A Model-based Health Monitoring and Diagnostic System for the UH-60 Helicopter Ann Patterso
某小型肉牛屠宰场产气荚膜梭菌定量风险评估
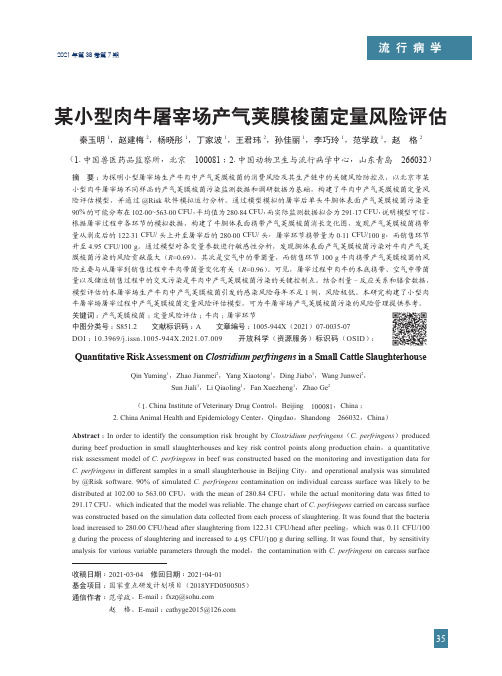
产气荚膜梭菌(Clostridium perfringens)食物 中毒在世界上非常普遍,是发达国家最常见的胃肠 道疾病之一 [1]。产气荚膜梭菌是引起美国食源性疾 病的第二大常见细菌,每年约造成 100 万人感染患 病 [2];在英国,由产气荚膜梭菌引起的食源性疾病 在英格兰和威尔士细菌性食源性疾病中排第三 [3]。
Quantitative Risk Assessment on Clostridium perfringens in a Small Cattle Slaughterhouse
Qin Yuming1,Zhao Jianmei2,Yang Xiaotong1,Ding Jiabo1,Wang Junwei2, Sun Jiali1,Li Qiaoling1,Fan Xuezheng1,Zhao Ge2
利用 @Risk 风险评估软件,对 37 个环节的数 据进行随机分布拟合,将风险评估中的变量和参数 用特定的值、公式或分布表示;采用蒙特卡罗模拟 技术,建立活牛屠宰工艺流程风险评估模型。模型 1 次模拟结果经过 10 000 次迭代运算,每次运算采 用拉丁超立方抽样方法;从模型各变量的概率分布 中取 1 个值,以随机抽取的数进行运算。 1.3 评估模型构建
1 材料与方法 1.1 屠宰加工数据资料
采用活牛屠宰工艺流程参数,通过参考《畜 禽屠宰操作规程 牛》(GB/T 19477—2018),在 采样现场调研和咨询获得。以某小型肉牛屠宰场为 研究模型(每日屠宰活牛 100~200 头),采集标准 屠宰操作程序中的牛体表、牛肛肠、牛胴体以及工 具、环境及零销环节样品等共 260 份,进行产气荚 膜梭菌分离鉴定,获得 37 个环节的牛肉和环境中 产气荚膜梭菌污染定量和定性数据。 1.2 评估方法和工具
智慧病房系统英文建设方案
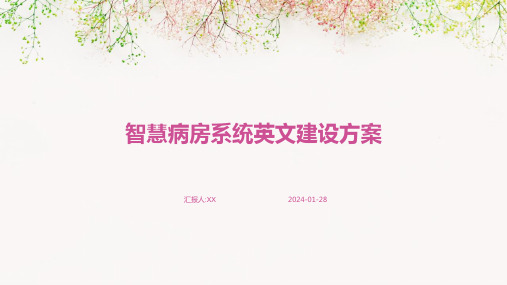
01
03 02
Project Timeline
• Conduct rigorous testing to ensure system reliability and security
Project Timeline
01
Iterate on the design based on user feedback
Better utilization of hospital resources
Increased patient satisfaction and engagement
Benefits and Applications
Applications Remote patient monitoring Early warning systems for critical conditions
02
Collect feedback and make necessary adjustments
03
Phase 4: Full Rollout and Ongoing Support (Months 9-12)
Project Timeline
01
Roll out the system to all wards
Project Timeline
Develop the system components, including hardware and software Phase 2: Development and Testing (Months 36)
Structural Health Monitoring
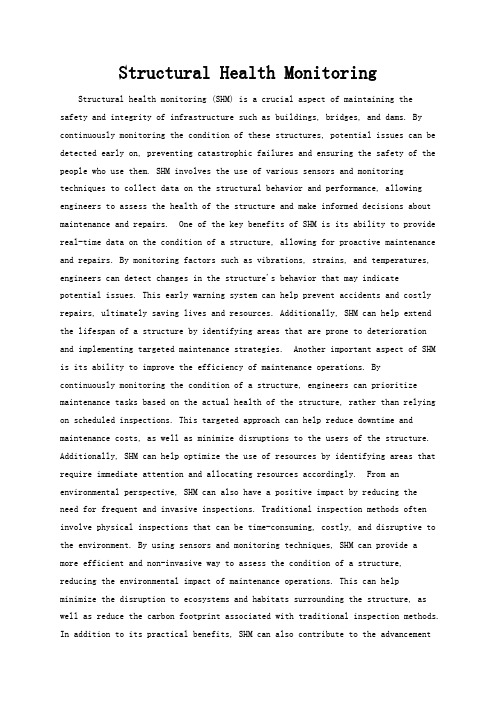
Structural Health Monitoring Structural health monitoring (SHM) is a crucial aspect of maintaining thesafety and integrity of infrastructure such as buildings, bridges, and dams. By continuously monitoring the condition of these structures, potential issues can be detected early on, preventing catastrophic failures and ensuring the safety of the people who use them. SHM involves the use of various sensors and monitoring techniques to collect data on the structural behavior and performance, allowing engineers to assess the health of the structure and make informed decisions about maintenance and repairs. One of the key benefits of SHM is its ability to provide real-time data on the condition of a structure, allowing for proactive maintenance and repairs. By monitoring factors such as vibrations, strains, and temperatures, engineers can detect changes in the structure's behavior that may indicatepotential issues. This early warning system can help prevent accidents and costly repairs, ultimately saving lives and resources. Additionally, SHM can help extend the lifespan of a structure by identifying areas that are prone to deteriorationand implementing targeted maintenance strategies. Another important aspect of SHMis its ability to improve the efficiency of maintenance operations. Bycontinuously monitoring the condition of a structure, engineers can prioritize maintenance tasks based on the actual health of the structure, rather than relying on scheduled inspections. This targeted approach can help reduce downtime and maintenance costs, as well as minimize disruptions to the users of the structure. Additionally, SHM can help optimize the use of resources by identifying areas that require immediate attention and allocating resources accordingly. From an environmental perspective, SHM can also have a positive impact by reducing theneed for frequent and invasive inspections. Traditional inspection methods often involve physical inspections that can be time-consuming, costly, and disruptive to the environment. By using sensors and monitoring techniques, SHM can provide amore efficient and non-invasive way to assess the condition of a structure,reducing the environmental impact of maintenance operations. This can helpminimize the disruption to ecosystems and habitats surrounding the structure, as well as reduce the carbon footprint associated with traditional inspection methods. In addition to its practical benefits, SHM can also contribute to the advancementof engineering knowledge and technology. By collecting and analyzing data on the behavior of structures under various conditions, engineers can gain valuable insights into the performance of different materials and designs. This knowledge can be used to improve the design and construction of future structures, making them more resilient and sustainable. Furthermore, the development of new sensor technologies and monitoring techniques for SHM can drive innovation in the field of structural engineering, leading to more efficient and effective ways to monitor and maintain infrastructure. Overall, structural health monitoring plays acrucial role in ensuring the safety, efficiency, and sustainability of infrastructure. By continuously monitoring the condition of structures, engineers can detect potential issues early on, prioritize maintenance tasks, and optimize the use of resources. Additionally, SHM can help reduce the environmental impact of maintenance operations and contribute to the advancement of engineering knowledge and technology. As we continue to rely on infrastructure for our daily lives, the importance of SHM in maintaining the safety and integrity of these structures cannot be overstated.。
基于噪声测量的车辆变速箱故障诊断 英文翻译 中英文全
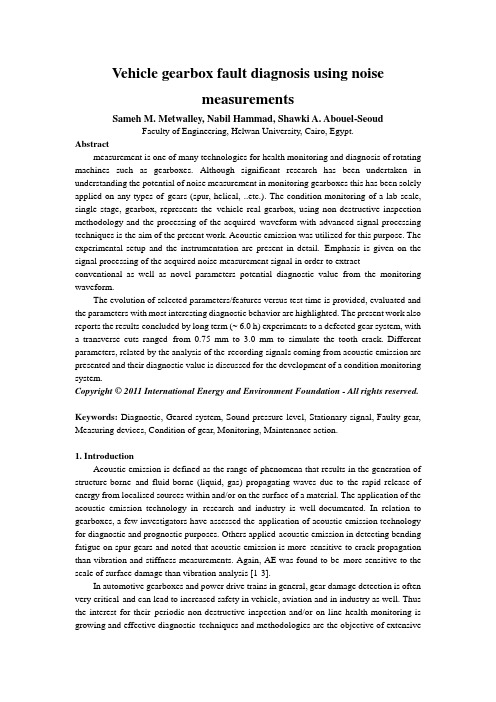
