Molecular Modeling
计算化学简介

计算化学基本概念分子模拟(Molecular Modeling)泛指用于模拟分子或分子体系性质的方法,定位于表述和处理基于三维结构的分子结构和性质。
Quantum Mechanics (QM) 量子力学Molecular Mechanics (MM) 分子力学Theoretical Chemistry 理论化学Computational Chemistry 计算化学Computer Chemistry 计算机化学Molecular Modeling 分子模拟量子化学简介量子化学的研究范围和内容9稳定和不稳定分子的结构、性能,及其结构与性能之间的关系9分子和分子之间的相互作用9分子和分子之间的相互碰撞和相互反应等问题计算与预测各种分子性质(如分子几何构型、偶极矩、分子内旋势能、NMR、振动频率与光谱强度)预测化学反应过程中的过渡态及中间体、研究反应机理理解分子间作用力及溶液、固体中的分子行为计算热力学性质(熵、Gibbs函数、热容等)量子力学与经典力学的差别首先表现在对粒子的状态和力学量的描述及其变化规律上。
在量子力学中,粒子的状态用波函数来描述,它是坐标和时间的复函数。
为了描述粒子状态变化的规律,就需要找出波函数所满足的运动方程。
这个方程是薛定谔在1926年首先提出的,被称为薛定谔方程。
求解薛定谔方程,即可从电子结构层面来阐明分子的能量、性质及分子间相互作用的本质。
Schrödinger 方程The ab initio Molecular Orbital TheoryThe Hartree-Fock EquationThe Self-Consistent Field TheoryLinear Combination of Atomic OrbitalsBasis Sets: Slater-Type Orbitals(STO) and Gaussian-Type Orbitals(GTO) 当我们决定由原子轨道线性组合成分子轨道时,就要考虑采取什么数学形式来表示原子轨道。
Lec 00 概述
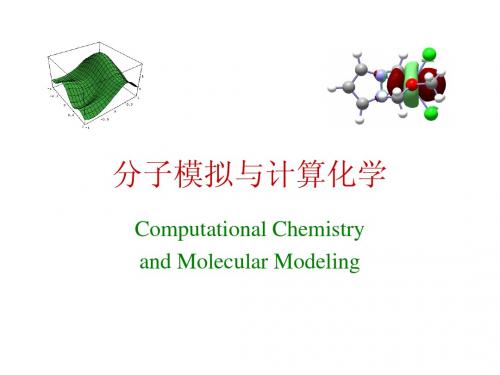
必备条件
量子化学基础
学习计算化学, 需要一些必要的知识和条件, 大家还要边学习, 边做练习, 这样才能真正理解各种概念和理论的含义.
一般而言, 学习需要一定的量子化学基础, 但是大家学习过结构化学, 知 道薛定谔方程, 电子组态也就足够了.
PC机
计算机是必须的, 软件大家自己准备,
熟悉Windows以及Linux操作系统
•需要很多经验参数, 这些经验参数需要仔细测试和校准
分子力学方法
• 计算成本低
因为其对象仅仅是原子及其化学键, 因此计算成本低, 可以计算大体系, 应 该在10万个原子左右。
• 只能得到稳定几何结构
• 但是由于分子力学方法依赖于力场模型, 同时又有很多经验参数, 因此其得 到的几何结构是粗略的, 相当不准确的。
• 学习分子模拟与计算化学的目的是为了解 决化学问题 • 1:集中讲授计算化学的理论体系。 • 2:确定研究课题,熟悉计算软件。 • 课程讨论与考核:
– 进行课程讨论占20分。 – 课程研究总结占40分。 – 理论考核占40分
参考资料
• 量子化学: 基本原理和从头计算法(上,中册), 徐光宪, 黎乐民 • Quantum Chemistry, 第五版, I. Levine • Molecular Modeling – Principles and Applications, A. R. Leach (Addison Wesley Longman) • Computational Chemistry – Introduction to the theory and applications of molecular and quantum mechanics, E. Lewars (Kluwer) • A Chemist’s Guide to Density Functional Theory, W. Koch and M. C. Holthausen (Wiley)
MOE-基于结构的药物设计及在药物发现方面的应用

药物发现/设计
基于大分子结构的 基于小分子配体的 基于片段的
上市
转化医学
药代动力学 吸收/分布/排除 代谢 毒性检测 先导化合物优化 临床数据管理 2010 © CloudScientific All Rights Reserved 临床数据统计分析
药物开发 (临床前及临床试验)
about moe
2010 © CloudScientific All Rights Reserved
我们代理如下公司产品在中国的业务
ChemAxon
2010 © CloudScientific All Rights Reserved
我们提供的解决方案
生物学
生物信息学 基因组学 蛋白组学 结构生物学 信号转导通路
about cloudscientific
2010 © CloudScientific All Rights Reserved
Best Solutions for Best Research
公司介绍
上海康昱盛信息科技有限公司
2010 © CloudScientific All Rights Reserved
2010 © CloudScientific All Rights Reserved
我们能为您提供以下方面的价值
• •
产品: 世界领先的生命科学软件产品 解决方案:针对客户的专业需求,提供专业的产品组合建议和科学应 用方面咨询,并可做客户化的定制开发
•
培训: 不仅为客户提供产品定制的专业培训,并且定期邀请行业专家 提供专业知识和行业进展的高级培训
2010 © CloudScientific All Rights Reserved
Molecular Operating Environment MOETM
分子动力学软件选择

分子动力学软件选择There are widely used packages like AMBER, CHARMm and X-PLOR/amber/amber.html//CHARMm and X-PLOR both use the same forcefield. Amber's is different.If you're Wintel-bound, you could try Hyperchem, which has a free downloadable demo: /products/hc5_features.htmlIt has a nice structure build capability (the other packages havepowerful languages, but can be intimidating to new users).OpenSource adherents can find a wealth of free packages at SAL, anexcellent site:/Z/2/index.shtmlMy personal favourites are MMTK, EGO and VMD/NAMD.I compiled a list of free and commerical programs at/chemistry/soft_mod_en.htmlmodeling in solution is possible e.g. with these programs (to the best of my knowledge):commercial: AMSOL, GROMOS, Titanfree: GAMESOL, GROMACS, MOIL, OMNISOL, TinkerYou find links to all of these programs at/chemistry/soft_mod_en.htmlPAPA (计算粒状物料的三维并行分子动力学计算程序)【URL】http://www.ica1.uni-stuttgart.de/Research/Software_P3T/papa.html【作者】 ICA 1 Group, Institute of Computer Applications (ICA) of the University of Stuttgart【语言版本】 English【收费情况】免费【用途】 Characteristic:dissipative interaction for rotating, rough, spherical particlesgeometry elements: walls, cylinders, spheres, etc freely configurablematerial properties of walls and particles freely configurable for an arbitray number of materialsobject oriented, written in C++full checkpointing supportedseveral compilation options: support of X11 graphics, reduction to 2D, debugging aids, etc. Applications:simulation of granular media, silo filling and steady flow problems, sphere packings of mono- and polydisperse systemProtoMol (分子动力学并行计算软件)【URL】/~lcls/Protomol.html【作者】 LCLS Group at the University of Notre Dame【语言版本】 English【操作系统】 SunOS 5.8, IRIX 6.5, Linux 2.4, AIX 5.1【收费情况】免费【用途】 PROTOMOL is an object-oriented component based framework for molecular dynamics simulations. The framework supports the CHARMM 19 and 28a2 force fields and is able to process PDB, PSF, XYZ and DCD trajectory files. It is designed for high flexibility, easy extendibility and maintenance, and high performance demands, including parallelization. The technique of multiple time-stepping has been used to improvelong-term efficiency, and the use of fast electrostatic force evaluation algorithms like plain Ewald, Particle Mesh Ewald, and Multigrid summation further improves performance. Longer time steps are possible using MOLLY, Langevin Molly and Hybrid Monte Carlo, Nose-Hoover, and Langevin integrators. In addition, PROTOMOL has been designed to interact with VMD, a visualization engine developed by the University of Illinois that is used for displaying large biomolecular systems in three dimensions. PROTOMOL is free distributed software, and the source code is in cluded.【相关链接】VMD (分子可视化软件)美国圣母大学:计算生命科学实验室Claessen站点的分子模型化软件【URL】/chemistry/soft_mod_en.html【简介】Molecular ModelingCommercial Software3D Viewer: converts 2D structures into 3D with simple MM2Alchemy 2000: semi empirical, QSAR, Protein, Polymer, LogPAMPAC: semiempirical quantum mechanical programAMSOL: semi empirical, solvation models for free energies of solvation in aqueous solutions and in alkane solventsPersonal CAChe: visualize molecules in 3D, search for conformations, analyze chemical reactivity and predict properties of compoundsQuantum CAChe: Personal CaChe plus molecular dynamics and semi-empirical MOPAC and ZINDO quantum mechanicsChem3D: MOPAC and Gaussian integration, ChemProp, ...Gaussian 98W: MP2, MP3, MP4, MP5, HF, CASSCF, GVB, QCISD, BD, CCSD, G1, G2, ZINDO, ONIOM calculations, DFT excited states, VCD intensities, ...GROMOS: general-purpose molecular dynamics computer simulation package for the study of biomolecular systemsHyperchem Suite: semi empirical, RMS Fit, Molecule Presentations, Sequence Editor, Crystal Builder, Sugar Builder, Conformational Search, QSAR Properties, ScriptEditor ...(Hyperchem Pro, Hyperchem Std.)Jaguar: electronic structure calculationMacroModel: allows the graphical construction of complex chemical structures mechanics and dynamics techniques in vacuo or in solutionMOPAC 2000: the latest version of MOPACSpartan: MM, semiempirical, ab initio, DFT, ...Titan: TITAN is the union of Wavefunction's versatile, easy-to-use interface with fast, computational algorithms from Schr鰀inger's JaguarWinMOPAC: based on MOPACShareware/Freeware3D Viewer for ISIS Draw: converts 2D structures into 3D with simple MM2Biomer: online java applet, model builders for polynucleotides (DNA/RNA), polysaccharides and proteins, interactive molecule editor, AMBER force-field based geometry optimization, simulated annealing with molecular dynamics, and the ability to save gif, jpeg, and ppm imagesChem3D Net: demo version of Chem3DCOLUMBUS: high-level ab initio molecular electronic structure calculationsDalton: quantum chemistry programGAMESOL: calculate free energies of solvation based on fixed, gas-phase solute geometries interfacing GAMESSGAMESS: General Atomic and Molecular Electronic Structure System is a general ab initio quantum chemistry packageGaussian Basis Set: get any Gaussian basis set you can imagineGROMACS: fully automated topology builder for proteins, molecular dynamics, leap-frog integrator, position langevin dynamics, normal mode analysis, electrostatics,non-equilibrium MD, NMR refinement with NOE data, large number of powerful analysis tools, ...Hückel: constructs the Hückel matrix, the programs then calculate, displayMOIL: molecular modeling, energy minimization and molecular dynamics simulation for biomolecules like proteinsMoldy: molecular dynamics simulation program, liquids, solids, rigid surfacesMOPAC: general purpose semiempirical molecular orbital package for the study of chemical structures and reactionsMOPAC 5.08mn: modified version of MOPACNWChem: quantum package for supercomputers and Linux, SCF, RHF, UHF, DFT, CASSCF, interface to Python programming languageOMNISOL: calculating free energies of solvation for organic molecules containing H, C, N, O, F, S, Cl, Br, and I in water and organic solventsPC GAMESS: GAMESS for the Intel communityQ: molecular dynamics package designed for free energy calculations in biomolecular systemTinker: molecular modeling software is a complete and general package for molecular mechanics and dynamicsVMD (分子可视化软件)【URL】/Research/vmd/【作者】 Biophysics Group,University of Illinois at Urbana-Champaign (UIUC)【语言版本】 English【收费情况】免费【用途】 VMD is a molecular visualization program for displaying, animating, and analyzing large biomolecular systems using 3-D graphics and built-in scripting. VMD supports computers running MacOS-X, Unix, or Windows, is distributed free of