CHAPTER 1 THREE GENERATIONS OF COEVOLUTIONARY ROBOTICS
人工智能英文参考文献(最新120个)
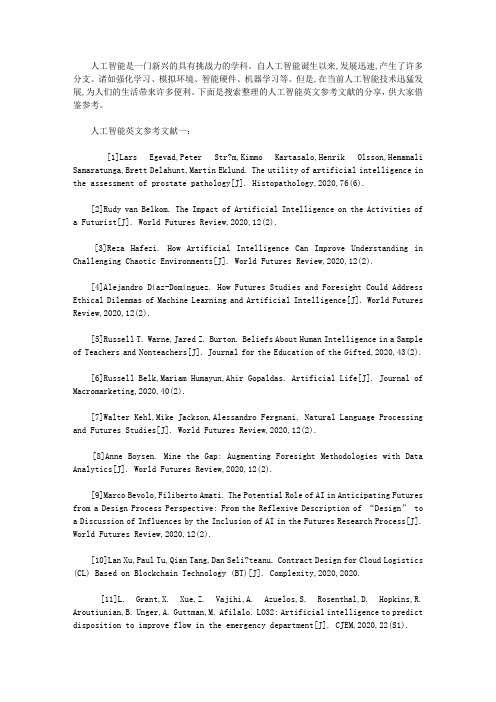
人工智能是一门新兴的具有挑战力的学科。
自人工智能诞生以来,发展迅速,产生了许多分支。
诸如强化学习、模拟环境、智能硬件、机器学习等。
但是,在当前人工智能技术迅猛发展,为人们的生活带来许多便利。
下面是搜索整理的人工智能英文参考文献的分享,供大家借鉴参考。
人工智能英文参考文献一:[1]Lars Egevad,Peter Str?m,Kimmo Kartasalo,Henrik Olsson,Hemamali Samaratunga,Brett Delahunt,Martin Eklund. The utility of artificial intelligence in the assessment of prostate pathology[J]. Histopathology,2020,76(6).[2]Rudy van Belkom. The Impact of Artificial Intelligence on the Activities ofa Futurist[J]. World Futures Review,2020,12(2).[3]Reza Hafezi. How Artificial Intelligence Can Improve Understanding in Challenging Chaotic Environments[J]. World Futures Review,2020,12(2).[4]Alejandro Díaz-Domínguez. How Futures Studies and Foresight Could Address Ethical Dilemmas of Machine Learning and Artificial Intelligence[J]. World Futures Review,2020,12(2).[5]Russell T. Warne,Jared Z. Burton. Beliefs About Human Intelligence in a Sample of Teachers and Nonteachers[J]. Journal for the Education of the Gifted,2020,43(2).[6]Russell Belk,Mariam Humayun,Ahir Gopaldas. Artificial Life[J]. Journal of Macromarketing,2020,40(2).[7]Walter Kehl,Mike Jackson,Alessandro Fergnani. Natural Language Processing and Futures Studies[J]. World Futures Review,2020,12(2).[8]Anne Boysen. Mine the Gap: Augmenting Foresight Methodologies with Data Analytics[J]. World Futures Review,2020,12(2).[9]Marco Bevolo,Filiberto Amati. The Potential Role of AI in Anticipating Futures from a Design Process Perspective: From the Reflexive Description of “Design” to a Discussion of Influences by the Inclusion of AI in the Futures Research Process[J]. World Futures Review,2020,12(2).[10]Lan Xu,Paul Tu,Qian Tang,Dan Seli?teanu. Contract Design for Cloud Logistics (CL) Based on Blockchain Technology (BT)[J]. Complexity,2020,2020.[11]L. Grant,X. Xue,Z. Vajihi,A. Azuelos,S. Rosenthal,D. Hopkins,R. Aroutiunian,B. Unger,A. Guttman,M. Afilalo. LO32: Artificial intelligence to predict disposition to improve flow in the emergency department[J]. CJEM,2020,22(S1).[12]A. Kirubarajan,A. Taher,S. Khan,S. Masood. P071: Artificial intelligence in emergency medicine: A scoping review[J]. CJEM,2020,22(S1).[13]L. Grant,P. Joo,B. Eng,A. Carrington,M. Nemnom,V. Thiruganasambandamoorthy. LO22: Risk-stratification of emergency department syncope by artificial intelligence using machine learning: human, statistics or machine[J]. CJEM,2020,22(S1).[14]Riva Giuseppe,Riva Eleonora. OS for Ind Robots: Manufacturing Robots Get Smarter Thanks to Artificial Intelligence.[J]. Cyberpsychology, behavior and social networking,2020,23(5).[15]Markus M. Obmann,Aurelio Cosentino,Joshy Cyriac,Verena Hofmann,Bram Stieltjes,Daniel T. Boll,Benjamin M. Yeh,Matthias R. Benz. Quantitative enhancement thresholds and machine learning algorithms for the evaluation of renal lesions using single-phase split-filter dual-energy CT[J]. Abdominal Radiology,2020,45(1).[16]Haytham H. Elmousalami,Mahmoud Elaskary. Drilling stuck pipe classification and mitigation in the Gulf of Suez oil fields using artificial intelligence[J]. Journal of Petroleum Exploration and Production Technology,2020,10(10).[17]Rüdiger Schulz-Wendtland,Karin Bock. Bildgebung in der Mammadiagnostik –Ein Ausblick <trans-title xml:lang="en">Imaging in breast diagnostics—an outlook [J]. Der Gyn?kologe,2020,53(6).</trans-title>[18]Nowakowski Piotr,Szwarc Krzysztof,Boryczka Urszula. Combining an artificial intelligence algorithm and a novel vehicle for sustainable e-waste collection[J]. Science of the Total Environment,2020,730.[19]Wang Huaizhi,Liu Yangyang,Zhou Bin,Li Canbing,Cao Guangzhong,Voropai Nikolai,Barakhtenko Evgeny. Taxonomy research of artificial intelligence for deterministic solar power forecasting[J]. Energy Conversion and Management,2020,214.[20]Kagemoto Hiroshi. Forecasting a water-surface wave train with artificial intelligence- A case study[J]. Ocean Engineering,2020,207.[21]Tomonori Aoki,Atsuo Yamada,Kazuharu Aoyama,Hiroaki Saito,Gota Fujisawa,Nariaki Odawara,Ryo Kondo,Akiyoshi Tsuboi,Rei Ishibashi,Ayako Nakada,Ryota Niikura,Mitsuhiro Fujishiro,Shiro Oka,Soichiro Ishihara,Tomoki Matsuda,Masato Nakahori,Shinji Tanaka,Kazuhiko Koike,Tomohiro Tada. Clinical usefulness of a deep learning‐based system as the first screening on small‐bowel capsule endoscopy reading[J]. Digestive Endoscopy,2020,32(4).[22]Masashi Fujii,Hajime Isomoto. Next generation of endoscopy: Harmony with artificial intelligence and robotic‐assisted devices[J]. Digestive Endoscopy,2020,32(4).[23]Roberto Verganti,Luca Vendraminelli,Marco Iansiti. Innovation and Design in the Age of Artificial Intelligence[J]. Journal of Product Innovation Management,2020,37(3).[24]Yuval Elbaz,David Furman,Maytal Caspary Toroker. Modeling Diffusion in Functional Materials: From Density Functional Theory to Artificial Intelligence[J]. Advanced Functional Materials,2020,30(18).[25]Dinesh Visva Gunasekeran,Tien Yin Wong. Artificial Intelligence in Ophthalmology in 2020: A Technology on the Cusp for Translation and Implementation[J]. Asia-Pacific Journal of Ophthalmology,2020,9(2).[26]Fu-Neng Jiang,Li-Jun Dai,Yong-Ding Wu,Sheng-Bang Yang,Yu-Xiang Liang,Xin Zhang,Cui-Yun Zou,Ren-Qiang He,Xiao-Ming Xu,Wei-De Zhong. The study of multiple diagnosis models of human prostate cancer based on Taylor database by artificial neural networks[J]. Journal of the Chinese Medical Association,2020,83(5).[27]Matheus Calil Faleiros,Marcello Henrique Nogueira-Barbosa,Vitor Faeda Dalto,JoséRaniery Ferreira Júnior,Ariane Priscilla Magalh?es Tenório,Rodrigo Luppino-Assad,Paulo Louzada-Junior,Rangaraj Mandayam Rangayyan,Paulo Mazzoncini de Azevedo-Marques. Machine learning techniques for computer-aided classification of active inflammatory sacroiliitis in magnetic resonance imaging[J]. Advances in Rheumatology,2020,60(1078).[28]Balamurugan Balakreshnan,Grant Richards,Gaurav Nanda,Huachao Mao,Ragu Athinarayanan,Joseph Zaccaria. PPE Compliance Detection using Artificial Intelligence in Learning Factories[J]. Procedia Manufacturing,2020,45.[29]M. Stévenin,V. Avisse,N. Ducarme,A. de Broca. Qui est responsable si un robot autonome vient à entra?ner un dommage ?[J]. Ethique et Santé,2020.[30]Fatemeh Barzegari Banadkooki,Mohammad Ehteram,Fatemeh Panahi,Saad Sh. Sammen,Faridah Binti Othman,Ahmed EL-Shafie. Estimation of Total Dissolved Solids (TDS) using New Hybrid Machine Learning Models[J]. Journal of Hydrology,2020.[31]Adam J. Schwartz,Henry D. Clarke,Mark J. Spangehl,Joshua S. Bingham,DavidA. Etzioni,Matthew R. Neville. Can a Convolutional Neural Network Classify Knee Osteoarthritis on Plain Radiographs as Accurately as Fellowship-Trained Knee Arthroplasty Surgeons?[J]. The Journal of Arthroplasty,2020.[32]Ivana Nizetic Kosovic,Toni Mastelic,Damir Ivankovic. Using Artificial Intelligence on environmental data from Internet of Things for estimating solar radiation: Comprehensive analysis[J]. Journal of Cleaner Production,2020.[33]Lauren Fried,Andrea Tan,Shirin Bajaj,Tracey N. Liebman,David Polsky,Jennifer A. Stein. Technological advances for the detection of melanoma: Part I. Advances in diagnostic techniques[J]. Journal of the American Academy of Dermatology,2020.[34]Mohammed Amoon,Torki Altameem,Ayman Altameem. Internet of things Sensor Assisted Security and Quality Analysis for Health Care Data Sets Using Artificial Intelligent Based Heuristic Health Management System[J]. Measurement,2020.[35]E. Lotan,C. Tschider,D.K. Sodickson,A. Caplan,M. Bruno,B. Zhang,Yvonne W. Lui. Medical Imaging and Privacy in the Era of Artificial Intelligence: Myth, Fallacy, and the Future[J]. Journal of the American College of Radiology,2020.[36]Fabien Lareyre,Cédric Adam,Marion Carrier,Juliette Raffort. Artificial Intelligence in Vascular Surgery: moving from Big Data to Smart Data[J]. Annals of Vascular Surgery,2020.[37]Ilesanmi Daniyan,Khumbulani Mpofu,Moses Oyesola,Boitumelo Ramatsetse,Adefemi Adeodu. Artificial intelligence for predictive maintenance in the railcar learning factories[J]. Procedia Manufacturing,2020,45.[38]Janet L. McCauley,Anthony E. Swartz. Reframing Telehealth[J]. Obstetrics and Gynecology Clinics of North America,2020.[39]Jean-Emmanuel Bibault,Lei Xing. Screening for chronic obstructive pulmonary disease with artificial intelligence[J]. The Lancet Digital Health,2020,2(5).[40]Andrea Laghi. Cautions about radiologic diagnosis of COVID-19 infection driven by artificial intelligence[J]. The Lancet Digital Health,2020,2(5).人工智能英文参考文献二:[41]K. Orhan,I. S. Bayrakdar,M. Ezhov,A. Kravtsov,T. ?zyürek. Evaluation of artificial intelligence for detecting periapical pathosis on cone‐beam computed tomography scans[J]. International Endodontic Journal,2020,53(5).[42]Avila A M,Mezi? I. Data-driven analysis and forecasting of highway traffic dynamics.[J]. Nature communications,2020,11(1).[43]Neri Emanuele,Miele Vittorio,Coppola Francesca,Grassi Roberto. Use of CT andartificial intelligence in suspected or COVID-19 positive patients: statement of the Italian Society of Medical and Interventional Radiology.[J]. La Radiologia medica,2020.[44]Tau Noam,Stundzia Audrius,Yasufuku Kazuhiro,Hussey Douglas,Metser Ur. Convolutional Neural Networks in Predicting Nodal and Distant Metastatic Potential of Newly Diagnosed Non-Small Cell Lung Cancer on FDG PET Images.[J]. AJR. American journal of roentgenology,2020.[45]Coppola Francesca,Faggioni Lorenzo,Regge Daniele,Giovagnoni Andrea,Golfieri Rita,Bibbolino Corrado,Miele Vittorio,Neri Emanuele,Grassi Roberto. Artificial intelligence: radiologists' expectations and opinions gleaned from a nationwide online survey.[J]. La Radiologia medica,2020.[46]?. ? ? ? ? [J]. ,2020,25(4).[47]Savage Rock H,van Assen Marly,Martin Simon S,Sahbaee Pooyan,Griffith Lewis P,Giovagnoli Dante,Sperl Jonathan I,Hopfgartner Christian,K?rgel Rainer,Schoepf U Joseph. Utilizing Artificial Intelligence to Determine Bone Mineral Density Via Chest Computed Tomography.[J]. Journal of thoracic imaging,2020,35 Suppl 1.[48]Brzezicki Maksymilian A,Bridger Nicholas E,Kobeti? Matthew D,Ostrowski Maciej,Grabowski Waldemar,Gill Simran S,Neumann Sandra. Artificial intelligence outperforms human students in conducting neurosurgical audits.[J]. Clinical neurology and neurosurgery,2020,192.[49]Lockhart Mark E,Smith Andrew D. Fatty Liver Disease: Artificial Intelligence Takes on the Challenge.[J]. Radiology,2020,295(2).[50]Wood Edward H,Korot Edward,Storey Philip P,Muscat Stephanie,Williams George A,Drenser Kimberly A. The retina revolution: signaling pathway therapies, genetic therapies, mitochondrial therapies, artificial intelligence.[J]. Current opinion in ophthalmology,2020,31(3).[51]Ho Dean,Quake Stephen R,McCabe Edward R B,Chng Wee Joo,Chow Edward K,Ding Xianting,Gelb Bruce D,Ginsburg Geoffrey S,Hassenstab Jason,Ho Chih-Ming,Mobley William C,Nolan Garry P,Rosen Steven T,Tan Patrick,Yen Yun,Zarrinpar Ali. Enabling Technologies for Personalized and Precision Medicine.[J]. Trends in biotechnology,2020,38(5).[52]Fischer Andreas M,Varga-Szemes Akos,van Assen Marly,Griffith L Parkwood,Sahbaee Pooyan,Sperl Jonathan I,Nance John W,Schoepf U Joseph. Comparison of Artificial Intelligence-Based Fully Automatic Chest CT Emphysema Quantification to Pulmonary Function Testing.[J]. AJR. American journal ofroentgenology,2020,214(5).[53]Moore William,Ko Jane,Gozansky Elliott. Artificial Intelligence Pertaining to Cardiothoracic Imaging and Patient Care: Beyond Image Interpretation.