Vehicle gearbox fault diagnosis using noisemeasurementsSameh M. Metwalley, Nabil Hammad, Shawki A. Abouel-SeoudFaculty of Engineering, Helwan University, Cairo, Egypt.Abstractmeasurement is one of many technologies for health monitoring and diagnosis of rotating machines such as gearboxes. Although significant research has been undertaken in understanding the potential of noise measurement in monitoring gearboxes this has been solely applied on any types of gears (spur, helical, ..etc.). The condition monitoring of a lab-scale, single stage, gearbox, represents the vehicle real gearbox, using non-destructive inspection methodology and the processing of the acquired waveform with advanced signal processing techniques is the aim of the present work. Acoustic emission was utilized for this purpose. The experimental setup and the instrumentation are present in detail. Emphasis is given on the signal processing of the acquired noise measurement signal in order to extract conventional as well as novel parameters potential diagnostic value from the monitoring waveform.The evolution of selected parameters/features versus test time is provided, evaluated and the parameters with most interesting diagnostic behavior are highlighted. The present work also reports the results concluded by long term (~ 6.0 h) experiments to a defected gear system, with a transverse cuts ranged from 0.75 mm to 3.0 mm to simulate the tooth crack. Different parameters, related by the analysis of the recording signals coming from acoustic emission are presented and their diagnostic value is discussed for the development of a condition monitoring system.Copyright © 2011 International Energy and Environment Foundation - All rights reserved.Keywords: Diagnostic, Geared system, Sound pressure level, Stationary signal, Faulty gear, Measuring devices, Condition of gear, Monitoring, Maintenance action.1. IntroductionAcoustic emission is defined as the range of phenomena that results in the generation of structure-borne and fluid-borne (liquid, gas) propagating waves due to the rapid release of energy from localised sources within and/or on the surface of a material. The application of the acoustic emission technology in research and industry is well-documented. In relation to gearboxes, a few investigators have assessed the application of acoustic emission technology for diagnostic and prognostic purposes. Others applied acoustic emission in detecting bending fatigue on spur gears and noted that acoustic emission is more sensitive to crack propagation than vibration and stiffness measurements. Again, AE was found to be more sensitive to the scale of surface damage than vibration analysis [1-3].In automotive gearboxes and power drive trains in general, gear damage detection is often very critical and can lead to increased safety in vehicle, aviation and in industry as well. Thus the interest for their periodic non-destructive inspection and/or on line health monitoring is growing and effective diagnostic techniques and methodologies are the objective of extensiveresearch efforts over the last 50 years. Few research teams have published experimental data coming from long-term testing to see the effect of natural gear pitting mostly upon vibration recordings. In [4, 5], some excellent experimental work at GRC/NASA and published interesting results from extensive gear testing at a special test-rig utilizing vibration and oil debris measurements. With the clear goal to improve the performance of the current helicopter gearbox health monitoring systems, they have tested gears at high shaft speed for multi-hour periods (up to 250 h) and correlated special features extracted from the vibration recordings with the Fedebris mass accumulated during the tests.The interest for applications of acoustic emission for condition monitoring in rotating machinery is relatively new and has grown significantly over the last decade. Acoustic emission in rotating machinery is defined as elastic waves generated by the interaction of two media in motion, i.e., a pair of gears.Sources of acoustic emission in rotating machinery include asperities contact, cyclic fatigue, friction,material loss, cavitations, leakage, etc. Acoustic emission technique has drawn attention as it offers some advantages over classical vibration monitoring. First of all, as acoustic emission is a non-directional technique, one acoustic emission sensor is sufficient in contrast to vibration monitoring which may require information from three axes. Since acoustic emission is produced at microscopic level it is highly sensitive and offers opportunities for identifying defects at an earlier stage when compared to other condition monitoring techniques. As acoustic emission mainly defects high-frequency elastic waves, it is not affected by structural resonances and typical mechanical background noise (under 20 kHz). In [6],acoustic emission to spur gears in a gearbox test rig has been applied. It is simulated pits of constant depth but variable size and acoustic emission parameters such as energy, amplitude and counts were monitored during the test. Acoustic emission was proved superior over vibration data on early detection of small defects in gears. Also, acoustic emission technique in condition monitoring of test-rig gearboxes has been applied, while vibration methods was used for comparative purposes by placing accelerometers on the gearbox casing [7, 8]. The influence of oil temperature and the oil film thickness on acoustic emission activity and on acoustic emission RMS varied with time as the gearbox reached a stabilized temperature and the variation acoustic emission activity RMS could be as much as 33%.The effect of oil temperature on the acoustic emission was discussed in [9, 10] and concluded that the source of acoustic emission mechanism that produced the gear mesh bursts was from asperities contact. Moreover, some interesting observations on acoustic emission activity due to misalignment and natural pitting, where the acoustic emission technique is applicable for monitoring gear damage.Researchers in the field have focused mainly on advanced signal processing techniques applied on acoustic recordings coming mainly from artificial gear defects in short tests rather than including gear pitting damage in multi-hour testing. However, the condition monitoring of a