Computation of singular integral operators in wavelet coordinates
常用数学词汇汇总

隶属度grade of membership 隶属函数membership function 均方差mean square deviation 先验分布 a priori distribution先验估计 a priori estimate先验概率 a priori probability稳定性stability矩阵A的根radical of matrix A积分方程integral equation射影projection横坐标abscissa收敛convergence横轴abscissa axis连续的continuous凸闭集convex closed set单调的monotonic无穷infinity加性additive多项式polynomial算子operator函子functor微分方程differential equation微分式differential expression 转置伴随adjugacy肯定的affirmative代数范数algebraic norm任意平均数arbitrary average任意常数arbitrary constant任意递归arbitrary recurrence树形图arborescence算术距离arithmetic distance不等式inequality算术平均arithmetic mean几何平均geometric mean人工神经网络artificial neural network相伴图associated diagram度量空间metric space格lattice自协方差auto-covariance自相关autocorrelation自动机理论automata theory公理axiom公理的axiomatic坐标轴axis of coordinates纵轴axis of ordinates整数部分integer part不动点定理fixed point theorem Bayes序贯决策函数Bayessequenetial decision function基向量base vector基本权base weight重心barycenter最优调和优函数best harmonicmajorant双轴坐标biaxial coordinates有偏估计biased estimate双调和biharmonic二项分布binomial distribution边界集boundary set有界闭包bounded closure弱有界的bounded-weak大括号brace括号,方括号bracket分支树branch tree差分法calculus of differences微积分calculus of fluxion典型的,标准的canonical标准型canonical form标准同构canonical isomorphism基数cardinal number基数积cardinal product支集carried set不变量invariant引理lemma舍去casting out范畴,类型category圆心center of a circle点划线chain line重心center of gravity必然事件certain event等价的equivalent变换change基变换change of base信道channel特征超平面characteristichyperplane特征超曲面characteristichypersurface特征根characteristic root特征列characteristic series特征解characteristic solution固有值,特征值characteristic value最佳逼近特征characteristic of bestapproximation分层,谱系hierarchy2χ检验chi square criterion2χ分布chi square distribution中国邮递问题Chinese postmanproblem主列chief series中国剩余定理Chinese remaindertheorem论题thesis误差error组平均class mean经典概率论classical theory ofprobability类,组,级,班class闭开的clopen闭开集clopen set闭集closed collection闭包closure聚类分析cluster analysis粗糙的coarse子集subset偏相关系数coefficient of partialcorrelation回归系数coefficient of regression上包络coenvelope偏序集partially ordered set协调系统coherent system重合coincidence列矩阵column matrix列置换column permutation公共基common base紧凸集compact convex set均衡equilibrium相容的compatible余,补complementary完全,完备completeN维空间n-dimension space复合数composite number合成测度compound measure计算复杂性computation complexity不稳定性instability凹的concave条件熵conditional entropy条件概率密度conditionalprobability density条件完备的conditionally complete置信区间confidence interval置信下限confidence lower limit转置矩阵,伴随矩阵transposematrix相容条件consistency condition约束问题constrained problem上连续continuity from above 相反命题contrary proposition变量variable收敛证明convergence proof规划program卷积convolution坐标基向量coordinate basis vector 相关指数correlation indices陪集coset余弦cosine耦合couple耦合变量coupling variable耦合关系coupled relation协方差covariance临界点critical point临界值critical value临界集critical set三次的cubic割集cutset割平面cutting plane最小二乘法least square method疏散化decentralization疏散求和decentral summary解藕decoupling递减函数decreasing function定义公设defining postulate逆fuzzy defuzzify退化degenerateδ分布delta distribution线性linear行列式determinant确定性系统deterministic system展开development可展函数developable function差集difference set直接推导direct derivation正半锥direct half conoid直接方法direct manner直积direct product拓扑topology有向的directedDirichlet 边界条件Dirichlet boundary conditionDirichlet 级数Dirichlet series间断性,不连续性discontinuity 离散discrete直和direct sum不相交集disjoint subset不对称dissymmetry特异序列distinguished