charge, and includes source code.VMD is designed for the visualization and analysis of biological systems such as proteins, nucleic acids, lipid bilayer assemblies, etc. It may be used to view more general molecules, as VMD can read standard Protein Data Bank (PDB) files and display the contained structure. VMD provides a wide variety of methods for rendering and coloring a molecule: simple points and lines, CPK spheres and cylinders, licorice bonds, backbone tubes and ribbons, cartoon drawings, and others. VMD can be used to animate and analyze the trajectory of a molecular dynamics (MD) simulation. In particular, VMD can act as a graphical front end for an external MD program by displaying and animating a molecule undergoing simulation on a remote computer. VMD uses OpenGL to provide high performance 3-D molecular graphics【相关链接】RasMol:3D分子结构显示程序PDB文件显示程序KineMage美国伊利诺依大学:理论生物物理学研究组JMV (Java分子可视化工具)ProtoMol (分子动力学并行计算软件)ORAC (用于模拟溶剂化生物分子的分子动力学计算程序, 意大利佛罗伦萨大学)【URL】http://www.chim.unifi.it/orac/【作者】 Massimo Marchi and P. Procacci【语言版本】 English【操作系统】 UNIX【收费情况】免费【用途】 ORAC is a program for running classical simulations of biomolecules. Simulations can be carried out in the NVE, NPT, NHP, and NVT thermodynamic ensembles. The integration of the equations of motion in any ensemble can be carried out with the r-RESPA multiple time step integrator and electrostatic interactions can be handled with the Smooth Particle Mesh Ewald method.【备注】A parallel version of ORAC4.0 (MPI/T3E) is available upon request to:Massimo MarchiSection de Biophysique des Proteines et des Membranes,DBCM, DSV, CEA, Centre d'Etudes,Saclay, 91191 Gif-sur-Yvette Cedex, FRANCEVirtual Molecular Dynamics Laboratory (分子动力学软件)【URL】/vmdl/index.html【作者】 Amit Bansil, Lidia Braunstein【语言版本】 English【收费情况】免费【用途】 The Virtual Molecular Dynamics Laboratory enables the student to visualize atomic motion, manipulate atomic interactions, and quantitatively investigate the resulting macroscopic properties of biological, chemical, and physical systems.The Virtual Laboratory is a suite of research-based molecular dynamics software toolsand project-based curriculum guides. The software tools are: "Simple Molecular Dynamics (SMD)", "Universal Molecular Dynamics", and "Water".【相关链接】美国波士顿大学聚合物研究中心(可视化模拟)DL_POLY (分子动力学模拟软件)【URL】/msi/software/DL_POLY/【作者】 W. Smith and T.R. Forester【语言版本】 English【收费情况】免费DL_POLY is supplied to individuals under a licence and is free of cost to academic scientists pursuing scientific research of a non-commercial nature. A group licence is also available for academic research groups. All recipients of the code must first agree to the terms of the licence.Commercial organisations interested in acquiring the package should approach Dr. W. Smith at Daresbury Laboratory in the first instance. Daresbury Laboratory is the sole centre for distribution of the package.【用途】 DL_POLY is a general purpose serial and parallel molecular dynamics simulation package originally developed at Daresbury Laboratory by W. Smith and T.R. Forester under the auspices of the Engineering and Physical Sciences Research Council (EPSRC) for the EPSRC's Collaborative Computational Project for the Computer Simulation of Condensed Phases (CCP5) and the Molecular Simulation Group (MSG) at Daresbury Laboratory. The package is the property of the Central Laboratory of the Research Councils.Two versions of DL_POLY are currently available. DL_POLY_2 is the original version which has been parallelised using the Replicated Data strategy and is useful for simulations of up to 30,000 atoms on 100 processors. DL_POLY_3 is a version which uses Domain Decomposition to achieve parallelism and is suitable for simulations of order 1 million atoms on 8-1024 processors.DL_POLY (分子动力学模拟软件)【URL】/msi/software/DL_POLY/【作者】 W. Smith and T.R. Forester【语言版本】 English【收费情况】免费DL_POLY is supplied to individuals under a licence and is free of cost to academicscientists pursuing scientific research of a non-commercial nature. A group licence is also available for academic research groups. All recipients of the code must first agree to the terms of the licence.Commercial organisations interested in acquiring the package should approach Dr. W. Smith at Daresbury Laboratory in the first instance. Daresbury Laboratory is the sole centre for distribution of the package.【用途】 DL_POLY is a general purpose serial and parallel molecular dynamics simulation package originally developed at Daresbury Laboratory by W. Smith and T.R. Forester under the auspices of the Engineering and Physical Sciences Research Council (EPSRC) for the EPSRC's Collaborative Computational Project for the Computer Simulation of Condensed Phases (CCP5) and the Molecular Simulation Group (MSG) at Daresbury Laboratory. The package is the property of the Central Laboratory of the Research Councils.Two versions of DL_POLY are currently available. DL_POLY_2 is the original version which has been parallelised using the Replicated Data strategy and is useful for simulations of up to 30,000 atoms on 100 processors. DL_POLY_3 is a version which uses Domain Decomposition to achieve parallelism and is suitable for simulations of order 1 million atoms on 8-1024 processors.PMDS (并行分子动力学模板库)【URL】http://stencil.koma.jaeri.go.jp/【作者】 Japan Atomic Energy Research Institute【语言版本】 English【收费情况】免费【用途】 Parallel Molecular Dynamics Stencil (PMDS) is an assembly of subroutine programs for executing parallel short-range molecular-dynamics simulations of solids. PMDS is written in C language using MPI for parallelization, and is designed to separate and conceal parts of the programs for parallel algorithms such as inter-processor communications so that parallel programming for force calculation can be done in the same way as serial programming; it can be easily revised according to physical models.MDRANGE (分子动力学计算ion ranges)【URL】http://beam.helsinki.fi/~knordlun/mdh/mdh_program.html【作者】 Kai Nordlund【语言版本】 English【收费情况】免费【用途】 The official name of the program is MDRANGE. However, in the actual program files the shorter, more convenient name mdh (abbreviated from Molecular Dynamics High-energy) is used. Both names therefore (at least for now) mean exactly the same program. The program is a molecular dynamics (MD) simulation program tailored for effective calculation of ion ranges. The word effective used here must be understood in the context of high-energy molecular dynamics calculations.What it doesCalculates ion ranges in solidsCalculates deposited energiesCalculates the primary recoil spectrumObtaining stopping powers possible indirectlyIon and sample elements which can be used: anyEnergy range in which calculation can be done: roughly 1 eV/amu - 10 MeV/amuEnergy range in which use is justified: roughly 100 eV/amu - 100 keV/amuMDRANGE3.0: option for Puska-Echenique-Nieminen-Ritchie(PENR)-electronic stopping model [Sil00]. Needs charge density file from user.MDRANGE3.0: option for Brandt-Kitakawa(BK)-electronic stopping model. Needs charge density file from user.【备注】Kai NordlundAccelerator Laboratory, University of Helsinki, P.O. BOX 43, FIN-00014 Helsinki, Finland (email kai.nordlund@helsinki.fi)Car-Parrinello分子动力学(CPMD, ab-initio分子动力学计算软件)【URL】/【作者】 Jurg Hutter【语言版本】 English【操作系统】 Unix/Linux【下载】 /ftp.html【收费情况】免费【用途】泛函:LDA,LSD,GGA,自由能密度泛函。
Recent development of in silico molecular modeling for gas and

Available online at Recent development of in silico molecular modeling for gas and liquid separations in metal–organic frameworksJianwen JiangAs a new family of nanoporous materials,metal–organic frameworks(MOFs)are considered versatile materials for widespread applications.Majority of current studies in MOFs have been experimentally based,thus little fundamental guidance exists for the judicious screening and design of task-specific MOFs.With synergistic advances in mathematical methods,computational hardware and software,in silico molecular modeling has become an indispensable tool to unravel microscopic properties in MOFs that are otherwise experimentally inaccessible or difficult to obtain.In this article,the recent development of molecular modeling is critically highlighted for gas and liquid separations in MOFs.Bottom-up strategies have been proposed for gas separation in MOFs,particularly CO2capture.Meanwhile, interest for liquid separation in MOFs is growing and modeling is expected to provide in-depth mechanistic understanding. Despite considerable achievements,substantial challenges and new opportunities are foreseeable in more practical modeling endeavors for economically viable separationsin MOFs.AddressDepartment of Chemical and Biomolecular Engineering,National University of Singapore,117576,SingaporeCorresponding author:Jiang,Jianwen(chejj@.sg)Current Opinion in Chemical Engineering2012,1:138–144This review comes from a themed issue onNanotechnologyEdited by Hua Chun ZengAvailable online23rd December20112211-3398/$–see front matter#2011Elsevier Ltd.All rights reserved.DOI10.1016/j.coche.2011.11.002IntroductionDuring the past decade,metal–organic frameworks (MOFs)have emerged as a new family of nanoporous materials[1,2].In