bus arrival time prediction method for its application
利用梯度提升树预测公交车到站时间

第37卷 第4期 福 建 电 脑 Vol.37 No.42021年4月Journal of Fujian ComputerApr. 2021———————————————李文锋(通信作者),男,1978年生,主要研究领域为智能交通行业应用。
E-mail:**************。
程远,男,1989年生,主要研究领域为智能交通行业应用。
E-mail:****************。
曹辉彬,男,1997年生,主要研究领域为数据挖掘、图像视觉分析。
E-mail:****************。
赖永炫,男,1981年生,主要研究领域为智能交通、大数据分析。
E-mail:*************.cn 。
张鹏,男, 1989年生,主要研究领域为智能交通行业应用。
E-mail: ****************。
赖颖琦,女,1992年生,主要研究领域为智能交通行业应用。
E-mail:*******************。
利用梯度提升树预测公交车到站时间李文锋1 程远1 曹辉彬2 赖永炫2 张鹏1 赖颖琦11(厦门卫星定位应用股份有限公司 福建 厦门 361000)2(厦门大学信息学院软件工程系 福建 厦门 361000)摘 要 当前我国公交公司普遍采用让具有丰富经验的公交调度人员以人工估计车辆到站的方法来调度车辆的发车。
这种方式缺少计算辅助,加上工作量大,经常容易出现错误预估导致无法缓解道路上常发生的同路公交车遇到一起(串车)或者相隔太远(大间隔)的情况。
公交到站时间受道路交通、乘客人数、时间、天气等诸多因素影响,具有不确定性。
本文基于该现实问题从公交公司角度出发,提出了一种基于动态特征选择和梯度提升树的公交到站时间预测算法。
其动态主要体现在对于不同线路、同一线路不同方向经过特征选择分别选取对该线路该方向站点停留和站间行驶影响较大的特征。
该算法用于辅助公交调度人员参考到站时间,从而使得调度人员可以作出更准确有效的调度策略。
公交客流预测的英语
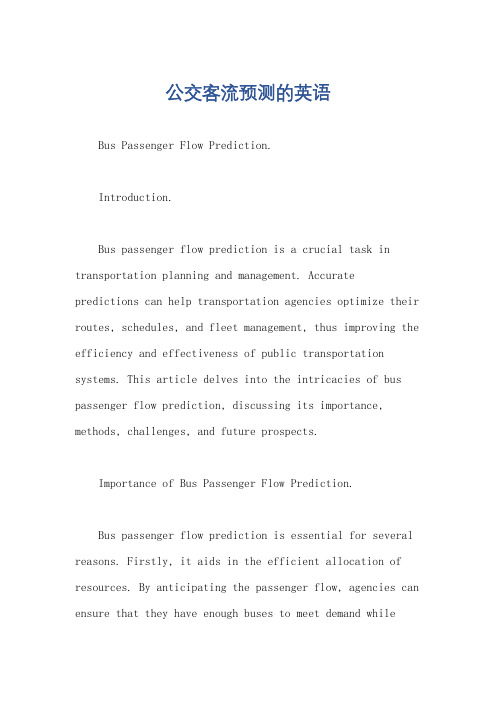
公交客流预测的英语Bus Passenger Flow Prediction.Introduction.Bus passenger flow prediction is a crucial task in transportation planning and management. Accuratepredictions can help transportation agencies optimize their routes, schedules, and fleet management, thus improving the efficiency and effectiveness of public transportation systems. This article delves into the intricacies of bus passenger flow prediction, discussing its importance, methods, challenges, and future prospects.Importance of Bus Passenger Flow Prediction.Bus passenger flow prediction is essential for several reasons. Firstly, it aids in the efficient allocation of resources. By anticipating the passenger flow, agencies can ensure that they have enough buses to meet demand whilealso avoiding unnecessary over-capacity. This helps in reducing costs and waste.Secondly, passenger flow prediction enables agencies to optimize their routing and scheduling decisions. By understanding when and where demand is highest, agencies can design routes that better serve passengers, reduce travel time, and enhance the overall passenger experience.Lastly, accurate predictions can help agencies prepare for突发事件 such as special events, weather changes, or emergencies. By anticipating changes in passenger flow, agencies can plan accordingly, ensuring that their transportation systems remain robust and responsive.Methods of Bus Passenger Flow Prediction.There are several methods used for bus passenger flow prediction, each with its own strengths and limitations.1. Historical Data Analysis: This approach relies on analyzing historical passenger flow data to identifypatterns and trends. It can be used to forecast future passenger flows based on past performance. However, it may not account for sudden changes in demand caused by external factors such as weather or special events.2. Statistical Models: Statistical models, such as regression analysis or time series analysis, are often used to predict passenger flow. These models rely on historical data and various explanatory variables to estimate future passenger numbers. While they can provide valuable insights, they may not capture the complex interactions between various factors that influence passenger flow.3. Machine Learning Algorithms: Machine learning algorithms, such as neural networks, random forests, or support vector machines, have been increasingly used for passenger flow prediction. These algorithms can learn from historical data and identify complex patterns and relationships. They can handle nonlinearities and interactions between various factors, making them more suitable for dynamic and uncertain environments. However, they require large amounts of data for training and may beprone to overfitting if not used carefully.4. Real-Time Monitoring and Prediction: Real-time monitoring systems can provide up-to-date information on passenger flow, enabling agencies to make timelyadjustments to their operations. By combining real-timedata with predictive models, agencies can achieve more accurate predictions and respond more quickly to changes in demand.Challenges in Bus Passenger Flow Prediction.Despite the importance of bus passenger flow prediction, there are several challenges that need to be addressed.1. Data Availability and Quality: Accurate predictions rely on high-quality data. However, obtaining comprehensive and reliable data on passenger flow can be challenging, especially in developing countries or regions with limited infrastructure. Additionally, data quality issues such as missing values, outliers, or inconsistencies can affect the accuracy of predictions.2. Dynamic and Uncertain Environments: Passenger flow is influenced by various dynamic and uncertain factors such as weather, traffic conditions, special events, or public holidays. Modeling these factors accurately can be difficult, and their impact on passenger flow may vary depending on the context and location.3. Complex Interdependencies: Passenger flow is influenced by multiple factors that interact in complex ways. Understanding and capturing these interdependencies can be challenging, especially when dealing with large-scale transportation systems.Future Prospects.Despite these challenges, there are several promising avenues for further research and development in bus passenger flow prediction.1. Advanced Data Collection Techniques: The development of new data collection techniques such as sensor networks,mobile phone data, or social media analytics can provide