On-line Reorganization of Data in Scalable Continuous Media Servers
The statistical treatment of data and the Analysis of MOlecular VAriance (AMOVA) in molecul

Annals of Microbiology, 52, 95-101 (2002)The statistical treatment of data and the Analysisof MOlecular V Ariance (AMOV A) in molecularmicrobial ecologyA. MENGONI*, M. BAZZICALUPODipartimento di Biologia Animale e Genetica ‘Leo Pardi’Via Romana 17, 50125 Firenze, ItalyAbstract - Molecular ecological studies are often characterised by an extremely high num-ber of samples and by an equal high number of molecular analyses to whom the samples have been subjected. The analysis of molecular variance (AMOV A) is a powerful statisti-cal method for the description of factors influencing the structure of populations. AMOV A has been applied to different biological systems, trying to answer as many different ques-tions. To date, AMOV A has helped the elucidation of the factors influencing the shaping of the population genetic structure in different bacterial species, showing the effects of differ-ent variables such as time, soil, drug-sensitivity, plant genotype, location in the plant root system and culture media on the genetic diversity of the bacterial populations.Key words:microbial ecology, molecular fingerprinting, analysis of molecular variance (AMOV A), bacterial populations.INTRODUCTIONThe knowledge of the genetic diversity of the bacterial populations has increased considerably over the last fifteen years, following the application of molecular techniques to ecological studies. Quantitative resolution has improved as a large number of haplotypic markers are found within each sample and as a large num-ber of samples can be simultaneously investigated. The study of molecular ecol-ogy can therefore be characterised by an extremely high number of samples and by a high number of molecular analyses to whom the samples are subjected aim-ing to provide the most complete description of the population under investiga-tion. As a consequence, an immediate interpretation of the results can be difficult, unless powerful statistical techniques are used in order to describe the structure of the populations and to highlight the contribution of its components. These tech-niques are in general methodologies to uncover the effects of categorical inde-95pendent variables on an interval dependent variable. Examples of such techniques are the Analysis of Variance (ANOV A) (Miller, 1997) and the Principal Compo-nent Analysis (PCA) (Chatfield and Collins, 1997), which can handle different data, but which are not specific for the analysis of molecular data. Moreover the significance in ANOV A is tested assuming a normal distribution of data, which is not the case of the molecular polymorphisms. The Analysis of Molecular Vari-ance (AMOV A, Excoffier et al., 1992) is a methodology for the analysis of vari-ance that makes use of molecular data derived from the analysis of DNA. Yet AMOV A is adaptable to different kind of assumption on the evolution of a genetic system. Starting from data of various origin (sequences, isoenzymatic patterns, microsatellites, RFLP, RAPD, REP, PCR-RFLP, RFLP, AFLP), AMOV A makes use of the molecular information gathered in the population study to investigate the genetic differentiation of the sampled populations. Moreover the significance in AMOV A procedure is tested via a permutational approach, eliminating the need of normal distribution that is required for the analysis of variance but inap-propriate for molecular data.The effectiveness and the usefulness of AMOV A for the analysis of molecu-lar data in microbial ecology have been widely demonstrated (Carelli et al., 2000; Chiarini et al., 2000; Dalmastri et al., 1999; Di Cello et al., 1997; Paffetti et al., 1998; Paffetti et al., 1996; Picard et al., 2000; Richner et al., 1999; Tabacchioni et al., 2000).HOW DOES AMOV A WORK?Population genetic structure has traditionally been studied using departures of allele frequencies from panmittic expectations using estimation procedures related to Wright’s F-statistics (Wright, 1951, 1965). F-statistics have been pro-posed for the treatment of several polymorphisms (for detailed references see Excoffier et al., 1992), but few studies only have tried to translate the molecular information, specific of each technique, into estimate of magnitude of intraspe-cific subdivision (Lynch and Crease, 1990; Takhata and Palumbi, 1985). AMOV A was proposed by Excoffier, Smouse and Quattro (1992) to design an alternative methodology that makes use of the available molecular information provided in population studies. AMOVA differs from analysis of variance in that it can accommodate different evolutionary assumptions without modifying the basic structure of the analysis, and in that hypotheses are tested using permutational methods so that normal distribution assumption is not required. Considering a large set of samples, they can be sorted among different groups any of which con-tains in turn different populations each formed by several individuals. The aim of the analysis is to find out and describe that hierarchical structure splitting the total variance into covariance components due to intra-populations, inter-populations and inter-groups differences (Excoffier, 2000). AMOV A computes the differences between groups, among populations within group and among strains (or individ-uals) within the population (Fig. 1).Molecular biology techniques (i.e. RFLP, RAPD, REP, PCR-RFLP, AFLP) produces bands after electrophoresis that can be interpreted in a binary form, i.e. in the form of 0s and 1s, where 1 denotes the presence of a band and zero its absence. On these data a distance matrix can be computed. For binary data, dis-96 A.MENGONI and M. BAZZICALUPOtances can be defined as Euclidean distance (the number of allelic differences between two haplotypes, see Nei and Tajima, 1981). The formalisation for the Euclidean distance is:E=n(1-2n xy /2n),where n is the total number of bands and n xy the number of shared bands.AMOV A can also handle sequence data computing the distance using several parameters (i.e. pairwise differences, Jukes-Cantor, Kimura 2-parameter, Tamura,Tajima-Nei, Tamura-Nei, for a review see Li, 1997), and can analyse allozyme and microsatellite data.To understand the working principle of AMOV A the equation for the i-th haplotype frequency vector should be considered. This equation is in the form:X ijk =x+a k +b jk +c ijkfrom the j-th population in the k-th group. The vector x is the unknown expecta-tion of X ijk , averaged over the whole study. To generate the real haplotype (X ijk ),the deviations from the expected haplotype (x ) are taken into account. These could be subdivided into three component: a , b and c ; a is the effect due to the group, b for being into a population, and c for being different from the other hap-lotypes into the same population. These effects are assumed to be additive, ran-dom, independent, and to have associated covariance components. The total mol-ecular variance is the sum of covariance components due to differences among haplotypes within a population, those due to differences among haplotypes in different populations within the same group, and those due to differences among Ann. Microbiol., 52, 95-101 (2002)97FIG. 1 –Representation of the AMOV A working. The circles indicate the groups and the populations (filled with diagonal rows). The black dots indicate the strains (individuals). The double arrows show the comparisons made: among strains within the population, among populations, among groups.groups. AMOV A computes the percentage that each set of differences (covariance components) has on the total genetic variance found. The covariance components are used to compute fixation (Φ) indices (Wright, 1951, 1965): ΦCT is the corre-lation of random pairs of haplotypes drawn from a group relative to the correla-tion pairs of random haplotypes drawn from the whole population. ΦSC is the cor-relation of random pairs of haplotypes drawn from a population relative to the correlation pairs of random haplotypes drawn from the whole group, averaged over all populations. ΦST is the correlation of random pairs of haplotypes drawn from within populations relative to the correlation pairs of random haplotypes drawn from the whole population. Statistical significance of the distribution of variance components is tested via a test of permutation. In practice, the signifi-cance of fixation indices is tested using a non-parametric permutation approach (Excoffier et al ., 1992), which is based in a permutation of haplotypes, individu-als, or populations, among individuals, populations, or groups of populations.After each round of permutation, all statistics are recomputed to obtain their null distribution.In Table 1 an example of AMOV A result is reported. The hypothesis tested is the existence of an uneven distribution of the genetic variation of nodulating bac-teria (Sinorhizobium meliloti ) linked to the difference among plant cultivars (Medicago sativa ). Groups were formed joining together isolates from different plants belonging to the same cultivar. The total variance derived from the molec-ular data has been divided into the three hierarchical partitions aiming to test the hypothesis of a genetic differentiation based on the different plant cultivar.Columns show the percent of total variance attributed to the partition, the Φsta-tistics and its significance (P-value). In general, the differences within popula-tions (plants) (3rd row) are the main component in the variance partition (highest percentage and ΦST value). P-value is highly significant in the first partition indi-cating that the plant cultivar is a factor influencing the shaping of populations.Moreover, the highly significant values in the second and third partition indicates that the different plants belonging to the same cultivar represent different bacter-ial populations and that in the same plant the different isolates can be distin-guished.A flow-chart of the steps to be performed in an AMOV A approach can be summarised as follows: i) binary scoring of the molecular fingerprint of each iso-98 A.MENGONI and M. BAZZICALUPO TABLE 1 –An example of Analysis of Molecular Variance (AMOV A) from populations of nodulating Sinorhizobium meliloti analysed with RAPD markers Source of variation% of total ΦStatistics P-value Among cultivars12.8ΦCT =0.128<0.0001Plants within cultivars25.7ΦSC =0.295<0.0001Isolates within plant 61.5ΦST =0.385<0.0001The nodulating bacterial populations have been isolated from different plant cultivars (groups). Rows indicate the three hierarchical partitions: among groups, among popula-tions (plants) within groups and within populations (isolates from different nodules within the same plant).late; ii) computing of the distance matrix; iii) assuming an hypothesis of variance partition; iv) testing the hypothetical partition. A software suite (Arlequin, Schneider et al., 2000) has been developed which allows for the computation of AMOV A(Arlequin can be freely downloaded from http://lgb.unige.ch/arlequin/).SOME APPLICATIONS OF AMOV A TO QUESTIONSOF MOLECULAR MICROBIAL ECOLOGYAMOV A was first used to analyse RFLP profiles of human mitochondrial DNA (Excoffier et al., 1992). Later on, AMOV A was utilised for the study of plant and animal ecological problems using RAPD, microsatellites and sequences (Huff et al., 1993; Haig et al., 1994; Roewer et al., 1996; Barbujani et al., 1996; Mengoni et al., 2000a, 2000b).The first report on the use of AMOV A to address questions of microbial ecol-ogy was by Paffetti and co-workers (1996). In that study the genetic polymor-phism of a population of Sinorhizobium meliloti strains was analysed using RAPD markers, aiming at factors that could play a role in shaping the genetic dif-ferentiation of the population. Strains were recovered from nodules of four alfalfa (Medicago sativa) varieties grown in two different soils. AMOV A was utilised to show the correlation of the detected genetic variation with the different environ-mental conditions. In two other studies (Paffetti et al., 1998; Carelli et al., 2000) the question of the different weights of plant variety, soil and season in shaping the population genetic structure of nodulating S. meliloti strains was addressed. AMOV A results indicated that plant genotype was one of the main factor in struc-turing the population and that its contribute, together with that of the soil type, changed over the time allowing the evolution of the bacterial population to be described.AMOVA has also been applied to RAPD data of Burkholderia cepacia colonising maize rhizosphere with the aim to show the influence of variables such as plant growth stage, soil type, cultivar, and position along the plant root system on the genetic structure of bacterial populations (Di Cello et al., 1997; Dalmastri et al., 1999; Chiarini et al., 2000). Here AMOV A successfully allowed to describe the influence of each variable on the genetic differentiation of the population. In particular, a high percentage of the genetic variability was attrib-uted to differences among populations isolated at various soil depths. Moreover, soil was shown to be a major component in affecting the genetic diversity of maize-root associated B. cepacia populations. AMOV A has recently been applied to similar questions with RAPD profiles of Pseudomonas strains (Picard et al., 2000) isolated from roots and rhizosphere of maize. Here AMOV A showed that plant age plays an important role in the genetic variability of the rhizosphere bac-teria, but not of the root bacteria.A third example of application of AMOV A to the ecology of microbial popu-lations came from the analysis of clinical isolates of Mycobacterium tuberculosis (Richner et al., 1999). In this case the aim was to relate the genetic differentiation to the sensitivity to drugs and to the site of isolation (rural or urban). AMOV A indicated the existence of a degree of population subdivision between drug sensi-tive and drug-resistant isolates higher than between isolates from urban and rural areas.Ann. Microbiol., 52, 95-101 (2002)99Finally, AMOV A was recently used in to address the question of possible biases caused by the use of different culture media for the isolation of a bacterial population (Tabacchioni et al., 2000). Strains of B. cepacia from maize rhizos-phere were isolated in two different media and a RAPD fingerprint of each strain was performed. AMOV A was able to recognise two different groups, accordingly with the two media used for the isolation.From these case-studies stand out that AMOV A is a powerful technique to cope with the ecological problems of microbial populations when those are stud-ied at the molecular level. Other statistical methods are available for the analysis of ecological data such as canonical correlation analysis, Multidimensional scal-ing and Principal Component Analysis. These methods have been applied to the ecology of bacterial populations (see for example Buchrieser et al., 1994, and more recently Bernal and Graham, 2001), but the lack of a specific interpretation of the molecular information in these approaches limits their wide-scale use as principal statistical method in the molecular ecology of microbial populations.REFERENCESBarbujani G., Sterico M., Excoffier L., Nigro L. (1996). Mitochondrial DNA sequence variation across linguistic and geographic boundaries in Italy. Hum. Biol., 68: 201-215.Bernal G., Graham P.H. (2001). Diversity in the rhizobia associated with Phaseolus vul-garis L. in Ecuador, and comparisons with Mexican bean rhizobia. Can. J. Micro-biol., 47: 526-534.Buchrieser C., Weagant S.D., Kaspar C.W. (1994). Molecular characterization of Yersinia enterocolitica by pulsed-field gel electrophoresis and hybridization of DNA frag-ments to ail and pYV probes. Appl. Environ. Microbiol., 60: 4371-4379.Carelli M., Gnocchi S., Fancelli S., Mengoni A., Paffetti D., Scotti C., Bazzicalupo M.