21_人脸表情识别_章毓晋
人脸表情识别的写作方法与创新点
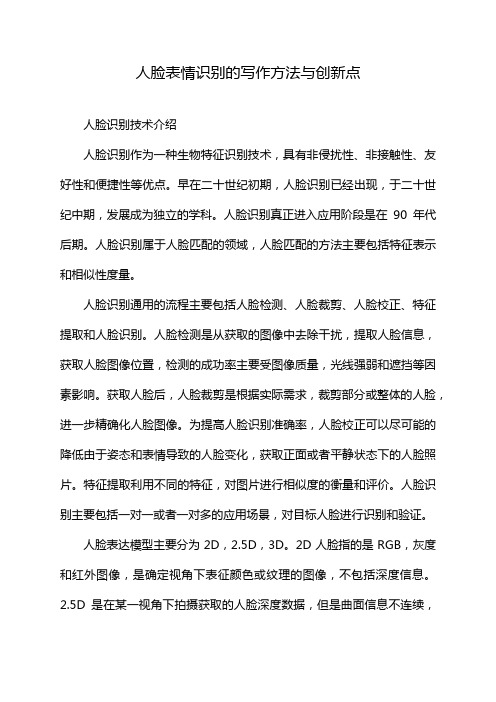
人脸表情识别的写作方法与创新点人脸识别技术介绍人脸识别作为一种生物特征识别技术,具有非侵扰性、非接触性、友好性和便捷性等优点。
早在二十世纪初期,人脸识别已经出现,于二十世纪中期,发展成为独立的学科。
人脸识别真正进入应用阶段是在90年代后期。
人脸识别属于人脸匹配的领域,人脸匹配的方法主要包括特征表示和相似性度量。
人脸识别通用的流程主要包括人脸检测、人脸裁剪、人脸校正、特征提取和人脸识别。
人脸检测是从获取的图像中去除干扰,提取人脸信息,获取人脸图像位置,检测的成功率主要受图像质量,光线强弱和遮挡等因素影响。
获取人脸后,人脸裁剪是根据实际需求,裁剪部分或整体的人脸,进一步精确化人脸图像。
为提高人脸识别准确率,人脸校正可以尽可能的降低由于姿态和表情导致的人脸变化,获取正面或者平静状态下的人脸照片。
特征提取利用不同的特征,对图片进行相似度的衡量和评价。
人脸识别主要包括一对一或者一对多的应用场景,对目标人脸进行识别和验证。
人脸表达模型主要分为2D,2.5D,3D。
2D人脸指的是RGB,灰度和红外图像,是确定视角下表征颜色或纹理的图像,不包括深度信息。
2.5D是在某一视角下拍摄获取的人脸深度数据,但是曲面信息不连续,没有被遮挡部分的深度数据信息。
3D人脸由多张不同角度的深度图像合成,具有完整连续的曲面信息,包含深度信息。
2D图像人脸识别的研究时间较长,软硬件技术较为完备,得到了广泛的应用。
但是由于2D图像反映二维平面信息,不包含深度数据,不能够完整的表达出真实人脸模型。
相比于二维人脸图像,三维图像不受光照等影响,具有更强的描述能力,能够更为真实的反映人脸信息,在人脸合成、人脸迁移、三维人脸识别等场景中应用。
3D人脸识别一般采用深度相机获取人脸深度信息,主要包括双目相机,基于结构光原理的RGB-D相机和基于光飞行时间原理的TOF相机。
常见的三维人脸识别算法主要包括传统识别方法和深度学习识别方法。
1.传统识别方法(1)基于点云数据的人脸识别点云是3D人脸数据的一种表征方式,每一个点都对应一个三维坐标,扫描设备使用这种数据格式存储采集的三维人脸信息,甚至可以将稀疏坐标也拼接到形状信息上,更为完善的反映人脸信息。
图像处理和分析教程章毓晋
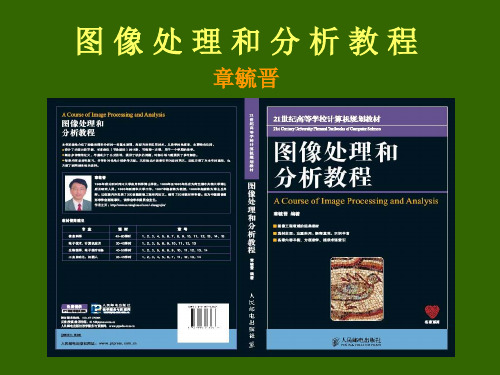
第12章
12-16
12.3 特色的取阈值技术
借助过渡区选择阈值
剪切变换
把被剪切了的部分设成剪切值,避免了一般 剪切在剪切边缘造成大的反差而产生的不良影响
第12章
高端剪切
L
f high (i,
j)
f
(i,
j)
当 f (i, j) L 当 f (i, j) L
低端剪切
f (i, j) flow (i, j) L
12.3 特色的取阈值技术
多分辨率阈值选取
图像在小波变换后可分解为一系列尺度不同 的分量。图像直方图在小波变换后也可进行多分 辨率分析
首先利用在粗分辨率下的直方图细节信息确 定分割区域的类数,即检测出真正的峰点和谷点
确定类数后,可利用多分辨率的层次结构在 直方图的相邻峰之间确定最优阈值,即对峰点和 谷点进行较精确的定位
第12章
12-9
12.2 主动轮廓模型
设计能量函数
外部能量 将变形模板向感兴趣的特征吸引
Eext (vi ) mEmag (vi ) gEgrad (vi )
图像灰度能量函数为正将轮廓向低灰度区域 移动,为负将轮廓将向高灰度区域移动
图像梯度能量函数将变形轮廓吸向图像中的 边缘
第12章
12-10
图像处理和分析教程
章毓晋
第12章 典型图像分割算法
随着各学科许多新理论和方法的提出, 人们也提出了许多结合一些特定理论、方法和 工具的分割技术
图像分割至今为止尚无通用的自身理 论。所以,每当有新的数学工具或方法提出 来,人们就试着将其用于图像分割,因而提出 了不少特殊的或者说有特色的分割算法
介绍几个具有比较特殊思路的典型方法
当 f (i, j) L 当 f (i, j) L
图像处理章毓晋课后答案
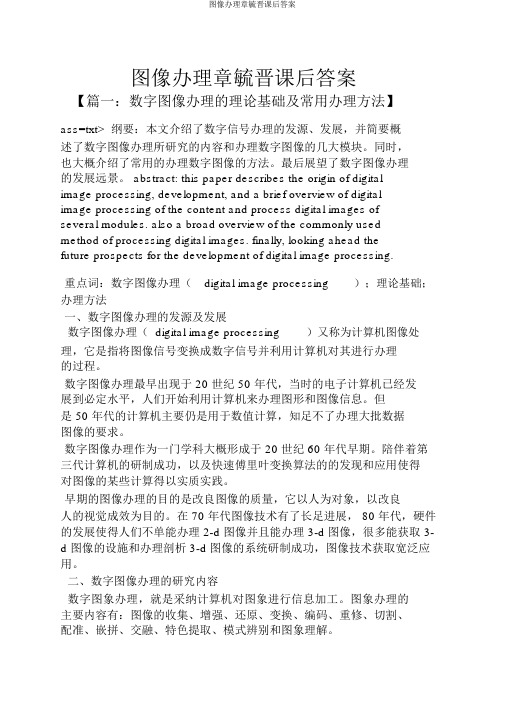
图像办理章毓晋课后答案【篇一:数字图像办理的理论基础及常用办理方法】ass=txt> 纲要:本文介绍了数字信号办理的发源、发展,并简要概述了数字图像办理所研究的内容和办理数字图像的几大模块。
同时,也大概介绍了常用的办理数字图像的方法。
最后展望了数字图像办理的发展远景。
abstract: this paper describes the origin of digitalimage processing, development, and a brief overview of digitalimage processing of the content and process digital images ofseveral modules. also a broad overview of the commonly usedmethod of processing digital images. finally, looking ahead thefuture prospects for the development of digital image processing.重点词:数字图像办理(digital image processing);理论基础;办理方法一、数字图像办理的发源及发展数字图像办理( digital image processing)又称为计算机图像处理,它是指将图像信号变换成数字信号并利用计算机对其进行办理的过程。
数字图像办理最早出现于 20 世纪 50 年代,当时的电子计算机已经发展到必定水平,人们开始利用计算机来办理图形和图像信息。
但是 50 年代的计算机主要仍是用于数值计算,知足不了办理大批数据图像的要求。
数字图像办理作为一门学科大概形成于 20 世纪 60 年代早期。
陪伴着第三代计算机的研制成功,以及快速傅里叶变换算法的的发现和应用使得对图像的某些计算得以实质实践。
基于ROI-KNN卷积神经网络的面部表情识别
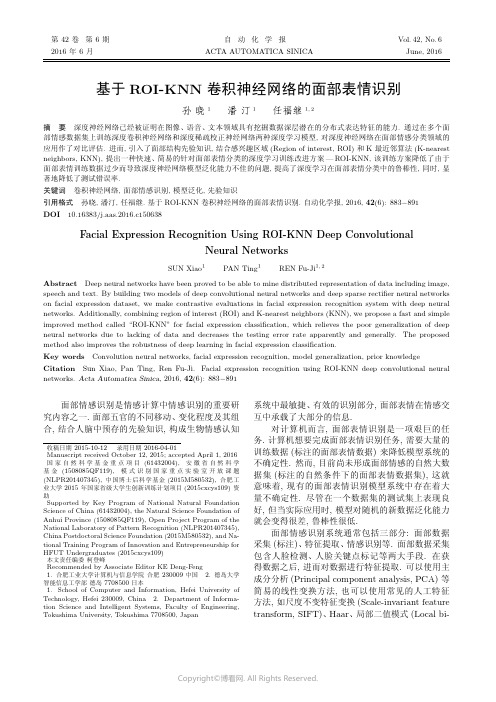
第42卷第6期自动化学报Vol.42,No.6 2016年6月ACTA AUTOMATICA SINICA June,2016基于ROI-KNN卷积神经网络的面部表情识别孙晓1潘汀1任福继1,2摘要深度神经网络已经被证明在图像、语音、文本领域具有挖掘数据深层潜在的分布式表达特征的能力.通过在多个面部情感数据集上训练深度卷积神经网络和深度稀疏校正神经网络两种深度学习模型,对深度神经网络在面部情感分类领域的应用作了对比评估.进而,引入了面部结构先验知识,结合感兴趣区域(Region of interest,ROI)和K最近邻算法(K-nearest neighbors,KNN),提出一种快速、简易的针对面部表情分类的深度学习训练改进方案—ROI-KNN,该训练方案降低了由于面部表情训练数据过少而导致深度神经网络模型泛化能力不佳的问题,提高了深度学习在面部表情分类中的鲁棒性,同时,显著地降低了测试错误率.关键词卷积神经网络,面部情感识别,模型泛化,先验知识引用格式孙晓,潘汀,任福继.基于ROI-KNN卷积神经网络的面部表情识别.自动化学报,2016,42(6):883−891DOI10.16383/j.aas.2016.c150638Facial Expression Recognition Using ROI-KNN Deep ConvolutionalNeural NetworksSUN Xiao1PAN Ting1REN Fu-Ji1,2Abstract Deep neural networks have been proved to be able to mine distributed representation of data including image, speech and text.By building two models of deep convolutional neural networks and deep sparse rectifier neural networks on facial expression dataset,we make contrastive evaluations in facial expression recognition system with deep neural networks.Additionally,combining region of interest(ROI)and K-nearest neighbors(KNN),we propose a fast and simple improved method called“ROI-KNN”for facial expression classification,which relieves the poor generalization of deep neural networks due to lacking of data and decreases the testing error rate apparently and generally.The proposed method also improves the robustness of deep learning in facial expression classification.Key words Convolution neural networks,facial expression recognition,model generalization,prior knowledge Citation Sun Xiao,Pan Ting,Ren Fu-Ji.Facial expression recognition using ROI-KNN deep convolutional neural networks.Acta Automatica Sinica,2016,42(6):883−891面部情感识别是情感计算中情感识别的重要研究内容之一.面部五官的不同移动、变化程度及其组合,结合人脑中预存的先验知识,构成生物情感认知收稿日期2015-10-12录用日期2016-04-01Manuscript received October12,2015;accepted April1,2016国家自然科学基金重点项目(61432004),安徽省自然科学基金(1508085QF119),模式识别国家重点实验室开放课题(NLPR201407345),中国博士后科学基金(2015M580532),合肥工业大学2015年国家省级大学生创新训练计划项目(2015cxcys109)资助Supported by Key Program of National Natural Foundation Science of China(61432004),the Natural Science Foundation of Anhui Province(1508085QF119),Open Project Program of the National Laboratory of Pattern Recognition(NLPR201407345), China Postdoctoral Science Foundation(2015M580532),and Na-tional Training Program of Innovation and Entrepreneurship for HFUT Undergraduates(2015cxcys109)本文责任编委柯登峰Recommended by Associate Editor KE Deng-Feng1.合肥工业大学计算机与信息学院合肥230009中国2.