Statistical Characterization of a real video on demand service_ user behaviour and streaming-media
地质统计学的反演流程

地质统计学的反演流程Geostatistics is a branch of statistics that deals with the study of spatial or spatiotemporal phenomena. In the field of geology, geostatistics plays a crucial role in the analysis and interpretation of geological data. 地质统计学是处理空间或时空现象研究的统计学分支。
在地质领域,地质统计学在地质数据的分析和解释中起着至关重要的作用。
The process of geostatistical inversion involves the use of statistical and mathematical techniques to infer the spatial distribution of geological properties based on limited observations. 地质统计反演的流程涉及利用统计和数学技术,根据有限观测推断地质特性的空间分布。
One of the key aspects of geostatistical inversion is the characterization of uncertainty in the estimation of geological properties. This involves quantifying the variability and reliability of the inferred spatial distribution, taking into account the limited amount of data available. 地质统计反演的一个关键方面是表征地质特性估计的不确定性。
定性与定量研究
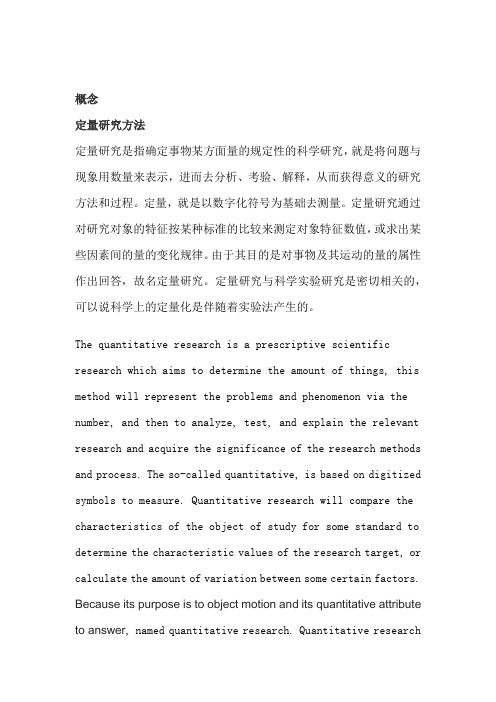
概念定量研究方法定量研究是指确定事物某方面量的规定性的科学研究,就是将问题与现象用数量来表示,进而去分析、考验、解释,从而获得意义的研究方法和过程。
定量,就是以数字化符号为基础去测量。
定量研究通过对研究对象的特征按某种标准的比较来测定对象特征数值,或求出某些因素间的量的变化规律。
由于其目的是对事物及其运动的量的属性作出回答,故名定量研究。
定量研究与科学实验研究是密切相关的,可以说科学上的定量化是伴随着实验法产生的。
The quantitative research is a prescriptive scientific research which aims to determine the amount of things, this method will represent the problems and phenomenon via the number, and then to analyze, test, and explain the relevant research and acquire the significance of the research methods and process. The so-called quantitative, is based on digitized symbols to measure. Quantitative research will compare the characteristics of the object of study for some standard to determine the characteristic values of the research target, or calculate the amount of variation between some certain factors. Because its purpose is to object motion and its quantitative attribute to answer, named quantitative research. Quantitative researchand scientific experimental research are closely related, it can be said that scientific quantitative along with the experimental method。
写长法对高中英语写作影响的实证研究
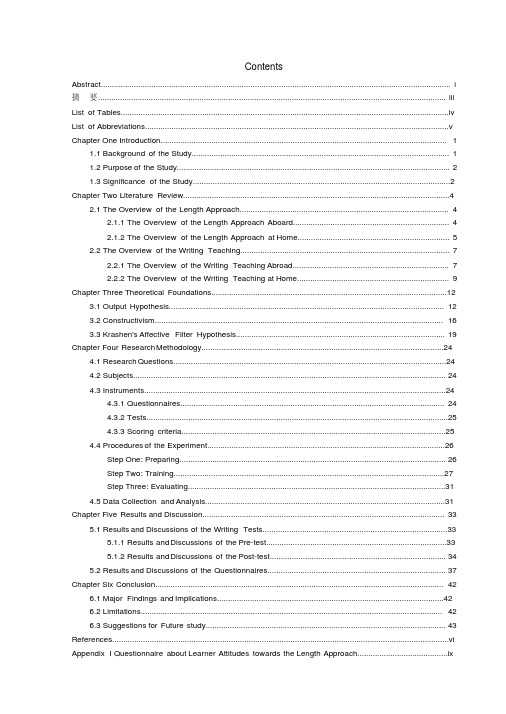
ContentsAbstract (i)摘要 (iii)List of Tables (iv)List of Abbreviations (v)Chapter One Introduction (1)1.1Background of the Study (1)1.2Purpose of the Study (2)1.3Significance of the Study (2)Chapter Two Literature Review (4)2.1The Overview of the Length Approach (4)2.1.1The Overview of the Length Approach Aboard (4)2.1.2The Overview of the Length Approach at Home (5)2.2The Overview of the Writing Teaching (7)2.2.1The Overview of the Writing Teaching Abroad (7)2.2.2The Overview of the Writing Teaching at Home (9)Chapter Three Theoretical Foundations (12)3.1Output Hypothesis (12)3.2Constructivism (16)3.3Krashen's Affective Filter Hypothesis (19)Chapter Four Research Methodology (24)4.1Research Questions (24)4.2Subjects (24)4.3Instruments (24)4.3.1Questionnaires (24)4.3.2Tests (25)4.3.3Scoring criteria (25)4.4Procedures of the Experiment (26)Step One:Preparing (26)Step Two:Training (27)Step Three:Evaluating (31)4.5Data Collection and Analysis (31)Chapter Five Results and Discussion (33)5.1Results and Discussions of the Writing Tests (33)5.1.1Results and Discussions of the Pre-test (33)5.1.2Results and Discussions of the Post-test (34)5.2Results and Discussions of the Questionnaires (37)Chapter Six Conclusion (42)6.1Major Findings and Implications (42)6.2Limitations (42)6.3Suggestions for Future study (43)References (vi)Appendix I Questionnaire about Learner Attitudes towards the Length Approach (ix)Appendix II Pre-test (xi)Appendix III Scoring criteria (xii)Appendix IV Post-test (xiii)Appendix V A student's writing compositions (xiv) (xv) (xxii)Acknowledgements (xxiii)AbstractEnglish writing,as one of productive skills and communication ways of students'language,is anessential aspect in English learning and teaching.However,writing teaching has always been considered asin foreign language teaching.For many years,researchers have devoted themselves to find out “bottleneck”effective ways to solve this problem and many approaches have been put forward,such as productapproach,process approach and genre approach etc.To some extent,these approaches have improvedE nglish writing students’w riting proficiency.However,the current situation of college students’performance in China is still far from satisfactory.A majority of students lack enthusiasm and are not interested in writing.Given problems existing in English writing,it is urgent to find out new effective ways so as to comprehensively improve students'writing