(2000). Genetic diversity and dynamics of Sinorhizobium meliloti populations nodu-lating different alfalfa varieties in italian soils. Appl. Environ. Microbiol., 66: 4785-4789.Chatfield C., Collins A.J. (1997). Introduction to Multivariate Analysis. Chapman & Hall, London, UK.Chiarini L., Giovannelli V., Bevivino A., Dalmastri C., Tabacchioni S. (2000). Different portions of the maize root system host Burkholderia cepacia populations with differ-ent degrees of genetic polymorphism. Environ Microbiol., 2: 111-118.Dalmastri C., Chiarini L., Cantale C., Bevinino A., Tabacchioni S. (1999). Soil type and maize cultivar affect the genetic diversity of maize root-associated Burkholderia cepacia populations. Microb. Ecol., 38: 273-284.Di Cello F., Bevivino A., Chiarini L., Fani R., Paffetti D., Tabacchioni S., Dalmastri C.(1997). Biodiversity of a Burkholderia cepacia population isolated from the maize rhizosphere at different plant growth stages. Appl. Environ. Microbiol., 63: 4485-4493.Excoffier L., Smouse P.E., Quattro J.M. (1992). Analysis of molecular variance inferred from metric distances among DNA haplotypes: application to human mitochondrial DNA restriction data. Genetics, 131: 479-491.Excoffier L. (2000). Analysis of population subdivision. In: Balding D., Bishop M., Can-nings C., eds, Handbook of Statistical Genetics. Wiley and Sons, Ltd.Haig S.M., Rymer J.M., Heckel D.G. (1994). Population differentiation in randomly 100 A.MENGONI and M. BAZZICALUPOamplified polymorphic DNA of red-cockaded woodpeckers Picoides borealis. Mol.Ecol., 3: 581-595.Huff D.R., Peakall R., Smouse P.E. (1993). RAPD variation within and among natural populations of outcrossing buffalograss [Buchloe dactyloides(Nutt.) Engelm]. Theor.Appl. Genet., 86: 927-934.Li W.-H. (1997). Molecular Evolution. Sinauer, Sunderland, Massachusetts, USA. Lynch M., Crease T.J. (1990). The analysis of population survey data on DNA sequence variation. Mol. Biol. Evol., 7: 377-394.Mengoni A., Gori A., Bazzicalupo M. (2000a). The use of RAPD and SSR (microsatellite) variation for assessing genetic relationships among tetraploid alfalfa. Plant Breeding, 119: 311-318.Mengoni A., Ruggini C., Vendramin G.G., Bazzicalupo M. (2000b). Chloroplast microsatellite variations in tetraploid alfalfa (Medicago sativa L.). Plant Breeding, 119: 509-512.Miller R.G. Jr. (1997). Beyond ANOV A. Basics of Applied Statistics. Chapman & Hall, London, UK.Nei M., Tajima F. (1981). DNA polymorphism detectable by restriction endonucleases.Genetics, 97: 145-163.Paffetti D., Scotti C., Gnocchi S., Fancelli S., Bazzicalupo M. (1996). Genetic diversity of an Italian Rhizobium meliloti population from different Medicago sativa varieties.Appl. Environ. Microbiol., 62: 2279-2285.Paffetti D., Daguin F., Fancelli S., Gnocchi S., Lippi F., Scotti C., Bazzicalupo M. (1998).Influence of plant genotype on the selection of nodulating Sinorhizobium meliloti strains by Medicago sativa. Antonie Van Leeuwenhoek, 73: 3-8.Picard C., Di Cello F., Ventura M., Fani R., Guckert A. (2000). Frequency and biodiversity of 2,4-diacetylphloroglucinol-producing bacteria isolated from the maize rhizosphere at different stages of plant growth. Appl. Environ. Microbiol., 66: 948-955. Richner S., Meiring J., Kirby R. (1999). DNA profiling of Mycobacterium tuberculosis from the Eastern Cape Province of South Africa and the detection of a high level of genetic diversity. Electrophoresis, 20 (1): 800-806.Roewer L., Kayser M., Dieltjes P., Nagy M., Bakker E., Krawczak M., de Knijff P. (1996).Analysis of molecular variance (AMOV A) of Y-chromosome-specific microsatel-lites in two closely related human populations. Hum. Mol. Genet., 5: 1029-1033. Schneider S., Roessli D., Excoffier L., (2000). Arlequin 2.000: a Software for Population Genetic Data Analysis. Genetics and Biometry Laboratory, University of Geneva, Switzerland.Tabacchioni S., Chiarini L., Bevivino A., Cantale C., Dalmastri C. (2000). Bias caused by using different isolation media for assessing the genetic diversity of a natural micro-bial population. Microb. Ecol., 40: 169-176.Takahata N., Palumbi S.R. (1985). Extranuclear differentiation and gene flow in the finite island model. Genetics, 109: 441-457.Wright S. (1951). The genetic structure of populations. Ann. Eugen., 1: 323-334. Wright S. (1965). The interpretation of population structure by F-statistics with special regards to systems of mating. Evolution, 19: 395-420.Ann. Microbiol., 52, 95-101 (2002)101。
Fiscal Policy Effectiveness in Japan
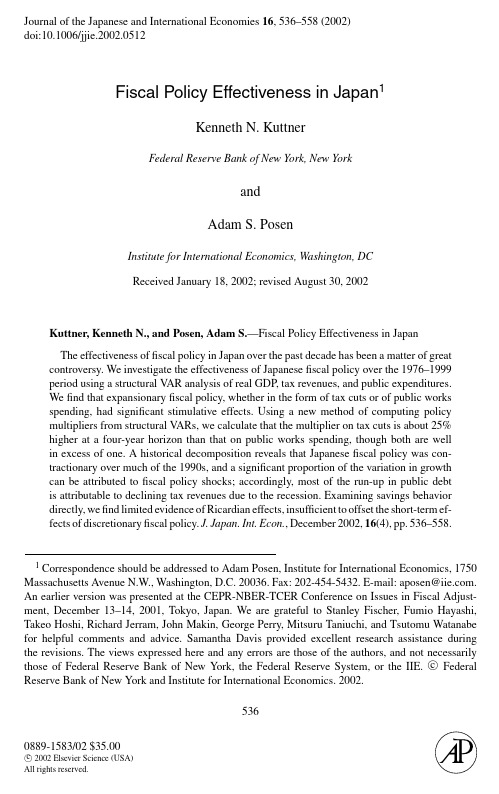
Journal of the Japanese and International Economies16,536–558(2002)doi:10.1006/jjie.2002.0512Fiscal Policy Effectiveness in Japan1Kenneth N.KuttnerFederal Reserve Bank of New York,New YorkandAdam S.PosenInstitute for International Economics,Washington,DCReceived January18,2002;revised August30,2002Kuttner,Kenneth N.,and Posen,Adam S.—Fiscal Policy Effectiveness in JapanThe effectiveness offiscal policy in Japan over the past decade has been a matter of greatcontroversy.We investigate the effectiveness of Japanesefiscal policy over the1976–1999period using a structural V AR analysis of real GDP,tax revenues,and public expenditures.Wefind that expansionaryfiscal policy,whether in the form of tax cuts or of public worksspending,had significant stimulative ing a new method of computing policymultipliers from structural V ARs,we calculate that the multiplier on tax cuts is about25%higher at a four-year horizon than that on public works spending,though both are wellin excess of one.A historical decomposition reveals that Japanesefiscal policy was con-tractionary over much of the1990s,and a significant proportion of the variation in growthcan be attributed tofiscal policy shocks;accordingly,most of the run-up in public debtis attributable to declining tax revenues due to the recession.Examining savings behaviordirectly,wefind limited evidence of Ricardian effects,insufficient to offset the short-term ef-fects of discretionaryfiscal policy.J.Japan.Int.Econ.,December2002,16(4),pp.536–558.1Correspondence should be addressed to Adam Posen,Institute for International Economics,1750 Massachusetts Avenue N.W.,Washington,D.C.20036.Fax:202-454-5432.E-mail:aposen@. An earlier version was presented at the CEPR-NBER-TCER Conference on Issues in Fiscal Adjust-ment,December13–14,2001,Tokyo,Japan.We are grateful to Stanley Fischer,Fumio Hayashi, Takeo Hoshi,Richard Jerram,John Makin,George Perry,Mitsuru Taniuchi,and Tsutomu Watanabe for helpful comments and advice.Samantha Davis provided excellent research assistance during the revisions.The views expressed here and any errors are those of the authors,and not necessarily those of Federal Reserve Bank of New York,the Federal Reserve System,or the IIE. c Federal Reserve Bank of New York and Institute for International Economics.2002.5360889-1583/02$35.00c 2002Elsevier Science(USA)All rights reserved.FISCAL POLICY EFFECTIVENESS IN JAPAN537 Federal Reserve Bank of New York,New York,and Institute for International Economics,Washington,DC.c 2002Elsevier Science(USA)Journal of Economic Literature Classification Numbers:E62,E65,E21.The effectiveness offiscal policy in Japan in the1990s has been at least as controversial as the currently more public disputes over monetary policy.There has been open debate over the degree to which expansionaryfiscal policy has even been tried,let alone whether it has been effective,along with widespread assertions about the degree of forward-looking behavior by Japanese savers.The highly visible and rapid,more than doubling of Japanese public debt in less than a decade speaks for itself to a surprising number of observers:thefiscal deficit has grown sharply,yet the economy has continued to stagnate,sofiscal stabilization failed.No less an economist than Milton Friedman recently wrote,”[D]oesfiscal stimulus stimulate?Japan’s experience in the‘90s is dramatic evidence to the contrary.Japan resorted repeatedly to large doeses offiscal stimulus in the form of extra government spending....The result:stagnation at best,depression at worst, for most of the past decade.”2But it is easy to demonstrate from just charting publicly available data that the bulk of the increase in Japanese public debt is due to a plateau in tax revenue rather than to increased public expenditure or even discretionary tax cuts.This of course reflects the inverse cyclical relationship between output and tax revenue.If one applied a plausible tax elasticity of1.25to reasonable measures of the widening output gap(e.g.,those estimated in Kuttner and Posen(2001)),the result would be a much-reduced estimate of the structural budget deficit.In fact,using the measure of potential based on a constant productivity trend growth rate of2.5%a year all but eliminates the non-social security portion of the deficit.Moreover,as measured by thefiscal shocks derived from our estimates in this paper,fiscal policy has been generally contractionary since1997.More tellingly,the massive increase in Japanese government debt outstanding over the period has had little apparent effect to date on either the level of long-term interest rates or the steepness of the yield curve,or the yen–dollar exchange rate. This is commonly attributed to the passivity of Japanese savers,and there surely has been no sign of crowding out or of inflation fears.This fact has not gone unremarked upon in thefinancial press.3Nevertheless,citing the eventual need to pay obligations,including those off of the government balance sheet(such as pen-sions),the ratings agencies downgraded Japanese local currency sovereign debt to 2Friedman,“No More Economic Stimulus Needed,”Wall Street Journal,October10,2001,p.A17. See also Ian Campbell,“Friedman Opposes Stimulus Package,”UPI Newswire,October9,2001.3The Economist observed,“[government bond yields]fell as the government pumped the economy with...fiscal stimulus,as the yen plummeted by40%from its high in the middle of1995,and even as the government’s debt climbed to100%of GDP.By late[1997]the Japanese government was able to borrow more cheaply than any other government in recorded history.”“Japanese Bonds:That Sinking Feeling,”The Economist,February21,1998,pp.74–75.538KUTTNER AND POSENAA-(by Standard and Poor’s,April15,2002)and A2(by Moody’s,May30, 2002).4But with the exception of a brief panic-induced spike in rates in Jan-uary1999,more than half of which was reversed within two months,holders of Japanese government bonds have yet to take any significant capital losses. Against this background of declining tax revenues and relatively stable long-term nominal interest rates,the actual course of Japanesefiscal policy has been almost tumultuous,rather than one of unremitting spend-spend-spend,as often assumed.The divergence of common perceptions from reality may be due in part to the fact that Japan has a centralized,if arcane,fiscal system.5Every year since 1994has brought announcements of various tax reforms,but their actual impact is difficult to ascertain.6On the public spending side,estimating the mamizu(“true water”)of any Japanesefiscal stimulus requires great care,given institutional complications.7Meanwhile,in terms of revenue collection,the Japanese tax base is rather small by developed economy standards,especially on the household side, where salaried urban workers pay a disproportionate share of the taxes,and small business owners and rural residents pay almost none.8The absence of obvious interest rate,inflation,or crowding out effects from the fiscal measures undertaken leads us to examine what really happened withfiscal policy in Japan in the1990s.If standard theory tells us that expansionaryfiscal policy drives up interest rates,limiting that policy’s effectiveness,then perhaps the absence of an interest rate rise is indicative of the opposite.Ourfirst considera-tion therefore is simply whether thefiscal impulses had Keynesian countercyclical signs and what impact those impulses had.As many observers have stressed,tra-ditional public works in Japan more closely approximate the building of pyramids in hinterlands,famous to macroeconomics undergraduates,than do those in any other OECD country.9Some have indicated that they would expect the multiplier on such wasteful expenditures to be less than one.10Of course,although Keynes 4See Arkady Ostrovsky and Christopher Swann,“Japan hit by downgrade in credit rating,”Financial Times,April16,2002,p.13,and David Ibison,“Japan’s sovereign debt rating downgraded,”, May31,2002.5See Ishi(2000)for a historical perspective;Balassa and Noland(1988),Bayoumi(1998),and OECD Economic Survey:Japan(1999),for institutional descriptions;and Schick(1996)for a com-parison of U.S.and Japanese budget processes.Tax Bureau(2000)gives the official account of the tax system.6See Watanabe et al.