Motion estimation in temporal subbands for quality scalable motion coding
多目标跟踪的多伯努利平滑方法
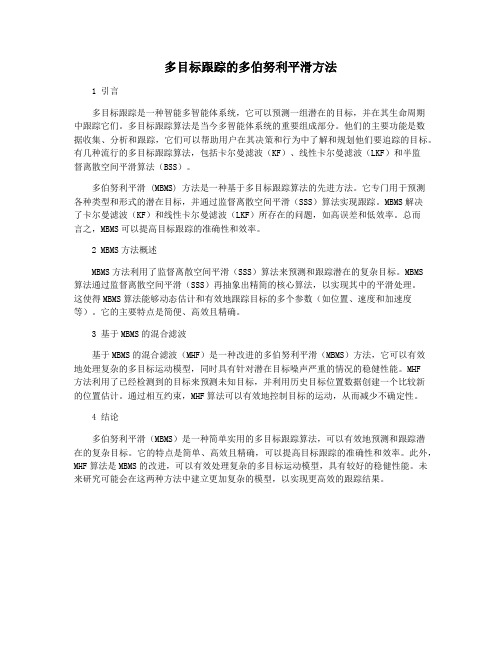
多目标跟踪的多伯努利平滑方法1 引言多目标跟踪是一种智能多智能体系统,它可以预测一组潜在的目标,并在其生命周期中跟踪它们。
多目标跟踪算法是当今多智能体系统的重要组成部分。
他们的主要功能是数据收集、分析和跟踪,它们可以帮助用户在其决策和行为中了解和规划他们要追踪的目标。
有几种流行的多目标跟踪算法,包括卡尔曼滤波(KF)、线性卡尔曼滤波(LKF)和半监督离散空间平滑算法(BSS)。
多伯努利平滑 (MBMS) 方法是一种基于多目标跟踪算法的先进方法。
它专门用于预测各种类型和形式的潜在目标,并通过监督离散空间平滑(SSS)算法实现跟踪。
MBMS解决了卡尔曼滤波(KF)和线性卡尔曼滤波(LKF)所存在的问题,如高误差和低效率。
总而言之,MBMS可以提高目标跟踪的准确性和效率。
2 MBMS方法概述MBMS方法利用了监督离散空间平滑(SSS)算法来预测和跟踪潜在的复杂目标。
MBMS算法通过监督离散空间平滑(SSS)再抽象出精简的核心算法,以实现其中的平滑处理。
这使得MBMS算法能够动态估计和有效地跟踪目标的多个参数(如位置、速度和加速度等)。
它的主要特点是简便、高效且精确。
3 基于MBMS的混合滤波基于MBMS的混合滤波(MHF)是一种改进的多伯努利平滑(MBMS)方法,它可以有效地处理复杂的多目标运动模型,同时具有针对潜在目标噪声严重的情况的稳健性能。
MHF方法利用了已经检测到的目标来预测未知目标,并利用历史目标位置数据创建一个比较新的位置估计。
通过相互约束,MHF算法可以有效地控制目标的运动,从而减少不确定性。
4 结论多伯努利平滑(MBMS)是一种简单实用的多目标跟踪算法,可以有效地预测和跟踪潜在的复杂目标。
它的特点是简单、高效且精确,可以提高目标跟踪的准确性和效率。
此外,MHF算法是MBMS的改进,可以有效处理复杂的多目标运动模型,具有较好的稳健性能。
未来研究可能会在这两种方法中建立更加复杂的模型,以实现更高效的跟踪结果。
矿山采动覆岩内部岩移原位监测技术进展及应用
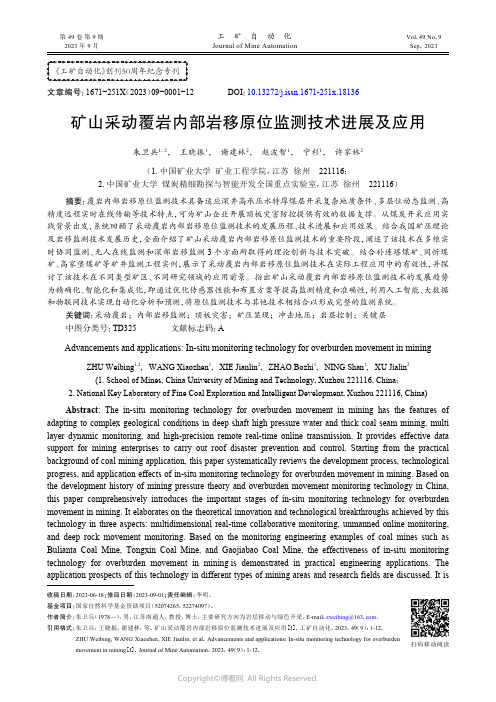
矿山采动覆岩内部岩移原位监测技术进展及应用朱卫兵1,2, 王晓振1, 谢建林2, 赵波智1, 宁杉1, 许家林2(1. 中国矿业大学 矿业工程学院,江苏 徐州 221116;2. 中国矿业大学 煤炭精细勘探与智能开发全国重点实验室,江苏 徐州 221116)摘要:覆岩内部岩移原位监测技术具备适应深井高承压水特厚煤层开采复杂地质条件、多层位动态监测、高精度远程实时在线传输等技术特点,可为矿山企业开展顶板灾害防控提供有效的数据支撑。
从煤炭开采应用实践背景出发,系统回顾了采动覆岩内部岩移原位监测技术的发展历程、技术进展和应用效果。
结合我国矿压理论及岩移监测技术发展历史,全面介绍了矿山采动覆岩内部岩移原位监测技术的重要阶段,阐述了该技术在多维实时协同监测、无人在线监测和深部岩移监测3个方面所取得的理论创新与技术突破。
结合补连塔煤矿、同忻煤矿、高家堡煤矿等矿井监测工程实例,展示了采动覆岩内部岩移原位监测技术在实际工程应用中的有效性,并探讨了该技术在不同类型矿区、不同研究领域的应用前景。
指出矿山采动覆岩内部岩移原位监测技术的发展趋势为精确化、智能化和集成化,即通过优化传感器性能和布置方案等提高监测精度和准确性,利用人工智能、大数据和物联网技术实现自动化分析和预测,将原位监测技术与其他技术相结合以形成完整的监测系统。
关键词:采动覆岩;内部岩移监测;顶板灾害;矿压显现;冲击地压;岩层控制;关键层中图分类号:TD325 文献标志码:AAdvancements and applications: In-situ monitoring technology for overburden movement in miningZHU Weibing 1,2, WANG Xiaozhen 1, XIE Jianlin 2, ZHAO Bozhi 1, NING Shan 1, XU Jialin 2(1. School of Mines, China University of Mining and Technology, Xuzhou 221116, China ;2. National Key Laboratory of Fine Coal Exploration and Intelligent Development, Xuzhou 221116, China)Abstract : The in-situ monitoring technology