richer and more granular data on passenger flow. This will enable more accurate predictions and better understanding of passenger behavior.2. Integration of Multiple Data Sources: Integrating multiple data sources such as historical data, real-time monitoring data, and external factors can provide a more comprehensive picture of passenger flow. This integration can help improve the accuracy and reliability of predictions.3. Advancements in Machine Learning and Artificial Intelligence: The ongoing advancements in machine learning and artificial intelligence (AI) provide new opportunities for bus passenger flow prediction. Techniques such as deep learning or reinforcement learning can handle complex patterns and interactions better, enabling more accurate and robust predictions.4. Collaborative and Multi-Stakeholder Approaches: Collaboration between transportation agencies, privatesector partners, and academic institutions can foster innovation and improve the overall quality of passengerflow prediction. Multi-stakeholder approaches can also help ensure that predictions align with the needs and objectives of all parties involved.In conclusion, bus passenger flow prediction is acrucial task that requires a combination of data analysis, modeling techniques, and technological advancements. By addressing the challenges and leveraging the opportunities discussed in this article, transportation agencies can improve their understanding of passenger flow and make more informed decisions about their transportation systems. This, in turn, can lead to more efficient, responsive, and sustainable public transportation services that betterserve the needs of the community.。
GA-Elman公交车辆到站时间预测模型
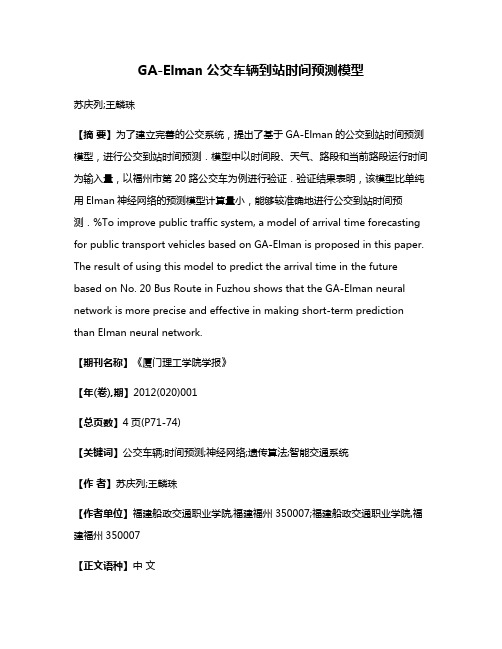
GA-Elman公交车辆到站时间预测模型苏庆列;王麟珠【摘要】为了建立完善的公交系统,提出了基于GA-Elman的公交到站时间预测模型,进行公交到站时间预测.模型中以时间段、天气、路段和当前路段运行时间为输入量,以福州市第20路公交车为例进行验证.验证结果表明,该模型比单纯用Elman神经网络的预测模型计算量小,能够较准确地进行公交到站时间预测.%To improve public traffic system, a model of arrival time forecasting for public transport vehicles based on GA-Elman is proposed in this paper. The result of using this model to predict the arrival time in the future based on No. 20 Bus Route in Fuzhou shows that the GA-Elman neural network is more precise and effective in making short-term prediction than Elman neural network.【期刊名称】《厦门理工学院学报》【年(卷),期】2012(020)001【总页数】4页(P71-74)【关键词】公交车辆;时间预测;神经网络;遗传算法;智能交通系统【作者】苏庆列;王麟珠【作者单位】福建船政交通职业学院,福建福州350007;福建船政交通职业学院,福建福州350007【正文语种】中文【中图分类】U491近年来,作为我国城市化进程的一个缩影,城区规模不断扩大,公交线路不断增多,公交网络急剧复杂化,许多学者都在为建立智能交通系统 (ITS)而努力.公交车辆到站时间预测在完善公共交通智能化调度系统、电子站牌显示系统及公交信息服务系统等方面起着重要作用.研究公交车辆到站时间预测方法,提高公交车辆到站时间预测精度,不仅可方便公众出行、提高公交效率,同时对缓解城市交通拥堵等状况具有重要意义.为此,各国学者做了大量的研究.傅惠等[1]采用了Kalman滤波算法进行公交到站时间预测,熊桂喜等[2]针对Kalman滤波算法需假设系统噪声和观测噪声为零均值的白色噪声的缺点,建立了基于改进Sage滤波器的公交到站时间预测.于滨等[3-4]采用SVM的方法建立公交到站时间预测模型,并针对SVM静态预测的缺点,提出了基于SVM和Kalman的到站时间预测模型.针对SVM方法所需数据量大的缺点,本文提出了基于GA-Elman的公交到站时间预测模型,该模型不仅具有动态预测特性,计算量小,还提高了公交到站时间的预测准确度.1 GA-Elman预测模型1.1 Elman神经网络Elman网络是一种典型的动态神经元网络,它在BP网络的输入层、隐含层和输出层基础上,增加了一个承接层[5],如图1所示.承接层用于记忆隐含层过去的状态,并且在下一时刻连同网络输入一起作为隐含层单元的输入,相当于状态反馈,具有一步延迟的特性,从而使得部分递归网络具有动态记忆功能.Elman网络无须存储所有的输入信息却能在网络中反映出所有历史信号对当前系统的影响,适合用来建立时间序列的预测模型.设Elman网络输入矢量为u(k),输出矢量为y(k),隐含层输出矢量为x(k),承接层输出矢量为xc(k),则Elman网络输入输出关系为:式中:w1为承接层到隐含层的连接权值;w2为输入层到隐含层的连接权值;w3为隐含层到输出层的连接权值;f(·)为隐含层神经元的传递函数;g(·)为输出神经元的传递函数.1.2 基于GA的Elman网络优化遗传算法是模拟生物在自然环境力的遗传和进化过程而形成的一种自适应全局优化概率搜索算法.在遗传算法的每一代中,按照适者生存和优胜劣汰的原理,产生一个新的近似解,获得的新个体比原个体更能适应环境.通过模拟生物进化过程中繁殖、杂交和变异的自然选择规律,实现最优化[6-7].将遗传算法用于优化Elman网络的初始权值、阈值过程如下:步骤1 设定参数:个体编码串长度L、群体大小M、交叉算子PC变异算子Pm、终止代数T、代沟G.经调试后选择L=12,种群规模n=100,Pc=0.8,Pm=0.004,G=20.步骤2 编码、生成初始种群.步骤3 计算个体评价函数.步骤4 将选择算子、交叉算子、变异算子作用于种群,产生下一代种群.步骤5 终止条件判断:第G代之内最高适应值无显著变化.若未找到满意个体,则重复步骤3、4,直至满足评价函数的预设标准.2 GA-Elman公交到站时间预测2.1 预测模型建立采用GA-Elman遗传神经网络算法和Elman神经网络分别建立时间预测模型.基于GA-Elman遗传神经网络算法建立公交到站时间预测模型,该模型输入层神经元个数为4,分别为时间段 (节假日高峰期,节假日非高峰期,非节假日高峰期,非节假日非高峰期)、天气 (非雨天,雨天)、路段(人流集中区,人流非集中区)、当前路段的运行时间;隐含层和承接层的个数为8;输出层神经元个数为1,即公交到站时间.基于Elman神经网络模型建立公交到站时间预测模型,在这里采用的输入层为4个神经元,输出层为1个神经元,隐含层为6个神经元.2.2 样本采集为了验证模型的精度,利用福州市第20路公交车的数据进行校验.20路公交行程为:从省公安学校站到火车站,共27个站,线路总长13.691 km.实际选取从省公安学校站至对湖站的6个站点5个路段为例,途经省交通学院站、仓山镇站、程埔头站和师大站,区间的相关信息如图2所示.该路段从省公安学校站起点,途径省交通学院站和仓山镇站为非人流集中区.从首山路和上山路的交叉路口后为师大学生街范围,故程埔头站和师大站地处人流集中区,再到非分流集中区的对湖站.在该GA-Elman的时间预测模型中以时间段、天气、路段和当前路段运行时间为输入量.2010年以来对该路段进行了随车调查,调查数据包括车辆到达这6个站点的时刻,时间段 (节假日高峰期6∶30 ~7∶30,节假日非高峰期10∶00 ~11∶00,非节假日高峰期6∶30~7∶30,非节假日非高峰期10∶00~11∶00)、天气 (非雨天,雨天)、路段 (人流集中区,非人流集中区),当前路段的运行时间,共采集160组数据样本.考虑到非雨天市民出行率比雨天高、高峰期市民出行压力大且该时段路况较不稳定等因素,加大对非雨天和高峰期的数据样本采集量.具体数据样本组数分布如表1所示.2.3 数据预处理由于学习方法要求输入层的输入值在[0,1]之间,其输出范围也要求在[0,1]之间,因此,对以上收集到的160组路段运行时间的随车调查数据进行归一化处理.总共采用120组样本分别对基于Elman和基于GA-Elman的两种时间预测模型进行训练,在每项中预留5组样本作为测试样本.2.4 预测结果分析Elman神经网络时间预测模型和GA-Elman时间预测模型的训练过程和结果比较如图3、图4所示.从图3中可以看出Elman训练迭代次数为204次,而GA-Elman仅为72次,可见基于GA-Elman时间预测模型收敛速度快、训练时间短、训练精度高,符合公交到站时间预测要求.表1 调查样本分布情况Tab.1 Distribution of samples个样本样本量非雨天F雨天Y FF 20 15非节假日高峰期FG 25 20节假日非高峰期JF 20 15节假日高峰期JG 25 20合计非节假日非高峰期90 70如图4所示,对基于GA-Elman遗传神经网络算法和Elman神经网络的时间预测模型的公交到站时间预测误差进行比较.其中,图4(a)为非节假日非高峰期非雨天(FFF类)的公交到站时间预测误差对比,图4(b)为节假日高峰期雨天(JGY类)的公交到站时间预测误差对比.由图4时间预测误差比较结果可以看出,基于GA-Elman遗传神经网络算法的到站时间预测精度比基于单一Elman神经网络的到站时间预测精度高,预测曲线也比较平稳,较Elman神经网络波动小,误差也较小.3 结语针对公交到站时间预测在完善公共交通智能化调度系统、电子站牌显示系统及公交信息服务系统等方面的重要作用,在分析公交车辆行驶特性的基础上,用遗传算法对Elman神经网络进行优化,建立GA-Elman遗传神经网络算法的时间预测模型,并将其应用于公交车辆到站时间预测.仿真模拟结果表明,基于GA-Elman遗传神经网络算法与单纯的Elman神经网络的时间预测模型相比,迭代次数少、计算能力和训练精度提高,可有效预测公交车辆到站时间,为市民出行提供方便.[参考文献][1]傅惠,徐建闽.基于卡尔曼滤波的路径行程时间预测方法[J].微计算机信息,2007,23(8-1):290-292.[2]熊桂喜,刘铭志.基于改进Sage滤波器的车辆行程时间预测模型[J].计算机技术与发展,2008,18(9):162-164.[3]于滨,杨忠振,曾庆成.基于SVM和Kalman滤波的公交车到站时间预测模型[J].中国公路学报,2008,21(2):89-92.[4]PADMANABAN R P S.Development of a real-time bus arrival prediction system for Indian traffic conditions[J].IET Intell Transp Syst,2010,4(3):189-200.[5]JEFFREY L,ELMAN.Finding structure in time [J].Cognitive Science,1990,14:179-211.[6]乔晶晶,潘宏侠.基于遗传算法优化神经网络的齿轮故障诊断[J].水电能源科学,2010,28(6):106-108.[7]MURPHY L A,ABDEL-ATY-ZOHDY H S,SHERIF M H.A genetic algorithm tracking model for product deployment in telecom services[C]//48th IEEE International Midwest Sympusium on Circuits andSystem.Covington:IEEE Press,2005.。