[J]. Journal of thoracic imaging,2020,35(3).[54]Hwang Eui Jin,Park Chang Min. Clinical Implementation of Deep Learning in Thoracic Radiology: Potential Applications and Challenges.[J]. Korean journal of radiology,2020,21(5).[55]Mateen Bilal A,David Anna L,Denaxas Spiros. Electronic Health Records to Predict Gestational Diabetes Risk.[J]. Trends in pharmacological sciences,2020,41(5).[56]Yao Xiang,Mao Ling,Lv Shunli,Ren Zhenghong,Li Wentao,Ren Ke. CT radiomics features as a diagnostic tool for classifying basal ganglia infarction onset time.[J]. Journal of the neurological sciences,2020,412.[57]van Assen Marly,Banerjee Imon,De Cecco Carlo N. Beyond the Artificial Intelligence Hype: What Lies Behind the Algorithms and What We Can Achieve.[J]. Journal of thoracic imaging,2020,35 Suppl 1.[58]Guzik Tomasz J,Fuster Valentin. Leaders in Cardiovascular Research: Valentin Fuster.[J]. Cardiovascular research,2020,116(6).[59]Fischer Andreas M,Eid Marwen,De Cecco Carlo N,Gulsun Mehmet A,van Assen Marly,Nance John W,Sahbaee Pooyan,De Santis Domenico,Bauer Maximilian J,Jacobs Brian E,Varga-Szemes Akos,Kabakus Ismail M,Sharma Puneet,Jackson Logan J,Schoepf U Joseph. Accuracy of an Artificial Intelligence Deep Learning Algorithm Implementing a Recurrent Neural Network With Long Short-term Memory for the Automated Detection of Calcified Plaques From Coronary Computed Tomography Angiography.[J]. Journal of thoracic imaging,2020,35 Suppl 1.[60]Ghosh Adarsh,Kandasamy Devasenathipathy. Interpretable Artificial Intelligence: Why and When.[J]. AJR. American journal of roentgenology,2020,214(5).[61]M.Rosario González-Rodríguez,M.Carmen Díaz-Fernández,Carmen Pacheco Gómez. Facial-expression recognition: An emergent approach to the measurement of tourist satisfaction through emotions[J]. Telematics and Informatics,2020,51.[62]Ru-Xi Ding,Iván Palomares,Xueqing Wang,Guo-Rui Yang,Bingsheng Liu,Yucheng Dong,Enrique Herrera-Viedma,Francisco Herrera. Large-Scale decision-making: Characterization, taxonomy, challenges and future directions from an Artificial Intelligence and applications perspective[J]. Information Fusion,2020,59.[63]Abdulrhman H. Al-Jebrni,Brendan Chwyl,Xiao Yu Wang,Alexander Wong,Bechara J. Saab. AI-enabled remote and objective quantification of stress at scale[J]. Biomedical Signal Processing and Control,2020,59.[64]Gillian Thomas,Elizabeth Eisenhauer,Robert G. Bristow,Cai Grau,Coen Hurkmans,Piet Ost,Matthias Guckenberger,Eric Deutsch,Denis Lacombe,Damien C. Weber. The European Organisation for Research and Treatment of Cancer, State of Science in radiation oncology and priorities for clinical trials meeting report[J]. European Journal of Cancer,2020,131.[65]Muhammad Asif. Are QM models aligned with Industry 4.0? A perspective on current practices[J]. Journal of Cleaner Production,2020,258.[66]Siva Teja Kakileti,Himanshu J. Madhu,Geetha Manjunath,Leonard Wee,Andre Dekker,Sudhakar Sampangi. Personalized risk prediction for breast cancer pre-screening using artificial intelligence and thermal radiomics[J]. Artificial Intelligence In Medicine,2020,105.[67]. Evaluation of Payer Budget Impact Associated with the Use of Artificial Intelligence in Vitro Diagnostic, Kidneyintelx, to Modify DKD Progression:[J]. American Journal of Kidney Diseases,2020,75(5).[68]Rohit Nishant,Mike Kennedy,Jacqueline Corbett. Artificial intelligence for sustainability: Challenges, opportunities, and a research agenda[J]. International Journal of Information Management,2020,53.[69]Hoang Nguyen,Xuan-Nam Bui. Soft computing models for predicting blast-induced air over-pressure: A novel artificial intelligence approach[J]. Applied Soft Computing Journal,2020,92.[70]Benjamin S. Hopkins,Aditya Mazmudar,Conor Driscoll,Mark Svet,Jack Goergen,Max Kelsten,Nathan A. Shlobin,Kartik Kesavabhotla,Zachary A Smith,Nader S Dahdaleh. Using artificial intelligence (AI) to predict postoperative surgical site infection: A retrospective cohort of 4046 posterior spinal fusions[J]. Clinical Neurology and Neurosurgery,2020,192.[71]Mei Yang,Runze Zhou,Xiangjun Qiu,Xiangfei Feng,Jian Sun,Qunshan Wang,Qiufen Lu,Pengpai Zhang,Bo Liu,Wei Li,Mu Chen,Yan Zhao,Binfeng Mo,Xin Zhou,Xi Zhang,Yingxue Hua,Jin Guo,Fangfang Bi,Yajun Cao,Feng Ling,Shengming Shi,Yi-Gang Li. Artificial intelligence-assisted analysis on the association between exposure to ambient fine particulate matter and incidence of arrhythmias in outpatients of Shanghai community hospitals[J]. Environment International,2020,139.[72]Fatemehalsadat Madaeni,Rachid Lhissou,Karem Chokmani,Sebastien Raymond,Yves Gauthier. Ice jam formation, breakup and prediction methods based on hydroclimatic data using artificial intelligence: A review[J]. Cold Regions Science and Technology,2020,174.[73]Steve Chukwuebuka Arum,David Grace,Paul Daniel Mitchell. A review of wireless communication using high-altitude platforms for extended coverage and capacity[J]. Computer Communications,2020,157.[74]Yong-Hong Kuo,Nicholas B. Chan,Janny M.Y. Leung,Helen Meng,Anthony Man-Cho So,Kelvin K.F. Tsoi,Colin A. Graham. An Integrated Approach of Machine Learning and Systems Thinking for Waiting Time Prediction in an Emergency Department[J]. International Journal of Medical Informatics,2020,139.[75]Matteo Terzi,Gian Antonio Susto,Pratik Chaudhari. Directional adversarial training for cost sensitive deep learning classification applications[J]. Engineering Applications of Artificial Intelligence,2020,91.[76]Arman Kilic. Artificial Intelligence and Machine Learning in Cardiovascular Health Care[J]. The Annals of Thoracic Surgery,2020,109(5).[77]Hossein Azarmdel,Ahmad Jahanbakhshi,Seyed Saeid Mohtasebi,Alfredo Rosado Mu?oz. Evaluation of image processing technique as an expert system in mulberry fruit grading based on ripeness level using artificial neural networks (ANNs) and support vector machine (SVM)[J]. Postharvest Biology and Technology,2020,166.[78]Wafaa Wardah,Abdollah Dehzangi,Ghazaleh Taherzadeh,Mahmood A. Rashid,M.G.M. Khan,Tatsuhiko Tsunoda,Alok Sharma. Predicting protein-peptide binding sites with a deep convolutional neural network[J]. Journal of Theoretical Biology,2020,496.[79]Francisco F.X. Vasconcelos,Róger M. Sarmento,Pedro P. Rebou?as Filho,Victor Hugo C. de Albuquerque. Artificial intelligence techniques empowered edge-cloud architecture for brain CT image analysis[J]. Engineering Applications of Artificial Intelligence,2020,91.[80]Masaaki Konishi. Bioethanol production estimated from volatile compositions in hydrolysates of lignocellulosic biomass by deep learning[J]. Journal of Bioscience and Bioengineering,2020,129(6).人工智能英文参考文献三:[81]J. Kwon,K. Kim. Artificial Intelligence for Early Prediction of Pulmonary Hypertension Using Electrocardiography[J]. Journal of Heart and Lung Transplantation,2020,39(4).[82]C. Maathuis,W. Pieters,J. van den Berg. Decision support model for effects estimation and proportionality assessment for targeting in cyber operations[J]. Defence Technology,2020.[83]Samer Ellahham. Artificial Intelligence in Diabetes Care[J]. The American Journal of Medicine,2020.[84]Yi-Ting Hsieh,Lee-Ming Chuang,Yi-Der Jiang,Tien-Jyun Chang,Chung-May Yang,Chang-Hao Yang,Li-Wei Chan,Tzu-Yun Kao,Ta-Ching Chen,Hsuan-Chieh Lin,Chin-Han Tsai,Mingke Chen. Application of deep learning image assessment software VeriSee? for diabetic retinopathy screening[J]. Journal of the Formosan Medical Association,2020.[85]Emre ARTUN,Burak KULGA. Selection of candidate wells for re-fracturing in tight gas sand reservoirs using fuzzy inference[J]. Petroleum Exploration and Development Online,2020,47(2).[86]Alberto Arenal,Cristina Armu?a,Claudio Feijoo,Sergio Ramos,Zimu Xu,Ana Moreno. Innovation ecosystems theory revisited: The case of artificial intelligence in China[J]. Telecommunications Policy,2020.[87]T. Som,M. Dwivedi,C. Dubey,A. Sharma. Parametric Studies on Artificial Intelligence Techniques for Battery SOC Management and Optimization of Renewable Power[J]. Procedia Computer Science,2020,167.[88]Bushra Kidwai,Nadesh RK. Design and Development of Diagnostic Chabot for supporting Primary Health Care Systems[J]. Procedia Computer Science,2020,167.[89]Asl? Bozda?,Ye?im Dokuz,?znur Begüm G?k?ek. Spatial prediction of PM 10 concentration using machine learning algorithms in Ankara, Turkey[J]. Environmental Pollution,2020.[90]K.P. Smith,J.E. Kirby. Image analysis and artificial intelligence in infectious disease diagnostics[J]. Clinical Microbiology and Infection,2020.[91]Alklih Mohamad YOUSEF,Ghahfarokhi Payam KAVOUSI,Marwan ALNUAIMI,Yara ALATRACH. Predictive data analytics application for enhanced oil recovery in a mature field in the Middle East[J]. Petroleum Exploration and Development Online,2020,47(2).[92]Omer F. Ahmad,Danail Stoyanov,Laurence B. Lovat. Barriers and pitfalls for artificial intelligence in gastroenterology: Ethical and regulatory issues[J]. Techniques and Innovations in Gastrointestinal Endoscopy,2020,22(2).[93]Sanne A. Hoogenboom,Ulas Bagci,Michael B. Wallace. Artificial intelligence in gastroenterology. The current state of play and the potential. How will it affect our practice and when?[J]. Techniques and Innovations in Gastrointestinal Endoscopy,2020,22(2).[94]Douglas K. Rex. Can we do resect and discard with artificial intelligence-assisted colon polyp “optical biopsy?”[J]. Techniques and Innovations in Gastrointestinal Endoscopy,2020,22(2).[95]Neal Shahidi,Michael J. Bourke. Can artificial intelligence accurately diagnose endoscopically curable gastrointestinal cancers?[J]. Techniques and Innovations in Gastrointestinal Endoscopy,2020,22(2).[96]Michael Byrne. Artificial intelligence in gastroenterology[J]. Techniques and Innovations in Gastrointestinal Endoscopy,2020,22(2).[97]Piet C. de Groen. Using artificial intelligence to improve adequacy of inspection in gastrointestinal endoscopy[J]. Techniques and Innovations in Gastrointestinal Endoscopy,2020,22(2).[98]Robin Zachariah,Andrew Ninh,William Karnes. Artificial intelligence for colon polyp detection: Why should we embrace this?[J]. Techniques and Innovations in Gastrointestinal Endoscopy,2020,22(2).[99]Alexandra T. Greenhill,Bethany R. Edmunds. A primer of artificial intelligence in medicine[J]. Techniques and Innovations in Gastrointestinal Endoscopy,2020,22(2).[100]Tomohiro Tada,Toshiaki Hirasawa,Toshiyuki Yoshio. The role for artificial intelligence in evaluation of upper GI cancer[J]. Techniques and Innovations in Gastrointestinal Endoscopy,2020,22(2).[101]Yahui Jiang,Meng Yang,Shuhao Wang,Xiangchun Li,Yan Sun. Emerging role of deep learning‐based artificial intelligence in tumor pathology[J]. Cancer Communications,2020,40(4).[102]Kristopher D. Knott,Andreas Seraphim,Joao B. Augusto,Hui Xue,Liza Chacko,Nay Aung,Steffen E. Petersen,Jackie A. Cooper,Charlotte Manisty,Anish N. Bhuva,Tushar Kotecha,Christos V. Bourantas,Rhodri H. Davies,Louise A.E. Brown,Sven Plein,Marianna Fontana,Peter Kellman,James C. Moon. The Prognostic Significance of Quantitative Myocardial Perfusion: An Artificial Intelligence–Based Approach Using Perfusion Mapping[J]. Circulation,2020,141(16).[103]Muhammad Asad,Ahmed Moustafa,Takayuki Ito. FedOpt: Towards Communication Efficiency and Privacy Preservation in Federated Learning[J]. Applied Sciences,2020,10(8).[104]Wu Wenzhi,Zhang Yan,Wang Pu,Zhang Li,Wang Guixiang,Lei Guanghui,Xiao Qiang,Cao Xiaochen,Bian Yueran,Xie Simiao,Huang Fei,Luo Na,Zhang Jingyuan,Luo Mingyan. Psychological stress of medical staffs during outbreak of COVID-19 and adjustment strategy.