德岛大学智能信息工学部德岛7708500日本1.School of Computer and Information,Hefei University of Technology,Hefei230009,China2.Department of Informa-tion Science and Intelligent Systems,Faculty of Engineering, Tokushima University,Tokushima7708500,Japan 系统中最敏捷、有效的识别部分,面部表情在情感交互中承载了大部分的信息.对计算机而言,面部表情识别是一项艰巨的任务.计算机想要完成面部表情识别任务,需要大量的训练数据(标注的面部表情数据)来降低模型系统的不确定性.然而,目前尚未形成面部情感的自然大数据集(标注的自然条件下的面部表情数据集),这就意味着,现有的面部表情识别模型系统中存在着大量不确定性.尽管在一个数据集的测试集上表现良好,但当实际应用时,模型对随机的新数据泛化能力就会变得很差,鲁棒性很低.面部情感识别系统通常包括三部分:面部数据采集(标注)、特征提取、情感识别等.面部数据采集包含人脸检测、人脸关键点标记等两大手段.在获得数据之后,进而对数据进行特征提取.可以使用主成分分析(Principal component analysis,PCA)等简易的线性变换方法,也可以使用常见的人工特征方法,如尺度不变特征变换(Scale-invariant feature transform,SIFT)、Haar、局部二值模式(Local bi-884自动化学报42卷nary pattern,LBP)等.最后,将提取到的特征数据输入到判别分类器当中,得到识别结果.随着深度神经网络的提出,图像识别领域的“先提取特征,后模式识别”这一框架被打破.Krizhevsky 等[1]在ILSVRC-2012图像识别竞赛中,利用深度卷积神经网络的自适应特征提取能力,使得模型的测试成绩远远超过了SIFT 等具有旋转缩放不变性的人工特征.最近,在面部情感识别任务上,Lopes 等[2]尝试引入了卷积神经网络模型,将特征提取和判别分类两个步骤统一结合,在Extended CohnKanade (CK+)[3]静态情感数据集上取得了很好的测试结果.然而,目前大多数针对面部表情的深度学习模型是在标准数据集上训练并获得较好的结果,在实际应用中却出现精度急剧下降,无法重现实验室模型的准确率,这部分原因在于基于CK+等标准数据集上训练的模型有两个比较明显的缺陷:1)其数据都是摄像机通过正规的角度采集,这与实际系统获得的Wild 数据有很大的差别,会导致模型的泛化能力很差.如图1所示.在实验部分,通过设计相应实验验证了这点.图1CK+与Wild 数据集样例Fig.1Samples from CK+and Wild2)CK+数据集有593幅面部表情图像,包括愤怒、厌恶、恐惧、高兴、悲伤、惊讶六种基本情绪,这意味着平均每种表情有不到100个训练样本.即便引入非表情峰值的图像进行扩展,或采用Lopes 等[2]的对单张图片旋转采样生成30张的扩展训练样本方法,最后得到的大部分图像携带的信息都有重复(接近于样本简单复制),与同数量的不同样本在信息量上还是有不少差距.而目前小型图像数据集的原始数据量基准都是60k (不包括采样生成),如MNIST 、Cifar10等数据集.相对这些数据集,在CK+上的训练更容易达到过拟合.鉴于以上两点问题分析,目前基于CK+数据集训练并得到的高准确率(95%)测试结果并不意味着当前模型系统已经胜任真实的面部情感识别任务,或超越人类的识别结果.本文第1节将介绍深度神经网络在模型结构上的一些新变化.第2节将介绍两种基本的深度神经网络结构以及针对小数据集的先验改良方法.第3节介绍混合CK+与从互联网上收集Wild 面部表情数据形成的新数据集,包含实验测试结果与分析.第4节是结论、归纳.本文中使用的基于Theano 深度学习框架的相关代码和训练参数可从Github 1获取.1相关工作1.1神经网络神经网络的出发点是“参数”拟合“函数”,Bishop [4]从贝叶斯概率体系角度证明了拟合学习算法的判别根据:p (t |x,t ,α,β)=N(t |m T N Φ(x ),σ2N (x ))(1)m T N Φ(x )=y (x,m N )=N n =1kernel (x,x n )t n (2)式(1)表明了预测数据t ,在训练数据t 、x ,以及训练数据高斯方差β、参数高斯方差α的概率分布同样是一个高斯分布.式(2)表明了该高斯分布的均值为一个等价核函数(即Smooth 矩阵)与训练目标的乘积.该核函数衡量着预测输入x 与训练输入x n 的距离.距离越近,数值越大,预测目标t 就越接近训练目标t n ,反之亦然.Bengio [5]指出,参数模型如支持向量机(Sup-port vector machine,SVM)、浅层神经网络,非参数模型如K 最近邻算法(K-nearest neighbors,KNN),最基本的特性都是基于训练样本与预测样本输入的空间距离而做出预测结果的,称之为平滑先验(Smoothness-prior).这个先验在目标函数随输入空间变化敏感时,只能采集到局部特征(Local representation),会得到很差的泛化结果,而图像数据的输入空间恰好如此.因而,不可以直接在图像任务中使用这些分类器,而需要先提取特征.从流形学习的观点看,SIFT 、Haar 、LBP 等人工特征或是PCA 这类的简单线性变换特征将输入空间的流形面从高维降至低维,如图2,由于流形面是局部光滑的,从而使得具有平滑判别能力的分类器在流形面区域变换后,仍然可以很好地分类.1.2深度卷积神经网络LeCun 等[6]在1990年提出的深度卷积神经网络,如图3.以Fukushima [7]的感知机结构为基础,借助Rumelhart 等[8]的反向传播训练算法,首先在文字图像识别领域取得巨大成功[9].卷积神经网络与一般的全连接式神经网络相比较,除了在模型中注入Smooth 这样的先验知识之外,还注入一些针对图像数据特点的先验知识.1https:///neopenx/6期孙晓等:基于ROI-KNN 卷积神经网络的面部表情识别885图2输入空间的流形面Fig.2Manifold side of inputspace图3卷积神经网络的局部块状连接与基本结构Fig.3Local connection and structure of convolutionalneural network (CNN)1.2.1局部性图像中包含的隐含信息在输入空间内具有局部平滑性,因此卷积神经网络针对像素块建立块状神经元的局部连接(Locally-connection).传统基于像素点的连接称为全连接(Fully-connection)或稠密连接(Dense-connection).块状神经元显著减少了每层神经元参数个数,这使得误差从输出层开始,以较小的广度发散[5],同时可以增加神经网络深度,来保持结构中深度和广度之间的平衡.Szegedy 等[10]利用此特性构建出22层的GoogLeNet,赢得了ILSVRC-2014图像识别竞赛冠军.1.2.2权值共享/局部感受野当二维神经元块维度小于二维数据块(特征图)时,意味着神经元块参数会在数据块的不同区域重复加权计算,这构成权值共享,数学形式即二维离散卷积.权值共享的做法借鉴了视觉神经感受野的概念,Fukushima [7]认为局部感受野使得模型获得图像中的平移不变性,增强泛化能力.1.2.3降采样降采样Pooling 层是一个非参数层,作用是将一定邻域内的像素块压缩成一个像素点,使图像缩放.它通常紧接着卷积层,根据缩放算法的不同,分为锐化(Max pooling)、平滑(Avg pooling).对输入图像数据块的逐层缩放,让各层获得不同比例的局部感受野,使得模型获得图像中的缩放不变性,增强泛化能力.1.3深度稀疏校正神经网络Glorot 等[11]提出深度稀疏校正神经网络(Deep sparse rectifier neural networks)从结构上仍然属于全连接神经网络,唯一变化是将Sigmoid 型(logistic/tanh)激活函数全部替换成了ReLU.1.3.1深度结构的有效性Barron [12]证明了拥有一个隐层、N 个神经元的全连接神经网络可以将任何函数拟合至1/N 精度.这意味着,如果需要增加拟合精度,只要广度,而无需考虑深度.而Bengio [5]认为如果一个函数可以由多个函数组合得到,在数据有限的情况下,使用过浅的深度会影响拟合的效果,引起训练周期过长、泛化能力很差等问题.Hubel 等[13]在实验中发现猫的视觉皮层由多层抽象神经结构完成,V1层提取图像边缘特征,V2层开始逐层组合出部分形状,直至最后组合抽象出完整的视觉目标.这从生理学角度证明了图像识别函数可以由多个函数组合而成,增加神经网络的深度要比广度有效得多.1.3.2ReLU 激活函数Dayan 等[14]通过拟合数据,发现生物神经元输入电信号与激活频率之间的函数图像具有相对的不对称性与对称性,如图4所示,不对称区域出现了一段很突兀的“0”,这与主流的Sigmoid 函数有很大区别,而与ReLU 函数比较相似.Attwell 等[15]通过实验观察到,脑神经元在一定时刻,平均只有1%∼4%被激活,这段“0”起到了很强的校正作用,让大部分神经元处于完全不激活状态,这是生物神经网络具有数以千亿计的神经元,而不会像模型神经网络一样引发“维数灾难”的原因.ReLU 激活函数定义为:ReLU (x )=max(0,x )Softplus 函数是它的平滑版本:Softplus (x )=log(1+e x )Softplus 与ReLU 都是非饱和函数,它们输出的上下界不被限制在[–1,1]之内,这大大缓解了深度结构带来的梯度发散(Gradient vanish)问题,促进梯886自动化学报42卷图4不同激活函数的函数图像(图片源自Glorot [11])Fig.4Graphs for different activation functions from Glorot [11]度在反向传播中路径中的流动,在训练庞大神经网络时,有数倍的提速效果[1].另外,校正“0”为模型注入了大量稀疏性,与L1Regularization 效果相同.目前已知,稀疏性有助于推动线性不可分转化为线性可分,缩小做逐层贪心预训练与不做之间在泛化效果上的差异[11].1.4DropoutHinton 等[16]提出的Dropout 层在大量实验中已经被证实可以有效改善任意神经网络的过拟合问题.Dropout 分为两个阶段:1)训练阶段:此时经过该层的所有输入点x ,都会以一定概率p 被置为0,即该神经元被剔除.定义式:DropoutT rain (x )=RandomZero (p )×x这是一个随机过程,意味着每次正向传播,网络的有效结构都会产生变化.2)测试阶段:此时应该激活所有神经元,变成完整结构.激活全部神经元等效于多个随机神经网络的叠加求和,需要对输入x 做一个平均处理,不然会出现数值问题.定义式:DropoutT est (x )=(1−p )×xDropout 能有效改善过拟合可以从两个角度理解.首先,Dropout 引入了随机化的稀疏性,让庞大的神经网络模型在同一时刻只工作一部分,这与Attwell 等[15]在生物神经方面的工作不谋而合.其次,由于每次网络的结构都在变化,参数会不停受到惩罚,被迫向一个稳定的方向调整,而不是简单地做拟合.这与Darwin [17]提出的“自然竞争选择”概念切合,拉近了模型神经网络与生物神经网络的距离.1.5初始化1.5.1权值初始化传统的神经网络权值初始化为:W =Uniform−1√N ,1√NXavier 等[18]提出了更适合Sigmoid 函数的方案:W =Uniform−1√F in +F out ,1√F in +F out其中,F in 为输入维度,F out 为输出维度.Bishop [4]指出,在N →∞时,均匀分布会演变为高斯分布,更一般地,任意连续的随机变量都可以假定符合高斯分布.而贝叶斯拟合模型引入的关于W 的共轭先验分布也是假定P (W )服从高斯分布.