基于LSTM和Kalman滤波的公交车到站时间预测

基于LSTM和Kalman滤波的公交车到站时间预测范光鹏;孙仁诚;邵峰晶【摘要】The construction of intelligent transportation system has become the main problem facing the development of urban transportation.The prediction of bus arrival time is an important part of intelligent transportation system.Bus arrival time data is time-series data with long-term and short-term characteristics,and the bus is susceptible to external factors,so the bus arrival time is also dynamic.Based on the above problems,a bus arrival time prediction model based on LSTM and Kalman filtering was proposed,in which LSTM model was used to predict the basic time series of bus arrival and arrival,and Kalman filtering model was used to dynamically adjust the basic time series.Finally,the adjusted prediction accuracy,mean square deviation and mean absolute deviation were respectively compared with those predicted by LSTM,SVM and SVM + Kalman models.It was proved that the accuracy,mean square deviation and average absolute deviation of LSTM + Kalman model prediction were better than the comparative model.%智能交通系统的建设已成为城市交通发展面临的主要问题,其中公交车到站时间预测是智能交通系统的重要组成部分.公交车到站时间数据是具有长期和短期特性的时间序列数据,而且公交车易受到外来因素的影响,因此公交车到站时间也是动态变化的.基于上述问题,提出基于LSTM和Kalman滤波的公交车到站时间预测模型,其中LSTM模型用来预测公交车到站的基础时间序列,Kalman滤波模型用于对基础时间数据序列进行动态调整,最终将调整后的预测值的正确率、均方差、平均绝对偏差分别与LSTM、SVM、SVM+Kalman模型预测结果进行对比,证明LSTM+ Kalman模型预测值的正确率,均方差和平均绝对偏差均优于对比模型.【期刊名称】《计算机应用与软件》【年(卷),期】2018(035)004【总页数】6页(P91-96)【关键词】智能交通;公交车到站时间;LSTM模型;Kalman滤波;时间序列【作者】范光鹏;孙仁诚;邵峰晶【作者单位】青岛大学计算机科学技术学院山东青岛266071;青岛大学计算机科学技术学院山东青岛266071;青岛大学计算机科学技术学院山东青岛266071【正文语种】中文【中图分类】TP391.90 引言随着国民经济的发展,私家车数量越来越多,给城市交通带来巨大压力,交通问题已成为城市发展面临的巨大问题,因此建设智能交通系统是城市交通建设的主要任务。
公交到达时间预测误差分析及模型改进

Science &Technology Vision 科技视界均值N 标准差t Sig.(双侧)短时精度87.83%200 3.52%11.0750.000长时精度85.08%2004.27%0引言公交到达时间预测是智能交通领域研究的热点问题之一,而公交到达时间预测是一个持续改进的过程。
目前以存在了许多公交到达时间预测的方法,如人工神经网络算法,支持向量机、自回归时间序列,卡尔曼滤波等等[1-3],这些算法均有自身的优点,但也存在一定的局限性,预测精度还有待进一步的提高。
文献[1]已经建立了公交到达时间预测模型,并且经实验验证能取得较好的结果,而进一步分析,其精度还可以进一步提高。
因此,为进一步提高公交到达时间预测的精度,本文在已有模型的基础上,通过利用海量的公交车GPS 数据进行长期的测试,对分析测试结果做详细的分析,同时结合公交车GPS 数据,分析产生误差产生的原因(特别是大误差产生的原因),针对具体的问题提出相应的解决方案,对公交到达时间预测模型做进一步的改善和优化,以提高模型的精度。
1旧模型公交到达时间预测结果分析1.1原有模型简介文献[3]将公交到达时间分成了路段行程时间、车站区域停留时间和其他延误时间3部分,提出了公交达到时间预测模型,如下所示:T ntk =T mtk +n -1b =m∑T b (b -1)t +n -1b =m∑T btd +qp =1∑T psd(1)其中T mtk 和n -1b =m ∑T b (b -1)t 为共同组成路段行程时间,n -1b =m∑Tbtd为车辆靠站停留时间,q p =1∑T psd 为信号灯延误时间。
本文在文献[1]的基础上建立公交到达时间预测模型,以重庆市典型公交线路如886(日间车线路)和0811(夜间车线路)为例,并利用从2012年-2013年期间的公交GPS 实际数据进行测试。
1.2评价指标介绍公交到达时间预测精度的评价是一个相对的指标,既需要考虑到绝对误差,又要考虑到乘客的主观感受,因此,本文采用短时精度和长时精度两个指标进行评价。
基于粒子滤波的公交车辆到站时间预测研究

基于粒子滤波的公交车辆到站时间预测研究任远;吕永波;马继辉;陈鑫杰;余明捷【摘要】准确预测公交车辆到站时间对于改善公交服务水平、提升公交吸引力、缓解交通拥堵具有重要意义.公交车辆到站时间受到实际路面情况影响很大,粒子滤波算法对于这种非线性、非高斯的随机系统具有很好的适用性.因此本文探索性地应用粒子滤波算法建立公交车辆到站时间预测模型(BAT-PF),并以北京市公交300路内环线位置数据为基础,选取高峰和平峰时刻进行实例研究,并将预测结果与卡尔曼滤波算法所得预测结果进行对比分析.结果表明,本文建立的公交车辆到站时间粒子滤波预测模型具有更好的适用性和稳定性,而且预测精度高.%Providing accurate bus arrival time (BAT) can help to improve the service quality of a transit system, enhance bus attractiveness and ease traffic jams. BAT is greatly influenced by the complex road conditions, particle filter algorithm can be well applied to this kind of nonlinear and non-Gaussian systems. Therefore, a BAT based on particle filter algorithm prediction model (BAT-PF) is proposed tentatively. Then, based on the location data, a case study of the inner line 300 of Beijing is conducted. Bus arrival time during the morning peak hours and off-peak hours are forecasted by both the BAT-PF and the Kalman filter (KF). The results show that the BAT-PF is more applicable and stable to predict bus arrival time and has a higher accuracy.【期刊名称】《交通运输系统工程与信息》【年(卷),期】2016(016)006【总页数】5页(P142-146)【关键词】城市交通;适用性;粒子滤波;公交到站时间;卡尔曼滤波;卫星定位数据【作者】任远;吕永波;马继辉;陈鑫杰;余明捷【作者单位】北京交通大学交通运输学院,北京100044;北京交通大学交通运输学院,北京100044;北京交通大学交通运输学院,北京100044;北京交通大学交通运输学院,北京100044;北京交通大学交通运输学院,北京100044【正文语种】中文【中图分类】U491.17目前我国城市公共交通系统存在信息化水平低、服务质量差、车速慢,以及车辆准点率低等问题,降低了公交分担率,增加了交通压力.随着定位、通信等高新技术的发展,使预测公交车辆到站时间成为可能.公交到站时间的准确预测不仅可以节约乘客出行时间,改善公交服务水平,提高公共交通的吸引力,方便运营者进行实时调度管理.因此,研究公交车辆到站时间预测具有重要意义.国内外学者在公交到站时间预测领域开展了广泛研究.汪磊等[1]建立多元线性回归模型对大连市21路公交车辆行程时间进行了预测和评价分析.杨兆升等[2]建立了基于BP神经网络的行程时间预测模型,编制了行程时间预测软件系统,并利用长春市的交通实测数据对行程时间进行了预测. Kumar等[3]通过自动车辆定位系统开发了一个基于不同模式的Kalman滤波预测模型,并利用金奈市公交线路的卫星定位数据对混杂交通条件下的车辆行驶时间进行了预测.Ranhee Jeong等[4]建立了人工神经网络模型,将准点率、停站时间和到站时间作为输入变量,利用休斯顿市公交线路的AVL数据进行了预测.Lin等[5]开发了两个神经网络模型ANN和HANN,并以济南市某路公交车的运行数据进行实证研究.Chen等[6]建立了基于ANN和Kalman滤波的组合预测模型,实现了公交车辆到站的动态预测.Yu等[7]提出了基于改进支持向量机模型预测公交车辆运行时间,并利用大连市23路公交车数据进行了模型效果检验.上述预测模型中,多元回归模型适用性差,预测精度较低.神经网络模型可以很好地拟合非线性系统,但使用前需要大量的历史数据对其进行训练,而且存在欠学习和过学习,以及局部最优的问题.支持向量机具有很强的学习能力和容错能力,其泛化能力优于神经网络,但也需要对其进行训练,难以用于实时预测.Kalman滤波模型预测精度高且能够在线预测,具有很强的实时性,但对非线性和非高斯分布的状态模型,其精度很难保证[8].