sequence扰动理论disturbed theory发散的divergent有效性域domain of validity对偶约束dual constraint对偶最优解dual optimal solution动态规划流程图dynamicprogramming flow chart有效性分析efficiency analysis有效估计efficiency estimate不变量invariant初等的elementary经验假定empirical assumption空集empty set端end终端条件end condition端结点end node端值end value可枚举的enumerableε循环epsilon-cycle相等约束equated constraint同等强度equally strong等价类equivalence class等价测度equivalent measures等值投影equivalent projection等价集equivalent set等变化的equivariant遍历ergode遍历的ergodic遍历类ergodic class估计值estimate value公式formula偶数even integer奇偶效验even-odd check精确解exact solution存在性证明existence proof互斥事件exclusive event显式公式explicit formula指数增长exponential growth广义基extended basis极值点extreme point失效率failure rate子集簇family of subsets故障树fault tree可行基feasible basis反馈feedback集合域field of sets有穷自动机finite automaton数学归纳法finite induction增量increment前向差分forward difference函数族function family多元函数function of severalvariables函数级数function series基本类fundamental classFuzzy聚类fuzzy clustering贴近度nearness松弛relaxation增益gain恒等式identity一般集合论general set theory广义解generalized solution全局约束global constraint全局可靠性global reliability图论graph theory群论group theory分组抽样grouping sampling调和分析harmonic analysis半连续的hemi-continuous插值interpolation双线性泛函bilinear functional启发式搜索heuristic search谱系分类hierarchical subdivision高阶差分higher difference立方cube齐次的homogeneous超平面hyperplane第i分量i-th component恒等的identical恒等identically equal病态方程ill-conditioned equation不可比的incomparable不相容的,非一致的inconsistent不确定性indeterminacy不确定的indeterminate归纳induction归纳推理inductioninference/reasoning归纳学习inductive learning无穷大infinity不稳定性instability整数部分integral part逆函数inverse function可逆函数invertible function可逆性invertibility不可约的irreducible等熵的isentropic孤立边界点isolated boundary point等距的isometric迭代iteration迭代的iterative迭代奇异值分解iterative singular value decomposition判断judgement滤波filtering扩张extension必要条件Lagrange necessary conditionlambda特征根latent root等价律law of equivalence最不利分布least favorable distribution最小二乘曲线拟合least square curve fitting左陪集left coset左极限left limit左递归规则left recursive rule小于或等于less than or equal to 似然函数likelihood function线性假设line hypothesis线性演绎linear deduction局部分析local analysis半群semigroup对数logarithm对数的logarithmic主码majority code多值函数many-valued function 多值性many-valuedness匹配matching矩阵求逆matrix inversion矩阵变换matrix transformation 矩阵转置matrix transposition 极大极小原则maximin criterion 极大极大maximax极小极大Minimax均方误差mean square error可测的measurable 元知识metaknowledge等效法method of equal effects归纳法method of induction极小极大定理min-max theorem最小生成树minimal spanning tree冗余度极小化minimization ofredundancy混合问题mixed problem模函数modular function模,模数modulo多维分布multidimensional allocation超几何的hypergeometric正态分布normal distribution多值,重值multiple value重数multiplicity多阶段决策multistage decision多元判别分析multivariatediscriminant analysis互斥问题mutual exclusion problem互斥(不相容)集mutually exclusivesets互不相交子集mutually disjointsubsets互素的mutually prime互相分离集mutually separated sets互相奇异的mutually singularN元n-aryN元关系n-ary relationN连通图n-connected graphN维随机变量n-dimensionalrandom variableN倍,N重n-foldN元组n-tupleN次幂n-th power负二项分布negative binomial否命题negative proposition嵌套递归式nested recursion非等距的non-isometric非交叉集non-overlapping set非自反关系non-reflexive relation非对称的non-symmetrical非稳定的non-stable非对称关系non-symmetric relation非平凡的non-trivial非空的non-vacuous非零向量non-vanishing vector非经典的nonclassical非相容nonconforming非紧的noncompact非合作noncooperative非退化nondegenerateNondegenerative不确定的nondeterministic非空集nonempty set不可行的nonfeasible不完整约束nonholonomicconstraint非增nonincreasing非劣解noninferior