lab-scale, single stage,gearbox, represents the vehicle real gearbox, using non-destructive inspection methodology and the processing of the acquired waveform with advanced signal processing techniques is the aim of the present work.2. Stationary signal data analysisThere are numerous signal processing techniques in the literature for fault diagnostics ofmechanical systems. Case-dependent knowledge and investigation are required to select appropriate signal processing tools among a number of possibilities. The most common waveform data in condition monitoring are vibration signals and acoustic emissions. Other waveform data are ultrasonic signals,motor current, partial discharge, etc. In the literature, there are two main categories of stationary waveform data analysis; time-domain analysis and frequency-domain analysis.3. Time-domain analysisTime-domain analysis is directly based on the time waveform itself. Traditional time-domain analysis calculates characteristic features from time waveform signals as descriptive statistics such as mean, peak, peak-to-peak interval, standard deviation, crest factor and high order statistics (root mean square, skewness, kurtosis, etc.). These features are usually called time-domain features. A popular time-domain analysis approach is Time Synchronous Average (TSA). The idea of TSA is to use the ensemble average of the raw signal over a number of evolutions in an attempt to remove or reduce noise and effects from other sources to enhance the signal components of interest.More advanced approaches of time-domain analysis apply time series models to waveform data. The main idea of time series modelling is to fit the waveform data to a parametric time model and extract features based on this parametric model. The popular models used in the literature are the Auto Regressive (AR) model and the Auto Regressive Moving Average (ARMA) model [11].In this paper, only high order statistic of root mean square (RMS) is used. This feature is usually called time-domain features. RMS is a kind of average of signal, for discrete signals, the RMS value is definedas:4. Frequency-domain analysisFrequency-domain analysis is based on the transformed signal in frequency domain. The advantage of frequency–domain analysis over time-domain analysis is its ability to easily identify and isolate certain frequency components of interest. The most widely used conventional analysis is the spectrum analysis by mean of fast Fourier transform (FFT). The main idea of spectrum analysis is to either look at the whole spectrum or look closely at certain frequency components of interest and thus extract features from the signal [12].5. Constant percentage bandwidth (CPB)The basic choice to be made is between constant absolute bandwidth and constant proportional(percentage) bandwidth where the absolute bandwidth is a fixed percentage of the tuned centre frequency. Constant percentage bandwidth gives uniform resolution on a linear frequency scale, and this for example, gives equal resolution and separation of harmonically related components and this will facilitate detection of a harmonic pattern. However, the linear frequency scale automatically gives a restriction of the useful frequency range to (at the most)two decades.It is worth paying particular attention to two special classes of constant percentage bandwidth filter, viz.octave and third octave filters since these are widely used, in particular for acoustic measurements. The former have a bandwidth such that the upper limiting frequency of the pass band is always twice the lower limited frequency, resulting in the band width of 70.0%.6. Measuring system and test procedureFigure 1 shows the experimental setup used for the gearbox testing. The gearbox consists of two helical gears with a module of 2 mm, pressure angle 20°, which have 64 and 26 teeth with 40 mm face width.The axes of the gears are supported by two ball bearings each. The entire system is settled in an oil basin in order to ensure proper lubrication. The gearbox is powered by an electric motor and consumes its power on a hydraulic disc brake, while the speed is measured by photo electric probe. Bruel & Kjaer(B&K) portable and multi-channel PULSE type 3560-B-X05 (Figure 2) with condenser 1/2- microphone and preamplifier type 4189A-021 was positioned in the center of gearbox front casing away from the casing and the ground by 1.0 m and 0.50 m respectively [13]. The B&K PULSE labshop is the measurement software type 7700 is used to analyse the results (Figure 3). In terms of various parameters evolution during the test –from a representative test on a gear system with a cut of root thickness to simulate the tooth crack (Figure 4) will be presented and detailed in this study. Many tests were conducted on the same configuration yield similar parameters behaviour. Small cracks were made artificially with wire electrical discharge machining at the root of gear of one tooth to create a stress concentration which eventually led to a propagating crack. The crack depths are ranged from 0.75 mm to 3.0 mm with thickness of almost 0.5 mm. Recordings every 15 min were acquired and a total of 24 recordings (~ 6.0 h of test duration) were resulted until the termination of the test. This type of test was preferred in order to have the opportunity to monitor bath damage modes, i.e., the natural crack propagation. Damage is assured by increasing the test period to the point of where the remaining metal in the tooth area has enough stress to be in the plastic deformation region. Careful monitoring of the SPLresponses reveals some subtle and increasing changes in responses. When the gear tooth is brought under load, all the response are seen declining slightly over initial few hours, or 'break-in period'. Break-in period is followed by a long period with little or no change in the responses, 'or stable period'. Finally,often several hours