《神经网络与深度学习综述DeepLearning15May2014
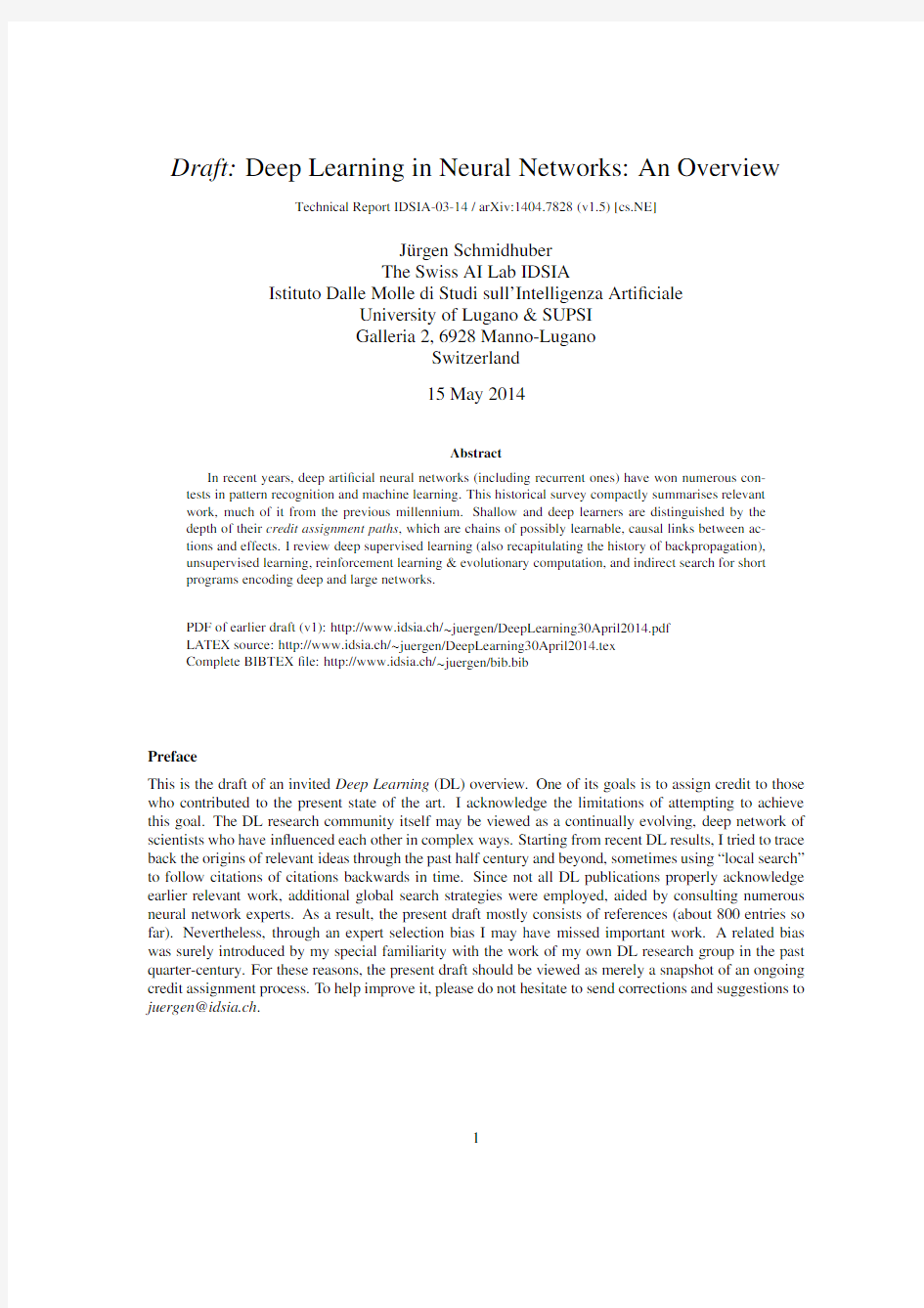
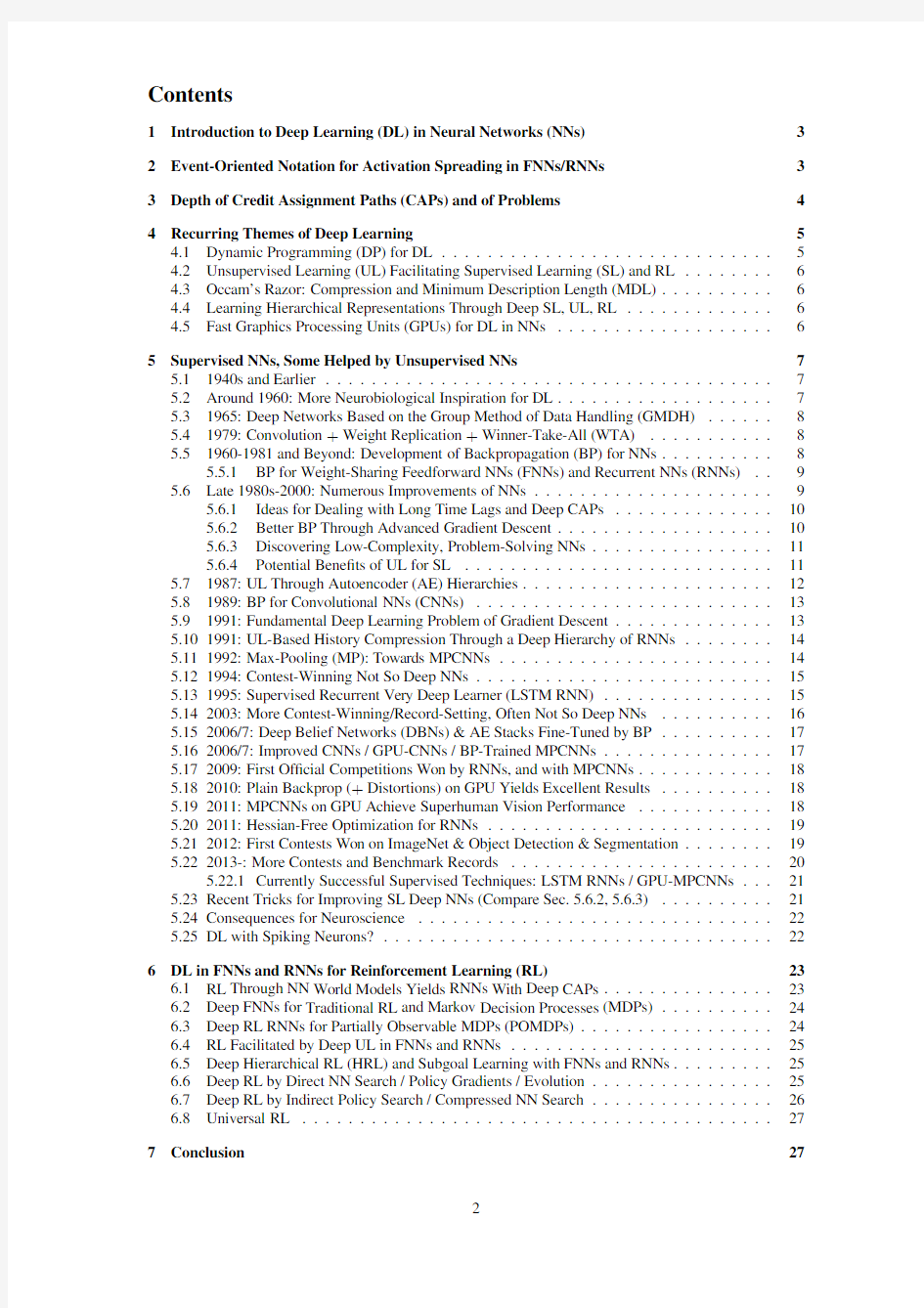
Draft:Deep Learning in Neural Networks:An Overview
Technical Report IDSIA-03-14/arXiv:1404.7828(v1.5)[cs.NE]
J¨u rgen Schmidhuber
The Swiss AI Lab IDSIA
Istituto Dalle Molle di Studi sull’Intelligenza Arti?ciale
University of Lugano&SUPSI
Galleria2,6928Manno-Lugano
Switzerland
15May2014
Abstract
In recent years,deep arti?cial neural networks(including recurrent ones)have won numerous con-tests in pattern recognition and machine learning.This historical survey compactly summarises relevant
work,much of it from the previous millennium.Shallow and deep learners are distinguished by the
depth of their credit assignment paths,which are chains of possibly learnable,causal links between ac-
tions and effects.I review deep supervised learning(also recapitulating the history of backpropagation),
unsupervised learning,reinforcement learning&evolutionary computation,and indirect search for short
programs encoding deep and large networks.
PDF of earlier draft(v1):http://www.idsia.ch/~juergen/DeepLearning30April2014.pdf
LATEX source:http://www.idsia.ch/~juergen/DeepLearning30April2014.tex
Complete BIBTEX?le:http://www.idsia.ch/~juergen/bib.bib
Preface
This is the draft of an invited Deep Learning(DL)overview.One of its goals is to assign credit to those who contributed to the present state of the art.I acknowledge the limitations of attempting to achieve this goal.The DL research community itself may be viewed as a continually evolving,deep network of scientists who have in?uenced each other in complex ways.Starting from recent DL results,I tried to trace back the origins of relevant ideas through the past half century and beyond,sometimes using“local search”to follow citations of citations backwards in time.Since not all DL publications properly acknowledge earlier relevant work,additional global search strategies were employed,aided by consulting numerous neural network experts.As a result,the present draft mostly consists of references(about800entries so far).Nevertheless,through an expert selection bias I may have missed important work.A related bias was surely introduced by my special familiarity with the work of my own DL research group in the past quarter-century.For these reasons,the present draft should be viewed as merely a snapshot of an ongoing credit assignment process.To help improve it,please do not hesitate to send corrections and suggestions to juergen@idsia.ch.
Contents
1Introduction to Deep Learning(DL)in Neural Networks(NNs)3 2Event-Oriented Notation for Activation Spreading in FNNs/RNNs3 3Depth of Credit Assignment Paths(CAPs)and of Problems4 4Recurring Themes of Deep Learning5
4.1Dynamic Programming(DP)for DL (5)
4.2Unsupervised Learning(UL)Facilitating Supervised Learning(SL)and RL (6)
4.3Occam’s Razor:Compression and Minimum Description Length(MDL) (6)
4.4Learning Hierarchical Representations Through Deep SL,UL,RL (6)
4.5Fast Graphics Processing Units(GPUs)for DL in NNs (6)
5Supervised NNs,Some Helped by Unsupervised NNs7
5.11940s and Earlier (7)
5.2Around1960:More Neurobiological Inspiration for DL (7)
5.31965:Deep Networks Based on the Group Method of Data Handling(GMDH) (8)
5.41979:Convolution+Weight Replication+Winner-Take-All(WTA) (8)
5.51960-1981and Beyond:Development of Backpropagation(BP)for NNs (8)
5.5.1BP for Weight-Sharing Feedforward NNs(FNNs)and Recurrent NNs(RNNs)..9
5.6Late1980s-2000:Numerous Improvements of NNs (9)
5.6.1Ideas for Dealing with Long Time Lags and Deep CAPs (10)
5.6.2Better BP Through Advanced Gradient Descent (10)
5.6.3Discovering Low-Complexity,Problem-Solving NNs (11)
5.6.4Potential Bene?ts of UL for SL (11)
5.71987:UL Through Autoencoder(AE)Hierarchies (12)
5.81989:BP for Convolutional NNs(CNNs) (13)
5.91991:Fundamental Deep Learning Problem of Gradient Descent (13)
5.101991:UL-Based History Compression Through a Deep Hierarchy of RNNs (14)
5.111992:Max-Pooling(MP):Towards MPCNNs (14)
5.121994:Contest-Winning Not So Deep NNs (15)
5.131995:Supervised Recurrent Very Deep Learner(LSTM RNN) (15)
5.142003:More Contest-Winning/Record-Setting,Often Not So Deep NNs (16)
5.152006/7:Deep Belief Networks(DBNs)&AE Stacks Fine-Tuned by BP (17)
5.162006/7:Improved CNNs/GPU-CNNs/BP-Trained MPCNNs (17)
5.172009:First Of?cial Competitions Won by RNNs,and with MPCNNs (18)
5.182010:Plain Backprop(+Distortions)on GPU Yields Excellent Results (18)
5.192011:MPCNNs on GPU Achieve Superhuman Vision Performance (18)
5.202011:Hessian-Free Optimization for RNNs (19)
5.212012:First Contests Won on ImageNet&Object Detection&Segmentation (19)
5.222013-:More Contests and Benchmark Records (20)
5.22.1Currently Successful Supervised Techniques:LSTM RNNs/GPU-MPCNNs (21)
5.23Recent Tricks for Improving SL Deep NNs(Compare Sec.5.6.2,5.6.3) (21)
5.24Consequences for Neuroscience (22)
5.25DL with Spiking Neurons? (22)
6DL in FNNs and RNNs for Reinforcement Learning(RL)23
6.1RL Through NN World Models Yields RNNs With Deep CAPs (23)
6.2Deep FNNs for Traditional RL and Markov Decision Processes(MDPs) (24)
6.3Deep RL RNNs for Partially Observable MDPs(POMDPs) (24)
6.4RL Facilitated by Deep UL in FNNs and RNNs (25)
6.5Deep Hierarchical RL(HRL)and Subgoal Learning with FNNs and RNNs (25)
6.6Deep RL by Direct NN Search/Policy Gradients/Evolution (25)
6.7Deep RL by Indirect Policy Search/Compressed NN Search (26)
6.8Universal RL (27)
7Conclusion27
1Introduction to Deep Learning(DL)in Neural Networks(NNs) Which modi?able components of a learning system are responsible for its success or failure?What changes to them improve performance?This has been called the fundamental credit assignment problem(Minsky, 1963).There are general credit assignment methods for universal problem solvers that are time-optimal in various theoretical senses(Sec.6.8).The present survey,however,will focus on the narrower,but now commercially important,sub?eld of Deep Learning(DL)in Arti?cial Neural Networks(NNs).We are interested in accurate credit assignment across possibly many,often nonlinear,computational stages of NNs.
Shallow NN-like models have been around for many decades if not centuries(Sec.5.1).Models with several successive nonlinear layers of neurons date back at least to the1960s(Sec.5.3)and1970s(Sec.5.5). An ef?cient gradient descent method for teacher-based Supervised Learning(SL)in discrete,differentiable networks of arbitrary depth called backpropagation(BP)was developed in the1960s and1970s,and ap-plied to NNs in1981(Sec.5.5).BP-based training of deep NNs with many layers,however,had been found to be dif?cult in practice by the late1980s(Sec.5.6),and had become an explicit research subject by the early1990s(Sec.5.9).DL became practically feasible to some extent through the help of Unsupervised Learning(UL)(e.g.,Sec.5.10,5.15).The1990s and2000s also saw many improvements of purely super-vised DL(Sec.5).In the new millennium,deep NNs have?nally attracted wide-spread attention,mainly by outperforming alternative machine learning methods such as kernel machines(Vapnik,1995;Sch¨o lkopf et al.,1998)in numerous important applications.In fact,supervised deep NNs have won numerous of-?cial international pattern recognition competitions(e.g.,Sec.5.17,5.19,5.21,5.22),achieving the?rst superhuman visual pattern recognition results in limited domains(Sec.5.19).Deep NNs also have become relevant for the more general?eld of Reinforcement Learning(RL)where there is no supervising teacher (Sec.6).
Both feedforward(acyclic)NNs(FNNs)and recurrent(cyclic)NNs(RNNs)have won contests(Sec.
5.12,5.14,5.17,5.19,5.21,5.22).In a sense,RNNs are the deepest of all NNs(Sec.3)—they are general computers more powerful than FNNs,and can in principle create and process memories of ar-bitrary sequences of input patterns(e.g.,Siegelmann and Sontag,1991;Schmidhuber,1990a).Unlike traditional methods for automatic sequential program synthesis(e.g.,Waldinger and Lee,1969;Balzer, 1985;Soloway,1986;Deville and Lau,1994),RNNs can learn programs that mix sequential and parallel information processing in a natural and ef?cient way,exploiting the massive parallelism viewed as crucial for sustaining the rapid decline of computation cost observed over the past75years.