(2001)and Tax Bureau(2000).7See Posen(1998).8See Balassa and Noland(1988).9Sixty percent of the Japanese coastline is today reportedly encased in concrete(Ian Buruma,“The Japanese Malaise,”New York Review of Books,July5,2001,p.5).Similar examples are easy to come by:see,for example,Martin Wolf,“Japan’s Economic Black Holes,”Financial Times,January17, 2001,p.21,and Bergsten,Ito,and Noland(2001,pp.64–65).10In June1998the then—Vice Minister of Finance for International Affairs Eisuke Sakakibara (1999,p.45),expressed a contrary point of view:“Concerning the currentfiscal package,I know that there have been various criticisms of it,but I think there is now a wider acceptance,even in the international community,of public works as a more effective means than tax cuts.In addition,under current circumstances,a strong multiplier effect can be expected....”FISCAL POLICY EFFECTIVENESS IN JAPAN539 maintained that even overtly wasteful public works projects were an effective source offiscal expansion,several observers have stressed that in the Japanese context tax cuts are likely to be more effective.We then turn to historical decompositions of the effect offiscal policy on the Japanese economy in the1990s.The ample variation in Japanesefiscal policy,mov-ing from contractionary to expansionary and back to contractionary,with some tax measures temporary and others permanent,provides a rich basis for econometric investigation.Upon that investigation,it becomes clear thatfiscal policy provides an apparent explanation for a surprisingly large amount of the variation in Japanese economic growth over the period.Meanwhile,on the tax side,all tax cuts were preceded and accompanied by loud declarations by government officials that even-tually taxes would have to go up—whether due to the looming demographic threat, to the unsustainability of Japanese public debt,or to the supposedly declining po-tential growth rate.Even though wefind that these well-publicized dangers from debt did not have any obvious short-run effect on multipliers,we also directly examine the possibility of Ricardian equivalence.Finally,we conclude by consid-ering some of the questions raised by the apparentfiscal power granted through savers’passivity in Japan.The analysis here builds on earlier work applying a structural V AR approach tofiscal policy in Japan(Kuttner and Posen,2001),but extends that paper’s in-vestigations in four important ways.First,impulse response functions and their standard errors are calculated,allowing a clear sense of the significance and inter-action offiscal policy shocks.Second,fiscal shocks and their contributions to GDP growth in the1990s are computed and plotted,yielding an analysis of the historical record.Third,a new approach is introduced to compute“pure”policy multipliers from structural vector autoregressions(V ARs)in order to give a clearer picture of the effects of tax and expenditure changes in isolation.And fourth,throughout the paper,a variety of robustness checks are considered,especially with regard to the results’sensivity to the identifying assumptions.1.THE SHORT-RUN EFFECTS OF FISCAL POLICYTo assess the impact offiscal policy on the economy,we employ a structural three-variable V AR model adapted from Blanchard and Perotti(1999),which is designed to identify the impact offiscal policy while explicitly allowing for con-temporaneous interdependence among output,taxes,and spending.The one-lag version of the structural V AR can be expressed succinctly asA0Y t=A1Y t−1+Bεt,(1) where Y t=(T t,E t,X t) is the vector of the logarithms of real tax revenue,real expenditure,and real GDP,andεt is interpreted as a vector of mutually orthogonal shocks to the three jointly endogenous variables.540KUTTNER AND POSENFollowing Blanchard and Perotti,a key identifying assumption is that real GDP is allowed to have a contemporaneous effect on tax receipts,but not on expenditure. (As discussed below,however,plausible changes to this assumption make no substantive difference to the results.)The model also assumes that taxes do not depend contemporaneously on expenditure(or vice versa)although tax shocks are allowed to affect spending within the year.This assumption reflects the institutional setup forfiscal policy in Japan,where taxes are mostly collected from withholding and consumption,spending is mostly implemented with a lag,and both automatic stabilizers and the size of the public sector are limited.With these assumptions imposed,the model can be written asT t=a130X t+a111T t−1+a121E t−1+a131X t−1+εT tE t=a211T t−1+a221E t−1+a231X t−1+b21εT t+εE t(2)X t=a310T t+a320E t+a311T t−1+a321E t−1+a331X t−1+εX t,where a i j0,a i j1,and b i j represent the i,j th elements of the A0,A1,and B matrices.Thus a130captures the within-period elasticity of tax receipts with respect to GDP,b21is the effect of tax shocks on expenditure,and a310and a32allow taxes andexpenditure to affect real GDP contemporaneously.With seven parameters to estimate from the six unique elements of the covariance matrix of reduced-form V AR residuals,the model in(2)is not identified,however.11 Our strategy,like that of Blanchard and Perotti,is to use independent informationon the elasticity of tax revenue with respect to real GDP(i.e.,a130)to identifythe model.Drawing on Giorno et al.(1995),we set this parameter equal to1.25, yielding an exactly identified model.Reliable comprehensive quarterlyfiscal data for Japan are not available to the public or to the internationalfinancial organizations,unfortunately,and so we fit the model instead to annual consolidated central,state,and localfiscal data, compiled by the IMF,spanningfiscal years1976through1999.12Tax receipts are defined as direct and indirect tax revenue,excluding social security contributions. Expenditure corresponds to the sum of current and capital expenditure,less so-cial security and interest payments.13The estimated model also includes a linear trend and a trend interacted with a post-1990dummy to capture the post-1990 11See Hamilton(1994,chapter11)for a complete discussion of identification and estimation of structural V ARs.12This lack of timely higher frequency data is of course of policy significance,as well as presenting a difficulty for research.As Stanley Fischer(2001,p.163)observes,“Indeed,there is a general problem offiscal transparency in Japan...the key issues are lack of consolidation among differentfiscal units and the absence of quarterly data,which means thatfiscal information is on average about eight months out of date.”13As noted by Blanchard and Perotti(1999),estimating the third equation in the structural V AR is equivalent to using a measure of“cyclically adjusted”tax receipts(and a similarly adjusted measure of spending)as instruments for taxes and spending in a two-stage least squares regression.FISCAL POLICY EFFECTIVENESS IN JAPAN541TABLE IThe Relationship between Taxes,Spending,and GDP:Estimated Parameters of the Structural V AREquationIndependent variable Lag Tax Expenditure GDP Tax receipts t——−0.03 Expenditures t——0.17∗∗Real GDP t 1.25——Tax receipts t−10.71∗∗∗−0.12−0.25∗∗Expenditures t−10.030.78∗∗∗0.02 Real GDP t−1−0.58∗∗0.66∗0.59∗∗∗Tax shock t—−0.03—Trend−0.004−0.0020.033∗∗∗Trend×(t>1990)−0.018−0.010−0.038∗∗∗Adjusted R20.9960.9950.997 Durbin–Watson 1.66 2.30 1.85 Source.Authors’calculations,based on trivariate structural V AR including real tax revenue,real government expenditures and real GDP,estimated on24annual observations spanningfiscal years 1976through1999.Note.Asterisks indicate statistical signficance:∗∗∗for0.01,∗∗for0.05,and∗for0.10.The coefficient of1.25on real GDP in the tax equation is imposed a priori as an identifying assumption.The adjusted R-squared and Durbin–Watson statistics are from the reduced form V AR equations.Further details can be found in the text.slowdown in trend GDP growth.14The estimated parameters are displayed in Table I.Interpreting individual coefficients of a simultaneous equation model is difficult,of course,but it is worth noting that expenditures have a positive,statis-tically significant impact on real GDP.Figure1plots the impulse response functions for the four-year time-horizon relevant for policy analysis,along with90%confidence bands associated with the estimates.As shown in thefirst two panels of the last row of thefigure,tax cuts and expenditure increases both have expansionary effects.Moreover,the estimated effects are statistically significant at a one-to two-year horizon,as well as for the current year in the case of expenditure shocks.The estimated magnitudes of both tax and expenditure effects are comparable as well.The upper left-hand panel of Fig.1shows that tax revenue shocks tend to be relatively transitory,effectively vanishing after one year,notwithstanding the characterization of most Japanese tax law changes as permanent in intent.15,16In contrast,the center panel of the 14The model makes no explicit distinction between temporary and permanent tax and expenditure changes,in part because the temporary tax changes enacted in Japan have been much smaller in magnitude than the permanent ones(see Watanabe et al.,2001).Many of the supposedly permanent tax changes were offset by subsequent tax legislation,however,and this pattern should be picked up by the model’s dynamics.15This pattern is documented in Watanabe et al.(2001).Because of the feedback between tax revenues and GDP,and the greater-than-unit elasticity of tax revenue with respect to GDP,the impact of a10%tax shock on tax revenue is slightly less than10%.16The lack of a significant response of expenditures to tax shocks may appear atfirst to contradict the results of Ihori et al.(2001),who found Granger causality from the taxes to expenditures,expressed542KUTTNER AND POSEN years after shockp e r c e n t effect of tax shock on tax 01234-14-7714effect of tax shock on spending 01234-10-5051015effect of tax shock on GDP 01234-551015effect of spending shock on tax 01234-14-70714effect of spending shock on spending 01234-10-551015effect of spending shock on GDP 01234-5051015effect of GDP shock on tax 01234-14-70714effect of GDP shock on spending 01234-10-5051015effect of GDP shock on GDP01234-5051015FIG.1.Estimated impulse responses from structural V AR.Standard errors were computed via Monte-Carlo.Dashed lines represent 90%con fidence intervals.No standard errors are given for the contemporaneous effects of GDP and spending shocks on spending,as these are fixed by assumption.The tax shock represents a tax cut,and the spending shock represents a spending increase.figure shows that public works spending shocks are highly persistent,in keeping with institutional and journalistic accounts of government behavior in Japan (and elsewhere).The dynamic effects of tax and spending shocks,including the expansionary effect of tax cuts on GDP,are easier to interpret (and more dramatic)when put in yen terms,as is done in Table I.To do so requires scaling up the response by the inverse of the share of taxes in GDP,which averaged 19%during the 1990s.This adjustment results in a cumulative Y =484increase in GDP in response to a Y =100tax cut.One explanation for the size of the response is that,over the sample period,tax cuts have tended to be associated with spending increases;in fact,the cumulative increase in spending is roughly equal to the decrease in taxes (although this effect as a share of GDP.A closer look shows that the results are consistent,however:in our model,positive tax shocks decrease the level of real GDP in our model while leaving expenditures largely unchanged in the near term,which leads in turn to an increase in expenditures as a share of GDP.FISCAL POLICY EFFECTIVENESS IN JAPAN543 is estimated rather imprecisely).17Overall,GDP rises by more than twice the sum of the spending and tax effects.The immediate impact of a10%positive spending shock on GDP is1.6%, however,which translates into Y=84for a Y=100spending increase,and the stimulus builds only slightly over time.One reason for the smaller estimated effect of spending than of tax shocks is that taxes tend to rise in response to positive spending shocks in this sample,partly offsetting the expansionary impact of the spending increase.This can be interpreted as evidence of the expensive maintenance of unproductive Japanese public works projects.Overall,the increase in GDP is about1.75times the net effect of the spending minus the tax increases—smaller than the effect of tax shocks,but still a respectable economic impact.Deriving a model with sufficient structure to assess the impact offiscal pol-icy clearly requires a number of strong identifying assumptions.As noted above, three such assumptions are embedded in a Blanchard–Perotti framework:first,that current taxes do not depend directly on current expenditures;second,that current expenditures to not respond directly to current GDP;and third,that the within-year elasticity of tax revenues with respect to GDP is1.25.Since the model is exactly identified,these restrictions are not formally testable,of course,but the reported results are robust to plausible changes in all three of these assumptions.18 In particular,allowing for a contemporaneous effect from spending shocks to tax revenues(instead of the other way around)has virtually no effect on the results. The results are slightly more sensitive to changes to the assumed elasticity of tax revenues,but for plausible values of the parameter(i.e.,ranging from1.0to 1.5),the estimates are qualitatively similar to those reported above.And it turns out that assuming a plausible,negative elasticity of expenditures with respect to GDP(reflecting a presumed countercyclical use offiscal policy)actually increases the estimated effects offiscal shocks.These robustness checks therefore indicate that thefindings are not merely an artifact of the model’s identifying assump-tions.This analysis shows that,when it has been used,discretionaryfiscal policy in Japan has in fact had the effects predicted in standard closed-economy macroe-conomic analyses.Both tax cuts and spending increases lead to higher real GDP, although the tendency for taxes and spending to move together has reduced the impact of spending increases.19The commonly held perception offiscal policy’s 17Blanchard and Perotti(1999)found a qualitatively similar pattern in the U.S.data.18The full results obtained under these alternative assumptions are available from the authors upon request.19Further work is needed to reconcile our results on the sizable effects offiscal policy in Japan with thefindings(using very different econometric approaches)of Bayoumi(2001)and Perri(1999) thatfiscal policy had the expected sign but very small effects,and of Ramaswamy and Rendu(2000) that“public consumption had a dampening impact on activity in the1990s.”A likely explanation is that these analyses did not take full account of the dynamic interactions among GDP,tax revenue, and expenditure in the way that we were able to.544KUTTNER AND POSENineffectiveness in all likelihood stems from a failure to recognize the dependence of tax receipts with respect to GDP:as GDP falls,tax revenue shrinks,but to conclude from this that changes in the deficit have not affected growth would be incorrect.2.MULTIPLIERS ON TAX CUTS AND PUBLIC SPENDINGThe difficulty in reading off a simple multiplier from our estimations is that in the data(and therefore in Japanese reality over the period)tax cuts generally have been accompanied by spending increases;expenditure increases,on the other hand, have generally been accompanied by tax increases.So,for example,in Table II, where we list the Y=484estimate of the effect on GDP of a Y=100tax cut,we are actually reporting the four-year cumulative effect of the tax cut and of the accompanying expenditure increase seen in the data.A fair comparison of the effects of(or multiplier on)tax cuts and expenditure increases therefore requires taking into account any correlation between taxes and expenditures.To do this,we examine the responses to linear combinations of tax and spending shocks calculated to generate a cumulative1%change in the variable of interest, and a cumulative zero response to the other variable,measured at a four-year hori-zon.The response of GDP to this combination of shocks is then used to calculate a “pure”multiplier on tax or spending shocks.For example,a−0.66%(expansion-ary)tax shock combined with a−0.21%(contractionary)spending shock gives a1%reduction in tax revenues over four years,with no cumulative impact on spending,and a net0.47%increase in real GDP.Scaling this response by the inverse of the share of taxes in GDP(using the 1990–1999)average of19%)yields a multiplier for tax cuts of2.5;a similar calculation for spending increases gives a multiplier of2.0.As a result of this difference in magnitudes,the cumulative four-year gain to Japanese GDP from a revenue neutral shift of Y=100from public works spending to tax cuts is Y=47.TABLE IIThe Dynamic Impact of Fiscal Policy:Estimated Yen-Denominated Impulse Responses(Effects of expansionary Y=100shocks,in yen)Impact of−Y=100tax shock Impact of+Y=100spending shockTaxes Spending GDP Taxes Spending GDP Year0−963162010084Year1−32161583487105Year2036168377789Four-year cumulative−111104484127332353Source.Authors’calculations based on the estimated structural V AR.Note.The impact of Y=100tax and spending shocks are computed assuming taxes andspending represent19%of GDP.FISCAL POLICY EFFECTIVENESS IN JAPAN545 These estimates also understate the beneficial effects of tax cuts,because they do not directly capture the allocative efficiency gains from changes in Japanese tax code,just the immediate macroeconomic impact.Though such gains can be exaggerated,there is good reason to believe that such supply-side effects would be large in Japan today.These effects atfirst glance may seem rather large,relative to