缺陷的多少;杂质的多少
1 Ln i
Jx=-DCx
J x d xJ x ( J x ) d x D C x x ( D C x ) dx
x x+dx
x
净 增 JJ x + 量 d xJ x x(DC x)dx
J(DC) x x x
又JCC(DC)D2C x t t x x x2
三维表C 达 D (式 2C为 .2C : 2C)
缺陷的多少
(3) 稳定扩散(恒源扩散)
不稳定扩散
C
C
C
J
C/ x=常数
C/ t0
J/ x 0
t
x
.
t
8
x
三维表达式:
J= iJx
jJy
kJz
D(iC j CkC) x y z
用途:
可直接用于求解扩散质点浓度分布不随 时间变化的稳定扩散问题。
.
9
二、 Fick第II定律
推导:取一体积元,分析x→x+dx间质点数 在单位时间内 x 方向的改变,即考虑两个相距为 dx 的平行平面。
散, 质点所受的力
推导D:
高u
Fi
ui x
Vi 低u Fi
对象:一体积元中 多组分中i 组分质点的扩散
i质点所受的力:
Fi
DTI原理及临床应用
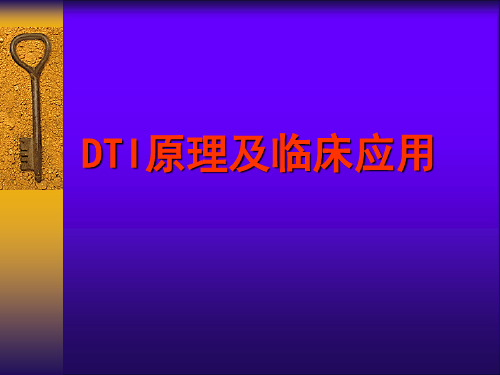
一、概述
DTI是在DWI基础上出现的一种MRI成像
技术, 不仅能发现常规MRI难以显示的病 变,而且能以三维方式显示神经纤维束的
走行方向,为无创性评价白质纤维束间的
联系及其病变一种成像技术。
二、DTI的原理
DTI是利用组织中水分子弥散的各向异性
来探测组织微观结构的成像方法。是一
肿瘤细胞使细胞密度增大的缘故。
利用白质内水分子扩散各向异性的原理,
二维彩色编码的DTI或三维白质束示踪影 像能直观显示脑内白质束的走行方向以
及与邻近肿瘤之间的关系,在指导神经
外科手术中有临床应用价值。
左桥小脑角 区脑膜瘤
右小脑半球 淋 巴瘤
右额叶胶质瘤
左顶叶胶质瘤
种定量显示白质纤维束的成像方法。
与DTI相关的一些概念
1、扩散系数(diffusion coefficient,DC):
表示单位时间内分子自由扩散的范围。
2、扩散敏感因子b值(b value)是反映MRI
各成像序列(如SE、FE、EPI)对扩散运动
表现的敏感程度,体现成像序列检测扩
散的能力。
(四)癫痫
DTI可显示其部分各向异性的降低、扩散
的增加以及白质纤维束的移位。
(五)精神病 精神分裂症病人白质的各向
异性降低,虽然白质的体积未见异常,
但可说明白质纤维束的完整性受损。
FA的降低发生在慢性精神分裂症病人胼
胝体压部及附近枕叶白质,全脑白质和
灰质的平均扩散值增加,这也说明慢性
3、表观扩散系数(apparent diffusion
coeffcient,ADC)描述磁共振扩散加权成
像中不同方面水分子扩散运动的速度和
DTI原理及应用 ppt课件
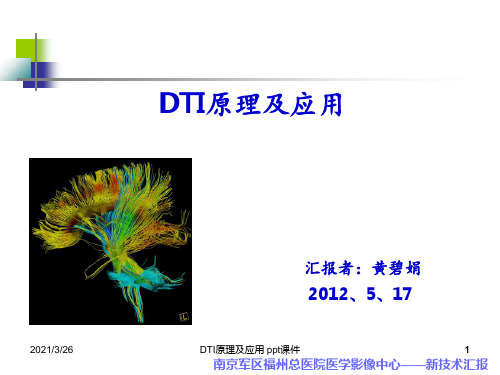
2021/3/26
DTI原理及应用 ppt课件
6
南京军区福州总医院医学影像中心——新技术汇报
Diffusion Tensor imaging 弥散张量成像
• 如果分子扩散取决 于方向,方向不一 致,成为各向异性 (anisotropic diffusion),可用 扩散椭圆形表示
2021/3/26
DTI原理及应用 ppt课件
12
南京军区福州总医院医学影像中心——新技术汇报
部分各向异性 (fractional anisotropy, FA)
➢ 目前描述脑白质纤维束各向异性特征的主要参数 ➢ 其值的大小与髓鞘的完整性、纤维致密性及平行
性有密切关系,能够较真实全面地反映白质纤维 是否完整。
3
南京军区福州总医院医学影像中心——新技术汇报
Diffusion Tensor imaging 弥散张量成像
解剖成像组织的形态学研究
分子水平 (细胞内外水分子跨膜运动) 目前已应用于脑、心脏、脊髓微细结构的研究
2021/3/26
DTI原理及应用 ppt课件
4
南京军区福州总医院医学影像中心——新技术汇报
水分子在自由状态下的弥散是各向同性的南京军区福州总医院医学影像中心新技术汇报diffusiontensorimaging弥散张量成像在纯水中分子在所有方向的扩散一致称各向同性isotropicdiffusion可用扩散球形体表示南京军区福州总医院医学影像中心新技术汇报diffusiontensorimaging弥散张量成像如果分子扩散取决于方向方向不一致成为各向异性anisotropicdiffusion可用扩散椭圆形表示南京军区福州总医院医学影像中心新技术汇报diffusiontensorimaging弥散张量成像dti就是一种用数学的方法来表示脑组织内水分子弥散的各向异性南京军区福州总医院医学影像中心新技术汇报diffusiontensorimaging弥散张量成像正常组织结构水分子各向异性病理组织结构成分改变扩散扩散南京军区福州总医院医学影像中心新技术汇报在脑白质中由于髓鞘的阻挡水分子的弥散被限制在与纤维走向一致的方向上具有较高的各向异性根据脑白质水分子沿神经纤维方向运动快垂直方向运动慢的特点mr图像显示出神经纤维的方向diffusiontensorimaging10南京军区福州总医院医学影像中心新技术汇报diffusiontensorimaging弥散张量成像diffusiontensorfibertractographydtftdti数据处理软件纤维束11南京军区福州总医院医学影像中心新技术汇报diffusiontensorimaging各向异性指标
DWI原理及在中枢神经系统中的临床应用
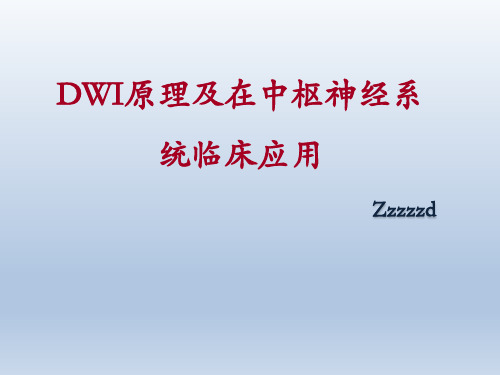
隙性梗死灶中鉴别出急
性期病灶,以指导临床
ADC
eADC
治疗。