remarkable contrast to traditional inor-ganic zeolites,MOFs can be synthesized from various inorganic clusters and organic linkers,thus possess a wide range of surface area and pore size.More fascinatingly, the judicious selection of building blocks allows the pore volume and functionality to be tailored in a rational manner.With such salient features,MOFs are considered versatile materials for widespread potential applications [3,4]as illustrated in Figure1.Indeed,MOFs have been identified as a topical area in materials science and technology because of their implications for global and national economies[5].To date,thousands of MOFs have been synthesized in this vibrantfield and several(Cu-BTC,ZIF-8,MIL-53,etc.) are commercially available under the trade name Basoli-te TM[6].However,massive research efforts on MOFs have been primarily based on experiments.It is impractical to search for task-specific MOFs by trial-and-error from infinitely large number of possible candidates.Therefore, quantitative guidelines are desired for the high-throughput screening of enormous MOFs and the rational design of new MOFs towards practical applications.In this context, clear and deep microscopic understanding from a molecu-lar level is indispensable.With synergistic advances in mathematical methods,computational hardware and soft-ware,in silico molecular modeling has played an increas-ingly important role in unraveling microscopic properties in MOFs[7 ,8 ,9 ].Sophisticated modeling and simu-lation provide molecular insights that are experimentally intractable,if not impossible,thus elucidate underlying physics from bottom-up.Among many potential appli-cations of MOFs,separations are of central importance in chemical industry and have been actively investigated [10].In this article,the recent development of molecular modeling is critically highlighted for both gas and liquid separations in MOFs,and the foreseeable challenges and opportunities are discussed.Gas separationThe overwhelming majority of studies for gas separation in MOFs have been focused on CO2capture.This is because the combustion of fossil fuels produces a huge quantity of CO2emissions into the atmosphere.Carbon capture and sequestration is crucial to environmental protection and sustainable economy.As an essential pre-requisite,CO2has to be captured fromflue gas/ shifted syngas in post-/pre-combustion processes. Another important gas separation involving CO2is puri-fication of natural gas,in which impurities such as CO2 need to be separated to enhance calorie content.MOF adsorbentsMost synthesized MOFs are crystallites and tested as adsorbents for gas separation.Several reviews have sum-marized numerous experimental studies for CO2capture in MOF adsorbents[11–13].Nevertheless,nearly all these experiments examined the adsorption of pure gases (e.g.CO2,N2,CH4,and H2)due to the formidable difficulty associated with mixtures.By contrast,simu-lation can be readily used for single or multi-componentsystems.Thus,quantitative understanding of mixture adsorption in MOFs has been obtained,to a large extent,from simulation studies.Several bottom-up strategies as illustrated in Figure 2have been proposed to tune CO 2capture performance,for example,using specific MOFs with small pores,catenation,functionalization,ionic fra-meworks,exposed metals or metal doping.Yang and Zhong [14]simulated the adsorption of CO 2/CH 4/H 2mixture in two MOFs (IRMOF-1and Cu-BTC)and found pore size strongly affects separation efficiency.However,IRMOF-1and Cu-BTC do not possess iden-tical topology,leading to ambiguous interplay with the effect of pore size.In this regard,Babarao et al.[15]examined the separation of CO 2/CH 4mixture in isostruc-tural MOFs (Cu-BTC and PCN-60)and observed that the selectivity in Cu-BTC with small pores is nearly twice of that in PCN-60.This strategy of small pores is also reflected in framework catenation that can induce con-stricted pores and greater potential overlaps.For example,catenated IRMOF-13and PCN-6exhibit a larger selectivity for CO 2/CH 4mixture than non-cate-nated counterparts [15].An appealing strategy is to use ionic MOFs as demonstrated by Jiang and co-workers [16,17 ,18]for the separation of CO 2-containing mixtures.Simulation reveals that CO 2molecules are strongly adsorbed onto the ionic frameworks and nonframeworkions,and the predicted selectivity is significantly higher than in neutral MOFs and many other nanoporous materials.On the other hand,Yazaydin et al.[19]screened a diverse set of 14MOFs for low-pressure CO 2capture from flue gas combining simulation and experiment.The results show that M/DOBDC (M =Zn,Mg,Co or Ni)with high density of exposed metals strongly interact with CO 2.By physical and chemical doping,Xu et al.[20 ]estimated the separation of CO 2/CH 4mixtures in Li-modified MOF-5.Owing to the enhancement of electro-static potentials,adsorption selectivity was predicted to be much higher than in MOF-5.In a separate study,Lan et al.[21 ]simulated CO 2capture in covalent-organic frameworks doped by alkali,alkaline-earth and transition metals,and concluded that Li is the best surface modifier for CO 2capture.The strategies outlined in Figure 2have been compre-hensively discussed [24 ,25 ].Two of them (ionic fra-meworks and metal doping)appear to be more efficient to enhance CO 2capture.It should be noted that these strategies also can tune the separation of other mixtures,for example,the selectivity of alkane isomers was found to be enhanced by framework catenation [26].In a recent perspective,Krishna and van Baten [27 ]highlighted the potency of simulation in screening of best MOFs for CO 2capture and hydrocarbon separation,and they furtherRecent development of in silico molecular modeling Jiang 139Figure 1Purification Toxics RemovalDrug DeliveryFuel Cell SystemsStorageStorage and SeparationCarbon SequestrationSensingMOPWidespread potential applications of MOFs (/ees6/clathrates/index.shtml ).compared MOFs against traditional zeolites with regard to separation characteristics.As an alternative to simulation,analytical theories have been developed for gas separation in MOFs.Liu et al.[28,29]proposed a density functional theory (DFT)in 3D-nanoconfined space.The theory was applied to adsorption and separation in 3D-MOFs with complex pore networks,whereas most DFT studies are limited in simple confined geometries (e.g.slit and cylindrical pores).Good agree-ment was obtained between theoretical predictions,simu-lation and experimental data.Coudert et al.[30]developed the osmotic framework adsorbed solution theory (OFAST)in terms of a competition between host’s free energy and adsorption energy.This theory is based exclusively on pure-component adsorption and has the superior capability to describe flexible MOFs.For illustration,the authors used the OFAST to examine the effect of breathing on separation of CO 2/CH 4mixtures in MIL-53.The modeling studies discussed above for gas separation in MOF adsorbents are primarily focused on adsorption selectivity.However,several other factors (e.g.working capacity,regenerability,etc.)should be included in prac-tice as discussed by Bae and Snurr [31 ].Another crucial issue is how moisture in gas mixtures would affect sep-aration performance?From systematical simulation stu-dies in various neutral and ionic MOFs,Jiang and coworkers observed four different intriguing effects ofH 2O on CO 2capture [25 ].It is also instructive to examine structural change in flexible MOFs that might occur upon adsorption [32].The incorporation of flexi-bility to simulate structural change would need a robust force field.However,a general force field is currently unavailable for MOFs and first-principles modeling is expected to play a pivotal role [33 ].In addition,the chemical and thermal stability of MOFs are important for separation [34].A large number of MOFs are unstable in atmosphere or under moisture,which impedes their util-ization.Therefore,it is indispensable to develop molecu-lar guidelines for the design of stable MOFs.Nevertheless,unraveling what govern the stability of MOFs at a microscopic level is a challenge.MOF membranesCompared with adsorptive separation,membrane-based separation is considered to be energetically more effi-cient,lower capital cost and larger separation capability.However,the fabrication of MOF membranes is a for-midable task [35].Only in recent years,have there been active experimental endeavors to explore MOF mem-branes for gas separation [36 ].Since both equilibrium and dynamic properties are required,simulation for gas separation in MOF mem-branes is more time-consuming than in MOF adsorbents.Nevertheless,a handful of simulation studies have been reported.Keskin and Sholl [37 ]examined the separation140NanotechnologyFigure 2functionalizationmetal dopingionic frameworksexposed metalssmall porescatenationBottom-up strategies to tune CO 2capture performance.The representative MOFs are from [15,17 ,20 ,22,23].performance of diverse MOFs for CO2/CH4and CO2/H2 mixtures.They found that all the MOFs examined exhi-bit unfavorably low CO2selectivities and mixture effects play a crucial role in determining the performance.By combining simulation and IR microscopy,Bux et al.