other published estimates;in fact they are quite close to comparably calculated multipliers for the United States,such as those of Blanchard and Perotti(1999).The“multipliers”reported there,however,are defined differently from those we calculate.Blanchard and Perotti reported multipliers defined as the ratio of the peak response of GDP to the size of the initial shock to taxes or spending.That method can be misleading, however,as it fails to take into account either the dynamics of the response or the tendency for taxes and spending to move together.20Using our method to calculate comparable multipliers from Blanchard and Perotti’s trend-stationary estimates, we obtain a multiplier of roughly4.0for tax shocks—considerably larger than our estimate for Japan.Our estimated spending multiplier for Japan is somewhat higher than the comparable multiplier for the United States calculated from the Blanchard–Perotti results,but quite close to similar calculations based on their estimated response to military spending shocks.In contrast to these results,the Economic Planning Agency(EPA)of the Japanese government(now the Cabinet and Fiscal Office)has published declining esti-mates of the multiplier onfiscal policy for the past several years.In May1995, the EPA World Economic Model5th Version reported cumulative multipliers on government investment of1.32in thefirst year,1.75in the second year,and2.13 in the third year(down from1.39,1.88,and2.33in the4th Version),and far lower multipliers on income tax reductions(0.46,0.91,and1.26,down from0.53,1.14, and1.56in the4th Version).21In October2001,the EPA released the multipli-ers from the1998revised version of the model,with the cumulative multipliers on government investment declining to1.12,1.31,and1.10,and on income tax reductions of0.62,0.59,and0.05.22Leaving aside the question of whether these changes represent statistically significant differences,given the difficulties of esti-mating these multipliers,it is worth considering the source of this divergence from our results.The difficulty in making a strict comparison lies in the unavailability(at least publicly,in English)of the details of the EPA’s large-scale macro model,partic-ularly with regard to the assumed response of monetary policy built in.As the discussion in OECD(2000,pp.60–64)makes clear,while there are a number of20Basing the multiplier on the peak response could,for example,yield a nonzero multiplier even if the effect on GDP were completely reversed in subsequent periods.21See“The EPA World Economic Model5th Version:Basic Structure and Multipliers,”Economic Analysis Series139,May,1995,www.esri.cao.go.jp/en/archive/bun/abstract/139-e.html.22See“The ESRI Short-Run Macroeconometric Model of Japanese Economy:Basic Structure and Multipliers,”October2001,www.esri.cao.go.jp/en/archive/e-dis/abstract/006-e.html.。
ON THE MEAN DISTANCE IN SCALE FREE GRAPHS

Keywords and phrases : scale free graphs, mean distance
1
Introduction
In the first subsection of this introduction, we introduce the graph model, discuss its relevance, and present our main results. In Section 1.2, we review related work, whereas in Section 1.3 we explain the organization of this paper.
x j =1 fj ,
where x is the largest integer smaller than or equal to x. As in [22] we will assume that
for some τ > 3 and some positive c, 1 − F (x) ≤ cx−τ +1 , x > 0. (2)
∗
Delft University of Technology, Electrical Engineering, Mathematics and Computer Science, P.O. Box 5031, 2600
GA Delft, The Netherlands. E-mail: G. Hooghiemstra@ewi.tudelft.nl, P.VanMieghem@ewi.tudelft.nl
This graph model that includes heavy tail degrees with finite variance is a variant of the configuration model, which, given a degree sequence, is the random graph with that given degree sequence. For a graph, the degree sequence of that graph is the vector, of which the k th coordinate equals the frequency of nodes with degree k . In our model, the degree sequence is very close to the distribution of the nodal degree D of which D1 , . . . , DN are i.i.d. copies. We further define µ = E[D], ν= E[D(D − 1)] . E[D] (3)
N-adic Summation-Shrinking Generator Basic properties and empirical evidences
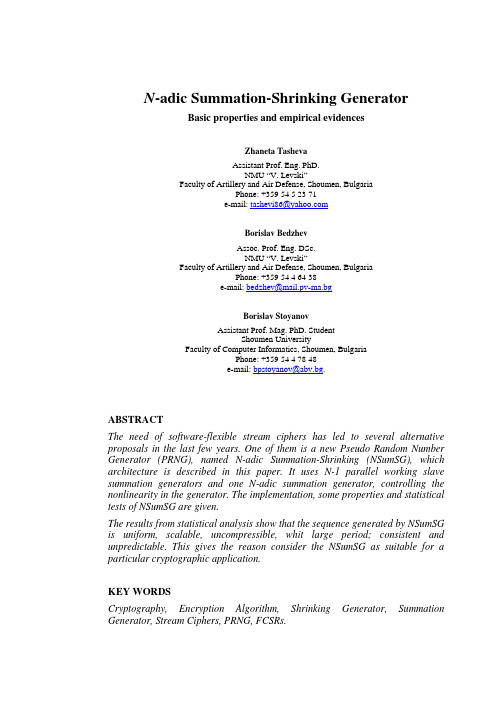
N-adic Summation-Shrinking GeneratorBasic properties and empirical evidencesZhaneta TashevaAssistant Prof. Eng. PhD.NMU “V. Levski”Faculty of Artillery and Air Defense, Shoumen, BulgariaPhone: +359 54 5 23 71e-mail: tashevi86@Borislav BedzhevAssoc. Prof. Eng. DSc.NMU “V. Levski”Faculty of Artillery and Air Defense, Shoumen, BulgariaPhone: +359 54 4 64 38e-mail: bedzhev@mail.pv-ma.bgBorislav StoyanovAssistant Prof. Mag. PhD. StudentShoumen UniversityFaculty of Computer Informatics, Shoumen, BulgariaPhone: +359 54 4 78 48e-mail: bpstoyanov@abv.bg.ABSTRACTThe need of software-flexible stream ciphers has led to several alternative proposals in the last few years. One of them is a new Pseudo Random Number Generator (PRNG), named N-adic Summation-Shrinking (NSumSG), which architecture is described in this paper. It uses N-1 parallel working slave summation generators and one N-adic summation generator, controlling the nonlinearity in the generator. The implementation, some properties and statistical tests of NSumSG are given.The results from statistical analysis show that the sequence generated by NSumSG is uniform, scalable, uncompressible, whit large period; consistent and unpredictable. This gives the reason consider the NSumSG as suitable for a particular cryptographic application.KEY WORDSCryptography, Encryption Algorithm, Shrinking Generator, Summation Generator, Stream Ciphers, PRNG, FCSRs.SECTION 1IntroductionThe proliferation of computers and communications systems in the 1960s brought with it a demand from the private sector for means to protect information in digital form and to provide security services. The stream ciphers are an important tool for solving this problem. Despite of their large application, it is very hard or may be impossible to describe all factors, which influence over the performance quality of the stream ciphers. Anyway, surely it depends on their crypto resistance, velocity and effectiveness of hardware implementation. Mostly the crypto resistance of a stream cipher is connected with it ability to generate pseudo random sequence (PRS or gamma) with following properties:(1) it should have enormous period;(2) it should demonstrate uniform distribution of d-tuples (for a large range of d);(3) it should exhibit a good structure (usually a lattice structure) in high dimensions.Unfortunately, the mentioned factors are in contradiction, because if the structure of the stream cipher is simple in order to provide high performance velocity and cost-effective hardware implementation, then the crypto reliability is low. For instance, the classical fast and cheap Linear Feedback Shift Registers(LFSRs) are vulnerable to the so - named “Berlekamp–Massey crypto attack” [4], [5], [8]. This attack allows finding of all bits of a LFSR output sequence, if 2n its consequent bits are known. Here n is the number of the cells connected in the LFSR. Having in mind the advantages of the stream ciphers with simple structure, recently some theorists [3], [4], [6] proposed a new approach to stream cipher design. The basic idea of this approach is building devices with high crypto reliability combining in some appropriate way crypto vulnerable, but fast and cheap elements (including LFSR). This meaning of stream cipher design leaded to introducing of a few new architectures. It should be mentioned the so-named summation generator, shrinking generator and N-adic Feedback with Carry Shift Register (N-FCSR) [2], [3], [13]. They are promising candidates for high-speed encryption applications due to their simplicity and provable properties.With regard to positive features of the summation generator, shrinking generator and N-FCSRs, our paper is focused on the problem of synthesis of a derivative structure, named summation-shrinking generator.The paper is organized as follows. First, the basics of the summation generator and shrinking generator are recalled. Second one their derivative structure, called N-adic Summation-Shrinking Generator (NSumSG) is presented. After then, the implementation and statistical analysis of NSumSG properties are given. Finally, the advantages and possible areas of application of our algorithm are discussed.SECTION 2Basic theory of the summation and shrinking generatorsPrincipally the crypto resistance of a stream cipher, based on LFSR s, can be enhanced by two alternative methods. The first method uses an appropriate combining of the outputs of some LFSR s, as it is shown on Fig.1a. These gamma generators are called “Combination Generators”. The other alternative is to generate the gamma as a non-linear function from conditions of the single LFSR triggers (Fig.1b). In this case the gamma generators are named “Filter Generators”.Fig. 1a:Combination generator Fig.1b:Filter-generatorHaving in minded that:- the filter-generators could be studied as a particular case of the combination generators when S = 1 on Fig. 1a;- the combination generators are still being applied in some real communication and information systems [5], [7];in the rest part of this report our attention shall be focused on the derivative structures of the combination generator.As mentioned, the basic idea of the combination generator method is to create a hard-to-crack gamma sequence by an appropriate combining of some PRSs, whose technical realization is simple and cost-effective. The scheme, shown on Fig.1a, is an object of intensive research since 1984, because it is easy to generate PRSs with LFSRs. As a result of these efforts [6] the cryptologist Rueppel has proved that the combination generators have maximal linear complexity L(x) if: - the all LFSR s have a feed-back loop, described with primitive irreducible polynomial (i.e. the created PRSs are maximum length sequences (shortly m-sequences));-the periods T i,i = 1, 2, …, s of the PRSs, generated by LFSR s, are different.Here linear complexity L(x ) means the length of the binary LFSR , which can beconstructed in the result of the Berlekamp-Massey crypto attack.The Rueppel conditions are easy to realizing as a s-bit adder. This means that ffrom Fig.1a must be a full adder, which has 1log 2 s triggers. In order tosimplify the explanation, we shall suppose, that the LFSRs are only two. In thiscase, during the time interval from 0.W j to 0).1(W j (here 0W is the period of theLFSR s clock-pulses) in LFSR triggers the sequences 11,...,, r j j j a a a A and11,...,, r j j j b b b B are placed. In the adder the numbers, corresponding to thesequences A and B :,2....2.,2....2.111111j j r r j j j r r j b b b b a a a a (1)are summed with carry. Then in the outputs of the adder the total sum b a z isobtained. Here:,1,...,1,,...1,...,1,,,2....2.,,...,,11111111 r j j j i b a b a r j j j i b a z z z z z z z z Z i i i i i i i i i i i j j r r j r j j j V V V V (2)and:-z j is the j th element of combination generator output sequence;-ıi is the carry from the (i-1)th digit.The basic idea of the combining generator can be realized as a shrinkinggenerator also. In the shrinking generator, a control LFSR R 0 is used to select aportion of the output sequence of a second LFSR R 1. Therefore, the producedgamma (or the keystream ) is a shrunken version (also known as an irregularlydecimated subsequence ) of the output sequence of R 1, as depicted in Fig. 2.The algorithm of shrinking generator consists of the following steps:(1) Registers R 0 and R 1 are clocked.(2) If the output of R 0 is 1, the output bit of R 1 forms a part of thekeystream.(3) If the output of R 0 is 0, the output bit of R 1 is discarded.Let R 0 and R 1 be maximum-length LFSRs of lengths L 0 and L 1, respectively, andlet z be an output sequence of the shrinking generator formed by R 0 and R 1. Ifgcd(L 0,L 1) = 1, the z has period (12L – 1). 102 L [7]. The linear complexity L (z )of z satisfies Eq. (3) [7]:1012012.)(2. d L L L z L L (3)Suppose that the connection polynomials of R 0 and R 1 are chosen uniformly atrandom from the set of all primitive polynomials of degrees L 0 and L 1 over Z 2.Then the distribution of patterns in z is almost uniform [7].For maximum security, R 0 and R 1 should be maximum-length LFSRs , and theirlengths should satisfy the condition gcd (L 0,L 1) = 1. Moreover, secret connectionshould be used. Subject to these constraints, if L 0| m and L 1| m , the shrinkinggenerator has a security level approximately equal to 22m . Thus, if L 0| 64 andL 1| 64, the generator appears to be secure against all presently known attacks [5],[7].Fig. 2:Shrinking generatorSECTION 3N-adic Summation-Shrinking Generator ArchitectureIn this section the basic architecture of new N-adic Summation-ShrinkingGenerator (NSumG ) and some basic NSumG properties will be present.The NSumG architecture, proposed recently in [12], uses an increased number ofslaved registers in comparison with Shrinking Generator as in the Shrinking-Multiplexing Generator [11]. The control and slave registers in shrinking-multiplexing generator are replaced with N -adic and 2-adic summation generatorsin the NSumG (fig. 3) respectively. The using of N-adic control summationgenerator enhances the number of the used 2-adic slave summation generatorsfrom 1 in shrinking generator to N 1 in NSumG .Every summation generator consists of two FCSRs , depicted as R j 1y R j 2,()1...,,1,0 N j . It ought to be underlined that slave FCSRs R j 1y R j 2()1...,2,1 N j are 2 FCSRs and hence, the corresponding adders m j consist onebit for m j and one bit for sign. The control FCSRs R 01and R 02 are N -FCSRs andtheir adder m 0 have 1)(0 jN m ind bits for ||0mand an extra bit for sign. clockoutput b i discard b iAs shown, a summation generator selects a portion of the output sequences of several summation generators.Definition 1. The algorithm of the N-adic Summation-Shrinking Generator consists of the following steps:FCSRs from R01y R02 to R N-1 1y R N-1 2 are clocked with clock All(1)sequence with period 0W.