The rest of this paper is structured as follows.Sec.2introduces a compact,event-oriented notation that is simple yet general enough to accommodate both FNNs and RNNs.Sec.3introduces the concept of Credit Assignment Paths(CAPs)to measure whether learning in a given NN application is of the deep or shallow type.Sec.4lists recurring themes of DL in SL,UL,and RL.Sec.5focuses on SL and UL,and on how UL can facilitate SL,although pure SL has become dominant in recent competitions(Sec.5.17-5.22). Sec.5is arranged in a historical timeline format with subsections on important inspirations and technical contributions.Sec.6on deep RL discusses traditional Dynamic Programming(DP)-based RL combined with gradient-based search techniques for SL or UL in deep NNs,as well as general methods for direct and indirect search in the weight space of deep FNNs and RNNs,including successful policy gradient and evolutionary methods.
2Event-Oriented Notation for Activation Spreading in FNNs/RNNs Throughout this paper,let i,j,k,t,p,q,r denote positive integer variables assuming ranges implicit in the given contexts.Let n,m,T denote positive integer constants.
An NN’s topology may change over time(e.g.,Fahlman,1991;Ring,1991;Weng et al.,1992;Fritzke, 1994).At any given moment,it can be described as a?nite subset of units(or nodes or neurons)N= {u1,u2,...,}and a?nite set H?N×N of directed edges or connections between nodes.FNNs are acyclic graphs,RNNs cyclic.The?rst(input)layer is the set of input units,a subset of N.In FNNs,the k-th layer(k>1)is the set of all nodes u∈N such that there is an edge path of length k?1(but no longer path)between some input unit and u.There may be shortcut connections between distant layers.
The NN’s behavior or program is determined by a set of real-valued,possibly modi?able,parameters or weights w i(i=1,...,n).We now focus on a single?nite episode or epoch of information processing and activation spreading,without learning through weight changes.The following slightly unconventional notation is designed to compactly describe what is happening during the runtime of the system.
During an episode,there is a partially causal sequence x t(t=1,...,T)of real values that I call events.Each x t is either an input set by the environment,or the activation of a unit that may directly depend on other x k(k winning NNs(Sec.5.19,5.21,5.22)there also are events of the type x t=max k∈in t (x k);some network types may also use complex polynomial activation functions(Sec.5.3).x t may directly affect certain x k(k>t)through outgoing connections or links represented through a current set out t of indices k with t∈in k.Some non-input events are called output events. Note that many of the x t may refer to different,time-varying activations of the same unit in sequence-processing RNNs(e.g.,Williams,1989,“unfolding in time”),or also in FNNs sequentially exposed to time-varying input patterns of a large training set encoded as input events.During an episode,the same weight may get reused over and over again in topology-dependent ways,e.g.,in RNNs,or in convolutional NNs(Sec.5.4,5.8).I call this weight sharing across space and/or time.Weight sharing may greatly reduce the NN’s descriptive complexity,which is the number of bits of information required to describe the NN (Sec.4.3). In Supervised Learning(SL),certain NN output events x t may be associated with teacher-given,real-valued labels or targets d t yielding errors e t,e.g.,e t=1/2(x t?d t)2.A typical goal of supervised NN training is to?nd weights that yield episodes with small total error E,the sum of all such e t.The hope is that the NN will generalize well in later episodes,causing only small errors on previously unseen sequences of input events.Many alternative error functions for SL and UL are possible. SL assumes that input events are independent of earlier output events(which may affect the environ-ment through actions causing subsequent perceptions).This assumption does not hold in the broader?elds of Sequential Decision Making and Reinforcement Learning(RL)(Kaelbling et al.,1996;Sutton and Barto, 1998;Hutter,2005)(Sec.6).In RL,some of the input events may encode real-valued reward signals given by the environment,and a typical goal is to?nd weights that yield episodes with a high sum of reward signals,through sequences of appropriate output actions. Sec.5.5will use the notation above to compactly describe a central algorithm of DL,namely,back-propagation(BP)for supervised weight-sharing FNNs and RNNs.(FNNs may be viewed as RNNs with certain?xed zero weights.)Sec.6will address the more general RL case. 3Depth of Credit Assignment Paths(CAPs)and of Problems To measure whether credit assignment in a given NN application is of the deep or shallow type,I introduce the concept of Credit Assignment Paths or CAPs,which are chains of possibly causal links between events. Let us?rst focus on SL.Consider two events x p and x q(1≤p More general,possibly indirect,Potential Causal Connections(PCC)are expressed by the recursively de?ned Boolean predicate pcc(p,q),which in the SL case is true only if pdcc(p,q),or if pcc(p,k)for some k and pdcc(k,q).In the latter case,appending q to any CAP from p to k yields a CAP from p to q(this is a recursive de?nition,too).The set of such CAPs may be large but is?nite.Note that the same weight may affect many different PDCCs between successive events listed by a given CAP,e.g.,in the case of RNNs, or weight-sharing FNNs. Suppose a CAP has the form(...,k,t,...,q),where k and t(possibly t=q)are the?rst successive elements with modi?able w v(k,t).Then the length of the suf?x list(t,...,q)is called the CAP’s depth (which is0if there are no modi?able links at all).This depth limits how far backwards credit assignment can move down the causal chain to?nd a modi?able weight.1 Suppose an episode and its event sequence x1,...,x T satisfy a computable criterion used to decide whether a given problem has been solved(e.g.,total error E below some threshold).Then the set of used weights is called a solution to the problem,and the depth of the deepest CAP within the sequence is called the solution’s depth.There may be other solutions(yielding different event sequences)with different depths.Given some?xed NN topology,the smallest depth of any solution is called the problem’s depth. Sometimes we also speak of the depth of an architecture:SL FNNs with?xed topology imply a problem-independent maximal problem depth bounded by the number of non-input layers.Certain SL RNNs with?xed weights for all connections except those to output units(Jaeger,2001;Maass et al.,2002; Jaeger,2004;Schrauwen et al.,2007)have a maximal problem depth of1,because only the?nal links in the corresponding CAPs are modi?able.In general,however,RNNs may learn to solve problems of potentially unlimited depth. Note that the de?nitions above are solely based on the depths of causal chains,and agnostic of the temporal distance between events.For example,shallow FNNs perceiving large“time windows”of in-put events may correctly classify long input sequences through appropriate output events,and thus solve shallow problems involving long time lags between relevant events. At which problem depth does Shallow Learning end,and Deep Learning begin?Discussions with DL experts have not yet yielded a conclusive response to this question.Instead of committing myself to a precise answer,let me just de?ne for the purposes of this overview:problems of depth>10require Very Deep Learning. The dif?culty of a problem may have little to do with its depth.Some NNs can quickly learn to solve certain deep problems,e.g.,through random weight guessing(Sec.5.9)or other types of direct search (Sec.6.6)or indirect search(Sec.6.7)in weight space,or through training an NN?rst on shallow problems whose solutions may then generalize to deep problems,or through collapsing sequences of(non)linear operations into a single(non)linear operation—but see an analysis of non-trivial aspects of deep linear networks(Baldi and Hornik,1994,Section B).In general,however,?nding an NN that precisely models a given training set is an NP-complete problem(Judd,1990;Blum and Rivest,1992),also in the case of deep NNs(S′?ma,1994;de Souto et al.,1999;Windisch,2005);compare a survey of negative results(S′?ma, 2002,Section1). Above we have focused on SL.In the more general case of RL in unknown environments,pcc(p,q) is also true if x p is an output event and x q any later input event—any action may affect the environment and thus any later perception.(In the real world,the environment may even in?uence non-input events computed on a physical hardware entangled with the entire universe,but this is ignored here.)It is possible to model and replace such unmodi?able environmental PCCs through a part of the NN that has already learned to predict(through some of its units)input events(including reward signals)from former input events and actions(Sec.6.1).Its weights are frozen,but can help to assign credit to other,still modi?able weights used to compute actions(Sec.6.1).This approach may lead to very deep CAPs though. Some DL research is about automatically rephrasing problems such that their depth is reduced(Sec.4). In particular,sometimes UL is used to make SL problems less deep,e.g.,Sec.5.10.Often Dynamic Programming(Sec.4.1)is used to facilitate certain traditional RL problems,e.g.,Sec.6.2.Sec.5focuses on CAPs for SL,Sec.6on the more complex case of RL. 4Recurring Themes of Deep Learning 4.1Dynamic Programming(DP)for DL One recurring theme of DL is Dynamic Programming(DP)(Bellman,1957),which can help to facili-tate credit assignment under certain assumptions.For example,in SL NNs,backpropagation itself can 1An alternative would be to count only modi?able links when measuring depth.In many typical NN applications this would not make a difference,but in some it would,e.g.,Sec.6.1. be viewed as a DP-derived method(Sec.5.5).In traditional RL based on strong Markovian assumptions, DP-derived methods can help to greatly reduce problem depth(Sec.6.2).DP algorithms are also essen-tial for systems that combine concepts of NNs and graphical models,such as Hidden Markov Models (HMMs)(Stratonovich,1960;Baum and Petrie,1966)and Expectation Maximization(EM)(Dempster et al.,1977),e.g.,(Bottou,1991;Bengio,1991;Bourlard and Morgan,1994;Baldi and Chauvin,1996; Jordan and Sejnowski,2001;Bishop,2006;Poon and Domingos,2011;Dahl et al.,2012;Hinton et al., 2012a). 4.2Unsupervised Learning(UL)Facilitating Supervised Learning(SL)and RL Another recurring theme is how UL can facilitate both SL(Sec.5)and RL(Sec.6).UL(Sec. 5. 6.4) is normally used to encode raw incoming data such as video or speech streams in a form that is more convenient for subsequent goal-directed learning.In particular,codes that describe the original data in a less redundant or more compact way can be fed into SL(Sec.5.10,5.15)or RL machines(Sec.6.4),whose search spaces may thus become smaller(and whose CAPs shallower)than those necessary for dealing with the raw data.UL is closely connected to the topics of regularization and compression(Sec.4.3,5.6.3). 4.3Occam’s Razor:Compression and Minimum Description Length(MDL) Occam’s razor favors simple solutions over complex ones.Given some programming language,the prin-ciple of Minimum Description Length(MDL)can be used to measure the complexity of a solution candi-date by the length of the shortest program that computes it(e.g.,Solomonoff,1964;Kolmogorov,1965b; Chaitin,1966;Wallace and Boulton,1968;Levin,1973a;Rissanen,1986;Blumer et al.,1987;Li and Vit′a nyi,1997;Gr¨u nwald et al.,2005).Some methods explicitly take into account program runtime(Al-lender,1992;Watanabe,1992;Schmidhuber,2002,1995);many consider only programs with constant runtime,written in non-universal programming languages(e.g.,Rissanen,1986;Hinton and van Camp, 1993).In the NN case,the MDL principle suggests that low NN weight complexity corresponds to high NN probability in the Bayesian view(e.g.,MacKay,1992;Buntine and Weigend,1991;De Freitas,2003), and to high generalization performance(e.g.,Baum and Haussler,1989),without over?tting the training data.Many methods have been proposed for regularizing NNs,that is,searching for solution-computing, low-complexity SL NNs(Sec.5.6.3)and RL NNs(Sec.6.7).This is closely related to certain UL methods (Sec.4.2,5.6.4). 4.4Learning Hierarchical Representations Through Deep SL,UL,RL Many methods of Good Old-Fashioned Arti?cial Intelligence(GOFAI)(Nilsson,1980)as well as more recent approaches to AI(Russell et al.,1995)and Machine Learning(Mitchell,1997)learn hierarchies of more and more abstract data representations.For example,certain methods of syntactic pattern recog-nition(Fu,1977)such as grammar induction discover hierarchies of formal rules to model observations. The partially(un)supervised Automated Mathematician/EURISKO(Lenat,1983;Lenat and Brown,1984) continually learns concepts by combining previously learnt concepts.Such hierarchical representation learning(Ring,1994;Bengio et al.,2013;Deng and Yu,2014)is also a recurring theme of DL NNs for SL (Sec.5),UL-aided SL(Sec.5.7,5.10,5.15),and hierarchical RL(Sec.6.5).Often,abstract hierarchical representations are natural by-products of data compression(Sec.4.3),e.g.,Sec.5.10. 