这意味着,使用均匀分布来初始化W 不是一个很好的方案.Krizhevsky 等[1]、Hinton 等[16]在ILSVRC-2012图像识别竞赛的冠军模型中,对W 的初始化使用了零均值、常数方差的高斯分布而不是传统的均匀分布,从实验角度证明了高斯分布初始化的合理性.1.5.2偏置初始化Krizhevsky 等[1]、Hinton 等[16]将神经网络隐层(非输出层)的偏置初始化为1而不是0,让训练在初期阶段得到很大加速.目前尚无数学解释,只是经验规则.2结构、超参数与改良方法2.1深度卷积神经网络如图5,针对输入大小为32×32的灰度图(彩色维度为1),构建了3个卷积与Max pooling 层、1个全连接层、1个Softmax 层.根据各层神经元个数的不同,又分为CNN-64、CNN-96、CNN-128.CNN-64:[32,32,64,64]CNN-96:[48,48,96,200]CNN-128:[64,64,128,300]6期孙晓等:基于ROI-KNN 卷积神经网络的面部表情识别887图5深度卷积神经网络的结构(?表示不确定超参数,有多种优选方案.)Fig.5Structure of DNN(?represents uncertain parameters with many candidatesolutions.)为了减轻过拟合问题,全连接层后连接着一个p =0.5的Dropout 层,而不是使用L2Regulariza-tion.除Softmax 层之外,其余各层激活函数均为ReLU,卷积层输出激活后,再输入到Max pooling 层.权值W 的初始化采用Krizhevsky 等[1]的零均值、常数标准差(Standard deviation,STD)方案.各层STD 分别为:[0.0001,0.001,0.001,0.01,0.1]偏置的初始化采用Krizhevsky 等[1]的方案.2.2深度稀疏校正神经网络如图6,针对输入大小为32×32的灰度图(彩色维度为1),构建了3个全连接层、1个Softmax 层.图6深度稀疏校正网络的结构Fig.6Structure of deep sparse rectifier net根据各层神经元个数的不同,又分为DNN-1000,DNN-2000.DNN-1000:[1000,1000,1000]DNN-2000:[2000,2000,2000]为了减轻过拟合问题,三个全连接层后各连接着三个p =0.2的Dropout 层.除Softmax 层之外,其余各层激活函数均为ReLU.权值W 的初始化各层STD 分别为:[0.1,0.1,0.1,0.1].在测试中发现,隐层偏置全部设为1对于深度稀疏校正神经网络效果并不好,所以设为0.2.3数据预处理、训练参数控制本文的数据处理中只做了均值标准化,取训练数据32×32的各个维度计算1024个均值并序列化保存.训练、测试时,减去均值.特别地,DNN 在均值标准化后,对数值缩小128.0倍.训练过程中使用交叉验证与早终止(Early stopping).两个模型学习率lr 为常数0.01,动量momentum 为常数0.9.交叉验证中发现验证集错误率不再下降或上升时,即判定为学习率lr 过大,停止并降低一个数量级,再次训练,重复直至学习率在0.0001阶段结束,经历3个数量级的训练.2.4ROI-KNNXavier 等[18−19]在利用深度卷积神经网络训练人脸特征时,采取对单张图片不同尺度区域切割的方法,来扩大数据集.本文借鉴了此方法,并针对面部表情识别做了改进,根据人脸的面部结构,设置了9个不同的感兴趣区域(Region of interest,ROI),如图7,主动引导神经网络关注与表情相关的面部区域.图79个ROI 区域(切割、翻转、遮盖、中心聚焦)Fig.7Nine ROI regions (cut,flip,cover,center focus)设置ROI 区域使用的都是图像处理中的基本手段,包含切割、翻转、遮盖、中心聚焦.为了确保不同面部的ROI 区域不会有太大偏差,需预先进行人脸检测提取人脸,使面部填充图像的大部分区域,让面部中轴线近似与图像中轴线重合.切割方案重点关注眼、鼻、嘴在不同表情中的区别,为了尽量让处理手段简单,并没有预先检测面部关键点来切割.翻转方案考虑了拍摄方式的不同.遮盖方案是对切割方案的联合.中心聚焦方案去除了一定噪声(如头发).ROI 方法让训练数据扩大至9倍,这种扩大是否有效,取决于这些迥异ROI 区域之间是否存在着某些联系,有助于增强预测目标的信度.这里的增强更强调ROI 区域对测试原始图像的增强、不同ROI 区域之间的增强(如左眼对上半脸),而不仅仅是相同ROI 区域间的增强(如左眼对左眼).Bengio [5]指出了这两者的区别:前者的成功源于模型挖掘出了分布式表达特征(Distributed representation),分布式表达特征让模型对未观测的数据有着很好的泛化和归纳.而后者的成功则受Smooth-prior 作用下的局部表达特征(Local representation)影响较大,与训练数据、测试数据在输入空间的距离有很大关888自动化学报42卷系.在下一节的实验会证明ROI区域确实对判别原始图像有一定增强.ROI数据倍增的效果是针对训练阶段的,而在测试阶段最直接的方法是对测试图像直接判别.但因为这会浪费模型中记忆的关于ROI区域的分布式表达特征.尽管这些特征在直接做判别时具有推动作用,但未起到更大作用.众多机器学习模型中,KNN具有出色且简单的归并能力,它通过建立贪心投票机制,让多个判别目标联合,缩小最终的判别范围,强化最终的判别信度.鉴于此,提出ROI-KNN方法,在测试时,对9个ROI区域的判别结果投票,取票数最多的判别结果作为最终结果,在线归并原始结果.ROI-KNN的最大缺陷是对原始模型训练的Distributed representation有很高的要求,因为这些ROI的输入信息较完整图片要小很多,直观上来看,就是放大关注细节.训练ROI与测试ROI之间细微的差别,被放大之后,模型中的Local represen-tation会对判别产生很大干扰.在实验中最直接的体现就是ROI区域的测试错误率要大于原始图像错误率,若基于这种情况下投票,那么最后的投票结果反而比不投票要差.下一节将设计相应的实验验证.2.5旋转生成采样Lopes等[2]扩大数据集的方法是将原始图像轻微旋转一定角度,生成大量变化的训练样本.这种做法看起来似乎是没有问题的,因为深度卷积神经网络本身具有挖掘图像缩放不变性、平移不变性的能力,唯独缺少旋转不变性.在这里必须考虑一个问题:强行注入旋转变换的样本能否让模型获得旋转不变性?本文对此的答案是否定的.卷积神经网络得到的平移、缩放不变性是模型不断提炼、泛化的成果,而直接注入的旋转样本可能只会让模型出现过拟合,因为模型本身并没有提炼旋转不变性的能力,而本文提出的ROI方法是基于平移、缩放不变性的,没有这种潜在问题.如果测试数据与训练数据较为接近,那么过拟合问题就不会暴露,反之亦然.本文认为Lopes等[2]注入旋转样本后的模型有过拟合的可能,因为他们的测试数据与训练数据很接近,注入旋转样本得到的改善很有可能是过拟合得到的.在下一节的实验中会使用旋转样本,对Wild数据进行测试来验证.3实验本节使用第2.1节和第2.2节中构建的两个深度神经网络模型做对比评估,评估环节的目标包括: ROI辅助评估、旋转生成样本评估和ROI-KNN辅助评估.最后将评估深度学习模型与非深度学习模型.3.1数据集为了解决CK+数据集过于正规的问题.从互联网各大搜索引擎中收集了4类,每类500张Wild 数据,分别是高兴、悲伤、惊讶、愤怒.此外,由于CK+数据集的原始类别标签不含有“中性”表情,从合肥工业大学教务管理系统中抽取了1200张学生面部照片,这些照片除了表情呈中性之外,与CK+一样,都是很正规的摄像机取景,方便在测试集中与Wild数据作对比评估.训练集由CK+的高兴、悲伤、惊讶、愤怒各700张混合互联网下载的图片各200张以及“中性”的900张构成.共计5类,每类900张图片.测试集由互联网下载的图片各300张混合“中性”的300张构成.共计5类,每类300张图片.3.2ROI辅助评估ROI辅助评估是本文关注的重点,它反映着模型内部Distributed representation的训练情况.使用的是第3.1节给出的5类共4500张面部训练数据、5类共1500张测试数据.训练4500张数据经过ROI处理后,为4500×9=40500张,测试数据不做变化.实验结果如表1,基准为无ROI强化,“∗”表示ROI强化.从整体实验结果来看,ROI的引入对两套模型的各个规模都有4%∼5%的精度提升,符合预期.深度卷积神经网络随着规模的提升,效果也在提升,达到最好的整体错误率25.8%.逐一对各个表情分析,可以发现一些问题.首先,就是中性测试集相对于其他测试集,测试成绩非常高.这是在第3.1节数据有意如此设置:测试集里,只有中性集没有使用Wild数据,而选择了与训练集较为相似的正规数据,这个成绩符合预期,同时证明了Lopes等[2]基于CK+的高准确率测试结果并不一定意味着模型拥有良好的泛化能力.其次,悲伤测试集表现最差,这与Lopes等[2]的结果一致,说明面部悲伤情感比较难被准确识别,而高兴、惊讶、愤怒的测试结果则比较接近.表1ROI辅助评估的测试集错误率(%) Table1Test set error rate of ROI auxiliary(%)中性高兴悲伤惊讶愤怒整体CNN-64 4.732.754.33340.333.3 CNN-64* 5.636.359.320.031.730.6 CNN-96* 5.036.753.320.724.728.6 CNN-128 3.332.051.027.037.730.2 CNN-128* 3.031.055.718.724.326.6 DNN-1000 3.037.765.338.336.736.2 DNN-1000* 2.339.052.030.031.731.0 DNN-2000* 2.043.355.024.732.731.56期孙晓等:基于ROI-KNN卷积神经网络的面部表情识别8893.3旋转生成样本评估在第2.5节推测旋转采样生成的样本可能会导致神经网络模型产生过拟合,为了验证该假设的可能性,设计了两份新的训练数据:1)数据集I.针对CK+与高考录取照片两类正规数据,以图像中心为原点,进行旋转采样.旋转方法同文献[2],令旋转角α服从零均值高斯分布:α∼N(0,3o)对源训练集5类,每类700张执行高斯随机数11次,加上第3.1节4500张训练图像,共有5×700×11+4500=43000张,构成新训练集,测试集不变化.2)数据集II.将数据集I中的43000张采样数据,与第3.2节中的40500张数据混合,共计83500张训练数据,构成新训练集,测试集不变化.以第3.2节中的无ROI测试结果作为对比基准,实验结果如表2,“*”表示使用数据集I,“+”表示使用数据集II+ROI,“∧”表示使用数据集II结合ROI-KNN.表2旋转生成样本评估的测试集错误率(%) Table2Test set error rate of rotating generatedsample(%)中性高兴悲伤惊讶愤怒整体CNN-128 3.332.051.027.037.730.2 CNN-128* 4.741.352.732.735.033.2 CNN-128+ 3.037.051.715.724.026.3 CNN-128ˆ0.030.054.013.026.724.7 DNN-1000 3.037.765.338.336.736.2 DNN-1000* 1.339.762.037.342.036.5 DNN-1000+ 2.341.357.030.035.733.3 DNN-1000ˆ 1.343.067.731.033.735.3从整体实验结果来看,旋转生成样本的引入暴露了不少问题.首先,对于数据集I,CNN-128、DNN-1000用43000张原始与生成的混合大数据,得出了比4500的小数据还差的结果,说明38500张旋转生成样本不仅没有促进归纳和泛化,反而对Wild数据的直接判别产生了干扰,这与Lopes等[2]的结果截然相反,本文认为是基于CK+的测试集掩盖了过拟合问题.