[38] simulated ethene/ethane separation in ZIF-8membrane. They found that ethane adsorbs more strongly than ethene,but ethene diffuses faster;and the interplay results in a membrane permeation selectivity for ethene. Krishna and van Baten[27 ]underlined the advantages of using simulation tools in the screening of MOF mem-branes for CO2capture.Along with considerable interest in MOF membranes, MOF-based composite membranes have received increasing attention for gas separation.In this emerging area,a handful of experiments have been conducted[39], but modeling studies are ing atomistic simulation and continuum model,Keskin and Sholl[40]attempted to select MOF/polymer membranes for high-perform-ance gas separation.A highly selective MOF was ident-ified and predicted to enhance the performance of Matrimid and other polymers for CO2/CH4separation. Chen et al.[41]proposed a composite with ionic liquid (IL)supported on IRMOF-1.The simulation reveals that ions in the composite act as favorable sites for CO2adsorption,and the selectivity for CO2/N2mixture is higher than in neat IL,IRMOF-1and many other supported IL membranes.It is worthwhile to note that defects and inter-crystalline interstices usually exist in synthesized MOF membranes. Nevertheless,most simulation studies use perfect and rigid models for MOF membranes.How to incorporate defects and interstices into practical modeling is challenging.On the other hand,theflexibility of MOF structures may have a larger influence in membrane separation than adsorbent separation[36 ],and should be implemented as well into modeling.Another essential issue is the mech-anical properties of MOFs[42].The high pressure exerted for membrane separation may distort MOF structures and deteriorate performance.It is thus crucial to quantitatively understand how pressure affects pore geometries and framework dimensionalities.For MOF-based composite membranes,microscopic insights into the interactions between MOF and other species(e.g.polymer or ionic liquid)are strikingly important and fundamental studies at a molecular level are desired.Liquid separationWhile gas separation in MOFs has been extensively investigated,endeavors for liquid separation are lagged behind[43 ].A recent trend has been to explore the use of MOF adsorbents and membranes for liquid separation. By combining chromatographic and breakthrough exper-iments,Alaerts et al.determined the adsorption and separation of ortho-substituted alkylaromatics(xylenes, ethylbenzene,ethyltoluenes and cymenes)in a column packed with MIL-53crystallites[44].Jin and coworkers tested the separation of water/organics mixtures in MIL-53membrane and observed a high selectivity for water removal from ethyl acetate solution[45].Simulation for liquid separation in MOFs is scarce owing to the significant amount of computational time required to sample liquid phase.Consequently,the microscopic understanding of liquid separation in MOFs is far from complete.To the best of our knowledge,only two simu-lation studies have been reported in this area,one for water desalination and the other for biofuel purification. Recent development of in silico molecular modeling Jiang141Figure3Selectivities of biofuel in Na-rho-ZMOF and Zn4O(bdc)(bpz)2by pervaporation[47 ].Specifically,Hu et al.[46]performed simulation on the desalination of NaCl aqueous solution through a ZIF-8 membrane by reverse osmosis.Because of the sieving effect of small apertures in ZIF-8,Na+and ClÀions could not transport through ZIF-8membrane and water desa-lination was observed.Theflux of water permeating the membrane was found to scale linearly with external pressure.In a separate study,Nalaparaju et al.[47 ] examined hydrophilic Na-rho-ZMOF and hydrophobic Zn4O(bdc)(bpz)2for biofuel purification.The selectiv-ities between water and ethanol in the two MOFs are largely determined by adsorption behavior.As indicated in Figure3,Na-rho-ZMOF is preferable to remove water, whereas Zn4O(bdc)(bpz)2is promising to enrich ethanol. The simulation provides molecular guidelines for the selection of appropriate MOFs towards efficient biofuel purification.Currently,modeling for liquid separation in MOFs is very limited.With increasing demands for clean water,liquid fuels and other liquid-based applications,more efforts are expected in order to provide deep molecular insights.A pre-requisite for liquid separation is that the MOFs used should be stable in water or other liquids[48],it is crucial to understand what factors govern the stability of MOFs, which would allow to produce stable MOFs for liquid separation.ConclusionAs a burgeoningfield,research activities in MOFs are rather hectic.In addition to enormous experimental stu-dies,we have witnessed the recent development of in silico molecular modeling for MOFs.Microscopic under-standing has been achieved for gas separation particularly CO2capture in MOFs,and bottom-up strategies have been proposed to enhance separation efficiency.How-ever,liquid separation in MOFs remains largely unex-plored at a molecular level and more endeavors are desired towards this end.It is obvious that current molecular modeling for separ-ations using MOFs is still in an infant stage.As discussed above,substantial challenges are foreseen for more prac-tical modeling and precise description.A number of issues should be considered in future modeling,such as the stability and mechanical properties of MOFs, structuralflexibility,material regenerability,and effect of moisture(in gas separation).These challenges provide new opportunities for modeling studies to unravel in-depth microscopic insights and thus provide quantitative guidelines on the rational screening and design of novel MOFs.Furthermore,for energy-efficient and cost-effec-tive separations,process requirements are essential to be integrated with material properties at a system level.In this context,molecular modeling,process optimization, as well as material synthesis,should be synergized holi-stically towards the development of best MOFs for economically viable separations and other practical appli-cations.AcknowledgementsThe author gratefully acknowledges the National University of Singapore, the Singapore National Research Foundation,and the Ministry of Education of Singapore for support.References and recommended readingPapers of particular interest,published within the period of review, have been highlighted as:of special interestof outstanding interest1.Yaghi OM,O’Keefe M,Ockwig NW,Chae HK,Eddaoudi M,Kim J:Reticular synthesis and design of new materials.Nature2003, 423:705-714.2.Long JR,Yaghi OM:The pervasive chemistry of metal–organicframeworks.Chem Soc Rev2009,38:1213-1214.3.MacGillivray LR(Ed):Metal–Organic Frameworks:Design andApplication.Hoboken,New Jersey:John Wiley&Sons,Inc.;2010.4.Farrusseng D(Ed):Metal–Organic Frameworks:Applications fromCatalysis to Gas Storage.Weinheim,Germany:Wiley-VCH;2011.5.Adams J,Pendlebury D:Global Research Report:MaterialsScience and Technology.Leeds,UK:Thomson Reuters;2011. 6.Czaja AU,Trukhan N,Muller U:Industrial applications of metal–organic frameworks.Chem Soc Rev2009,38:1284-1293.7.Keskin S,Liu J,Rankin RB,Johnson JK,Sholl DS:Progress,opportunities,and challenges for applying atomically detailed modeling to molecular adsorption and transport in metal–organic framework materials.Ind Eng Chem Res2009,48:2355-2371.This article reviews the applications of atomically detailed modeling for MOFs.Quantum mechanical calculations are used to examine structural and electronic properties,while molecular modeling calculations are used to study adsorption and diffusion in MOFs.8.DU¨ren T,Bae YS,Snurr RQ:Using molecular simulation tocharacterise metal–organic frameworks for adsorptionapplications.Chem Soc Rev2009,38:1203-1212.This review gives an overview of how molecular simulation can be used to characterize MOFs for gas adsorption,and how molecular insights can be combined to develop design principles for specific applications.9.Jiang JW,Babarao R,Hu ZQ:Molecular simulations for energy, environmental and pharmaceutical applications of nanoporous materials:from zeolites,metal–organic frameworks to protein crystals.Chem Soc Rev2011,40:3599-3612.This review summarizes the recent simulation studies for energy,envir-onmental and pharmaceutical applications of nanoporous materials ran-ging from zeolites,MOFs to protein crystals with increasing degree of complexity in building blocks.Major challenges in future simulation exploration are discussed.10.Li JR,Sculley J,Zhou HC:Metal–organic frameworks forseparations.Chem Rev2012,112,doi:10.1021/cr200190s,inpress.11.Keskin S,van Heest TM,Sholl DS:Can metal–organicframework materials play a useful role in large-scale carbon dioxide separations?ChemSusChem2010,3:879-891.12.Fe´rey G,Serre C,Devic T,Maurin G,Jobic H,Llewellyn PL,Weireld GD,Vimont A,Daturi M,Chang JS:Why hybrid porous solids capture greenhouse gases?Chem Soc Rev2011,40:550-562.13.Li JR,Ma YG,McCarthy MC,Sculley J,Yu JM,Jeong HK,Balbuena PB,Zhou