for overburden movement in mining has the features of adapting to complex geological conditions in deep shaft high pressure water and thick coal seam mining, multi layer dynamic monitoring, and high-precision remote real-time online transmission. It provides effective data support for mining enterprises to carry out roof disaster prevention and control. Starting from the practical background of coal mining application, this paper systematically reviews the development process, technological progress, and application effects of in-situ monitoring technology for overburden movement in mining. Based on the development history of mining pressure theory and overburden movement monitoring technology in China,this paper comprehensively introduces the important stages of in-situ monitoring technology for overburden movement in mining. It elaborates on the theoretical innovation and technological breakthroughs achieved by this technology in three aspects: multidimensional real-time collaborative monitoring, unmanned online monitoring,and deep rock movement monitoring. Based on the monitoring engineering examples of coal mines such as Bulianta Coal Mine, Tongxin Coal Mine, and Gaojiabao Coal Mine, the effectiveness of in-situ monitoring technology for overburden movement in mining is demonstrated in practical engineering applications. The application prospects of this technology in different types of mining areas and research fields are discussed. It is收稿日期:2023-06-18;修回日期:2023-09-01;责任编辑:李明。
gtsam 常用因子

gtsam 常用因子English answers:IMU Preintegration.Inertial Measurement Unit (IMU) preintegration is a technique used to integrate the IMU measurements over a period of time, typically between two keyframes. This reduces the computational cost of integrating the measurements online and allows for more efficient optimization. GTSAM provides a robust and accurate implementation of IMU preintegration.Stereo Vision.Stereo vision is a technique used to estimate the depth of a scene using two or more cameras. GTSAM provides a variety of stereo vision