solution非迭代的noniterative非极限的nonlimit非线性的nonlinear正规化方程normalized equation数字的numerical一对多的one-to-many单值函数one-valued function单向函数one-way function开集opener逆范畴,对偶范畴oppositecategory异号opposite sign最优的optimal停止stopping有序和ordered sum序数,有序的ordinal定向orientation正交基orthogonal basic两两的pairwise两两独立pairwise independence平行parallel部分展开partial expansion偏序partial order峰值peak value罚函数penalty function完全相关perfect correlation完全对偶性perfect duality性能评价performance evaluation允许值permissible value扰动问题perturbation problem分段连续piecewise continuity逐点遍历定理pointwise ergodictheorem势,基数potency优先图precedence diagram偏爱序preference ordering素数prime先验分布prior distribution范围range线性linearity伪随机的pseudo-random纯策略pure strategy二次对偶quadratic dual四重的,四倍的quadruple,quadrupling,quadruplex径向基函数radial basis function径向偏差radial deviation径向开集radially open set可归约的reducible正则基数regular cardinal多面体polyhedron可靠性计算reliability calculation 可去集removable set累极限repeated limit剩余类residue class可解的resoluble回溯集retraceable set可逆的reversible直角right angle严格上界rigorous upper bound 鲁棒性,稳健性robustness均方根root-mean-square粗糙集rough set粗逻辑rough logic舍入round-off舍入的rounded程序routine行坐标row-coordinates行向量row vector消元规则rule of elimination鞍点saddle point同类same kind样本中心矩sample central moment 样本方差sample variance散布的scattered单叶函数schlicht function二次多项式second degree of polynomial二次导数算法second derivative algorithm二阶必要条件second order necessary condition自相容性self-consistency自相关性self-correlation自对偶self-dual 半同余semi-congruence半等价的semi-equivalent偏序集semi-ordered set半完全的semi-perfect可分的separable七倍的,七维的septuple七的septimal时序sequence in time子集序列sequence of subsets实数集set of real numbers集值函数set-valued function收缩算法shrinking algorithmσ域sigma-field单纯形法simplex method单值函数single-valued function稀疏索引sparse index谱估计spectral estimate样条拟合spline fitting稳态误差steady state error步长,阶段step步长step size逐步法stepwise随机分布stochastic distribution严格不等式strict inequality严格单调递增strictly monotoneincreasing严格正定算子strictly positivedefinite operator强对偶strong duality较强一致性stronger uniformity连通有向图connected directedgraph次加性的subadditive次调和解subharmonic solution下标subscript下标变量subscript variable辅助条件subsidiary condition充分集sufficient set上确界sup上标superscript支撑集support set表面散度surface divergence前向搜索sweep forward后向搜索sweep backward常微分方程组system of ordinarydifferential equation剩余系system of residues逐项term by term三元的,三进制的ternary假设检验test of hypothesis正态性检验test of normality显著性检验test of significance最后决定terminal decision择一定理theorem of alternative互补松弛定理theorem ofcomplementary slackness赋值论theory of valuations消除论theory of elimination数论theory of numbers置换理论theory of permutation排队论theory of queue集合论theory of sets象限quadrant自顶向下top-down全部单调的totally monotonic变换范围transformation range瞬态响应transient response超限评估transfinite evaluation传递闭包transitive closure转移概率矩阵transition probabilitymatrix转置,对换transposition梯形的trapezoidal梯形公式trapezoidal formula遍历traverse类树的tree-like树图tree graph试错搜索trial-and-error search三角数triangular number平凡序trivial ordered双重的two-foldTwo-person nash game超因子ultra-divisor超指数ultra-exponential超无穷点ultra-infinite point超弱的ultra-weak无偏置置信区间unbiasedconfidence interval无偏估计unbiased estimate无偏估计量unbiased estimator无偏α水平检验unbiased levelalpha test不确定推理uncertain reasoning无条件的unconditional不相关的uncorrelated不等方差unequal variance非本质的unessential一致性uniformity酋矩阵unitary matrix普通范畴universal category泛算子universal operator无序的unordered全域,总体universe无下标变量unsubscripted variable 非零解,非平凡解untrivial solution 上界upper limit变分类variational class向量不变式vector invariant通用的,多用途的versatile/versal 锥顶vertex of a cone顶点图vertex graph抛物线的顶点vertex of a parabola 垂直射影vertical projection小波wavlet弱闭包weak closure弱完备性weak completion弱耦合weak coupling弱序化weak ordering弱耦合系统weak coupled system 价格加权综合weighted aggregate of price良序子集well-ordered subset整数whole numberzeta零和对策zero sum game零序列zero-sequence零熵zero entropy笛卡尔积Cartesian product折扣tradeoff交集intersection推论corollary引理lemma证明proof公式formula定理theorem。