prior to failure, one generally sees the responses decrease during the 'divergence period [14].Figure 1. Experimental setup Figure 2. Bruel & Kjaer (B&K) portable andmulti-channel PULSEFigure 3. The B&K PULSE labshop Figure 4. Gear tooth crackFive gear wheels with one pinion whose details mentioned in Table 1 have been used. One was a new wheel and was assumed to be free from defects (g o). In the other four gear wheels, defects were created using EDM in order to keep the size of the defect under control. The details of the various defects are depicted in Table 2 and its view is shown in Figure 4. The size of cracks is a little bigger than one can encounter in the practical situation. The sound pressure level signal from the microphone mounted on front of the test structure was taken, after allowing initial running of the system for sometime.Table 1. Gear and pinion wheels specificationsTable 2. Details of gear wheels various defectsAt crack size (g4), Table 2, recordings every 15 min were acquired and a total of 24 recordings (~ 6.0 h of test duration) were resulted until the termination of the test. This type of test was preferred in order to have the opportunity to monitor bath damage modes, i.e., the natural crack propagation. Damage is assured by increasing the test period to the point of where the remaining metal in the tooth area has enough stress to be in the plastic deformation region. Careful monitoring of the SPL responses reveals some subtle and increasing changes in responses.7. Results and discussionIn Figure 5, where the speed is 400 rpm, and load is 10 Nm for healthy gear, the sound pressure level(SPL) measured at a location of 1.0 m away from the gearbox face in time domain (Figure 5a) and in frequency domain (Figure 5b). This indicates high levels in the frequency ranges of 200 Hz-300 Hz, 400Hz-500 Hz and 600Hz-700 Hz (Figure 5b), while the levels of the remaining frequency are lower and almost constant. The influence of the load on the measured SPLs at speed of 400 rpm is presented in Figure 6, where the 1/3-octave SPL is increased with the increase of the load dispite some small discrepancies exsited in the 1/3-octaves up to 63 Hz (Figure 6b). This may be attributed to the influnce of gear meshing frequencies, rotating shafts frequencies and structure rigidity resonance frequencies.In Figure 7, where the speed is 400 rpm, and load is 10 Nm for faulty gear, the sound pressure level(SPL) measured at a location of 1.0 m away from the gearbox face in time domain (Figure 7a) and in frequency domain (Figure 7b). The whole spectrum levels are higher when compared with those presentin Figure 5b, particularly towards the higher harmonics of tooth-mesh of the output gear, indicating crack. Furthermore, for healthy gears (Figure 5b), the averaged signal is normally dominated by tooth meshing harmonics modulation by the rotation of the gear shaft. When a localized tooth defect, such as tooth crack (g4) of dimension of 3.0 x 0.5 x 40, the engagement of the cracked tooth will induces an impulsive change with comparatively low energy to the gear mesh signal. This can produce some higher shaft-order modulations and may excite structure resonance.The influence of the crack size on the measured SPLs at speed of 400 rpm and load 10 Nm is presented in Figure 8, where the 1/3-octave SPL is increased with the increase of the crack sizes stated in Table 2 dispite some small discrepancies exsited in the 1/3-octaves up to 63 Hz (Figure 6b).Figure 5. Sound pressure level spectra: (a) time history of sound pressure level; (b) frequencydomain of sound pressure levelFigure 6. 1/3-Octave sound pressure level spectra: (a) 1/3-octave sound pressure level; and (b)1/3-octave sound pressure levelFigure 7. Sound pressure level spectra: (a) time history of sound pressure level; and (b)frequency domain of sound pressure levelFigure 8. 1/3-Octave sound pressure level spectra: (a) 1/3-octave sound pressure level; and (b)1/3-octave sound pressure levelTo highlight the noise signal components generated by crack damage only, the influence of the regular sound pressure levels (SPL) components are to be removed for obtaining the residual SPL signal. When there is no crack in the gear, the obtained noise signal can be considered to be regular signals. Thus, if the sound pressure signals with 0% crack has been selected as a reference signal and remove it from each set of cracked gear SPL signals, the information contained in the remaining part is supposed to be only related to the gear crack. The aforementioned equation (1) for RMS is applied to the residual signal,where their results are shown in Figure 9. The influence of load and crack size on the RMS SPL averages are present in Figure 9a and b respectively. It is clearly seen that the SPL in terms of RMS value increased as the increase of load output gear tooth crack size. This significant increase indicates the deterioration in condition.However, when analyzing the noise signal measured from the single-stage gearbox structure in frequency-domain (Figure 10), firstly, each gear's shaft rotating frequency and meshing frequency are calculated. Table 3 tabulates them at motor speed of 400 rpm and load of 2.5 Nm, where their shaft rotating frequencies, ƒpr and ƒgr and meshing frequencies, ƒpm and ƒgm are listed. The spectrum of healthy gearbox is shown in Figure 10a which can be considered to represent the new condition, while Figure 10b represents the faulty gear at crack size (g4) with the dimension of 3.0 x 0.5 x 40 mm. It is found that the spectra are dominated entirely by these frequencies as shown by the arrows. The other significant components in the spectra are an inter-modulation sideband with the same spacing from the first tooth-mesh harmonic as that of the ghost frequency from the fundamental tooth-meshing frequency. Some sidebands are presented but at a relatively low SPL levels.Table 