factors, including the pinhole model and the fisheye model. These factors can be used to estimate the pose of the cameras and the depth of the scene.Lidar.Lidar (Light Detection and Ranging) is a remote sensing technology that uses laser pulses to measure the distance to objects. GTSAM provides a variety of lidar factors, including the point-to-plane, point-to-line, and plane-to-plane models. These factors can be used to estimate the pose of the lidar sensor and the location of objects in the scene.GPS.Global Positioning System (GPS) is a satellite-based navigation system that provides location and time information. GTSAM provides a variety of GPS factors, including the position-only model and the velocity-aided model. These factors can be used to estimate the pose of the GPS receiver and the velocity of the vehicle.Odomery.Odometry is a technique used to estimate the pose of a vehicle using the measurements from its wheel encoders. GTSAM provides a variety of odometry factors, including the differential drive model and the unicycle model. These factors can be used to estimate the pose of the vehicle and the velocity of the wheels.Chinese answers:IMU预积分。
自适应运动估计算法
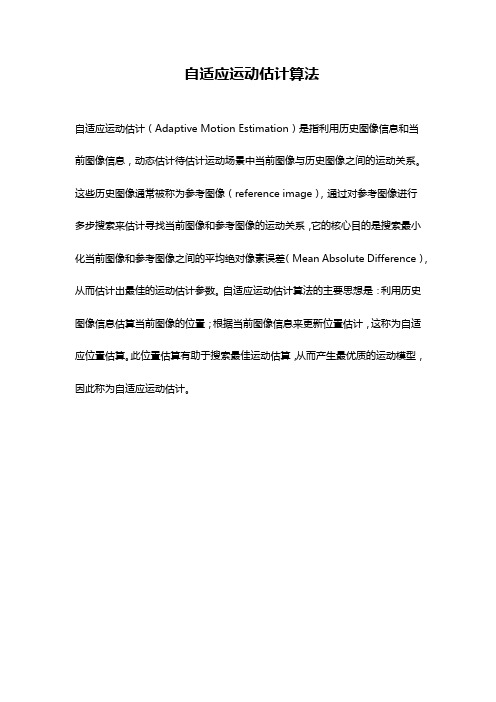
自适应运动估计算法
自适应运动估计(Adaptive Motion Estimation)是指利用历史图像信息和当前图像信息,动态估计待估计运动场景中当前图像与历史图像之间的运动关系。
这些历史图像通常被称为参考图像(reference image),通过对参考图像进行多步搜索来估计寻找当前图像和参考图像的运动关系,它的核心目的是搜索最小化当前图像和参考图像之间的平均绝对像素误差(Mean Absolute Difference),从而估计出最佳的运动估计参数。
自适应运动估计算法的主要思想是:利用历史图像信息估算当前图像的位置;根据当前图像信息来更新位置估计,这称为自适应位置估算。
此位置估算有助于搜索最佳运动估算,从而产生最优质的运动模型,因此称为自适应运动估计。
fast motion deblurring 中文翻译
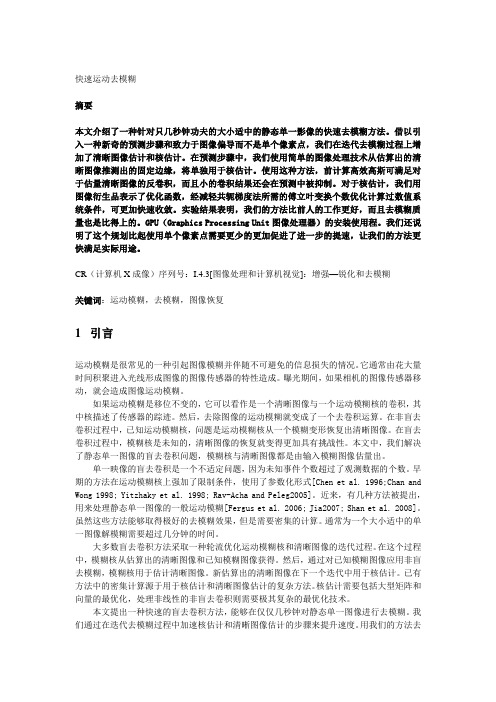
快速运动去模糊摘要本文介绍了一种针对只几秒钟功夫的大小适中的静态单一影像的快速去模糊方法。
借以引入一种新奇的预测步骤和致力于图像偏导而不是单个像素点,我们在迭代去模糊过程上增加了清晰图像估计和核估计。
在预测步骤中,我们使用简单的图像处理技术从估算出的清晰图像推测出的固定边缘,将单独用于核估计。
使用这种方法,前计算高效高斯可满足对于估量清晰图像的反卷积,而且小的卷积结果还会在预测中被抑制。
对于核估计,我们用图像衍生品表示了优化函数,经减轻共轭梯度法所需的傅立叶变换个数优化计算过数值系统条件,可更加快速收敛。
实验结果表明,我们的方法比前人的工作更好,而且去模糊质量也是比得上的。
GPU(Graphics Processing Unit图像处理器)的安装使用程。
我们还说明了这个规划比起使用单个像素点需要更少的更加促进了进一步的提速,让我们的方法更快满足实际用途。
CR(计算机X成像)序列号:I.4.3[图像处理和计算机视觉]:增强—锐化和去模糊关键词:运动模糊,去模糊,图像恢复1引言运动模糊是很常见的一种引起图像模糊并伴随不可避免的信息损失的情况。