linux lapack矩阵运算
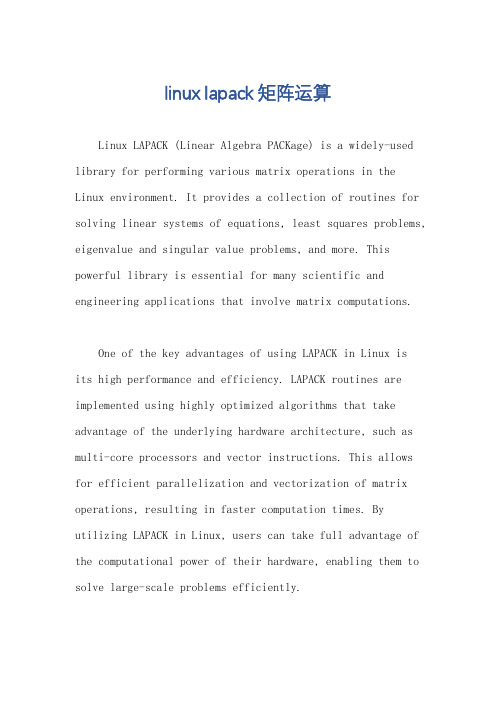
linux lapack矩阵运算Linux LAPACK (Linear Algebra PACKage) is a widely-used library for performing various matrix operations in the Linux environment. It provides a collection of routines for solving linear systems of equations, least squares problems, eigenvalue and singular value problems, and more. This powerful library is essential for many scientific and engineering applications that involve matrix computations.One of the key advantages of using LAPACK in Linux isits high performance and efficiency. LAPACK routines are implemented using highly optimized algorithms that take advantage of the underlying hardware architecture, such as multi-core processors and vector instructions. This allows for efficient parallelization and vectorization of matrix operations, resulting in faster computation times. By utilizing LAPACK in Linux, users can take full advantage of the computational power of their hardware, enabling them to solve large-scale problems efficiently.Another benefit of using LAPACK in Linux is its extensive functionality. LAPACK provides a wide range of routines for various matrix operations, including matrix factorizations, matrix-vector operations, and matrix eigenvalue and singular value computations. These routines are designed to be highly robust and reliable, ensuring accurate results even for ill-conditioned or singular matrices. With LAPACK, users have access to a comprehensive set of tools for solving complex linear algebra problems.Furthermore, LAPACK in Linux offers excellent interoperability with other scientific computing libraries and programming languages. LAPACK routines are typically written in Fortran, but they can be easily accessed and used from other programming languages such as C, C++, and Python. Many popular numerical computing libraries, such as NumPy and SciPy in Python, provide LAPACK bindings, making it straightforward to integrate LAPACK into existing codebases. This interoperability allows users to leverage LAPACK's capabilities within their preferred programming environment, enhancing productivity and code reusability.In addition to its performance and functionality, LAPACK in Linux benefits from a large and active user community. The LAPACK library has been widely adopted inthe scientific and engineering communities, and as a result, there is a wealth of resources and support available. Users can find extensive documentation, tutorials, and example code online, as well as participate in forums and mailing lists to seek assistance from experienced users. Thisvibrant community ensures that LAPACK remains well-maintained, continuously improving, and adaptable to evolving needs.Lastly, LAPACK's open-source nature aligns with the principles of the Linux ecosystem. Being open source, LAPACK can be freely used, modified, and distributed, allowing users to customize and extend its functionality to suit their specific requirements. This openness fosters