[J]. Journal of medical virology,2020.[105]. Eyenuk Fulfills Contract for Artificial Intelligence Grading of Retinal Images[J]. Telecomworldwire,2020.[106]Kim Tae Woo,Duhachek Adam. Artificial Intelligence and Persuasion: A Construal-Level Account.[J]. Psychological science,2020,31(4).[107]McCall Becky. COVID-19 and artificial intelligence: protecting health-care workers and curbing the spread.[J]. The Lancet. Digital health,2020,2(4).[108]Alca?iz Mariano,Chicchi Giglioli Irene A,Sirera Marian,Minissi Eleonora,Abad Luis. [Autism spectrum disorder biomarkers based on biosignals, virtual reality and artificial intelligence].[J]. Medicina,2020,80 Suppl 2.[109]Cong Lei,Feng Wanbing,Yao Zhigang,Zhou Xiaoming,Xiao Wei. Deep Learning Model as a New Trend in Computer-aided Diagnosis of Tumor Pathology for Lung Cancer.[J]. Journal of Cancer,2020,11(12).[110]Wang Fengdan,Gu Xiao,Chen Shi,Liu Yongliang,Shen Qing,Pan Hui,Shi Lei,Jin Zhengyu. Artificial intelligence system can achieve comparable results to experts for bone age assessment of Chinese children with abnormal growth and development.[J]. PeerJ,2020,8.[111]Hu Wenmo,Yang Huayu,Xu Haifeng,Mao Yilei. Radiomics based on artificial intelligence in liver diseases: where we are?[J]. Gastroenterology report,2020,8(2).[112]Batayneh Wafa,Abdulhay Enas,Alothman Mohammad. Prediction of the performance of artificial neural networks in mapping sEMG to finger joint angles via signal pre-investigation techniques.[J]. Heliyon,2020,6(4).[113]Aydin Emrah,Türkmen ?nan Utku,Namli G?zde,?ztürk ?i?dem,Esen Ay?e B,Eray Y Nur,Ero?lu Egemen,Akova Fatih. A novel and simple machine learning algorithm for preoperative diagnosis of acute appendicitis in children.[J]. Pediatric surgery international,2020.[114]Ellahham Samer. Artificial Intelligence in Diabetes Care.[J]. The Americanjournal of medicine,2020.[115]David J. Winkel,Thomas J. Weikert,Hanns-Christian Breit,Guillaume Chabin,Eli Gibson,Tobias J. Heye,Dorin Comaniciu,Daniel T. Boll. Validation of a fully automated liver segmentation algorithm using multi-scale deep reinforcement learning and comparison versus manual segmentation[J]. European Journal of Radiology,2020,126.[116]Binjie Fu,Guoshu Wang,Mingyue Wu,Wangjia Li,Yineng Zheng,Zhigang Chu,Fajin Lv. Influence of CT effective dose and convolution kernel on the detection of pulmonary nodules in different artificial intelligence software systems: A phantom study[J]. European Journal of Radiology,2020,126.[117]Georgios N. Kouziokas. A new W-SVM kernel combining PSO-neural network transformed vector and Bayesian optimized SVM in GDP forecasting[J]. Engineering Applications of Artificial Intelligence,2020,92.[118]Qingsong Ruan,Zilin Wang,Yaping Zhou,Dayong Lv. A new investor sentiment indicator ( ISI ) based on artificial intelligence: A powerful return predictor in China[J]. Economic Modelling,2020,88.[119]Mohamed Abdel-Basset,Weiping Ding,Laila Abdel-Fatah. The fusion of Internet of Intelligent Things (IoIT) in remote diagnosis of obstructive Sleep Apnea: A survey and a new model[J]. Information Fusion,2020,61.[120]Federico Caobelli. Artificial intelligence in medical imaging: Game over for radiologists?[J]. European Journal of Radiology,2020,126.以上就是关于人工智能参考文献的分享,希望对你有所帮助。
机器人学导论(英) 第三讲
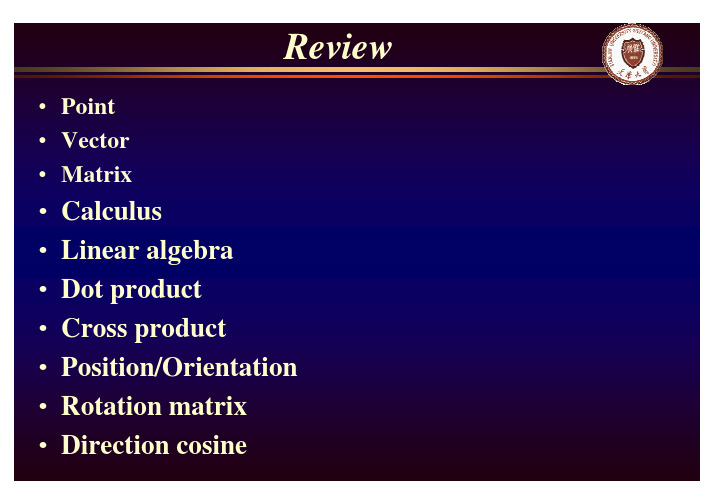
•Point•Vector•Matrix•Calculus•Linear algebra •Dot product •Cross product •Position/Orientation •Rotation matrix •Direction cosine•Coordinate system •Frame•Mapping •Translation •Rotation •Homogeneous transorm •Cross product •Operator •Translational operator •Rotational operator•Transformation operator •Compound transformation •Inverting a transformChapter3 Manipulator Kinematics•What is kinematics ?Kinematics is the sciences of motion that treats the subject without regard to the forces that cause it.•Example:With the knowledge of manipulator’s link length and joint angles, how to compute the position and orientation of the manipulatorsIn kinematics, we consider about the position, the velocity,the acceleration, and the higher derivatives of the position.The study of kinematics refers to all the geometrical and time-based properties of the motion.•Forward kinematicsGiven the joint variables of the robot, determine the position and orientation of the end-effector.[]12...T n θθθΘ=),,,,,(αβγz y x Y =•Kinematic ChainA robot can be treated as a set of right bodies (rigid links) connected together at various joints.•Lower pair:describe the connection between a pair of bodies when relative motion is characterized by two surface sliding over on another.•Joint type (lower pair):1. Revolute2. Prismatic3. Cylindrical4. Planar5. Screw6. Spherical•We consider the joint with 1degree-of-freedom (DOF). A joint with m DOF can be modeled as m joints of 1DOF connected by m-1links of zero length.•The action of joint can be described by a single real number: the angle of rotation in the case of a revolute joint, or the displacement in the case of a prismatic joint.•Number of the links starts from the immobile base (link 0), the first moving body is link 1and so on, out to the freed end of the arm, which is link n .…link1link2link0link6•In kinematics, a link is considered as a rigid body that define the relationship between two neighboring joint axes.1−i a axis i-1axis ilink i-11−i α1−i α:twist angle•Two parameters: link length and link twist angle are used to define the relative location of the two axes.•L ink length: the distance between two axes is measured along a line that is mutually perpendicular to both axes. This line is unique except in the case that two axes are parallel.axis i-1axis ilink i-1a1−ia:link length1−i•Imagine that a plane, whose normal is the mutuallyperpendicular line just constructed, we can project the axis i-1and axis i onto that plane and measure the angle from axis i-1to axis i by the right-hand rule .axis i-1axis ilink n-11−i α:twist angle1−i a•Intermediate links in the chain:Neighboring links has a common joint axis between them. The distance along this common axis from one link to another is defined as “link offset”. It will be variable for prismatic joint.axis i-1axis ilink i-11−iαid1−iaiθiaid:link offset•The angle that rotates about this common axis between one link and its neighboring link is defined as “joint angle ”. It will be variable for revolute joint.axis i-1axis ilink i-11−i αid 1−i a iθia i θ:joint angle•Link length and link twist angle depend on joint axes i and i+1.•For first link in the chain, we set and .00=a 00=α•For last link in the chain, we set and .0=n a 0=n α•If joint 1 is revolute, is set to be 0, zero position ofwill be chosen arbitrarily.1d 1θ•If joint 1 is prismatic, is set to be 0; zero position ofwill be chosen arbitrarily.1θ1d•Link parameters¾For revolute joint, the joint angle is called “joint variable”, and the other three quantities are fixed link parameters.¾For prismatic joint, the link offset is called “jointvariable”, and the other three link quantities are fixed link parameters.θd •Any robot can be described kinematically by giving the values of four quantities for each link, i.e., Denavit-Hartenberg notation[]i i iid a θαvariableconstant• A frame is attached to each link to describe the location of a link relative to its neighbors.•The link frame are named by number according to the link to which they are attached. Step1: frame {i}link {i}link1link2link0link6{0}{1}{2}{6}•Step2: intermediate link in the chain:iZ ˆjoint axis i frame {i}axis i-1axis ilink i-11−i αid 1−i a iθia 1ˆ−i Z 1ˆ−i X 1ˆ−i Y iZ ˆiX ˆiY ˆlink i•Step 3: direction of -along , from joint i to joint i+1iX ˆi a axis i-1axis ilink i-11−i αid 1−i a iθia 1ˆ−i Z 1ˆ−i X 1ˆ−i Y iZ ˆiX ˆiY ˆlink i•Step 4: direction of can be determined by right-hand rule ˆiY•How about if intersect ?is normal to the plane of and .i Z ˆ1ˆ+i Z iX ˆi Z ˆ1ˆ+i Z •First link in the chainsSelection of frame {0} is arbitrarily, will be selected along .0ˆZ 1ˆZ •Last link in the chainsFor revolute joint is chosen along when , the origin of frame {n} is chosen to set . n X ˆ0=n d 1ˆ−n X 0=n θFor prismatic joint, the direction of is chose to make and the origin of {n} is chosen at the intersection of and joint axis n when .0=n θnX ˆ1ˆ−n X 0=n daxis i-1axis ilink i-11−i αid 1−i a iθia 1ˆ−i Z 1ˆ−i X 1ˆ−i Y iZ ˆiX ˆiY ˆlink i•Summary of link parameters: the distance from to measured along : the angle from to measured about : the distance from to measured along : the angle from to measured about iX ˆi a i αi Z ˆ1ˆ+i Z i Z ˆ1ˆ+i Z iX ˆi d i θ1ˆ−i X i X ˆi Z ˆ1ˆ−i X iX ˆi Z ˆ•Summary of link-frame attachment procedure:Step1: identify the join axis (revolute joint/prismatic joint)Step2: identify the common perpendicular between two neighbouring joint axes, at the point of where the common perpendicular meets the i-th axis, assign the link-frame origin. (special case: the two axes intersect)Step3: assign the axis pointing along the i-th joint axis Step4: assign the axis pointing along the common perpendicular (special case: the two axes intersect)iX ˆi Z ˆStep5: assign the axis to complete a right-hand coordinate system.iY ˆStep6: assign the {0} to match {1} when first joint variable is 0.For {n}, chosen origin and directly of freely, but so as to cause as many link parameters as possible to be zero.nX ˆExample 3.3 in the text book(a)(b)Example 3.4 in the text bookwhere two adjacentaxes intersect(a)(b)D-H Tablei1−i a 1−i αid i θ11ˆZ 1ˆX 2ˆZ 2ˆX 3ˆZ 3ˆX 2L Link1Link2Link323D 90000)(2t d 2L )(1t θ)(3t θFrame {0} is coincident with frame {1}.Example 3.5 in the text bookwhere two adjacentaxes intersect•It is possible that there is no unique attachment of frames to link and result in several possible D-H representation.¾There are two choice of direction in which to point .¾In the case of intersecting axes, there are two choices fordirection of . 2ˆZ iX ˆ01=a 901−=α01=d 22L a =02=α12L d =1ˆZ 1ˆX 2ˆZ 2ˆX 3ˆZ 1L 2L case 111()t θθ=22()t θθ=−1=a 901=α01=d 22L a =02=α12L d −=1ˆZ 1ˆX 2ˆZ 2ˆX 3ˆZ 1L 2L case 2•When the direction of is changed, some link parameters willalso be changed. There are four more possible assignments offrames with pointing down.1X 11()t θθ=22()t θθ=1ˆZ•The above problem will be broken into four sub-sub-problems.Each sub-sub problem will be a function of only one link parameter.[]i i i i d a θα11−−Ti i1−•Sub-problem: construct the transform that define frame {i} to frame {i-1}•Problem statement: solve for the position and orientation of link n relative to link 0.•The problem can be broken into n sub-problem. Each sub-problem will involve transformation from one link to its neighboring link.•Introduce three intermediate frames: {P}, {Q}, and {R} for each link for transformationaxis i-1axis ilink n-11−iαid1−iaiθia 1ˆ−iZ1ˆ−iXiZˆiXˆRZˆRXˆQXˆQZˆPZˆPXˆ•Step1: {i}--->{P}, translation transform•Step2: {P}--->{Q}, rotation transform•Step3: {Q}--->{R}, translation transform•Step4: {R}--->{i-1}, rotation transform)(ˆi z P id D T i =)(ˆi z Q Pi R T θ=)(1ˆ1−−=i X R Qa D T i )(1ˆ11−−−=i X i Ri R T α)()()()(ˆˆ1ˆ1ˆ111i Z i Z i X i X i id D R a D R T iii i θα−−−−−=We can write))()()((11T T T T T P iQ P R Q i R i i−−=⎥⎥⎥⎥⎦⎤⎢⎢⎢⎢⎣⎡−−−=−−−−−−−−−−10001111111111i i i i i i i i i i i i i i i i i i i d c c s c s s d s s c c c s a s c T αααθαθαααθαθθθBy substituting the link parameters into above equation, we have•The Unimation Puma 560 is a robot with 6 degree of freedom, and all rotational joints (6R).