它通常由花大量时间积聚进入光线形成图像的图像传感器的特性造成。
曝光期间,如果相机的图像传感器移动,就会造成图像运动模糊。
如果运动模糊是移位不变的,它可以看作是一个清晰图像与一个运动模糊核的卷积,其中核描述了传感器的踪迹。
然后,去除图像的运动模糊就变成了一个去卷积运算。
在非盲去卷积过程中,已知运动模糊核,问题是运动模糊核从一个模糊变形恢复出清晰图像。
在盲去卷积过程中,模糊核是未知的,清晰图像的恢复就变得更加具有挑战性。
本文中,我们解决了静态单一图像的盲去卷积问题,模糊核与清晰图像都是由输入模糊图像估量出。
单一映像的盲去卷积是一个不适定问题,因为未知事件个数超过了观测数据的个数。
早期的方法在运动模糊核上强加了限制条件,使用了参数化形式[Chen et al. 1996;Chan and Wong 1998; Yitzhaky et al. 1998; Rav-Acha and Peleg2005]。
Spatio-temporal motion estimation for transparency and occlusion

S-
2
where Ω is the support of χ. To make use of Gauss’ theorem in the plane, we denote by B the boundary of Ω, by N the unit normal to B , and by ds the arc-length element of B . We finally obtain the following equality: (u − v ) · ∇χ, φ =
I P IEEE I C I P (ICIP’03), B, S, S 14–17, 2003, . III, . 69–72.
SPATIO-TEMPORAL MOTION ESTIMATION FOR TRANSPARENCY AND OCCLUSIONS Erhardt Barth∗ , Ingo Stukeα , Til Aachα , and Cicero Motaβ Institute for Neuro- and Bioinformatics, University of L¨ ubeck Ratzeburger Allee 160, 23538 L¨ ubeck, Germany α Institute for Signal Processing, University of L¨ ubeck Institute for Applied Physics, University of Frankfurt, Germany
motion trajectory fields -回复

motion trajectory fields -回复Motion trajectory fields是指运动轨迹场,在计算机视觉和计算机图形学领域应用广泛。
它们可以用于识别运动物体的轨迹、分析运动模式和预测物体未来的运动路径。
在本文中,我将一步一步回答一些关于motion trajectory fields的问题,并介绍它们在不同领域的应用。
首先,什么是motion trajectory fields?motion trajectory fields是描述运动物体在空间中的轨迹和运动方向的数学模型。
它们通过分析运动物体在连续时间段内的位置变化,确定物体的运动路径和速度。
其次,motion trajectory fields是如何计算的?计算motion trajectory fields的一种常见方法是使用光流估计技术。
光流估计是通过分析图像序列中相邻帧之间的亮度变化来计算物体的运动模式。
这可以通过追踪像素的灰度值变化或特征点的移动来实现。
根据这些运动信息,可以生成运动轨迹场。
然后,motion trajectory fields的应用有哪些?motion trajectory fields在很多领域都有广泛的应用。
在计算机视觉领域,它们被用于目标跟踪、行为分析和物体识别。
例如,在目标跟踪方面,可以通过观察运动物体的轨迹来确定其位置和速度,从而实现精确的跟踪。
在行为分析方面,利用运动轨迹场可以识别和分析人的动作模式,如散步、跑步或跳跃。
此外,motion trajectory fields也被应用于虚拟现实和增强现实中,为用户提供更真实的交互体验。
最后,motion trajectory fields的未来发展方向是什么?随着计算机视觉和计算机图形学技术的不断进步,motion trajectory fields的应用潜力也在不断扩大。
未来的发展方向可以包括更精细的运动分析和预测,以及更高效的计算算法。
spatio-temporall...