3. Gearbox shafts frequencies at motor speed of 400 rpm and load of 2.5 NFigure 9. RMS sound pressure level: (a) influence of load; and (b) influence of crack dimensionFigure 10. Locations of tooth meshing and shaft rotation frequencies: (a) healthy gear; and (b)faulty gear (g4), 3.0 x 0.5 x 40 mmSamples from SPL responses at speed of 400 rpm, load 15 NM and 330 min for faulty gear with crack dimension of 3.0 x 0.5 x 40 mm in terms of time history and frequency domain are shown in Figure 11a and b respectively, while Figure 12a and b show the 1/3-octave RMS averages for different testing time up to 6.0 hours. The evaluation of RMS average parameter with respect of testing time ranged from 0.0 min to 360 min (6.0 hours) is depicted in Figure 13. To assist the more accurate observation of this parameter evaluation during the range of testing time, a magnification was seen in the Figure 13, where the first transition period is obtained at the end of testing time near 135 min, while the second transition period is observed from 135 to 360 min. These transition periods are important and possess diagnostic value as they can be used to define and characterize critical changes of the gears damage accumulation and evaluation.Figure 11. Sound pressure level spectra: (a) time history (g4), 3.0 x 0.5 x 40 mm; and (b)frequency domain (g4), 3.0 x 0.5 x 40 mmFigure 12. 1/3-Octave sound pressure level spectra: (a) 1/3-octave sound pressure level; and (b)1/3-octave sound pressure levelFigure 13. Relationship between RMS of SPL and testing time8. Conclusion1- The experimental methodology capability developed in this work could be utilized for diagnostic regime. Furthermore, the obvious periodical impulses caused by the cracked tooth appear in time history,frequency domain and in 1.3-octave band averages signals as the crack level increases, these carry diagnostic information which is important for extracting features of tooth crack damage.2- The FFT technique and the high order statistic of RMS reflect in the Sound pressure level (SPL)responses of the gearbox. This can be an effective way to carry out the predictive maintenance regime and consequently to save money and look promising.3- The identification of gearbox noise in terms of SPL is introduced. When applied to thegearbox, the method resulted in an accurate account of the state of the gear, even, when applied to real data taken from the gear test. The results look promising. Moreover, the proposed noise in terms of sound pressure level (SPL) signature methodology has to be tested on the other test rig also. RMS average value analysis could be a good indicator for early detection and characterization of faults.4- In order to study the development of damage in artificially induced cracks in the gearbox, multi-hour tests were conducted and recordings were acquired using noise in terms of SPL monitoring, where the RMS average was calculated. In the recordings, the transitions in the RMS values with the recording time were highlighted suggesting critical changes in the operation of the gearbox.References[1] Singh A, Houser DR. and Vijayakar S. "Detecting gear tooth breakage using acoustic emission: a feasibility and sensor placement study" J Mech Design Trans. ASME 1999;121(4):587–93, 1999.[2] Miyachika K., Zheng Y. and Tsubokura K. "Acoustic Emission of bending fatigue process of super car burized spur gear teeth" Progress in Acoustic Emission XI. Anonymous. The Japanese Society for NDI; 2002. pp. 304 310, 2002.[3] Singh, A., Houser, D.R. and Vijayakar, S. "Detecting gear tooth breakage using acoustic emission:a feasibility and sensor placement study" Journal of Mechanical Design 21(1999) pp. 587–593,1999.[4] Tandon N. and Mata S. "Detection of defects in gears by acoustic emission" J. Acoustic. Emission 1999;17(1–2):23–7.1999.[5] Kramberger, J., Sraml, M., Glodez, S. Flasker, J. and Potrc, I."Computational model for the analysis of bending fatigue in gears", Computers and structures 82 (23-26), pp 2261-2269, 2004.[6] Loutas, T. H., Sotiriades, G.,Kalaitzoglou, I. and KOstopoulos, V."Condition monitoring ofa single-stage gearbox with artificially induced gear cracks utilizing on-line vibration and acoustic emission measurements" Applied Acoustics 70, pp. 1148-1159, 2009.[7] Tan,CK., Irving, P. and Mba, D. "A comparative experimental study on the diagnostic and prognostic capabilities of acoustic emission, vibration and spectrometric oil analysis for spur gears" Mechanical System signal Process, 21(1), pp. 208-233, 2007.[8] Toutountzakis, T., Tan, C. K. and Mba, D. "Application of acoustic emission to seeded gear fault detection" NDT&E Int. 2004;37, pp. 1-10, 2004.[9] Tan, C. K. and Mba, D. "Identification of the acoustic emission source during a comparative study on diagnosis of a spur gearbox" Tribology Int. 2005;38, pp. 469-480, 2005.[10] Eftekharnejad, B. and Mba, D. "Seeded fault detection on helical gears with acoustic. Emission "Applied Acostic 70, pp. 547-555, 2009.[11] Abouel-seoud, S. A., Hammad, N., Abd-elhalim, N., Mohamed, E. and Abdel-hady, M. "Vehicle Gearbox Condition Monitoring Using Vibration Signatural Analysis" SAE Paper No. 2008-01-1654, 2008.[12] Yuan, X. and Cai, L. "Variable amplitude Fourier series with its application in gearbox diagnosis-Part II: Experimental and application" Mechanical Systems and Signal Processing 19, pp. 1067-1081, 2005.[13] Rebbechi, B., Howard, C. and Hasen, C."Active control of gearbox vibration" ACTIVE 99, Fort Lauderdale, Florda USA, December 02-04, 1999.[14] Mille, R.K. and McIntire, P. "Acoustic Emission Testing, vol. 5, second ed" Non- destructiveTesting Handbook, American Society for Non-destructive Testing, 1987, pp. 275–310,1987.基于噪声测量的车辆变速箱故障诊断摘要噪声测量的方法是众多对旋转机械的健康监测和诊断的技术之一,比如变速箱的监测和诊断。
关于健康系统的演讲稿英语

关于健康系统的演讲稿英语Good morning, ladies and gentlemen,。