•The link frames are assigned in the position corresponding to all joint angles equal to 0.forearm•Join axes of joint 4,5,6 intersect at a common point, and the joint axes 4,5,6 are mutually orthogonal. This design is used in many industrial robot.Schematic of the 3R wrist of PUMA 560•Link parameters of the PUMA 560 is listed in the following D-H table.•Transformation of each link can be computed as follows:•The transformation of all six link:•For a manipulator with n DOF, the position of all the links can be specified with a set of n joint variable. This set is often called as joint vector.•The space of joint vectors is referred as joint space.•Cartensian space: position is measured along orthogonal axes, and orientation is described by the rotation matrix wespecified before.•Cartensian space is often called as task-oriented space or operational space.•In the case that joint is not actuated directly by actuator, and the position sensors are often located at the actuators, actuator position should be considered.•Actuator vector: a set of actuator position•Actuator space: the space of actuator vectorsActuator SpaceJoint Space Cartesian Space•Base frame {B}, or frame {0}. It is attached to a non-moving part of the robot, i.e., the base, sometimes called link 0link1link2link0link6{B}{W}{T}{S}{G}link1link2link0link6{B}{W }{T }{S}{G}•The station frame {S}, located in a task-relevant location. Sometimes is called universe frame , task frame or world frame . It is often specified with respect to frame {B} as:TS B S =}{link1link2link0link6{B}{W }{T }{S}{G}•The wrist frame {W}, attached to the last link of themanipulator (frame {N}). It is defined relative to the base frame {B} asTT W N B W 0}{==link1link2link0link6{B}{W }{T }{S}{G}•The tool frame {T}, attached to the end of tool that the robot isholding. It can be defined according to wrist frame {W}.TT W T=}{link1link2link0link6{B}{W}{T }{S}{G}•The goal frame is utilized to describe the location to which therobot is to move the tool. It is specified relative to the station frame {S}.TG S G=}{•Where is the tool?The problem is to locate the position and the orientation of the tool that the robot is holding.))(()(1T T T T W TB W BS ST −=}{}{S T →•Example of the assignment of standard frames:Thank You!。
机器人毕业设计参考文献
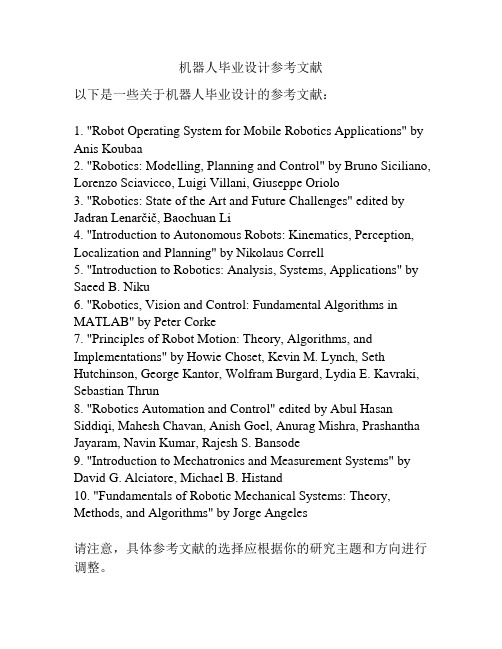
机器人毕业设计参考文献以下是一些关于机器人毕业设计的参考文献:1. "Robot Operating System for Mobile Robotics Applications" by Anis Koubaa2. "Robotics: Modelling, Planning and Control" by Bruno Siciliano, Lorenzo Sciavicco, Luigi Villani, Giuseppe Oriolo3. "Robotics: State of the Art and Future Challenges" edited by Jadran Lenarčič, Baochuan Li4. "Introduction to Autonomous Robots: Kinematics, Perception, Localization and Planning" by Nikolaus Correll5. "Introduction to Robotics: Analysis, Systems, Applications" by Saeed B. Niku6. "Robotics, Vision and Control: Fundamental Algorithms in MATLAB" by Peter Corke7. "Principles of Robot Motion: Theory, Algorithms, and Implementations" by Howie Choset, Kevin M. Lynch, Seth Hutchinson, George Kantor, Wolfram Burgard, Lydia E. Kavraki, Sebastian Thrun8. "Robotics Automation and Control" edited by Abul Hasan Siddiqi, Mahesh Chavan, Anish Goel, Anurag Mishra, Prashantha Jayaram, Navin Kumar, Rajesh S. Bansode9. "Introduction to Mechatronics and Measurement Systems" by David G. Alciatore, Michael B. Histand10. "Fundamentals of Robotic Mechanical Systems: Theory, Methods, and Algorithms" by Jorge Angeles请注意,具体参考文献的选择应根据你的研究主题和方向进行调整。
可越障爬壁机器人研究与设计
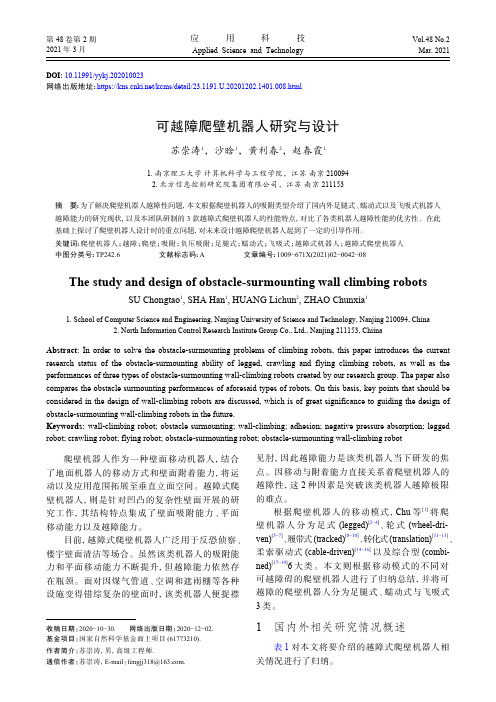
DOI: 10.11991/yykj.202010023网络出版地址:https:///kcms/detail/23.1191.U.20201202.1401.008.html可越障爬壁机器人研究与设计苏崇涛1,沙晗1,黄利春2,赵春霞11. 南京理工大学 计算机科学与工程学院,江苏 南京 2100942. 北方信息控制研究院集团有限公司,江苏 南京 211153摘 要:为了解决爬壁机器人越障性问题,本文根据爬壁机器人的吸附类型介绍了国内外足腿式、蠕动式以及飞吸式机器人越障能力的研究现状,以及本团队研制的3款越障式爬壁机器人的性能特点,对比了各类机器人越障性能的优劣性。
在此基础上探讨了爬壁机器人设计时的重点问题,对未来设计越障爬壁机器人起到了一定的引导作用。
关键词:爬壁机器人;越障;爬壁;吸附;负压吸附;足腿式;蠕动式;飞吸式;越障式机器人;越障式爬壁机器人中图分类号:TP242.6 文献标志码:A 文章编号:1009−671X(2021)02−0042−08The study and design of obstacle-surmounting wall climbing robotsSU Chongtao 1, SHA Han 1, HUANG Lichun 2, ZHAO Chunxia 11. School of Computer Science and Engineering, Nanjing University of Science and Technology, Nanjing 210094, China2. North Information Control Research Institute Group Co., Ltd., Nanjing 211153, ChiinaAbstract : In order to solve the obstacle-surmounting problems of climbing robots, this paper introduces the current research status of the obstacle-surmounting ability of legged, crawling and flying climbing robots, as well as the performances of three types of obstacle-surmounting wall-climbing robots created by our research group. The paper also compares the obstacle surmounting performances of aforesaid types of robots. On this basis, key points that should be considered in the design of wall-climbing robots are discussed, which is of great significance to guiding the design of obstacle-surmounting wall-climbing robots in the future.Keywords: wall-climbing robot; obstacle surmounting; wall-climbing; adhesion; negative pressure absorption; legged robot; crawling robot; flying robot; obstacle-surmounting robot; obstacle-surmounting wall-climbing robot爬壁机器人作为一种壁面移动机器人,结合了地面机器人的移动方式和壁面附着能力,将运动以及应用范围拓展至垂直立面空间。
自动化外文参考文献(精选120个最新)
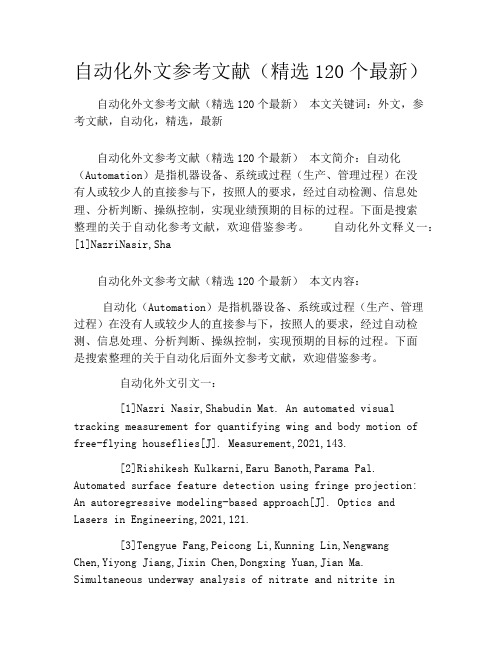
自动化外文参考文献(精选120个最新)自动化外文参考文献(精选120个最新)本文关键词:外文,参考文献,自动化,精选,最新自动化外文参考文献(精选120个最新)本文简介:自动化(Automation)是指机器设备、系统或过程(生产、管理过程)在没有人或较少人的直接参与下,按照人的要求,经过自动检测、信息处理、分析判断、操纵控制,实现业绩预期的目标的过程。
下面是搜索整理的关于自动化参考文献,欢迎借鉴参考。
自动化外文释义一:[1]NazriNasir,Sha自动化外文参考文献(精选120个最新)本文内容:自动化(Automation)是指机器设备、系统或过程(生产、管理过程)在没有人或较少人的直接参与下,按照人的要求,经过自动检测、信息处理、分析判断、操纵控制,实现预期的目标的过程。
下面是搜索整理的关于自动化后面外文参考文献,欢迎借鉴参考。
自动化外文引文一:[1]Nazri Nasir,Shabudin Mat. An automated visual tracking measurement for quantifying wing and body motion of free-flying houseflies[J]. Measurement,2021,143.[2]Rishikesh Kulkarni,Earu Banoth,Parama Pal. Automated surface feature detection using fringe projection: An autoregressive modeling-based approach[J]. Optics and Lasers in Engineering,2021,121.[3]Tengyue Fang,Peicong Li,Kunning Lin,NengwangChen,Yiyong Jiang,Jixin Chen,Dongxing Yuan,Jian Ma. Simultaneous underway analysis of nitrate and nitrite inestuarine and coastal waters using an automated integrated syringe-pump-based environmental-water analyzer[J]. Analytica Chimica Acta,2021,1076.[4]Shengfeng Chen,Jian Liu,Xiaosong Zhang,XinyuSuo,Enhui Lu,Jilong Guo,Jianxun Xi. Development ofpositioning system for Nuclear-fuel rod automated assembly[J]. Robotics and Computer Integrated Manufacturing,2021,61.[5]Cheng-Ta Lee,Yu-Ching Lee,Albert Y. Chen. In-building automated external defibrillator location planning and assessment through building information models[J]. Automation in Construction,2021,106.[6]Torgeir Aleti,Jason I. Pallant,Annamaria Tuan,Tom van Laer. Tweeting with the Stars: Automated Text Analysis of the Effect of Celebrity Social Media ications on ConsumerWord of Mouth[J]. Journal of Interactive Marketing,2021,48.[7]Daniel Bacioiu,Geoff Melton,MayorkinosPapaelias,Rob Shaw. Automated defect classification of SS304 TIG welding process using visible spectrum camera and machine learning[J]. NDT and E International,2021,107.[8]Marcus von der Au,Max Schwinn,KatharinaKuhlmeier,Claudia Büchel,Bj?rn Meermann. Development of an automated on-line purification HPLC single cell-ICP-MS approach for fast diatom analysis[J]. Analytica ChimicaActa,2021,1077.[9]Jitendra Mehar,Ajam Shekh,Nethravathy M. U.,R. Sarada,Vikas Singh Chauhan,Sandeep Mudliar. Automation ofpilot-scale open raceway pond: A case study of CO 2 -fed pHcontrol on Spirulina biomass, protein and phycocyanin production[J]. Journal of CO2 Utilization,2021,33.[10]John T. Sloop,Henry J.B. Bonilla,TinaHarville,Bradley T. Jones,George L. Donati. Automated matrix-matching calibration using standard dilution analysis withtwo internal standards and a simple three-port mixing chamber[J]. Talanta,2021,205.[11]Daniel J. Spade,Cathy Yue Bai,ChristyLambright,Justin M. Conley,Kim Boekelheide,L. Earl Gray. Corrigendum to “Validation of an automated counting procedure for phthalate-induced testicular multinucleated germ cells” [Toxicol. Lett. 290 (2021) 55–61][J]. Toxicology Letters,2021,313.[12]Christian P. Janssen,Shamsi T. Iqbal,Andrew L. Kun,Stella F. Donker. Interrupted by my car? Implications of interruption and interleaving research for automatedvehicles[J]. International Journal of Human - Computer Studies,2021,130.[13]Seunguk Lee,Si Kuan Thio,Sung-Yong Park,Sungwoo Bae. An automated 3D-printed smartphone platform integrated with optoelectrowetting (OEW) microfluidic chip for on-site monitoring of viable algae in water[J]. Harmful Algae,2021,88.[14]Yuxia Duan,Shicai Liu,Caiqi Hu,Junqi Hu,Hai Zhang,Yiqian Yan,Ning Tao,Cunlin Zhang,Xavier Maldague,Qiang Fang,Clemente Ibarra-Castanedo,Dapeng Chen,Xiaoli Li,Jianqiao Meng. Automated defect classification in infrared thermography based on a neural network[J]. NDT and E International,2021,107.[15]Alex M. Pagnozzi,Jurgen Fripp,Stephen E. Rose. Quantifying deep grey matter atrophy using automated segmentation approaches: A systematic review of structural MRI studies[J]. NeuroImage,2021,201.[16]Jin Ye,Zhihong Xuan,Bing Zhang,Yu Wu,LiLi,Songshan Wang,Gang Xie,Songxue Wang. Automated analysis of ochratoxin A in cereals and oil by iaffinity magnetic beads coupled to UPLC-FLD[J]. Food Control,2021,104.[17]Anne Bech Risum,Rasmus Bro. Using deep learning to evaluate peaks in chromatographic data[J].Talanta,2021,204.[18]Faris Elghaish,Sepehr Abrishami,M. Reza Hosseini,Soliman Abu-Samra,Mark Gaterell. Integrated project delivery with BIM: An automated EVM-based approach[J]. Automation in Construction,2021,106.[19]Carl J. Pearson,Michael Geden,Christopher B. Mayhorn. Who's the real expert here? Pedigree's unique bias on trust between human and automated advisers[J]. Applied Ergonomics,2021,81.[20]Vibhas Mishra,Dani?l M.J. Peeters,Mostafa M. Abdalla. Stiffness and buckling analysis of variablestiffness laminates including the effect of automated fibre placement defects[J]. Composite Structures,2021,226.[21]Jenny S. Wesche,Andreas Sonderegger. When computers take the lead: The automation of leadership[J]. Computers in Human Behavior,2021,101.[22]Murat Ayaz,Hüseyin Yüksel. Design of a new cost-efficient automation system for gas leak detection in industrial buildings[J]. Energy &amp; Buildings,2021,200.[23]Stefan A. Mann,Juliane Heide,Thomas Knott,Razvan Airini,Florin Bogdan Epureanu,Alexandru-FlorianDeftu,Antonia-Teona Deftu,Beatrice Mihaela Radu,Bogdan Amuzescu. Recording of multiple ion current components and action potentials in human induced pluripotent stem cell-derived cardiomyocytes via automated patch-clamp[J]. Journal of Pharmacological and Toxicological Methods,2021,100.[24]Rhar? de Almeida Cardoso,Alexandre Cury,Flavio Barbosa. Automated real-time damage detection strategy using raw dynamic measurements[J]. Engineering Structures,2021,196.[25]Mengmeng Zhong,Tielong Wang,Chengdu Qi,Guilong Peng,Meiling Lu,Jun Huang,Lee Blaney,Gang Yu. Automated online solid-phase extraction liquid chromatography tandem mass spectrometry investigation for simultaneous quantification of per- and polyfluoroalkyl substances, pharmaceuticals and personal care products, and organophosphorus flame retardants in environmental waters[J]. Journal of Chromatography A,2021,1602.