ability.Recently,a new approach-LA,based on some important L2theories as well as the insightful analyses of the situation of writing teaching in China,was initiated by Professor Wang Chuming.The basic assumption of this new approach is that the teachercurrent level of foreign language. constantly adjusts the writing requirements on the basis of students’Through gradually increasing the number of words in composition,students are encouraged to writeincreasingly long compositions so as to strengthen their sense of achievement,enhance their confidence intheir ability to learn foreign language,stimulate further learning and eventually ensure progress in foreignlanguage learning.This new approach has attracted great attention both at home and abroad.The currentstudy,on the basis of the previous researches,aims to investigate the feasibility and validity of applying LAto high school English writing teaching,hoping to change the current situation in writing field and improvestudents'writing confidence and proficiency of their English.80students coming from two parallel classesof high school in Minghu school in Taian participated in this research.By employing instrumentscontaining two English writing tests,two questionnaires and SPSS(Statistical Program for Social Science17.0),this thesis adopted quantitative and qualitative analysis methods in order to answer the followingresearchquestions:1.Can the application of the length approach help senior high school students develop theirself-confidence in their English writing?2.Can the application the length approach in English writing help senior high school students improvetheir writing proficiency in their English writing?After fourteen weeks'experiment,the author comes to the conclusion as follows:i。
高精度室内定位算法与技术综述
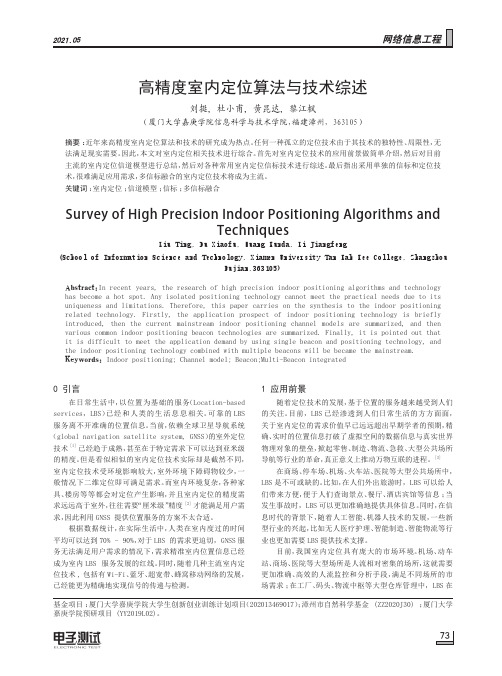
0 引言在日常生活中,以位置为基础的服务(Location-based services,LBS)已经和人类的生活息息相关。
可靠的LBS 服务离不开准确的位置信息。
当前,依赖全球卫星导航系统(global navigation satellite system, GNSS)的室外定位技术[1]已经趋于成熟,甚至在于特定需求下可以达到亚米级的精度。
但是看似相似的室内定位技术实际却是截然不同,室内定位技术受环境影响较大,室外环境下障碍物较少,一般情况下二维定位即可满足需求。
而室内环境复杂,各种家具、楼房等等都会对定位产生影响,并且室内定位的精度需求远远高于室外,往往需要“厘米级”精度[2]才能满足用户需求,因此利用GNSS 提供位置服务的方案不太合适。
根据数据统计,在实际生活中,人类在室内度过的时间平均可以达到70% - 90%,对于LBS 的需求更迫切,GNSS 服务无法满足用户需求的情况下,需求精准室内位置信息已经成为室内LBS 服务发展的红线。
同时,随着几种主流室内定位技术,包括有Wi-Fi、蓝牙、超宽带、蜂窝移动网络的发展,已经能更为精确地实现信号的传递与检测。
1 应用前景随着定位技术的发展,基于位置的服务越来越受到人们的关注。
目前,LBS 已经渗透到人们日常生活的方方面面,关于室内定位的需求价值早已远远超出早期学者的预期,精确、实时的位置信息打破了虚拟空间的数据信息与真实世界物理对象的壁垒,掀起零售、制造、物流、急救、大型公共场所导航等行业的革命,真正意义上推动万物互联的进程。
[3]在商场、停车场、机场、火车站、医院等大型公共场所中,LBS 是不可或缺的。
比如,在人们外出旅游时,LBS 可以给人们带来方便,便于人们查询景点、餐厅、酒店宾馆等信息;当发生事故时,LBS 可以更加准确地提供具体信息。
同时,在信息时代的背景下,随着人工智能、机器人技术的发展,一些新型行业的兴起,比如无人医疗护理、智能制造、智能物流等行业也更加需要LBS 提供技术支撑。
无线电通信原理 第四章(英文)
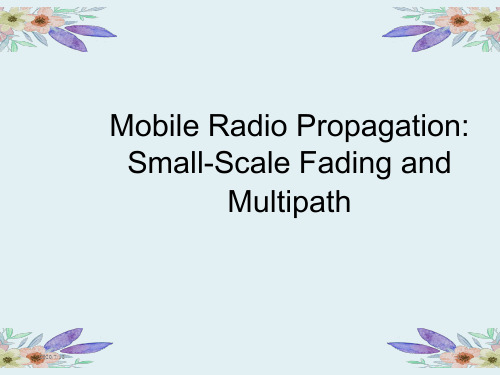
2020/7/18
10
the time varying discrete-time impulse response model for a multipath radio channel
2020/7/18
11
N 1
hb (t,t ) ai (t,t ) exp[ ji (t,t )] (t ti (t)) i0
Local area: no greater than 6m outdoor Local area: no greater than 2m indoor
2020/7/18
14
Parameters of Mobile Multipath Channels
• Time Dispersion Parameters • Coherent bandwidth • Doppler Spread • Coherence Time
•We need a few major parameters for easy –Compare different channels (delay, bandwidth, spectrum, etc) –Develop design guide lines for wireless signals
-If the vehicle is moving directly towards the transmitter
f 26.82 165Hz 0.162
-If the vehicle is moving perpendicular to the angle of arrival of
the transmitted signal
t : time variation due to motion/Doppler shift
A Formal Characterisation of

1 Introduction
It is a commonplace feature of legal systems, and other norm-governed organisations, that particular agents are empowered to create certain types of states, by means of the performance of speci ed types of acts. Typically, the states created will have a normative character according to which obligations and rights are established for some agents vis-a-vis others, as for instance when a contract is made, or a marriage is e ected, or ownership of an item is transferred. The performances by means of which these states are established will often be of a clearly prescribed, perhaps ritualised nature, involving the utterance of a particular form of words (e.g., the utterance of a speci c type of performative
A Formtionalised Power
Andrew J.I. Jones Marek Sergot y June 1994; June 1995
6603_Lecture2(3)
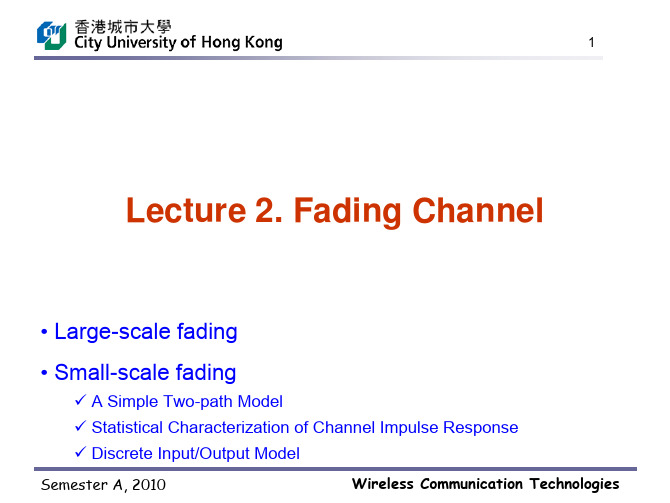
• •
The difference between the Dopper shifts of the two paths is:
Ds D2 D1 2 fv / c
Tc
~
1 Ds
Doppler Spread Ds
Semester A, 2010
Wireless Communication Technologies
The statistics do not change with time (frequency).