4.5Fast Graphics Processing Units(GPUs)for DL in NNs While the previous millennium saw several attempts at creating fast NN-speci?c hardware(e.g.,Jackel et al.,1990;Faggin,1992;Ramacher et al.,1993;Widrow et al.,1994;Heemskerk,1995;Korkin et al., 1997;Urlbe,1999),and at exploiting standard hardware(e.g.,Anguita et al.,1994;Muller et al.,1995; Anguita and Gomes,1996),the new millennium brought a DL breakthrough in form of cheap,multi-processor graphics cards or GPUs.GPUs are widely used for video games,a huge and competitive market that has driven down hardware prices.GPUs excel at fast matrix and vector multiplications required not only for convincing virtual realities but also for NN training,where they can speed up learning by a factor of50and more.Some of the GPU-based FNN implementations(Sec.5.16-5.19)have greatly contributed to recent successes in contests for pattern recognition(Sec.5.19-5.22),image segmentation(Sec.5.21), and object detection(Sec.5.21-5.22). 5Supervised NNs,Some Helped by Unsupervised NNs The main focus of current practical applications is on Supervised Learning(SL),which has dominated re-cent pattern recognition contests(Sec.5.17-5.22).Several methods,however,use additional Unsupervised Learning(UL)to facilitate SL(Sec.5.7,5.10,5.15).It does make sense to treat SL and UL in the same section:often gradient-based methods,such as BP(Sec.5.5.1),are used to optimize objective functions of both UL and SL,and the boundary between SL and UL may blur,for example,when it comes to time series prediction and sequence classi?cation,e.g.,Sec.5.10,5.12. A historical timeline format will help to arrange subsections on important inspirations and techni-cal contributions(although such a subsection may span a time interval of many years).Sec.5.1brie?y mentions early,shallow NN models since the1940s,Sec.5.2additional early neurobiological inspiration relevant for modern Deep Learning(DL).Sec.5.3is about GMDH networks(since1965),perhaps the?rst (feedforward)DL systems.Sec.5.4is about the relatively deep Neocognitron NN(1979)which is similar to certain modern deep FNN architectures,as it combines convolutional NNs(CNNs),weight pattern repli-cation,and winner-take-all(WTA)mechanisms.Sec.5.5uses the notation of Sec.2to compactly describe a central algorithm of DL,namely,backpropagation(BP)for supervised weight-sharing FNNs and RNNs. It also summarizes the history of BP1960-1981and beyond.Sec.5.6describes problems encountered in the late1980s with BP for deep NNs,and mentions several ideas from the previous millennium to overcome them.Sec.5.7discusses a?rst hierarchical stack of coupled UL-based Autoencoders(AEs)—this concept resurfaced in the new millennium(Sec.5.15).Sec.5.8is about applying BP to CNNs,which is important for today’s DL applications.Sec.5.9explains BP’s Fundamental DL Problem(of vanishing/exploding gradients)discovered in1991.Sec.5.10explains how a deep RNN stack of1991(the History Compressor) pre-trained by UL helped to solve previously unlearnable DL benchmarks requiring Credit Assignment Paths(CAPs,Sec.3)of depth1000and more.Sec.5.11discusses a particular WTA method called Max-Pooling(MP)important in today’s DL FNNs.Sec.5.12mentions a?rst important contest won by SL NNs in1994.Sec.5.13describes a purely supervised DL RNN(Long Short-Term Memory,LSTM)for problems of depth1000and more.Sec.5.14mentions an early contest of2003won by an ensemble of shallow NNs, as well as good pattern recognition results with CNNs and LSTM RNNs(2003).Sec.5.15is mostly about Deep Belief Networks(DBNs,2006)and related stacks of Autoencoders(AEs,Sec.5.7)pre-trained by UL to facilitate BP-based SL.Sec.5.16mentions the?rst BP-trained MPCNNs(2007)and GPU-CNNs(2006). Sec.5.17-5.22focus on of?cial competitions with secret test sets won by(mostly purely supervised)DL NNs since2009,in sequence recognition,image classi?cation,image segmentation,and object detection. Many RNN results depended on LSTM(Sec.5.13);many FNN results depended on GPU-based FNN code developed since2004(Sec.5.16,5.17,5.18,5.19),in particular,GPU-MPCNNs(Sec.5.19). 5.11940s and Earlier NN research started in the1940s(e.g.,McCulloch and Pitts,1943;Hebb,1949);compare also later work on learning NNs(Rosenblatt,1958,1962;Widrow and Hoff,1962;Grossberg,1969;Kohonen,1972; von der Malsburg,1973;Narendra and Thathatchar,1974;Willshaw and von der Malsburg,1976;Palm, 1980;Hop?eld,1982).In a sense NNs have been around even longer,since early supervised NNs were essentially variants of linear regression methods going back at least to the early1800s(e.g.,Legendre, 1805;Gauss,1809,1821).Early NNs had a maximal CAP depth of1(Sec.3). 5.2Around1960:More Neurobiological Inspiration for DL Simple cells and complex cells were found in the cat’s visual cortex(e.g.,Hubel and Wiesel,1962;Wiesel and Hubel,1959).These cells?re in response to certain properties of visual sensory inputs,such as the orientation of https://www.360docs.net/doc/4210009725.html,plex cells exhibit more spatial invariance than simple cells.This inspired later deep NN architectures(Sec.5.4)used in certain modern award-winning Deep Learners(Sec.5.19-5.22). 5.31965:Deep Networks Based on the Group Method of Data Handling(GMDH) Networks trained by the Group Method of Data Handling(GMDH)(Ivakhnenko and Lapa,1965; Ivakhnenko et al.,1967;Ivakhnenko,1968,1971)were perhaps the?rst DL systems of the Feedforward Multilayer Perceptron type.The units of GMDH nets may have polynomial activation functions imple-menting Kolmogorov-Gabor polynomials(more general than traditional NN activation functions).Given a training set,layers are incrementally grown and trained by regression analysis,then pruned with the help of a separate validation set(using today’s terminology),where Decision Regularisation is used to weed out super?uous units.The numbers of layers and units per layer can be learned in problem-dependent fashion. This is a good example of hierarchical representation learning(Sec.4.4).There have been numerous ap-plications of GMDH-style networks,e.g.(Ikeda et al.,1976;Farlow,1984;Madala and Ivakhnenko,1994; Ivakhnenko,1995;Kondo,1998;Kord′?k et al.,2003;Witczak et al.,2006;Kondo and Ueno,2008). 5.41979:Convolution+Weight Replication+Winner-Take-All(WTA) Apart from deep GMDH networks(Sec.5.3),the Neocognitron(Fukushima,1979,1980,2013a)was per-haps the?rst arti?cial NN that deserved the attribute deep,and the?rst to incorporate the neurophysiolog-ical insights of Sec.5.2.It introduced convolutional NNs(today often called CNNs or convnets),where the(typically rectangular)receptive?eld of a convolutional unit with given weight vector is shifted step by step across a2-dimensional array of input values,such as the pixels of an image.The resulting2D array of subsequent activation events of this unit can then provide inputs to higher-level units,and so on.Due to massive weight replication(Sec.2),relatively few parameters may be necessary to describe the behavior of such a convolutional layer. Competition layers have WTA subsets whose maximally active units are the only ones to adopt non-zero activation values.They essentially“down-sample”the competition layer’s input.This helps to create units whose responses are insensitive to small image shifts(compare Sec.5.2). The Neocognitron is very similar to the architecture of modern,contest-winning,purely super-vised,feedforward,gradient-based Deep Learners with alternating convolutional and competition lay-ers(e.g.,Sec.5.19-5.22).Fukushima,however,did not set the weights by supervised backpropagation (Sec.5.5,5.8),but by local un supervised learning rules(e.g.,Fukushima,2013b),or by pre-wiring.In that sense he did not care for the DL problem(Sec.5.9),although his architecture was comparatively deep indeed.He also used Spatial Averaging(Fukushima,1980,2011)instead of Max-Pooling(MP,Sec.5.11), currently a particularly convenient and popular WTA mechanism.Today’s CNN-based DL machines pro?t a lot from later CNN work(e.g.,LeCun et al.,1989;Ranzato et al.,2007)(Sec.5.8,5.16,5.19). 5.51960-1981and Beyond:Development of Backpropagation(BP)for NNs The minimisation of errors through gradient descent(Hadamard,1908)in the parameter space of com-plex,nonlinear,differentiable,multi-stage,NN-related systems has been discussed at least since the early 1960s(e.g.,Kelley,1960;Bryson,1961;Bryson and Denham,1961;Pontryagin et al.,1961;Dreyfus,1962; Wilkinson,1965;Amari,1967;Bryson and Ho,1969;Director and Rohrer,1969;Griewank,2012),ini-tially within the framework of Euler-LaGrange equations in the Calculus of Variations(e.g.,Euler,1744). Steepest descent in such systems can be performed(Bryson,1961;Kelley,1960;Bryson and Ho,1969)by iterating the ancient chain rule(Leibniz,1676;L’H?o pital,1696)in Dynamic Programming(DP)style(Bell-man,1957).A simpli?ed derivation of the method uses the chain rule only(Dreyfus,1962). The methods of the1960s were already ef?cient in the DP sense.However,they backpropagated derivative information through standard Jacobian matrix calculations from one“layer”to the previous one, explicitly addressing neither direct links across several layers nor potential additional ef?ciency gains due to network sparsity(but perhaps such enhancements seemed obvious to the authors). Given all the prior work on learning in multilayer NN-like systems(compare also Sec.5.3),it seems surprising in hindsight that a book(Minsky and Papert,1969)on the limitations of simple linear perceptrons (Sec.5.1)discouraged some researchers from further studying NNs. Explicit,ef?cient error backpropagation(BP)in arbitrary,discrete,possibly sparsely connected,NN-like networks apparently was?rst described in a1970master’s thesis(Linnainmaa,1970,1976),albeit without reference to NNs.BP is also known as the reverse mode of automatic differentiation(Griewank, 2012),where the costs of forward activation spreading essentially equal the costs of backward derivative calculation.See early FORTRAN code(Linnainmaa,1970);see also(Ostrovskii et al.,1971).Ef?cient BP was soon explicitly used to minimize cost functions by adapting control parameters(weights)(Dreyfus, 1973).Compare some NN-speci?c discussion(Werbos,1974,section5.5.1),a method for multilayer threshold NNs(Bobrowski,1978),and a computer program for automatically deriving and implementing BP for given differentiable systems(Speelpenning,1980). To my knowledge,the?rst NN-speci?c application of ef?cient BP was described in1981(Werbos, 1981,2006).See also(Parker,1985;LeCun,1985,1988).A paper of1986signi?cantly contributed to the popularisation of BP(Rumelhart et al.,1986).See generalisations for sequence-processing recur-rent NNs(e.g.,Williams,1989;Robinson and Fallside,1987;Werbos,1988;Williams and Zipser,1988, 1989b,a;Rohwer,1989;Pearlmutter,1989;Gherrity,1989;Williams and Peng,1990;Schmidhuber,1992a; Pearlmutter,1995;Baldi,1995;Kremer and Kolen,2001;Atiya and Parlos,2000),also for equilibrium RNNs(Almeida,1987;Pineda,1987)with stationary inputs.See also natural gradients(Amari,1998). 5.5.1BP for Weight-Sharing Feedforward NNs(FNNs)and Recurrent NNs(RNNs) Using the notation of Sec.2for weight-sharing FNNs or RNNs,after an episode of activation spreading through differentiable f t,a single iteration of gradient descent through BP computes changes of all w i in proportion to?E ?w i = t?E?net t?net t?w i as in Algorithm5.5.1(for the additive case),where each weight w i is associated with a real-valued variable△i initialized by0. Alg.5.5.1:One iteration of BP for weight-sharing FNNs or RNNs for t=T,...,1do to compute?E ?net t ,inititalize real-valued error signal variableδt by0; if x t is an input event then continue with next iteration; if there is an error e t thenδt:=x t?d t; add toδt the value k∈out t w v(t,k)δk;(this is the elegant and ef?cient recursive chain rule application collecting impacts of net t on future events) multiplyδt by f′t(net t); for all k∈in t add to△w v(k,t)the value x kδt end for Finally,to?nish one iteration of steepest descent,change all w i in proportion to△i and a small learning rate.The computational costs of the backward(BP)pass are essentially those of the forward pass(Sec.2). Forward and backward passes are re-iterated until suf?cient performance is reached. As of2013,this simple BP method is still the central learning algorithm for FNNs and RNNs.Notably, most contest-winning NNs up to2013(Sec.5.12,5.14,5.17,5.19,5.21,5.22)did not augment supervised BP by some sort of un supervised learning as discussed in Sec.5.7,5.10,5.15. 5.6Late1980s-2000:Numerous Improvements of NNs By the late1980s it seemed clear that BP by itself(Sec.5.5)was no panacea.Most FNN applications focused on FNNs with few hidden layers.Many practitioners found solace in a theorem(Kolmogorov, 1965a;Hecht-Nielsen,1989;Hornik et al.,1989)stating that an NN with a single layer of enough hidden units can approximate any multivariate continous function with arbitrary accuracy.Additional hidden layers often did not seem to offer empirical bene?ts. Likewise,most RNN applications did not require backpropagating errors far.Many researchers helped their RNNs by?rst training them on shallow problems(Sec.3)whose solutions then generalized to deeper problems.In fact,some popular RNN algorithms restricted credit assignment to a single step back-wards(Elman,1990;Jordan,1986,1997),also in more recent studies(Jaeger,2002;Maass et al.,2002; Jaeger,2004). Generally speaking,although BP allows for deep problems in principle,it seemed to work only for shallow problems.The late1980s and early1990s saw a few ideas with a potential to overcome this problem,which was fully understood only in1991(Sec.5.9). 