[26]Pau Climent-Pér ez,Susanna Spinsante,Alex Mihailidis,Francisco Florez-Revuelta. A review on video-based active and assisted living technologies for automated lifelogging[J]. Expert Systems With Applications,2021,139.[27]William Snyder,Marisa Patti,Vanessa Troiani. An evaluation of automated tracing for orbitofrontal cortexsulcogyral pattern typing[J]. Journal of Neuroscience Methods,2021,326.[28]Juan Manuel Davila Delgado,LukumonOyedele,Anuoluwapo Ajayi,Lukman Akanbi,OlugbengaAkinade,Muhammad Bilal,Hakeem Owolabi. Robotics and automated systems in construction: Understanding industry-specific challenges for adoption[J]. Journal of Building Engineering,2021,26.[29]Mohamed Taher Alrefaie,Stever Summerskill,Thomas W Jackon. In a heart beat: Using driver’s physiological changes to determine the quality of a takeover in highly automated vehicles[J]. Accident Analysis andPrevention,2021,131.[30]Tawseef Ayoub Shaikh,Rashid Ali. Automated atrophy assessment for Alzheimer's disease diagnosis from brain MRI images[J]. Magnetic Resonance Imaging,2021,62.自动化外文参考文献二:[31]Vaanathi Sundaresan,Giovanna Zamboni,Campbell Le Heron,Peter M. Rothwell,Masud Husain,Marco Battaglini,Nicola De Stefano,Mark Jenkinson,Ludovica Griffanti. Automatedlesion segmentation with BIANCA: Impact of population-level features, classification algorithm and locally adaptive thresholding[J]. NeuroImage,2021,202.[32]Ho-Jun Suk,Edward S. Boyden,Ingrid van Welie. Advances in the automation of whole-cell patch clamp technology[J]. Journal of Neuroscience Methods,2021,326.[33]Ivana Duznovic,Mathias Diefenbach,Mubarak Ali,Tom Stein,Markus Biesalski,Wolfgang Ensinger. Automated measuring of mass transport through synthetic nanochannels functionalized with polyelectrolyte porous networks[J]. Journal of Membrane Science,2021,591.[34]James A.D. Cameron,Patrick Savoie,Mary E.Kaye,Erik J. Scheme. Design considerations for the processing system of a CNN-based automated surveillance system[J]. Expert Systems With Applications,2021,136.[35]Ebrahim Azadniya,Gertrud E. Morlock. Automated piezoelectric spraying of biological and enzymatic assays for effect-directed analysis of planar chromatograms[J]. Journal of Chromatography A,2021,1602.[36]Lilla Z?llei,Camilo Jaimes,Elie Saliba,P. Ellen Grant,Anastasia Yendiki. TRActs constrained by UnderLying INfant anatomy (TRACULInA): An automated probabilistic tractography tool with anatomical priors for use in the newborn brain[J]. NeuroImage,2021,199.[37]Kate?ina Fikarová,David J. Cocovi-Solberg,María Rosende,Burkhard Horstkotte,Hana Sklená?ová,Manuel Miró. A flow-based platform hyphenated to on-line liquid chromatography for automatic leaching tests of chemical additives from microplastics into seawater[J]. Journal of Chromatography A,2021,1602.[38]Darko ?tern,Christian Payer,Martin Urschler. Automated age estimation from MRI volumes of the hand[J]. Medical Image Analysis,2021,58.[39]Jacques Blum,Holger Heumann,Eric Nardon,Xiao Song. Automating the design of tokamak experiment scenarios[J]. Journal of Computational Physics,2021,394.[40]Elton F. de S. Soares,Carlos Alberto V.Campos,Sidney C. de Lucena. Online travel mode detection method using automated machine learning and feature engineering[J]. Future Generation Computer Systems,2021,101.[41]M. Marouli,S. Pommé. Autom ated optical distance measurements for counting at a defined solid angle[J].Applied Radiation and Isotopes,2021,153.[42]Yi Dai,Zhen-Hua Yu,Jian-Bo Zhan,Bao-Shan Yue,Jiao Xie,Hao Wang,Xin-Sheng Chai. Determination of starch gelatinization temperatures by an automated headspace gas chromatography[J]. Journal of Chromatography A,2021,1602.[43]Marius Tarp?,Tobias Friis,Peter Olsen,MartinJuul,Christos Georgakis,Rune Brincker. Automated reduction of statistical errors in the estimated correlation functionmatrix for operational modal analysis[J]. Mechanical Systems and Signal Processing,2021,132.[44]Wenxia Dai,Bisheng Yang,Xinlian Liang,ZhenDong,Ronggang Huang,Yunsheng Wang,Wuyan Li. Automated fusionof forest airborne and terrestrial point clouds throughcanopy density analysis[J]. ISPRS Journal of Photogrammetry and Remote Sensing,2021,156.[45]Jyh-Haur Woo,Marcus Ang,Hla Myint Htoon,Donald Tan. Descemet Membrane Endothelial Keratoplasty Versus Descemet Stripping Automated Endothelial Keratoplasty andPenetrating Keratoplasty[J]. American Journal of Ophthalmology,2021,207.[46]F. Wilde,S. Marsen,T. Stange,D. Moseev,J.W. Oosterbeek,H.P. Laqua,R.C. Wolf,K. Avramidis,G.Gantenbein,I.Gr. Pagonakis,S. Illy,J. Jelonnek,M.K. Thumm,W7-X team. Automated mode recovery for gyrotrons demonstrated at Wendelstein 7-X[J]. Fusion Engineering and Design,2021,148.[47]Andrew Kozbial,Lekhana Bhandary,Shashi K. Murthy. Effect of yte seeding density on dendritic cell generation in an automated perfusion-based culture system[J]. Biochemical Engineering Journal,2021,150.[48]Wen-Hao Su,Steven A. Fennimore,David C. Slaughter. Fluorescence imaging for rapid monitoring of translocation behaviour of systemic markers in snap beans for automatedcrop/weed discrimination[J]. Biosystems Engineering,2021,186.[49]Ki-Taek Lim,Dinesh K. Patel,Hoon Se,JanghoKim,Jong Hoon Chung. A fully automated bioreactor system for precise control of stem cell proliferation anddifferentiation[J]. Biochemical Engineering Journal,2021,150.[50]Mitchell L. Cunningham,Michael A. Regan,Timothy Horberry,Kamal Weeratunga,Vinayak Dixit. Public opinion about automated vehicles in Australia: Results from a large-scale national survey[J]. Transportation Research Part A,2021,129.[51]Yi Xie,Qiaobei You,Pingyang Dai,Shuyi Wang,Peiyi Hong,Guokun Liu,Jun Yu,Xilong Sun,Yongming Zeng. How to achieve auto-identification in Raman analysis by spectral feature extraction &amp; Adaptive Hypergraph[J].Spectrochimica Acta Part A: Molecular and Biomolecular Spectroscopy,2021,222.[52]Ozal Yildirim,Muhammed Talo,Betul Ay,Ulas Baran Baloglu,Galip Aydin,U. Rajendra Acharya. Automated detection of diabetic subject using pre-trained 2D-CNN models with frequency spectrum images extracted from heart ratesignals[J]. Computers in Biology and Medicine,2021,113.[53]Marius Kern,Laura Tusa,Thomas Lei?ner,Karl Gerald van den Boogaart,Jens Gutzmer. Optimal sensor selection for sensor-based sorting based on automated mineralogy data[J]. Journal of Cleaner Production,2021,234.[54]Karim Keddadouche,Régis Braucher,Didier L.Bourlès,Mélanie Baroni,Valéry Guillou,La?titia Léanni,Georges Auma?tre. Design and performance of an automated chemical extraction bench for the preparation of 10 Be and 26 Al targets to be analyzed by accelerator mass spectrometry[J]. Nuclear Inst. and Methods in Physics Research, B,2021,456.[55]Christian P. Janssen,Stella F. Donker,Duncan P. Brumby,Andrew L. Kun. History and future of human-automation interaction[J]. International Journal of Human - Computer Studies,2021,131.[56]Victoriya Orlovskaya,Olga Fedorova,Michail Nadporojskii,Raisa Krasikova. A fully automated azeotropic drying free synthesis of O -(2-[ 18 F]fluoroethyl)- l -tyrosine ([ 18 F]FET) using tetrabutylammonium tosylate[J]. Applied Radiation and Isotopes,2021,152.[57]Dinesh Krishnamoorthy,Kjetil Fjalestad,Sigurd Skogestad. Optimal operation of oil and gas production usingsimple feedback control structures[J]. Control Engineering Practice,2021,91.[58]Nick Oliver,Thomas Calvard,Kristina Poto?nik. Safe limits, mindful organizing and loss of control in commercial aviation[J]. Safety Science,2021,120.[59]Bo Sui,Nils Lubbe,Jonas B?rgman. A clustering approach to developing car-to-two-wheeler test scenarios for the assessment of Automated Emergency Braking in China using in-depth Chinese crash data[J]. Accident Analysis and Prevention,2021,132.[60]Ji-Seok Yoon,Eun Young Choi,Maliazurina Saad,Tae-Sun Choi. Automated integrated system for stained neuron detection: An end-to-end framework with a high negative predictive rate[J]. Computer Methods and Programs in Biomedicine,2021,180.自动化外文参考文献八:[61]Min Wang,Barbara E. Glick-Wilson,Qi-Huang Zheng. Facile fully automated radiosynthesis and quality control of O -(2-[ 18 F]fluoroethyl)- l -tyrosine ([ 18 F]FET) for human brain tumor imaging[J]. Applied Radiation andIsotopes,2021,154.[62]Fabian Pütz,Finbarr Murphy,Martin Mullins,LisaO'Malley. Connected automated vehicles and insurance: Analysing future market-structure from a business ecosystem perspective[J]. Technology in Society,2021,59.[63]Victoria A. Banks,Neville A. Stanton,Katherine L. Plant. Who is responsible for automated driving? A macro-level insight into automated driving in the United Kingdom using the Risk Management Framework and Social NetworkAnalysis[J]. Applied Ergonomics,2021,81.[64]Yingjun Ye,Xiaohui Zhang,Jian Sun. Automated vehicle’s behavior decision making using deep reinforcement learning and high-fidelity simulation environment[J]. Transportation Research Part C,2021,107.[65]Hasan Alkaf,Jameleddine Hassine,TahaBinalialhag,Daniel Amyot. An automated change impact analysis approach for User Requirements Notation models[J]. TheJournal of Systems &amp; Software,2021,157.[66]Zonghua Luo,Jiwei Gu,Robert C. Dennett,Gregory G. Gaehle,Joel S. Perlmutter,Delphine L. Chen,Tammie L.S. Benzinger,Zhude Tu. Automated production of a sphingosine-1 phosphate receptor 1 (S1P1) PET radiopharmaceutical [ 11C]CS1P1 for human use[J]. Applied Radiation andIsotopes,2021,152.[67]Sarfraz Qureshi,Wu Jiacheng,Jeroen Anton van Kan. Automated alignment and focusing system for nuclear microprobes[J]. Nuclear Inst. and Methods in Physics Research, B,2021,456.[68]Srikanth Sagar Bangaru,Chao Wang,MarwaHassan,Hyun Woo Jeon,Tarun Ayiluri. Estimation of the degreeof hydration of concrete through automated machine learning based microstructure analysis – A study on effect of image magnification[J]. Advanced Engineering Informatics,2021,42.[69]Fang Tengyue,Li Peicong,Lin Kunning,Chen Nengwang,Jiang Yiyong,Chen Jixin,Yuan Dongxing,Ma Jian. Simultaneous underway analysis of nitrate and nitrite in estuarine and coastal waters using an automated integrated syringe-pump-based environmental-water analyzer.[J]. Analytica chimica acta,2021,1076.[70]Ramos Inês I,Carl Peter,Schneider RudolfJ,Segundo Marcela A. Automated lab-on-valve sequential injection ELISA for determination of carbamazepine.[J]. Analytica chimica acta,2021,1076.[71]Au Marcus von der,Schwinn Max,Kuhlmeier Katharina,Büchel Claudia,Meermann Bj?rn. Development of an automated on-line purification HPLC single cell-ICP-MS approach for fast diatom analysis.[J]. Analytica chimica acta,2021,1077.[72]Risum Anne Bech,Bro Rasmus. Using deep learning to evaluate peaks in chromatographic data.[J].Talanta,2021,204.[73]Spade Daniel J,Bai Cathy Yue,LambrightChristy,Conley Justin M,Boekelheide Kim,Gray L Earl. Corrigendum to "Validation of an automated counting procedure for phthalate-induced testicular multinucleated germ cells" [Toxicol. Lett. 290 (2021) 55-61].[J]. Toxicologyletters,2021,313.[74]Zhong Mengmeng,Wang Tielong,Qi Chengdu,Peng Guilong,Lu Meiling,Huang Jun,Blaney Lee,Yu Gang. Automated online solid-phase extraction liquid chromatography tandem mass spectrometry investigation for simultaneousquantification of per- and polyfluoroalkyl substances, pharmaceuticals and personal care products, and organophosphorus flame retardants in environmental waters.[J]. Journal of chromatography. A,2021,1602.[75]Stein Christopher J,Reiher Markus. autoCAS: A Program for Fully Automated MulticonfigurationalCalculations.