H ( f , t ) H ( f f , t t ) R ( f , t ) E
Semester A, 2010 Wireless Communication Technologies
1
Lecture 2. Fading Channel
• Large-scale fading • Small-scale fading
A Simple Two-path Model Statistical Characterization of Channel Impulse Response Discrete Input/Output Model
3
Large-scale Fading
• Large-scale fading -- Log-normal shadowing
– Attributed to the random variation of propagation environment. – Empirically modeled as a log-normal random variable with mean and variance 2. – Mean is determined by the path loss: ~ d-.
MULTIPLE CHOICE QUESTIONS英文考试题含答案
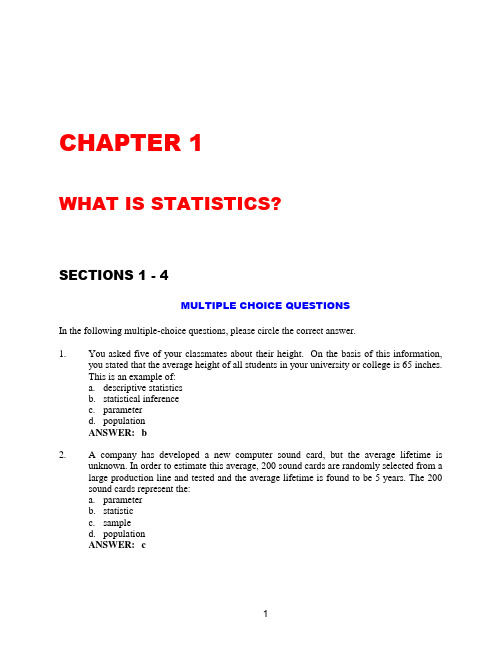
CHAPTER 1WHAT IS STATISTICS?SECTIONS 1 - 4MULTIPLE CHOICE QUESTIONSIn the following multiple-choice questions, please circle the correct answer.1. You asked five of your classmates about their height. On the basis of this information,you stated that the average height of all students in your university or college is 65 inches.This is an example of:a.descriptive statisticsb.statistical inferencec.parameterd.populationANSWER: b2. A company has developed a new computer sound card, but the average lifetime isunknown. In order to estimate this average, 200 sound cards are randomly selected from a large production line and tested and the average lifetime is found to be 5 years. The 200 sound cards represent the:a.parameterb.statisticc.sampled.populationANSWER: c2 Chapter One3. A summary measure that is computed from a sample to describe a characteristic of thepopulation is called aa.parameterb.statisticc.populationd.sampleANSWER: b4. A summary measure that is computed from a population is called aa.parameterb.statisticc.populationd.sampleANSWER: a5. When data are collected in a statistical study for only a portion or subset of all elementsof interest, we are using a:a.sampleb.parameterc.populationd.statisticANSWER: a6. Which of the following is not the goal of descriptive statistics?a.Summarizing datab.Displaying aspects of the collected datac.Reporting numerical findingsd.Estimating characteristics of the populationANSWER: d7. Which of the following statements is not true?a.One form of descriptive statistics uses graphical techniquesb.One form of descriptive statistics uses numerical techniquesc.In the language of statistics, population refers to a group of peopled.Statistical inference is used to draw conclusions or inferences about characteristics ofpopulations based on sample dataANSWER: c8. Descriptive statistics deals with methods of:anizing datab.summarizing datac.presenting data in a convenient and informative wayd.All of the aboveANSWER: dWhat Is Statistics? 3 9. A politician who is running for the office of governor of a state with 4 million registeredvoters commissions a survey. In the survey, 54% of the 5,000 registered voters interviewed say they plan to vote for her. The population of interest is the:a. 4 million registered voters in the stateb.5,000 registered voters interviewedc.2,700 voters interviewed who plan to vote for her.d.2,300 voters interviewed who plan not to vote for herANSWER: a10. A company has developed a new battery, but the average lifetime is unknown. In order toestimate this average, a sample of 500 batteries is tested and the average lifetime of this sample is found to be 225 hours. The 225 hours is the value of a:a.parameterb.statisticc.sampled.populationANSWER: b11. The process of using sample statistics to draw conclusions about true populationparameters is calleda.inferential statisticsb.the scientific methodc.sampling methodd.descriptive statisticsANSWER: a12. Which of the following is most likely a population as opposed to a sample?a.Respondents to a magazine surveyb.The first 10 students completing a final examc.Every fifth student to arrive at the book store on your campusd.Registered voters in the State of MichiganANSWER: d13. Researchers suspect that the average number of credits earned per semester by collegestudents is rising. A researcher at Michigan State University (MSU) wished to estimate the number of credits earned by students during the fall semester of 2003 at MSU. To do so, he randomly selects 500 student transcripts and records the number of credits each student earned in the fall term 2003. He found that the average number of semester credits completed was 14.85 credits per student. The population of interest to the researcher isa.all MSU studentsb.all college students in Michiganc.all MSU students enrolled in the fall semester of 2003d.all college students in Michigan enrolled in the fall semester of 2003ANSWER: c4 Chapter One14. The collection and summarization of the graduate degrees and research areas of interestof the faculty in the University of Michigan of a particular academic institution is an example ofa.inferential statisticsb.descriptive statisticsc. a parameterd. a statisticANSWER: b15. Those methods involving the collection, presentation, and characterization of a set of datain order to properly describe the various features of that set of data are calleda.inferential statisticsb.the scientific methodc.sampling methodd.descriptive statisticsANSWER: d16. Which of the following is most likely a parameter as opposed to a statistic?a.The average score of the first ten students completing an assignmentb.The proportion of females registered to vote in Kent County, Michiganc.The average height of 100 students randomly selected from a community colleged.The proportion of cars stopped yesterday on US 131 that were cited for speedingANSWER: b17. A study is under way in a national forest to determine the adult height of pine trees.Specifically, the study is attempting to determine what factors aid a tree in reaching heights greater than 50 feet tall. It is estimated that the forest contains 32,000 pine trees.The study involves collecting heights from 500 randomly selected adult pine trees and analyzing the results. The sample in the study isa.the 500 randomly selected adult pine treesb.the 32,000 adult pine trees in the forestc.all the adult pine trees taller than 50 feetd.all pine trees, of any age in the forestANSWER: a18. The estimation of the population average student expenditure on education based on thesample average expenditure of 1,000 students is an example ofa.inferential statisticsb.descriptive statisticsc. a parameterd. a statisticANSWER: aWhat Is Statistics? 5TRUE / FALSE QUESTIONS19. When the purpose of the statistical inference is to draw a conclusion about a population,the significance level, as a measure of reliability, measures how frequently the conclusion will be correct in the long run.ANSWER: F20. A summary measure that is computed from a sample to describe a characteristic of thepopulation is called a statistic.ANSWER: T21. The confidence level is the proportion of times that an estimating procedure will be wrong.ANSWER: F22. Conclusions and estimates about a population based on sample data are not always goingto be correct. For this reason measures of reliability, such as the significance level and the confidence level, should be built into the statistical inference.ANSWER: T23. A university employs 2500 faculty and staff. To ascertain how the employees feelregarding a health insurance plan, 250 of the employees are surveyed. The proportion of the 250 employees who favor the health insurance plan is a parameter.ANSWER: F24. In a sample of 400 students in a college of business, 120 of them or 30% are marketingmajors. The 30% is an example of statistical inference as opposed to descriptive statistics.ANSWER: F25. Twenty-five percent of a sample of 200 tennis players indicated that their parents did notplay tennis. Based on this sample, we estimate that the 25% figure holds for the parents of all professional tennis players. This is an example of descriptive statistics as opposed to statistical inference.ANSWER: F26. A population is the totality of items or things of interest under consideration.ANSWER: T27. A local cable system using a sample of 800 subscribers estimates that forty percent of itssubscribers watch premium channel at most four times a week. This is an example of statistical inference as opposed to descriptive statisticsANSWER: T28. A statistic is usually unobservable while a parameter is usually observable.ANSWER: F29. Statistical inference is the process of making an estimate, prediction, or decision about apopulation based on sample data.6 Chapter OneANSWER: T30. A summary measure that is computed from a population is called a parameter.ANSWER: T31. A sample is the portion of the universe that is selected for analysis and making inferencesabout the population from which the sample is selected.ANSWER: T32. As a population becomes large, it is usually better to obtain statistical information fromthe entire population.ANSWER: F33. A statistic is usually used to provide an estimate for a usually unobserved parameter.ANSWER: T34. A sample is always a good representation of the target population.ANSWER: F35. Estimating characteristics of the population is the main goal of descriptive statistics.ANSWER: FWhat Is Statistics? 