5.6.1Ideas for Dealing with Long Time Lags and Deep CAPs To deal with long time lags between relevant events,several sequence processing methods were pro-posed,including Focused BP based on decay factors for activations of units in RNNs(Mozer,1989,1992), Time-Delay Neural Networks(TDNNs)(Lang et al.,1990)and their adaptive extension(Bodenhausen and Waibel,1991),Nonlinear AutoRegressive with eXogenous inputs(NARX)RNNs(Lin et al.,1995,1996), certain hierarchical RNNs(Hihi and Bengio,1996),RL economies in RNNs with WTA units and local learning rules(Schmidhuber,1989b),and other methods(e.g.,Ring,1993,1994;Plate,1993;de Vries and Principe,1991;Sun et al.,1993a;Bengio et al.,1994).However,these algorithms either worked for shal-low CAPs only,could not generalize to unseen CAP depths,had problems with greatly varying time lags between relevant events,needed external?ne tuning of delay constants,or suffered from other problems.In fact,it turned out that certain simple but deep benchmark problems used to evaluate such methods are more quickly solved by randomly guessing RNN weights until a solution is found(Hochreiter and Schmidhuber, 1996). While the RNN methods above were designed for DL of temporal sequences,the Neural Heat Ex-changer(Schmidhuber,1990c)consists of two parallel deep FNNs with opposite?ow directions.Input patterns enter the?rst FNN and are propagated“up”.Desired outputs(targets)enter the“opposite”FNN and are propagated“down”.Using a local learning rule,each layer in each net tries to be similar(in infor-mation content)to the preceding layer and to the adjacent layer of the other net.The input entering the?rst net slowly“heats up”to become the target.The target entering the opposite net slowly“cools down”to become the input.The Helmholtz Machine(Dayan et al.,1995;Dayan and Hinton,1996)may be viewed as an unsupervised(Sec.5.6.4)variant thereof(Peter Dayan,personal communication,1994). A hybrid approach(Shavlik and Towell,1989;Towell and Shavlik,1994)initializes a potentially deep FNN through a domain theory in propositional logic,which may be acquired through explanation-based learning(Mitchell et al.,1986;DeJong and Mooney,1986;Minton et al.,1989).The NN is then?ne-tuned through BP(Sec.5.5).The NN’s depth re?ects the longest chain of reasoning in the original set of logical rules.An extension of this approach(Maclin and Shavlik,1993;Shavlik,1994)initializes an RNN by domain knowledge expressed as a Finite State Automaton(FSA).BP-based?ne-tuning has become important for later DL systems pre-trained by UL,e.g.,Sec.5.10,5.15. 5.6.2Better BP Through Advanced Gradient Descent Numerous improvements of steepest descent through BP(Sec.5.5)have been proposed.Least-squares methods(Gauss-Newton,Levenberg-Marquardt)(Levenberg,1944;Marquardt,1963)and quasi-Newton methods(Broyden-Fletcher-Goldfarb-Shanno,BFGS)(Broyden et al.,1965;Fletcher and Powell,1963; Goldfarb,1970;Shanno,1970)are computationally too expensive for large NNs.Partial BFGS(Battiti, 1992;Saito and Nakano,1997)and conjugate gradient(Hestenes and Stiefel,1952;M?ller,1993)as well as other methods(Solla,1988;Schmidhuber,1989a;Cauwenberghs,1993)provide sometimes useful fast alternatives.BP can be treated as a linear least-squares problem(Biegler-K¨o nig and B¨a rmann,1993), where second-order gradient information is passed back to preceding layers. To speed up BP,momentum was introduced(Rumelhart et al.,1986),ad-hoc constants were added to the slope of the linearized activation function(Fahlman,1988),or the nonlinearity of the slope was exaggerated(West and Saad,1995).Only the signs of the error derivatives are taken into account by the successful and widely used BP variant R-prop(Riedmiller and Braun,1993). The local gradient can be normalized based on the NN architecture(Schraudolph and Sejnowski,1996), through a diagonalized Hessian approach(Becker and Le Cun,1989),or related ef?cient methods(Schrau-dolph,2002). Some algorithms for controlling BP step size adapt a global learning rate(Lapedes and Farber,1986; V ogl et al.,1988;Battiti,1989;LeCun et al.,1993;Yu et al.,1995),others compute individual learning rates for each weight(Jacobs,1988;Silva and Almeida,1990).In online learning,where BP is applied af-ter each pattern presentation,the vario-ηalgorithm(Neuneier and Zimmermann,1996)sets each weight’s learning rate inversely proportional to the empirical standard deviation of its local gradient,thus normal-izing the stochastic weight?https://www.360docs.net/doc/4210009725.html,pare a local online step size adaptation method for nonlinear NNs(Almeida et al.,1997). Many additional tricks for improving NNs have been described(e.g.,Orr and M¨u ller,1998;Montavon et al.,2012).Compare Sec.5.6.3and recent developments mentioned in Sec.5.23. 5.6.3Discovering Low-Complexity,Problem-Solving NNs Many researchers used BP-like methods to search for simple,low-complexity NNs(Sec.4.3)with high generalization capability.Most approaches address the bias/variance dilemma(Geman et al.,1992)through strong prior assumptions.For example,weight decay(Hanson and Pratt,1989;Weigend et al.,1991; Krogh and Hertz,1992)encourages small weights,by penalizing large weights.In a Bayesian framework, weight decay can be derived from Gaussian or Laplace weight priors(Hinton and van Camp,1993);see also(Murray and Edwards,1993).An extension of this approach postulates that a distribution of networks with many similar weights generated by Gaussian mixtures is“better”a priori(Nowlan and Hinton,1992). Often weight priors are implicit in additional penalty terms(MacKay,1992)or in methods based on validation sets(Mosteller and Tukey,1968;Stone,1974;Eubank,1988;Hastie and Tibshirani,1990; Craven and Wahba,1979;Golub et al.,1979),Akaike’s information criterion and?nal prediction er-ror(Akaike,1970,1973,1974),or generalized prediction error(Moody and Utans,1994;Moody,1992). See also(Holden,1994;Wang et al.,1994;Amari and Murata,1993;Wang et al.,1994;Guyon et al.,1992; Vapnik,1992;Wolpert,1994). Similar priors are implicit in constructive and pruning algorithms,e.g.,layer-by-layer sequential net-work construction(Fahlman,1991;Ash,1989;Moody,1989;Gallant,1986;Utgoff and Stracuzzi,2002), input pruning(Moody,1992;Refenes et al.,1994),unit pruning(White,1989;Mozer and Smolensky, 1989;Levin et al.,1994),weight pruning,e.g.,optimal brain damage(LeCun et al.,1990b),and optimal brain surgeon(Hassibi and Stork,1993). A very general but not always practical approach for discovering low-complexity SL NNs or RL NNs searches among weight matrix-computing programs written in a universal programming language,with a bias towards fast and short programs(Schmidhuber,1995,1997)(Sec.6.7). Flat Minimum Search(FMS)(Hochreiter and Schmidhuber,1997a,1999)searches for a“?at”min-imum of the error function:a large connected region in weight space where error is low and remains approximately constant,that is,few bits of information are required to describe low-precision weights with high https://www.360docs.net/doc/4210009725.html,pare perturbation tolerance conditions(Minai and Williams,1994;Murray and Ed-wards,1993;Neti et al.,1992;Matsuoka,1992;Bishop,1993;Kerlirzin and Vallet,1993;Carter et al., 1990).An MDL-based,Bayesian argument suggests that?at minima correspond to“simple”NNs and low expected over?https://www.360docs.net/doc/4210009725.html,pare Sec.5.6.4and more recent developments mentioned in Sec.5.23. 5.6.4Potential Bene?ts of UL for SL The SL notation of Sec.2focused on teacher-given labels d t.Many papers of the previous millennium, however,were about unsupervised learning(UL)without a teacher(e.g.,Hebb,1949;von der Malsburg, 1973;Kohonen,1972,1982,1988;Willshaw and von der Malsburg,1976;Grossberg,1976a,b;Watanabe, 1985;Rumelhart and Zipser,1986;Pearlmutter and Hinton,1986;Barrow,1987;Field,1987;Oja,1989; Barlow et al.,1989;Baldi and Hornik,1989;Rubner and Tavan,1989;Sanger,1989;Ritter and Koho-nen,1989;Rubner and Schulten,1990;F¨o ldi′a k,1990;Martinetz et al.,1990;Kosko,1990;Mozer,1991; Palm,1992;Atick et al.,1992;Schmidhuber and Prelinger,1993;Miller,1994;Saund,1994;F¨o ldi′a k and Young,1995;Deco and Parra,1997);see also post-2000work(e.g.,Klapper-Rybicka et al.,2001; Carreira-Perpinan,2001;Wiskott and Sejnowski,2002;Franzius et al.,2007;Waydo and Koch,2008). Many UL methods are designed to maximize information-theoretic objectives(e.g.,Linsker,1988; Barlow et al.,1989;MacKay and Miller,1990;Plumbley,1991;Schmidhuber,1992b,c;Schraudolph and Sejnowski,1993;Redlich,1993;Zemel,1993;Zemel and Hinton,1994;Field,1994;Hinton et al.,1995; Dayan and Zemel,1995;Amari et al.,1996;Deco and Parra,1997).Many do this to uncover and disen-tangle hidden underlying sources of signals(e.g.,Jutten and Herault,1991;Schuster,1992;Andrade et al., 1993;Molgedey and Schuster,1994;Comon,1994;Cardoso,1994;Bell and Sejnowski,1995;Karhunen and Joutsensalo,1995;Belouchrani et al.,1997;Hyv¨a rinen et al.,2001;Szab′o et al.,2006). Many UL methods automatically and robustly generate distributed,sparse representations of input pat-terns(F¨o ldi′a k,1990;Hinton and Ghahramani,1997;Lewicki and Olshausen,1998;Hyv¨a rinen et al.,1999; Hochreiter and Schmidhuber,1999;Falconbridge et al.,2006)through well-known feature detectors(e.g., Olshausen and Field,1996;Schmidhuber et al.,1996),such as off-center-on-surround-like structures,as well as orientation sensitive edge detectors and Gabor?lters(Gabor,1946).They extract simple features related to those observed in early visual pre-processing stages of biological systems(e.g.,De Valois et al., 1982;Jones and Palmer,1987). UL can help to encode input data in a form advantageous for further processing.In the context of DL,one important goal of UL is redundancy reduction.Ideally,given an ensemble of input patterns, redundancy reduction through a deep NN will create a factorial code(a code with statistically independent components)of the ensemble(Barlow et al.,1989;Barlow,1989),to disentangle the unknown factors of variation(compare Bengio et al.,2013).Such codes may be sparse and can be advantageous for(1)data compression,(2)speeding up subsequent BP(Becker,1991),(3)trivialising the task of subsequent naive yet optimal Bayes classi?ers(Schmidhuber et al.,1996). Most early UL FNNs had a single layer.Methods for deeper UL FNNs include hierarchical self-organizing maps(e.g.,Koikkalainen and Oja,1990;Lampinen and Oja,1992;Versino and Gambardella, 1996;Dittenbach et al.,2000;Rauber et al.,2002),hierarchical Gaussian potential function networks(Lee and Kil,1991),the Self-Organising Tree Algorithm(SOTA)(Herrero et al.,2001),and nonlinear Autoen-coders(AEs)with more than3(e.g.,5)layers(Kramer,1991;Oja,1991;DeMers and Cottrell,1993). Such an AE NN(Rumelhart et al.,1986)can be trained to map input patterns to themselves,for example, by compactly encoding them through activations of units of a narrow bottleneck hidden layer.See(Baldi, 2012)for limitations of certain nonlinear AEs. Other nonlinear UL methods include Predictability Minimization(PM)(Schmidhuber,1992c),where nonlinear feature detectors?ght nonlinear predictors,trying to become both informative and as unpre-dictable as possible,and L OCOCODE(Hochreiter and Schmidhuber,1999),where FMS(Sec.5.6.3)?nds low-complexity AEs with low-precision weights describable by few bits of information,often yielding sparse or factorial codes. 5.71987:UL Through Autoencoder(AE)Hierarchies Perhaps the?rst work to study potential bene?ts of UL-based pre-training was published in1987.It proposed unsupervised AE hierarchies(Ballard,1987),closely related to certain post-2000feedforward Deep Learners based on UL(Sec.5.15).The lowest-level AE NN with a single hidden layer is trained to map input patterns to themselves.Its hidden layer codes are then fed into a higher-level AE of the same type,and so on.The hope is that the codes in the hidden AE layers have properties that facilitate subsequent learning.In one experiment,a particular AE-speci?c learning algorithm(different from traditional BP of Sec.5.5.1)was used to learn a mapping in an AE stack pre-trained by this type of UL(Ballard,1987).This was faster than learning an equivalent mapping by BP through a single deeper AE without pre-training. On the other hand,the task did not really require a deep AE,that is,the bene?ts of UL were not that obvious from this https://www.360docs.net/doc/4210009725.html,pare an early survey(Hinton,1989)and the somewhat related Recursive Auto-Associative Memory(RAAM)(Pollack,1988,1990;Melnik et al.,2000),originally used to encode linguistic structures,but later also as an unsupervised pre-processor to facilitate deep credit assignment for RL(Gisslen et al.,2011)(Sec.6.4). In principle,many UL methods(Sec.5.6.4)could be stacked like the AEs above,the history-compressing RNNs of Sec.5.10,the Restricted Boltzmann Machines(RBMs)of Sec.5.15,or hierarchical Kohonen nets(Sec.5.6.4),to facilitate subsequent https://www.360docs.net/doc/4210009725.html,pare Stacked Generalization(Wolpert,1992; Ting and Witten,1997),and FNNs that pro?t from pre-training by competitive UL(Rumelhart and Zipser, 1986)prior to BP-based?ne-tuning(Maclin and Shavlik,1995). 