(2) If the N-adic output b i = j of the control summation generator is not equal to 0, the output bit of j th slave summation generator forms a part of the keystream. Otherwise, if the output b i = 0 of the control summation generator is equal to 0, the all output bits of slaved summation generators are discarded (fig. 3).Fig. 3: N-adic Summation-Shrinking GeneratorTherefore, the produced keystream is a shrunken and mixed version of the outputsequences 1...,,2,1, N i a ij of the N -1 slaved summation generators.It is straightforward that the N -adic Summation-Shrinking Generator succeeds allpositive features of the summation generator, shrinking generator and N -adicFCSR .The proposed new pseudo random number generator architecture takes advantages of feedback with carry shift registers over )/(N Z for any integerN > 1 (N -FCSRs) (see fig. 4).Definition 2 [13]. Let N > 1 be an integer and }10:{ d d N a a S . For anyinteger 1t r , the state of a feedback with carry shift register over )/(N Z consistof r integers S a a a r 110,,, and arbitrary integer 1 r M M , the memory.The state change function is determined by 1 r integers S d d d g r ,,,,21 ,such that gcd (g ,N ) = 1 and 0z r d as follows (fig. 4):(1) Compute the integer sum r r r r d a d a d a M 022111 V ;(2) Compute S a r ,Z M r such that N M ga r r V ; (3) Change the memory 1 r M to r M ;(4) Output the cell 0a and use the cell r a to shift the register loadingcells, replacing ),,(01a a r by ),,(1a a r .For r n t ,n a is defined by both the memory and the running register cells. In theentire operating ),,,,(21r d d d g are fixed. The following integer r r N d N d N d g d 221 is called the connection number. Consequently,g d 0 and ¦ ri i i N d d 0.Fig. 4:N-adic Feedback with Carry Shift RegisterFor maximum security one must choose the triples of integers ),,(N p d satisfying the next conditions:(1)d is prime; (2)12 p d and p is odd prime; (3) N is prime;(4)N is primitive modulo d and primitive modulo p .In particular case when N = 2 the 2SumSG consists of only one slave 2-adicsummation generator. Let the connection integers of two 2-FCSRs R 01y R 02 ofcontrol summation generator be d 01 and d 02. Let the slave summation generatorcombines two 2-FCSRs R 11y R 12 with connection numbers d 11 and d 12. The period of control summation generator is))1(),1((lcm ))1(),1gcd(()1)(1(0201020102010 d d d d d d T (4) and the period of slave summation generator is))1(),1((lcm ))1(),1gcd(()1)(1(1211121112111 d d d d d d T , (5) according to the [6] and the using of triples ),,(N p d with properties mentionedabove.Then the period 2S of the 2 adic Summation-Shrinking Generator is:),gcd(101*02T T T T S . (6) Here the *0T denotes the total number of ones of the control summation generator.According to [6] the linear complexities 0L and 1L of the summation generators are close to their periods, i.e. ))1)(1gcd(()1)(1(020102010 d d d d L ,))1)(1gcd(()1)(1(121112111 d d d d L .Then from [1] the linear complexity L of the 2SumSG is at most*01.T T L . (7)As one can see from equation (4)y (7), the proposed new architecture ofpseudorandom number generator even with N = 2 allows to produce PRSs withperiod and linear complexity larger than the respective parameters of the PRSsformed by a classic shrinking generator [1].SECTION 4Implementation and output files generationThe N SumSG is software implemented in Visual C++ 6.0 environment for Windows/32 bits. There are used the class p_adic to produce the output N SumSG sequence. The application and N SumSG statistical tests were executed on PC AMD Athlon™ XP 2200+ / 256 MB RAM.Two different setups are applied to generate 1 000 sequences by 1 000 000 bits each to test the N-adic Summation-Shrinking Generator:N = 2. Thereby the N SumSG consists of one controlling 2-adic (1)summation generator with connection integers d01 = 10 000 139 and d02 = 10 000 189. The slave 2-adic summation generator has first connection number d11 = 10 000 229. The second connection number d12 is in every 1 000 000 bits, taking consequently 1 000 values, which are strong 2-primes [9] in the range [81 467, 2 283 803]. So the seed of constructed N SumSG is different at every 1 000 000 bits. The size of generated N SumSG output file is 983 Mbytes.(2) N = 3. In this configuration the controlling 3-adic summation generator gets two connection numbers d01 = 5 000 011 and d02 = 5 000 201. The first slave summation 2-adic generator has a seed comprising the numbers d11 = 10 000 139 and d12 = 10 000 189. The second summation generator has the first connection number d21= 10000229. The second connection number d22 is changed in every 1 000 000 bits, taking consequently 1 000 values, which are strong2-primes in the range [981 467, 2 283 803]. In this way were generated 1 000 sequences by 1 000 000 bits, in which the seed were changed at every 1 000 000 bits. The size of generated N SumSG output file is 983 Mbytes.The connection FCSR numbers were chosen randomly in the two above mention setups.SECTION 5Statistical analysis and interpretation of empirical resultsTo test the randomness of binary sequences generated by N SumSG the so-named NIST suite, proposed by National Institute of Standards and Technology, is used. The NIST suite [7], [10] includes sixteen tests. The tests fix on a variety of different types of non-randomness that could exist in a sequence. These tests are: frequency (monobit), frequency within a block, runs, longest-run-of-ones in a block, binary matrix rank, discrete Fourier transform (spectral), non-overlapping template matching, overlapping template matching, Maurer’s “Universal statistical”, Lempel-Ziv compression, linear complexity, serial, approximate entropy, cumulative sums, random excursions, random excursions variant.The testing process consists of the following steps [7], [10]:(1) State the null hypothesis. Assume that the binary sequence is random.(2) Compute a sequence test statistic. Testing is carried out at the bit level. (3) Compute the p-value, ]1,0[value p .(4) Compare the D to value p . Fix D , where ]01.0,0001.0( D .Successis declared whenever D t value p ; otherwise, failure is declared.Given the empirical results for a particular statistical test, the NIST suitecomputes the proportion of sequences that pass. The range of acceptable proportion is determined using the confidence interval defined as,mp p p )ˆ1(ˆ3ˆ r , where D 1ˆp , and m is the number of binary tested sequences. In our two setups 1000 m . Thus the confidence interval is0094392.099.01000)01.0(99.030.99r r . The proportion should lie above 0.9805607.The distribution of p-values is examined to ensure uniformity. The intervalbetween 0 and 1 is divided into 10 sub-intervals, and the p-values that lie withineach sub-interval are counted. Uniformity may also be specified trough anapplication of a 2F test and the determination of a p-value corresponding to theGoodness-of-Fit Distributional Test on the p-values obtained for an arbitrary statistical test, p-value of the p-values. This is implemented by computing¦ 1012210/)10/(i i m m F F , where i F is the number of p-values in sub-interval i , andm is the number of tested sequences. A p-value is calculated such that )2/,2/9(value -p 2F igamc Ɍ . If 0001.0value -p !Ɍ, then the sequences canbe regarded to be uniformly distributed.Table 1 lists the results from the NIST test suite with input file from the first setup(N = 2). The detailed result of Non-overlapping template matching test, Randomexcursion test and Random excursion – variant test and the numbers of the p-values in the subintervals, when N = 2, can be found in Appendix 1.Table 1: The results from NSumSG statistical tests, when N = 2 Statistical TestResult Proportion P-value T Comment Frequency (monobit)Pass 0.9920 0.260930 Frequency within a blockPass 0.9810 0.896345 Pass 0.9870 0.524101Cumulative sums Pass 0.9910 0.832561Runs Pass 0.9830 0.326749 Longest-run-of-ones in a block Pass 0.9850 0.465415Binary matrix rank Pass 0.9890 0.757790Discrete Fourier transform (spectral) Pass 0.9970 0.186566Non-overlapping template matching Pass 0.9894 0.531028 Avg. valuesStatistical Test Result Proportion P-value T CommentOverlapping template matching Pass 0.9940 0.618385Maurer’s “Universal statistical” Pass 0.9880 0.086634Approximate entropy Pass 0.9890 0.476911Random excursions Pass 0.9870 0.598233 Avg. valuesRandom excursions variant Pass 0.9901 0.431378 Avg. valuesSerialPass 0.9930 0.227180Pass 0.9910 0.849708Lempel-Ziv compression Pass 0.9960 0.037320Linear complexity Pass 0.9960 0.355364The minimum pass rate for theRandom Excursion - (variant) test isapproximately 0.977854.The minimum pass rate for eachstatistical test with the exception ofthe Random Excursion - variant testis approximately = 0.980561.The Table 2 lists the results from the NIST test suite with input file from thesecond setup (N = 3). The detailed result of Non-overlapping template matchingtest, Random excursion test and Random excursion – variant test and the numbersof the p-values in the subintervals, when N = 3, can be found in Appendix 2.Table 2: The results from NSumSG statistical tests, when N = 3Statistical Test Result Proportion P-value T CommentFrequency (monobit) Pass 0.9890 0.881662Frequency within a block Pass 0.9880 0.254411Cumulative sumsPass 0.9820 0.534146Pass 0.9850 0.8272790.4280950.9930Runs PassLongest-run-of-ones in a block Pass 0.9870 0.187581Binary matrix rank Pass 0.9860 0.618385Discrete Fourier transform (spectral) Pass 0.9910 0.647530Non-overlapping template matching Pass 0.9899 0.476221 Avg. valuesOverlapping template matching Pass 0.9900 0.045088Maurer’s “Universal statistical” Pass 0.9850 0.662091Approximate entropy Pass 0.9950 0.508172Random excursions Pass 0.9907 0.476154 Avg. valuesRandom excursions variant Pass 0.9895 0.461205 Avg. valuesSerialPass 0.9880 0.672470Pass 0.9940 0.159910Lempel-Ziv compression Pass 0.9820 0.532132Linear complexity Pass 0.9900 0.869278The minimum pass rate for theRandom Excursion - (variant) test isapproximately 0.978117.The minimum pass rate for eachstatistical test with the exception ofthe Random Excursion - variant testis approximately = 0.980561.CONCLUSIONS AND FUTURE WORKSThe results from statistical analysis show that the sequence generated by NSumSGis uniform, scalable, uncompressible, whit large period; consistent and unpredictable.This gives the reason to consider that the NSumSG as a very interesting pseudorandom generator and it can be useful as a part of stream ciphers.We will be glad to thanks everyone who helps us to make some strong cryptanalysis of NSumSG.References:[1] D. Coppersmith, H. Krawczyk, Y. Mansour, “The Shrinking Generator”,Proceedings of Crypto 93, Springer-Verlag, 1994., pp. 22-39[2] A. Klapper, M. Goresky, “2-adic Shift Register. Fast Software Encryption”,Second International Workshop. (Lecture Notes in Computer Science, vol.950, Springer Verlag, N. Y., 1994.) pp.174-178[3] A. Klapper, J. Xu, “Algebraic Feedback Shift Registers” (submitted toElsevier Preprint), 2003.[4] R. Lidl, H. Niederreiter, “Finite Fields”, Addison – Wesley PublishingCompany, London, England, 1983.[5] P. van Oorshot, A. Menezes, S. Vanstone, “Handbook of AppliedCryptography”, CRC Press, 1997.[6] R. Rueppel, “Analysis and Design of Stream Siphers”, Springer Verlag, N.Y., 1986.[7] A. Rukhin, J. Soto, J. Nechvatal, M. Smid, E. Barker, S. Leigh, M. Levenson,M. Vangel, D. Banks, A. Heckert, J. Dray, S. Vo, “A Statistical Test Suite for Random and Pseudo-Random Number Generators for Cryptographic Application”, NIST Special Publication 800-22 (with revision May 15, 2001) /rng/.[8] B. Schneier, “Applied Cryptography”, John Wiley & Sons, New York, 1996.[9] Ch. Seo, S. Lee, Y. Sung, K. Han, S. Kim, “A Lower Bound on the LinearSpan an FCSR”, IEEE Transaction on Information Theory, Vol. 46, No 2, March 2000.[10] J. Soto, “Statistical Testing of Random Number Generators”,/rng/.[11] Zh. N. Tasheva, B. Y. Bedzhev, V. A. Mutkov, “An Shrinking DataEncryption Algorithm with p-adic Feedback with Carry Shift Register”, XII International Symposium of Theoretical Electrical Engineering ISTET 03, Warsaw, Poland, 6-9 July, 2003., Conference Proceedings, vol.II, pp.397 400.[12] Zh. N. Tasheva, B. Y. Bedzhev, B. P. Stoyanov, “Summation-ShrinkingGenerator”, Conference Proceeding of International Conference “Information Technology and Sequrity ITS – 2004”, June 22-26, 2004, Partenit, Crimea, Ukraine, pp.119-127.[13] Xu, J., “Stream Cipher Analysis Based on FCSRs”, PhD Dissertation,University of Kentucky, 2000.APPENDIX 1Results from setup 1The Uniformity of p-values and the Proportion of passing sequencesC1 C2 C3 C4 C5 C6 C7 C8 C9 C10p-values T Proportion Test113 117 91 111 85 97 86 10096 1040.2609300.9920 frequency91 98 112101 111 96 10198 93 99 0.8963450.9810 block-frequency114 105 96 91 82 95 10797 1061070.5241010.9870 cumulative-sums106 104 10997 88 92 96 94 1081060.8325610.9910 cumulative-sums122 90 108104 99 108 86 92 96 95 0.3267490.9830 runs108 95 94 96 118 94 84 11010398 0.4654150.9850 longest-run109 106 97 95 99 86 10211495 97 0.7577900.9890 rank94 107 109109 121 100 93 99 84 84 0.1865660.9970 fft102 92 99 113 83 92 90 11512193 0.1202070.9900 nonperiodic-templates 108 118 90 95 96 104 95 11196 87 0.4597170.9860 nonperiodic-templates99 95 87 101 106 106 96 10190 1190.5893410.9910 nonperiodic-templates106 112 10196 108 107 10181 85 1030.4317540.9940 nonperiodic-templates104 86 98 101 102 104 12067 1111070.0255350.9840 nonperiodic-templates97 117 10693 79 99 92 10992 1160.1671840.9900 nonperiodic-templates90 92 12196 121 120 87 85 87 1010.0163740.9910 nonperiodic-templates108 133 91 92 89 94 11210186 94 0.0316370.9810 nonperiodic-templates83 109 12299 95 91 10198 98 1040.3619380.9910 nonperiodic-templates89 109 10893 100 106 10510410482 0.6038410.9890 nonperiodic-templates122 91 92 111 89 99 98 10610389 0.3175650.9840 nonperiodic-templates 108 105 83 97 120 88 10194 10797 0.3298500.9860 nonperiodic-templates89 116 10195 105 93 97 99 90 1150.5221000.9930 nonperiodic-templates94 90 11391 93 109 11210110097 0.6683210.9860 nonperiodic-templates90 94 93 115 101 108 10310010096 0.8343080.9920 nonperiodic-templates85 99 106106 100 98 11695 11184 0.3838270.9910 nonperiodic-templates97 101 103111 106 81 96 10111292 0.5728470.9910 nonperiodic-templates107 89 94 95 113 103 10394 10399 0.8644940.9900 nonperiodic-templates 103 111 10196 95 98 78 99 1021170.3889900.9940 nonperiodic-templates99 89 100106 99 90 1061001031080.9311850.9870 nonperiodic-templates99 99 98 84 102 101 1041051041040.9463080.9900 nonperiodic-templates 105 98 97 111 107 97 82 10990 1040.5976200.9860 nonperiodic-templates98 91 79 88 111 102 1071171021050.2355890.9910 nonperiodic-templates94 107 11594 98 109 10510586 87 0.4885340.9910 nonperiodic-templates102 93 99 114 98 98 10896 91 1010.8977630.9910 nonperiodic-templates106 113 92 101 95 111 11289 93 88 0.4616120.9930 nonperiodic-templatesC1 C2 C3 C4 C5 C6 C7 C8 C9 C10p-values T Proportion Test99 115 80 92 104 125 10294 87 1020.0795380.9910 nonperiodic-templates 93 113 89 108 115 90 88 10699 99 0.4280950.9920 nonperiodic-templates 92 104 98 105 91 93 12310992 93 0.3821150.9890 nonperiodic-templates 97 112 102101 113 90 99 10781 98 0.4924360.9910 nonperiodic-templates 94 87 113107 97 109 96 98 96 1030.7811060.9890 nonperiodic-templates 104 98 86 99 94 105 10792 11699 0.6910810.9910 nonperiodic-templates 104 105 98 91 99 90 11190 96 1160.6163050.9890 nonperiodic-templates 102 106 10595 94 94 10710694 97 0.9673820.9930 nonperiodic-templates 103 100 91 103 92 100 96 11210598 0.9400800.9830 nonperiodic-templates 104 107 10793 98 93 98 10579 1160.3994420.9910 nonperiodic-templates 104 87 97 107 98 111 11084 95 1070.5361630.9960 nonperiodic-templates 104 107 10683 100 102 10110491 1020.8377810.9900 nonperiodic-templates 96 96 12384 89 97 1061011061020.3314080.9950 nonperiodic-templates 100 115 98 97 96 103 84 1001071000.7714690.9830 nonperiodic-templates 110 99 106117 85 93 10211288 88 0.2518370.9920 nonperiodic-templates 91 103 10194 96 103 10510393 1110.9379190.9870 nonperiodic-templates 103 74 94 108 99 102 96 99 1161090.2467500.9920 nonperiodic-templates 101 102 86 100 108 100 11210894 89 0.7095580.9850 nonperiodic-templates 105 97 98 100 97 122 95 91 92 1030.6267090.9880 nonperiodic-templates 92 110 10399 95 102 10295 98 1040.9803410.9920 nonperiodic-templates 103 100 102100 90 115 95 90 10798 0.8201430.9860 nonperiodic-templates 104 111 93 104 82 81 11890 1081090.1037530.9810 nonperiodic-templates 105 116 85 89 96 96 10610095 1120.4711460.9840 nonperiodic-templates 91 104 10795 96 90 10610810796 0.8739870.9830 nonperiodic-templates 110 100 106104 107 96 99 98 10377 0.5749030.9890 nonperiodic-templates 125 91 10794 101 111 90 91 10090 0.2167130.9890 nonperiodic-templates 96 93 11294 97 109 91 10195 1120.7538440.9920 nonperiodic-templates 102 100 95 107 106 104 99 10684 97 0.8891180.9870 nonperiodic-templates 104 98 119103 99 94 85 90 1001080.5181060.9930 nonperiodic-templates 95 84 11395 91 101 11398 1081020.5361630.9890 nonperiodic-templates 106 106 90 89 113 105 98 92 98 1030.7714690.9880 nonperiodic-templates 97 101 99 95 110 90 95 93 12397 0.4865880.9900 nonperiodic-templates 101 91 10099 97 104 90 11311392 0.7298700.9910 nonperiodic-templates 110 97 10179 104 105 10011576 1130.0752540.9860 nonperiodic-templates 107 86 105115 91 97 89 10798 1050.5503470.9850 nonperiodic-templates 88 111 102100 94 96 10010211493 0.7695270.9950 nonperiodic-templates 105 111 98 94 94 96 99 89 1081060.8676920.9870 nonperiodic-templates 86 117 99 113 100 96 12094 91 84 0.1075120.9900 nonperiodic-templates 105 107 100112 98 92 95 10794 90 0.8377810.9920 nonperiodic-templates 79 111 97 104 98 100 11310589 1040.4172190.9930 nonperiodic-templates 86 81 112104 115 104 10685 11196 0.1388600.9920 nonperiodic-templates 92 91 10795 114 100 10111489 97 0.5934780.9900 nonperiodic-templates 111 99 99 107 95 97 95 11597 85 0.6475300.9900 nonperiodic-templates 90 117 83 115 96 96 10010792 1040.3011940.9910 nonperiodic-templates 100 94 102105 96 108 92 10391 1090.9240760.9880 nonperiodic-templates 94 121 88 100 105 82 99 10811093 0.2224800.9950 nonperiodic-templates 116 93 120105 91 94 11679 99 87 0.0465680.9890 nonperiodic-templates 106 85 89 100 93 116 10211510094 0.3907210.9870 nonperiodic-templates 102 92 99 114 82 93 89 11711993 0.1031380.9900 nonperiodic-templates 103 95 101127 102 84 10089 92 1070.1825500.9900 nonperiodic-templates。