collaboration and innovation, as users can contribute improvements and bug fixes back to the community. The open-source nature of LAPACK in Linux promotes transparency, reproducibility, and the sharing of knowledge, which are fundamental values in scientific computing.In conclusion, Linux LAPACK provides a powerful and efficient framework for performing matrix operations in the Linux environment. Its high performance, extensive functionality, interoperability, active user community, and open-source nature make it an excellent choice for solving complex linear algebra problems. Whether you are working on scientific research, engineering simulations, or data analysis tasks, LAPACK in Linux offers the tools and resources needed to tackle a wide range of matrix computations effectively.。
riemann-roch定理

riemann-roch定理The Riemann-Roch Theorem is a fundamental theorem in complex analysis, algebraic geometry, and arithmetic geometry concerning the behavior of linear series on algebraic curves. It plays a major role in the study of divisors on an algebraic curve, particularly in algebraic geometry. It was first proved by Bernhard Riemann in 1854. The statement of the theorem is a complex relationship between the degree and number of effective divisors, singular points, and the genus of the curve.The theorem is used to prove results in a number of areas, including the existence of trigonal curves and the Brill–Noether theorem. It also has applications in coding theory and the computation of zeta functions. The theorem is a generalization of the genus formula for algebraic curves, which is a special case of Riemann-Roch for curves with no points of multiplicity.The Riemann-Roch theorem has seen numerous generalizations, including to higher-dimensional varieties and to non-commutative rings. It has also been used as the starting point for proving a number of deep results, such as Grothendieck's Riemann-Roch theorem in algebraic geometry.The Riemann-Roch Theorem has been a cornerstone of modern mathematics, allowing for the study of algebraic curves with much greater accuracy and detail. It has been further developed and adapted to many different contexts, including non-commutative rings, higher-dimensional varieties and non-archimedean geometry. As a result, it has been used to prove a number of deep and important results, such as the Brill-Noether Theorem and Grothendieck's Riemann-Roch theorem.The Riemann-Roch Theorem allows for a deep understanding of algebraic geometry; it gives us new and powerful tools to study divisors onalgebraic curves and to better understand their properties. It also provides new ways of bounding the number of effective divisors, singular points, and the genus of a curve. With continued research and development, this theorem promises to provide further breakthroughs in the field of algebraic geometry.。
克隆巴赫系数的英文
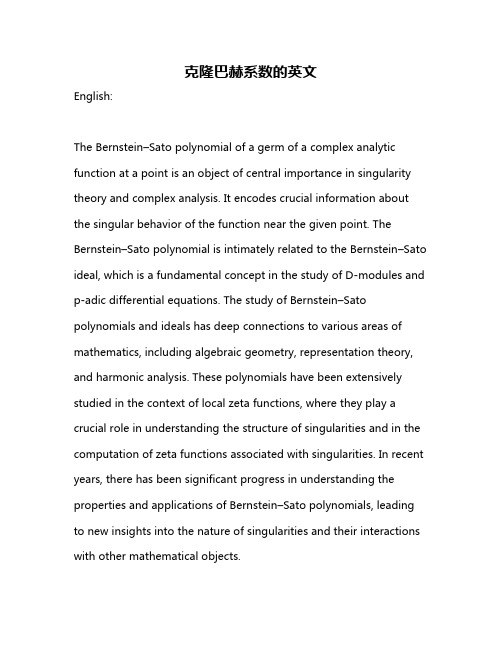