5.81989:BP for Convolutional NNs(CNNs) In1989,backpropagation(Sec.5.5)was applied(LeCun et al.,1989,1990a,1998)to weight-sharing convolutional neural layers with adaptive connections(compare Sec.5.4).This combination,augmented by max-pooling(Sec.5.11,5.16),and sped up on graphics cards(Sec.5.19),has become an essential ingredient of many modern,competition-winning,feedforward,visual Deep Learners(Sec.5.19-5.21). This work also introduced the MNIST data set of handwritten digits(LeCun et al.,1989),which over time has become perhaps the most famous benchmark of Machine https://www.360docs.net/doc/4210009725.html,Ns helped to achieve good performance on MNIST(LeCun et al.,1990a)(CAP depth5)and on?ngerprint recognition(Baldi and Chauvin,1993);similar CNNs were used commercially in the1990s. 5.91991:Fundamental Deep Learning Problem of Gradient Descent A diploma thesis(Hochreiter,1991)represented a milestone of explicit DL research.As mentioned in Sec.5.6,by the late1980s,experiments had indicated that traditional deep feedforward or recurrent net-works are hard to train by backpropagation(BP)(Sec.5.5).Hochreiter’s work formally identi?ed a major reason:Typical deep NNs suffer from the now famous problem of vanishing or exploding gradients.With standard activation functions(Sec.1),cumulative backpropagated error signals(Sec.5.5.1)either shrink rapidly,or grow out of bounds.In fact,they decay exponentially in the number of layers or CAP depth (Sec.3),or they explode.This is also known as the long time lag problem.Much subsequent DL re-search of the1990s and2000s was motivated by this https://www.360docs.net/doc/4210009725.html,pare(Bengio et al.,1994),who also studied basins of attraction and their stability under noise from a dynamical systems point of view:either the dynamics are not robust to noise,or the gradients vanish.See also(Hochreiter et al.,2001a;Tiˇn o and Hammer,2004).Over the years,several ways of partially overcoming the Fundamental Deep Learning Problem were explored: I A Very Deep Learner of1991(the History Compressor,Sec.5.10)alleviates the problem through unsupervised pre-training for a hierarchy of RNNs.This greatly facilitates subsequent supervised credit assignment through BP(Sec.5.5).Compare conceptually related AE stacks(Sec.5.7)and Deep Belief Networks(DBNs)(Sec.5.15)for the FNN case. II LSTM-like networks(Sec.5.13,5.17,5.22)alleviate the problem through a special architecture unaffected by it. III Today’s GPU-based computers have a million times the computational power of desktop machines of the early1990s.This allows for propagating errors a few layers further down within reasonable time, even in traditional NNs(Sec.5.18).That is basically what is winning many of the image recognition competitions now(Sec.5.19,5.21,5.22).(Although this does not really overcome the problem in a fundamental way.) IV Hessian-free optimization(Sec.5.6.2)can alleviate the problem for FNNs(M?ller,1993;Pearlmut-ter,1994;Schraudolph,2002;Martens,2010)(Sec.5.6.2)and RNNs(Martens and Sutskever,2011) (Sec.5.20). V The space of NN weight matrices can also be searched without relying on error gradients,thus avoid-ing the Fundamental Deep Learning Problem altogether.Random weight guessing sometimes works better than more sophisticated methods(Hochreiter and Schmidhuber,1996).Certain more complex problems are better solved by using Universal Search(Levin,1973b)for weight matrix-computing programs written in a universal programming language(Schmidhuber,1995,1997).Some are bet-ter solved by using linear methods to obtain optimal weights for connections to output events,and evolving weights of connections to other events—this is called Evolino(Schmidhuber et al.,2007). Compare related RNNs pre-trained by certain UL rules(Steil,2007),also for the case of spiking neurons(Klamp?and Maass,2013)(Sec.5.25).Direct search methods are relevant not only for SL but also for more general RL,and are discussed in more detail in Sec.6.6. 5.101991:UL-Based History Compression Through a Deep Hierarchy of RNNs A working Very Deep Learner(Sec.3)of1991(Schmidhuber,1992b,2013a)could perform credit as-signment across hundreds of nonlinear operators or neural layers,by using unsupervised pre-training for a stack of RNNs. The basic idea is still relevant today.Each RNN is trained for a while in unsupervised fashion to predict its next input;e.g.,(Connor et al.,1994;Dorffner,1996).From then on,only unexpected inputs(errors) convey new information and get fed to the next higher RNN which thus ticks on a slower,self-organising time scale.It can easily be shown that no information gets lost.It just gets compressed(much of machine learning is essentially about compression,e.g.,Sec.4.3,5.6.3,6.7).For each individual input sequence,we get a series of less and less redundant encodings in deeper and deeper levels of this History Compressor, which can compress data in both space(like feedforward NNs)and time.This is another good example of hierarchical representation learning(Sec.4.4).There also is a continuous variant(Schmidhuber et al., 1993). The RNN stack is essentially a deep generative model of the data,which can be reconstructed from its compressed form.Adding another RNN to the stack improves a bound on the data’s description length—equivalent to the negative logarithm of its probability(Huffman,1952;Shannon,1948)—as long as there is remaining local learnable predictability in the data representation on the corresponding level of the hierarchy. The system was able to learn many previously unlearnable DL tasks.One ancient illustrative DL experiment(Schmidhuber,1993b)required CAPs(Sec.3)of depth1200.The top level code of the ini-tially unsupervised RNN stack,however,got so compact that(previously infeasible)sequence classi?cation through additional BP-based SL became possible.Essentially the system used UL to greatly reduce prob-lem https://www.360docs.net/doc/4210009725.html,pare earlier BP-based?ne-tuning of NNs initialized by rules of propositional logic(Shavlik and Towell,1989)(Sec.5.6.1). There is a way of compressing higher levels down into lower levels,thus fully or partially collapsing the RNN stack.The trick is to retrain a lower-level RNN to continually imitate(predict)the hidden units of an already trained,slower,higher-level RNN(the“conscious”chunker),through additional predictive output neurons(Schmidhuber,1992b).This helps the lower RNN(the“automatizer”)to develop appropriate, rarely changing memories that may bridge very long time lags.Again,this procedure can greatly reduce the required depth of the BP process. The1991system was a working Deep Learner in the modern post-2000sense,and also a?rst Neu-ral Hierarchical Temporal Memory(HTM).It is conceptually similar to previous AE hierarchies(1987, Sec.5.7)and later Deep Belief Networks(2006,Sec.5.15),but more general in the sense that it uses sequence-processing RNNs instead of FNNs with unchanging inputs.More recently,well-known en-trepreneurs(Hawkins and George,2006;Kurzweil,2012)also got interested in HTMs;compare also hier-archical HMMs(e.g.,Fine et al.,1998).Stacks of RNNs were used in later work with great success,e.g., Sec.5.13,5.17,5.22.Clockwork RNNs(Koutn′?k et al.,2014)also consist of interacting RNN modules with different clock rates,but do not require UL to set those rates. 5.111992:Max-Pooling(MP):Towards MPCNNs The Neocognitron(Sec.5.4)inspired the Cresceptron(Weng et al.,1992),which adapts its topology during training;compare the incrementally growing and shrinking GMDH networks(Sec.5.3). Instead of using alternative local WTA methods(e.g.,Fukushima,1980;Schmidhuber,1989b;Maass, 2000;Fukushima,2013a),the Cresceptron uses Max-Pooling(MP)layers.MP is very simple:a2-dimensional layer or array of unit activations is partitioned into smaller rectangular arrays.Each is replaced in a down-sampling layer by the activation of its maximally active unit.A later,more complex version of the Cresceptron(Weng et al.,1997)also included“blurring”layers. The neurophysiologically plausible topology of the feedforward HMAX model(Riesenhuber and Pog-gio,1999)is very similar to the one of the1992Cresceptron.HMAX does not learn though.Its units have hand-crafted weights;biologically plausible learning rules were later proposed for similar models, e.g.,(Serre et al.,2002;Teichmann et al.,2012). When CNNs or convnets(Sec.5.4,5.8)are combined with MP,they become Cresceptron-like or HMAX-like MPCNNs with alternating convolutional and max-pooling layers.Unlike Cresceptron and HMAX,however,MPCNNs are trained by BP(Sec.5.5,5.16)(Ranzato et al.,2007).Advantages of doing this were pointed out subsequently(Scherer et al.,2010).BP-trained MPCNNs have become an essential ingredient of many modern,competition-winning,feedforward,visual Deep Learners(Sec.5.17,5.19-5.21). 5.121994:Contest-Winning Not So Deep NNs Back in the1990s,certain NNs already won certain controlled pattern recognition contests with secret test sets.Notably,an NN with internal delay lines won the Santa Fe time-series competition on chaotic intensity pulsations of an NH3laser(Wan,1994;Weigend and Gershenfeld,1993).No very deep CAPs(Sec.3) were needed though. 5.131995:Supervised Recurrent Very Deep Learner(LSTM RNN) Supervised Long Short-Term Memory(LSTM)RNN(Hochreiter and Schmidhuber,1997b;Gers et al., 2000;P′e rez-Ortiz et al.,2003)could eventually perform similar feats as the deep RNN hierarchy of1991 (Sec.5.10),overcoming the Fundamental Deep Learning Problem(Sec.5.9)without any unsupervised pre-training.LSTM could also learn DL tasks without local sequence predictability(and thus un learnable by the partially unsupervised1991History Compressor,Sec.5.10),dealing with very deep problems(Sec.3), e.g.,(Gers et al.,2002). The basic LSTM idea is very simple.Some of the units are called Constant Error Carrousels(CECs). Each CEC uses as an activation function f,the identity function,and has a connection to itself with?xed weight of1.0.Due to f’s constant derivative of1.0,errors backpropagated through a CEC cannot vanish or explode but stay as they are(unless they“?ow out”of the CEC to other,typically adaptive parts of the NN).CECs are connected to several nonlinear adaptive units(some with multiplicative activation functions)needed for learning nonlinear behavior.Weight changes of these units often pro?t from error signals propagated far back in time through CECs.CECs are the main reason why LSTM nets can learn to discover the importance of(and memorize)events that happened thousands of discrete time steps ago, while previous RNNs already failed in case of minimal time lags of10steps. Many different LSTM variants and topologies are allowed.It is possible to evolve good problem-speci?c topologies(Bayer et al.,2009).Some LSTM variants also use modi?able self-connections of CECs(Gers and Schmidhuber,2001). To a certain extent,LSTM is biologically plausible(O’Reilly,2003).LSTM learned to solve many pre-viously unlearnable DL tasks involving:Recognition of the temporal order of widely separated events in noisy input streams;Robust storage of high-precision real numbers across extended time intervals;Arith-metic operations on continuous input streams;Extraction of information conveyed by the temporal dis-tance between events;Recognition of temporally extended patterns in noisy input sequences(Hochreiter and Schmidhuber,1997b;Gers et al.,2000);Stable generation of precisely timed rhythms,as well as smooth and non-smooth periodic trajectories(Gers and Schmidhuber,2000).LSTM clearly outperformed previous RNNs on tasks that require learning the rules of regular languages describable by determinis-tic Finite State Automata(FSAs)(Watrous and Kuhn,1992;Casey,1996;Siegelmann,1992;Blair and Pollack,1997;Kalinke and Lehmann,1998;Zeng et al.,1994;Manolios and Fanelli,1994;Omlin and Giles,1996;Vahed and Omlin,2004),both in terms of reliability and speed.LSTM also worked better on tasks involving context free languages(CFLs)that cannot be represented by HMMs or similar FSAs discussed in the RNN literature(Sun et al.,1993b;Wiles and Elman,1995;Andrews et al.,1995;Steijvers and Grunwald,1996;Tonkes and Wiles,1997;Rodriguez et al.,1999;Rodriguez and Wiles,1998).CFL recognition(Lee,1996)requires the functional equivalent of a stack.Some previous RNNs failed to learn small CFL training sets(Rodriguez and Wiles,1998).Those that did not(Rodriguez et al.,1999;Bod′e n and Wiles,2000)failed to extract the general rules,and did not generalize well on substantially larger test sets.Similar for context-sensitive languages(CSLs)(e.g.,Chalup and Blair,2003).LSTM generalized well though,requiring only the30shortest exemplars(n≤10)of the CSL a n b n c n to correctly predict the possible continuations of sequence pre?xes for n up to1000and more.A combination of a decoupled extended Kalman?lter(Kalman,1960;Williams,1992b;Puskorius and Feldkamp,1994;Feldkamp et al., 1998;Haykin,2001;Feldkamp et al.,2003)and an LSTM RNN(P′e rez-Ortiz et al.,2003)learned to deal correctly with values of n up to10million and more.That is,after training the network was able to read sequences of30,000,000symbols and more,one symbol at a time,and?nally detect the subtle differences between legal strings such as a10,000,000b10,000,000c10,000,000and very similar but illegal strings such as a10,000,000b9,999,999c10,000,https://www.360docs.net/doc/4210009725.html,pare also more recent RNN algorithms able to deal with long time lags(Sch¨a fer et al.,2006;Martens and Sutskever,2011;Zimmermann et al.,2012;Koutn′?k et al.,2014). Bi-directional RNNs(BRNNs)(Schuster and Paliwal,1997;Schuster,1999)are designed for input sequences whose starts and ends are known in advance,such as spoken sentences to be labeled by their phonemes;compare(Fukada et al.,1999).To take both past and future context of each sequence element into account,one RNN processes the sequence from start to end,the other backwards from end to start. At each time step their combined outputs predict the corresponding label(if there is any).BRNNs were successfully applied to secondary protein structure prediction(Baldi et al.,1999).DAG-RNNs(Baldi and Pollastri,2003;Wu and Baldi,2008)generalize BRNNs to multiple dimensions.They learned to predict properties of small organic molecules(Lusci et al.,2013)as well as protein contact maps(Tegge et al., 2009),also in conjunction with a growing deep FNN(Di Lena et al.,2012)(Sec.5.21).BRNNs and DAG-RNNs unfold their full potential when combined with the LSTM concept(Graves and Schmidhuber,2005, 2009;Graves et al.,2009). Particularly successful in recent competitions are stacks(Sec.5.10)of LSTM RNNs(Fernandez et al., 2007;Graves and Schmidhuber,2009)trained by Connectionist Temporal Classi?cation(CTC)(Graves et al.,2006),a gradient-based method for?nding RNN weights that maximize the probability of teacher-given label sequences,given(typically much longer and more high-dimensional)streams of real-valued input vectors.CTC-LSTM performs simultaneous segmentation(alignment)and recognition(Sec.5.22). In the early2000s,speech recognition was still dominated by HMMs combined with FNNs(e.g., Bourlard and Morgan,1994).Nevertheless,when trained from scratch on utterances from the TIDIG-ITS speech database,in2003LSTM already obtained results comparable to those of HMM-based sys-tems(Graves et al.,2003;Beringer et al.,2005;Graves et al.,2006).A decade later,LSTM achieved best known results on the famous TIMIT phoneme recognition benchmark(Graves et al.,2013)(Sec.5.22).Be-sides speech recognition and keyword spotting(Fern′a ndez et al.,2007),important applications of LSTM include protein analysis(Hochreiter and Obermayer,2005),robot localization(F¨o rster et al.,2007)and robot control(Mayer et al.,2008),handwriting recognition(Graves et al.,2008,2009;Graves and Schmid-huber,2009;Bluche et al.,2014),optical character recognition(Breuel et al.,2013),and others.RNNs can also be used for metalearning(Schmidhuber,1987;Schaul and Schmidhuber,2010;Prokhorov et al.,2002), because they can in principle learn to run their own weight change algorithm(Schmidhuber,1993a).A suc-cessful metalearner(Hochreiter et al.,2001b)used an LSTM RNN to quickly learn a learning algorithm for quadratic functions(compare Sec.6.8). More recently,LSTM RNNs won several international pattern recognition competitions and set bench-mark records on large and complex data sets,e.g.,Sec.5.17,5.21,5.22.Gradient-based LSTM is no panacea though—other methods sometimes outperformed it at least on certain tasks(Jaeger,2004;Schmid-huber et al.,2007;Martens and Sutskever,2011;Pascanu et al.,2013b;Koutn′?k et al.,2014)(compare Sec.5.20). 