7 STATISTICAL CONCEPTS & APPLIED QUESTIONS36. In statistics, what name do we give to a numerical characteristic of a sample?ANSWER:Statistic37. In statistics, what name do we give to a numerical characteristic of a population?ANSWER:Parameter38. A manufacturer of children toys claims that less than 3% of his products are defective.When 500 toys were drawn from a large production run, 5% were found to be defective.a.What is the population of interest?b.What is the sample?c.What is the parameter?d.What is the statistic?e.Does the value 3% refer to the parameter or the statistic? Why?f.Does the value 5% refer to the parameter or the statistic? Why?g.Explain briefly how the statistic can be used to make inferences about the parameterto test the claim.ANSWER:a.The complete production run of toysb.The 500 toys drawn from the production runc.Proportion of the production run toys that are defectived.Proportion of sample toys that are defectivee.Parameter; since it is a summary measure that is computed from the populationf.Statistic; since it is a summary measure that is computed from the sampleg.Since the sample proportion is more than 3%, we conclude that the claim is false.39. The Human Resources Director of a large insurance company wishes to develop anemployee health benefits package and decides to select 400 employees from a list of employees in order to study their preferences for the various components of a potential package. The 400 employees who will participate in this study constitute the __________.ANSWER:sample8 Chapter One40. The Human Resources Director of a large hospital in California wishes to develop anemployee health benefits package and decides to select 300 employees from a list of all employees in order to study their preferences for the various components of a potential package. Information obtained from the sample will be used to draw conclusions about the true population __________.ANSWER:parameters41. The Commissioner of Health in California State wanted to study malpractice litigation inLos Angeles. A sample of 32,000 medical records was drawn from a population of 3.5 million patients who were discharged during the year 2003. Using the information obtained from the sample to predict population characteristics with respect to malpractice litigation is an example of __________.ANSWER:inferential statistics42. The Human Resources Director of a large automobile corporation in Michigan wishes todevelop an employee pension package and decides to select 500 employees from a list of all auto workers in order to study their preference for the various components of a potential package. In this study, methods that result in decisions concerning population characteristics based only on the sample results are called __________.ANSWER:inferential statistics43. The Commissioner of Health in California State wanted to study malpractice litigation inLos Angeles. A sample of 32,000 medical records was drawn from a population of 3.5 million patients who were discharged during the year 2003. The true proportion of malpractice claims filed from the population of 3.5 million patients is a __________.ANSWER:parameter44. A businessman who is running for the vacant City Mayor seat with 25,000 registeredvoters conducts a survey. In the survey, 55% of the 500 registered voters interviewed say they planned to vote for him.a.What is the population of interest?b.What is the sample?c.Is the 55% a parameter or a statistic? Why?ANSWER:a.The political choices of the 25,000 registered voters.b.The political choices of the 500 registered voters interviewed.c.Statistic; since it is a summary measure that is computed from the sampleWhat Is Statistics? 945. Define each of the following statistical terms:a.Descriptive statisticsb.Statistical inferencec.Confidence leveld.Significance levele.Populationf.SampleANSWER:a.Descriptive statistics deals with methods of organizing, summarizing, and presentingdata in a convenient and informative way.b. Statistical inference is the process of making estimate, prediction, or decision about apopulation based on sample data.c.The confidence level is a measure of reliability that measures the proportion of timesthat an estimating procedure will be correct.d.The significance level is a measure of reliability that measures how frequently theconclusion about a population will be wrong in the long run.e. A population is the group of all items of interest to a statistics practitioner. It isfrequently very large and may, in fact, be infinitely large.f. A sample is a set of data drawn from the population.46. Identify each of the following studies as either descriptive statistics or statistical inference.a.Examine the weights of a sample of 75 manufacturer parts to see if the average weightof all the parts produced by the process is 2 pounds.b.Post the average final score for your statistics class.c.Estimate the percentage of the US population that will vote for John Kerry in the2004 presidential election.d.Select a random sample of 100 babies born in 2003 and estimate the birth weight ofall babies born during the same year.e.Examine the weights of a sample of 10 cans of corn to see if their average weight is16 ounces.ANSWER:a.Statistical inferenceb.Descriptive statisticsc.Statistical inferenced.Statistical inferencee.Descriptive statistics47. The Commissioner of Health in California State wanted to study malpractice litigation inLos Angeles. A sample of 32,000 medical records was drawn from a population of 3.510 Chapter Onemillion patients who were discharged during the year 2003. The collection, presentation, and characterization of the data from patient medical records are examples of __________.ANSWER:descriptive statistics48. The Human Resources Director of a large automobile corporation in Michigan wishes todevelop an employee pension package and decides to select 500 employees from a list of all auto workers in order to study their preferences for the various components of a potential package. In this study, methods involving the collection, presentation, and characterization of the data are called __________.ANSWER:descriptive statistics49. The Commissioner of Health in California State wanted to study malpractice litigation inLos Angeles. A sample of 30,000 medical records was drawn from a population of 3.5 million patients who were discharged during the year 2003. The proportion of malpractice claims filed from the 32,000 patients is a __________.ANSWER:statistic50. The Human Resources Director at Michigan State University wishes to develop anemployee benefits package and decides to select 500 employees from a list of all employees in order to study their preferences for the various components of a potential package. The Director will use the data from the sample to compute __________.ANSWER:statistics51. The Human Resources Director at Florida State University wishes to develop anemployee benefits package and decides to select 200 employees from a list of employees in order to study their preferences for the various components of a potential package. All the employees in the university constitute the __________.ANSWER:population52. At Grand Rapids Community College 150 students are randomly selected and asked thedistance of their commute to campus. From this group a mean of 18.2 miles is computed.a.What is the parameter?What Is Statistics? 