其次,对于数据集II, ROI的引入几乎抵消了旋转样本的影响,但是此时ROI-KNN的效果不佳,在DNN-1000中尤为明显.第3.4节中的实验结果表明,ROI-KNN对模型中的Distributed representation有很高的要求,ROI-KNN的效果不佳,从另一个角度表明了引入旋转生成样本可能对Distributed representation产生了影响.基于以上两个数据集的测试,可以判断在面部情感分析任务上,引入旋转生成样本来扩大数据集并不是一个可取的方案.它并不能让具有缩放、平移不变性的深度卷积神经网络获得旋转不变性,反而因为旋转输入空间的引入,对缩放、平移不变性的效果产生干扰,构成由于模型挖掘数据能力不足,导致的不可避免型过拟合,这种过拟合不是由于参数空间过大引起的,没有方法通过扩大数据集避免.当测试数据与训练数据有较大偏差和变化时,便会显现出来,若模型训练按照这种方式训练,则是无法在实际中应用的.3.4ROI-KNN辅助评估ROI-KNN辅助评估将考察KNN的贪心投票机制对结果的影响,按照第2.4节中的推测,它对模型内部的Distributed representation有很高的要求.实验结果如表3,基准为ROI强化,“*”表示ROI-KNN强化.表3ROI-KNN辅助评估的测试集错误率(%)Table3Test set error rate with ROI-KNN(%)中性高兴悲伤惊讶愤怒整体CNN-64 5.636.359.320.031.730.6 CNN-64* 1.029.756.017.030.026.7CNN-96 5.036.753.320.724.728.6 CNN-96*0.326.056.316.026.725.8 CNN-128 3.031.055.718.724.326.6 CNN-128*0.622.757.012.026.323.7 DNN-1000 2.339.052.030.031.731.0 DNN-1000*0.337.361.031.731.032.2 DNN-2000 2.043.355.024.732.731.5 DNN-2000*0.340.068.026.333.333.6从整体实验结果来看,KNN的投票机制让深度卷积神经网络各个规模又得到了4%∼5%的精度提升,但在深度稀疏校正神经网络中,不仅没有提升,反而让整体结果略微变差.逐一对各个表情分析,在深度卷积神经网络中,除了悲伤集外,其他测试集均有一定提升.在深度稀疏校正神经网络中,中性、高兴集有一定提升,悲伤集变差幅度最大,其他测试集几乎无变化.此实验结果表明了KNN投票机制对模型的泛化能力(或Distributed representation)有很高的要求,直接体现在泛化最差的悲伤集上,各个模型表现均不好.另一方面,卷积神经网络整体又比深度稀疏校正神经网络好得多,可能是得益于内部针对图像处理的先验知识.3.5与非深度学习模型的对比为了比较所提出的ROI-KNN方法与SVM等非深度学习方法的性能,设计了另一组实验,在公开JAFFE数据集上,与SVM、PCA等非深度学习方法进行了比较,其中本文的模型选取了CNN-128结合ROI-KNN.从表4中可以看出,相对SVM等浅层机器学习模型,本文提出的深度学习模型在传统的数据集上有非常优异的表现.。
基于深度学习技术的热图像人脸表情识别(IJIGSP-V11-N10-1)
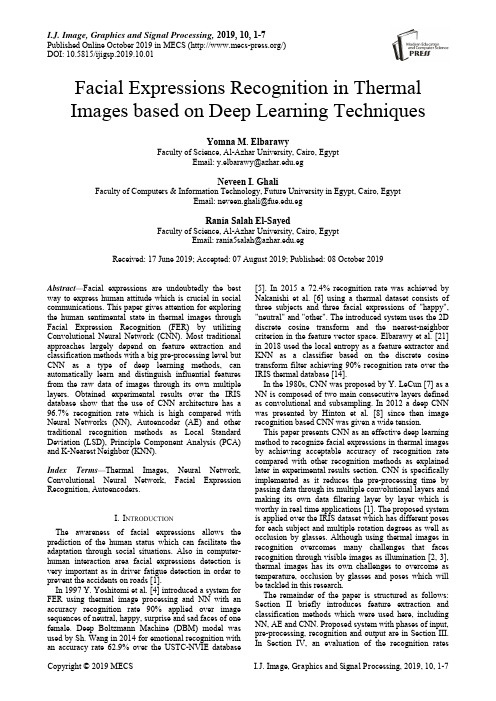
I.J. Image, Graphics and Signal Processing, 2019, 10, 1-7Published Online October 2019 in MECS (/)DOI: 10.5815/ijigsp.2019.10.01Facial Expressions Recognition in Thermal Images based on Deep Learning TechniquesYomna M. ElbarawyFaculty of Science, Al-Azhar University, Cairo, EgyptEmail: y.elbarawy@.egNeveen I. GhaliFaculty of Computers & Information Technology, Future University in Egypt, Cairo, EgyptEmail: neveen.ghali@.egRania Salah El-SayedFaculty of Science, Al-Azhar University, Cairo, EgyptEmail: rania5salah@.egReceived: 17 June 2019; Accepted: 07 August 2019; Published: 08 October 2019Abstract—Facial expressions are undoubtedly the best way to express human attitude which is crucial in social communications. This paper gives attention for exploring the human sentimental state in thermal images through Facial Expression Recognition (FER) by utilizing Convolutional Neural Network (CNN). Most traditional approaches largely depend on feature extraction and classification methods with a big pre-processing level but CNN as a type of deep learning methods, can automatically learn and distinguish influential features from the raw data of images through its own multiple layers. Obtained experimental results over the IRIS database show that the use of CNN architecture has a 96.7% recognition rate which is high compared with Neural Networks (NN), Autoencoder (AE) and other traditional recognition methods as Local Standard Deviation (LSD), Principle Component Analysis (PCA) and K-Nearest Neighbor (KNN).Index Terms—Thermal Images, Neural Network, Convolutional Neural Network, Facial Expression Recognition, Autoencoders.I.I NTRODUCTIONThe awareness of facial expressions allows the prediction of the human status which can facilitate the adaptation through social situations. Also in computer-human interaction area facial expressions detection is very important as in driver fatigue detection in order to prevent the accidents on roads [1].In 1997 Y. Yoshitomi et al. [4] introduced a system for FER using thermal image processing and NN with an accuracy recognition rate 90% applied over image sequences of neutral, happy, surprise and sad faces of one female. Deep Boltzmann Machine (DBM) model was [5]. In 2015 a 72.4% recognition rate was achieved by Nakanishi et al. [6] using a thermal dataset consists of three subjects and three facial expressions of "happy", "neutral" and "other". The introduced system uses the 2D discrete cosine transform and the nearest-neighbor criterion in the feature vector space. Elbarawy et al. [21] in 2018 used the local entropy as a feature extractor and KNN as a classifier based on the discrete cosine transform filter achieving 90% recognition rate over the IRIS thermal database [14].In the 1980s, CNN was proposed by Y. LeCun [7] as a NN is composed of two main consecutive layers defined as convolutional and subsampling. In 2012 a deep CNN was presented by Hinton et al. [8] since then image recognition based CNN was given a wide tension.This paper presents CNN as an effective deep learning method to recognize facial expressions in thermal images by achieving acceptable accuracy of recognition rate compared with other recognition methods as explained later in experimental results section. CNN is specifically implemented as it reduces the pre-processing time by passing data through its multiple convolutional layers and making its own data filtering layer by layer which is worthy in real time applications [1]. The proposed system is applied over the IRIS dataset which has different poses for each subject and multiple rotation degrees as well as occlusion by glasses. Although