5.142003:More Contest-Winning/Record-Setting,Often Not So Deep NNs In the decade around2000,many practical and commercial pattern recognition applications were domi-nated by non-neural machine learning methods such as Support Vector Machines(SVMs)(Vapnik,1995; Sch¨o lkopf et al.,1998).Nevertheless,at least in certain domains,NNs outperformed other techniques. A Bayes NN(Neal,2006)based on an ensemble(Breiman,1996;Schapire,1990;Wolpert,1992; Hashem and Schmeiser,1992;Ueda,2000;Dietterich,2000a)of NNs won the NIPS2003Feature Se-lection Challenge with secret test set(Neal and Zhang,2006).The NN was not very deep though—it had two hidden layers and thus rather shallow CAPs(Sec.3)of depth3. Important for many present competition-winning pattern recognisers(Sec.5.19,5.21,5.22)were de-velopments in the CNN department.A BP-trained(LeCun et al.,1989)CNN(Sec.5.4,Sec.5.8)set a new MNIST record of0.4%(Simard et al.,2003),using training pattern deformations(Baird,1990)but no unsupervised pre-training(Sec.5.10,5.15).A standard BP net achieved0.7%(Simard et al.,2003).Again, the corresponding CAP depth was https://www.360docs.net/doc/4210009725.html,pare further improvements in Sec.5.16,5.18,5.19. Good image interpretation results(Behnke,2003)were achieved with rather deep NNs trained by the BP variant R-prop(Riedmiller and Braun,1993)(Sec.5.6.2). Deep LSTM RNNs started to obtain certain?rst speech recognition results comparable to those of HMM-based systems(Graves et al.,2003);compare Sec.5.13,5.21,5.22. 5.152006/7:Deep Belief Networks(DBNs)&AE Stacks Fine-Tuned by BP While DL-relevant networks date back at least to1965(Sec.5.3),and explicit DL research results have been published at least since1991(Sec.5.9,5.10),the expression Deep Learning was actually coined around2006,when unsupervised pre-training of deep FNNs helped to accelerate subsequent SL through BP(Hinton and Salakhutdinov,2006;Hinton et al.,2006).Compare BP-based(Sec.5.5)?ne-tuning of not so deep FNNs pre-trained by competitive UL(Maclin and Shavlik,1995). The Deep Belief Network(DBN)is a stack of Restricted Boltzmann Machines(RBMs)(Smolensky, 1986),which in turn are Boltzmann Machines(BMs)(Hinton and Sejnowski,1986)with a single layer of feature-detecting units;compare also Higher-Order BMs(Memisevic and Hinton,2010).Each RBM perceives pattern representations from the level below and learns to encode them in unsupervised fashion. At least in theory under certain assumptions,adding more layers improves a bound on the data’s negative log probability(Hinton et al.,2006)(equivalent to the data’s description length—compare Sec.5.10).There are extensions for Temporal RBMs(Sutskever et al.,2008). Without any training pattern deformations(Sec.5.14),a DBN?ne-tuned by BP achieved1.2%error rate(Hinton and Salakhutdinov,2006)on the MNIST handwritten digits(Sec.5.8,5.14).This result helped to arouse interest in DBNs.DBNs also achieved good results on phoneme recognition,with an error rate of26.7%on the TIMIT core test set(Mohamed et al.,2009;Mohamed and Hinton,2010);compare further improvements through FNNs(Hinton et al.,2012a;Deng and Yu,2014)and LSTM RNNs(Sec.5.22). A DBN-based technique called Semantic Hashing(Salakhutdinov and Hinton,2009)maps semantically similar documents(of variable size)to nearby addresses in a space of document representations.It outper-formed previous searchers for similar documents,such as Locality Sensitive Hashing(Buhler,2001;Datar et al.,2004). Autoencoder(AE)stacks(Ballard,1987)(Sec.5.7)became a popular alternative way of pre-training deep FNNs in unsupervised fashion,before?ne-tuning them through BP(Sec.5.5)(Bengio et al.,2007; Erhan et al.,2010).Denoising AEs(Vincent et al.,2008)discourage hidden unit perturbations in response to input perturbations,similar to how FMS(Sec.5.6.3)for L OCOCODE AEs(Sec.5.6.4)discourages output perturbations in response to weight perturbations.Sparse coding(Sec.5.6.4)was also formulated as a combination of convex optimization problems(Lee et al.,2007a).A survey(Bengio,2009)of stacked RBM and AE methods focuses on post-2006developments;compare also(Arel et al.,2010).Unsupervised DBNs and AE stacks are conceptually similar to,but in a certain sense less general than,the unsupervised RNN stack-based History Compressor of1991(Sec.5.10),which can process and re-encode not only stationary input patterns,but entire pattern sequences. 5.162006/7:Improved CNNs/GPU-CNNs/BP-Trained MPCNNs Also in2006,a BP-trained(LeCun et al.,1989)CNN(Sec.5.4,Sec.5.8)set a new MNIST record of 0.39%(Ranzato et al.,2006),using training pattern deformations(Sec.5.14)but no unsupervised https://www.360docs.net/doc/4210009725.html,pare further improvements in Sec.5.18,5.19.Similar CNNs were used for off-road obstacle avoidance(LeCun et al.,2006).A combination of CNNs and TDNNs later learned to map?xed-size representations of variable-size sentences to features relevant for language processing,using a combination of SL and UL(Collobert and Weston,2008). 2006also saw an early GPU-based CNN implementation(Chellapilla et al.,2006);compare earlier GPU-FNNs with a reported speed-up factor of20(Oh and Jung,2004).GPUs or graphics cards have become more and more important for DL in subsequent years(Sec.5.18-5.21). In2007,BP(Sec.5.5)was applied(Ranzato et al.,2007)for the?rst time to Neocognitron-inspired (Sec.5.4),Cresceptron-like or HMAX-like MPCNNs(Sec.5.11)with alternating convolutional and max- pooling layers.MPCNNs have become an essential ingredient of many modern,competition-winning, feedforward,visual Deep Learners(Sec.5.17,5.19-5.21). 5.172009:First Of?cial Competitions Won by RNNs,and with MPCNNs Stacks(Sec.5.10)of LSTM RNNs(Fernandez et al.,2007;Graves and Schmidhuber,2009)trained by Connectionist Temporal Classi?cation(CTC)(Graves et al.,2006)(Sec.5.13)became the?rst RNNs to win of?cial international pattern recognition contests(with secret test sets known only to the organisers). More precisely,three connected handwriting competitions at ICDAR2009in three different languages (French,Arab,Farsi)were won by deep LSTM RNNs without any a priori linguistic knowledge,perform-ing simultaneous segmentation and https://www.360docs.net/doc/4210009725.html,pare(Graves and Schmidhuber,2005;Graves et al., 2009;Schmidhuber et al.,2011;Graves et al.,2013)(Sec.5.22). To detect human actions in surveillance videos,a3-dimensional CNN(e.g.,Jain and Seung,2009; Prokhorov,2010),combined with SVMs,was part of a larger system(Yang et al.,2009)using a bag of features approach(Nowak et al.,2006)to extract regions of interest.The system won three2009TRECVID competitions.These were possibly the?rst of?cial international contests won with the help of(MP)CNNs (Sec.5.16).An improved version of the method was published later(Ji et al.,2013). 2009also saw an impressive GPU-DBN implementation(Raina et al.,2009)orders of magnitudes faster than previous CPU-DBNs(see Sec.5.15);see also(Coates et al.,2013).The Convolutional DBN(Lee et al.,2009a)(with a probabilistic variant of MP,Sec.5.11)combines ideas from CNNs and DBNs,and was successfully applied to audio classi?cation(Lee et al.,2009b). 5.182010:Plain Backprop(+Distortions)on GPU Yields Excellent Results In2010,a new MNIST record of0.35%error rate was set by good old BP(Sec.5.5)in deep but otherwise standard NNs(Ciresan et al.,2010),using neither unsupervised pre-training(e.g.,Sec.5.7,5.10,5.15)nor convolution(e.g.,Sec.5.4,5.8,5.14).However,training pattern deformations(e.g.,Sec.5.14)were im-portant to generate a big training set and avoid over?tting.This success was made possible mainly through a GPU implementation of BP that was up to50times faster than standard CPU versions.A good value of0.95%was obtained without distortions except for small saccadic eye movement-like translations—compare Sec.5.15. Since BP was3-5decades old by then(Sec.5.5),and pattern deformations2decades(Baird,1990) (Sec.5.14),these results seemed to suggest that advances in exploiting modern computing hardware were more important than advances in algorithms. 5.192011:MPCNNs on GPU Achieve Superhuman Vision Performance In2011,the?rst GPU-implementation(Ciresan et al.,2011a)of Max-Pooling(MP)CNNs/Convnets was described(the GPU-MPCNN),building on earlier work on MP(Sec.5.11)CNNs(Sec.5.4,5.8,5.16),and on early GPU-based CNNs without MP(Chellapilla et al.,2006)(Sec.5.16);compare GPU-DBNs(Raina et al.,2009)(Sec.5.17)and early GPU-NNs(Oh and Jung,2004).MPCNNs have alternating convolutional layers(Sec.5.4)and max-pooling layers(MP,Sec.5.11)topped by standard fully connected layers.All weights are trained by BP(Sec.5.5,5.8,5.16)(LeCun et al.,1989;Ranzato et al.,2007;Scherer et al., 2010).GPU-MPCNNs have become essential for many contest-winning FNNs(Sec.5.21,Sec.5.22). Multi-Column(MC)GPU-MPCNNs(Ciresan et al.,2011b)are committees(Breiman,1996;Schapire, 1990;Wolpert,1992;Hashem and Schmeiser,1992;Ueda,2000;Dietterich,2000a)of GPU-MPCNNs with simple democratic output averaging.Several MPCNNs see the same input;their output vectors are used to assign probabilities to the various possible classes.The class with the on average highest probability is chosen as the system’s classi?cation of the present https://www.360docs.net/doc/4210009725.html,pare earlier,more sophisticated ensemble methods(Schapire,1990),and the contest-winning ensemble Bayes-NN(Neal,2006)of Sec.5.14. An MC-GPU-MPCNN was the?rst system to achieve superhuman visual pattern recognition(Ciresan et al.,2012b,2011b)in a controlled competition,namely,the IJCNN2011traf?c sign recognition contest in San Jose(CA)(Stallkamp et al.,2011).This is of interest for fully autonomous,self-driving cars in traf?c(e.g.,Dickmanns et al.,1994).The MC-GPU-MPCNN obtained0.56%error rate and was twice better than human test subjects,three times better than the closest arti?cial NN competitor(Sermanet and LeCun,2011),and six times better than the best non-neural method. A few months earlier,the qualifying round was won in a1st stage online competition,albeit by a much smaller margin:1.02%(Ciresan et al.,2011b)vs1.03%for second place(Sermanet and LeCun, 2011).After the deadline,the organisers revealed that human performance on the test set was1.19%.That is,the best methods already seemed human-competitive.However,during the qualifying it was possible to incrementally gain information about the test set by probing it through repeated submissions.This is illustrated by better and better results obtained by various teams over time(Stallkamp et al.,2011)(the organisers eventually imposed a limit of10resubmissions).In the?nal competition this was not possible. This illustrates a general problem with benchmarks whose test sets are public,or at least can be probed to some extent:competing teams tend to over?t on the test set even when it cannot be directly used for training,only for evaluation. In1997many thought it a big deal that human chess world champion Kasparov was beaten by an IBM computer.But back then computers could not at all compete with little kids in visual pattern recognition, which seems much harder than chess from a computational perspective.Of course,the traf?c sign domain is highly restricted,and kids are still much better general pattern recognisers.Nevertheless,by2011,deep NNs could already learn to rival them in important limited visual domains. An MC-GPU-MPCNN was also the?rst method to achieve human-competitive performance(around 0.2%)on MNIST(Ciresan et al.,2012c). Given all the prior work on(MP)CNNs(Sec.5.4,5.8,5.11,5.16)and GPU-CNNs(Sec.5.16),GPU-MPCNNs are not a breakthrough in the scienti?c sense.But they are a commercially relevant breakthrough in ef?cient coding that has made a difference in several contests since2011.Today,most feedforward competition-winning deep NNs are GPU-MPCNNs(Sec.5.21,Sec.5.22). 