11b.What is the statistics?c.What is the population?d.What is the sample?ANSWER:a.The mean commute distance for all students at the collegeb.The computed 18.2 milesc.All students enrolled at the colleged.The 150 randomly selected students53. Consider the population of all tennis players in the world. Define five samples that can betaken from this population.ANSWER:US tennis players, Female tennis players, Left-handed tennis players, Pro tennis players, and European tennis players who won US open54. An office supply warehouse has boxes of pencils, 100 pencils to the box. Informationabout the entire warehouse as well as a sample of the boxes is shown below:A Quality Control inspector is interested in the number of boxes with three or moredefectives.a.What is the value of the statistic?b.What is the value of the parameter?ANSWER:a. 6b.7055. Briefly describe the difference between parameter and statistic, and give an example ofeach.12 Chapter OneANSWER:A parameter is a descriptive measure of a population.Example: The mean number of soft drinks consumed by all students at the University of Michigan.A statistics is a descriptive measure of a sampleExample: The mean number of soft drinks consumed last week by a sample of 250 students from the University of Michigan.。
- 1、下载文档前请自行甄别文档内容的完整性,平台不提供额外的编辑、内容补充、找答案等附加服务。
- 2、"仅部分预览"的文档,不可在线预览部分如存在完整性等问题,可反馈申请退款(可完整预览的文档不适用该条件!)。
- 3、如文档侵犯您的权益,请联系客服反馈,我们会尽快为您处理(人工客服工作时间:9:00-18:30)。
Statistical characterization of a real video on demandservice:User behaviour and streaming-media workload analysisRoberto Garcı´a *,Xabiel G.Pan ˜eda,Victor Garcı´a,David Melendi,Manuel Vilas Department of Computer Science,University of Oviedo,Campus de Viesques,sn,33204Xixo´n-Asturies,Spain Received 14September 2006;received in revised form 20December 2006;accepted 10February 2007Available online 25February 2007AbstractThe video on demand service characterized in this article has surpassed 4years of service,and the access log database stores information of more than 160,000reproductions and 900videos.The wide variety of subjects,the range of content lengths and a frequent update of contents (several new videos every day),clearly differentiate this study from other pre-vious research limited to specific users,subjects or environments.We present a statistical study of user behaviour and streaming traffic,analyzing session characteristics,mistaken reproductions,amount of media delivered,number and length of pauses and jumps in the reproduction,popularity and daily access profile.The results of the analysis will allow us to develop simulation models and workload generators to evaluate different scenarios and situations of the service.Ó2007Elsevier B.V.All rights reserved.Keywords:Video on demand;Multimedia streaming;Network traffic measurement;Workload characterization1.IntroductionThe speeds offered by the Internet access providers have been increasing continuously in recent years.As penetration grows,broadband providers in the OECD are increasingly offering voice and video services over their platforms [19].The bandwidth enhancement in subscribers’access capabilities has given rise to the appearance of Internet audio/video services,based mainly on streaming technology.Streaming media has been widely used over the Internet for an increasingly demanding and growing consumer population [35].Thus,the characterization of streaming access workloads has become an essential factor to evaluate the per-formance of these services and their implications on the rest of the services in the network.In this paper,a user behaviour analysis and workload characterization performed on the video on demand service of www.lne.es (La Nueva Espan ˜a Digital )is presented.This digital news service is one of the most suc-cessful in Spain and its video on demand service,called LNE TV ,has interesting characteristics,such as a wide1569-190X/$-see front matter Ó2007Elsevier B.V.All rights reserved.doi:10.1016/j.simpat.2007.02.004*Corresponding author.Tel.:+34985182488;fax:+34985181986.E-mail addresses:garciaroberto@uniovi.es (R.Garcı´a),xabiel@uniovi.es (X.G.Pan ˜eda),victor@uniovi.es (V.Garcı´a),melendi@uniovi.es (D.Melendi),vilasmanuel@uniovi.es (M.Vilas).URL:http://www.it.uniovi.es (R.Garcı´a).Simulation Modelling Practice and Theory 15(2007)672–689R.Garcı´a et al./Simulation Modelling Practice and Theory15(2007)672–689673 variety of subjects,a wide range of content lengths,a continuous content update(several new videos every day),etc.Over a period of4years the access logfiles have been captured and stored in a database to be ana-lyzed.The obtained results have been compared with some previous classical studies,such as[2,6,12,16,33]. The results of the analysis have been used to develop user behaviour and workload traffic models which can be integrated in video on demand service models and load generators with the aim of evaluating future situations in the service.This paper makes the following contributions:A primary contribution of our work is its statistical analysis of user behaviour in a commercial video on demand service over a4-year period.The mistaken reproductions,amount of media delivered,number and length of pauses and jumps in the reproduction,session characteristics,popularity and daily access profile have been characterized.Unlike most previous media workload characterizations,which are focused on an educational content,the workload analyzed consists of accesses to a news site in Spain,containing vari-able-length and variable-subject entertainment content.Thus,the results improve and enrich current knowl-edge of typical media workload patterns.Secondly,a streaming-media workload characterization has been performed,differentiating traffic per pro-tocol,source device,audio/videoflow and workload,during both regular load and buffering time intervals. The service uses RealNetworks technology.Finally,we propose a simulation model of the audio/video on demand service.This model is oriented to services with different types of information and lengths.The rest of the paper is organized as follows:in Section2other related works are analyzed.A general description of the case study is carried out in Section3.The user behaviour analysis and characterization are presented in Section4.A measurement study and characterization of RealMedia streaming traffic is carried out in Section5.In Section6we propose a simulation model of the video on demand service.Finally,con-clusions are presented in Section7.2.Related workVideo on demand analysis is a relatively recentfield in the research world.Video on demand services are not widely deployed on the Internet,and,therefore,analysis studies are not abundant.In spite of that,some interesting papers on streaming service analysis have appeared during the last few years.These papers study different elements of user behaviour,quality of service and content popularity.Elements such as session length,delivered time,user’s interactions,etc.have been studied in[2,6].In[33], the study is centred on the user’s connection,user’s origin,and quality requested.The quality of service has been evaluated in[16,33].Different parameters,such as packet loss,jitter,and user perceived quality have been the main study points of these papers.Another element widely analyzed is document popularity[2,5,6,9,12,30].The distribution of users’repro-ductions among the videos is compared with the Zipf-like distribution and theÉparameter is calculated.Ach-arya et al.