统计学术语中英文对照详解

统计学术语中英文对照Absolute deviation 绝对离差Absolute number 绝对数Absolute residuals 绝对残差Acceleration array 加速度立体阵Acceleration in an arbitrary direction 任意方向上的加速度Acceleration normal 法向加速度Acceleration space dimension 加速度空间的维数Acceleration tangential 切向加速度Acceleration vector 加速度向量Acceptable hypothesis 可接受假设Accumulation 累积Accuracy 准确度Actual frequency 实际频数Adaptive estimator 自适应估计量Addition 相加Addition theorem 加法定理Additivity 可加性Adjusted rate 调整率Adjusted value 校正值Admissible error 容许误差Aggregation 聚集性Alternative hypothesis 备择假设Among groups 组间Amounts 总量Analysis of correlation 相关分析Analysis of covariance 协方差分析Analysis of regression 回归分析Analysis of time series 时间序列分析Analysis of variance 方差分析Angular transformation 角转换ANOVA (analysis of variance)方差分析ANOVA Models 方差分析模型Arcing 弧/弧旋Arcsine transformation 反正弦变换Area under the curve 曲线面积AREG 评估从一个时间点到下一个时间点回归相关时的误差ARIMA 季节和非季节性单变量模型的极大似然估计Arithmetic grid paper 算术格纸Arithmetic mean 算术平均数Arrhenius relation 艾恩尼斯关系Assessing fit 拟合的评估Associative laws 结合律Asymmetric distribution 非对称分布Asymptotic bias 渐近偏倚Asymptotic efficiency 渐近效率Asymptotic variance 渐近方差Attributable risk 归因危险度Attribute data 属性资料Attribution 属性Autocorrelation 自相关Autocorrelation of residuals 残差的自相关Average 平均数Average confidence interval length 平均置信区间长度Average growth rate 平均增长率Bar chart 条形图Bar graph 条形图Base period 基期Bayes' theorem Bayes定理Bell—shaped curve 钟形曲线Bernoulli distribution 伯努力分布Best—trim estimator 最好切尾估计量Bias 偏性Binary logistic regression 二元逻辑斯蒂回归Binomial distribution 二项分布Bisquare 双平方Bivariate Correlate 二变量相关Bivariate normal distribution 双变量正态分布Bivariate normal population 双变量正态总体Biweight interval 双权区间Biweight M—estimator 双权M估计量Block 区组/配伍组BMDP(Biomedical computer programs)BMDP统计软件包Boxplots 箱线图/箱尾图Breakdown bound 崩溃界/崩溃点Canonical correlation 典型相关Caption 纵标目Case-control study 病例对照研究Categorical variable 分类变量Catenary 悬链线Cauchy distribution 柯西分布Cause-and-effect relationship 因果关系Cell 单元Censoring 终检Center of symmetry 对称中心Centering and scaling 中心化和定标Central tendency 集中趋势Central value 中心值CHAID —χ2 Automatic Interaction Detector 卡方自动交互检测Chance 机遇Chance error 随机误差Chance variable 随机变量Characteristic equation 特征方程Characteristic root 特征根Characteristic vector 特征向量Chebshev criterion of fit 拟合的切比雪夫准则Chernoff faces 切尔诺夫脸谱图Chi—square test 卡方检验/χ2检验Choleskey decomposition 乔洛斯基分解Circle chart 圆图Class interval 组距Class mid-value 组中值Class upper limit 组上限Classified variable 分类变量Cluster analysis 聚类分析Cluster sampling 整群抽样Code 代码Coded data 编码数据Coding 编码Coefficient of contingency 列联系数Coefficient of determination 决定系数Coefficient of multiple correlation 多重相关系数Coefficient of partial correlation 偏相关系数Coefficient of production-moment correlation 积差相关系数Coefficient of rank correlation 等级相关系数Coefficient of regression 回归系数Coefficient of skewness 偏度系数Coefficient of variation 变异系数Cohort study 队列研究Column 列Column effect 列效应Column factor 列因素Combination pool 合并Combinative table 组合表Common factor 共性因子Common regression coefficient 公共回归系数Common value 共同值Common variance 公共方差Common variation 公共变异Communality variance 共性方差Comparability 可比性Comparison of bathes 批比较Comparison value 比较值Compartment model 分部模型Compassion 伸缩Complement of an event 补事件Complete association 完全正相关Complete dissociation 完全不相关Complete statistics 完备统计量Completely randomized design 完全随机化设计Composite event 联合事件Composite events 复合事件Concavity 凹性Conditional expectation 条件期望Conditional likelihood 条件似然Conditional probability 条件概率Conditionally linear 依条件线性Confidence interval 置信区间Confidence limit 置信限Confidence lower limit 置信下限Confidence upper limit 置信上限Confirmatory Factor Analysis 验证性因子分析Confirmatory research 证实性实验研究Confounding factor 混杂因素Conjoint 联合分析Consistency 相合性Consistency check 一致性检验Consistent asymptotically normal estimate 相合渐近正态估计Consistent estimate 相合估计Constrained nonlinear regression 受约束非线性回归Constraint 约束Contaminated distribution 污染分布Contaminated Gausssian 污染高斯分布Contaminated normal distribution 污染正态分布Contamination 污染Contamination model 污染模型Contingency table 列联表Contour 边界线Contribution rate 贡献率Control 对照Controlled experiments 对照实验Conventional depth 常规深度Convolution 卷积Corrected factor 校正因子Corrected mean 校正均值Correction coefficient 校正系数Correctness 正确性Correlation coefficient 相关系数Correlation index 相关指数Correspondence 对应Counting 计数Counts 计数/频数Covariance 协方差Covariant 共变Cox Regression Cox回归Criteria for fitting 拟合准则Criteria of least squares 最小二乘准则Critical ratio 临界比Critical region 拒绝域Critical value 临界值Cross—over design 交叉设计Cross—section analysis 横断面分析Cross—section survey 横断面调查Crosstabs 交叉表Cross-tabulation table 复合表Cube root 立方根Cumulative distribution function 分布函数Cumulative probability 累计概率Curvature 曲率/弯曲Curvature 曲率Curve fit 曲线拟和Curve fitting 曲线拟合Curvilinear regression 曲线回归Curvilinear relation 曲线关系Cut-and-try method 尝试法Cycle 周期Cyclist 周期性D test D检验Data acquisition 资料收集Data bank 数据库Data capacity 数据容量Data deficiencies 数据缺乏Data handling 数据处理Data manipulation 数据处理Data processing 数据处理Data reduction 数据缩减Data set 数据集Data sources 数据来源Data transformation 数据变换Data validity 数据有效性Data—in 数据输入Data—out 数据输出Dead time 停滞期Degree of freedom 自由度Degree of precision 精密度Degree of reliability 可靠性程度Degression 递减Density function 密度函数Density of data points 数据点的密度Dependent variable 应变量/依变量/因变量Dependent variable 因变量Depth 深度Derivative matrix 导数矩阵Derivative—free methods 无导数方法Design 设计Determinacy 确定性Determinant 行列式Determinant 决定因素Deviation 离差Deviation from average 离均差Diagnostic plot 诊断图Dichotomous variable 二分变量Differential equation 微分方程Direct standardization 直接标准化法Discrete variable 离散型变量DISCRIMINANT 判断Discriminant analysis 判别分析Discriminant coefficient 判别系数Discriminant function 判别值Dispersion 散布/分散度Disproportional 不成比例的Disproportionate sub—class numbers 不成比例次级组含量Distribution free 分布无关性/免分布Distribution shape 分布形状Distribution—free method 任意分布法Distributive laws 分配律Disturbance 随机扰动项Dose response curve 剂量反应曲线Double blind method 双盲法Double blind trial 双盲试验Double exponential distribution 双指数分布Double logarithmic 双对数Downward rank 降秩Dual-space plot 对偶空间图DUD 无导数方法Duncan's new multiple range method 新复极差法/Duncan新法Effect 实验效应Eigenvalue 特征值Eigenvector 特征向量Ellipse 椭圆Empirical distribution 经验分布Empirical probability 经验概率单位Enumeration data 计数资料Equal sun-class number 相等次级组含量Equally likely 等可能Equivariance 同变性Error 误差/错误Error of estimate 估计误差Error type I 第一类错误Error type II 第二类错误Estimand 被估量Estimated error mean squares 估计误差均方Estimated error sum of squares 估计误差平方和Euclidean distance 欧式距离Event 事件Event 事件Exceptional data point 异常数据点Expectation plane 期望平面Expectation surface 期望曲面Expected values 期望值Experiment 实验Experimental sampling 试验抽样Experimental unit 试验单位Explanatory variable 说明变量Exploratory data analysis 探索性数据分析Explore Summarize 探索-摘要Exponential curve 指数曲线Exponential growth 指数式增长EXSMOOTH 指数平滑方法Extended fit 扩充拟合Extra parameter 附加参数Extrapolation 外推法Extreme observation 末端观测值Extremes 极端值/极值F distribution F分布F test F检验Factor 因素/因子Factor analysis 因子分析Factor Analysis 因子分析Factor score 因子得分Factorial 阶乘Factorial design 析因试验设计False negative 假阴性False negative error 假阴性错误Family of distributions 分布族Family of estimators 估计量族Fanning 扇面Fatality rate 病死率Field investigation 现场调查Field survey 现场调查Finite population 有限总体Finite-sample 有限样本First derivative 一阶导数First principal component 第一主成分First quartile 第一四分位数Fisher information 费雪信息量Fitted value 拟合值Fitting a curve 曲线拟合Fixed base 定基Fluctuation 随机起伏Forecast 预测Four fold table 四格表Fourth 四分点Fraction blow 左侧比率Fractional error 相对误差Frequency 频率Frequency polygon 频数多边图Frontier point 界限点Function relationship 泛函关系Gamma distribution 伽玛分布Gauss increment 高斯增量Gaussian distribution 高斯分布/正态分布Gauss-Newton increment 高斯-牛顿增量General census 全面普查GENLOG (Generalized liner models)广义线性模型Geometric mean 几何平均数Gini’s mean difference 基尼均差GLM (General liner models) 通用线性模型Goodness of fit 拟和优度/配合度Gradient of determinant 行列式的梯度Graeco-Latin square 希腊拉丁方Grand mean 总均值Gross errors 重大错误Gross-error sensitivity 大错敏感度Group averages 分组平均Grouped data 分组资料Guessed mean 假定平均数Half-life 半衰期Hampel M-estimators 汉佩尔M估计量Happenstance 偶然事件Harmonic mean 调和均数Hazard function 风险均数Hazard rate 风险率Heading 标目Heavy-tailed distribution 重尾分布Hessian array 海森立体阵Heterogeneity 不同质Heterogeneity of variance 方差不齐Hierarchical classification 组内分组Hierarchical clustering method 系统聚类法High—leverage point 高杠杆率点HILOGLINEAR 多维列联表的层次对数线性模型Hinge 折叶点Histogram 直方图Historical cohort study 历史性队列研究Holes 空洞HOMALS 多重响应分析Homogeneity of variance 方差齐性Homogeneity test 齐性检验Huber M-estimators 休伯M估计量Hyperbola 双曲线Hypothesis testing 假设检验Hypothetical universe 假设总体Impossible event 不可能事件Independence 独立性Independent variable 自变量Index 指标/指数Indirect standardization 间接标准化法Individual 个体Inference band 推断带Infinite population 无限总体Infinitely great 无穷大Infinitely small 无穷小Influence curve 影响曲线Information capacity 信息容量Initial condition 初始条件Initial estimate 初始估计值Initial level 最初水平Interaction 交互作用Interaction terms 交互作用项Intercept 截距Interpolation 内插法Interquartile range 四分位距Interval estimation 区间估计Intervals of equal probability 等概率区间Intrinsic curvature 固有曲率Invariance 不变性Inverse matrix 逆矩阵Inverse probability 逆概率Inverse sine transformation 反正弦变换Iteration 迭代Jacobian determinant 雅可比行列式Joint distribution function 分布函数Joint probability 联合概率Joint probability distribution 联合概率分布K means method 逐步聚类法Kaplan-Meier 评估事件的时间长度Kaplan—Merier chart Kaplan-Merier图Kendall's rank correlation Kendall等级相关Kinetic 动力学Kolmogorov-Smirnove test 柯尔莫哥洛夫—斯米尔诺夫检验Kruskal and Wallis test Kruskal及Wallis检验/多样本的秩和检验/H检验Kurtosis 峰度Lack of fit 失拟Ladder of powers 幂阶梯Lag 滞后Large sample 大样本Large sample test 大样本检验Latin square 拉丁方Latin square design 拉丁方设计Leakage 泄漏Least favorable configuration 最不利构形Least favorable distribution 最不利分布Least significant difference 最小显著差法Least square method 最小二乘法Least—absolute-residuals estimates 最小绝对残差估计Least-absolute-residuals fit 最小绝对残差拟合Least—absolute—residuals line 最小绝对残差线Legend 图例L-estimator L估计量L-estimator of location 位置L估计量L—estimator of scale 尺度L估计量Level 水平Life expectance 预期期望寿命Life table 寿命表Life table method 生命表法Light—tailed distribution 轻尾分布Likelihood function 似然函数Likelihood ratio 似然比line graph 线图Linear correlation 直线相关Linear equation 线性方程Linear programming 线性规划Linear regression 直线回归Linear Regression 线性回归Linear trend 线性趋势Loading 载荷Location and scale equivariance 位置尺度同变性Location equivariance 位置同变性Location invariance 位置不变性Location scale family 位置尺度族Log rank test 时序检验Logarithmic curve 对数曲线Logarithmic normal distribution 对数正态分布Logarithmic scale 对数尺度Logarithmic transformation 对数变换Logic check 逻辑检查Logistic distribution 逻辑斯特分布Logit transformation Logit转换LOGLINEAR 多维列联表通用模型Lognormal distribution 对数正态分布Lost function 损失函数Low correlation 低度相关Lower limit 下限Lowest—attained variance 最小可达方差LSD 最小显著差法的简称Lurking variable 潜在变量Main effect 主效应Major heading 主辞标目Marginal density function 边缘密度函数Marginal probability 边缘概率Marginal probability distribution 边缘概率分布Matched data 配对资料Matched distribution 匹配过分布Matching of distribution 分布的匹配Matching of transformation 变换的匹配Mathematical expectation 数学期望Mathematical model 数学模型Maximum L-estimator 极大极小L 估计量Maximum likelihood method 最大似然法Mean 均数Mean squares between groups 组间均方Mean squares within group 组内均方Means (Compare means) 均值—均值比较Median 中位数Median effective dose 半数效量Median lethal dose 半数致死量Median polish 中位数平滑Median test 中位数检验Minimal sufficient statistic 最小充分统计量Minimum distance estimation 最小距离估计Minimum effective dose 最小有效量Minimum lethal dose 最小致死量Minimum variance estimator 最小方差估计量MINITAB 统计软件包Minor heading 宾词标目Missing data 缺失值Model specification 模型的确定Modeling Statistics 模型统计Models for outliers 离群值模型Modifying the model 模型的修正Modulus of continuity 连续性模Morbidity 发病率Most favorable configuration 最有利构形Multidimensional Scaling (ASCAL)多维尺度/多维标度Multinomial Logistic Regression 多项逻辑斯蒂回归Multiple comparison 多重比较Multiple correlation 复相关Multiple covariance 多元协方差Multiple linear regression 多元线性回归Multiple response 多重选项Multiple solutions 多解Multiplication theorem 乘法定理Multiresponse 多元响应Multi—stage sampling 多阶段抽样Multivariate T distribution 多元T分布Mutual exclusive 互不相容Mutual independence 互相独立Natural boundary 自然边界Natural dead 自然死亡Natural zero 自然零Negative correlation 负相关Negative linear correlation 负线性相关Negatively skewed 负偏Newman—Keuls method q检验NK method q检验No statistical significance 无统计意义Nominal variable 名义变量Nonconstancy of variability 变异的非定常性Nonlinear regression 非线性相关Nonparametric statistics 非参数统计Nonparametric test 非参数检验Nonparametric tests 非参数检验Normal deviate 正态离差Normal distribution 正态分布Normal equation 正规方程组Normal ranges 正常范围Normal value 正常值Nuisance parameter 多余参数/讨厌参数Null hypothesis 无效假设Numerical variable 数值变量Objective function 目标函数Observation unit 观察单位Observed value 观察值One sided test 单侧检验One-way analysis of variance 单因素方差分析Oneway ANOVA 单因素方差分析Open sequential trial 开放型序贯设计Optrim 优切尾Optrim efficiency 优切尾效率Order statistics 顺序统计量Ordered categories 有序分类Ordinal logistic regression 序数逻辑斯蒂回归Ordinal variable 有序变量Orthogonal basis 正交基Orthogonal design 正交试验设计Orthogonality conditions 正交条件ORTHOPLAN 正交设计Outlier cutoffs 离群值截断点Outliers 极端值OVERALS 多组变量的非线性正规相关Overshoot 迭代过度Paired design 配对设计Paired sample 配对样本Pairwise slopes 成对斜率Parabola 抛物线Parallel tests 平行试验Parameter 参数Parametric statistics 参数统计Parametric test 参数检验Partial correlation 偏相关Partial regression 偏回归Partial sorting 偏排序Partials residuals 偏残差Pattern 模式Pearson curves 皮尔逊曲线Peeling 退层Percent bar graph 百分条形图Percentage 百分比Percentile 百分位数Percentile curves 百分位曲线Periodicity 周期性Permutation 排列P-estimator P估计量Pie graph 饼图Pitman estimator 皮特曼估计量Pivot 枢轴量Planar 平坦Planar assumption 平面的假设PLANCARDS 生成试验的计划卡Point estimation 点估计Poisson distribution 泊松分布Polishing 平滑Polled standard deviation 合并标准差Polled variance 合并方差Polygon 多边图Polynomial 多项式Polynomial curve 多项式曲线Population 总体Population attributable risk 人群归因危险度Positive correlation 正相关Positively skewed 正偏Posterior distribution 后验分布Power of a test 检验效能Precision 精密度Predicted value 预测值Preliminary analysis 预备性分析Principal component analysis 主成分分析Prior distribution 先验分布Prior probability 先验概率Probabilistic model 概率模型probability 概率Probability density 概率密度Product moment 乘积矩/协方差Profile trace 截面迹图Proportion 比/构成比Proportion allocation in stratified random sampling 按比例分层随机抽样Proportionate 成比例Proportionate sub-class numbers 成比例次级组含量Prospective study 前瞻性调查Proximities 亲近性Pseudo F test 近似F检验Pseudo model 近似模型Pseudosigma 伪标准差Purposive sampling 有目的抽样QR decomposition QR分解Quadratic approximation 二次近似Qualitative classification 属性分类Qualitative method 定性方法Quantile-quantile plot 分位数-分位数图/Q—Q图Quantitative