Today, I am honored to have the opportunity to talk to you about the topic of health system. Health is a fundamental human right, and a well-functioning health system is essential to ensure the well-being of individuals and communities.First and foremost, a good health system should be accessible to all, regardless of their social or economic status. This means that everyone should have access to affordable and quality healthcare services, including preventive, curative, and rehabilitative care. In addition, the health system should be geographically accessible, with healthcare facilities distributed evenly across the country to ensure that no one is left behind.Secondly, a strong health system should be responsive to the needs of the population it serves. This involves not only providing the necessary medical treatments, but also addressing the social, economic, and environmental determinants of health. For example, the health system should be able to respond to outbreaks of infectious diseases, as well as provide support for mental health issues and chronic diseases.Furthermore, a sustainable health system requires adequate financing and resource allocation. Governments should invest in healthcare infrastructure, medical supplies, and human resources to ensure that the health system can meet the growing demands of the population. This also involves effective governance and management of healthcare resources to prevent wastage and ensure efficiency.Moreover, a well-functioning health system should be of high quality, with a focus on patient safety and satisfaction. This includes continuous monitoring and evaluation of healthcare services, as well as the implementation of evidence-based practices and clinical guidelines. Patient-centered care should be at the heart of the health system, with a strong emphasis on communication, empathy, and respect for individual preferences.In conclusion, a robust health system is essential for the overall well-being of a society. It should be accessible, responsive, sustainable, and of high quality. As we continue to face new health challenges and opportunities, it is crucial that we work together to strengthen our health systems and ensure the health and prosperity of future generations.Thank you for your attention.。
人工智能在医疗领域英语作文

人工智能在医疗领域英语作文The Role of Artificial Intelligence in Healthcare.In recent years, artificial intelligence (AI) has emerged as a transformative force across various industries, and healthcare is no exception. The potential of AI in medicine is vast, spanning from diagnosis and treatment to patient care and administrative tasks. This essay delvesinto the various applications of AI in healthcare, its benefits, challenges, and the future of this technology in the medical field.Applications of AI in Healthcare.1. Diagnostics.AI algorithms can analyze medical images with remarkable accuracy, often surpassing human experts. These algorithms can detect subtle abnormalities in radiological images, such as X-rays, MRIs, and CT scans, enablingearlier and more accurate diagnoses. AI-powered tools have also shown promise in diagnosing diseases like cancer, stroke, and heart disease based on symptoms and medical history.2. Treatment Planning.AI systems can analyze a patient's genetic information, medical history, and lifestyle habits to recommend personalized treatment plans. This precision medicine approach ensures that patients receive the most effective treatment based on their unique needs. AI can also help doctors predict how a patient may respond to specific medications or therapies, enabling more informed decisions.3. Patient Care.AI-enabled robots and virtual assistants are being increasingly used to provide patient care. These systems can monitor patients' health remotely,提醒患者按时服药,提供健康咨询,甚至在紧急情况下 alert medical personnel. Such technologies not only improve patient outcomes but alsoreduce the workload on healthcare professionals.4. Administrative Tasks.AI systems can automate many administrative tasks in healthcare, such as scheduling appointments, managing patient records, and billing. This automation reduces the burden on medical staff, allowing them to focus more on patient care. It also improves the efficiency of healthcare facilities and reduces errors in administrative processes.Benefits of AI in Healthcare.1. Improved Accuracy and Efficiency.AI systems can process and analyze vast amounts of medical data much faster than humans, enabling more accurate and efficient diagnoses and treatments. This improved accuracy can lead to better patient outcomes and reduced medical errors.2. Personalized Medicine.AI allows for the development of personalized treatment plans based on a patient's unique characteristics. This approach is more effective and often leads to betterpatient outcomes.3. Cost Savings.By automating administrative tasks and improving the efficiency of healthcare facilities, AI can help reduce overall healthcare costs. This cost savings can be passed on to patients, making healthcare more accessible.4. Improved Patient Experience.AI-enabled tools like remote monitoring and virtual assistants can enhance the patient experience by providing more convenient and personalized care. Patients can receive real-time health updates and alerts, and they can consult with doctors remotely, reducing the need for hospital visits.Challenges of AI in Healthcare.1. Data Privacy and Security.Healthcare data is highly sensitive, and ensuring its privacy and security is crucial. AI systems that handle medical data must comply with strict data protection regulations to prevent breaches and misuse of information.2. Regulatory Challenges.Regulating AI in healthcare can be complex, as it involves balancing innovation with patient safety and ethical considerations. Developing appropriate regulatory frameworks that encourage innovation while protecting patient rights is crucial.3. Integration with Existing Systems.Integrating AI systems into existing healthcare infrastructure