克隆巴赫系数的英文English:The Bernstein–Sato polynomial of a germ of a complex analytic function at a point is an object of central importance in singularity theory and complex analysis. It encodes crucial information about the singular behavior of the function near the given point. The Bernstein–Sato polynomial is intimately related to the Bernstein–Sato ideal, which is a fundamental concept in the study of D-modules and p-adic differential equations. The study of Bernstein–Sato polynomials and ideals has deep connections to various areas of mathematics, including algebraic geometry, representation theory, and harmonic analysis. These polynomials have been extensively studied in the context of local zeta functions, where they play a crucial role in understanding the structure of singularities and in the computation of zeta functions associated with singularities. In recent years, there has been significant progress in understanding the properties and applications of Bernstein–Sato polynomials, leading to new insights into the nature of singularities and their interactions with other mathematical objects.中文翻译:克隆巴赫多项式是复解析函数在某一点处的一个基本概念,它在奇点理论和复分析中具有重要的地位。
对称矩阵特征值分解的FPGA实现
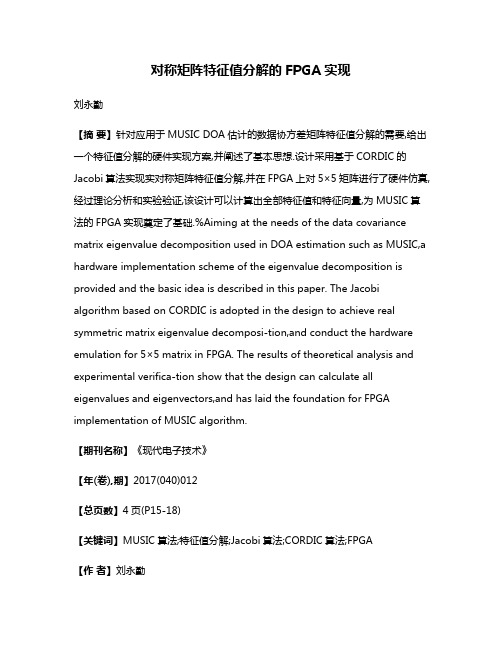
对称矩阵特征值分解的FPGA实现刘永勤【摘要】针对应用于MUSIC DOA估计的数据协方差矩阵特征值分解的需要,给出一个特征值分解的硬件实现方案,并阐述了基本思想.设计采用基于CORDIC的Jacobi算法实现实对称矩阵特征值分解,并在FPGA上对5×5矩阵进行了硬件仿真,经过理论分析和实验验证,该设计可以计算出全部特征值和特征向量,为MUSIC算法的FPGA实现奠定了基础.%Aiming at the needs of the data covariance matrix eigenvalue decomposition used in DOA estimation such as MUSIC,a hardware implementation scheme of the eigenvalue decomposition is provided and the basic idea is described in this paper. The Jacobi algorithm based on CORDIC is adopted in the design to achieve real symmetric matrix eigenvalue decomposi-tion,and conduct the hardware emulation for 5×5 matrix in FPGA. The results of theoretical analysis and experimental verifica-tion show that the design can calculate all eigenvalues and eigenvectors,and has laid the foundation for FPGA implementation of MUSIC algorithm.【期刊名称】《现代电子技术》【年(卷),期】2017(040)012【总页数】4页(P15-18)【关键词】MUSIC算法;特征值分解;Jacobi算法;CORDIC算法;FPGA【作者】刘永勤【作者单位】西安理工大学自动化与信息工程学院,陕西西安 710048;渭南师范学院数学与物理学院,陕西渭南 714099【正文语种】中文【中图分类】TN911-34;TN929.1多信号分类(MUSIC)[1]算法是波达方向(DOA)估计技术中最具代表性的高分辨力算法之一,因其突破了传统方法的瑞利极限而广受人们青睐。
Synopsis

Form101Part II:Research ProposalSynopsisProvide a concise overview of the scientific and technical objectives,approach,and the new knowledge,expertise,or technology that could be transferred to Canadian industry.Indicate the benefits expected to accrue to Canadian industry,to the academic institution,and to the scientific or engineering disciplineThe industrial partner for this project is Waterloo Maple Inc.,a Canadian company,whose main commercial product is Maple.Maple is a mathematical software system which con-tains facilities for exact algebraic computation and numerical computation.It is used by scientists and engineers in many disciplines in Canada,the US,and worldwide,for research and development.Maple’s main algebraic capability is polynomial computation over various rings,most importantly,the integers,finitefields,algebraic numberfields,and functionfields.Most user level application facilities in Maple,such as solving systems of algebraic and differen-tial equations,make extensive use of polynomial operations.This includes basic polynomial arithmetic,polynomial greatest common divisors(GCDs),polynomial factorization,poly-nomial resultants,and Gr¨o bner basis computation.However,except for Z n[x],Maple does not have any dedicated polynomial data structures and it is not possible to implement many of the best algorithms,in particular the modular algorithms,efficiently in the Maple lan-guage because it is interpreted.Consequently,Maple’s competitiveness is falling behind that of other commercial systems,for example,Mathematica and Magma.Moreover,Maple is becoming less helpful as a