左图示双侧基底节区散在多 发小腔隙性脑梗死灶,其中 左侧内囊膝部病灶为本次新 发病灶。
27
急性脑梗死(3)
• DWI高信号机制:
– 脑梗死病生 – 细胞源性水肿(数秒)
正常脑组织水分子布朗运动 细胞内水肿水分子运动受限
– 血管源性水肿(数小时-数天, 无扩散受限)
频率编码梯度 Gx
MR信号
FID
回波
TE TR
SE序列中90脉冲后将产生一个最大的宏观横向磁化矢量,由于主磁场的不均匀和组织的T2弛豫的双 重作用,宏观横向磁化矢量呈指数式衰减,表现为MR信号很快减弱,这种信号变化方式即自由感 应衰减(FID)。由于180脉冲的聚相位作用,在TE时刻将产生一个自旋回波,回波是从无到有,从 小到大,到最大强度后又逐渐变小直到零的MR信号。
急性脑梗死-不同部位脑梗死(1)
DWI图
ADC图
T2WI
M
DWI
27Y
4
AD C
急性脑梗死(2)
• 大面积脑梗死
T2WI
DWI
图
DWI图
❖ 多发腔隙性脑梗死灶中发现新发病灶
T2WI
常规DWI
DWI能早期发现深部脑
白质穿支小动脉闭塞所
致细胞毒性水肿,尤其
是能从不同时期多发腔
DWI在脑梗死中的演变
急性、超急性期脑梗死: 脑动脉阻塞后,缺血中 心区脑组织严重缺血,数分钟内出现不可逆损伤, 即细胞毒性水肿。DWI能超早期(发病30min) 发现严重缺血中心区,在常规DWI上呈明显高信 号,ADC图示扩散明显受限 ADC值演变:5-10d ADC值逐渐接近正常, DWI 高信号;10d –数月, ADC值大于正常, DWI信 号降低
各向异性扩散滤波

各向异性扩散滤波各向异性扩散滤波(Anisotropic Diffusion Filter)是一种在图像处理中常用的非线性滤波算法,用于去噪和边缘增强。
与其他线性滤波算法相比,各向异性扩散滤波能够更好地保持图像的边缘细节,因此被广泛应用于图像增强、图像分割等领域。
该算法的核心思想是根据像素的梯度大小来控制邻域像素的扩散程度。
梯度大小可以反映像素间的强度变化,因此边缘处的梯度较大,说明在该区域内像素的变化较剧烈。
而对于相似的区域,像素值的变化较小,导致梯度较低。
因此,为了保持边缘细节,各向异性扩散滤波根据梯度大小调整每个像素的更新强度。
具体而言,各向异性扩散滤波的更新模型可以表示为:\[\frac{{\partial I}}{{\partial t}} = c(\,\nabla I\,)\nabla^2 I\]其中,\(\frac{{\partial I}}{{\partial t}}\)表示图像强度在时间上的变化率,\(\nabla I\)表示图像梯度,\(\nabla^2 I\)表示图像的二阶导数,负责传播强度变化。
函数\(c(\,\nabla I\,)\)表示更新强度,它是梯度的函数,用于控制不同梯度大小下的扩散程度。
常用的更新强度函数有多种,其中一种常用的形式是:\[c(\,\nabla I\,) = \exp(-\frac{{\,\nabla I\,^2}}{{k^2}})\]其中,\(\,\nabla I\,\)表示梯度的大小,可以使用Sobel算子等计算得到。
参数\(k\)用于控制扩散强度,较小的\(k\)值可以增强边缘,而较大的\(k\)值则会更强化平滑效果。
\[I^{n+1}(x,y) = I^n(x,y) + \lambda \nabla \cdot (c(\,\nabla I^n(x,y)\,) \nabla I^n(x,y))\]其中,\(I^{n+1}(x,y)\)表示第\(n+1\)次迭代后,像素点\((x,y)\)的数值;\(I^n(x,y)\)表示第\(n\)次迭代的像素点\((x,y)\)的数值;\(\nabla\)表示梯度算子;\(\lambda\)表示时间步长,用于控制每次迭代更新的强度。
- 1、下载文档前请自行甄别文档内容的完整性,平台不提供额外的编辑、内容补充、找答案等附加服务。
- 2、"仅部分预览"的文档,不可在线预览部分如存在完整性等问题,可反馈申请退款(可完整预览的文档不适用该条件!)。
- 3、如文档侵犯您的权益,请联系客服反馈,我们会尽快为您处理(人工客服工作时间:9:00-18:30)。
8 Anisotropic diffusion filteringImages contain of the image itself and noiseNoise: random, little disturbances of the imageTo improve the segmentation: the noise should be reduced Condition:•Remove noise•But: keep the imageinformation unchangedDiffusionPhysical process for balancing concentration changes Now:•the image intensity can be seen as a “concentration”•The noise can be modelled as little concentrationinhomogeneitiesThese inhomogeneities could be smoothed by diffusion Diffusion should only be perpendicular e.g. to edgesPhysical background of diffusionGiven: a concentration distribution u Fick’s law:Concentration gradient causes a flux j j aims to compensate the gradientD : diffusion tensor, in general a positive definite, symmetric matrixu∇⋅−=D jPhysical background of diffusion (2) Diffusion is mass transport without destroying mass or creating new massContinuity equationt denotes the time, t u the deviation of u with respect to t∂∂+∂∂−=−=∂y x u t j j j divPhysical background of diffusion (3) diffusion equationApplication in many physical transport process, e.g. for heat transfer it is called heat-transfer-equation Image processing:Identify the concentration with the grey value at a certain location()u u t ∇⋅=∂D divPhysical background of diffusion(4)Diffusion tensor:•Constant over the whole image: homogeneous (orlinear) diffusion•Often it is a function of the structure of the image itself: nonlinear diffusion•Isotropic: j and the concentration gradient are parallel•Anisotropic: otherwiseLinear isotropic diffusionMostly used for smoothing images The image I itself is the initial starting for the diffusion processWe use D = 1 since D only influences the speed of the diffusion),()0,,(div y x I y x u uu t ==∂Linear isotropic diffusion(2)t=4t=8t=12t=20t=16t=24t=40t=0Linear isotropic diffusion(3)Advantages:•Continuously simplifying of the image•Reducing the noise in the imageDisadvantages:•Linear isotropic diffusion does not only reduce noise •It also blues important features like edges•No a-priori knowledge is taken into account•Result: it makes edges harder to identify8.3 Nonlinear diffusionNonlinear diffusionImprovement:•Preservation of the edges, only smooting between edges •Need to assign a position specific diffusivityAdapting the diffusivity g to the gradient in the actualimage u(x,y,t)We obtain the equation()u()∂2div gu=∇u∇tu for 1u for0→∇→∞→∇→g g Nonlinear diffusion (2)Conditions for gPerona & Malik:()22211λu u g ∇+=∇Nonlinear diffusion (3)t=4t=8t=12t=20t=16t=24t=40t=0Nonlinear diffusion (4)t=4t=20t=40t=40t=20t=4LineardiffusionNonlineardiffusionNonlinear diffusion(5)Advantages•Steering of the diffusion at each point of the image is possible•So diffusion can be reduced on edges•The result: edge-preserving image smoothing•But: the smoothing of the edges cannot be completely circumventedNext: anisotropic approachesNonlinear anisotropic diffusionUntil now: determination of the amount of diffusion depending on local features (here: the gradient).Other features could be possibleIdea: we could define the diffusion tensor so that the diffusion goes around some structuresHere: diffusion should