公交系统是一个时变性非常强的非线性系统,而粒子滤波(Particle Filter,PF)算法适用于各种状态空间模型表示的非线性随机系统,能有效改善最优估计的效果,但目前在交通领域,粒子滤波算法主要被应用于交通状态的预测[9-11],因此本文探索性地应用粒子滤波算法预测公交车辆到站时间,建立了基于粒子滤波公交车辆到站时间的预测模型,并对该算法的适用性及精度进行研究.1.1 离散化公交线路为了提高预测精度,本文将公交线路的站间路段以相等的长度l划分成多个子区间(不足长度l的按实际长度计算,本文中l取20 m),区间端点(包括站点)定义为关键点,关键点k和关键点k+1之间的区间编号为K,如图1所示.1.2 数据匹配本文使用的公交车辆位置数据来源于车载卫星定位设备,将公交车辆经纬度、时间、瞬时速度等信息提取出来后,利用地理信息系统中缓冲区分析思想将这些信息同线路、区间、关键点进行匹配,以获得车辆在1.1节中提出的区间K的平均速度,作为预测的基础.1.3 技术路线采用逆向查找法,根据实时公交车辆位置信息,获得距离请求站点最近的公交车位置、瞬时速度等信息.判定其所在区间K或关键点k,将瞬时速度与历史平均速度进行融合,运用粒子滤波算法预测该车到达站点的时间.2.1 粒子滤波算法基本原理粒子滤波是一种基于蒙特卡罗方法和递推贝叶斯估计的统计滤波方法,依据大数定理采用蒙特卡罗方法来求解贝叶斯估计中的积分运算[12],其动态空间模型如下:状态方程观测方程式中:xk为k时刻系统的状态值;yk为k时刻系统的观测值;wk、nk分别为系统的状态噪声和观测噪声,假定1到k时段的一组观测值Zk={y1:i|i=1,2,…,k}已知,根据贝叶斯估计理论可得由于式(7)中的积分很难直接计算得出,粒子滤波算法中通过蒙特卡洛方法和重要性采样对方程进行求解.其基本原理是生成一组随机样本,即粒子,根据重要密度函数对后验概率分布函数进行估计,具体计算方式为式中:x0:k是0到k时刻的状态集;wik为k时刻第i个粒子对应的权值,且满足是重要密度函数.粒子滤波算法对线性和非线性系统都有较好的应用性,但普遍存在粒子退化现象,即在经过一定的递推之后,重要性权值有可能集中到少数粒子上,紧靠这些粒子已不能有效表达出后验概率密度函数.为了克服粒子退化现象,提高预测精度,本文采用了应用最广泛的随机重采样方法进行粒子重采样,方法如下:(1)产生N个在[0,1]上均匀分布的随机数形成一个具有N个随机数的集合;(2)通过搜索算法找到满足以下条件的整数n,使得记录样本并将其作为新样本集中的采样;(3)将区间[0,1]按分成N个小区间,当随机数ui落在第n个区间In=(λn-1,λ]时,对应样本xnk进行复制.在采样总数保持不变的情况下,权值较大的样本被多次复制,从而实现重采样过程.2.2 基于粒子滤波公交车辆到站时间预测模型(BAT-PF)BAT-PF模型由状态方程和观测方程两部分组成.(1)状态方程.公交车到站时间是模型的状态变量,其中,xk表示公交车到达关键点k的时间;sk-1表示关键点k-1与关键点k之间的距离;vc,k-1表示公交车辆在区间k-1上的计算速度;A为状态转移系数;B为控制变量系数;wk-1为过程噪声.为了提升实时数据对交通状况的反映,并解决卫星定位瞬时车速等于零的问题,引入权重系数α,对卫星定位获取的实时速度和区间历史平均速度进行加权,求得计算速度,其计算公式为式中:vik表示公交车经过关键点k的瞬时速度;vhk表示公交车经过关键点k的历史平均速度.(2)观测方程.式中:yk表示公交车到达关键点k的观测时间;H为观测值系数;nk表示观测噪声.综上所述,公交车辆到站时间粒子滤波预测模型为本文设定A、B、H均取单位矩阵E,过程噪声wk-1和观测噪声nk相互独立. 2.3 基于粒子滤波的公交到站时间预测流程基于粒子滤波算法的公交车辆到站时间预测流程如下:Step 1初始化,设置算法所需参数,如初始迭代变量k=0,迭代次数为M,粒子数为N.Step 2由初始概率密度函数p(x0)确定N个粒子的参数所有粒子的初始权重为1 N.Step 3在关键点k,利用状态方程(1)和观测方程(3)计算xik(i=1,2,…,N)和对应的yik.Step 4更新粒子权值.根据公式)计算出每个粒子的权值,并通过公式归一化得到Step 5重采样.评估退化程度,判断是否需要进行重采样.本文采用Geweke等[12]提出的相对效率(RNE)来度量粒子的退化程度.有效抽样尺度Neff的定义为设定一个有效样本数Nth作为阈值,当Neff>Nth时,转到Step6;当Neff≤Nth时,则应用随机采样方法进行重采样.Step6进行状态估计.根据公式计算后验概率密度函数,然后根据计算状态变量Step 7判断算法是否结束.若k=M,则算法结束,即公交车辆到达请求站点,若k <M,则k=k+1,并转到Step3.本文选用北京市300内环线路公交车的卫星定位数据对模型进行检验.该数据涵盖了2013年3月份所有工作日(周一~周五),22天中5 190趟车次的运行数据.300内环公交线路行程为草桥到草桥的环线,线长49.0 km,共34个车站.按照l=20 m等分线路(不足部分按实际长度计算),得到2 450个区间.实际选取从六里桥南到安贞桥西这段具有代表性的线路对模型进行检验,该部分线路共包括13个站点、12个站间路段、850个区间.选取其中20天(1,4~8,11~15,18~22,25~26,29日)的数据作为基础数据以获得各个区间和关键点的不同时段的历史平均车速,对27、28日两天早高峰中08:00和上午平峰中11:00两个时刻进行预测.经过实验方法,确定α较优取值为0.6.预测值和实际值对比如图2所示.由图2可以看出,应用BAT-PF模型预测公交车辆到站时间可以很好地拟合实际情况,具有相同的变化趋势,且预测误差基本控制在2.5 min之内,因此BAT-PF 模型可以用来进行公交车辆到站时间的预测,具有适用性.为了进一步评价该算法的准确性和稳定性,将其预测结果同Kalman滤波算法所得结果进行对比,选择平均绝对误差(MAE)作为评价指标,结果如图3所示.式中:treal为实际值,tpred为预测值.由图3可以看出,不同时刻BAT-PF模型预测结果的平均绝对误差都要小于Kalman滤波算法预测结果的平均绝对误差.此外,不同时刻应用BAT-PF模型预测结果的波动性明显小于Kalman滤波算法,而且只是在一个很小的范围内扰动,平均绝对误差范围在40~150 s之间,而Kalman滤波算法的平均绝对误差波动范围为60~290 s.本文将粒子滤波算法应用到公交到站时间预测中,建立了基于粒子滤波的公交到站时间预测模型(BAT-PF),并引入权重系数α以提升预测的实时性,最终将预测结果与Kalman滤波算法所得结果进行对比分析.结果表明,粒子滤波算法适用于公交到站时间预测,且具有很好的准确性和稳定性.【相关文献】[1]汪磊,左忠义.基于MLR的公交车行程时间预测模型[J].大连交通大学学报,2015,36(2):1-5.[WANG L, ZUO Z Y.Bus travel time prediction based on MLR[J]. Journal of Dalian Jiaotong University,2015,36(2):1-5.][2]杨兆升,朱中.基于BP神经网络的路径行程时间实时预测模型[J].系统工程理论与实践,1999,19(8):59-64.[YANG Z S,ZHU Z.Real-time prediction of bus travel time based onBP artificial neural network[J]. System Engineering-Theory&Practice,1999,19(8):59-64.] [3]KUMAR B A,et al.Pattern-based travel time prediction underheterogeneoustrafficconditions[C]. Transportation Research Board 93rd Annual Meeting, 2014.[4]JEONG R,RILETT L R.Bus arrival time prediction using artificial neural networkmodel[C].2004 IEEE Intelligent Transportation Systems Conference,2004: 988-993.[5]LIN Y,YANG X,et al.Real-time bus arrival time prediction:case study forJinan,China[J].Journal of Transportation Engineering,2014,139(11):1133-1140.[6]CHEN M,LIU X B,XIA J X.A dynamic bus-arrival time prediction model based on APCdata[J].Computer-Aided Civil and Infrastructure Engineering,2004(19): 364-376.[7]YU B,YE T,et al.Bus travel-time prediction with a forgetting factor[J].Journal ofComputing in Civil Engineering,2014,28(3).[8]陈旭梅,龚辉波,王景楠.基于SVM和Kalman滤波的BRT行程时间预测模型研究[J].交通运输系统工程与信息,2012,12(4):29-34.[CHEN X M,GONG X B, WANG J N.BRT vehicle travel time prediction based on SVM and Kalman filter[J].Journal of Transportation Systems Engineering and Information Technology,2012,12(4):29-34.][9]MIHAYLOVA L,BOEL R.A particle filter for freeway traffic estimation[C].IEEE Conference on Decision& Control,2005(2):608-616.[10]CHEN H,RAKHA H A,SADEK S.Real-time freeway traffic state prediction:A particle filter approach[C]. IEEE Conference on Intelligent Transportation Systems, 2011:626-631. [11]BI J,CHANG C,FAN Y.Particle filter for estimatingfreewaytrafficstateinBeijing[J].Mathematical Problems in Engineering,2013,70(2):717-718.[12]朱志宇.粒子滤波算法及其应用[M].北京:科学出版社,2010.[ZHU Z Y.Particle filtering algorithm and application[M].Beijing:Science Press,2010.]。
交通事件持续时间预测方法综述
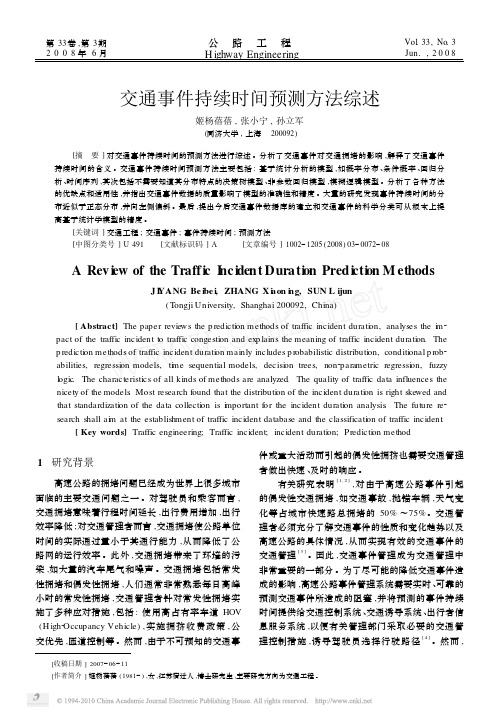
件或重大活动而引起的偶发性拥挤也需要交通管理 者做出快速 、及时的响应 。
有关研究表明 [ 1, 2 ] ,对由于高速公路事件引起 的偶发性交通拥堵 ,如交通事故 ,抛锚车辆 ,天气变 化等占城市快速路总拥堵的 50% ~75%。交通管 理者必须充分了解交通事件的性质和变化趋势以及 高速公路的具体情况 ,从而实现有效的交通事件的 交通管理 [ 3 ] 。因此 ,交通事件管理成为交通管理中 非常重要的一部分 。为了尽可能的降低交通事件造 成的影响 ,高速公路事件管理系统需要实时 、可靠的 预测交通事件所造成的阻塞 ,并将预测的事件持续 时间提供给交通控制系统 、交通诱导系统 、出行者信 息服务系统 ,以便有关管理部门采取必要的交通管 理控制措施 ,诱导驾驶员选择行驶路径 [ 4 ] 。然而 ,
的交通事件数据进行 Kolmogorov2Sm irnov检验 , 得
出所有的交通事件和每一类特殊的交通事件分别以
从 0. 31到 0. 99的显著水平服从对数正态分布 。但
是研究中交通事件数据仅包括事件总的持续时间 ,
因此不能证明每一阶段的持续时间都受到前一阶段
持续时间影响的这一假设 。 Golob的这一研究是对