platform for the development of new algorithms.The main objectives of this project are(i)to design and implement a good data struc-ture for polynomials over the rationals,finitefields and algebraic numberfields,that will enable an efficient implementation of modular algorithms,(ii)to implement the best mod-ular algorithms for the critical polynomial operations above so that Maple’s core algebraic facilities are the best available for general purpose computation,and(iii)to develop and im-plement new efficient modular algorithms for polynomial operations over algebraic number and functionfields.Because the focus of this proposal is the“core”algebraic facilities of Maple,this project will be of immediate benefit to most users of Maple and it will provide a solid foundation for future research and development.The research contribution to thefield of computational algebra will be the development of efficient algorithms for computing with polynomials over algebraic number and functionfields and improved reconstruction techniques. BackgroundRelate the proposal to current scientific,technical and commercial developments in thefield, referring to the current literature and market conditions.Describe the background research on which the project is built.The three main problems addressed in this project proposal are the polynomial GCD prob-lem,the polynomial factorization problem and the Gr¨o bner basis computation problem.Thescientific literature for each is quite extensive hence our summary is necessarily selective.The texts of Geddes,Czapor,Labahn,[1],Zippel[2]and von zur Gathen and Gerhard[3],contain descriptions of algorithms for the polynomial GCD and factorization problems over Q and GF(q),and some material on computing Gr¨o bner bases.The Becker,Weisspfenning text[4] contains a deeper treatment of Gr¨o bner basis computation.1:The polynomial GCD problem.Thefirst effective algorithm for multivariate polynomial GCD computation over Z was Brown’s algorithm[5].Subsequent research focussed on improving the time complexity of Brown’s algorithm for sparse polynomials.Results include Wang’s EEZ-GCD algorithm [6],Zippel’s sparse modular GCD algorithm[7]and Kaltofen and Trager’s black box GCD algorithm[8].The heuristic algorithm GCDHEU of Char,Geddes,Gonnet in[9]provides an alternative approach in which a polynomial GCD problem is reduced to a single large integer GCD computation.All general purpose computer algebra systems are using some combination of these algorithms.For GCDs overfinitefields,the main difficulty is how to deal with smallfields where there may be insufficient evaluation points for the interpolation based algorithms.An obvious solution is to work in an extensionfield of suitably large size.Kaltofen and Monagan[10] show how to do this more efficiently.This is implemented in Maple for GF(p)[x,y]only.For GCDs over numberfields,based on previous work of Langemyr and McCallum[11], Encarnacion shows in[12]how to compute univariate GCDs over a numberfield presented with onefield extension.Encarnacion’s algorithm uses rational reconstruction,a technique developed by Wang,Guy and Davenport in[13]which has turned out to have wide appli-cation.Encarnacion’s algorithm is implemented in Axiom,Maple,and Magma.Maple also uses the heuristic algorithm of Gonnet et.al.