preserve edges•On edges: no diffusion over edges, but diffusion parallel to edges should be enabledNonlinear anisotropic diffusion(2)Combination of two features•Non-linearity-The diffusion at border is much less than thediffusion elsewhere•Anisotropy-Diffusion should be perpendicular to edge-No diffusion over edgesNonlinear anisotropic diffusion(3)How to define the diffusion tensor D?Remember: D is a positive definite symmetric matrix •It has two different eigenvalues and two eigenvectors•Therewith the eigenvectors are perpendicular•The eigenvectors gives the main diffusion direction•The corresponding eigenvalues the strength of diffusion in the direction of the eigenvectors.Definition of the eigenvectorsWe want to stop the diffusion over the edge. Therewith we need as one eigenvector the direction of the gradient. The second is perpendicular and can be seen as thetangential vector to the edge [][]−=∇∇=x y v v v u u v 1121Nonlinear anisotropic diffusion (4)u v uv ∇⊥∇21||Definition of the eigenvaluesFirst: We want to stop the diffusion over the edge (direction v1). We use the non-linearity and definePerpendicular to the edge the diffusion should not bestopped ()21ug ∇=λNonlinear anisotropic diffusion (5)12=λNow, we obtain D asResult is an edge-enhancing anisotropic diffusionT⋅⋅ =||||00||||212121v v v v D λλNonlinear anisotropic diffusion (6)Nonlinear anisotropic diffusion (7)t=4t=8t=12t=20t=16t=24t=40t=0Nonlinear anisotropic diffusion (8)t=4t=20t=40t=40t=20t=4NonlineardiffusionNonlinearanisotropicdiffusionNumerical implementationWe haveLooking for a solution u(x,y,t)Either for a specific t = t 0Or for tProblem: the equation cannot be analytically solved Numerical solution is necessary),()0,,(y x I y x u =1. step: discretizing the time axis•Determination of a time step-depends on the numerical stability •only the timesare taken into accountWe obtain u(x,y,t i )Numerical implementation (2)τ∆⋅==i t t i2. step: finding an approximation for the derivative with respect to tThe Taylor serie for u(x,y,t) leads toNeglecting the remain and transform the equation leads to ()ζττR t y x u tt y x u t y x u +∂∂⋅∆+=∆+),,(),,(),,(Numerical implementation (3)),,(),,(),,(1i i i t y x u tt y x u t y x u ∂∂⋅∆+=+τConditions to the step size:Dimensions: m , grid width: hCosts per iteration: very lowEfficiency: low (many iterations needed to get a result)mh 22<∆τNumerical implementation (4)Until now we used the so-called explicit scheme