Spatio-Temporal LSTM with Trust Gates for3D Human Action Recognition817 respectively,and utilized a SVM classifier to classify the actions.A skeleton-based dictionary learning utilizing group sparsity and geometry constraint was also proposed by[8].An angular skeletal representation over the tree-structured set of joints was introduced in[9],which calculated the similarity of these fea-tures over temporal dimension to build the global representation of the action samples and fed them to SVM forfinal classification.Recurrent neural networks(RNNs)which are a variant of neural nets for handling sequential data with variable length,have been successfully applied to language modeling[10–12],image captioning[13,14],video analysis[15–24], human re-identification[25,26],and RGB-based action recognition[27–29].They also have achieved promising performance in3D action recognition[30–32].Existing RNN-based3D action recognition methods mainly model the long-term contextual information in the temporal domain to represent motion-based dynamics.However,there is also strong dependency between joints in the spatial domain.And the spatial configuration of joints in video frames can be highly discriminative for3D action recognition task.In this paper,we propose a spatio-temporal long short-term memory(ST-LSTM)network which extends the traditional LSTM-based learning to two con-current domains(temporal and spatial domains).Each joint receives contextual information from neighboring joints and also from previous frames to encode the spatio-temporal context.Human body joints are not naturally arranged in a chain,therefore feeding a simple chain of joints to a sequence learner can-not perform well.Instead,a tree-like graph can better represent the adjacency properties between the joints in the skeletal data.Hence,we also propose a tree structure based skeleton traversal method to explore the kinematic relationship between the joints for better spatial dependency modeling.In addition,since the acquisition of depth sensors is not always accurate,we further improve the design of the ST-LSTM by adding a new gating function, so called“trust gate”,to analyze the reliability of the input data at each spatio-temporal step and give better insight to the network about when to update, forget,or remember the contents of the internal memory cell as the representa-tion of long-term context information.The contributions of this paper are:(1)spatio-temporal design of LSTM networks for3D action recognition,(2)a skeleton-based tree traversal technique to feed the structure of the skeleton data into a sequential LSTM,(3)improving the design of the ST-LSTM by adding the trust gate,and(4)achieving state-of-the-art performance on all the evaluated datasets.2Related WorkHuman action recognition using3D skeleton information is explored in different aspects during recent years[33–50].In this section,we limit our review to more recent RNN-based and LSTM-based approaches.HBRNN[30]applied bidirectional RNNs in a novel hierarchical fashion.They divided the entire skeleton tofive major groups of joints and each group was fedSpatio-Temporal LSTM with Trust Gates for3D Human Action RecognitionJun Liu1,Amir Shahroudy1,Dong Xu2,and Gang Wang1(B)1School of Electrical and Electronic Engineering,Nanyang Technological University,Singapore,Singapore{jliu029,amir3,wanggang}@.sg2School of Electrical and Information Engineering,University of Sydney,Sydney,Australia******************.auAbstract.3D action recognition–analysis of human actions based on3D skeleton data–becomes popular recently due to its succinctness,robustness,and view-invariant representation.Recent attempts on thisproblem suggested to develop RNN-based learning methods to model thecontextual dependency in the temporal domain.In this paper,we extendthis idea to spatio-temporal domains to analyze the hidden sources ofaction-related information within the input data over both domains con-currently.Inspired by the graphical structure of the human skeleton,wefurther propose a more powerful tree-structure based traversal method.To handle the noise and occlusion in3D skeleton data,we introduce newgating mechanism within LSTM to learn the reliability of the sequentialinput