HC:Carbon dioxide capture-related gasadsorption and separation in metal–organic frameworks.Coord Chem Rev2011,255:1791-1823.14.Yang QY,Zhong CL:Molecular simulation of CO2/CH4/H2mixture adsorption in metal–organic frameworks.J Phys Chem B2006,110:17776-17783.142Nanotechnology15.Babarao R,Jiang JW,Sandler SI:Adsorptive separation of CO2/CH4mixture in metal–exposed,catenated and charged metal–organic frameworks:insight from molecular simulation.Langmuir2009,25:5239-5247.16.Jiang JW:Charged soc metal–organic framework for high-efficacy H2adsorption and syngas purification:atomisticsimulation study.AIChE J2009,55:2422-2432.17. Babarao R,Jiang JW:Unprecedentedly high selective adsorption of gas mixtures in rho zeolite-like metal–organic framework:a molecular simulation study.J Am Chem Soc 2009,131:11417-11425.A simulation study is reported for the separation of CO2-containingmixtures in rho zeolite-like MOF(ZMOF)with anionic framework.Forthefirst time,this study characterizes nonframework Na+ions,examines gas separation in ionic ZMOF,and reveals that rho-ZMOF is a promisingcandidate for CO2capture.18.Babarao R,Eddaoudi M,Jiang JW:Highly porous ionic rhtmetal–organic framework for H2and CO2storage andngmuir2010,26:11196-11203.19.Yazaydin AO,Snurr RQ,Park TH,Koh K,Liu J,LeVan MD,Benin AI,Jakubczak P,Lanuza M,Galloway DB et al.:Screeningof MOFs for CO2capture fromflue gas using a combinedexperimental and modeling approach.J Am Chem Soc2009,131:18198-18199.20. Xu Q,Liu DH,Yang QY,Zhong CL,Mi JG:Li-modified metal–organic frameworks for CO2/CH4separation.J Mater Chem 2010,20:706-714.The separation of CO2/CH4mixtures is simulated in Li-modified MOF-5. The selectivity is found to be greatly improved owing to the enhancement of electrostatic potential by Li doping.21. Lan JH,Cao DP,Wang WC,Smit B:Doping of alkali,alkaline-earth,and transition metals in covalent-organic frameworks for enhancing CO2capture byfirst-principles calculations and molecular simulations.ACS Nano2010,4:4225-4237.This study examines the doping of a series of alkali(Li,Na,and K), alkaline-earth(Be,Mg,and Ca),and transition(Sc and Ti)metals in covalent-organic frameworks,and the effects of the doped metals on CO2capture.22.Dietzel PDC,Johnsen RE,Fjellvag H,Bordiga S,Groppo E,Chavan S,Blom R:Adsorption properties and structure of CO2 adsorbed on open coordination sites of metal–organicframework Ni2(dhtp)from gas adsorption.IR spectroscopyand X-ray diffraction.Chem Commun2008,44:5125-5127.23.Babarao R,Dai S,Jiang DE:Functionalizing porous aromaticframeworks with polar organic groups for high-capacity and selective CO2separation:a molecular simulation study.Langmuir2011,27:3451-3460.24. Liu DH,Zhong CL:Understanding gas separation in metal–organic frameworks.J Mater Chem2010,20:10308-10318.This feature article summarizes the recent advances of computer model-ing on gas separation in MOFs and demonstrates how computer model-ing can help to understand the separation characteristics of MOFs.Several strategies are proposed to improve the separation efficiency of MOFs.25. Jiang JW:Metal–organic frameworks for CO2capture:what are learned from molecular simulations.In Coordination Polymers and Metal Organic Frameworks.Edited by OO L,Ramı´rez LD.Nova Science Publishers;2011.In this book chapter,recent simulation studies are summarized for CO2 capture in MOFs.A number of strategies are discussed towards improv-ing capture performance.In addition,the effects of moisture in various MOFs on CO2adsorption and separation are presented.26.Babarao R,Tong YH,Jiang JW:Molecular insight intoadsorption and diffusion of alkane isomer mixtures in metal–organic frameworks.J Phys Chem B2009,113:9129-9136.27. Krishna R,van Baten JM:In silico screening of metal–organic frameworks in separation applications.Phys Chem Chem Phys 2011,13:10510-10593.This perspective highlights the potency of molecular simulation in deter-mining the best MOF for a given separation task.A variety of metrics that quantify separation performance such as adsorption selectivity,working capacity,diffusion selectivity and membrane permeability are determined by simulation.28.Liu Y,Liu HL,Hu Y,Jiang JW:Development of a densityfunctional theory in three-dimensional nanoconfined space:H2storage in metal–organic frameworks.J Phys Chem B2009, 113:12326-12331.29.Liu Y,Liu HL,Hu Y,Jiang JW:Density functional theory foradsorption of gas mixtures in metal–organic frameworks.J Phys Chem B2010,114:2820-2827.30.Coudert FX,Mellot-Draznieks C,Fuchs AH,Boutin A:Predictionof breathing and gate-opening transitions upon binary mixture adsorption in metal–organic frameworks.J Am Chem Soc2009,131:11329-11331.31.Bae YS,Snurr RQ:Development and evaluation of porousmaterials for carbon dioxide separation and capture.AngewChem Int Ed2011,50:11586-11596.The question of how a large number of MOFs can be quickly evaluated for CO2separation is addressed.Five adsorbent evaluation criteria are described and used to assess over40MOFs for their potential in CO2 separation processes for natural gas purification,landfill gas separation, and CO2capture from power-plantflue gas.32.Horike S,Shimomura S,Kitagawa S:Soft porous crystals.NatChem2009,1:695-704.33.Tafipolsky M,Amirjalayer S,Schmid R:Atomistic theoreticalmodels for nanoporous hybrid materials.MicroporousMesoporous Mater2010,129:304-318.Available atomistic theoretical models are overviewed for the new class of functional porous hybrid materials such as MOFs and COFs.The current status of both periodic and non-periodic quantum mechanic,as well as molecular mechanic models are discussed.34.Kang IJ,Khan NA,Haque E,Jhung SH:Chemical and thermalstability of isotypic metal–organic frameworks.Chem Eur J2011,17:6437-6442.35.Shekhah O,Liu J,Fischer RA,Woll C:MOF thinfilms:existingand future applications.Chem Soc Rev2011,40:1081-1106. 36.Caro J:Are MOF membranes better in gas separation thanthose made of zeolites.Curr Opin Chem Eng2011,1:77-83. MOF membranes developed and tested for gas separation during the past5years have been reviewed.The structuralflexibility of MOFs prevents a sharp molecular sieving effect.Mixed-matrix membranes containing MOFs are predicted for the near future.37.Keskin S,Sholl DS:Efficient methods for screening of metalorganic framework membranes for gas separations usingatomically detailed ngmuir2009,25:11786-11795. An efficient approximate method is introduced to screen MOF mem-branes for gas separation with a connection between mixture adsorption and mixture self-diffusion properties.The method is applied to MOF membranes with chemical diversity for light gas separation.38.Bux H,Chmelik C,Krishna R,Caro J:Ethene/ethane separationby ZIF-8membrane:molecular correlation of permeation,adsorption,diffusion.J Membr Sci2011,369:284-289.39.Vinh-Thang H,Kaliaguine S:MOF-based mixed-matrix-membranes for industrial applications.In CoordinationPolymers and Metal Organic Frameworks.Edited by Ortiz OL,Ramı´rez LD.Nova Science Publishers;2011.40.Keskin S,Sholl DS:Selecting metal organic frameworks asenabling materials in mixed matrix membranes for highefficiency natural gas purification.Energy Environ Sci2010,3:343-351.41.Chen YF,Hu ZQ,Gupta KM,Jiang JW:Ionic liquid/metal–organicframework composite for CO2capture:a computationalinvestigation.J Phys Chem C2011,115:21736-21742.42.Tan JC,Cheetham AK:Mechanical properties of hybridinorganic-organic framework materials:establishingfundamental structure–property relationships.Chem Soc Rev 2011,40:1059-1080.43.Cychosz KA,Ahmad R,Matzger AJ:Liquid phase separation by crystalline microporous coordination polymers.Chem Sci2010,1:293-302.This perspective details the experimental studies reported on liquid-phase separation using microporous coordination polymers(MCPs).Guest mole-cules examined include those as small as water to large organic dyes.In many cases,MCPs outperform zeolites and activated carbons in both kinetics and efficiency.Recent development of in silico molecular modeling Jiang143。
MOE-基于结构的药物设计及在药物发现方面的应用

2010 © CloudScientific All Rights Reserved
2D Matching: (Fingerprint) Similarity Searching
Homology Model
by using more than one template in the presence of ligands, prosthetic groups, or other molecular entities as multimers: dimers, trimers, or tetramers
MOE‐Dock
Easy‐to‐use, fast, reliable Placement Methods: Triangle Matcher Alpha Triangle Alpha PMI Proxy Triangle Triangle Matcher Pharmacophore FlexX* GOLD* Scoring Function: London dG Alpha HB Affinity dG ASE
Leads
Known binding site? Yes No
Potency ? Selectivity? Physical Properties? ADMET?
Good, nothing need to do
Find Binding Site
Optimization
Not Good
2010 © CloudScientific All Rights Reserved
Receptor‐Based Drug Design
Given Protein
Known 3D Structure? Yes No
Pharmacophore Search Docking / Scoring
人源化单克隆抗体制备工艺

速度极快, 最快在1 周内即可完成抗体的分离工作。
核糖体展示技术
1997 年Hanes 等在Mattheakis 等的多聚核糖体展示技术的基础上 进行改进建立了核糖体展示技术。
基本ne,September 26, 2012
一、Molecular Modeling and Structural Analysis of D9 Fv
D9 VH and VL mRNA was extracted from D9 hybridoma cells, amplified by Reverse Transcription-PCR and then sequenced. The deduced amino acid sequences are shown here.