analysis 定量分析Quartile 四分位数Quick Cluster 快速聚类Radix sort 基数排序Random allocation 随机化分组Random blocks design 随机区组设计Random event 随机事件Randomization 随机化Range 极差/全距Rank correlation 等级相关Rank sum test 秩和检验Rank test 秩检验Ranked data 等级资料Rate 比率Ratio 比例Raw data 原始资料Raw residual 原始残差Rayleigh’s test 雷氏检验Rayleigh's Z 雷氏Z值Reciprocal 倒数Reciprocal transformation 倒数变换Recording 记录Redescending estimators 回降估计量Reducing dimensions 降维Re—expression 重新表达Reference set 标准组Region of acceptance 接受域Regression coefficient 回归系数Regression sum of square 回归平方和Rejection point 拒绝点Relative dispersion 相对离散度Relative number 相对数Reliability 可靠性Reparametrization 重新设置参数Replication 重复Report Summaries 报告摘要Residual sum of square 剩余平方和Resistance 耐抗性Resistant line 耐抗线Resistant technique 耐抗技术R—estimator of location 位置R估计量R-estimator of scale 尺度R估计量Retrospective study 回顾性调查Ridge trace 岭迹Ridit analysis Ridit分析Rotation 旋转Rounding 舍入Row 行Row effects 行效应Row factor 行因素RXC table RXC表Sample 样本Sample regression coefficient 样本回归系数Sample size 样本量Sample standard deviation 样本标准差Sampling error 抽样误差SAS(Statistical analysis system ) SAS统计软件包Scale 尺度/量表Scatter diagram 散点图Schematic plot 示意图/简图Score test 计分检验Screening 筛检SEASON 季节分析Second derivative 二阶导数Second principal component 第二主成分SEM (Structural equation modeling) 结构化方程模型Semi-logarithmic graph 半对数图Semi—logarithmic paper 半对数格纸Sensitivity curve 敏感度曲线Sequential analysis 贯序分析Sequential data set 顺序数据集Sequential design 贯序设计Sequential method 贯序法Sequential test 贯序检验法Serial tests 系列试验Short-cut method 简捷法Sigmoid curve S形曲线Sign function 正负号函数Sign test 符号检验Signed rank 符号秩Significance test 显著性检验Significant figure 有效数字Simple cluster sampling 简单整群抽样Simple correlation 简单相关Simple random sampling 简单随机抽样Simple regression 简单回归simple table 简单表Sine estimator 正弦估计量Single-valued estimate 单值估计Singular matrix 奇异矩阵Skewed distribution 偏斜分布Skewness 偏度Slash distribution 斜线分布Slope 斜率Smirnov test 斯米尔诺夫检验Source of variation 变异来源Spearman rank correlation 斯皮尔曼等级相关Specific factor 特殊因子Specific factor variance 特殊因子方差Spectra 频谱Spherical distribution 球型正态分布Spread 展布SPSS(Statistical package for the social science)SPSS统计软件包Spurious correlation 假性相关Square root transformation 平方根变换Stabilizing variance 稳定方差Standard deviation 标准差Standard error 标准误Standard error of difference 差别的标准误Standard error of estimate 标准估计误差Standard error of rate 率的标准误Standard normal distribution 标准正态分布Standardization 标准化Starting value 起始值Statistic 统计量Statistical control 统计控制Statistical graph 统计图Statistical inference 统计推断Statistical table 统计表Steepest descent 最速下降法Stem and leaf display 茎叶图Step factor 步长因子Stepwise regression 逐步回归Storage 存Strata 层(复数)Stratified sampling 分层抽样Stratified sampling 分层抽样Strength 强度Stringency 严密性Structural relationship 结构关系Studentized residual 学生化残差/t化残差Sub—class numbers 次级组含量Subdividing 分割Sufficient statistic 充分统计量Sum of products 积和Sum of squares 离差平方和Sum of squares about regression 回归平方和Sum of squares between groups 组间平方和Sum of squares of partial regression 偏回归平方和Sure event 必然事件Survey 调查Survival 生存分析Survival rate 生存率Suspended root gram 悬吊根图Symmetry 对称Systematic error 系统误差Systematic sampling 系统抽样Tags 标签Tail area 尾部面积Tail length 尾长Tail weight 尾重Tangent line 切线Target distribution 目标分布Taylor series 泰勒级数Tendency of dispersion 离散趋势Testing of hypotheses 假设检验Theoretical frequency 理论频数Time series 时间序列Tolerance interval 容忍区间Tolerance lower limit 容忍下限Tolerance upper limit 容忍上限Torsion 扰率Total sum of square 总平方和Total variation 总变异Transformation 转换Treatment 处理Trend 趋势Trend of percentage 百分比趋势Trial 试验Trial and error method 试错法Tuning constant 细调常数Two sided test 双向检验Two-stage least squares 二阶最小平方Two-stage sampling 二阶段抽样Two-tailed test 双侧检验Two—way analysis of variance 双因素方差分析Two—way table 双向表Type I error 一类错误/α错误Type II error 二类错误/β错误UMVU 方差一致最小无偏估计简称Unbiased estimate 无偏估计Unconstrained nonlinear regression 无约束非线性回归Unequal subclass number 不等次级组含量Ungrouped data 不分组资料Uniform coordinate 均匀坐标Uniform distribution 均匀分布Uniformly minimum variance unbiased estimate 方差一致最小无偏估计Unit 单元Unordered categories 无序分类Upper limit 上限Upward rank 升秩Vague concept 模糊概念Validity 有效性VARCOMP (Variance component estimation)方差元素估计Variability 变异性Variable 变量Variance 方差Variation 变异Varimax orthogonal rotation 方差最大正交旋转Volume of distribution 容积W test W检验Weibull distribution 威布尔分布Weight 权数Weighted Chi-square test 加权卡方检验/Cochran检验Weighted linear regression method 加权直线回归Weighted mean 加权平均数Weighted mean square 加权平均方差Weighted sum of square 加权平方和Weighting coefficient 权重系数Weighting method 加权法W-estimation W估计量W-estimation of location 位置W估计量Width 宽度Wilcoxon paired test 威斯康星配对法/配对符号秩和检验Wild point 野点/狂点Wild value 野值/狂值Winsorized mean 缩尾均值Withdraw 失访Youden’s index 尤登指数Z test Z检验Zero correlation 零相关Z-transformation Z变换。
统计学专业名词(中英对照)
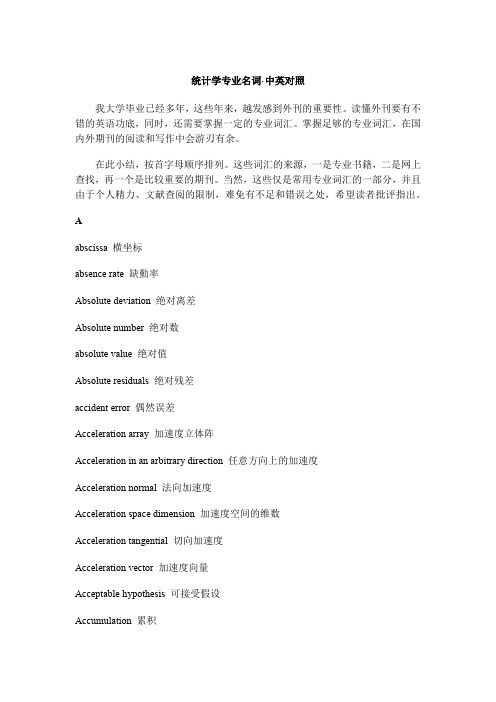
统计学专业名词·中英对照我大学毕业已经多年,这些年来,越发感到外刊的重要性。
读懂外刊要有不错的英语功底,同时,还需要掌握一定的专业词汇。
掌握足够的专业词汇,在国内外期刊的阅读和写作中会游刃有余。
在此小结,按首字母顺序排列。
这些词汇的来源,一是专业书籍,二是网上查找,再一个是比较重要的期刊。
当然,这些仅是常用专业词汇的一部分,并且由于个人精力、文献查阅的限制,难免有不足和错误之处,希望读者批评指出。
Aabscissa 横坐标absence rate 缺勤率Absolute deviation 绝对离差Absolute number 绝对数absolute value 绝对值Absolute residuals 绝对残差accident error 偶然误差Acceleration array 加速度立体阵Acceleration in an arbitrary direction 任意方向上的加速度Acceleration normal 法向加速度Acceleration space dimension 加速度空间的维数Acceleration tangential 切向加速度Acceleration vector 加速度向量Acceptable hypothesis 可接受假设Accumulation 累积Accumulated frequency 累积频数Accuracy 准确度Actual frequency 实际频数Adaptive estimator 自适应估计量Addition 相加Addition theorem 加法定理Additive Noise 加性噪声Additivity 可加性Adjusted rate 调整率Adjusted value 校正值Admissible error 容许误差Aggregation 聚集性Alpha factori ng α因子法Alternative hypothesis 备择假设Among groups 组间Amounts 总量Analysis of correlation 相关分析Analysis of covariance 协方差分析Analysis of data 分析资料Analysis Of Effects 效应分析Analysis Of Variance 方差分析Analysis of regression 回归分析Analysis of time series 时间序列分析Analysis of variance 方差分析Angular transformation 角转换ANOVA (analysis of variance)方差分析ANOVA Models 方差分析模型ANOVA table and eta 分组计算方差分析Arcing 弧/弧旋Arcsine transformation 反正弦变换Area 区域图Area under the curve 曲线面积AREG 评估从一个时间点到下一个时间点回归相关时的误差ARIMA 季节和非季节性单变量模型的极大似然估计Arithmetic grid paper 算术格纸Arithmetic mean 算术平均数Arithmetic weighted mean 加权算术均数Arrhenius relation 艾恩尼斯关系Assessing fit 拟合的评估Associative laws 结合律Assumed mean 假定均数Asymmetric distribution 非对称分布Asymmetry coefficient 偏度系数Asymptotic bias 渐近偏倚Asymptotic efficiency 渐近效率Asymptotic variance 渐近方差Attributable risk 归因危险度Attribute data 属性资料Attribution 属性Autocorrelation 自相关Autocorrelation of residuals 残差的自相关Average 平均数Average confidence interval length 平均置信区间长度average deviation 平均差Average growth rate 平均增长率BBar chart/graph 条形图Base period 基期Bayes' theorem Bayes 定理Bell-shaped curve 钟形曲线Bernoulli distribution 伯努力分布Best-trim estimator 最好切尾估计量Bias 偏性Biometrics 生物统计学Binary logistic regression 二元逻辑斯蒂回归Binomial distribution 二项分布Bisquare 双平方Bivariate Correlate 二变量相关Bivariate normal distribution 双变量正态分布Bivariate normal population 双变量正态总体Biweight interval 双权区间Biweight M-estimator 双权M 估计量Block 区组/配伍组BMDP(Biomedical computer programs) BMDP 统计软件包Box plot 箱线图/箱尾图Breakdown bound 崩溃界/崩溃点CCanonical correlation 典型相关Caption 纵标目Cartogram 统计图Case fatality rate 病死率Case-control study 病例对照研究Categorical variable 分类变量Catenary 悬链线Cauchy distribution 柯西分布Cause-and-effect relationship 因果关系Cell 单元Censoring 终检census 普查Center of symmetry 对称中心Centering and scaling 中心化和定标Central tendency 集中趋势Central value 中心值CHAID -χ2 Automatic Interaction Detector 卡方自动交互检测Chance 机遇Chance error 随机误差Chance variable 随机变量Characteristic equation 特征方程Characteristic root 特征根Characteristic vector 特征向量Chebshev criterion of fit 拟合的切比雪夫准则Chernoff faces 切尔诺夫脸谱图chi-sguare(X2) test 卡方检验卡方检验/χ2 检验Choleskey decomposition 乔洛斯基分解Circle chart 圆图Class interval 组距Classification 分组、分类Class mid-value 组中值Class upper limit 组上限Classified variable 分类变量Cluster analysis 聚类分析Cluster sampling 整群抽样Code 代码Coded data 编码数据Coding 编码Coefficient of contingency 列联系数Coefficient of correlation 相关系数Coefficient of determination 决定系数Coefficient of multiple correlation 多重相关系数Coefficient of partial correlation 偏相关系数Coefficient of production-moment correlation 积差相关系数Coefficient of rank correlation 等级相关系数Coefficient of regression 回归系数Coefficient of skewness 偏度系数Coefficient of variation 变异系数Cohort study 队列研究Collection of data 资料收集Collinearity 共线性Column 列Column effect 列效应Column factor 列因素Combination pool 合并Combinative table 组合表Combined standard deviation 合并标准差Combined variance 合并方差Common factor 共性因子Common regression coefficient 公共回归系数Common value 共同值Common variance 公共方差Common variation 公共变异Communality variance 共性方差Comparability 可比性Comparison of bathes 批比较Comparison value 比较值Compartment model 分部模型Compassion 伸缩Complement of an event 补事件Complete association 完全正相关Complete dissociation 完全不相关Complete statistics 完备统计量Complete survey 全面调查Completely randomized design 完全随机化设计Composite event 联合事件Composite events 复合事件Concavity 凹性Conditional expectation 条件期望Conditional likelihood 条件似然Conditional probability 条件概率Conditionally linear 依条件线性Confidence interval 置信区间Confidence level 可信水平,置信水平Confidence limit 置信限Confidence lower limit 置信下限Confidence upper limit 置信上限Confirmatory Factor Analysis 验证性因子分析Confirmatory research 证实性实验研究Confounding factor 混杂因素Conjoint 联合分析Consistency 相合性Consistency check 一致性检验Consistent asymptotically normal estimate 相合渐近正态估计Consistent estimate 相合估计Constituent ratio 构成比,结构相对数Constrained nonlinear regression 受约束非线性回归Constraint 约束Contaminated distribution 污染分布Contaminated Gausssian 污染高斯分布Contaminated normal distribution 污染正态分布Contamination 污染Contamination model 污染模型Continuity 连续性Contingency table 列联表Contour 边界线Contribution rate 贡献率Control 对照质量控制图Control group 对照组Controlled experiments 对照实验Conventional depth 常规深度Convolution 卷积Coordinate 坐标Corrected factor 校正因子Corrected mean 校正均值Correction coefficient 校正系数Correction for continuity 连续性校正Correction for grouping 归组校正Correction number 校正数Correction value 校正值Correctness 正确性Correlation 相关,联系Correlation analysis 相关分析Correlation coefficient 相关系数Correlation 相关性Correlation index 相关指数Correspondence 对应Counting 计数Counts 计数/频数Covariance 协方差Covariant 共变Cox Regression Cox 回归Criteria for fitting 拟合准则Criteria of least squares 最小二乘准则Critical ratio 临界比Critical region 拒绝域Critical value 临界值Cross-over design 交叉设计Cross-section analysis 横断面分析Cross-section survey 横断面调查Crosstabs 交叉表Crosstabs 列联表分析Cross-tabulation table 复合表Cube root 立方根Cumulative distribution function 分布函数Cumulative frequency 累积频率Cumulative probability 累计概率Curvature 曲率/弯曲Curvature 曲率Curve Estimation 曲线拟合Curve fit 曲线拟和Curve fitting 曲线拟合Curvilinear regression 曲线回归Curvilinear relation 曲线关系Cut-and-try method 尝试法Cycle 周期Cyclist 周期性DD test D 检验data 资料Data acquisition 资料收集Data bank 数据库Data capacity 数据容量Data deficiencies 数据缺乏Data handling 数据处理Data manipulation 数据处理Data processing 数据处理Data reduction 数据缩减Data set 数据集Data sources 数据来源Data transformation 数据变换Data validity 数据有效性Data-in 数据输入Data-out 数据输出Dead time 停滞期Degree of freedom 自由度degree of confidence 可信度,置信度degree of dispersion 离散程度Degree of precision 精密度Degree of reliability 可靠性程度degree of variation 变异度Degression 递减Density function 密度函数Density of data points 数据点的密度Dependent variableDepth 深度Derivative matrix 导数矩阵Derivative-free methods 无导数方法Design 设计design of experiment 实验设计Determinacy 确定性Determinant 行列式Determinant 决定因素Deviation 离差Deviation from average 离均差diagnose accordance rate 诊断符合率Diagnostic plot 诊断图Dichotomous variable 二分变量Differential equation 微分方程Direct standardization 直接标准化法Direct Oblimin 斜交旋转Discrete variable 离散型变量DISCRIMINANT 判断Discriminant analysis 判别分析Discriminant coefficient 判别系数Discriminant function 判别值Dispersion 散布/分散度Disproportional 不成比例的Disproportionate sub-class numbers 不成比例次级组含量Distribution free 分布无关性/免分布Distribution shape 分布形状Distribution-free method 任意分布法Distributive laws 分配律Disturbance 随机扰动项Dose response curve 剂量反应曲线Double blind method 双盲法Double blind trial 双盲试验Double exponential distribution 双指数分布Double logarithmic 双对数Downward rank 降秩Dual-space plot 对偶空间图DUD 无导数方法Duncan's new multiple range method 新复极差法/Duncan 新法EError Bar 均值相关区间图Effect 实验效应Effective rate 有效率Eigenvalue 特征值Eigenvector 特征向量Ellipse 椭圆Empirical distribution 经验分布Empirical probability 经验概率单位Enumeration data 计数资料Equal sun-class number 相等次级组含量Equally likely 等可能Equation of linear regression 线性回归方程Equivariance 同变性Error 误差/错误Error of estimate 估计误差Error of replication 重复误差Error type I 第一类错误Error type II 第二类错误Estimand 被估量Estimated error mean squares 估计误差均方Estimated error sum of squares 估计误差平方和Euclidean distance 欧式距离Event 事件Exceptional data point 异常数据点Expectation plane 期望平面Expectation surface 期望曲面Expected values 期望值Experiment 实验Experiment design 实验设计Experiment error 实验误差Experimental group 实验组Experimental sampling 试验抽样Experimental unit 试验单位Explained variance (已说明方差)Explanatory variable 说明变量Exploratory data analysis 探索性数据分析Explore Summarize 探索-摘要Exponential curve 指数曲线Exponential growth 指数式增长EXSMOOTH 指数平滑方法Extended fit 扩充拟合Extra parameter 附加参数Extrapolation 外推法Extreme observation 末端观测值Extremes 极端值/极值FF distribution F 分布F test F 检验Factor 因素/因子Factor analysis 因子分析Factor Analysis 因子分析Factor score 因子得分Factorial 阶乘Factorial design 析因试验设计False negative 假阴性False negative error 假阴性错误Family of distributions 分布族Family of estimators 估计量族Fanning 扇面Fatality rate 病死率Field investigation 现场调查Field survey 现场调查Finite population 有限总体Finite-sample 有限样本First derivative 一阶导数First principal component 第一主成分First quartile 第一四分位数Fisher information 费雪信息量Fitted value 拟合值Fitting a curve 曲线拟合Fixed base 定基Fluctuation 随机起伏Forecast 预测Four fold table 四格表Fourth 四分点Fraction blow 左侧比率Fractional error 相对误差Frequency 频率Freguency distribution 频数分布Frequency polygon 频数多边图Frontier point 界限点Function relationship 泛函关系GGamma distribution 伽玛分布Gauss increment 高斯增量Gaussian distribution 高斯分布/正态分布Gauss-Newton increment 高斯-牛顿增量General census 全面普查Generalized least squares 综合最小平方法GENLOG (Generalized liner models) 广义线性模型Geometric mean 几何平均数Gini's mean difference 基尼均差GLM (General liner models) 通用线性模型Goodness of fit 拟和优度/配合度Gradient of determinant 行列式的梯度Graeco-Latin square 希腊拉丁方Grand mean 总均值Gross errors 重大错误Gross-error sensitivity 大错敏感度Group averages 分组平均Grouped data 分组资料Guessed mean 假定平均数HHalf-life 半衰期Hampel M-estimators 汉佩尔M 估计量Happenstance 偶然事件Harmonic mean 调和均数Hazard function 风险均数Hazard rate 风险率Heading 标目Heavy-tailed distribution 重尾分布Hessian array 海森立体阵Heterogeneity 不同质Heterogeneity of variance 方差不齐Hierarchical classification 组内分组Hierarchical clustering method 系统聚类法High-leverage point 高杠杆率点High-Low 低区域图Higher Order Interaction Effects,高阶交互作用HILOGLINEAR 多维列联表的层次对数线性模型Hinge 折叶点Histogram 直方图Historical cohort study 历史性队列研究Holes 空洞HOMALS 多重响应分析Homogeneity of variance 方差齐性Homogeneity test 齐性检验Huber M-estimators 休伯M 估计量Hyperbola 双曲线Hypothesis testing 假设检验Hypothetical universe 假设总体IImage factoring 多元回归法Impossible event 不可能事件Independence 独立性Independent variable 自变量Index 指标/指数Indirect standardization 间接标准化法Individual 个体Inference band 推断带Infinite population 无限总体Infinitely great 无穷大Infinitely small 无穷小Influence curve 影响曲线Information capacity 信息容量Initial condition 初始条件Initial estimate 初始估计值Initial level 最初水平Interaction 交互作用Interaction terms 交互作用项Intercept 截距Interpolation 内插法Interquartile range 四分位距Interval estimation 区间估计Intervals of equal probability 等概率区间Intrinsic curvature 固有曲率Invariance 不变性Inverse matrix 逆矩阵Inverse probability 逆概率Inverse sine transformation 反正弦变换Iteration 迭代JJacobian determinant 雅可比行列式Joint distribution function 分布函数Joint probability 联合概率Joint probability distribution 联合概率分布KK-Means Cluster 逐步聚类分析K means method 逐步聚类法Kaplan-Meier 评估事件的时间长度Kaplan-Merier chart Kaplan-Merier 图Kendall's rank correlation Kendall 等级相关Kinetic 动力学Kolmogorov-Smirnove test 柯尔莫哥洛夫-斯米尔诺夫检验Kruskal and Wallis test Kruskal 及Wallis 检验/多样本的秩和检验/H 检验Kurtosis 峰度LLack of fit 失拟Ladder of powers 幂阶梯Lag 滞后Large