然而如果将交通事件按照事件的类型严重程度分成子类则相似的交通事件的持续时间服从正态分布这一结论支持相似事件的持续时间是随机变量的推测使用西雅图的交通事件数据建立了事件持续时概率分布annering发现威布尔概率分布也可以用于描述一些事件数据个概率分布对数正态分布双对数分布威布尔分布的共同特点是概率分布的左侧特点它表明较短持续时间的事件占较大的比例见图但是该方法的缺点是在预测模型中较少考虑事件的相关影响因素右边项的总和服从正态分布因此lnygolob根据事件的类型和车道关闭数332个高速公路交通事件数据和193个匝道数据分成16kolmogorov2smirnov99的显著水平服从对数正态分布因此不能证明每一阶段的持续时间都受到前一阶段持续时间影响的这一假设golob的这一研究是对事件持续时间的统计分布的第一次研究此后这一研究结论鼓励其他许多研究者在基于持续时间对数正态分布模型的基础上对事件持续时间进行分析giuliano将对数正态分布应用于事件持续时间golobgiuliano采用近洛杉叽地区10高速公路的交通事件持续时间数据同样得出在相同类型的交通事giuliano不同事件giuliano分析得晚上交通事件的平均持续时间大于白天的持续时间
公交到站时间实时预测信息发布技术研究
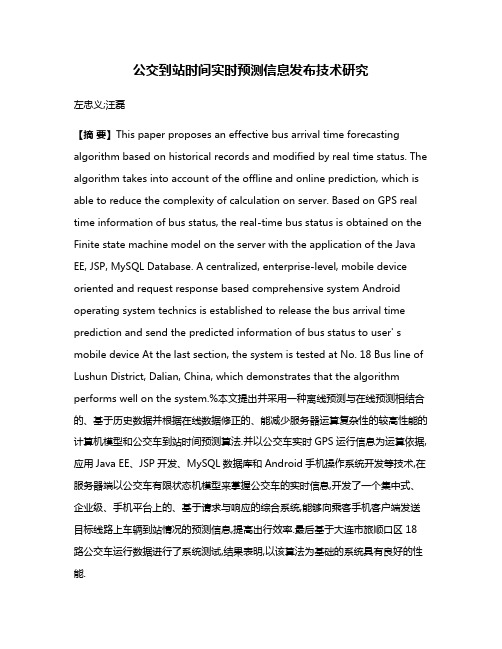
公交到站时间实时预测信息发布技术研究左忠义;汪磊【摘要】This paper proposes an effective bus arrival time forecasting algorithm based on historical records and modified by real time status. The algorithm takes into account of the offline and online prediction, which is able to reduce the complexity of calculation on server. Based on GPS real time information of bus status, the real-time bus status is obtained on the Finite state machine model on the server with the application of the Java EE, JSP, MySQL Database. A centralized, enterprise-level, mobile device oriented and request response based comprehensive system Android operating system technics is established to release the bus arrival time prediction and send the predicted information of bus status to user' s mobile device At the last section, the system is tested at No. 18 Bus line of Lushun District, Dalian, China, which demonstrates that the algorithm performs well on the system.%本文提出并采用一种离线预测与在线预测相结合的、基于历史数据并根据在线数据修正的、能减少服务器运算复杂性的较高性能的计算机模型和公交车到站时间预测算法.并以公交车实时GPS运行信息为运算依据,应用Java EE、JSP开发、MySQL数据库和Android手机操作系统开发等技术,在服务器端以公交车有限状态机模型来掌握公交车的实时信息,开发了一个集中式、企业级、手机平台上的、基于请求与响应的综合系统,能够向乘客手机客户端发送目标线路上车辆到站情况的预测信息,提高出行效率.最后基于大连市旅顺口区18路公交车运行数据进行了系统测试,结果表明,以该算法为基础的系统具有良好的性能.【期刊名称】《交通运输系统工程与信息》【年(卷),期】2013(013)001【总页数】7页(P63-68,75)【关键词】智能交通;到站时间预测;行程时间;公共交通;系统设计【作者】左忠义;汪磊【作者单位】大连交通大学交通运输工程学院辽宁大连116028;大连交通大学交通运输工程学院辽宁大连116028【正文语种】中文【中图分类】U491.7随着我国改革开放的不断深入、国民经济的不断发展,交通需求与通行能力、道路使用与道路建设之间存在的矛盾日益突出,发展容量大、承载高、污染低、快速准时的公共交通将是必然趋势[1].我国城市公共交通系统尚存在信息服务质量差、整体运营效率低等问题.随着信息时代的到来,计算机产业、互联网技术和通讯手段的不断进步,智能交通、智能社会距离人们越来越近,与此同时市民出行对公交信息的需求却日益增加.出行者在等候公交车时,经常会出现车辆久等不来、扎堆而来的情况,易导致出行者出现心情烦躁、焦虑等问题,同时公交车辆的到达情况也会影响出行者对下一步出行的规划和判断[2].方便出行者出行,节约出行者时间,是我们研究公交车信息提供和发布系统的目的所在[3].随着ITS的不断发展,公交车到站时间预测、电子站牌等技术在国外已经有了广泛研究和应用.在美国,Lin等人为美国弗吉尼亚州布莱克斯堡提出的基于GPS和时刻表的公交到站时间预测在郊区线路和长距离公交运输上得到了较多应用[4].在日本,政府大力支持公共交通及其附属服务的发展,电子站牌等技术已经比较成熟.在瑞士,公交车系统非常完善,其信息提供系统较为全面,车辆发车间隔短、利用率高,其到站时间发布的误差在1 min之内.国内各大高校的研究集中于理论算法上,杨兆升在智能交通关于综合路段的行程时间预测研究上奠定了理论基础[5],于滨等人提出的基于SVM和Kalman滤波器的公交车到站时间预测模型[6],充分说明利用支持向量机优化的 Kalman滤波器在预测公交车行程时间上具有非常精准的优越性.同济大学研制的“交通信息网格系统”在交通信息实时处理和发布上也有较强的实际意义.此外,国内许多软件公司业已着手公交信息系统的研发.在实际应用上,目前国内有部分城市如苏州、厦门已经实现了所有线路到站信息预报的覆盖并向公众发布信息,如苏州交通纵横网,由于它的查询过程依赖线路番号和站点编码,用户界面体验较差,只能反馈车辆距离目标站的站数而不提供距离、时间等预报信息,无法知晓车辆的运行、靠站状态,参考价值有限.南京、上海等城市已经在部分线路上设置了电子站牌,而重庆、广州等城市则在BRT线路上实现了车辆到站时间预测.除了苏州、厦门等城市,未见其它城市面向互联网或者乘客群体直接发布公交车到站信息.开发公交车到站时间预测系统,须权衡并满足以下要求:预测结果准确,精度较高;算法简洁,易于实现;充分利用互联网、移动网络,即时向乘客、用户提供信息.2.1 公交车到站时间的影响因素分析通常认为公交车辆运行时间分为正常运行时间和停车延误时间两个部分,停车延误时间又包括到站停车时间、交叉口随机延误、突发性事件延误等.公交车辆运行比较规范,正常运行时间里车辆的运行状态稳定,车辆运行速度总是围绕一个平均值作上下小幅波动,而停车延误时间则有很大的偶然性和随机性,通常公交车辆运行时间的比例如表1所示.(1)正常行驶时间.车辆在路段正常行驶时间是除去车辆停站、交叉口延误等路段,在道路路段行驶时所经历的时间.它的影响因素有:道路因素、车辆因素、交通因素、人的因素. (2)停车延误时间.停车延误时间是指车辆到站时间、交叉路口等待通行、突发事件停车等导致车辆停车所经历的时间.它的影响因素有:车辆到站、交叉口延误、突发事件停车.为便于计算机实现且细化路段从而提高车站间预测精度,在这里,我们将交叉口、车站、曲线点统一定义为关键点,连结两个关键点则形成路段,连结若干路段形成站间路段,连结若干站间路段则形成线路.后文的研究均基于关键点和路段.2.2 公交车辆到达时间预测模型(1)基于历史数据的预测模型.假设交通模式循环变化,历史数据是可以参考的,未来车辆运行情况与统计值的偏差不大为前提.在模型中以大量历史数据为基础,通过大量积累历史数据并进行统计和分析,得出统计层面上的公交车到达时间预测值.即算法的基线数据模型.式中 Tit为公交车在t时段从公交站点i到公交站点i+1的路段平均行程时间;Ait 为公交车从t时段出发到达公交站点i的平均行程时间;Dit为公交车从t时段出发到达公交站点i的平均滞留时间; Ai+1,t为公交车从t时段出发到达公交站点i+1的平均行程时间;T为一天所分割的时段数;N为公交车站点数.式中 Ajtk为公交车k从t时段出发到达公交站点j的平均行程时间;Amtk为公交车k从t时段出发到达公交站点m的平均行程时间.(2)其它预测模型.如Kalman滤波器模型、人工神经网络模型[7].这些模型可以由多参数变量的递推关系式表达出来,其运算结果的偏差可以层层反馈至顶端,因此它对非线性关系的预测有非常好的效果,然而其复杂的运算量并不适合访问量大的面向用户的在线预测,复杂性可达O(nk),国外的一些实践也表明基于Kalman滤波器模型、人工神经网络模型在线预测上存在迟滞、运算量大等问题,并不适合面向广大用户的在线预测和信息发布.(3)本系统采用的模型.系统采用历史统计量作为基线数据,车辆即时运行状态作一阶偏移量修正,GPS获得的瞬时速度对运行位置进行加乘,其流程如下.①历史数据模型和其统计量作为基线数据.②基线数据对于前一时刻车辆运行行程车速的修正.综合当前公交车k的运行状况针对基线数据的偏移量得出修正值,并在下一时刻的预测中利用修正值进行调值.式中 Vrl为预测时刻l时采用的修正后的公交车行程车速;Vblt为时刻l所在时段t 的基线行程车速; Vtrim为即时修正值,实时变量,等于上一时刻的实际行程车速减去上一时刻的基线行程车速.③基线数据修正后对于瞬时车速的加乘.基线数据及其修正的本质是行程车速,而GPS则可以获得当前时刻车辆的瞬时车速.瞬时车速的引入可满足突发事件下的时间预测,称为实时计算速度[2].式中 SM2为M-1站至M站之间未行使的路程; Si为第i站与i+1站之间的路程.2.3 公交车辆有限状态机有限状态机(Finite State Machine,FSM)[8],是一种在电路和控制领域里应用非常广泛的模型,在新兴的计算机科学和软件工程领域内也得到了充分应用和发展,它是面向对象的软件工程基础之一.类和对象是面向对象编程的基本概念,面向对象的设计可以非常好地实现这一模型,其核心包括状态和转换.公交车辆是系统中的核心实体.与客户和服务器基于请求与响应的简单交互过程不同,公交车辆包含了实时、监控、数据存储、动作、转换、状态等很多方面,其状态转换如图1所示.运行中的每一辆公交车在系统内存中都能找到一个实例与之一一对应,如图2所示,可以监控每一辆线上公交车的状态,以便实时计算用.式中 vc为实时计算速度;Vins为瞬时行驶速度; Si1,Si2为路段i上已行驶的和未行驶的路程.当车辆越靠近上一站点时,实时计算速度越依赖于修正后的基线数据,车辆越靠近下一站点时,实时计算速度越依赖于瞬时速度.④通过实时计算速度vc预测从M-1站出发到达车站j的时间Ajtk2.4 基线数据存储、挖掘和应用设系统运行时间内的基线时间段组成为式中 Tn为基线时间段;tn为基线分界时刻;tb为基线时刻设不同日期的不同样本车辆为i,j,则∀tb, i∈ Tn,tb,i-tb,j< tthreshold.①对于关键点编号为pointID的停靠时间.式中 tstop,pointID为编号为pointID的关键点停靠时间;tleave为编号为pointID的关键点的发车时刻; tarrive为编号为pointID的关键点的到达时刻;n为在有记录的日期范围内,pointID的记录总数.②对于关键点编号为pointID和pointID+1之间路段的运行时间式中 tline,lineID为编号为 lineID的路段的运行时间;tarrive,pointID+1为编号为pointID+1的关键点的到达时刻;tarrive,pointID为编号为 pointID的关键点的发车时刻.2.5 其它算法(1)车辆及所在路段的判定.①通过经纬度计算两点间距离式中 (xo,yo),(xe,ye)分别为任意两点的经纬度(用角度表示)[9].②通过判断距离来判断公交车是否在该路段式中 i表示公交车所在的一点;假设i点位于路段oe上,则Loi表示从这一路段起点到i点的距离; Lie表示从i点到这一路段终点之间的距离;Loe表示这一路段起点和终点之间的距离.若i点位于路段oe上,则必然有d=0.事实上位置信息必然有误差,因此须对d取阈值.③判断公交车是否到达关键点式中 l表示设定的允许公交车辆与关键点间距离偏移的阈值;(xpoint,ypoint)为关键点的经纬度; (xbus,ybus)为公交车辆的经纬度.(2)距离请求站点的最近车辆搜索.从请求站点起始,逆向查找法,直到查找到有车辆的存在即停止查找,返回该车辆信息. 系统采用Java语言编写.服务器采用 servlet和JSP运行在Tomcat7.0上[10],如图3所示.系统架构如图4所示,数据库采用MySQL和SQLite,客户端采用Android2.3开发,应用了软件工程[11]原理,开发了手机客户端如图5所示.