can be challenging, especially in older facilities. Ensuring compatibility with legacy systems andsmooth data transfer is essential for the successful implementation of AI in healthcare.4. Ethical Considerations.AI-based decisions can sometimes lack transparency, raising ethical concerns about accountability and fairness. Developers and policymakers need to ensure that AI systems are designed ethically, with mechanisms for oversight and appeals.The Future of AI in Healthcare.The future of AI in healthcare looks bright. As technology continues to evolve, we can expect even more innovative applications of AI in medicine. From more advanced diagnostic tools to fully automated patient care systems, AI will transform healthcare in the coming years. However, it is crucial that we address the challenges of data privacy, regulation, integration, and ethics to ensure that AI is used responsibly and benefits patients and society at large.In conclusion, artificial intelligence is revolutionizing healthcare by improving diagnosis, treatment, patient care, and administrative tasks. Its potential to transform healthcare is vast, but we must also be mindful of the challenges and ethical considerations involved. By addressing these issues, we can ensure that AI is used responsibly and effectively to improve healthcare outcomes for everyone.。
Structural Health Monitoring and Control
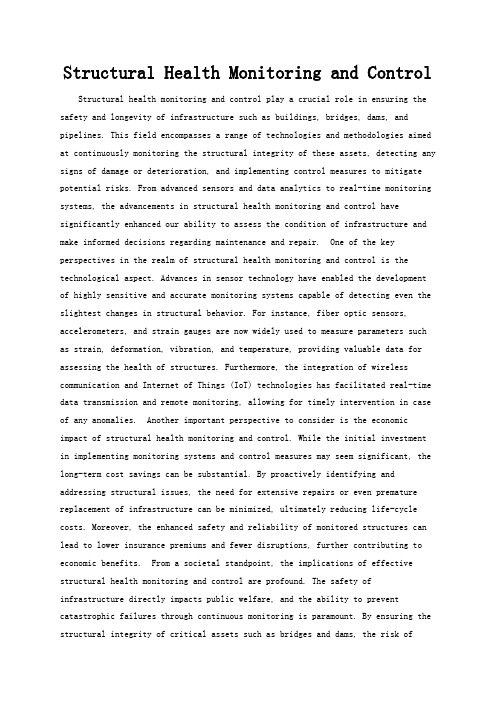
Structural Health Monitoring and Control Structural health monitoring and control play a crucial role in ensuring the safety and longevity of infrastructure such as buildings, bridges, dams, and pipelines. This field encompasses a range of technologies and methodologies aimed at continuously monitoring the structural integrity of these assets, detecting any signs of damage or deterioration, and implementing control measures to mitigate potential risks. From advanced sensors and data analytics to real-time monitoring systems, the advancements in structural health monitoring and control have significantly enhanced our ability to assess the condition of infrastructure and make informed decisions regarding maintenance and repair. One of the key perspectives in the realm of structural health monitoring and control is the technological aspect. Advances in sensor technology have enabled the developmentof highly sensitive and accurate monitoring systems capable of detecting even the slightest changes in structural behavior. For instance, fiber optic sensors, accelerometers, and strain gauges are now widely used to measure parameters such as strain, deformation, vibration, and temperature, providing valuable data for assessing the health of structures. Furthermore, the integration of wireless communication and Internet of Things (IoT) technologies has facilitated real-time data transmission and remote monitoring, allowing for timely intervention in case of any anomalies. Another important perspective to consider is the economic impact of structural health monitoring and control. While the initial investmentin implementing monitoring systems and control measures may seem significant, the long-term cost savings can be substantial. By proactively identifying and addressing structural issues, the need for extensive repairs or even premature replacement of infrastructure can be minimized, ultimately reducing life-cycle costs. Moreover, the enhanced safety and reliability of monitored structures can lead to lower insurance premiums and fewer disruptions, further contributing to economic benefits. From a societal standpoint, the implications of effective structural health monitoring and control are profound. The safety ofinfrastructure directly impacts public welfare, and the ability to prevent catastrophic failures through continuous monitoring is paramount. By ensuring the structural integrity of critical assets such as bridges and dams, the risk ofaccidents that could result in loss of life and property damage can besignificantly reduced. This aspect underscores the importance of investing in advanced monitoring and control technologies as a means of fulfilling our responsibility to safeguard the well-being of communities. In addition to the technological, economic, and societal aspects, the environmental dimension of structural health monitoring and control is also significant. By optimizing maintenance schedules and interventions based on actual structural condition rather than arbitrary timetables, resources can be utilized more efficiently, leading to reduced material waste and energy consumption. Furthermore, the ability to extend the service life of infrastructure through proactive monitoring and targeted maintenance contributes to sustainable development by minimizing the environmental impact associated with construction and replacement activities. In conclusion, structural health monitoring and control represent a critical domain with far-reaching implications for technology, economics, society, and the environment. The integration of advanced sensors, data analytics, and real-time monitoring capabilities has revolutionized our approach to maintaining and managing infrastructure. By recognizing the multifaceted benefits of investing in structural health monitoring and control, we can strive to create safer, more sustainable, and resilient built environments for current and future generations.。
16CH H.264 NVR使用说明书
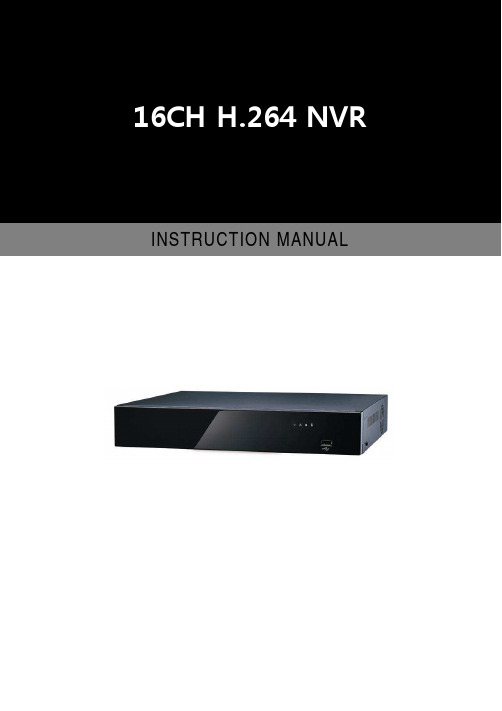
Auto Pan Zoom in Zoom out Preset 0 to 9
Perform auto pan feature Zoom in Zoom out Call preset Numerical keys
NVR remote control operational keyVR
INSTRUCTION MANUAL
Introduction
The 16-channel NVR L series Network Video Recorder offers a standalone solution for managing IP cameras and systems. It uses high quality H.264 decoders to deliver 3MP/1080P/720P/D1/VGA/CIF IP camera streams. Each channel can be individually configured to SD and or HD resolution to optimize storage space.