[J]. Journal of computationalchemistry,2021,40(25).[76]Alrefaie Mohamed Taher,Summerskill Stever,Jackon Thomas W. In a heart beat: Using driver's physiological changes to determine the quality of a takeover in highly automated vehicles.[J]. Accident; analysis andprevention,2021,131.[77]Shaikh Tawseef Ayoub,Ali Rashid. Automatedatrophy assessment for Alzheimer's disease diagnosis frombrain MRI images.[J]. Magnetic resonance imaging,2021,62.[78]Xie Yi,You Qiaobei,Dai Pingyang,Wang Shuyi,Hong Peiyi,Liu Guokun,Yu Jun,Sun Xilong,Zeng Yongming. How to achieve auto-identification in Raman analysis by spectral feature extraction &amp; Adaptive Hypergraph.[J]. Spectrochimica acta. Part A, Molecular and biomolecular spectroscopy,2021,222.[79]Azadniya Ebrahim,Morlock Gertrud E. Automated piezoelectric spraying of biological and enzymatic assays for effect-directed analysis of planar chromatograms.[J]. Journal of chromatography. A,2021,1602.[80]Fikarová Kate?ina,Cocovi-Solberg David J,Rosende María,Horstkotte Burkhard,Sklená?ová Hana,Miró Manuel. Aflow-based platform hyphenated to on-line liquid chromatography for automatic leaching tests of chemical additives from microplastics into seawater.[J]. Journal of chromatography. A,2021,1602.[81]Moitra Dipanjan,Mandal Rakesh Kr. Automated AJCC (7th edition) staging of non-small cell lung cancer (NSCLC) using deep convolutional neural network (CNN) and recurrent neural network (RNN).[J]. Health information science and systems,2021,7(1).[82]Ramos-Payán María. Liquid - Phase microextraction and electromembrane extraction in millifluidic devices:A tutorial.[J]. Analytica chimica acta,2021,1080.[83]Z?llei Lilla,Jaimes Camilo,Saliba Elie,Grant P Ellen,Yendiki Anastasia. TRActs constrained by UnderLying INfant anatomy (TRACULInA): An automated probabilistic tractography tool with anatomical priors for use in the newborn brain.[J]. NeuroImage,2021,199.[84]Sedghi Gamechi Zahra,Bons Lidia R,Giordano Marco,Bos Daniel,Budde Ricardo P J,Kofoed Klaus F,Pedersen Jesper Holst,Roos-Hesselink Jolien W,de Bruijne Marleen. Automated 3D segmentation and diameter measurement of the thoracic aorta on non-contrast enhanced CT.[J]. European radiology,2021,29(9).[85]Smith Claire,Galland Barbara C,de Bruin Willemijn E,Taylor Rachael W. Feasibility of Automated Cameras to Measure Screen Use in Adolescents.[J]. American journal of preventive medicine,2021,57(3).[86]Lambert Marie-?ve,Arsenault Julie,AudetPascal,Delisle Benjamin,D'Allaire Sylvie. Evaluating an automated clustering approach in a perspective of ongoing surveillance of porcine reproductive and respiratory syndrome virus (PRRSV) field strains.[J]. Infection, genetics and evolution : journal of molecular epidemiology and evolutionary genetics in infectious diseases,2021,73.[87]Slanetz Priscilla J. Does Computer-aided Detection Help in Interpretation of Automated Breast US?[J]. Radiology,2021,292(3).[88]Sander Laura,Pezold Simon,Andermatt Simon,Amann Michael,Meier Dominik,Wendebourg Maria J,Sinnecker Tim,Radue Ernst-Wilhelm,Naegelin Yvonne,Granziera Cristina,Kappos Ludwig,Wuerfel Jens,Cattin Philippe,Schlaeger Regina. Accurate, rapid and reliable, fully automated MRI brainstem segmentation for application in multiple sclerosis and neurodegenerative diseases.[J]. Human brainmapping,2021,40(14).[89]Pajkossy Péter,Sz?ll?si ?gnes,Racsmány Mihály. Retrieval practice decreases processing load of recall: Evidence revealed by pupillometry.[J]. International journal of psychophysiology : official journal of the International Organization of Psychophysiology,2021,143.[90]Kaiser Eric A,Igdalova Aleksandra,Aguirre Geoffrey K,Cucchiara Brett. A web-based, branching logic questionnaire for the automated classification ofmigraine.[J]. Cephalalgia : an international journal of headache,2021,39(10).自动化外文参考文献四:[91]Kim Jin Ju,Park Younhee,Choi Dasom,Kim Hyon Suk. Performance Evaluation of a New Automated Chemiluminescent Ianalyzer-Based Interferon-Gamma Releasing Assay AdvanSure I3 in Comparison With the QuantiFERON-TB Gold In-Tube Assay.[J]. Annals of laboratory medicine,2021,40(1).[92]Yang Shanling,Gao Xican,Liu Liwen,Shu Rui,Yan Jingru,Zhang Ge,Xiao Yao,Ju Yan,Zhao Ni,Song Hongping. Performance and Reading Time of Automated Breast US with or without Computer-aided Detection.[J]. Radiology,2021,292(3).[93]Hung Andrew J,Chen Jian,Ghodoussipour Saum,OhPaul J,Liu Zequn,Nguyen Jessica,Purushotham Sanjay,Gill Inderbir S,Liu Yan. A deep-learning model using automated performance metrics and clinical features to predict urinary continence recovery after robot-assisted radical prostatectomy.[J]. BJU international,2021,124(3).[94]Kim Ryan S,Kim Gene. Double Descemet Stripping Automated Endothelial Keratoplasty (DSAEK): Secondary DSAEK Without Removal of the Failed Primary DSAEK Graft.[J]. Ophthalmology,2021,126(9).[95]Sargent Alexandra,Theofanous Ioannis,Ferris Sarah. Improving laboratory workflow through automated pre-processing of SurePath specimens for human papillomavirus testing with the Abbott RealTime assay.[J]. Cytopathology : official journal of the British Society for Clinical Cytology,2021,30(5).[96]Saba Tanzila. Automated lung nodule detection and classification based on multiple classifiers voting.[J]. Microscopy research and technique,2021,82(9).[97]Ivan D. Welsh,Jane R. Allison. Automated simultaneous assignment of bond orders and formal charges[J]. Journal of Cheminformatics,2021,11(1).[98]Willem Jespers,MauricioEsguerra,Johan ?qvist,Hugo Gutiérrez-de-Terán. QligFEP: an automated workflow for small molecule free energycalculations in Q[J]. Journal of Cheminformatics,2021,11(1).[99]Manav Raj,Robert Seamans. Primer on artificial intelligence and robotics[J]. Journal of OrganizationDesign,2021,8(1).[100]Yvette Pronk,Peter Pilot,Justus M.Brinkman,Ronald J. Heerwaarden,Walter Weegen. Response rate and costs for automated patient-reported outcomes collection alone compared to combined automated and manual collection[J]. Journal of Patient-Reported Outcomes,2021,3(1).[101]Tristan Martin,Ana?s Moyon,Cyril Fersing,Evan Terrier,Aude Gouillet,Fabienne Giraud,BenjaminGuillet,Philippe Garrigue. Have you looked for “stranger things” in your automated PET dose dispensing system? A process and operators qualification scheme[J]. EJNMMI Radiopharmacy and Chemistry,2021,4(1).[102]Manuel Peuster,Michael Marchetti,Ger ardo García de Blas,Holger Karl. Automated testing of NFV orchestrators against carrier-grade multi-PoP scenarios using emulation-based smoke testing[J]. EURASIP Journal on Wireless ications and Networking,2021,2021(1).[103]R. Ferrús,O. Sallent,J. Pérez-Romero,R. Agustí. On the automation of RAN slicing provisioning: solution framework and applicability examples[J]. EURASIP Journal on Wireless ications and Networking,2021,2021(1).[104]Duo Li,Peter Wagner. Impacts of gradual automated vehicle penetration on motorway operation: a comprehensive evaluation[J]. European Transport Research Review,2021,11(1).[105]Abel Gómez,Ricardo J. Rodríguez,María-Emilia Cambronero,Valentín Valero. Profiling the publish/subscribe paradigm for automated analysis using colored Petri nets[J]. Software &amp; Systems Modeling,2021,18(5).[106]Dipanjan Moitra,Rakesh Kr. Mandal. Automated AJCC (7th edition) staging of non-small cell lung cancer (NSCLC) using deep convolutional neural network (CNN) and recurrent neural network (RNN)[J]. Health Information Science and Systems,2021,7(1).[107]Marta D’Alonzo,Laura Martincich,Agnese Fenoglio,Valentina Giannini,Lisa Cellini,ViolaLiberale,Nicoletta Biglia. Nipple-sparing mastectomy: external validation of a three-dimensional automated method to predict nipple occult tumour involvement on preoperative breast MRI[J]. European Radiology Experimental,2021,3(1).[108]N. V. Dozmorov,A. S. Bogomolov,A. V. Baklanov. An Automated Apparatus for Measuring Spectral Dependences ofthe Mass Spectra and Velocity Map Images of Photofragments[J]. Instruments and Experimental Techniques,2021,62(4).[109]Zhiqiang Sun,Bingzhao Gao,Jiaqi Jin,Kazushi Sanada. Modelling, Analysis and Simulation of a Novel Automated Manual Transmission with Gearshift Assistant Mechanism[J]. International Journal of Automotive Technology,2021,20(5).[110]Andrés Vega,Mariano Córdoba,Mauricio Castro-Franco,Mónica Balzarini. Protocol for automating errorremoval from yield maps[J]. Precision Agriculture,2021,20(5).[111]Bethany L. Lussier,DaiWai M. Olson,Venkatesh Aiyagari. Automated Pupillometry in Neurocritical Care: Research and Practice[J]. Current Neurology and Neuroscience Reports,2021,19(10).[112] B. Haskali,Peter D. Roselt,David Binns,Amit Hetsron,Stan Poniger,Craig A. Hutton,Rodney J. Hicks. Automated preparation of clinical grade [ 68 Ga]Ga-DOTA-CP04, a cholecystokinin-2 receptor agonist, using iPHASE MultiSyn synthesis platform[J]. EJNMMI Radiopharmacy andChemistry,2021,4(1).[113]Ju Hyun Ahn,Minho Na,Sungkwan Koo,HyunsooChun,Inhwan Kim,Jong Won Hur,Jae Hyuk Lee,Jong G. Ok. Development of a fully automated desktop chemical vapor deposition system for programmable and controlled carbon nanotube growth[J]. Micro and Nano Systems Letters,2021,7(1).[114]Kamellia Shahi,Brenda Y. McCabe,Arash Shahi. Framework for Automated Model-Based e-Permitting System forMunicipal Jurisdictions[J]. Journal of Management in Engineering,2021,35(6).[115]Ahmed Khalafallah,Yasmin Shalaby. Change Orders: Automating Comparative Data Analysis and Controlling Impacts in Public Projects[J]. Journal of Construction Engineering and Management,2021,145(11).[116]José ?. Martínez-Huertas,OlgaJastrzebska,Ricardo Olmos,José A. León. Automated summary evaluation with inbuilt rubric method: An alternative to constructed responses and multiple-choice testsassessments[J]. Assessment &amp; Evaluation in Higher Education,2021,44(7).[117]Samsonov,Koshel,Walther,Jenny. Automated placement of supplementary contour lines[J]. International Journal of Geographical Information Science,2021,33(10).[118]Veronika V. Odintsova,Peter J. Roetman,Hill F. Ip,René Pool,Camiel M. Van der Laan,Klodiana-DaphneTona,Robert R.J.M. Vermeiren,Dorret I. Boomsma. Genomics of human aggression: current state of genome-wide studies and an automated systematic review tool[J]. PsychiatricGenetics,2021,29(5).[119]Sebastian Eggert,Dietmar W Hutmacher. In vitro disease models 4.0 via automation and high-throughput processing[J]. Biofabrication,2021,11(4).[120]Asad Mahmood,Faizan Ahmad,Zubair Shafiq,Padmini Srinivasan,Fareed Zaffar. A Girl Has No Name: Automated Authorship Obfuscation using Mutant-X[J]. Proceedings on Privacy Enhancing Technologies,2021,2021(4).。
英语演讲练习-机器人发展史机械工程

The history of robots
The second stage - Technology development (1954-1978) 1962-1963 Application of sensors to improve the operability of the robot. 1965 Johns Hopkins University Applied Physics Laboratory developed the Beast robot 1969 Japan’s Wsaseda University developed the first robot to walk with its feet. 1978 Unimation company developed industrial robot PUMA which means the industrial robot technology has fully matured.
Classification of robots
Industrial robot - the carrier robot hold Lamborghini in the production line.
Classification of robots
military robot : military robot help the army to avoid death in battle and beat the enemy efficiently.
A
B
The purpose of creating robots
Thousands of years we human are burdened by heavy labor. And today there is a trend for us to solve the problem step by step since the robot was invented.