[1]characterized user accesses to video objects on the web(VOW),showing that,contrary to previous studies,popularity did not follow a Zipf distribution.Using some of the results obtained in these analyses,other studies and tools have appeared.These were able to simulate real service behaviour and carry out performance evaluations.That is the case of[3]where the server capacity is evaluated using a simulated workload.The work of[13]follows the same line.In their paper a tool for video on demand service analysis using simulated workload is presented[31]presents a character-ization of live streaming workloads from a server used by one of the top ten content providers in Brazil[27] analyzes the live streaming workload from a large content delivery network.They study the workload char-acterizing popularity,arrival process,session duration and transport protocol used.Although the analysis of Internet traffic usually includes streaming multimedia traffic,there are few specific works about RealMedia streaming traffic[17]presents an exhaustive study of RealAudio traffic from a popular Internet audio service.They found that RealAudio traffic is dominated by specific packet sizes[6]analyzes a client-based streaming-media workload generated by clients from the University of Washington connecting to servers in the Internet.Aspects about workload characterization,such as bandwidth utilization,session char-acteristics and server popularity are treated in detail.Further research on RealMedia traffic is presented in[14]. The authors study the traffic both in the application layer and the network layer and the relationship between674R.Garcı´a et al./Simulation Modelling Practice and Theory15(2007)672–689these two layers is investigated.More recently[5],analyzes an enterprise media server workload using access logs from two different servers in HP Laboratories.They divide the media workload properties into two groups:static and temporary,and propose two new metrics to characterize temporary properties of the media workload:newfiles impact metric and life span metric.Video popularity distributions and client behaviour have been treated in this research.A workload generator of streaming media services is presented in[29].This work focuses on the long term behaviour of network services.They proposed a generalized Zipf-like distribution to model the content pop-ularity and partition media workload properties in two groups,static and temporary,as in[5].Daytime access patterns are treated in this paper[10]proposed a new streaming media workload generator,parameterized from the real workload characterizations indicated in[9].3.Case studyThe presented study has been performed on the video on demand service of La Nueva Espan˜a Digital (www.lne.es)which has an important number of accesses and has reached8th position in the ranking of digital news sites in Spain.In2001,www.lne.es presented its video on demand service(LNE TV)developed by the Computer Science Department of the University of Oviedo.The number of visits and the volume of information have risen since then.3.1.Service descriptionThe multimedia section of www.lne.es has an architecture formed by two servers[28].One of them is the streaming server and the other supports the web pages used to access the videos,the analysis system,and a redundant streaming server.The analysis server stores all the modules of the analysis tool[22],including the database,the web server,and data loaders and analyzers.Helix from RealNetworks[24]is the technology used to stream the videos,delivered on demand when a subscriber performs a request.3.2.Content descriptionThe multimedia service contents have been classified into15subsections according to their subject.Some of these subjects are News,Music,Tourism,Science,Cinema,Comedy,Leisure,Sports,Others,etc.The lengths range from30s,the shortest,to more than an hour,the longest.Currently,the service has more than900 videos.3.3.Media server log formatsEvery request processed by the multimedia server is recorded in its logfiles.Logfiles in multimedia services usually provide detailed information about users,resources,requests,and data packet transmission[26].Table 1summarizes the overall statistics of the LNE TV service,obtained by analyzing the logfiles of this service with the tool Fesoria[22].Table1LNE TV service summarized statisticsDuration4Years Different subjects15 Total sessions47,000Storage requirements40GB Total reproductions160,000Bytes transferred188GB Unique clients31,902Bitrate(average)145,170bps Stored videos910Bitrate(standard deviation)84,116bpsR.Garcı´a et al./Simulation Modelling Practice and Theory15(2007)672–689675 er behaviour analysis and characterizationThe analysis presented in this paper has been performed using the information obtained from the logfiles of the multimedia server.Thefinal goal of the analysis is to construct a model of user behaviour.Thus,all the parameters which characterize this behaviour will be evaluated.A preliminary analysis has been presented in [32].Users access the service during a session,which is composed by one or more reproductions with reflection periods(think time)between them(Fig.1a).Each reproduction begins with a play interaction and continues to the end of the audio/video or to a stop interaction.During this time users can perform intermediate inter-actions(pause,forward and backward jumps,play,stop),generating paused periods,when no information is being delivered,and active periods,when users are receiving data.In the model proposed in[10]there is only one reproduction per user session.We have tried to go one step further than this previous definition,analyzing the user behaviour over longer periods,discovering the number of successive reproductions,and the reflection time between them.To distinguish one user session from another,we have considered a minimum gap of30min between two consecutive reproductions,the same as[21,9].Fig.1b shows the conceptual model of the user behaviour in a session,taking into account if the request for a video is correct or invalid,differentiating the characterization for short and long videos depending on the length of the requested video,and taking into account all the available user interactions(pause,jump,play, stop).At the end of a video reproduction the user can request a new video or end the session.The aim of this section is to determine the distributions for all the parameters needed to create an accurate model of user behaviour.Due to the variability in the lengths of the offered videos,we have differentiated the characterization for short(under5min)and long videos,the same as in[16].User behaviour characteristics are evaluated and the most suitable distributions arefitted to the empirically observed data,using maximum likelihood estimators(MLE)[15]and least squares differences.As in[9],in order tofind the distribution that best models the characteristic to be analyzed,we compared the least squares differences of the bestfitted curves for a set of alternative distributions.We also visually compared the curve fittings at the body and at the tail of the measured data,favouring a betterfit for the body or the tail,if nec-essary,in order to analyze its impact on the performance of the service.4.1.Session characteristicsAs Fig.1a shows,there are two essential elements in a session that must be determined:the number of reproductions and the inter-reproduction time(reflection or think time).Regarding the number of reproductions in a session,Fig.2a shows that 47.99%of the sessions present only one reproduction.The concatenation of two Zipf-like distributions,with h 1=1.77(94.28%of the sessions,1–6reproductions)and h 2=3.09(the remaining 5.72%,6to 25reproductions),closely match the empirical values.Fig.2a shows real accesses and estimated