using thermal images in recognition overcomes many challenges that faces recognition through visible images as illumination [2, 3], thermal images has its own challenges to overcome as temperature, occlusion by glasses and poses which will be tackled in this research.The remainder of the paper is structured as follows: Section II briefly introduces feature extraction and classification methods which were used here, including NN, AE and CNN. Proposed system with phases of input, pre-processing, recognition and output are in Section III.under different factors as network structure and pre-processing is illustrated. Finally, conclusions and results analysis are presented in Section V.II. P RELIMINARIESThis section briefly debates classic neural network, AEs and CNN applied on facial expression thermal images data to recognize expressions. A. Neural Networks Based FERNNs have two learning types, supervised and unsupervised techniques [9, 10]. This system uses the supervised technique feedforward with backpropagation training neural network [11] to train and produces a desired output as shown in Fig. 1. Number of inputimages x i are ∑x i 60i=1 and the number of the hidden layer neurons are adjusted according to the recognition accuracy. The numbers of decision classes are 3 denoting different facial expressions in the used dataset.Fig.1. NN based facial expression recognition.Scaled conjugate gradient backpropagation was used torecognize the facial expressions [12] and (1) represents the cross entropy function used for calculating the network error [13].C (X |Y )=−1n ∑y (i)ln (o(x (i)))+(1−y (i))ln(1−o(x (i)))n i=1(1)Where X ={x (1).…. x (n)} are the set of input images in the training data, Y ={y (1).…. y (n)} are the corresponding labels of input examples and o(x (i)) is the output of the neural network given input x i calculated as in (2)o =f(∑x (i)w (i))n i=1 (2)Where w i is the network weight for input x i . Neural network is introduced in algorithm 1. Algorithm 1: Neural Network Algorithm 1) Input pre-processed thermal images.2) Propagate forward through the network andrandomly initiate weights.3) Generate the output expressions.4) Calculate the network error using cross-entropyEquation 1.5) Re-adjust weights using (3).∆w (i)=rCx (i) (3)Where r is defined to be learning rate with proposed value 0.016) Goto 2 until acceptable output accuracy is reached B. Deep Autoencoder Neural Networks Based FER Autoencoder neural networks is an unsupervised learning for features extraction. It sets the output nodes with same dimensions as the input nodes. Therefore, a training goal is created which does not depend on existing labels but on the training data itself. This made an unsupervised optimization of the full neural network [16]. As in general deep learning models, AEs read the input data images as a matrix or array of images. AEs mainly has two parts encoders αand decoders β transited as in (4)α:X →Y.β:Y →X (4)Where X is the input vector and Y is the output one. The lower dimensional feature vector A is represented by (5).A =f(∑Wx +b) (5)Where W associated weight is vector with the input unit and hidden unit, b is the bias associated with the hidden unit.Networks can use more than one AE for feature extraction. Features extracted by the first AE behaves as an input for the second AE and so on. Finally, classification is done at the softmax layer which unlike AEs its training is supervised using the training data labels. The softmax layer uses a softmax function to calculate the probabilities distribution of the images over different expressions. Architecture of AEs network is illustrated in Fig. 2.The predicted probability for the j tℎclass given a sample vector x and a weighting vector w is given by (6).P (y =j |x )=ex T w j ∑ex T w n N n=1 (6)Where x Tw denotes the inner product of xand w.Fig.2. Autoencoders architecture of input vector X with length n.C. Convolutional Neural Network Based FERCNN differs from general deep learning models as it can directly accept 2D images as the input data, so that it has a unique advantage in the field of image recognition [18]. A four-layer CNN architecture designed to be applied over the used dataset, including two convolutional layers (C1, C2) and two subsampling layers (S1, S2). Finally, a Softmax classifier is used for image classification. General network architecture is illustrated in Fig. 3.Fig.3. General convolutional neural network architecture.Where C1 and C2 are used with different number of convolutions and kernel sizes. After each convolutional operation an additional operation is used called Rectified Linear Unit (ReLU) which is applied per pixel and replaces every negative pixel values in the feature map by zero value. Subsampling processes minimize the resolution of the functional map by max-pooling which takes the maximum response within the domestic features map size of the input (which is always the output of the convolutional layer) and reached a definite degree of invariance to deformity in the input [19]. At the fully connected layer, the output unit activation of the network made by softmax function which calculates