5.202011:Hessian-Free Optimization for RNNs Also in2011it was shown(Martens and Sutskever,2011)that Hessian-free optimization(e.g.,M?ller, 1993;Pearlmutter,1994;Schraudolph,2002)(Sec.5.6.2),can alleviate the Fundamental Deep Learning Problem(Sec.5.9)in RNNs,outperforming standard gradient-based LSTM RNNs(Sec.5.13)on several https://www.360docs.net/doc/4210009725.html,pare other RNN algorithms(Jaeger,2004;Schmidhuber et al.,2007;Pascanu et al.,2013b; Koutn′?k et al.,2014)that also at least sometimes yield better results than steepest descent for LSTM RNNs. 5.212012:First Contests Won on ImageNet&Object Detection&Segmentation In2012,an ensemble of GPU-MPCNNs(Sec.5.19)achieved best results on the ImageNet classi?cation benchmark(Krizhevsky et al.,2012),which is popular in the computer vision community.Here relatively large image sizes of256x256pixels were necessary,as opposed to only48x48pixels for the2011traf?c sign competition(Sec.5.19).See further improvements in Sec.5.22. Also in2012,the biggest NN so far(109free parameters)was trained in unsupervised mode(Sec.5.7, 5.15)on unlabeled data(Le et al.,2012),then applied to ImageNet.The codes across its top layer were used to train a simple supervised classi?er,which achieved best results so far on20,000classes.Instead of relying on ef?cient GPU programming,this was done by brute force on1,000standard machines with 16,000cores. So by2011/2012,excellent results had been achieved by Deep Learners in image recognition and classi?cation(Sec.5.19,5.21).The computer vision community,however,is especially interested in object detection in large images,for applications such as image-based search engines,or for biomedical diagnosis where the goal may be to automatically detect tumors etc in images of human tissue.Object detection presents additional challenges.One natural approach is to train a deep NN classi?er on patches of big images,then use it as a feature detector to be shifted across unknown visual scenes,using various rotations and zoom factors.Image parts that yield highly active output units are likely to contain objects similar to those the NN was trained on. 2012?nally saw the?rst DL system(an MC-GPU-MPCNN,Sec.5.19)to win a contest on visual object detection in large images of several million pixels(ICPR2012Contest on Mitosis Detection in Breast Cancer Histological Images,2012;Roux et al.,2013;Ciresan et al.,2013).Such biomedical applications may turn out to be among the most important applications of DL.The world spends over10%of GDP on healthcare(>6trillion USD per year),much of it on medical diagnosis through expensive experts.Partial automation of this could not only save lots of money,but also make expert diagnostics accessible to many who currently cannot afford it.It is gratifying to observe that today deep NNs may actually help to improve healthcare and perhaps save human lives. 2012also saw the?rst pure image segmentation contest won by DL,again through an MC-GPU-MPCNN(Segmentation of Neuronal Structures in EM Stacks Challenge,2012;Ciresan et al.,2012a).2 EM stacks are relevant for the recently approved huge brain projects in Europe and the US(e.g.,Markram, 2012).Given electron microscopy images of stacks of thin slices of animal brains,the goal is to build a detailed3D model of the brain’s neurons and dendrites.But human experts need many hours and days and weeks to annotate the images:Which parts depict neuronal membranes?Which parts are irrelevant background?This needs to be automated(e.g.,Turaga et al.,2010).Deep MC-GPU-MPCNNs learned to solve this task through experience with many training images,and won the contest on all three evaluation metrics by a large margin,with superhuman performance in terms of pixel error. Both object detection(Ciresan et al.,2013)and image segmentation(Ciresan et al.,2012a)pro?t from fast MPCNN-based image scans that avoid redundant computations.Recent MPCNN scanners speed up naive implementations by up to three orders of magnitude(Masci et al.,2013;Giusti et al.,2013);compare earlier ef?cient methods for CNNs without MP(Vaillant et al.,1994). Also in2012,a system consisting of growing deep FNNs and2D-BRNNs(Di Lena et al.,2012)won the CASP2012contest on protein contact map prediction.On the IAM-OnDoDB benchmark,LSTM RNNs(Sec.5.13)outperformed all other methods(HMMs,SVMs)on online mode detection(Otte et al., 2012;Indermuhle et al.,2012)and keyword spotting(Indermuhle et al.,2011).On the long time lag problem of language modelling,LSTM RNNs outperformed all statistical approaches on the IAM-DB benchmark(Frinken et al.,2012).Compare other recent RNNs for object recognition(Wyatte et al.,2012; OReilly et al.,2013),extending earlier work on biologically plausible learning rules for RNNs(O’Reilly, 1996). 5.222013-:More Contests and Benchmark Records A stack(Fernandez et al.,2007;Graves and Schmidhuber,2009)(Sec.5.10)of bi-directional LSTM recur-rent NNs(Graves and Schmidhuber,2005)trained by CTC(Sec.5.13,5.17)broke a famous TIMIT speech (phoneme)recognition record,achieving17.7%test set error rate(Graves et al.,2013),despite thousands of man years previously spent on Hidden Markov Model(HMMs)-based speech recognition https://www.360docs.net/doc/4210009725.html,-pare earlier DBN results(Sec.5.15).CTC-LSTM also helped to score?rst at NIST’s OpenHaRT2013 evaluation(Bluche et al.,2014).For Optical Character Recognition(OCR),LSTM RNNs outperformed commercial recognizers of historical data(Breuel et al.,2013). A new record on the ICDAR Chinese handwriting recognition benchmark(over3700classes)was set on a desktop machine by an MC-GPU-MPCNN(Sec.5.19)with almost human performance(Ciresan and Schmidhuber,2013). The MICCAI2013Grand Challenge on Mitosis Detection(Veta et al.,2013)also was won by an object-detecting MC-GPU-MPCNN(Ciresan et al.,2013).Its data set was even larger and more challenging than the one of ICPR2012(Sec.5.21):a real-world dataset including many ambiguous cases and frequently encountered problems such as imperfect slide staining. Deep GPU-MPCNNs(Sec.5.19)also helped to achieve new best results on important benchmarks of the computer vision community:ImageNet classi?cation(Zeiler and Fergus,2013)and—in conjunction with traditional approaches—PASCAL object detection(Girshick et al.,2013).They also learned to predict bounding box coordinates of objects in the Imagenet2013database,and obtained state-of-the-art results on tasks of localization and detection(Sermanet et al.,2013).GPU-MPCNNs also helped to recognise multi-digit numbers in Google Street View images(Goodfellow et al.,2014b),where part of the NN was trained to count visible digits;compare earlier work on detecting“numerosity”through DBNs(Stoianov and Zorzi, 2012).This system also excelled at recognising distorted synthetic text in reCAPTCHA puzzles.Additional 2It should be mentioned,however,that LSTM RNNs already performed simultaneous segmentation and recognition when they became the?rst recurrent Deep Learners to win of?cial international pattern recognition contests—see Sec.5.17. [关闭] 零基础入门深度学习(5) - 循环神经网络 机器学习深度学习入门 无论即将到来的是大数据时代还是人工智能时代,亦或是传统行业使用人工智能在云上处理大数据的时代,作为一个有理想有追求的程序员,不懂深度学习(Deep Learning)这个超热的技术,会不会感觉马上就out了?现在救命稻草来了,《零基础入门深度学习》系列文章旨在讲帮助爱编程的你从零基础达到入门级水平。零基础意味着你不需要太多的数学知识,只要会写程序就行了,没错,这是专门为程序员写的文章。虽然文中会有很多公式你也许看不懂,但同时也会有更多的代码,程序员的你一定能看懂的(我周围是一群狂热的Clean Code程序员,所以我写的代码也不会很差)。 文章列表 零基础入门深度学习(1) - 感知器 零基础入门深度学习(2) - 线性单元和梯度下降 零基础入门深度学习(3) - 神经网络和反向传播算法 零基础入门深度学习(4) - 卷积神经网络 零基础入门深度学习(5) - 循环神经网络 零基础入门深度学习(6) - 长短时记忆网络(LSTM) 零基础入门深度学习(7) - 递归神经网络 往期回顾 在前面的文章系列文章中,我们介绍了全连接神经网络和卷积神经网络,以及它们的训练和使用。他们都只能单独的取处理一个个的输入,前一个输入和后一个输入是完全没有关系的。但是,某些任务需要能够更好的处理序列的信息,即前面的输入和后面的输入是有关系的。比如,当我们在理解一句话意思时,孤立的理解这句话的每个词是不够的,我们需要处理这些词连接起来的整个序列;当我们处理视频的时候,我们也不能只单独的去分析每一帧,而要分析这些帧连接起来的整个序列。这时,就需要用到深度学习领域中另一类非常重要神经网络:循环神经网络(Recurrent Neural Network)。RNN种类很多,也比较绕脑子。不过读者不用担心,本文将一如既往的对复杂的东西剥茧抽丝,帮助您理解RNNs以及它的训练算法,并动手实现一个循环神经网络。 语言模型 RNN是在自然语言处理领域中最先被用起来的,比如,RNN可以为语言模型来建模。那么,什么是语言模型呢? 我们可以和电脑玩一个游戏,我们写出一个句子前面的一些词,然后,让电脑帮我们写下接下来的一个词。比如下面这句:我昨天上学迟到了,老师批评了____。 我们给电脑展示了这句话前面这些词,然后,让电脑写下接下来的一个词。在这个例子中,接下来的这个词最有可能是『我』,而不太可能是『小明』,甚至是『吃饭』。 语言模型就是这样的东西:给定一个一句话前面的部分,预测接下来最有可能的一个词是什么。 语言模型是对一种语言的特征进行建模,它有很多很多用处。比如在语音转文本(STT)的应用中,声学模型输出的结果,往往是若干个可能的候选词,这时候就需要语言模型来从这些候选词中选择一个最可能的。当然,它同样也可以用在图像到文本的识别中(OCR)。 使用RNN之前,语言模型主要是采用N-Gram。N可以是一个自然数,比如2或者3。它的含义是,假设一个词出现的概率只与前面N个词相关。我 深度神经网络及目标检测学习笔记 https://youtu.be/MPU2HistivI 上面是一段实时目标识别的演示,计算机在视频流上标注出物体的类别,包括人、汽车、自行车、狗、背包、领带、椅子等。 今天的计算机视觉技术已经可以在图片、视频中识别出大量类别的物体,甚至可以初步理解图片或者视频中的内容,在这方面,人工智能已经达到了3岁儿童的智力水平。这是一个很了不起的成就,毕竟人工智能用了几十年的时间,就走完了人类几十万年的进化之路,并且还在加速发展。 道路总是曲折的,也是有迹可循的。在尝试了其它方法之后,计算机视觉在仿生学里找到了正确的道路(至少目前看是正确的)。通过研究人类的视觉原理,计算机利用深度神经网络(Deep Neural Network,NN)实现了对图片的识别,包 括文字识别、物体分类、图像理解等。在这个过程中,神经元和神经网络模型、大数据技术的发展,以及处理器(尤其是GPU)强大的算力,给人工智能技术 的发展提供了很大的支持。 本文是一篇学习笔记,以深度优先的思路,记录了对深度学习(Deep Learning)的简单梳理,主要针对计算机视觉应用领域。 一、神经网络 1.1 神经元和神经网络 神经元是生物学概念,用数学描述就是:对多个输入进行加权求和,并经过激活函数进行非线性输出。 由多个神经元作为输入节点,则构成了简单的单层神经网络(感知器),可以进行线性分类。两层神经网络则可以完成复杂一些的工作,比如解决异或问题,而且具有非常好的非线性分类效果。而多层(两层以上)神经网络,就是所谓的深度神经网络。 神经网络的工作原理就是神经元的计算,一层一层的加权求和、激活,最终输出结果。深度神经网络中的参数太多(可达亿级),必须靠大量数据的训练来“这是苹在父母一遍遍的重复中学习训练的过程就好像是刚出生的婴儿,设置。.果”、“那是汽车”。有人说,人工智能很傻嘛,到现在还不如三岁小孩。其实可以换个角度想:刚出生婴儿就好像是一个裸机,这是经过几十万年的进化才形成的,然后经过几年的学习,就会认识图片和文字了;而深度学习这个“裸机”用了几十年就被设计出来,并且经过几个小时的“学习”,就可以达到这个水平了。 1.2 BP算法 神经网络的训练就是它的参数不断变化收敛的过程。像父母教婴儿识图认字一样,给神经网络看一张图并告诉它这是苹果,它就把所有参数做一些调整,使得它的计算结果比之前更接近“苹果”这个结果。经过上百万张图片的训练,它就可以达到和人差不多的识别能力,可以认出一定种类的物体。这个过程是通过反向传播(Back Propagation,BP)算法来实现的。 建议仔细看一下BP算法的计算原理,以及跟踪一个简单的神经网络来体会训练的过程。 神经网络最新发展综述 学校:上海海事大学 专业:物流工程 姓名:周巧珍 学号:201530210155 神经网络最新发展综述 摘要:作为联接主义智能实现的典范,神经网络采用广泛互联的结构与有效的学习机制来模拟人脑信息处理的过程,是人工智能发展中的重要方法,也是当前类脑智能研究中的有效工具。目前,模拟人脑复杂的层次化认知特点的深度学习成为类脑智能中的一个重要研究方向。通过增加网络层数所构造的“深层神经网络”使机器能够获得“抽象概念”能力,在诸多领域都取得了巨大的成功,又掀起了神经网络研究的一个新高潮。本文分8个方面综述了其当前研究进展以及存在的问题,展望了未来神经网络的发展方向。 关键词: 类脑智能;神经网络;深度学习;大数据 Abstract: As a typical realization of connectionism intelligence, neural network, which tries to mimic the information processing patterns in the human brain by adopting broadly interconnected structures and effective learning mechanisms, is an important branch of artificial intelligence and also a useful tool in the research on brain-like intelligence at present. Currently, as a way to imitate the complex hierarchical cognition characteristic of human brain, deep learning brings an important trend for brain-like intelligence. With the increasing number of layers, deep neural network entitles machines the capability to capture “abstract concepts” and it has achieved great success in various fields, leading a new and advanced trend in neural network research. This paper summarizes the latest progress in eight applications and existing problems considering neural network and points out its possible future directions. Key words : artificial intelligence; neural network; deep learning; big data 1 引言 实现人工智能是人类长期以来一直追求的梦想。虽然计算机技术在过去几十年里取得了长足的发展,但是实现真正意义上的机器智能至今仍然困难重重。伴随着神经解剖学的发展,观测大脑微观结构的技术手段日益丰富,人类对大脑组织的形态、结构与活动的认识越来越深入,人脑信息处理的奥秘也正在被逐步揭示。如何借助神经科学、脑科学与认知科学的研究成果,研究大脑信息表征、转换机理和学习规则,建立模拟大脑信息处理过程的智能计算模型,最终使机器掌握人类的认知规律,是“类脑智能”的研究目标。 类脑智能是涉及计算科学、认知科学、神经科学与脑科学的交叉前沿方向。类脑智能的 吴恩达深度学习课程:神经网络和深度学习[中英文字幕+ppt课件] 内容简介 吴恩达(Andrew Ng)相信大家都不陌生了。2017年8 月8 日,吴恩达在他自己创办的在线教育平台Coursera 上线了他的人工智能专项课程(Deep Learning Specialization)。此课程广受好评,通过视频讲解、作业与测验等让更多的人对人工智能有了了解与启蒙,国外媒体报道称:吴恩达这次深度学习课程是迄今为止,最全面、系统和容易获取的深度学习课程,堪称普通人的人工智能第一课。 关注微信公众号datayx 然后回复“深度学习”即可获取。 第一周深度学习概论: 学习驱动神经网络兴起的主要技术趋势,了解现今深度学习在哪里应用、如何应用。 1.1 欢迎来到深度学习工程师微专业 1.2 什么是神经网络? 1.3 用神经网络进行监督学习 1.4 为什么深度学习会兴起? 1.5 关于这门课 1.6 课程资源 第二周神经网络基础: 学习如何用神经网络的思维模式提出机器学习问题、如何使用向量化加速你的模型。 2.1 二分分类 2.2 logistic 回归 2.3 logistic 回归损失函数 2.4 梯度下降法 2.5 导数 2.6 更多导数的例子 2.7 计算图 2.8 计算图的导数计算 2.9 logistic 回归中的梯度下降法 2.10 m 个样本的梯度下降 2.11 向量化 2.12 向量化的更多例子 2.13 向量化logistic 回归 2.14 向量化logistic 回归的梯度输出 2.15 Python 中的广播 2.16 关于python / numpy 向量的说明 2.17 Jupyter / Ipython 笔记本的快速指南 2.18 (选修)logistic 损失函数的解释 第三周浅层神经网络: 深度神经网络全面概述从基本概念到实际模型和硬件基础 深度神经网络(DNN)所代表的人工智能技术被认为是这一次技术变革的基石(之一)。近日,由IEEE Fellow Joel Emer 领导的一个团队发布了一篇题为《深度神经网络的有效处理:教程和调研(Efficient Processing of Deep Neural Networks: A Tutorial and Survey)》的综述论文,从算法、模型、硬件和架构等多个角度对深度神经网络进行了较为全面的梳理和总结。鉴于该论文的篇幅较长,机器之心在此文中提炼了原论文的主干和部分重要内容。 目前,包括计算机视觉、语音识别和机器人在内的诸多人工智能应用已广泛使用了深度神经网络(deep neural networks,DNN)。DNN 在很多人工智能任务之中表现出了当前最佳的准确度,但同时也存在着计算复杂度高的问题。因此,那些能帮助DNN 高效处理并提升效率和吞吐量,同时又无损于表现准确度或不会增加硬件成本的技术是在人工智能系统之中广泛部署DNN 的关键。 论文地址:https://https://www.360docs.net/doc/4210009725.html,/pdf/1703.09039.pdf 本文旨在提供一个关于实现DNN 的有效处理(efficient processing)的目标的最新进展的全面性教程和调查。特别地,本文还给出了一个DNN 综述——讨论了支持DNN 的多种平台和架构,并强调了最新的有效处理的技术的关键趋势,这些技术或者只是通过改善硬件设计或者同时改善硬件设计和网络算法以降低DNN 计算成本。本文也会对帮助研究者和从业者快速上手DNN 设计的开发资源做一个总结,并凸显重要的基准指标和设计考量以评估数量快速增长的DNN 硬件设计,还包括学界和产业界共同推荐的算法联合设计。 读者将从本文中了解到以下概念:理解DNN 的关键设计考量;通过基准和对比指标评估不同的DNN 硬件实现;理解不同架构和平台之间的权衡;评估不同DNN 有效处理技术的设计有效性;理解最新的实现趋势和机遇。 一、导语 深度神经网络(DNN)目前是许多人工智能应用的基础[1]。由于DNN 在语音识别[2] 和图像识别[3] 上的突破性应用,使用DNN 的应用量有了爆炸性的增长。这些DNN 被部署到了从自动驾驶汽车[4]、癌症检测[5] 到复杂游戏[6] 等各种应用中。在这许多领域中,DNN 能够超越人类的准确率。而DNN 的出众表现源于它能使用统计学习方法从原始感官数据中提取高层特征,在大量的数据中获得输入空间的有效表征。这与之前使用手动提取特征或专家设计规则的方法不同。 然而DNN 获得出众准确率的代价是高计算复杂性成本。虽然通用计算引擎(尤其是GPU),已经成为许多DNN 处理的砥柱,但提供对DNN 计算更专门化的加速方法也越来越热门。本文的目标是提供对DNN、理解DNN 行为的各种工具、有效加速计算的各项技术的概述。 该论文的结构如下: 深度神经网络及目标检测学习笔记 https://youtu.be/MPU2HistivI 上面是一段实时目标识别的演示,计算机在视频流上标注出物体的类别,包括人、汽车、自行车、狗、背包、领带、椅子等。 今天的计算机视觉技术已经可以在图片、视频中识别出大量类别的物体,甚至可以初步理解图片或者视频中的内容,在这方面,人工智能已经达到了3岁儿童的智力水平。这是一个很了不起的成就,毕竟人工智能用了几十年的时间,就走完了人类几十万年的进化之路,并且还在加速发展。 道路总是曲折的,也是有迹可循的。在尝试了其它方法之后,计算机视觉在仿生学里找到了正确的道路(至少目前看是正确的)。通过研究人类的视觉原理,计算机利用深度神经网络(DeepNeural Network,NN)实现了对图片的识别,包括文字识别、物体分类、图像理解等。在这个过程中,神经元和神经网络模型、大数据技术的发展,以及处理器(尤其是GPU)强大的算力,给人工智能技术的发展提供了很大的支持。 本文是一篇学习笔记,以深度优先的思路,记录了对深度学习(Deep Learning)的简单梳理,主要针对计算机视觉应用领域。 一、神经网络 1.1 神经元和神经网络 神经元是生物学概念,用数学描述就是:对多个输入进行加权求和,并经过激活函数进行非线性输出。 