Other notationwith •I : identity matrix•u i values of u(x,y,t i )stacked in vector form•A l (u k )matrix version of the diffusion tensors Notation()i ml i l i u u A I u⋅ ⋅∆+= =+11τSemi-implicit schemeAdditive operator splitting()i ml i l i uu A I u ⋅ ⋅∆−=−=+ 111τImprovements()()i m l i l i m m u u A I u ⋅⋅∆⋅−=−=+ 1111τSemi-implicit scheme: We can write the derivative of u with respect to t asImplicit: because u i+1is usedSemi-implicit: operator A l (u i )is combined with u i+1 Transformation of the equation leads to ()111+=+⋅=∆− i ml i l i i A u u u u τSemi-implicit scheme()i m l i l i u u A I u⋅ ⋅∆−=−=+ 111τSemi-implicit scheme(2)Features:•Stable for < , but: convergence usually becomesslower for too large•Cost per iteratorion: high, because the matrix must bebuilt and inverted in each iteration, O(n2)•Efficiency: medium(better than the explicit schemebecause of the possible larger , but further improvement is possible)Question: How could the invertion of the matrix be more effective•Hint: each A l is a simple tri-diagonal matrixAdditive operator splitting(AOS)Objective: split the derivation operator for each spatial directionThen we get for each direction a simple tri-diagonal matrix Such matrices can be very efficiently invertedAdvantage:•Keep the unrestrictedness of•Make the calculation more effectiveOne can writeas()⋅∆− =ml i l 1u A I τAdditive operator splitting (2)()[]=⋅⋅∆−m l i m m 111u A I τFor the computation of the inverse matrix we use the approximationThenow occuring error is •onlyof second and higher order •This means: the first derivatives have no errors()111−−−+≈+B A B A Additive operator splitting (3)And we approximate the inverse matrix byTherewith we obtain the equation()()[]111111−=−= ⋅⋅∆−≈ ⋅∆−m l i m l i l m m u A I u A I ττAdditive operator splitting (4)()[]i m l i i m m u u A I u 11111−=+ ⋅⋅∆−=τAdditive operator splitting(5)Differences and improvements•A split in the operator for each spatial direction has been performed•The pixels can be arranged for each operator separately •As result each operator is tri-diagonal matrix and isinverted separately.•Such 3-diagonal matrices can be inverted in linear time O(n), not in O(n2)Additive operator splitting(6)Features•Stable for <•Cost per iteratorion: low, but a little higher than for the explicit schemeEfficiency: highhigh low <AOS medium high < Semi-implicitlow very low explicitefficiency Cost per iteration Stability SchemeConclusionm h 22<∆τ。