抗体药物市场销售额增势不减,世界各国纷纷投入巨资开发这座“金 矿”,全球医药巨头,如辉瑞、罗氏、诺华等更是不惜重金开发抗体 药物。国内方面,成都康弘的郎沐表现出色,2014年上市8个月实现销 售额1亿元,2015年销售额约3亿元。
在临床实践中,抗体药物也呈现愈发活跃的状态。美国詹森研究开发 有限责任公司副总裁威廉·R·斯特罗尔表示,过去十年间,多种抗体治 疗方法和新平台已被设计研发,未来抗体治疗的范围将进一步扩大。 “目前一些针对免疫肿瘤学的抗体已被研发,即将进入临床,为癌症 患者、免疫功能紊乱、代谢障碍等患者提供更多治疗方法。”
抗体的现状
从1992年首个抗体药物Orthoclone上市以来,截至2016年03月,欧美日 等主要市场共上市了61个抗体药物。2014年上市了6个抗体药物,2015 年上市了9个抗体药物,连续两年打破历史记录。2015年,61个抗体药 物合计销售额达到906亿美元,与2014年相比增长了8.2%。从销售数据 来看,前21位的抗体药物都超过了10亿美元。
介观模拟 相关翻译

How to predict diffusion of medium-sized molecules in polymer matrices. From atomistic to coarse grainsimulationsAbstract :The normal diffusion regime of many small and medium-sized molecules occurs on a time scale that is too long to be studied by atomistic simulations. Coarse-grained (CG) molecular simulations allow to investigate length and time scales that are orders of magnitude larger compared to classical molecular dynamics simulations, hence providing avaluable approach to span time and length scales where normal diffusion occurs. Here we develop a novel multi-scale method for the prediction of diffusivity in polymer matrices which combines classical and CG molecular simulations. We applied an atomistic-based method in order to parameterize the CG MARTINI force field, providing an extension for the study of diffusion behavior of penetrant molecules in polymer matrices. As a case study, we found the parameters for benzene (as medium sized penetrant molecule whose diffusivity cannot be determined through atomistic models) and Poly (vinyl alcohol) (PVA) as polymer matrix. We validated our extended MARTINI force field determining the self diffusion coefficient of benzene (2.27·10−9m2s−1) and the diffusion coefficient of benzene in PVA(0.263·10−12m2s−1). The obtained diffusion coefficients are in remarkable agreement with experimental data (2.20·10−9m2s−1 and 0.25·10−12m2s−1, respectively). We believe that this method can extend the application range of computational modeling, providing modeling tools to study the diffusion of larger molecules and complex polymeric materials.Keywords:Coarse grain . Diffusion .Molecular dynamics simulation . Multi scale models . Nanofiltration . Polymeric matricesIntroduction:Molecular dynamics (MD) simulations are a powerful tool in the material science field as they provide material’s structural and dynamics details that are difficult, cost- or time-consuming to be assessed with experimental techniques. In particular, MD simulations are a valid tool for the design of polymeric membranes with tuned permeability properties. Up to now, however, the design of barrier materials is based on trial and error experimental procedures, in which a large part of the effort is spent to synthesize and characterize materials and blends which finally turn out to be unsatisfactory.Atomistic simulation have been successfully applied in the past to obtain the diffusion coefficients of small molecules (like oxygen, carbon dioxide or water) inpolymeric membranes [1–7], polymeric blends [8, 9], biopolymers [10, 11] and organic-inorganic hybrid membranes [12]. However, despite the increasing computational power available to researchers and the improvements in the MD codes, atomistic simulations are still able to handle only systems with tens or hundreds of thousands of atoms and in the nanoseconds time scale. Several phenomena of interest at the material scale, however,cover time and space scales larger than those affordable with atomistic modeling. This is the case of normal diffusion regime. The diffusion coefficient D can be directly calculated from the motion of the particles extracted during a MD simulation, in particular from the mean square displacement (MSD) of the particles, using the Einstein equation [5]. This equation holds only in the case that the observation time (i.e., the simulation time) is large enough to allow the particles to show uncorrelated motion. This means that the MSD is linear with time, i.e., MSD∼t n where n=1. Conversely, if n<1 then the diffusion is in the anomalous diffusion regime. Therefore, in order to assess an accurate diffusion coefficient, MD simulations must reach the normal diffusive regime which, depending on the membrane and diffusive molecule, can be on time scales higher than few nanoseconds. This regime is therefore often difficult to reach for molecules larger than diatomic molecules, like water or benzene, hindering molecular simulations to assess an accurate diffusion coefficient [12, 13].Recently, the use of coarse grain (CG) modeling, in which a number of atoms are condensed into beads or interacting particles, has proven to be a suitable option to model large systems and long time scales, providing realistic results. The methods, assumptions and level of resolution greatly vary depending on the scope of the models and the properties of interests [14, 15]. CG models have been developed with particular focus on biomolecular systems, since biomolecules are often too large and their characteristic times too long to be treated with full atomistic simulations. However, there are no theoretical impediments to the application of CG methods to polymeric materials.In this view, of particular interest is the coarse grain force field developed by Marrink and co-workers and called MARTINI force field. This CG force field was originally developed to model the lipid bilayers forming the cellular membranes and then extended to proteins [16–18]. Unlike other CG models, which focus on accurate modeling of a particular state or a particular molecule, the philosophy of the MARTINI force field is to accurately parameterize the basic building blocks of the system (e.g., the single amino acids for proteins), thus allowing a broad spectrum of applications without the need of reparameterization.Relying on the same philosophy, in this work we present an atomistic-informed parameterization of the MARTINI force field for the modeling of penetrants diffusion in polymeric membranes. We used atomistic simulations to calculate the interaction free energy between the basic building blocks of the system, i.e., the penetrant molecule and the polymer monomers, and then we performed CG molecular dynamics simulations to assess the diffusion behavior. As a test case, we investigated the diffusion behavior of benzene in a matrix of Poly (vinyl alcohol), PVA. Benzene, an important industrial solvent and precursor in the production of drugs, plastics,synthetic rubber, and dyes, was chosen since it is a well known representative of medium-sized permeant molecules. On the other hand, the choice of PVA as the polymer matrix is based on the fact that this polymer is widely used in several fields and finds applications as membrane material due to its excellent chemical stability, film forming capacity, barrier properties and high hydrophilicity.Methods:Coarse grain mappingThe original MARTINI mapping scheme is based on the four-to-one rule, i.e., on average four heavy (nonhydrogen) atoms are grouped into a bead or interaction center. Here we used a similar mapping, where the benzene molecule is represented by one bead and the vinyl alcohol (VA) monomers are represented by a different bead (see Fig. 1). The mass of the two types of beads are calculated as the sum of the masses of the atoms grouped into the bead.Bonded and nonbonded interactionsThe chemically bonded beads interact through a bond interaction modeled as a harmonic potential Vbond(r):where r is the distance between two bonded beads, kbond is the force constant of the bond interaction and r0 is the equilibrium distance. In the system under investigation, only VA beads representing chemically bonded monomers along a PVA chain are subject to bond interactions.The beads i and j that are not chemically connected interact via nonbonded interactions, which are described by a Lennard-Jones 12-6 potential:where r is the distance between the two nonbonded beads, εij is the energy minimum depth (the strength of the interaction) and r0ij is the distance at the minimum of the potential.ParameterizationGiven the mapping scheme and the type of interaction, we needed to calculate one set of bonded parameters (for bonded VA–VA beads) and three sets of nonbonded parameters (VA–VA, benzene–benzene and VA–benzene). For each set we run a full atomistic simulation of the two groups involved in the interaction and we calculated the free energy of interaction between the two groups. The free energy calculationsare performed using the adaptative biasing force (ABF) framework [19] as implemented in the NAMD code [20, 21]. The atomistic MD simulations are carried out using the NAMD program and the all-atom CHARMM force field [22], for a simulation time of 40 ns (time step of 1 fs) at a temperature of 300 K. Nonbonded interactions are computed using a switching function between 20 and 22 Å. The free energy of interaction, computed via the ABF framework, is monitoredbetween the two groups of atoms as a function of their center-of-mass distance, in the range 2–20 Å using windows of 0.01 Å.Generation of the CG modelsWe generated three different molecular models: pure benzene, pure PVA and PVA with a small amount of benzene as penetrant molecule. All three systems were generated in the atomistic form using the Amorphous Cell construction tool of Materials Studio 4.4 (Accelrys, Inc.). The pure benzene system contained 500 benzene molecules in a cubic periodic box with initial density of 0.88 gcm−3. For the pure PVA system we considered six atactic PVA molecules (consisting of 200 repeat units) in a cubic periodic box with initial density of 1.25 gcm−3. Finally, the third system contained four PVA chains (of 200 repeat units) and 12 benzene molecules in a cubic periodic box with initial density of 1.25 gcm−3. The three atomistic systems are then converted into CG systems using the Coarse Grainer tool of Materials Studio according to the mapping scheme described above (see Fig. 2).CG simulationsCG molecular dynamics simulations are carried out using the Mesocite module and the