values in log–log plots.Zipf-like distribution [36]is represented by the following pdf distribution:p ði Þ¼C i H ;and C ¼1P n i ¼11i Hð1ÞThese results are consistent with [9],where the concatenation of two Zipf-like distributions is suggested as agood approximation model.In order to characterize the inter-reproduction time,a set of alternative distributions (Exponential,Pareto,Normal,Gamma,Weibull)have been tested,obtaining its parameters using MLE [15].By comparing the least squares differences between these best fitted curves and the real data,as in [9],the best adjust is done using a Weibull distribution [15],as is indicated in Fig.2b.Both the body and the tail of the empirical distribution are quite well fitted by the Weibull distribution.Moreover,Kolmogorov-Smirnov and Anderson-Darling tests [15]also demonstrate that Weibull is the most suitable distribution to characterize the inter-reproduction time.These results are consistent with the works in [9,2],where Weibull or Lognormal are the most accurate dis-tributions,depending on file size.The Weibull pdf distribution used in this paper is characterized by the a and b parameters,following the expression:f ðx Þ¼ab x b À1e Àa x bI ð0;1Þðx Þð2ÞFig.2b shows the cumulative distribution of the measured and simulated values,indicating the MLE parameters of the Weibull distribution and the mean of the inter-reproduction times in a session.As Fig.2b indicates,90%of the inter-reproduction times are below 200s,and the heavy-tailed Weibull distribu-tion represents non-zero probabilities of long inter-reproduction times.4.2.Mistaken reproductionsA mistaken reproduction is a user request which generates an error.It can be identified because it generates an entry in the error log or the duration reported in the entry of the access log is 0s.Generally,this type of reproduction is due to the fact that the user does not have the suitable program to watch the video.Despite the fact that Web masters issue warnings and develop plug-in detectors,a significant percentage of the total676R.Garcı´a et al./Simulation Modelling Practice and Theory 15(2007)672–689R.Garcı´a et al./Simulation Modelling Practice and Theory15(2007)672–689677 requests usually fail for this reason.Thus,the mistaken reproductions maintain a percentage of15%during the whole life of the service.4.3.Media delivered per reproductionOne important parameter in the analysis of video on demand services is the quantity of media delivered in a reproduction.Different characterizations have been carried out for short and long videos.Previous studies, like[16],have detected distributions,such as Lognormal and Exponential for short videos(0–5min),and Gamma+Pareto or Gamma+Lognormal for long videos(5–50min)[29]use an aggregated distribution, whose PDF is the sum of the PDFs of a set of normal distributions proportionally.However,the LNE TV service presents the interesting characteristic that a hybrid distribution,i.e.a composition of two distributions,fits well with the length of video reproductions(Fig.3).Using the length reproduction histogram of four representative examples of videos chosen at random (Fig.3),it is possible to appreciate afirst distribution from the(0–10%)interval to(90–100%)and another around100%.The weight of each distribution depends on the success of the analyzed video.Short videos(vid-eos1,2)usually have a second distribution heavier than thefirst,while for long videos(videos3,4)the weight of thefirst distribution is higher than the second.The empirical observed data are modelled,by least squares difference,using the composition of two expo-nential distributions with MLE parameters l1=0.16and l2=0.06,respectively for short videos,and MLE parameters l1=0.2and l2=0.27for long videos.Thefinal formula to calculate the reproduction length distribution is the following:fðxÞ¼pf1ðxÞþð1Àp1Þf2ðxÞð3Þ1f1ðxÞ¼1l1eÀx l10<x<10otherwise(and f2ðxÞ¼1l2eÀxÀ1l2x>10otherwise(where p1is the success probability of the video.The way these distributions combine depends on the type of user who reproduces the video:slightly inter-ested or highly interested.Normally,slightly interested users leave video reproductions during thefirst seconds and,moreover,their interest decreases quickly with time.On the other hand,highly interested users usually watch the entire video,and sometimes make backward jumps to re-watch some parts.In the case of previous studies,such as[6],despite the short length of a considerable amount of the repro-ductions,the majority are between2.5and4.5min.With regard to[16],the main difference can be that LNE TV offers news and entertainment programs,while[16]offered educational information.The visualization of the latter was essential for students to pass their courses,so the behaviour could have been conditioned. 4.4.Reproduction characteristicsThroughout the video reproductions,users can make pauses as well as backward and forward jumps.These user interactions have a significant influence on the on–offperiods in the transmission of media data and the buffer reloads on the clients’side.4.4.1.PausesPauses are important to determine the duration of the on–offperiods in the media ually, reproductions are modelled using on and offperiods to simulate receiving and paused times.Our analysis show that there are relatively few user interactions during the sessions.Fig.4shows the histogram with the percent-ages extracted from LNE TV.In short videos,only4.3%of the reproductions have user interactions,increasing to9.5%in long videos. These results are consistent with[9,2],which report a larger number of user interactions within a video session of longer videos.However,in[9,2]more than20%of the reproductions for longfiles have10or more inter-active requests.This difference is due to the educational content of the analyzed services in[9,2].In the enter-tainment service analyzed in[9],only4%of the reproductions have three or more pauses.A discrete distribution,with the values indicated in Fig.4,has been used to characterize the number of pauses.The length of paused time,which determines the offperiods in the user model,has also been characterized (Fig.5).We have compared the least squares difference of a set of alternative distributions,a Weibull being the most adequate distribution,both for long and short videos.The average value of the pause length is55.73s for short videos and95.09s for long videos.Fig.5shows the cumulative distribution function compared with the measured empirical data and the MLE parameters of the Weibull distribution used in our model.The presence678R.Garcı´a et al./Simulation Modelling Practice and Theory15(2007)672–689R.Garcı´a et al./Simulation Modelling Practice and Theory15(2007)672–689679of heavy-tailed distributions indicates non zero probabilities of long pauses,modelling the real usage of the users in the service.4.4.2.Forward and backward jumpsDespite the fact that forward and backward jumps do not change the on–offperiods of user behaviour,they are important in order to analyze the cache performance in this type of services.The number of forward and backward jumps has been modelled with a Zipf-like distribution,using least squares differences.Real accesses and estimated values for forward jumps are displayed in Fig.6.Similar results are obtained for backward jumps.Table2shows the interactions and the estimated h parameters.The high values of these parameters are due to the fact that most of the sessions do not have forward or backward jumps in the reproduction of the video (97.81%in Fig.6a and96.62%in Fig.6b).In the entertainment service analyzed in[9],asfile size increases the percentage of jumps increases.The percentage of reproductions with jumps in[9]is higher than that found in our analysis.The same results as in[9]were found in the educational workload analyzed in[21].The length of the jumps has also been analyzed and modelled with a Weibull distribution,using MLE and