一种基于3-UU并联机构的腕关节康复机器人研制
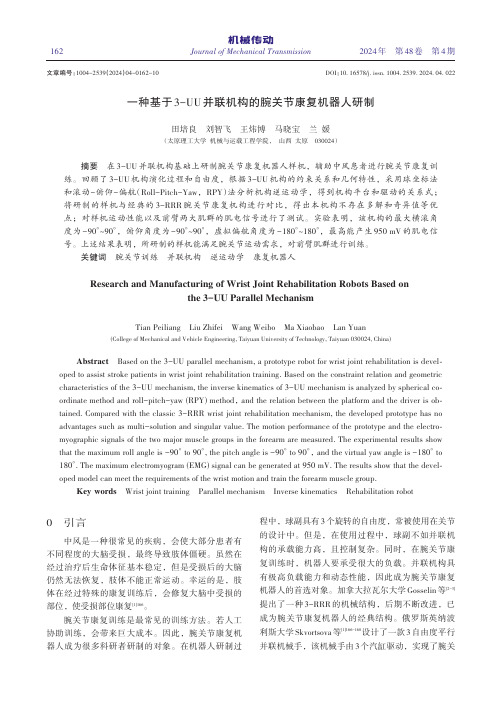
2024年第48卷第4期Journal of Mechanical Transmission一种基于3-UU并联机构的腕关节康复机器人研制田培良刘智飞王炜博马晓宝兰媛(太原理工大学机械与运载工程学院,山西太原030024)摘要在3-UU并联机构基础上研制腕关节康复机器人样机,辅助中风患者进行腕关节康复训练。
回顾了3-UU机构演化过程和自由度,根据3-UU机构的约束关系和几何特性,采用球坐标法和滚动-俯仰-偏航(Roll-Pitch-Yaw,RPY)法分析机构逆运动学,得到机构平台和驱动的关系式;将研制的样机与经典的3-RRR腕关节康复机构进行对比,得出本机构不存在多解和奇异值等优点;对样机运动性能以及前臂两大肌群的肌电信号进行了测试。
实验表明,该机构的最大横滚角度为-90°~90°,俯仰角度为-90°~90°,虚拟偏航角度为-180°~180°,最高能产生950 mV的肌电信号。
上述结果表明,所研制的样机能满足腕关节运动需求,对前臂肌群进行训练。
关键词腕关节训练并联机构逆运动学康复机器人Research and Manufacturing of Wrist Joint Rehabilitation Robots Based onthe 3-UU Parallel MechanismTian Peiliang Liu Zhifei Wang Weibo Ma Xiaobao Lan Yuan(College of Mechanical and Vehicle Engineering, Taiyuan University of Technology, Taiyuan 030024, China)Abstract Based on the 3-UU parallel mechanism, a prototype robot for wrist joint rehabilitation is devel⁃oped to assist stroke patients in wrist joint rehabilitation training. Based on the constraint relation and geometric characteristics of the 3-UU mechanism, the inverse kinematics of 3-UU mechanism is analyzed by spherical co⁃ordinate method and roll-pitch-yaw (RPY) method, and the relation between the platform and the driver is ob⁃tained. Compared with the classic 3-RRR wrist joint rehabilitation mechanism, the developed prototype has no advantages such as multi-solution and singular value. The motion performance of the prototype and the electro⁃myographic signals of the two major muscle groups in the forearm are measured. The experimental results show that the maximum roll angle is -90° to 90°, the pitch angle is -90° to 90°, and the virtual yaw angle is -180° to 180°. The maximum electromyogram (EMG) signal can be generated at 950 mV. The results show that the devel⁃oped model can meet the requirements of the wrist motion and train the forearm muscle group.Key words Wrist joint training Parallel mechanism Inverse kinematics Rehabilitation robot0 引言中风是一种很常见的疾病,会使大部分患者有不同程度的大脑受损,最终导致肢体僵硬。
基于逆强化学习与行为树的机械臂复杂操作技能学习方法

现代电子技术Modern Electronics Technique2024年4月1日第47卷第7期Apr. 2024Vol. 47 No. 70 引 言社会老龄化的加剧与各种意外事故的发生,导致老残人群数量日益增加,不同程度的肢体残障对老残人群的日常生活产生了较大影响。
为在一定程度上解决上述问题,除了提供社会支持和无障碍环境外,助老助残基于逆强化学习与行为树的机械臂复杂操作技能学习方法宋越杰, 马陈昊, 孟子晗, 刘元归(南京邮电大学 自动化学院 人工智能学院, 江苏 南京 210023)摘 要: 现有分层强化学习方法不仅在学习过程中存在样本效率低、奖励稀疏以及学习时间过长等问题,而且大多基于仿真环境,导致学习策略在机器人真实操作环境中部署困难。
针对上述问题,提出一种基于逆强化学习与行为树的机械臂复杂操作技能学习方法。
在对复杂操作任务进行分割的基础上,首先,根据专家演示轨迹确定分割后每个子任务的强化学习参数及其对应的专家策略;其次,根据子任务专家策略并使用生成对抗模仿学习算法进行预训练,得到每个子任务的奖励函数;再次,运用每个子任务的奖励函数并基于SAC 算法做进一步训练,获取到每个子任务的最优策略;最后,将子任务最优策略从仿真环境部署到真实环境,并将部署后的每个子任务作为叶节点构建行为树,实现机械臂复杂任务规划。
实验结果表明,所提方法能较好地学习机械臂复杂操作技能,并在性能上优于其他传统强化学习算法。
关键词: 机械臂; 逆强化学习; 行为树; 复杂操作任务; 专家策略; 奖励函数中图分类号: TN99⁃34; TP181 文献标识码: A 文章编号: 1004⁃373X (2024)07⁃0133⁃07Robotic manipulator complex manipulation skill learning methodbased on inverse reinforcement learning and behavior treeSONG Yuejie, MA Chenhao, MENG Zihan, LIU Yuangui(College of Automation & College of Artificial Intelligence, Nanjing University of Posts and Telecommunications, Nanjing 210023, China)Abstract : The existing hierarchical reinforcement learning methods not only face challenges such as low sample efficiency, sparse rewards, and prolonged learning times during the learning process, but also are predominantly based on simulated environ⁃ments, making it difficult to deploy learning strategies in real robotic manipulation environments. In view of this, a robotic ma⁃nipulator complex manipulation skill learning method based on inverse reinforcement learning and behavior tree is proposed. Based on the segmentation of complex manipulation tasks, the reinforcement learning (RL) parameters for each subtask after seg⁃mentation and their corresponding expert policies are determined according to expert demonstration trajectories. According to the expert policies for subtasks, a generative adversarial imitation learning (GAIL) algorithm is employed for pre⁃training, so as to ob⁃tain the reward functions for each subtask. The reward functions for each subtask are used to carry out further training based on the soft actor⁃critic (SAC) algorithm, so as to obtain the optimal policies. Finally, the optimal policies for the subtasks are de⁃ployed in real experiment. Each deployed subtask serves as a leaf node to construct a behavior tree, so as to realize complex task planning for the robotic manipulator. Experimental results demonstrate that the proposed method can effectively learn complex manipulation skills for the robotic arm and outperform the other traditional reinforcement learning algorithms in terms of the per⁃formance.Keywords : robotic manipulator; inverse reinforcement learning; behavior tree; complex operation task; expert policy; rewardfunctionDOI :10.16652/j.issn.1004⁃373x.2024.07.023引用格式:宋越杰,马陈昊,孟子晗,等.基于逆强化学习与行为树的机械臂复杂操作技能学习方法[J].现代电子技术,2024,47(7):133⁃139.收稿日期:2023⁃12⁃10 修回日期:2023⁃12⁃29基金项目:江苏省自然科学基金项目(BK20210599);江苏省博士后科研资助项目(2019K030)133现代电子技术2024年第47卷辅助设备的开发也非常重要。
- 1、下载文档前请自行甄别文档内容的完整性,平台不提供额外的编辑、内容补充、找答案等附加服务。
- 2、"仅部分预览"的文档,不可在线预览部分如存在完整性等问题,可反馈申请退款(可完整预览的文档不适用该条件!)。
- 3、如文档侵犯您的权益,请联系客服反馈,我们会尽快为您处理(人工客服工作时间:9:00-18:30)。
CHAPTER1THREE GENERATIONS OF COEVOLUTIONARY ROBOTICSJORDAN B.POLLACK,HOD LIPSON,PABLO FUNES,andGREGORY HORNBY1.1ROBOECONOMICSThefield of robotics today faces a practical economic problem:flexible machines with minds cost so much more than manual machines and their humans operators. Few would spend$2000on a vacuum cleaner when a manual one is$200,or half a million dollars on a driverless car when a regular car is$20,000,plus$6per hour for its driver.The high costs associated with designing,building,and controlling robots have led to a stasis[1],and robots in industry are only applied to simple and highly repetitive manufacturing tasks.Even though sophisticated teleoperated machines with sensors and actuators have found important applications(exploration of inac-cessible environments,for example),they leave very little decision,if at all,to the on-board software[2].The central issue addressed by our work is a low-cost way to get a higher level of complex physicality under control.We seek more controlled and moving mechan-ical parts,more sensors,more nonlinear interacting degrees of freedom,without entailing both the hugefixed costs of human design and programming,and the vari-able costs in manufacture and operation.We suggest that this can be achieved only when robot design and construction are fully automatic,and the results are inexpen-sive enough to be disposable.Traditionally,robots are designed on a disciplinary basis:mechanical engineers design complex articulated bodies,with state-of-the-art sensors,actuators,and mul-tiple degrees of freedom.These elaborate machines are then thrown over the wall to the control department,where software programmers and control engineers strug-gle to design a suitable controller.Even if an intelligent human can learn to control such a device,it does not follow that automatic autonomous control can be had at any price.Humans drastically underestimate animal brains:looking into nature,weComputational Intelligence:The Experts Speak.Edited by D.B.Fogel and C.J.RobinsonISBN0-471-27454-2#2003IEEE12THREE GENERATIONS OF COEVOLUTIONARY ROBOTICSsee animal brains of very high complexity,brains more complex than the bodies they inhabit,controlling bodies that have been selected by evolution precisely because they were controllable by those brains.In nature,the body and brain of a horse are coupled tightly,the fruit of a long series of small mutual adaptations;like chicken and egg,neither one was designedfirst.There is never a situation in which the hardware has no software,or where a growth or mutation—beyond the adaptive ability of the brain—survives.The key is thus to evolve both the brain and the body, simultaneously and continuously,from a simple controllable mechanism to one of sufficient complexity for a particular specialized task.The focus of our research is how to automate the integrated design of bodies and brains using a coevolutionary learning approach.The key is to evolve both the brain and the body simultaneously from a simple controllable mechanism to one of suffi-cient complexity for a task.Within a decade we see three technologies that are maturing past the threshold to make this possible.One is the increasingfidelity of advanced mechanical design simulation,stimulated by profits from successful soft-ware competition[3].The second is rapid,one-off prototyping and manufacture, which is proceeding from three-dimensional(3D)plastic layering to stronger com-posite and metal(sintering)technology[4].The third is our understanding of coevolutionary machine learning in the design and intelligent control of complex systems[5–7].1.2COEVOLUTIONCoevolution,when successful,dynamically creates a series of learning environ-ments each slightly more complex than the last,and a series of learners that are tuned to adapt in those environments.Sims’work[8]on body–brain coevolution and the more recent Framsticks simulator[9]demonstrated that the neural controllers and simulated bodies could be coevolved.The goal of our research in coevolutionary robotics is to replicate and extend results from virtual simulations such as these to the reality of computer-designed and constructed special-purpose machines that can adapt to real environments.We are working on coevolutionary algorithms to develop control programs that operate realistic physical-device simulators,both commercial-off-the-shelf and our own custom simulators,where wefinish the evolution inside real embodied robots.We are interested ultimately in mechanical structures that have complex physicality of more degrees of freedom than anything that has ever been controlled by human-designed algorithms,with lower engineering costs than cur-rently possible because of minimal human-design involvement in the product.It is not feasible that controllers for complete structures could be evolved(in simulation or otherwise)withoutfirst evolving controllers for simpler constructions. Compared to the traditional form of evolutionary robotics[10–14],which serially downloads controllers into a piece of hardware,it is relatively easy to explore the space of body constructions in simulation.Realistic simulation is also crucial for providing a rich and nonlinear universe.However,while simulation creates the ability to explore the space of constructions far faster than real-world buildingRESEARCH THRUSTS3 and evaluation could,there remains the problem of transfer to real constructions and scaling to the high complexities used for real-world designs.1.3RESEARCH THRUSTSWe describe three major thrusts in achieving fully automated design(FAD)and manufacture of high-part-count autonomous robots.Thefirst is evolution inside simulation,but in simulations more and more realistic,so the results are not simply visually believable,as in Sims’work[8],but also buildable.We investigated trans-ferring evolved high part-count,static structures from simulation to the real world. The second thrust is to evolve automatically buildable dynamic machines that are nearly autonomous in both their design and manufacture.The third thrust,and perhaps hardest,addresses scaling to more complex tasks:handling complex,high part-count structures through modularity.We have preliminary and promising results in each of these areas,which we outline below.1.3.1Buildable SimulationCommercial computer-aided design(CAD)models are in fact not constrained enough to be buildable,because they assume a human provides numerous con-straints to describe reality.In order to evolve both the morphology and behavior of autonomous mechanical devices that can be built,one must have a simulator that operates under many constraints,and a resultant controller that is adaptive enough to cover the gap between the simulated and real world.Features of a simu-lator for evolving morphology are:.Representation:should cover a universal space of mechanisms..Conservative:because simulation is never perfect,it should preserve a margin of safety..Efficient:it should be quicker to test in simulation than through physical production and test..Buildable:results should be convertible from a simulation to a real object.One approach is to custom build a simulator for modular robotic components,and then evolve either centralized or distributed controllers for them.In advance of a modular simulator with dynamics,we recently built a simulator for(static)Lego bricks,and used very simple evolutionary algorithms to create complex Lego struc-tures,which were then constructed manually[15–17].Our model considers the union between two bricks as a rigid joint between the centers of mass of each one,located at the center of the actual area of contact between them.This joint has a measurable torque capacity.That is,more than a certain amount of force applied at a certain distance from the joint will break the two bricks apart.The fun-damental assumption of our model is this idealization of the union of two Legobricks.The genetic algorithm reliably builds structures that meet simple fitness goals,exploiting physical properties implicit in the simulation.Building the results of the evolutionary simulation (by hand)demonstrated the power and possibility of fully automated design.The long bridge of Figure 1.1shows that our simple system discovered the cantilever,while the weight-carrying crane shows it discovered the basic triangular support.1.3.2Evolution and Construction of Electromechanical SystemsThe next step is to add dynamics to modular buildable physical components,and to insert their manufacturing constraints into the evolutionary process.We are experi-menting with a new process in which both robot morphology and control evolve in simulation and then replicate automatically into reality [18].The robots comprise only linear actuators and sigmoidal control neurons embodied in an arbitrary ther-moplastic body.The entire con figuration is evolved for a particular task and selected individuals are printed preassembled (except motors)using 3D solid printing (rapid prototyping)technology,later to be recycled into different forms.In doing so,we establish for the first time a complete physical evolution cycle.In this project,the evolutionary design approach assumes two main principles:(1)to minimize induc-tive bias,we must strive to use the lowest-level building blocks possible,and (2)we coevolve the body and the control,so that they stimulate and constrain each other.We use arbitrary networks of linear actuators and bars for the morphology,and arbi-trary networks of sigmoidal neurons for the control.Evolution is simulated starting with a soup of disconnected elements and continues over hundreds of generations of hundreds of machines,until creatures that are suf ficiently pro ficient at the given task emerge.The simulator used in this research is based on quasi-static motion.The basic principle is that motion is broken down into a series of statically stable frames solved independently.While quasi-static motion cannot describehigh-momentumFigure 1.1The FAD Lego bridge (cantilever)and crane (triangle).