MARTINI force field implemented in Materials Studio. Prior to the simulations, we modified the original force field including the parameters for bonded and nonbonded interactions between benzene and VA beads, as obtained from the ABF atomistic simulations. The CG systems are minimized for 1000 steps, then equilibrated for 1 ns (using a time step of 20 ps) at 300 K. Finally, production simulations are run for asimulated time of 10 ns (for pure benzene and pure PVA) and 200 ns (for benzene in PVA matrix). The diffusion coefficient Di of a single permeant molecule i is calculated by the Einstein relation, starting from the diffusion trajectory ~rietT which is determined during the production MD simulations:where represents the root mean square displacement (MSD) of the permeant molecule i averaged over all possible time origins and t represents the time. The computationally derived diffusion coefficient D for a given kind of permeant molecule is then obtained as average overthe diffusion coefficients for N permeant molecules:In this work the diffusion of all 500 benzene beads (for benzene self diffusion) and the 12 benzene beads (for benzene in PVA) was investigated during the CG simulations.Results:The parameters of the CG force field, i.e. the bonded and nonbonded interactions between the beads, are calculated through full atomistic simulations and by applying the ABF framework. The ABF calculations provide the free energy profile as a function of the distance between the group of atoms, as shown in Fig. 3. The free energy profile of two bonded VA monomers (Fig. 3a) is interpolated with a harmonic potential, giving the equilibrium distance and the force constant (see Table 1). On the other end, from the interaction free energy profiles between nonbonded VA monomers, benzene molecules and VA-benzene molecules (Fig. 3b–d) we obtained the three sets of r0 (energy minimum) and ε(energy minimum depth), used to feed the Lennard-Jones 12-6 potential of the CG force field (see Table 1).We used the CG approach to investigate three different systems: pure benzene, pure PVA and benzene molecules in a PVA matrix (see Fig. 2). The pure benzene system consisted of 500 benzene molecules, which were coarse grained into benzene beads. We measured the density of the system during the 1 ns equilibration obtaining a realistic value of 0.881±0.001 gcm−3 (where the experimental value is 0.876 gcm−3). After the equilibration we carried out a 10 ns simulation in which we monitored the MSD of the benzene molecules (see Fig. 4a) and, by applying Eq. 3, we determined the self diffusion coefficient of benzene, obtaining a value of 2.273±0.588·10−9m2s−1, very close to the experimental value of 2.203±0.004 ·10−9m2s−1 [23]. In the case of pure PVA the system consisted of a periodic box with six chains of 200 monomers each. The coarse grained system was equilibrated for 1 ns and the density of the CG PVA box was measured, giving a value of 1.305± 0.011 gcm−3, which lie in the experimental range (1.232– 1.329 gcm−3) [24]. Finally, the third system consisted of 12 benzene molecules diffusing in a PVA matrix. The density of the coarse grained system, measured at the end of 1 ns equilibration, was 1.293±0.003, similar to that of pure PVA. The MSD of the benzene beads was monitored during a 200 ns MD simulation (see Fig. 4b), and from the derivative of the curve, we calculated the diffusion coefficient of benzene in PVA. We obtained a diffusion coefficient of0.263±0.035·10−12m2s−1 which is in good agreement with the experimental value, that is 0.25·10−12m2s−1[12]. The 200 ns simulation of the 4 nm×4 nm×4 nm CG box (with 812 beads representative of ≈6000 atoms) took 36 hours on a single CPU. The results of the simulations as well as the experimental data are shown in Table 2.Table 1 Parameters of the CG force field, obtained fromfull atomistic free energy calculations.Within this approach bonded interactions (i.e., VA beads covalently connected) are modeled through a harmonic potential, while nonbonded interactions are approximated with a Lennard-Jones 12-6 potentialInteraction Bonded Nonbondedkbond (kcal/mol/Å2) r0 (Å) ε(kcal/mol) r0 (Å) VA–VA 40.36 2.76 1.306 4.115 VA–Benzene - - 0.957 5.51 Benzene–Benzene - - 0.9634 5.62 Table 2 Main results of the CG simulations. The conversion of the three systems under study fromatomistic to coarse grain reduces the interacting particles by a factor between 7 and 12, depending on the specific system. Despite the loss of atomistic details, the CG models feature realistic densities and are able to predict benzene diffusion coefficient very close to the experimentsSystems Totalatoms TotalbeadsFinal density(g/cm3)Experimentaldensity (g/cm3)PredictedDbenzene(m2/s)ExperimentalDbenzene(m2/s)500 benzene molecules 6000 500 0.881 0.876 2.273·10−9 2.203·10−9[23]6 PVA chains 8400 1200 1.305 1.23–1.32 [24] --4 PVA chains + 12 benzene 5744 812 1.293 -- 0.263·10−120.25·10−12[24]Discussion:In this work we present a multi-scale method for the parameterization of the MARTINI CG force field and its application for the calculation of the diffusivity of medium sized molecules in polymeric membranes. In the past, atomistic simulations have been already successfully applied for the calculation of diffusivity of small molecules in polymeric membranes. Nonetheless, the computational costs can hinder the ability of MD simulations to predict such a parameter. Indeed, the Einstein relation (Eq. 3) applied for the assessment of the diffusion coefficient can only be used when the simulation is in the regime of normal diffusion and this realm is reached when the slope of the function log[MSD(t)] = f [log(t)] equals 1. In the case of very small molecules, like oxygen or hydrogen, the normal diffusion regime can be reached within few nanoseconds [5] while, in the case of medium sized molecules like benzene [12] or even water [13], it cannot be reached within the limit of atomistic simulations. In this view, the use of CG simulations, where a number of atoms are condensed into beads or interacting particles, can be a useful approach to overcome the limitations of atomistic simulations.In order to test the feasibility of the CG approach, we investigated the self diffusion coefficient of benzene and its diffusivity in PVA matrix using an atomistic-informed CG force field (see Fig. 1). As shown in Table 2, the coarse graining reduces the number of interacting particles by a factor of ≈10. Furthermore,since the CG interactions are much smoother compared to atomistic interactions [16], it is possible to use a time step of 20–40 fs, much larger than that typical of classical MD (1–2 fs). Thus, the CG approach leads to a total speed-up factor of 200–400 with respect to atomistic simulations.In this work we did not rely on the standard MARTINI bead types but rather we defined two ad hoc bead types (one for benzene molecule and one for VA monomer) and we estimated the bonded and nonbonded parameters using atomistic free energy calculations.The predicted parameters are then used to feed the MARTINI force field. The extended force field is then used to perform CG molecular dynamics simulations of three different systems: pure benzene, pure PVA and benzene in PVA (see Fig. 2). The coarse grain systems underwent1 ns equilibration dynamics, during which they reached a stable density very close to the experimental values (Table 2), thus confirming that the outcome of the atomistic free energy calculations are reasonable. As a final validation of our approach, we ran longer CG simulations in order to assess the self diffusion coefficient of benzene and the diffusion coefficient of benzene in PVA. For the pure benzene, we ran a 10 ns simulations from which we estimated the diffusion coefficient. The calculated and the experimental values are shown in Table 2, and the comparison confirms that the CG model is able topredict the experimental value with good approximations. In the case of benzene diffusing in a PVA matrix, 10 ns of simulation were not enough to reach the normal diffusion regime, since the derivative of the function log[MSD(t)] =f [log(t)] was lower than 1. This result is in agreement with the observations of Pan et al. [12], which showed that MD simulations of a few nanoseconds are not long enough to reach the normal diffusion regime and provide a good estimation of benzene diffusivity in PVA. For this reason, we run a longer simulation of 200 ns, in order to reach the realm of normal diffusivity, as shown in Fig. 5. Indeed, the trajectory of this long simulation permitted us to obtain a diffusion coefficient very close to the experimental value (see Table 2). This result confirms the feasibility of the CG approach to reach the normal diffusion regime of medium sized molecules in polymer matrices and that our multi-scale approach is a valid method to treat this kind of problem.Fig. 5 Log(MSD) vs. log(t) plots (straight lines) and linear interpolation (dashed lines) for the self diffusion of benzene (panel a) and for the diffusion of benzene in PVA (panel b) obtained from CGsimulations. The plots show that in the case of pure benzene the normal diffusion regime is reached, as indicated by the slope very close to 1, already in the range from 10to 101ns, while in the case of benzene in PVA (panel b) a longer simulation time is required since the normal diffusion regime is reached in the range from 101.7 to 102.3ns (i.e., from 50 to 200 ns)The major limitation of atomistic computational techniques applied in the literature to solve diffusive problems is related to the restricted time scale and sample size which can be simulated, which are a few nanoseconds and a few nanometers, respectively. Thus, when the phenomena under investigation exceed these limits MD simulations fail to provide reliable values of the diffusivity. In order to overcome these limitations we developed a novel method, which consits of combining atomistic and coarse grain simulations in a multi-scale paradigm, where the parameters for the meso-scale model