least squares difference.Table3shows the parameters for the heavy-tailed distribution.680R.Garcı´a et al./Simulation Modelling Practice and Theory15(2007)672–689Table2Number of jumps characterizationInteraction Short videos Long videos #Forward jumps Zipf(h=5.80)Zipf(h=5.22) #Backward jumps Zipf(h=4.32)Zipf(h=3.73)Table3Jump length characterizationInteraction Distribution Short videos Long videosForward jump length Weibull a=0.13214Average a=0.10916Averageb=0.5863150.226s b=0.54129113.577s Backward jump length Weibull a=0.08108Average a=0.05959Averageb=0.5985100.097s b=0.59236187.383s These results are consistent with the general rule of[9,21],indicating that the distances of backward jumps are usually longer.In[9],the average jump in distance in either direction is under45s.Our results and the results in[9]are in sharp contrast with the very long average jump distance(over2000s)observed in the MANIC educational system[21].4.5.PopularityAnother important element in the workload study is the popularity of the offered ually, user accesses are compared with a Zipf-like distribution[2,6,9,27,33]with different values of the h parameter. The cacheability of the information will be widely successful when h parameter is high and unproductive when values are close to zero.Yu and Chou[34]propose a caching algorithm to characterize the empirical internal popularity distribution.The performed study has detected that document popularity follows a combination of two Zipf-like distributions with a parameter h1=0.56for98%of the accesses,when the analyzed period is long(Fig.7a).With shorter periods the results present a smaller h1(Fig.7b).The remaining2%of the accesses is considered negligible for caching policies.Previous studies have presented different conclusions[6]detected a low h equal to0.47and[2,9,27]the com-bination of two Zipf-like distributions[29]present a novel generalized Zipf-like distribution to capture the pop-ularity distribution.Fig.7shows,on the left,the results for the total analysis period(4years)and,on the right,for a period of one year.We have used a log–log scale to show the power-law structure of the Zipf law.R.Garcı´a et al./Simulation Modelling Practice and Theory15(2007)672–689681 4.6.Daily access profileAs in[29],the access distribution throughout the day has also been analyzed.There is a period between0 am and10am where the demand is low.The number of accesses increases in the morning to reach the max-imum demand at1pm.From2pm to3pm the number of accesses decreases slightly(this is the typical lunch time in Spain).The number of accesses rises from3pm to8pm,reaching the general maximum at7pm.This temporal evolution is used to model the average number of users who access the service throughout the day.5.Workload characterizationAs the last stage of video on demand service characterization,this section analyzes the basic characteristics of the streaming-media workload.We differentiate the traffic per protocol,device,audio and videoflow,reg-ular load and buffering time.Once the video is coded and stored,the media server transmits the data to the client through the network. Due to the effects of the network,the transmitted information reaches the receiver with a speed different to the playing rate of the client.Besides,the presence of a buffer in the receiver compensates the mismatch between transference and playing rates.Furthermore,the client could request a higher transference rate from the server to avoid the starvation of the receiver buffer.This situation,different from the regular load,is known as buf-fering time.In this paper we differentiate the characterization in regular load and in buffering time intervals.5.1.Streaming-media communicationIn this section we briefly describe the streaming media communications using RealNetworks tools.The media contents are created using RealProducer and stored in RealMedia File Format(RMFF)files.The Real-Time Streaming Protocol(RTSP)controls the streaming session,allowing the client to interact with the server by means of the play,pause,stop,fast-forward and rewind control functions[25].RTSP servers use different transport protocols to send data to RTSP clients.RealNetworks uses Real Data Transport (RDT)protocol as the transport mechanism to deliver data from servers to clients.The decision making pro-cess RealPlayer uses to choose either UDP or TCP as transport protocol to send media data is not publicly documented[8].In our study,we have detected the use of UDP.5.2.RealMedia workloadIn order to characterize the media workload,the most suitable audio/video qualities for the connection char-acteristics of the users that access the service were selected;most of the LNE TV users connect either through ADSL lines or through cable networks.Following the recommendations in[23],qualities of140-32-20and90-16-15have been selected.Thefiles nomenclature follows the format‘‘Total quality(Kbps)–Audio Quality (Kbps)–Frames/s’’.In Table4the most representative information from RMFFfiles is shown[14].Table4Summary information of the RMFFfilesItem140-32-2090-16-15Audio Video Audio Video Total packets53,19137,649Avg.packet size(bytes)465296320288 Max.packet size(bytes)465666320656 Avg.bit rate(bps)32,041107,95916,00074,000 Max.bit rate(bps)32,041107,95916,00074,000 Preroll(ms)185774839606870 Duration(ms)998,504998,502Target frame rate(frames/s)20155.3.Traffic collectionTo produce traces,eachfile is transmitted from the multimedia server exclusively for one user in non-over-lapped experiments.Providing that the main goal of the experiment is to define the traffic introduced in the communications network,traces are captured on the server’s side using Ethereal utility.Ethereal is used to analyze the behaviour of the RTSP and RDT protocols.Hence,we can differentiate audio streams from video streams,analyze control messages(resume,play,pause,buffering)and also obtain the timestamp of the deliv-ered data.Measurements with several qualities of media data indicate that UDP traffic from server to client represents more than96%of the packets and99%of the delivered bytes.Therefore,the consumption of network resources in LNE TV service,using RealNetworks technology,is mainly caused by the UDP-RDT traffic. For this reason we have carried out an accurate analysis to characterize this type of traffic.5.4.Streaming-media traffic characterizationIn this section we analyze and characterize the measured network-layer traffic of the RealMedia streams collected.We have focused the study on the UDP-RDT traffic,differentiating audio streams from video streams by means of the StreamIDfield in the RDT protocol.Moreover,the interaction and synchronism between the different trafficflows has been taken into account in order to construct a model of the media workload.As shown in Fig.8,after the initial negotiations between client and server,TCP traffic is also used to increase the transference rate during the preroll time(initial buffering time to load the buffer of the client [24])and posterior buffering intervals.Fig.8shows the preroll and buffering time intervals delimited by TCP client requests.5.4.1.Analysis summary for the streamsTables5and6show the analysis summary for audio and video streams,with the considered qualities in this research.Packet sizes include IP,UDP and RDT protocol overheads,which constitute38bytes over the media data.During the buffering time intervals the inter-arrival time decreases,approximately four times the usual load intervals,increasing the transference rate to quickly load the receiver buffer.These results are consistent with[7,4]for RealNetworks systems and[18],which work on Windows Stream-ing Media(WSM).We also observed that this increase in the transference rate is only made by reducing the inter-arrival times and not by increasing the sizes of the packets.During the buffering time and regular load intervals the packet size distributions remain invariable.。