sample 大样本Large sample test 大样本检验Latin square 拉丁方Latin square design 拉丁方设计Leakage 泄漏Least favorable configuration 最不利构形Least favorable distribution 最不利分布Least significant difference 最小显著差法Least square method 最小二乘法Least Squared Criterion,最小二乘方准则Least-absolute-residuals estimates 最小绝对残差估计Least-absolute-residuals fit 最小绝对残差拟合Least-absolute-residuals line 最小绝对残差线Legend 图例L-estimator L 估计量L-estimator of location 位置L 估计量L-estimator of scale 尺度L 估计量Level 水平Leveage Correction,杠杆率校正Life expectance 预期期望寿命Life table 寿命表Life table method 生命表法Light-tailed distribution 轻尾分布Likelihood function 似然函数Likelihood ratio 似然比line graph 线图Linear equation 线性方程Linear programming 线性规划Linear regression 直线回归Linear Regression 线性回归Linear trend 线性趋势Loading 载荷Location and scale equivariance 位置尺度同变性Location equivariance 位置同变性Location invariance 位置不变性Location scale family 位置尺度族Log rank test 时序检验Logarithmic curve 对数曲线Logarithmic normal distribution 对数正态分布Logarithmic scale 对数尺度Logarithmic transformation 对数变换Logic check 逻辑检查Logistic distribution 逻辑斯特分布Logit transformation Logit 转换LOGLINEAR 多维列联表通用模型Lognormal distribution 对数正态分布Lost function 损失函数Lower limit 下限Lowest-attained variance 最小可达方差LSD 最小显著差法的简称Lurking variable 潜在变量MMain effect 主效应Major heading 主辞标目Marginal density function 边缘密度函数Marginal probability 边缘概率Marginal probability distribution 边缘概率分布Matched data 配对资料Matched distribution 匹配过分布Matching of distribution 分布的匹配Matching of transformation 变换的匹配Mathematical expectation 数学期望Mathematical model 数学模型Maximum L-estimator 极大极小L 估计量Maximum likelihood method 最大似然法Mean 均数Mean squares between groups 组间均方Mean squares within group 组内均方Means (Compare means) 均值-均值比较Median 中位数Median effective dose 半数效量Median lethal dose 半数致死量Median polish 中位数平滑Median test 中位数检验Minimal sufficient statistic 最小充分统计量Minimum distance estimation 最小距离估计Minimum effective dose 最小有效量Minimum lethal dose 最小致死量Minimum variance estimator 最小方差估计量MINITAB 统计软件包Minor heading 宾词标目Missing data 缺失值Model specification 模型的确定Modeling Statistics 模型统计Models for outliers 离群值模型Modifying the model 模型的修正Modulus of continuity 连续性模Morbidity 发病率Most favorable configuration 最有利构形MSC(多元散射校正)Multidimensional Scaling (ASCAL) 多维尺度/多维标度Multinomial Logistic Regression 多项逻辑斯蒂回归Multiple comparison 多重比较Multiple correlation 复相关Multiple covariance 多元协方差Multiple linear regression 多元线性回归Multiple response 多重选项Multiple solutions 多解Multiplication theorem 乘法定理Multiresponse 多元响应Multi-stage sampling 多阶段抽样Multivariate T distribution 多元T 分布Mutual exclusive 互不相容Mutual independence 互相独立NNatural boundary 自然边界Natural dead 自然死亡Natural zero 自然零Negative correlation 负相关Negative linear correlation 负线性相关Negatively skewed 负偏Newman-Keuls method q 检验NK method q 检验No statistical significance 无统计意义Nominal variable 名义变量Nonconstancy of variability 变异的非定常性Nonlinear regression 非线性相关Nonparametric statistics 非参数统计Nonparametric test 非参数检验Nonparametric tests 非参数检验Normal deviate 正态离差Normal distribution 正态分布Normal equation 正规方程组Normal P-P 正态概率分布图Normal Q-Q 正态概率单位分布图Normal ranges 正常范围Normal value 正常值Normalization 归一化Nuisance parameter 多余参数/讨厌参数Null hypothesis 无效假设Numerical variable 数值变量OObjective function 目标函数Observation unit 观察单位Observed value 观察值One sided test 单侧检验One-way analysis of variance 单因素方差分析Oneway ANOVA 单因素方差分析Open sequential trial 开放型序贯设计Optrim 优切尾Optrim efficiency 优切尾效率Order statistics 顺序统计量Ordered categories 有序分类Ordinal logistic regression 序数逻辑斯蒂回归Ordinal variable 有序变量Orthogonal basis 正交基Orthogonal design 正交试验设计Orthogonality conditions 正交条件ORTHOPLAN 正交设计Outlier cutoffs 离群值截断点Outliers 极端值OVERALS 多组变量的非线性正规相关Overshoot 迭代过度PPaired design 配对设计Paired sample 配对样本Pairwise slopes 成对斜率Parabola 抛物线Parallel tests 平行试验Parameter 参数Parametric statistics 参数统计Parametric test 参数检验Pareto 直条构成线图(佩尔托图)Partial correlation 偏相关Partial regression 偏回归Partial sorting 偏排序Partials residuals 偏残差Pattern 模式PCA(主成分分析)Pearson curves 皮尔逊曲线Peeling 退层Percent bar graph 百分条形图Percentage 百分比Percentile 百分位数Percentile curves 百分位曲线Periodicity 周期性Permutation 排列P-estimator P 估计量Pie graph 构成图饼图Pitman estimator 皮特曼估计量Pivot 枢轴量Planar 平坦Planar assumption 平面的假设PLANCARDS 生成试验的计划卡PLS(偏最小二乘法)Point estimation 点估计Poisson distribution 泊松分布Polishing 平滑Polled standard deviation 合并标准差Polled variance 合并方差Polygon 多边图Polynomial 多项式Polynomial curve 多项式曲线Population 总体Population attributable risk 人群归因危险度Positive correlation 正相关Positively skewed 正偏Posterior distribution 后验分布Power of a test 检验效能Precision 精密度Predicted value 预测值Preliminary analysis 预备性分析Principal axis factoring 主轴因子法Principal component analysis 主成分分析Prior distribution 先验分布Prior probability 先验概率Probabilistic model 概率模型probability 概率Probability density 概率密度Product moment 乘积矩/协方差Profile trace 截面迹图Proportion 比/构成比Proportion allocation in stratified random sampling 按比例分层随机抽样Proportionate 成比例Proportionate sub-class numbers 成比例次级组含量Prospective study 前瞻性调查Proximities 亲近性Pseudo F test 近似F 检验Pseudo model 近似模型Pseudosigma 伪标准差Purposive sampling 有目的抽样QQR decomposition QR 分解Quadratic approximation 二次近似Qualitative classification 属性分类Qualitative method 定性方法Quantile-quantile plot 分位数-分位数图/Q-Q 图Quantitative analysis 定量分析Quartile 四分位数Quick Cluster 快速聚类RRadix sort 基数排序Random allocation 随机化分组Random blocks design 随机区组设计Random event 随机事件Randomization 随机化Range 极差/全距Rank correlation 等级相关Rank sum test 秩和检验Rank test 秩检验Ranked data 等级资料Rate 比率Ratio 比例Raw data 原始资料Raw residual 原始残差Rayleigh's test 雷氏检验Rayleigh's Z 雷氏Z 值Reciprocal 倒数Reciprocal transformation 倒数变换Recording 记录Redescending estimators 回降估计量Reducing dimensions 降维Re-expression 重新表达Reference set 标准组Region of acceptance 接受域Regression coefficient 回归系数Regression sum of square 回归平方和Rejection point 拒绝点Relative dispersion 相对离散度Relative number 相对数Reliability 可靠性Reparametrization 重新设置参数Replication 重复Report Summaries 报告摘要Residual sum of square 剩余平方和residual variance (剩余方差)Resistance 耐抗性Resistant line 耐抗线Resistant technique 耐抗技术R-estimator of location 位置R 估计量R-estimator of scale 尺度R 估计量Retrospective study 回顾性调查Ridge trace 岭迹Ridit analysis Ridit 分析Rotation 旋转Rounding 舍入Row 行Row effects 行效应Row factor 行因素RXC table RXC 表SSample 样本Sample regression coefficient 样本回归系数Sample size 样本量Sample standard deviation 样本标准差Sampling error 抽样误差SAS(Statistical analysis system ) SAS 统计软件包Scale 尺度/量表Scatter diagram 散点图Schematic plot 示意图/简图Score test 计分检验Screening 筛检SEASON 季节分析Second derivative 二阶导数Second principal component 第二主成分SEM (Structural equation modeling) 结构化方程模型Semi-logarithmic graph 半对数图Semi-logarithmic paper 半对数格纸Sensitivity curve 敏感度曲线Sequential analysis 贯序分析Sequence 普通序列图Sequential data set 顺序数据集Sequential design 贯序设计Sequential method 贯序法Sequential test 贯序检验法Serial tests 系列试验Short-cut method 简捷法Sigmoid curve S 形曲线Sign function 正负号函数Sign test 符号检验Signed rank 符号秩Significant Level 显著水平Significance test 显著性检验Significant figure 有效数字Simple cluster sampling 简单整群抽样Simple correlation 简单相关Simple random sampling 简单随机抽样Simple regression 简单回归simple table 简单表Sine estimator 正弦估计量Single-valued estimate 单值估计Singular matrix 奇异矩阵Skewed distribution 偏斜分布Skewness 偏度Slash distribution 斜线分布Slope 斜率Smirnov test 斯米尔诺夫检验Source of variation 变异来源Spearman rank correlation 斯皮尔曼等级相关Specific factor 特殊因子Specific factor variance 特殊因子方差Spectra 频谱Spherical distribution 球型正态分布Spread 展布SPSS(Statistical package for the social science) SPSS 统计软件包Spurious correlation 假性相关Square root transformation 平方根变换Stabilizing variance 稳定方差Standard deviation 标准差Standard error 标准误Standard error of difference 差别的标准误Standard error of estimate 标准估计误差Standard error of rate 率的标准误Standard normal distribution 标准正态分布Standardization 标准化Starting value 起始值Statistic 统计量Statistical control 统计控制Statistical graph 统计图Statistical inference 统计推断Statistical table 统计表Steepest descent 最速下降法Stem and leaf display 茎叶图Step factor 步长因子Stepwise regression 逐步回归Storage 存Strata 层(复数)Stratified sampling 分层抽样Stratified sampling 分层抽样Strength 强度Stringency 严密性Structural relationship 结构关系Studentized residual 学生化残差/t 化残差Sub-class numbers 次级组含量Subdividing 分割Sufficient statistic 充分统计量Sum of products 积和Sum of squares 离差平方和Sum of squares about regression 回归平方和Sum of squares between groups 组间平方和Sum of squares of partial regression 偏回归平方和Sure event 必然事件Survey 调查Survival 生存分析Survival rate 生存率Suspended root gram 悬吊根图Symmetry 对称Systematic error 系统误差Systematic sampling 系统抽样TTags 标签Tail area 尾部面积Tail length 尾长Tail weight 尾重Tangent line 切线Target distribution 目标分布Taylor series 泰勒级数Test(检验)Test of linearity 线性检验Tendency of dispersion 离散趋势Testing of hypotheses 假设检验Theoretical frequency 理论频数Time series 时间序列Tolerance interval 容忍区间Tolerance lower limit 容忍下限Tolerance upper limit 容忍上限Torsion 扰率Total sum of square 总平方和Total variation 总变异Transformation 转换Treatment 处理Trend 趋势Trend of percentage 百分比趋势Trial 试验Trial and error method 试错法Tuning constant 细调常数Two sided test 双向检验Two-stage least squares 二阶最小平方Two-stage sampling 二阶段抽样Two-tailed test 双侧检验Two-way analysis of variance 双因素方差分析Two-way table 双向表Type I error 一类错误/α错误Type II error 二类错误/β错误UUMVU 方差一致最小无偏估计简称Unbiased estimate 无偏估计Unconstrained nonlinear regression 无约束非线性回归Unequal subclass number 不等次级组含量Ungrouped data 不分组资料Uniform coordinate 均匀坐标Uniform distribution 均匀分布Uniformly minimum variance unbiased estimate 方差一致最小无偏估计Unit 单元Unordered categories 无序分类Unweighted least squares 未加权最小平方法Upper limit 上限Upward rank 升秩VVague concept 模糊概念Validity 有效性V ARCOMP (Variance component estimation) 方差元素估计Variability 变异性Variable 变量Variance 方差Variation 变异Varimax orthogonal rotation 方差最大正交旋转V olume of distribution 容积WW test W 检验Weibull distribution 威布尔分布Weight 权数Weighted Chi-square test 加权卡方检验/Cochran 检验Weighted linear regression method 加权直线回归Weighted mean 加权平均数Weighted mean square 加权平均方差Weighted sum of square 加权平方和Weighting coefficient 权重系数Weighting method 加权法W-estimation W 估计量W-estimation of location 位置W 估计量Width 宽度Wilcoxon paired test 威斯康星配对法/配对符号秩和检验Wild point 野点/狂点Wild value 野值/狂值Winsorized mean 缩尾均值Withdraw 失访X此组的词汇还没找到YYouden's index 尤登指数ZZ test Z 检验Zero correlation 零相关Z-transformation Z 变换。
Eclipse 之 用SCAL处理岩心实验数据

用SCAL 处理岩心实验数据1实验实验数据的数据的数据的预预处理相渗曲线和毛管力曲线可以分别作成输入文件输入到SCAL 里面,然后再统一保存为一个工程。
1.1岩心岩心相渗曲线相渗曲线相渗曲线数据的输入数据的输入如果有多条相渗曲线,需要将文件处理为RCI 文件,格式如下:UNITS LAB--------------- -- Record 1 --------------- RecordATTRIBUTE UNLINKS2TSAMPLENAME KROW1 KRBASIS Kair KAIR 2.85 PORO 0.332 CONTANGLE 140 INTERTENS 50RESERVOIR 'Reservoir 1' COREDEPTH 3016.64 KROW TABLE / 0 0 1 0.404 0 1 0.478 0.012 0.182 … …0.486 0.014 0.16 0.701 0.043 0 /关键字及参数说明如下:关键字参数说明备注/单位Record用来引入一个岩样数据 SAMPLENAME 实验岩样名-KRBASIS 计算相渗的基准渗透率 Kair 表示使用气测渗透率 KAIR 岩样气测渗透率 Md PORO岩样孔隙度 小数 CONTANGLE 实验流体润湿角 Degree INTERTENS 实验流体界面张力 Dyne/cm RESERVOIR 取样油藏/断块名 COREDEPTH 取样深度MKROW油水相渗曲线后跟一个表,Sw/Krw/Kro ,该表需要有一个反斜杠结束在RCI文件中可以包含多个Record记录,每个记录可以包含一个岩心的多个实验数据标,本例中给出的是一个记录包含一个岩心数据的一条相渗曲线。
在一个岩心的相渗曲线数据输完后,再用一个Record关键字引出下一个岩心的数据。
可以参考附件中的core_rel_perm.RCI文件。
1.2毛管力实验数据的输入毛管力实验室数据也较多,因此也采用生成RCI文件的方式输入。
Empirical processes of dependent random variables
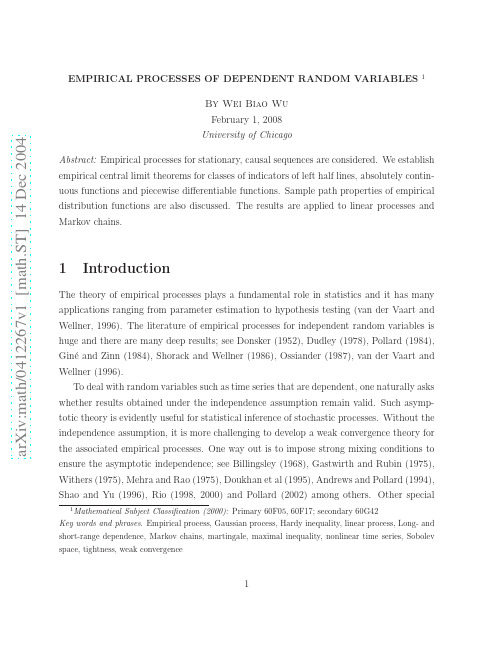
2
Preliminaries
n i=1
from R to R. The centered G -indexed empirical process is given by (P n − P )g = 1 n
n
the marginal and empirical distribution functions. Let G be a class of measurabrocesses that have been discussed include linear processes and Gaussian processes; see Dehling and Taqqu (1989) and Cs¨ org˝ o and Mielniczuk (1996) for long and short-range dependent subordinated Gaussian processes and Ho and Hsing (1996) and Wu (2003a) for long-range dependent linear processes. A collection of recent results is presented in Dehling, Mikosch and Sorensen (2002). In that collection Dedecker and Louhichi (2002) made an important generalization of Ossiander’s (1987) result. Here we investigate the empirical central limit problem for dependent random variables from another angle that avoids strong mixing conditions. In particular, we apply a martingale method and establish a weak convergence theory for stationary, causal processes. Our results are comparable with the theory for independent random variables in that the imposed moment conditions are optimal or almost optimal. We show that, if the process is short-range dependent in a certain sense, then the limiting behavior is similar to that of iid random variables in that the limiting distribution is a Gaussian process and the norming √ sequence is n. For long-range dependent linear processes, one needs to apply asymptotic √ expansions to obtain n-norming limit theorems (Section 6.2.2). The paper is structured as follows. In Section 2 we introduce some mathematical preliminaries necessary for the weak convergence theory and illustrate the essence of our approach. Two types of empirical central limit theorems are established. Empirical processes indexed by indicators of left half lines, absolutely continuous functions, and piecewise differentiable functions are discussed in Sections 3, 4 and 5 respectively. Applications to linear processes and iterated random functions are made in Section 6. Section 7 presents some integral and maximal inequalities that may be of independent interest. Some proofs are given in Sections 8 and 9.
- 1、下载文档前请自行甄别文档内容的完整性,平台不提供额外的编辑、内容补充、找答案等附加服务。
- 2、"仅部分预览"的文档,不可在线预览部分如存在完整性等问题,可反馈申请退款(可完整预览的文档不适用该条件!)。
- 3、如文档侵犯您的权益,请联系客服反馈,我们会尽快为您处理(人工客服工作时间:9:00-18:30)。
X0.0 X4.0 ...
X0.1
X4.1
...
X1.0
X5.0
...
X1.1
X5.1
...
X2.0
X6.0
...
X2.1
X6.1
...
C C C
012
X3.0
X7.0
...
X3.1
X7.1
...
C3
New Clusters
Introduced
finished Regular Service
Off-line Reorganization Regular Service (Display + Materialization)(Display + Materialization)
(No display)No New Services System Evacuation (2 hours)(20 mininutes)
System Evacuated
(Service existing requests only) Resume Regualr Service with higher number of displays
D = 40, d = 1, R_d = R_k = 40 (Mb/s), R_c = 4 (Mb/s)
C_orig = 40, C_new = 60, Capacity of one disk = 9 (GB)
Block size = 256 (KB), Object display length = 120 (min.)
Object Size = 3600 (MB)
Time Slot length = 0.05 (sec), Time Period length = 0.5 (sec)
Total Capacity = 360 (GB) = Total File System Size on disks
Number of Blocks per file = 14400, Number of files = 100
N_orig = 400
Time to Reorganize the file system = 20 minutes
New Clusters
Introduced finished
Regular Service
On-line Reorganization with Regular Service Regular Service (Display + Materialization)(Display + Materialization)
w/ 0% of N_orig (200 reorganizations): 20 min
w/ 20% of N_orig (80 displays + 160 reorganizations): 25 min
w/ 50% of N_orig (200 displays + 100 reorganizations): 40 min
w/ 80% of N_orig (320 displays + 40 reorganizations): 100 min
w/ 85% of N_orig (340 displays + 30 reorganizations): 133 min
w/ 90% of N_orig (360 displays + 20 reorganizations): 200 min
* N_orig (Number of displays with original configuration) = 400
Regular Service with
higher number of displays
14
1516
18
19
161722
23
: Reorganization Required : Type 1 (Read): Type 2 (Read): Type 3 (Write)
Time
Time
(Original Blocks, Reorganization Not Required) - Read
(New Blocks) - Write
: Read
: Write
Optimized
: Original Blocks : New Blocks : Retrieved Blocks
: Original Block : New Block
Time Beginning of Reorganization Switch from Old Scheduling with New Placement to New Scheduling with Old Placement Total file system
: Old Blocks : New Blocks 123671
2
3
4
5
67
8
9
10
11R R D
0,41,4,52,4,5,63,4,5,6,74,5,6,75,6,76,7,87,8,98,9,109,10,1110,1111Memory 891011W 78906045101145
New clusters
Introduced Reorganization finished Switch from Original Scheduling to
Regular Service
On-line Reorganization with Regular Service Regular Service Follow Original Scheduling
Follow New Scheduling (Display + Materialization)(Display + Materialization)
Regular Service with Increased Number of Displays
During A: Pre-fetch blocks for B
During B: Consume pre-fetched blocks
t t - LCM t + LCM
S S orig
new
Time (Time Periods)。