- 1、下载文档前请自行甄别文档内容的完整性,平台不提供额外的编辑、内容补充、找答案等附加服务。
- 2、"仅部分预览"的文档,不可在线预览部分如存在完整性等问题,可反馈申请退款(可完整预览的文档不适用该条件!)。
- 3、如文档侵犯您的权益,请联系客服反馈,我们会尽快为您处理(人工客服工作时间:9:00-18:30)。
A Model-based Health Monitoring and Diagnostic System for the UH-60 HelicopterAnn Patterson-HineWilliam HindsonDwight SanderferNASA Ames Research CenterSomnath DebChuck DomagalaQualtech Systems, Inc.AbstractModel-based reasoning techniques hold much promise in providing comprehensive monitoring and diagnostics capabilities for complex systems. We are exploring the use of one of these techniques, which utilizes multi-signal modeling and the TEAMS-RT real-time diagnostic engine, on the UH-60 Rotorcraft Aircrew Systems Concepts Airborne Laboratory (RASCAL) flight research aircraft. We focus on the engine and transmission systems, and acquire sensor data across the 1553 bus as well as by direct analog-to-digital conversion from sensors to the QHuMS computer. The QHuMS computer uses commercially available components and is rack-mounted in the RASCAL facility. The multi-signal model enables studies of system testability and analysis of optimal location for potential additional instrumentation. It also allows analysis of the degree of fault isolation available with various instrumentation suites. The model and examples of these analyses will be described and the data architectures enumerated. Flight tests of this system are scheduled to begin in October 2000, and the results will be summarized in the final paper.Model-based reasoning approachA coarse-grain, graphical dependency model was selected for this application in order to allow system-level diagnostics of several helicopter subsystems. Interfaces and dependencies between subsystems and their components were determined and modeled using multi-signal flow graphs [1]. The multi-signal modeling methodology is a hierarchical modeling methodology where the propagation paths of the effects of a failure are captured in terms of a directed graph. Propagation algorithms convert this graph to a single global fault dictionary for a given mode and state of the system. This dictionary contains the basic information needed to interpret test results and diagnose failures duringon-board monitoring. Qualtech Systems, Inc. (QSI) provides an integrated diagnostic tool suite based on the multi-signal model which includes TEAMS for system testability analysis and TEAMS-RT for real-time monitoring and diagnosis. QSI is a participant in the JAHUMS program providing a demonstration of an integrated support system with Sikorsky Aircraft [2]. Our work complements this program by providing an early proof-of-concept demonstration of on-board model-based diagnosis capability.RASCAL multi-signal model and testability analysisThe major components that are modeled in this effort appear in the top-level multi-signal model, shown in Figure 1. These include the fuel storage system, the engines, the transmission, main rotor, and tail rotor. Most of the modeling effort has focused on the engines and the transmission. An example of a lower level of detail in the hierarchical multi-signal model can be found in Figure 2. The components of the transmission system are modeled at this next level of detail, including the generators, accessory modules, input modules, main transmission, and hydraulics. Several instrumentation points, represented by circles, are shown in this view. The testability of these systems was analyzed using TEAMS to characterize expected coverage of an on-board monitoring and diagnostic system which would utilize currently available instrumentation and data paths.Figure 1. Top level multi-signal model.Figure 2. Model detail of transmission.The testability analysis determined that only a third of the potential failures in the transmission can be uniquely isolated even if an on-board reasoner had access to all pilot observables, while 90% of the failures were detectable (see Table 1). Comparisons of these measures for other instrumentation suites are shown in the table, including sensors tapped by the COSSI HUMS project, those presented to the pilot in the cockpit (Caution/Advisory panel and VIDS), vibration, and ground observable tests (called Group 1 and Group 2). Ground observable tests are those conducted by visual inspection or which could be implemented via signal processing or additional optical sensors. These results are being used to address the goal of routing more information to the QHuMS computer which initially only received data available on the 1553 databus. Analyses of AVA sensor locations for potential permanent installation is one example of current workwhich will be described in the final paper.Table 1: Testability Figures of Merit for the transmission system of a UH60A for different levels of instrumentation. (NOTE: Electrical or wiring failures were not modeled. Only Mechanical subsystems and sensor failures were taken intoconsideration)COSSI HUMSPilotObservablePilot Obs. +VibrationAll +Group IAll + GroupsI&IIFault Detection87.489.9797.66100100 Fault Isolation28.4334.5944.5857.7474.53 False Pulls62.2154.9341.2032.520.25QHuMS computerThe QHuMS computer is being developed by QSI under a NASA SBIR contract. It is designed to host the TEAMS-RT software on-board the RASCAL and perform real-time monitoring and diagnostic functions. The core system consists of a dual Pentium III 450 MHz CPU card, a 9GB hard drive, cooling fan, and standard power supply housed in a ruggedized chassis with an 8 slot PICMG industrial backplane. External power is supplied by the UH-60 which powers the chassis cooling fan and provides power to the internal supply. A Jaz drive is located outside the chassis. Several PCI cards are located on the backplane, including a 1553 card and A/D cards to receive data from the aircraft and a SCSI card to interface to the Jaz drive. Figure 3 shows the QhuMS computer mounted in the UH-60.Figure 3. TEAMS-RT computer installed on RASCAL.Flight testsFlight tests of this system are scheduled to begin in October 2000, and the results will be summarized in the final paper.References1.S. Deb et al “Multi-Signal Flow Graphs: A novel Approach for System TestabilityAnalysis and Fault Diagnosis,” Proc.IEEE AUTOTESTCON, Anaheim, CA, pp. 361-373, Sept. 1994.2.David J. Haas, et al, “Joint Advanced Health and Usage Monitoring System(JAHUMS) Advanced Concept Demonstration (ACTD),” Proc. of the 53rd American Helicopter Society Annual Forum, Virginia Beach, VA, May 2-4, 2000.。