(See /lego)4THREE GENERATIONS OF COEVOLUTIONARY ROBOTICSbehavior such as jumping,it can accurately and rapidly simulate low-momentum motion.This kind of motion is suf ficiently rich for the purpose of the experiment and,moreover,it is simple to induce in reality since all real-time control issues are eliminated.Several evolution runs were carried out for the task of locomotion.Fitness was awarded to machines according to the absolute average distance traveled over a speci fied period of neural activation.The evolved robots exhibited various methods of locomotion,including crawling,ratcheting,and some forms of pedalism (Fig.1.2).Selected robots are then replicated into reality:their bodies are first fleshed to accommodate motors and joints,and then copied into material using rapid prototyping technology.A temperature-controlled print head extrudes thermoplastic material layer by layer,so that the arbitrarily evolved morphology emerges preas-sembled as a solid 3D structure without tooling or human intervention.Motors are then snapped in,and the evolved neural network is activated (Fig.1.3).The robots then perform in reality as they did in simulation.1.3.3Modularity Through Generative EncodingsThe main dif ficulty for the use of evolutionary computation for design is that it is doubtful whether it will reach the high complexities necessary forpractical Figure 1.2(a)A tetrahedral mechanism that produces hingelike motion and advances by pushing the central bar against the floor.(b)Bipedalism:the left and right limbs are advanced in alternating thrusts.(c)Moves its two articulated components to produce crablike sideways motion.(d)While the upper two limbs push,the central body is retracted,and vice versa.(e)This simple mechanism uses the top bar to delicately shift balance from side to side,shifting the friction point to either side as it creates oscillatory motion and advances.(f)This mechanism has an elevated body,from which it pushes an actuator down directly onto the floor to create ratcheting motion.It has a few redundant bars dragged on the floor.(See /golem)RESEARCH THRUSTS 5engineering.Since the search space grows exponentially with the size of the pro-blem,search algorithms that use a direct encoding for designs will not scale to large designs.An alternative to a direct encoding is a generative speci fication,which is a grammatical encoding that speci fies how to construct a design [19,20].Similar to a computer program,a generative speci fication can allow the de finition of reusable subprocedures allowing the design system to scale to more complex designs than can be achieved with a direct encoding.Ideally,an automated design system would start with a library of basic parts and would iteratively create new,more complex modules from ones already in its library.The principle of modularity is well accepted as a general characteristic of design,as it typically promotes decoupling and reduces complexity [21].In contrast to a design in which every component is unique,a design built with a library of standard modules is more robust and more adaptable [22].Our system for automated modular design uses Lindenmayer sys-tems (L-systems)as the genotype evolved by the evolutionary algorithm.L-systems are a grammatical rewriting system introduced to model the biological development of multicellular organisms.Rules are applied in parallel to all characters in the string,just as cell divisions happen in parallel in multicellular plex objects are created by successively replacing parts of a simple object by using the set of rewriting ing this system we have evolved 3D static structures [23],andFigure 1.3(a)Fleshed joints,(b)replication progress,(c)preassembled robot,(d)final robot with assembled motor.(See /golem)6THREE GENERATIONS OF COEVOLUTIONARY ROBOTICSlocomoting mechanisms [24],some of which are shown in Figure 1.4,and trans-ferred successfully into reality,as seen in Figure 1.5[25].1.4CONCLUSIONCan evolutionary and coevolutionary techniques be applied to real physical sys-tems?In this chapter we have presented a selection of our work,each of which addresses a physical evolutionary substrate in one or more dimensions.Wehave Figure 1.4Examples of evolved,modular creatures.(See /tinkerbots)Figure 1.5Two parts of the locomotion cycle of a 2D,modular locomoting creature in both simulation and reality.(See /tinkerbots)CONCLUSION 78THREE GENERATIONS OF COEVOLUTIONARY ROBOTICSoverviewed research in the use of simulations for handling high-part-count static structures that are buildable,dynamic electromechanical systems with complex mor-phology that can be built automatically,and generative encodings as a means for scaling to complex structures.Our long-term vision is that both the morphology and control programs for robots arise directly through morphology and control-software coevolution:starting from primitive controllers attached to primitive bodies,the evolutionary system scales to complex,modular creatures by increasing the dictionary of components as stored in the creature encoding.Our current research moves toward the overall goal down multiple interacting paths,where what we learn in one thrust aids the others.We envision the improvement of our hardware-based evolution structures,expanding focus from static buildable structures to buildable robots.We see a path from evolution inside CAD/CAM (computer-aided manufacture)and buildable simulation,to rapid automatic construction of novel controlled mechanisms,andfinally the use of generative encodings to achieve highly complex,modular individuals.We believe such a broad program is the best way to ultimately construct complex autonomous robots whose corporate assemblages consist of simpler,automatically manufactured parts. ACKNOWLEDGMENTSThis research was supported in part by the National Science Foundation(NSF),the office of Naval Research(ONR),and the Defense Advanced Research Projects Agency(DARPA). REFERENCES1.H.P.Moravec.‘‘Rise of the Robots.’’Sci.Am.,124–135,Dec.1999.2.K.Morrison and T.Nguyen.‘‘On-Board Software for the Mars Pathfinder Microrover.’’InProceedings of the Second IAA International Conference on Low-Cost Planetary Missions, Johns Hopkins University Applied Physics Laboratory,Baltimore,MD,April1996. 3.M.Sincell.‘‘Physics Meets the Hideous Bog Beast.’’Science,vol.286,no.5439,398–399,Oct.1999.4.D.Dimos,S.C.Danforth,and M.J.Cima.‘‘Solid Freeform and Additive Fabrication.’’InJ.A.Floro(ed.),1998.5.P.J.Angeline,G.M.Saunders,and J.B.Pollack.‘‘An Evolutionary Algorithm thatConstructs Recurrent Networks.’’IEEE Trans.Neural Networks,vol.5,no.1,54–65,1994.6.H.Juille´and J.B.Pollack.‘‘Dynamics of Co-Evolutionary Learning.’’In Proceedings ofthe Fourth International Conference on Simulation of Adaptive Behavior,pp.526–534, MIT Press,Cambridge,MA,1996.7.J.B.Pollack and A.D.Blair.‘‘Coevolution in the Successful Learning of BackgammonStrategy.’’Mach.Learn.,vol.32,225–240,1998.8.K.Sims.‘‘Evolving3d Morphology and Behavior by Competition.’’In R.Brooks andP.Maes(eds.),Proceedings4th Artificial Life Conference,pp.28–39,MIT Press, Cambridge,MA,1994.REFERENCES9 9.M.Komosinski and S.Ulatowski.‘‘Framsticks:Towards a Simulation of a Nature-LikeWorld,Creatures and Evolution.’’In J.-D.N.D.Floreano and F.Mondada(eds.), Proceedings of5th European Conference on Artificial Life(ECAL99),vol.1674of Lecture Notes in Artificial Intelligence,pp.261–265,Springer-Verlag,New York,1999.10.D.Cliff,I.Harvey,and P.Husbands.‘‘Evolution of Visual Control Systems for Robot.’’InM.Srinivisan and S.Venkatesh(eds.),From Living Eyes to Seeing Machines,Oxford University Press,Oxford,1996.11.D.Floreano and F.Mondada.‘‘Evolution of Homing Navigation in a Real Mobile Robot.’’IEEE Trans.Syst.,Man,Cybern.,1996.12.J.C.Gallagher,R.D.Beer,K.S.Espenschield,and R.D.Quinn.‘‘Application of EvolvedLocomotion Controllers to a Hexapod Robot.’’Robotics Auton.Syst.,vol.19,95–103,1996.13.Y.Kawauchi,M.Inaba,and T.Fukuda.‘‘Genetic Evolution and Self-Organization ofCellular Robotic System.’’JSME Int.J.Ser.C.(Dyn.Control,Robotics,Des.Manuf.), vol.38,no.3,501–509,1999.14.H.Lund.‘‘Evolving Robot Control Systems.In Alexander(ed.),Proceedings of1NWGA.University of Vaasa,Vaasa,Finland,1995.15.P.Funes and J.B.Pollack.‘‘Computer Evolution of Buildable Objects.’’In P.Bentley(ed.),Evolutionary Design by Computers,pp.387–403.Morgan-Kaufmann,San Francisco, 1999.16.P.Funes and J.B.Pollack.‘‘Computer Evolution of Buildable Objects.’’In P.Husbands andI.Harvey(eds.),Fourth European Conference on Artificial Life,pp.358–367,MIT Press,Cambridge,MA,1997.17.P.Funes and J.B.Pollack.‘‘Evolutionary Body Building:Adaptive Physical Designs forRobots.’’Artif.Life,vol.4,no.4,337–357,1998.18.H.Lipson and J.B.Pollack.‘‘Automatic Design and Manufacture of Robotic Lifeforms.’’Nature,vol.406,no.6799,974–978,2000.19.M.Schoenauer.‘‘Shape Representations and Evolution Schemes.’’In L.J.Fogel,P.J.Angeline,and T.Ba¨ck(eds.),Proceedings of the5th Annual Conference on Evolutionary Programming,MIT Press,Cambridge,MA,1996.20.P.Bentley and S.Kumar.‘‘Three Ways to Grow Designs:AComparison of Embryogenies ofan Evolutionary Design Problem.’’In Banzhaf,Daida,Eiben,Garzon,Honavar,Jakiel,and Smith(eds.),Genetic and Evolutionary Computation Conference,pp.35–43,1999.21.N.P.Suh.The Principles of Design.Oxford University Press,Oxford,1990.22.H.Lipson,J.B.Pollack,and N.P.Suh,‘‘Promoting Modularity in Evolutionary Design.’’InProceedings of DETC’012001ASME Design Engineering Technical Conferences, Pittsburgh,PA,Sept.9–12,2001.23.G.S.Hornby and J.B.Pollack.‘‘Body-Brain Coevolution Using l-Systems as a GenerativeEncoding.’’In Genetic and Evolutionary Computation Conference,2001.24.G.S.Hornby and J.B.Pollack.‘‘The Advantages of Generative Grammatical Encodings forPhysical Design.’’In Congress on Evolutionary Computation,2001.25.G.S.Hornby,H.Lipson,and J.B.Pollack.‘‘Evolution of Generative Design Systems forModular Physical Robots.’’In IEEE International Conference on Robotics and Automation, 2001.26.P.J.Bentley.‘‘Generic Evolutionary Design of Solid Objects using a Genetic Algorithm.’’Ph.D.Thesis,Division of Computing and Control Systems,School of Engineering,The University of Huddersfield,Huddersfield,Yorks.,UK,1996.10THREE GENERATIONS OF COEVOLUTIONARY ROBOTICS27.P.Bentley,ed.Evolutionary Design by Computers.Morgan-Kaufmann,San Francisco,1999.28.R.Brooks.‘‘Intelligence Without Representation.’’Artif.Intell.,vol.47,no.1–3,139–160,1991.29.D.Cliff,and J.Noble.‘‘Knowledge-Based Vision and Simple Visual Machines.’’Philos.Trans.R.Soc.London:Ser.B,vol.352,1165–1175,1997.30.P.Husbands,G.Germy,M.McIlhagga,and R.Ives.‘‘Two Applications of GeneticAlgorithms to Component Design.’’In T.Fogarty,(ed.),Evolutionary Computing.LNCS 1143,pp.50–61,Springer-Verlag,New York,1996.31.N.Jakobi.‘‘Evolutionary Robotics and the Radical Envelope of Noise Hypothesis.’’Adapt.Behav.,vol.6,no.1,131–174,1997.32.C.Kane and M.Schoenauer.‘‘Genetic Operators for Two-Dimentional Shape Optimiza-tion.’’In J.-M.Alliot,E.Lutton,E.Ronald,M.Schoenauer,and D.Snyers(eds.),Artificial Evolution—EA95,Springer-Verlag,New York,1995.33.W.Lee,J.Hallam,and H.Lund.‘‘A Hybrid gp/ga Approach for Co-Evolving Controllersand Robot Bodies to Achieve Fitness-Specified Tasks.’’In Proceedings of IEEE3rd International Conference on Evolutionary Computation,pp.384–389,IEEE Press, New York,1996.34.H.Lund,J.Hallam,and W.Lee.‘‘Evolving Robot Morphology.’’In Proceedings of IEEEFourth International Conference on Evolutionary Computation,pp.197–202,IEEE Press, New York,1997.35.M.J.Mataric and D.Cliff.‘‘Challenges in Evolving Controllers for Physical Robots.’’Robotics Auton.Syst.,vol.19,no.1,67–83,1996.36.N.J.Nilsson.‘‘A Mobile Automaton:An Application of Artificial Intelligence Techni-ques.’’In Proceedings of the International Joint Conference on Artificial Intelligence, pp.509–520,1969.。