将上述信息汇总如表2所示.在大连市101路公交车上进行了软件工程开发的单元测试和集成测试[10],表明算法的可靠性;在大连市旅顺口区18路公交车上进行了实践性验证,如图6所示.实验将全线分成48个路段,图中抽取了关键点编号为31~40的典型路段在不同时段的预测情况,以及预测值和实际值的差值.定义差值的绝对值为误差,则计算48个路段的平均误差22.563 s.上述误差源于车辆到达信号控制交叉口所受延误的随机性,以及站点上下车乘客数的随机特征.图6中编号为31的交叉口,即兴发路与海慧街信号控制交叉口,其误差较大主要是由于信号控制交叉口所产生的延误所致.本文算法基于实时状态不断修正,预测值将始终围绕实际值呈波动性收敛.为评价预测结果的可接受度,引入北京公交集团“快一慢二”(即车辆早到1 min、晚到2 min以内为准点)的“准点率”概念,将预测值作为发布给使用者的时刻表,评价车辆到达与时刻表的符合度.统计分析表明,在48段数据中,仅1组非准点,其准点率达97.9%.满足预测的精度目标.本文以面向乘客发布公交车到站时间预测信息,直观地向乘客手机客户端发送目标线路上车辆到站情况的预测信息为切入点,研究了用户在上车前对未知车辆到站时间的预测,其结果可以应用在电子站牌、手机终端、互联网发布上,且同样适用于预告乘客所在车辆到目标车站的时间.鉴于其数据采集、分析、管理和应用上有相当程度的交叉和相互协调性,应把公交车到站时间预测和公交车GPS自动报站、公交车GPS监控和调度、公交车拥挤度检测、公交路线查询等模块为子系统纳入一个综合的公交车信息系统工程.在交通信息平台的架设上,本文为智能交通管理方面提供了很好的思路和模式.【相关文献】[1]张汝峰.我国城市公共交通优先发展对策研究[D].济南:山东大学,2010.[ZHANG R F.Study on the counter-policies for city public transport priority in China[D].Jinan:Shandong University,2010.][2]张迅.城市公共交通出行者信息服务选择行为研究[D].大连:大连理工大学,2010.[ZHANG X. Modeling information service choice behavior of urban transitpassengers[D].Dalian:Dalian University of Technology,2010.][3]罗虹.基于GPS的公交车辆到达时间预测技术研究[D].重庆:重庆大学,2007.[LUO H.Study on bus arrival time prediction based on GPS sata[D]. Chongqing:Chongqing University,2007.] [4]Lin W,Jian Z.An experimental study on real time bus arrival time prediction with GPS data.In TRB 78th annual meeting(CD-ROM),Washington,D.C.1999.[5]杨兆升,高学英.基于影响因素分类的路段行程时间融合研究[J].公路交通科技,2010,27(4): 116-127.[YANG Z S,GAO X Y.Research on travel time fusion based on influencing factors classification [J].Journal of Highway and Transportation Research and Development,2010,27(4):116-127.][6]于滨,杨忠振,曾庆成.基于SVM和Kalman滤波的公交车到站时间预测模型[J].中国公路学报, 2008,21(2):89-90.[YU B,YANG Z Z,ZENG Q C.Bus arrival time prediction model based on support vector machine and Kalman filter[J].China Journal of Highway and Transport,2008,21(2):89-90.][7]李劲,黄大荣.基于粗糙神经网络的交通优化控制模型[J].计算机工程与应用,2008,44(19):215-219.[LI J,HUANG D R.Traffic-flow optimal control model in intelligent transportsystems:Rough neural network approach[J].Computer Engineering and Applications,2008,44(19):215-219.][8]魏先民.有限状态机在嵌入式软件中的应用[J].潍坊学院学报,2006,6(4):24-25.[WEI X M. Finite state machine in embedded software application [J].Journal of WeifangUniversity,2006,6(4):24-25.][9]胡伍生,高成发.GPS测量原理及其运用[M].北京:人民交通出版社,2002.[HU W S,GAO C F. GPS measuring principle and application[M]. Beijing:China Communications Press,2002.] [10]郝玉龙.Java EE编程技术[M].北京:北京交通大学出版社,2008.[HAO Y L.Java EE programming techniques[M].Beijing:Beijing Jiaotong University Press,2008.][11]Stephen Schach.Object-oriented and classical software engineering(Seventh Edition)[M].New York City: McGraw-Hill,2008.。
- 1、下载文档前请自行甄别文档内容的完整性,平台不提供额外的编辑、内容补充、找答案等附加服务。
- 2、"仅部分预览"的文档,不可在线预览部分如存在完整性等问题,可反馈申请退款(可完整预览的文档不适用该条件!)。
- 3、如文档侵犯您的权益,请联系客服反馈,我们会尽快为您处理(人工客服工作时间:9:00-18:30)。
Bus Arrival Time Prediction Method forITS ApplicationBongsoo Son1, Hyung Jin Kim1, Chi-Hyun Shin2, and Sang-Keon Lee31Dept. of Urban Plannung and Engineering, Yonsei University, Seoul, Korea{sbs,hyungkim}@yonsei.ac.kr2Dept. of Transportation Engineering, Kyonggi University, Kyunggi-do, Koreachshin@kyonggi.ac.kr3Korea Research Institute For Human Settlements, Kyunggi-do, Koreasklee@krihs.re.krAbstract. It is known that stoppage times at signalized intersections cause thebiggest errors in bus arrival time prediction for real-time Bus Information Sys-tem (BIS) services and no particular method is proven successful so far. Thisstudy developed a prediction method that compares the predicted bus traveltimes from bus stop to the stop line at signalized intersections by using Kalmanfiltering technique with the state of green time indications of traffic signals, andthen incorporates possible stoppage into a next link travel times. From fieldsurveys and in-lab simulation, the proposed method was found superior to otherconventional techniques showing an average of more than 200% improvementin prediction accuracy.1 IntroductionIn order to combat the ever-increasing congestion in urban area, traffic officials and agencies have embraced Intelligent Transportation Systems (ITS) and technologies in a proactive, systematic way. In recent years interests has grown for granting preferen-tial service to public transport such as buses, since it is desirable to favor public transit over private auto travel due to the basic role of public transport in the city. A number of different public bus preferential treatments have been implemented in many urban areas around the world to offer better service to public buses than private autos. Among bus preferential schemes such as bus gate, bus malls, bus lanes, BIS, bus pri-ority signals, etc., bus information system is one utilized in many cities to balance public and private transport. In fact, the availability of accurate, real-time information is especially useful to operators of vehicle fleets as well as passengers.However, BIS has not much impacted on the reliability of bus arrival time infor-mation. The reason for uncertainty in predicted arrival times of public buses in BIS are waiting times for green phase at the signalized intersections, dwell-times at bus stops, delays resulted from incidents, and so on. Reliability of prediction has increased recently through numerous prediction methods that try to take some of these reasons into considerations [1, 2].Bus Arrival Time Prediction Method for ITS Application 89 As can be seen from Figure 1, it is well known that waiting times at the signalized intersection cause major