data and accordingly adjust its effect on updating the long-termcontext information stored in the memory cell.Our method achievesstate-of-the-art performance on4challenging benchmark datasets for3D human action analysis.Keywords:3D action recognition·Recurrent neural networks·Longshort-term memory·Trust gate·Spatio-temporal analysis1IntroductionIn recent years,action recognition based on the locations of major joints of the body in3D space has attracted a lot of attention.Different feature extraction and classifier learning approaches are studied for3D action recognition[1–3].For example,Yang and Tian[4]represented the static postures and the dynamics of the motion patterns via eigenjoints and utilized a Na¨ıve-Bayes-Nearest-Neighbor classifier learning.A HMM was applied by[5]for modeling the temporal dynam-ics of the actions over a histogram-based representation of3D joint locations. Evangelidis et al.[6]learned a GMM over the Fisher kernel representation of a succinct skeletal feature,called skeletal quads.Vemulapalli et al.[7]represented the skeleton configurations and actions as points and curves in a Lie group c Springer International Publishing AG2016B.Leibe et al.(Eds.):ECCV2016,Part III,LNCS9907,pp.816–833,2016.DOI:10.1007/978-3-319-46487-950。
- 1、下载文档前请自行甄别文档内容的完整性,平台不提供额外的编辑、内容补充、找答案等附加服务。
- 2、"仅部分预览"的文档,不可在线预览部分如存在完整性等问题,可反馈申请退款(可完整预览的文档不适用该条件!)。
- 3、如文档侵犯您的权益,请联系客服反馈,我们会尽快为您处理(人工客服工作时间:9:00-18:30)。
Motion estimation in temporal subbands for quality scalable motion codingM.Mrak,N.Sprljan and E.IzquierdoA new approach for estimation and modelling of layered motion vector fields for fully scalable video coding (SVC)is presented.Motion vector fields are evaluated according to their impact on the overall reconstruction error.This strategy leads to improved decoding perfor-mance when compared with previous methods for layered motion modelling in SVC.Introduction:Fully scalable video coding is needed to achieve highly flexible adaptation of video streams for various applications.It is well known that texture information usually demands a significant portion of the bit-stream while motion vectors require just a fraction of the total bandwidth.Texture coding is a well-understood technology which has attracted most of the efforts of the research community over the past decades,while less attention has been devoted to motion vector coding.However,at very low bit rates the ratio texture-motion becomes significant and the need for scalable motion coding becomes apparent.Scalable motion coding is a new concept,firstly introduced in [1].It has been shown that motion scalability can be beneficial at low bit rates and lower resolution [1–3].Motion information directly depends on the architecture of the video codec.Consequently,strategies for temporal and spatial scalability can be derived from the corresponding codec architecture.However,quality scalability of motion is a common problem for any architecture.Current approaches aimed at achieving quality scalability of motion are based on imposing scalability on both the structure of the motion vector field and the motion vector values.Low representations of the motion vectors’values are obtained either by using most significant bit-planes,averaging,or they are simply taken directly from the initial motion estimation (ME).In a layered structure motion is usually modelled using parameters from the initial estimation but without considering the distortion that would be introduced by the lower layer motion structure.In this Letter a method for the estimation of motion vector values and modelling of a layered motion structure is presented.The proposed strategy is based on finding the best motion vectors for a given macroblock partitioning and assessing that partition-ing regarding the reconstruction performance.Experimental results show improved performance when compared with other techniques.Frame reconstruction with lower-fidelity motion information:Impos-ing quality scalability on motion needs to be related to the process that decorrelates frames in the temporal domain:motion compensated temporal filtering (MCTF).For the Haar transform,temporal analysis between input frames {f i ,f i þ1}produces temporal frames,i.e.subbands {f i T ,f i þ1T }where f i Tis a lowpass frame and f i þ1T is a highpass frame.Temporal filtering is carried out in the direction of motion vectors mv ¼[mv H mv V ]T that are defined for motion units,such as blocks,of a specific motion structure for f i þ1.Specifically,temporal analysis uses motion vectors mv 0with the corresponding structure estimated in the initial ME.In a scalable video environment,for high bit rates only the scalability of texture is usually employed.However,for low bit rates scalability of motion is needed and its influence on the reconstruction is crucial.Generally,the reconstruction of the frames f R is obtained from{f ˆi T ,f ˆi þ1T },which are the quantised versions of temporal frames.This process uses a motion structure with the same or lower fidelity than the one used in the analysis and the corresponding reconstruction motion vectors mv R .Motion information for reconstruction is selected during the video bit-stream adaptation from available motion representations defined at the encoder side.In conventional approaches low representations of motion are defined at the encoder without optimising the quality of the reconstruction.However,low motion structure representation and related motion vectors can be carefully selected in the temporal subbands after MCTF .This fact is carefully exploited in the technique introduced in this Letter.The proposed scheme is called temporal motion estimation (TME)and is outlined in Fig.1.TME is used to estimate and model low representation of motion and is performed on temporal frames by searching for the motion vectors that minimise the distortion introduced in the reconstructed frames.The so estimateddistortion is proportional to the distortion resulting from scaling both texture andmotion.Fig.1Scalable encoder with TMEGeneralised motion structures:The block-based model is a popular representation of motion in which motion units are of rectangular shape.A regular partition of a basic motion unit,macroblock,con-sists of four square blocks,which can be further divided using the same principle.Such a partition can be described by a four-way tree structure.A tree T is associated to each macroblock of a motion compensated frame.A node n in T defines the position and the size of a blockB i þ1(n ,0)in a highpass frame.Here 0¼[00]T represents the 0-displacement relative to the block position defined by n in the frame f i þ1.Each final motion block used for MCTF corresponds to a tree leaf,which is also called an external node.All other motion tree nodes are internal nodes.Scalability can be imposed on the motion tree structure by dividing a motion tree into L layers.Motion tree layers (MTL)are then defined as MTL 0¼T 0and MTL l ¼T l n T l À1for l ¼1,...,L À1.T L À1is a tree used in MCTF while the inverse MCTF uses T l that is a subset of T L À1,i.e.T 0 T 1 ÁÁÁ T l ÁÁÁ T L À1.An example of such macroblock partitioning is given in Fig.2for L ¼3.In a non-scalable scenario motion parameters such as block mode and motion vectors are only defined for external nodes.However,if the decoder uses T l &T L À1the motion parameters of T l are required for framereconstruction.Fig.2Layered motion representation:partition of macroblock and corresponding motion treeEstimation for internal motion-tree nodes:In the proposed method the estimation of internal tree node parameters is performed using TME in temporal frames.A new measure,reconstruction square error (RSE),is used for evaluation of the distortion introduced into decoded frames.The proposed temporal motion estimation and modelling of motion layers is conducted according to the following algorithmic steps.Step 0Initialisation:Starting from the nodes closest to the leaves of the motion tree,each internal node is initialised with the motion vector mv 0obtained as the average of its child nodes.Step 1Estimation:For each internal node the estimation is performed in temporal subbands.A block B i (n ,mv )in the lowpass frame,displaced by mv ¼mv 0þj relative to B i þ1(n ,0),is searched within the search window of size w Âw .As a motion precision P from the initial ME is preserved,the components of j ¼[j H j V ]T are varied among j H ,j V 2{Àw ,Àw þ1=2P ,...,Àw þk =2P ,...,w }.Here