由多个神经元作为输入节点,则构成了简单的单层神经网络(感知器),可以进行线性分类。两层神经网络则可以完成复杂一些的工作,比如解决异或问题,而且具有非常好的非线性分类效果。而多层(两层以上)神经网络,就是所谓的深度神经网络。 神经网络的工作原理就是神经元的计算,一层一层的加权求和、激活,最终输出结果。深度神经网络中的参数太多(可达亿级),必须靠大量数据的训练来设置。训练的过程就好像是刚出生的婴儿,在父母一遍遍的重复中学习“这是苹 统计建模与R语言 全连接神经网络 学院航空航天学院 专业机械电子工程 年级 2019级 学生学号 19920191151134 学生姓名梅子阳 一、绪论 1、人工智能背景 信息技术是人类历史上的第三次工业革命,计算机、互联网、智能家居等技术的普及极大地方便了人们的日常生活。通过编程的方式,人类可以将提前设计好的交互逻辑交给机器重复且快速地执行,从而将人类从简单枯燥的重复劳动工作中解脱出来。但是对于需要较高智能水平的任务,如人脸识别、聊天机器人、自动驾驶等任务,很难设计明确的逻辑规则,传统的编程方式显得力不从心,而人工智能(Artificial Intelligence,简称 AI)是有望解决此问题的关键技术。 随着深度学习算法的崛起,人工智能在部分任务上取得了类人甚至超人的智力水平,如围棋上 AlphaGo 智能程序已经击败人类最强围棋专家之一柯洁,在 Dota2 游戏上OpenAI Five 智能程序击败冠军队伍 OG,同时人脸识别、智能语音、机器翻译等一项项实用的技术已经进入到人们的日常生活中。现在我们的生活处处被人工智能所环绕,尽管目前能达到的智能水平离通用人工智能(Artificial General Intelligence,简称 AGI)还有一段距离,但是我们仍坚定地相信人工智能的时代已经来临。 怎么实现人工智能是一个非常广袤的问题。人工智能的发展主要经历过三个阶段,每个阶段都代表了人们从不同的角度尝试实现人工智能的探索足迹。早期,人们试图通过总结、归纳出一些逻辑规则,并将逻辑规则以计算机程序的方式实现,来开发出智能系统。但是这种显式的规则往往过于简单,并且很难表达复杂、抽象的概念和规则。这一阶段被称为推理期。 1970 年代,科学家们尝试通过知识库加推理的方式解决人工智能,通过建庞大复杂的专家系统来模拟人类专家的智能水平。这些明确指定规则的方式存在一个最大的难题,就是很多复杂、抽象的概念无法用具体的代码实现。比如人类对图片的识别、对语言的理解过程,根本无法通过既定规则模拟。为了解决这类问题,一门通过让机器自动从数据中学习规则的研究学科诞生了,称为机器学习,并在 1980 年代成为人工智能中的热门学科。在机器学习中,有一门通过神经网络来学习复杂、抽象逻辑的方向,称为神经网络。神经网络方向的研究经历了两起两落。2012 年开始,由于效果极为显著,应用深层神经网络技术在计算机视觉、自然语言处理、机器人等领域取得了重大突破,部分任务上甚至超越了人类智能水平,开启了以深层神经网络为代表的人工智能的第三次复兴。深层神经网络有了一个新名字:深度学习。一般来讲,神经网络和深度学习的本质区别并不大,深度学习特指基于深层神经网络实现的模型或算法。 2、神经网络与深度学习 将神经网络的发展历程大致分为浅层神经网络阶段和深度学习阶段,以2006 年为分割点。2006 年以前,深度学习以神经网络和连接主义名义发展, BP神经网络及深度学习研究 摘要:人工神经网络是一门交叉性学科,已广泛于医学、生物学、生理学、哲学、信息学、计算机科学、认知学等多学科交叉技术领域,并取得了重要成果。BP(Back Propagation)神经网络是一种按误差逆传播算法训练的多层前馈网络,是目前应用最广泛的神经网络模型之一。本文将主要介绍神经网络结构,重点研究BP神经网络原理、BP神经网络算法分析及改进和深度学习的研究。 关键词:BP神经网络、算法分析、应用 1 引言 人工神经网络(Artificial Neural Network,即ANN ),作为对人脑最简单的一种抽象和模拟,是人们模仿人的大脑神经系统信息处理功能的一个智能化系统,是20世纪80 年代以来人工智能领域兴起的研究热点。人工神经网络以数学和物理方法以及信息处理的角度对人脑神经网络进行抽象,并建立某种简化模型,旨在模仿人脑结构及其功能的信息处理系统。 人工神经网络最有吸引力的特点就是它的学习能力。因此从20世纪40年代人工神经网络萌芽开始,历经两个高潮期及一个反思期至1991年后进入再认识与应用研究期,涌现出无数的相关研究理论及成果,包括理论研究及应用研究。最富有成果的研究工作是多层网络BP算法,Hopfield网络模型,自适应共振理论,自组织特征映射理论等。因为其应用价值,该研究呈愈演愈烈的趋势,学者们在多领域中应用[1]人工神经网络模型对问题进行研究优化解决。 人工神经网络是由多个神经元连接构成,因此欲建立人工神经网络模型必先建立人工神经元模型,再根据神经元的连接方式及控制方式不同建立不同类型的人工神经网络模型。现在分别介绍人工神经元模型及人工神经网络模型。 1.1 人工神经元模型 仿生学在科技发展中起着重要作用,人工神经元模型的建立来源于生物神经元结构的仿生模拟,用来模拟人工神经网络[2]。人们提出的神经元模型有很多,其中最早提出并且影响较大的是1943年心理学家McCulloch和数学家W. Pitts 在分析总结神经元基本特性的基础上首先提出的MP模型。该模型经过不断改进后,形成现在广泛应用的BP神经元模型。人工神经元模型是由人量处理单元厂泛互连而成的网络,是人脑的抽象、简化、模拟,反映人脑的基本特性。一般来说,作为人工神经元模型应具备三个要素: (1)具有一组突触或连接,常用表示神经元i和神经元j之间的连接强度。 w ij (2)具有反映生物神经元时空整合功能的输入信号累加器。 可用于自动驾驶的神经网络及深度学习 高级辅助驾驶系统(ADAS)可提供解决方案,用以满足驾乘人员对道路安全及出行体验的更高要求。诸如车道偏离警告、自动刹车及泊车辅助等系统广泛应用于当前的车型,甚至是功能更为强大的车道保持、塞车辅助及自适应巡航控制等系统的配套使用也让未来的全自动驾驶车辆成为现实。 作者:来源:电子产品世界|2017-02-27 13:55 收藏 分享 高级辅助驾驶系统(ADAS)可提供解决方案,用以满足驾乘人员对道路安全及出行体验的更高要求。诸如车道偏离警告、自动刹车及泊车辅助等系统广泛应用于当前的车型,甚至是功能更为强大的车道保持、塞车辅助及自适应巡航控制等系统的配套使用也让未来的全自动驾驶车辆成为现实。 如今,车辆的很多系统使用的都是机器视觉。机器视觉采用传统信号处理技术来检测识别物体。对于正热衷于进一步提高拓展ADAS功能的汽车制造业而言,深度学习神经网络开辟了令人兴奋的研究途径。为了实现从诸如高速公路全程自动驾驶仪的短时辅助模式到专职无人驾驶旅行的自动驾驶,汽车制造业一直在寻求让响应速度更快、识别准确度更高的方法,而深度学习技术无疑为其指明了道路。 以知名品牌为首的汽车制造业正在深度学习神经网络技术上进行投资,并向先进的计算企业、硅谷等技术引擎及学术界看齐。在中国,百度一直在此技术上保持领先。百度计划在2019 年将全自动汽车投入商用,并加大全自动汽车的批量生产力度,使其在2021 年可广泛投入使用。汽车制造业及技术领军者之间的密切合作是嵌入式系统神经网络发展的催化剂。这类神经网络需要满足汽车应用环境对系统大小、成本及功耗的要求。 1轻型嵌入式神经网络 卷积式神经网络(CNN)的应用可分为三个阶段:训练、转化及CNN在生产就绪解决方案中的执行。要想获得一个高性价比、针对大规模车辆应用的高效结果,必须在每阶段使用最为有利的系统。 训练往往在线下通过基于CPU的系统、图形处理器(GPU)或现场可编程门阵列(FPGA)来完成。由于计算功能强大且设计人员对其很熟悉,这些是用于神经网络训练的最为理想的系统。 在训练阶段,开发商利用诸如Caffe(Convolution Architecture For Feature Extraction,卷积神经网络架构)等的框架对CNN 进行训练及优化。参考图像数据库用于确定网络中神经元的最佳权重参数。训练结束即可采用传统方法在CPU、GPU 或FPGA上生成网络及原型,尤其是执行浮点运算以确保最高的精确度。 作为一种车载使用解决方案,这种方法有一些明显的缺点。运算效率低及成本高使其无法在大批量量产系统中使用。 CEVA已经推出了另一种解决方案。这种解决方案可降低浮点运算的工作负荷,并在汽车应用可接受的功耗水平上获得实时的处理性能表现。随着全自动驾驶所需的计算技术的进一步发展,对关键功能进行加速的策略才能保证这些系统得到广泛应用。 利用被称为CDNN的框架对网络生成策略进行改进。经过改进的策略采用在高功耗浮点计算平台上(利用诸如Caffe的传统网络生成器)开发的受训网络结构和权重,并将其转化为基于定点运算,结构紧凑的轻型的定制网络模型。接下来,此模型会在一个基于专门优化的成像和视觉DSP芯片的低功耗嵌入式平台上运行。图1显示了轻型嵌入式神经网络的生成 Computer Science and Application 计算机科学与应用, 2020, 10(9), 1625-1630 Published Online September 2020 in Hans. https://www.360docs.net/doc/4210009725.html,/journal/csa https://https://www.360docs.net/doc/4210009725.html,/10.12677/csa.2020.109171 深度神经网络知识蒸馏综述 韩宇 中国公安部第一研究所,北京 收稿日期:2020年9月3日;录用日期:2020年9月17日;发布日期:2020年9月24日 摘要 深度神经网络在计算机视觉、自然语言处理、语音识别等多个领域取得了巨大成功,但是随着网络结构的复杂化,神经网络模型需要消耗大量的计算资源和存储空间,严重制约了深度神经网络在资源有限的应用环境和实时在线处理的应用上的发展。因此,需要在尽量不损失模型性能的前提下,对深度神经网络进行压缩。本文介绍了基于知识蒸馏的神经网络模型压缩方法,对深度神经网络知识蒸馏领域的相关代表性工作进行了详细的梳理与总结,并对知识蒸馏未来发展趋势进行展望。 关键词 神经网络,深度学习,知识蒸馏 A Review of Knowledge Distillation in Deep Neural Networks Yu Han The First Research Institute, The Ministry of Public Security of PRC, Beijing Received: Sep. 3rd, 2020; accepted: Sep. 17th, 2020; published: Sep. 24th, 2020 Abstract Deep neural networks have achieved great success in computer vision, natural language processing, speech recognition and other fields. However, with the complexity of network structure, the neural network model needs to consume a lot of computing resources and storage space, which seriously restricts the development of deep neural network in the resource limited application environment and real-time online processing application. Therefore, it is necessary to compress the deep neural network without losing the performance of the model as much as possible. This article introduces CDA数据分析研究院出品,转载需授权 深度学习是机器学习的一个子领域,研究的算法灵感来自于大脑的结构和功能,称为人工神经网络。 如果你现在刚刚开始进入深度学习领域,或者你曾经有过一些神经网络的经验,你可能会感到困惑。因为我知道我刚开始的时候有很多的困惑,我的许多同事和朋友也是这样。因为他们在20世纪90年代和21世纪初就已经学习和使用神经网络了。 该领域的领导者和专家对深度学习的观点都有自己的见解,这些具体而细微的观点为深度学习的内容提供了很多依据。 在这篇文章中,您将通过听取该领域的一系列专家和领导者的意见,来了解什么是深度学习以及它的内容。 来让我们一探究竟吧。 深度学习是一种大型的神经网络 Coursera的Andrew Ng和百度研究的首席科学家正式创立了Google Brain,最终导致了大量Google服务中的深度学习技术的产品化。 他已经说了很多关于深度学习的内容并且也写了很多,这是一个很好的开始。 在深度学习的早期讨论中,Andrew描述了传统人工神经网络背景下的深度学习。在2013年的题为“ 深度学习,自学习和无监督特征学习”的演讲中“他将深度学习的理念描述为: 这是我在大脑中模拟的对深度学习的希望: - 使学习算法更好,更容易使用。 - 在机器学习和人工智能方面取得革命性进展。 我相信这是我们迈向真正人工智能的最好机会 后来他的评论变得更加细致入微了。 Andrew认为的深度学习的核心是我们现在拥有足够快的计算机和足够多的数据来实际训练大型神经网络。在2015年ExtractConf大会上,当他的题目“科学家应该了解深度学习的数据”讨论到为什么现在是深度学习起飞的时候,他评论道: 我们现在拥有的非常大的神经网络......以及我们可以访问的大量数据 他还评论了一个重要的观点,那就是一切都与规模有关。当我们构建更大的神经网络并用越来越多的数据训练它们时,它们的性能会不断提高。这通常与其他在性能上达到稳定水平的机器学习技术不同。 对于大多数旧时代的学习算法来说......性能将达到稳定水平。......深度学习......是第一类算法......是可以扩展的。...当你给它们提供更多的数据时,它的性能会不断提高 他在幻灯片中提供了一个漂亮的卡通片: 最后,他清楚地指出,我们在实践中看到的深度学习的好处来自有监督的学习。从2015年的ExtractConf演讲中,他评论道: 如今的深度学习几乎所有价值都是通过有监督的学习或从有标记的数据中学习 在2014年的早些时候,在接受斯坦福大学的题为“深度学习”的演讲时,他也发出了类似的评论。 深度学习疯狂发展的一个原因是它非常擅长监督学习 1. 自联想神经网络与深度网络 自联想神经网络是很古老的神经网络模型,简单的说,它就是三层BP网络,只不过它的输出等于输入。很多时候我们并不要求输出精确的等于输入,而是允许一定的误差存在。所以,我们说,输出是对输入的一种重构。其网络结构可以很简单的表示如下: 如果我们在上述网络中不使用sigmoid函数,而使用线性函数,这就是PCA模型。中间网络节点个数就是PCA模型中的主分量个数。不用担心学习算法会收敛到局部最优,因为线性BP网络有唯一的极小值。 在深度学习的术语中,上述结构被称作自编码神经网络。从历史的角度看,自编码神经网络是几十年前的事情,没有什么新奇的地方。 既然自联想神经网络能够实现对输入数据的重构,如果这个网络结构已经训练好了,那么其中间层,就可以看过是对原始输入数据的某种特征表示。如果我们把它的第三层去掉,这样就是一个两层的网络。如果,我们把这个学习到特征再用同样的方法创建一个自联想的三层BP网络,如上图所示。换言之,第二次创建的三层自联想网络的输入是上一个网络的中间层的输出。用同样的训练算法,对第二个自联想网络进行学习。那么,第二个自联想网络的中间层是对其输入的某种特征表示。如果我们按照这种方法,依次创建很多这样的由自联想网络组成的网络结构,这就是深度神经网络,如下图所示: 注意,上图中组成深度网络的最后一层是级联了一个softmax分类器。 深度神经网络在每一层是对最原始输入数据在不同概念的粒度表示,也就是不同级别的特征描述。 这种层叠多个自联想网络的方法,最早被Hinton想到了。 从上面的描述中,可以看出,深度网络是分层训练的,包括最后一层的分类器也是单独训练的,最后一层分类器可以换成任何一种分类器,例如SVM,HMM等。上面的每一层单独训练使用的都是BP算法。相信这一思路,Hinton早就实验过了。 2. DBN神经网络模型 使用BP算法单独训练每一层的时候,我们发现,必须丢掉网络的第三层,才能级联自联想神经网络。然而,有一种更好的神经网络模型,这就是受限玻尔兹曼机。使用层叠波尔兹曼机组成深度神经网络的方法,在深度学习里被称作深度信念网络DBN,这是目前非 学习方式 根据数据类型的不同,对一个问题的建模有不同的方式。在机器学习或者人工智能领域,人们首先会考虑算法的学习方式。在机器学习领域,有几种主要的学习方式。将算法按照学习方式分类是一个不错的想法,这样可以让人们在建模和算法选择的时候考虑能根据输入数据来选择最合适的算法来获得最好的结果。 监督式学习: 在监督式学习下,输入数据被称为“训练数据”,每组训练数据有一个明确的标识或结果,如对防垃圾邮件系统中“垃圾邮件”“非垃圾邮件”,对手写数字识别中的“1“,”2“,”3“,”4“等。在建立预测模型的时候,监督式学习建立一个学习过程,将预测结果与“训练数据”的实际结果进行比较,不断的调整预测模型,直到模型的预测结果达到一个预期的准确率。监督式学习的常见应用场景如分类问题和回归问题。常见算法有逻辑回归(Logistic Regression)和反向传递神经网络(Back Propagation Neural Network) 非监督式学习: 在非监督式学习中,数据并不被特别标识,学习模型是为了推断出数据的一些内在结构。常见的应用场景包括关联规则的学习以及聚类等。常见算法包括Apriori算法以及k-Means算法。 半监督式学习: 在此学习方式下,输入数据部分被标识,部分没有被标识,这种学习模型可以用来进行预测,但是模型首先需要学习数据的内在结构以便合理的组织数据来进行预测。应用场景包括分类和回归,算法包括一些对常用监督式学习算法的延伸,这些算法首先试图对未标识数据进行建模,在此基础上再对标识的数据进行预测。如图论推理算法(Graph Inference)或者拉普拉斯支持向量机(Laplacian SVM.)等。 强化学习: Draft:Deep Learning in Neural Networks:An Overview Technical Report IDSIA-03-14/arXiv:1404.7828(v1.5)[cs.NE] J¨u rgen Schmidhuber The Swiss AI Lab IDSIA Istituto Dalle Molle di Studi sull’Intelligenza Arti?ciale University of Lugano&SUPSI Galleria2,6928Manno-Lugano Switzerland 15May2014 Abstract In recent years,deep arti?cial neural networks(including recurrent ones)have won numerous con-tests in pattern recognition and machine learning.This historical survey compactly summarises relevant work,much of it from the previous millennium.Shallow and deep learners are distinguished by the depth of their credit assignment paths,which are chains of possibly learnable,causal links between ac- tions and effects.I review deep supervised learning(also recapitulating the history of backpropagation), unsupervised learning,reinforcement learning&evolutionary computation,and indirect search for short programs encoding deep and large networks. PDF of earlier draft(v1):http://www.idsia.ch/~juergen/DeepLearning30April2014.pdf LATEX source:http://www.idsia.ch/~juergen/DeepLearning30April2014.tex Complete BIBTEX?le:http://www.idsia.ch/~juergen/bib.bib Preface This is the draft of an invited Deep Learning(DL)overview.One of its goals is to assign credit to those who contributed to the present state of the art.I acknowledge the limitations of attempting to achieve this goal.The DL research community itself may be viewed as a continually evolving,deep network of scientists who have in?uenced each other in complex ways.Starting from recent DL results,I tried to trace back the origins of relevant ideas through the past half century and beyond,sometimes using“local search”to follow citations of citations backwards in time.Since not all DL publications properly acknowledge earlier relevant work,additional global search strategies were employed,aided by consulting numerous neural network experts.As a result,the present draft mostly consists of references(about800entries so far).Nevertheless,through an expert selection bias I may have missed important work.A related bias was surely introduced by my special familiarity with the work of my own DL research group in the past quarter-century.For these reasons,the present draft should be viewed as merely a snapshot of an ongoing credit assignment process.To help improve it,please do not hesitate to send corrections and suggestions to juergen@idsia.ch. 第10卷一第2期一2019年4月指挥信息系统与技术C o mm a n d I n f o r m a t i o nS y s t e ma n dT e c h n o l o g y V o l .10一N o .2A p r .2019 发展综述 d o i :10.15908/j .c n k i .c i s t .2019.02.002智能决策系统的深度神经网络加速与压缩方法综述? 黄一迪一刘一畅 (中国科学院大学计算机科学与技术学院一北京100049 )摘一要:深度神经网络凭借其出色的特征提取能力和表达能力,在图像分类二语义分割和物体检测 等领域表现出众,对信息决策支持系统的发展产生了重大意义.然而,由于模型存储不易和计算延 迟高等问题,深度神经网络较难在信息决策支持系统中得到应用.综述了深度神经网络中低秩分 解二网络剪枝二量化二知识蒸馏等加速与压缩方法.这些方法能够在保证准确率的情况下减小深度 神经网络模型二加快模型计算,为深度神经网络在信息决策支持系统中的应用提供了思路. 关键词:深度神经网络;低秩分解;网络剪枝;量化;知识蒸馏 中图分类号:T P 301.6一一文献标识码:A一一文章编号:1674G909X (2019)02G0008G06R e v i e wo fA c c e l e r a t i o na n dC o m p r e s s i o n M e t h o d s f o rD e e p N e u r a lN e t w o r k s i n I n t e l l i g e n tD e c i s i o nS y s t e m s HU A N G D i 一L I U C h a n g (S c h o o l o fC o m p u t e r S c i e n c e a n dT e c h n o l o g y ,U n i v e r s i t y o fC h i n e s eA c a d e m y o f S c i e n c e s ,B e i j i n g 100049,C h i n a )A b s t r a c t :F o r t h e e x c e l l e n t f e a t u r e e x t r a c t i o na b i l i t y a n de x p r e s s i o na b i l i t y ,t h ed e e p n e u r a l n e t Gw o r kd o e sw e l l i n t h e f i e l d s o f i m a g e c l a s s i f i c a t i o n ,s e m a n t i c s e g m e n t a t i o na n do b j e c t d e t e c t i o n ,e t c .,a n d i t p l a y s a s i g n i f i c a n t r o l eo nt h ed e v e l o p m e n to f t h e i n f o r m a t i o nd e c i s i o ns u p p o r t s y s Gt e m s .H o w e v e r ,f o r t h e d i f f i c u l t y o fm o d e l s t o r a g e a n dh i g hc o m p u t a t i o nd e l a y ,t h e d e e p n e u r a l n e t w o r k i sd i f f i c u l t t ob ea p p l i e d i nt h e i n f o r m a t i o nd e c i s i o ns u p p o r t s y s t e m s .T h ea c c e l e r a t i o n a n dc o m p r e s s i o n m e t h o d s f o r t h ed e e p n e u r a l n e t w o r k ,i n c l u d i n g l o w Gr a n kd e c o m p o s i t i o n ,n e t Gw o r k p r u n i n g ,q u a n t i z a t i o n a n dk n o w l e d g e d i s t i l l a t i o n a r e r e v i e w e d .T h em e t h o d s c a n r e d u c e t h e s i z e o fm o d e l a n d s p e e du p t h e c a l c u l a t i o nu n d e r t h e c o n d i t i o no f e n s u r i n g t h e a c c u r a c y ,a n dc a n p r o v i d e t h e i d e a o f t h e a p p l i c a t i o n i n t h e i n f o r m a t i o nd e c i s i o ns u p p o r t s y s t e m s .K e y w o r d s :d e e p n e u r a ln e t w o r k ;l o w G r a n k d e c o m p o s i t i o n ;n e t w o r k p r u n i n g ;q u a n t i z a t i o n ;k n o w l e d g e d i s t i l l a t i o n 一?基金项目:装备发展部 十三五 预研课题(31511090402)资助项目.收稿日期:2018G11G26引用格式:黄迪,刘畅.智能决策系统的深度神经网络加速与压缩方法综述[J ].指挥信息系统与技术,2019,10(2):8G13. HU A N GD i ,L I U C h a n g .R e v i e wo f a c c e l e r a t i o na n d c o m p r e s s i o nm e t h o d s f o r d e e p n e u r a l n e t w o r k s i n i n t e l l i Gg e n t d e c i s i o n s y s t e m s [J ].C o mm a n d I n f o r m a t i o nS y s t e ma n dT e c h n o l o g y ,2019,10(2):8G13.0一引一言 近年来,深度神经网络在人工智能领域表现非 凡,受到学界和业界的广泛关注,尤其在图像分类二 语义分割和物体检测等领域中,表现出了出色的特征提取和表达能力,如N e a g o e 等[1]提出过一种机器学习方法,可用于航空影像中军用地面车辆识别,为信息决策支持系统的改进提供了可能[2].然而,由于深度神经网络的模型复杂二计算量大和延时高等问题,将其应用于智能决策系统的技术零基础入门深度学习(5) - 循环神经网络
(完整版)深度神经网络及目标检测学习笔记(2)
最新神经网络最新发展综述汇编
吴恩达深度学习课程:神经网络和深度学习
(完整版)深度神经网络全面概述
深度神经网络及目标检测学习笔记
深度学习与全连接神经网络
BP神经网络及深度学习研究-综述(最新整理)
神经网络及深度学习
深度神经网络知识蒸馏综述
深度学习与神经网络
深度神经网络
机器学习算法汇总:人工神经网络、深度学习及其它
《神经网络与深度学习综述DeepLearning15May2014
智能决策系统的深度神经网络加速与压缩方法综述