errors in forecasting of bus arrival times. In the figure, bus travel trajectories between bus stop i-2 and bus stop i are not constant. More specifi-cally, bus type I is associated with the buses arrived at the traffic signal i-1 during the red time period. Bus type II represents the buses arrived at the signal during the period between ending of red time and beginning of green time and experienced delay for passing the traffic signal. Bus type III is related to the buses passed the traffic signal without any delay. The three types of bus trajectories indicate that the bus arrival times at bus stop i are widely different and greatly dependent upon whether or not buses await at traffic signal i-1, so bus travel times between the two bus stops significantly vary depending upon the state of signal i-2.Fig. 1. Time-space diagram for bus trajectoriesFigures 2 and 3 show the bus travel times measured between bus stop i-2 and traf-fic signal i-1 and between traffic signal i-1 and bus stop i, respectively, during the same time period. The bus travel times between bus stop i-2 and traffic signal i-1 are severely fluctuated, while those between traffic signal i-1 and bus stop i are relatively somewhat stable. The main cause of travel time fluctuation is the fact how long the bus does wait at traffic signal i-1. From the two figures, it is very clear that that waiting times at the signalized intersection cause major errors in forecasting of bus arrival times.Up to date there is no practical and easy method that accounts for the impact cre-ated by traffic signal operation along the bus routes. This paper is an attempt to im-prove the reliability of predicted bus travel time in urban signalized intersection net-works. In the paper, we have come to the result that we can figure out the waiting times at signalized intersections under the Time-Of-Day (TOD) type operation by comparing the state of signals at that very instant if the bus arrival times at the stop line of a signalized intersection are reasonably estimated. The prediction method is described below. The data for this paper came from several sources: specific sources will be given when individual data are discussed in Section 3.90B. Son et al.Fig. 2. Real data for bus travel times between bus stop i-2 and traffic signal i-1Fig. 3. Real data for bus travel times between traffic signal i-1 and bus stop i2 The Prediction MethodThe method proposed in this paper is based upon two forecasted link travel times of buses: one from a bus stop to a stop line of intersection and another from the stop line to the next downstream bus stop which is supposed to display the remaining time until a next bus of a particular bus line arrives. Conventional techniques have usually con-sidered the distance between two successive bus stops as one link. (See Figure 4)Bus Arrival Time Prediction Method for ITS Application 91GPS SignalThe information of local bus arrival times between consecutive bus stops needed for the prediction of link travel times can be obtained from ITS devices such as GPS devices with wireless data communication modules atop buses. Then, the GPS based modules have an electronic road map and do map-match for bus stops in order to transmit data. Each link travel time is predicted using Kalman Filtering in which state equations by time are formulated [3]. A particular concern is the link travel time from signalized intersections to immediate downstream bus stop for which two state equa-tions were developed to differentiate signal-awaiting trips at the signalized intersec-tions from non-stop trips.The computer algorithm used for this method works on a rigid space-time grid. For the analysis, the site interested are broken into sections bounded by grid points {i, i-1, . . . , i-n} located at each bus stop and at each stop line of signalized intersection a shown in Figure 2. The computational procedure starts at the most upstream point of the site interested, and works downstream (i.e., the bus traveling direction).Figure 5 is a schematic diagram of the computational procedure. In the figure, amount of waiting times at each signalized intersection can be estimated by comparing the predicted bus arrival times at the subject stop lines with the state of signals (i.e., Fig. 5. Schematic diagram of bus travel time computation procedureB. Son et al.92Time-Of-Day signal operation time schedule) at that very instant. For the TOD type signalized intersection, the signal operation time schedules are readily available from the traffic officials and agencies. When stopping at signal is anticipated, the waiting time is then added to the next link travel time that is also predicted. Otherwise, the predicted bus arrival time is determined by the next link travel time, where the next link travel time can be estimated based on the information associated with the pro-ceeding buses traveled on the downstream link at the same time period.3 The Data SetThe study site is located near Seoul Metropolitan Area. The study site is an 8-lane busy urban arterial highway and approximately 2 km long with four bus stops and three-signalized intersections. The route is therefore segmented into 6 links with consecutive links sharing start and end nodes. The links range from 0.3 to 1.0 km in length. A total of sixteen different bus- lines are in operation along this route. Among them, three bus-lines are currently in BIS service.Table 1. Real data set for the bus arrival times