are derived from atomistic MD simulations. Similar techniques are increasingly applied for the study of biological problems, but to the best of our knowledge, have not been used for the investigation of diffusion problems. Here we showed that this technique can be successfully applied to investigate the diffusion of penetrant molecules in polymer matrices, reliably predicting experimental data. A similar method as used in this paper could be applied to study the diffusion of larger molecules, which require a longer time to reach the normal diffusion regime, or the study of complex polymeric materials, for which representative volumes are larger than a few cubic nanometersConclusions:In conclusion, the main focus of this work has been to develop and validate a novel multi-scale method for the prediction of diffusivity in polymer matrices. We demonstrated that atomistic-informed CG simulations can be a valid approach to treat problems where the computational limits of classical MD simulations are too restrictive while, at the same time, strictly atomistic details are not mandatory. Thus, the multi-scale approach presented in this work extends the application range of computational modeling and provide a useful tool to investigate phenomena at the micro-scale which determine macroscopic physical properties of polymeric materials.In this view, multi-scale paradigm here discussed can further help computation aided molecular modeling to reduce the extent of the experimental trial-and-error approach during the design and investigation of new materials, thus resulting in a more cost and time efficient process.Acknowledgments:This research was partially supported by the Italian Institute of Technology (IIT). The authors declare no conflict ofinterest of any sort.References:1. Gestoso P, Karayiannis NC (2008) Molecular simulation of the effect of temperature and architecture on polyethylene barrier properties. J Phys Chem B 112:5646–56602. Chiessi E, Cavalieri F, Paradossi G (2007) Water and polymer dynamics in chemically cross-linked hydrogels of poly(vinyl alcohol): a molecular dynamics simulation study. J Phys Chem B 111:2820–28273. Zhang QG, Liu QL, Chen Y, Wu JY et al (2009) Microstructure dependent diffusion of water-ethanol in swollen poly(vinyl alcohol): a molecular dynamics simulation study. Chem Eng Sci 64:334–3404. Hofmann D, Fritz L, Ulbrich J, Schepers C et al (2000) Detailedatomistic molecular modeling of small molecule diffusion and solution processes in polymeric membrane materials. Macromol Theory Simul 9:293–3275. Hofmann D, Fritz L, Ulbrich J, Paul D (2000) Molecular simulation of small molecule diffusion and solution in dense amorphous polysiloxanes and polyimides. Comput Theor Polymer Sci 10:419–4366. Tocci E, Hofmann D, Paul D, Russo N et al (2001) A molecular simulation study on gas diffusion in a dense poly(ether-etherketone) membrane. Polymer 42:521–5337. Pavel D, Shanks R (2003) Molecular dynamics simulation of diffusion of O-2 and CO2 in amorphous poly(ethylene terephthalate) and related aromatic polyesters. Polymer 44:6713–67248. Fermeglia M, Cosoli P, Ferrone M, Piccarolo S et al (2006) PET/ PEN blends of industrial interest as barrier materials. Part 1. Many-scale molecular modeling of PET/PEN blends. Polymer 47:5979–59899. Pavel D, Shanks R (2005) Molecular dynamics simulation of diffusion of O-2 and CO2 in blends of amorphous poly(ethylene terephthalate) and related polyesters. Polymer 46:6135–614710. Ionita M, Silvestri D, Gautieri A, Votta E et al (2006) Diffusion of smallmolecules in bioartificialmembranes for clinical use:molecular modelling and laboratory investigation. Desalination 200:157–15911. Prathab B, Aminabhavi TM (2007) Molecular modeling study on surface, thermal, mechanical and gas diffusion properties of chitosan. J Polym Sci B Polym Phys45:1260–127012. Pan FS, Peng FB, Lu LY,Wang JT et al (2008)Molecular simulation on penetrants diffusion at the interface region of organic-inorganic hybrid membranes. Chem Eng Sci 63:1072–108013. Entrialgo-Castaño M, Salvucci AE, Lendlein A, Hofmann D (2008) An atomistic modeling and quantum mechanical approach to the hydrolytic degradation of aliphatic polyesters. Macromol Symp 269:47–6414. Tozzini V (2005) Coarse-grained models for proteins. Curr Opin Struct Biol 15:144–15015. Chng C-P, Yang L-W (2008) Coarse-grained models reveal functional dynamics - II. Molecular dynamics simulation at the coarse-grained level—theories and biological applications. Bioinf Biol Insights 2:171–18516. Marrink SJ, de Vries AH, Mark AE (2004) Coarse grained model for semiquantitative lipid simulations. J Phys Chem B 108:750–76017. Marrink SJ, Risselada HJ, Yefimov S, Tieleman DP et al (2007) The MARTINI force field: coarse grained model for biomolecular simulations. J Phys Chem B 111:7812–782418. Monticelli L, Kandasamy SK, Periole X, Larson RG et al (2008) The MARTINI coarse-grained force field: extension to proteins. J Chem Theory Comput 4:819–834 19. Darve E, Pohorille A (2001) Calculating free energies using average force. J Chem Phys 115:9169–918320. Darve E, Wilson MA, Pohorille A (2002) Calculating free energies using a scaled-force molecular dynamics algorithm. Mol Simul 28:113–14421. Phillips JC, Braun R, Wang W, Gumbart J et al (2005) Scalable molecular dynamics with NAMD. J Comput Chem 26:1781–180222. MacKerell AD, Bashford D, Bellott M, Dunbrack RL et al (1998) All-atom empirical potential for molecular modeling and dynamics studies of proteins. J Phys Chem B 102:3586–361623. Mills R (2002) Search for isotope effects in the self-diffusion of benzene and cyclohexane at 25.deg. J Phys Chem 79:852–85324. Mark J (1999) Polymer data handbook. Oxford University Press, New York从原子理论到粗粒理论来进行模拟研究预测中等大小的分子在聚合物模型中的扩散摘要:由于小分子和中等大小的分子的正常扩散所需要的时间太长,而以至于不能很好通过原子理论的模似进行研究。
- 1、下载文档前请自行甄别文档内容的完整性,平台不提供额外的编辑、内容补充、找答案等附加服务。
- 2、"仅部分预览"的文档,不可在线预览部分如存在完整性等问题,可反馈申请退款(可完整预览的文档不适用该条件!)。
- 3、如文档侵犯您的权益,请联系客服反馈,我们会尽快为您处理(人工客服工作时间:9:00-18:30)。
Bond Stretching Energy
Bond Stretching Energy
Estretch = ks/2 (l - l0)2
Energy, kcal/mol
350 300 250 200 150 100 50 0 0 1 2 3 Internuclear Distance
2 1.5 1 0.5 0 0 -0.5 1 2 3 4
LennardLennard-Jones or 6-12 potential
combination of a repulsive term [A] and an attractive term [B]
Nonbonded Internuclear Distance
Enthalpy of Formation
Equal to “steric energy” plus sum of group enthalpy values (CH2, CH3, C=O, etc.), with a few correction terms Not calculated by all molecular mechanics programs (e.g., HyperChem and Titan) Titan) Calculated values are generally quite close to experimental values for common classes of organic compounds.
7
Kcal / mol
6 5 4 3 2 1 0 0 30 60 90 120 150 180 210 240 270 300 330 360
Torsion Angle
Butane Barrier is Sum of Two Terms: V1(1+ cos φ) + V3(1 + cos 3φ)
CH3
CH3
H
CH3 CH3 H
H
gauche H
HCH3
H
HH
Eclipsed
H CH3
H
H
HH
eclipsed
H
CH3
H
Anti
CH3
H
Torsional Energy
Etorsion = 0.5 V1 (1 + cos φ) + 0.5 V2 (1 + cos 2φ) + 2φ 0.5 V3 (1 + cos 3φ) 3φ
Molecular Modeling
Part I. A Brief Introduction to Molecular Mechanics
Molecular Modeling (Mechanics)
Calculation of preferred (lowest energy) molecular structure and energy based on principles of classical (Newtonian) physics “Steric energy” based on energy increments due to deviation from some “ideal” geometry Components include bond stretching, bond angle bending, torsional angle deformation, dipoledipole-dipole interactions, van der Waals forces, H-bonding and other terms. H-
Properties Calculated
Optimized geometry (minimum energy conformation) Equilibrium bond lengths, bond angles, and dihedral (torsional) angles Dipole moment (vector sum of bond dipoles) Enthalpy of Formation (in some programs).
Use of Cut-offs
Van der Waals forces, hydrogen bonding, electrostatic forces, and dipole-dipole dipoleforces have dramatic distance dependencies; beyond a certain distance, the force is negligible, yet it still “costs” the computer to calculate it. To economize, “cut-offs” are often “cutemployed for these forces, typically somewhere between 10 and 15Å.
(cubic terms give better fit in region near minimum; inclusion of a fourth power term eliminates the maximum in the cubic fcn.)
Bond Angle Bending Energy
0 106
108
110
112
Bond Angle
Torsional Energy
Related to the rotation “barrier” (which also includes some other contributions, such as van der Waals interactions). The potential energy increases periodically as eclipsing interactions occur during bond rotation.
Bond Angle Deformation, C-C-C
Ebend = kb/2 (θ - θ0)2 (θ
Energy, kcal/mol
35 30 25 20 15 10 5
graph: sp3 C-C-C
(Likewise, cubic and higher terms are added for better fit with experimental observations)
Energy, kcal/mol
Electrostatic Energy
1 0.8 0.6 0.4 0.2 0 -0.2 0 -0.4 -0.6 -0.8 -1 Internuclear Distance 1 2 3 4
Dipole-Dipole Energy
Calculated as the three dimensional vector sum of the bond dipole moments, also considering the permitivity of the medium (typical default value is 1.5) (this is too complicated to demonstrate!!!)
2.5 2 Energy 1.5 1 0.5 0 0 30 60 90 120 150 180 210 240 270 300 330 360 Torsion Angle
van der Waals Energy
van der W aals Energy
2.5
EvdW = A/r12 - B/r6
Energy, kcal/mol
van der Waals Energy...
Buckingham Potential
9
EvdW = A (B/r ) - C/r6
Energy, kcal/mol
8 7 6 5 4 3 2 1 0 0 1 2 3 4 Nonbonded Internuclear Diatance
Buckingham potential (essentially repulsion only, especially at short distances)
Electrostatic Energy
E electrostatic = q1q2 / cε r cε (attractive or repulsive, repulsive, depending on relative signs of charge; value depends inversely on permitivity of free space, space, or the dielectric constant of the hypothetical medium)
2 1.8 1.6 1.4 1.2 Energy 1 0.8 0.6 0.4 0.2 0 0 30 60 90 120 150 180 210 240 270 300 330 360 Torsion Angle
2 1.8 1.6 1.4 1.2 Energy 1 0.8 0.6 0.4 0.2 0 0 30 60 90 120 150 180 210 240 270 300 330 360 Torsion Angle
(Hooke’s law force... harmonic oscillator) graph: C-C; C=O C-
Higher order terms give better fit
With cubic and higher terms: ν(l) = k/2(l - l0)2 [1- k’(l - l0) [1- k’’(l - l0)2 - k’’’(l - l0)3 - …]
Morse potential & Hooke’s Law