the probability distribution of K different possible outcomes. After training, the network uses the cross entropy to indicate the distance between the experimental output and the expected output [20].III.F ACIAL E XPRESSION R ECOGNITION S YSTEM U SINGC NNA. System OverviewThis section introduces CNN based facial expression recognition system. The system flow is shown in Fig. 4 where the input thermal images dataset under test is manipulated to detect face to reduce noise and unify size of images before feature extraction. CNN is implemented for feature extraction as discussed previously. From the extracted classes the analysis and accuracy is calculated. Details of each module are stated below.Fig.4. Scheme for facial expression recognition systemB. IRIS DatasetThe proposed system is applied over IRIS dataset [14], the standard facial expression dataset in the OCTBVS database which contain images in bitmap RGB format. The database contains thermal and visible images of 30 subject (28 males and 2 females) with size 320x240, collected by the long wave IR Camera (Thermal-Raythoen Palm IR-Pro) at the University of Tennessee having uneven illuminations and different poses. Each subject has three different expressions Surprise, Happy and Angry. Fig. 5 has samples of the visible thermal images in IRIS dataset with different rotations.Fig.5. IRIS: different rotation sample images.C. Images Pre-processingThe system uses 90 images (60 for training and 30 for testing) with different rotations, as well as occlusion by glasses and poses. Only poses less than 45° rotation were selected.Image Pre-processing was done to reduce unnecessaryregions in the original images through two main steps: First, face detection and extracting useful regions of the face and neglecting other images parts which hold non-essential background information using Viola-Jones algorithm [15]. Fig. 6 shows samples of detected faces with different expressions. Second step was resizing extracted faces with size 120x120 and preparing a two matrices one for training images and the other for the testing images.Fig.6. Sample of detected faces with different expressions.D. Feature Extraction Based CNNProposed CNN applied over pre-processed images to extract features. CNN was robust for expression recognition with different number of convolutions and kernel sizes. The architecture of our CNN is illustrated in Fig. 7.Fig.7. Proposed CNN architecture.The architecture includes two convolutional layers, two sub-sampling layers and one fully connected layer. The network has an input of 120x120 grayscale images and outputs the credit of each expression. The first layer of the CNN is a convolution layer its objective is to extract elementary visual features as corners and borders of face parts, applies a convolution kernel size of 3x3 with stride of 1 horizontally and vertically and outputs 12 images with size 116x116 pixels. This layer is trailed by a sub-sampling layer that utilizes max-pooling with kernel size 2x2 at stride 2 to lessen the image to half of its size, outputs 12 images with size 58x58 pixels. Therefore, a new convolution layer performs 36 convolutions with a 3x3 kernel size and stride of 1 to guide of the past layer and trailed by another sub-sampling, with a 2x2 kernel size at stride 2, their aim is to perform the same operations as earlier, but to handle features at a lower level, perceiving contextual elements rather than borders E. Output ModuleThe output module holds classification results and recognition rates which are calculated as explained earlier in section II. The general output consists of three classes, one for each expression. In case of applying CNN the outputs were given to a fully connected hidden layer that classifies images using the softmax function earlier in (6). The same process is done for NN and AE as feature extraction and classification techniques to be compared with CNN.IV.E XPERIMENTAL R ESULTSThree models were applied over the selected data to show how to overcome the challenge of thermal images as temperature, occlusion by glasses and poses.First, NN model applied over the selected data andrecognition accuracy of applying neural network with multiple different number of neurons 4, 6, 8, 10, 12 and 14. At 8 neurons testing recognition accuracy was the best which gives a 93.3% recognition rate.Table 1. Neural network recognition accuracy.Second model applied AE neural networks as a feature extraction and classification method. Applying different network structures could cause a great impact on the recognition rates hence a variant number of hidden layers were tested. Two level structured network were used and testing results are in Table 2. The maximum recognition rate was made when number of the hidden neurons was 16 and 32 for first level and 8 for the second, with testing accuracy 90%. Table 3 includes processing time for applying each structure which implicates that time is directly proportional to the number of hidden layers in each level.Table 2. Recognition accuracy (%) using AEs.The third applied model used a CNN with two convolutional layers. Convolutional layer one (C1) applied with different number of features map (4, 8,12 and 16) with size 3x3. The second convolutional layer (C2) applied with different number of features map (12, 24 and 36) with size 3x3 and both layers trained with 50 epochs, obtained results are shown in Table 4 with the highest recognition rate being 96.7% in testing. Table 5 shows the processing time for each applied CNN structure. related to the hardware specification which the proposed system uses. This study used a system has 64 bit operating system with 4GB RAMs and processor speed 2.20 GHz.Table 3. AEs processing time in seconds.Table 4. Recognition accuracy (%) using CNN.Table 5. CNN processing time in seconds.Table 6. Overall accuracy results for recognition.The overall results appear in Table 6 imply that using CNNs for expressions recognition in thermal images achieve high recognition rate with 96.7% in less time compared with other recognition methods (AE and NN), since it is easier to train with the pooling operations for down sampling and its many fewer parameters than stacked AEs with the same number of hidden units. Average processing time of deep recognition methods is illustrated in Fig. 8. Table 7 clarifies the confusion matrix of using the proposed architecture of CNN with accuracy 96.7%. Also illustrates the True Positive (TP) and False Negative (FN) rates respectively, where all images with happy and angry expressions are recognized with 100% accuracy. Surprised faces recognized with 90% TP rate, the other 10% FN rate confused with happiness expression.Table 7. Confusion matrix of CNN with accuracy (96.7%).Fig.8. Average elapsed time of recognition methods based deep learning.In real systems the subject image can be taken from a far distance which will make it hard to recognize. In order to add another challenge to the proposed system here, data augmentation was used to simulate the earlier difficulty by using a set of scaled images (horizontally and vertically). All training images were scaled by a randomly selected factor from the range vector [1 2]. Applying the same earlier CNN structure over the augmented images 10 times running for training and testing the average recognition rate was 70.9%.V.C ONCLUSIONGenerally, experience and continuous testing is reliable to get the best network structure for a particular classification task. This paper holds two main approaches of conducted results, the first approach uses traditional NN for feature extraction and classification achieving 93.3% recognition rate. Second approach applies deep learning techniques as AEs and CNNs over the selected data. AEs has the longest processing time and lowest recognition rate with 90%.A standard neural network with the same number of features as in CNN has more parameters, resulting an additional noise during the training process and larger memory requirements. CNN used the same features across the image in different locations at the convolution layer, thus immensely reducing the memory requirement. Therefore, the implementation of a standard neural system identical to a CNN will be permanently poorer. The proposed CNN architecture as a deep supervised learner of features, detects facial expressions in thermal images with high recognition accuracy and less time compared with other deep learning model AE achieving 96.7%. In future, other architecturesmay be experimented to produce a higher accuracy.R EFERENCES[1]S. Naz, Sh. Ziauddin and A. R. Shahid, "Driver FatigueDetection using Mean Intensity, SVM and SIFT", International Journal of Interactive Multimedia and Artificial Intelligence, In press, pp. 1 - 8, 2017.[2] F. Z. Salmam, A. Madani and M. Kissi, "EmotionRecognition from Facial Expression Based on Fiducial Points Detection and Using Neural Network", International Journal of Electrical and Computer Engineering (IJECE), Vol. 8(1), pp. 52-59, 2018.[3]Y. Wang, X. Yang and J. Zou, "Research of EmotionRecognition Based on Speech and Facial Expression", TELKOMNIKA (Telecommunication, Computing, Electronics and Control), Vol. 11(1), pp. 83-90, 2013. [4]Y. Yoshitomi, N. Miyawaki, S. Tomita and S. Kimura,"Facial expression recognition using thermal image processing and neural network", 6th IEEE International Workshop on Robot and Human Communication, Sendai, Japan, pp. 380- 385, 1997.[5]Sh. Wang, M. He, Z. Gao, Sh. He and Q. Ji, "Emotionrecognition from thermal infrared images using deep Boltzmann machine", Front. Comput. Sci., Vol. 8(4), pp.609-618, 2014.[6]Y. Nakanishi, Y. Yoshitomi, T. Asada et al., "Facialexpression recognition using thermal image processing and efficient preparation of training-data", Journal of Robotics, Networking and Artificial Life, Vol. 2(2), pp.79-84, 2015.[7]Y. Lecun, "Generalization and Network DesignStrategies", Pfeifer, Schreter, Fogelman and Steels (eds)’Connectionism in perspective’, Elsevier, 1989. [8] A. Krizhevsky, I. Sutskever, and G. E. Hinton, "ImageNetclassification with deep convolutional neural networks".Advances in Neural Information Processing Systems (NIPS), pp. 1106-1114, 2012.