collected from the field by manual Bus stop I – 2 Traffic signal i-1Number of bus line Arrival time Number of buslineArrival timeTravel timefrom bus stopi-2 to signal i-1333 8:00:00 333 8:02:06 0:01:56 51 8:00:28 51 8:02:19 0:01:51 820 8:00:37 820 8:02:20 0:01:43 57 8:00:56 57 8:02:28 0:01:32 736-1 8:03:27 736-1 8:05:27 0:02:00 700 8:04:58 700 8:05:38 0:00:40 1116 8:05:40 1116 8:06:00 0:00:20 333 8:06:03 333 8:08:18 0:02:15 220 8:06:22 220 8:08:27 0:02:05 51 8:08:53 51 8:09:16 0:00:23 17 8:11:39 17 8:14:18 0:02:39 220 8:11:54 220 8:12:09 0:00:15 820 8:12:11 820 8:14:23 0:02:12 116-3 8:12:29 116-3 8:14:30 0:02:01 57 8:14:37 57 8:14:56 0:00:19Bus Arrival Time Prediction Method for ITS Application 93The GPS data for the real time bus locations for the three bus-lines were obtained from SK-Entrac that the private agency provides BIS service in Korea. For the other bus-lines, the bus arrival times at signalized intersections and bus stops were directly collected from the field by manual for the three weekdays from October 14, 2003 to October 16, 2003 during the time period from 08:00 to 12:00. The signal operation time schedules were obtained from the police station. It should be noted that the signalized intersections are operated and/or controlled by the police in Korea.Table 1 shows real data set for the bus arrival times collected at two consecutive bus stops during the peak time period between 08:00 and 08:15. Average headway of buses during peak period between 08:00 and 10:00 is 44 seconds, and 57 seconds during non-peak period between 10:30 and 12:00.4 Validation TestFor the model validation test, the accuracy of the results obtained from the proposed method was measured by using three statistical errors such as Mean Relative Error (MRE), Root Squared Error (RSE) and Maximum Relative Error (MAX). Besides, the performances of proposed method were compared with those of four conventional methods such as 1) moving average method, 2) exponential smoothing method, 3) autoregressive moving average method (ARIMA) and 4) Kalman Filtering method. For these purposes, predicted bus arrival times obtained by using the four conven-tional techniques and the proposed method were compared with observed arrival times obtained from the field measurements.The results for the statistical analysis of the five methods were summarized in Table 2. As shown in the table, the proposed method is superior to the four conven-tional methods showing an average of more than 200% improvement in prediction accuracy. Among the conventional methods, Kalman Filtering method has produced the better results than the other three methods. The other methods show the same level of prediction performances in terms of statistical accuracy.Table 2. The statistical analysis results for the prediction techniques’ performancesPeriodError IndicesMoving Average Exponential Smoothing ARIMAKalman Filtering ProposedMethodMRE 0.1814 0.1809 0.19290.12800.0416 RSE 0.2163 0.2220 0.22320.15840.0586 Peak a.m. 8:00~9:00MAX 0.5703 0.5849 0.64240.32560.1400 MRE 0.2118 0.2121 0.20220.13950.0430 RSE 0.2461 0.2461 0.24160.17270.0546 Non-peak a.m. 10:30~11:30MAX0.6503 0.6056 0.62890.44490.1069B. Son et al.94Since most of signalized intersections along urban streets operate with cycle lengths of 120 seconds or more, the instance of waiting for the next green phase or non-stop passage of buses at critical intersections would create difficulty in bus arrival time forecasting efforts. It was concluded that the proposed method can offer prompt bus arrival time to bus passengers waiting at bus stops with relatively higher accuracy. Applicability of the proposed model is considered much higher in non-peak time and nighttime BIS service operation.5 ConclusionBIS is one utilized in many cities to balance public transport and private transport. The availability of accurate, real-time information is especially useful to operators of vehicle fleets as well as passengers. However, BIS has not much impacted on the reliability of bus arrival time information. It is well known that waiting times at the signalized intersection cause major errors in forecasting of bus arrival times. This paper is an attempt to improve the reliability of predicted bus travel time in urban signalized intersection networks. The emphasis of the proposed method is on estimat-ing waiting times at signalized intersections under the TOD type operation by compar-ing the state of signals at that very instant.The performances of the method proposed in this paper were measured by using three statistical errors such as MRE, RSE, and MAX. The performances of proposed method were also compared with those of four conventional methods by using the observed arrival times obtained from the field measurements. The proposed method is superior to the four conventional methods showing an average of more than 200% improvement in prediction accuracy. Among the conventional methods, Kalman Filter-ing method has produced the better results than the other three methods.References1.Wei-Hua Lin and Jian Zeng, “An Experimental Study on Real Time Bus Arrival Time Pre-diction with GPS Data”, Virginia Polytechnic Institute and State University, 1999.2.Amer Shalaby and Ali Farhan, “Bus Travel Time Prediction Model for Dynamic Opera-tions Control and Passenger Information System”, Transportation Research Board, 2003. 3.Grewal, M.S. and Andrews, A.P., “Kalman Filtering Theory and Practice”, Prentice Hall,1993.。