for integer precision P ¼0and for 1=2P precision P >0.The evaluation of the candidate motion vectors is carried out using the RSE on the block level:RSE ðn ;mv Þ¼B i þ1ðn ;0ÞÀB R i þ1ðn ;0ÞþB i ðn ;mv ÞÀB R i ðn ;mv Þð1ÞELECTRONICS LETTERS 15th September 2005Vol.41No.19where B R are obtained from blocks in f T and f according to the inverse MCTF relations for a given mv.As a result of the estimation for node n the motion vector that introduces the smallest distortion in the recon-struction framemv RðnÞ¼arg minmvðRSEðn;mvÞÞð2Þis considered.According to(2)mv R(n)is associated to the node as well as the corresponding RSE(n,mv R)that is used for the modelling in the following step.Step2Modelling of motion layers:Motion layers are optimised on a frame level by selecting the tree branches with the lowest RSE among all macroblocks.Here,the optimality of chosen motion vectors depends on the applied temporalfiltering.As MCTF affects both highpass and lowpass frames,the estimated motion vectors are only locally optimal(for the given block).However,for unconstrained MCTF,(1)reduces to RSE(n,mv)¼k B iþ1(n,0)ÀB iþ1R(n,0)k,where B iþ1R(n,0)is synthesised accordingly to mv.In this case the proposed technique selects the frame-wise optimal motion vectors for predic-tionfilters of any size.Selected experimental results:Several tests were performed in an SVC environment[4]using two-level unconstrained MCTF,motion block sizes of8Â8to64Â64pixels,1=4-pixel precision and L¼7 motion layers.The proposed TME was compared with methods that for motion vectors in the internal nodes use values estimated in initial ME and median values offiner block partitioning.The effectiveness of a new distortion measure RSE is compared with commonly used square error(SE),which is computed between original frames as SE(n,mv)¼k B i(n,mv)ÀB iþ1(n,0)k.Motion layers were modelled so that the same number of tree nodes is used for each technique, providing almost equal motion bit rate for each technique.Original temporal subbands were used for synthesis.The averaged PSNR for the luminance component of two test sequences are given in Table1. Relative number of nodes stands for a ratio between the number of nodes of motion trees T l at the decoder and the nodes of the initial tree T LÀ1.Table1:Decoding resultsSequence Motionvectorsin internalnodes DistortionevaluationMotion layer l;relative number of nodes0;0.21;0.32;0.43;0.54;0.65;0.7Decoding quality-PSNR Y[dB]‘Basket’Median SE27.8731.0234.4538.1841.2444.19RSE28.0631.3934.8738.6442.2345.75 Initial ME SE28.6031.8735.5738.9341.8444.62RSE28.9332.5236.1039.5443.0546.49TME29.2832.7736.3239.7443.1946.69‘Foreman’Median SE34.1636.8439.4841.9344.6847.85RSE33.9737.0439.9042.8846.0349.63 Initial ME SE36.6738.8340.9143.1646.0848.55RSE36.7339.5342.1044.6847.5650.93TME37.0739.9142.4945.0647.9051.24The results show that the application of the RSE provides better modelling of motion trees,which improves the decoding,when used with sub-optimal selections of motion vectors in internal nodes(median and initial ME).Moreover,the proposed TME,which uses the RSE with additional estimation in the temporal subbands,gives the best results in all test points as a result of optimal motion tree selection. #IEE20055August2005 Electronics Letters online no:20052863doi:10.1049/el:20052863M.Mrak,N.Sprljan and E.Izquierdo(Electronic Engineering Department,Queen Mary University of London,Mile End Road, London E14NS,United Kindom)E-mail:marta.mrak@References1Secker,A.,and Taubman,D.:‘Highly scalable video compression with scalable motion coding’,IEEE Trans.Image Process.,2004,13,(8), pp.1029–10412Mrak,M.,Sprljan,N.,Abhayaratne,G.C.K.,and Izquierdo,E.:‘Scalable generation and coding of motion vectors for highly scalable video coding’.Proc.Picture Coding Symp.,December20043Xiong,R.,Xu,J.,Wu,F.,Li,S.,and Zhang,Y.Q.:‘Layered motion estimation and coding for fully scalable3D wavelet video coding’.Proc.Int.Conf.on Image Processing,October2004,V ol.4,pp.2271–2274 4Sprljan,N.,Mrak,M.,Abhayaratne,G.C.K.,and Izquierdo,E.:‘A scalable coding framework for efficient video adaptation’.Proc.Int.Workshop on Image Analysis for Multimedia Interactive Services,April 2005ELECTRONICS LETTERS15th September2005Vol.41No.19。