[9]X. Liao and J. Yu, "Robust stability for interval Hopfieldneural networks with time delay", IEEE Transactions on Neural Networks, Vol. 9(5), pp. 1042–1045, 1998. [10]J. J. Hopfield, "Neurons with graded response havecollective computational properties like these of two-state neurons", Proceedings of the National Academy of Sciences, USA, Vol. 81(10), pp. 3088–3092, 1984. [11]R. Amardeep and Dr. K T. Swamy, "TrainingFeedforward Neural Network with Backpropogation Algorithm", International Journal of Engineering and Computer Science, Vol. 6(1), pp. 19860-19866, 2017. [12]M. F. Møller, "A scaled conjugate gradient algorithm forfast supervised learning", Neural Networks, Vol. 6(4), pp.525-533, 1993.[13]G. E. Nasr, E.A. Badr and C. Joun, "Cross Entropy ErrorFunction in Neural Networks: Forecasting Gasoline Demand", Proceedings of the Fifteenth International Florida Artificial Intelligence Research Society Conference, Florida, USA, pp. 381-384, 2002.[14]University of Tennessee: IRIS thermal-visible facedatabase: /pbvs/bench/, last accessed Jul. 2018.[15]P. Viola and M. Jones, "Rapid object detection using aboosted cascade of simple features", Proceedings of the 2001 IEEE Computer Society Conference on Computer Vision and Pattern Recognition, Vol. 1, Kauai, HI, USA, 2001.[16]U. Schmid, J. Günther and K. Diepold, "StackedDenoising and Stacked Convolutional Autoencoders. An Evaluation of Transformation Robustness for Spatial Data Representations", Technical Report, Technische Universität München, Munich, Germany, 2017.[17] B. Leng, S. Guo, X. Zhang, and Z. Xiong, "3d objectretrievalwith stacked local convolutional Autoencoder", Signal Processing, Vol. 112, pp. 119–128, 2015.[18]K. Shan, J. Guo, W. You, D. Lu and R. Bie, "AutomaticFacial Expression Recognition Based on a Deep Convolutional-Neural-Network Structure", Proceeding of IEEE 15th International Conference on Software Engineering Research, Management and Applications (SERA), London, UK, pp. 123-128, 2017. [19]Y. Yang, J. Yang, N. Xu and W. Han, "Learning 3D-FilterMap for Deep Convolutional Neural Networks", Proceeding of the 31st Conference on Neural Information Processing Systems (NIPS 2017), Long Beach, CA, USA, 2017.[20] A. Ruiz-Garcia, M. Elshaw, A. Altahhan and V. Palade,"Stacked Deep Convolutional Auto-Encoders for Emotion Recognition from Facial Expressions", International Joint Conference on Neural Networks (IJCNN), Anchorage, Alaska, pp. 1586-1593, 2017.[21]Y. M. Elbarawy, R. S. El-sayed and N. I. Ghali, "LocalEntropy and Standard Deviation for Facial Expressions Recognition in Thermal Imaging" Bulletin of Electrical Engineering and Informatics, Vol. 7(4), pp. 580-586, 2018. Authors’ ProfilesYomna M. Elbarawy received her B.Sc.and M.Sc. degrees in computer sciencefrom the Faculty of Science, Al-AzharUniversity, Cairo, Egypt in 2008 and2014 respectively. She is currently a Ph.D. student at the same university. Herresearch areas are social networksanalysis, computational intelligence,machine learning and deep learning technologies.Rania Salah El-Sayed is lecturer in the department of Mathematics & Computer Science, Faculty of science, Al-Azhar University, Cairo. Egypt. She received her Ph.D and M.Sc in Pattern Recognition and Network Security from Al-Azhar University in 2013 and 2009 respectively. Her B.Sc degree in Math & Computer Science was received in 2004 from Al-Azhar University. In 2012, she received CCNP security certification from Cisco. Her research interests include pattern recognition, machine learning & network security.Neveen I. Ghali received her B.Sc. from the Faculty of Science, Ain Shams University, Cairo, Egypt. Finished her M.Sc. and Ph.D. degrees in computer science from Faculty of Computers and Information, Helwan University, Cairo, Egypt in 1999 and 2003 respectively. She is currently a Professor in computer science and vice dean, Faculty of Computers and Information Technology in Future University in Egypt. Her research areas are artificial intelligence, computational intelligence and machine learning applications.How to cite this paper:Yomna M. Elbarawy, Neveen I. Ghali, Rania Salah El-Sayed, " Facial Expressions Recognition in Thermal Images based on Deep Learning Techniques", International Journal of Image, Graphics andSignal Processing(IJIGSP), Vol.11, No.10, pp. 1-7, 2019.DOI: 10.5815/ijigsp.2019.10.01。
人脸识别技术在人脸情绪识别中的应用

人脸识别技术在人脸情绪识别中的应用人类情绪是一种复杂而多变的心理状态,它能够通过面部表情来体现出来。
而人脸识别技术的迅猛发展,为人脸情绪识别提供了新的可能性。
本文将探讨人脸识别技术在人脸情绪识别中的应用,并对其潜在的影响进行分析。
首先,人脸识别技术在人脸情绪识别中的应用可以帮助我们更好地了解人类情绪。
通过分析人脸表情,计算机可以准确地识别出人的情绪状态,从而帮助我们更好地理解他人的情感体验。
这对于心理学研究、情感疾病的诊断以及人际交往等方面都具有重要意义。
例如,在心理学研究中,人脸情绪识别可以帮助研究者更加准确地了解被试者在实验过程中的情绪变化,从而提供更精确的实验数据。
其次,人脸识别技术在人脸情绪识别中的应用可以改善人机交互体验。
随着智能设备的普及和智能家居的发展,人机交互已经成为了我们生活中不可或缺的一部分。
通过人脸情绪识别技术,计算机可以准确地感知用户的情绪状态,并据此做出相应的反应,从而提供更加智能化、个性化的服务。
比如,智能音箱可以通过人脸情绪识别技术了解用户的情绪状态,然后播放适合用户情绪的音乐或提供相应的娱乐活动,从而提升用户的体验。
此外,人脸识别技术在人脸情绪识别中的应用还可以用于安全监控和犯罪预防。
通过人脸情绪识别技术,监控设备可以实时分析人们的情绪状态,从而及时发现异常情况。
例如,当监控摄像头识别到某人的情绪异常(如愤怒、恐惧等),系统可以自动发出警报,并通知相关人员进行处理。
这在公共场所的安全监控和犯罪预防方面具有重要的应用价值。
然而,人脸识别技术在人脸情绪识别中的应用也面临着一些潜在的问题和挑战。
首先,人类情绪是一种复杂而主观的心理状态,不同人对于相同的情绪可能有不同的表现方式。
因此,人脸情绪识别技术在面对这种多样性时可能存在一定的误判率。
其次,人脸情绪识别技术的应用需要涉及到个人隐私和数据安全的问题。
如果人脸情绪识别技术被滥用或泄露,可能会给个人带来一定的风险和困扰。
因此,在推广和应用人脸情绪识别技术时,我们需要加强相关的法律法规和隐私保护措施,确保技术的合理、安全使用。