[15]for onefield extension which is imple-mented for multivariate polynomials.In[16],van Hoeij and Monagan show how to compute GCDs over numberfields presented with multiplefield extensions.We have completed a mul-tivariate implementation of this algorithm in the Maple language in2001and in the Magma language in2003.Both implementations use a recursive dense polynomial data structure to facilitate an efficient implementation.The case of polynomial GCDs over algebraic functionfields has not,as far as we are aware of,been investigated in the literature.Maple is using the Euclidean algorithm and as a consequence,it grinds to a halt on polynomials of even modest degree.It is clear though that many of the techniques used for the algebraic numberfield case and multivariate polynomial GCDs over Z will apply.2:The polynomial Factorization problem.The polynomial factorization problem can be divided into four main cases,namely,univariate factorization over(i)finitefields,(ii)the integers,(iii)numberfields,and(iv)multivariate factorization over the preceding cases.Thefirst polynomial time algorithm for(i)was given by Berlekamp in[17]and for(ii)was given by Lenstra,Lenstra,and Lovasz,in[18]. Despite the latter result,all computer algebra systems have continued to use the Berlekamp-Hensel procedure for(ii)which,though not polynomial time,has a much better average caseperformance.The non-polynomial step in the Berlekamp-Hensel procedure was recently eliminated by van Hoeij in[19].This improvement is already implemented in Maple and Magma.As a consequence Trager’s algorithm in[20]for(iii),which previously was not polynomial time(even in the average case),is now also polynomial time.Multivariate factorization is polynomial time reducible to the univariate case for(ii)and (iii)and to the bivariate case for(i).Several polynomial time factorization algorithms for GF(q)[x,y]are known though they are not implemented in Axiom,Maple,Magma,and Mathematica.We mention those of von zur Gathen and Kaltofen in[21]and Noro and Yokoyama in[22].3:The Gr¨o bner basis computation problem.We do not mention here the research done in parallel Gr¨o bner basis computation and Gr¨o bner basis computation over non-standard rings because our focus is to build a solid algorithm for the most important cases in practice which can be plugged into Maple.Many of the algorithmic developments in Gr¨o bner basis computation have been modifica-tions of Buchberger’s original algorithm to reduce the number of unnecessary S-polynomials that are processed.We mention Buchberger’s“normal”selection strategy[23],the“sugar”selection strategy of Traverso et.al.[24],and Faugere’s new“F5”algorithm[25].We also mention two results of important practical significance.Thefirst is Faugere’s F4algorithm [26],which exploits sparse linear algebra to allow multiple pairs to be processed simultane-ously.The second is the“FGLM”algorithm of[27],which allows one to rapidly convert a Gr¨o bner basis for a zero dimensional ideal from one term ordering to another.A number of special purpose systems have been developed whose primary computational facility is Gr¨o bner basis computation.We mention Macaulay,Singular,CoCoa,and GB, each of which represents a substantial research project.Here we only give some details about the Gr¨o bner basis implementations in Maple,Mathematica,and Magma.Maple’s implementation is using the the sugar selection strategy.Mathematica’s and Magma’s are automatically using FGLM.Magma is also using the F4algorithm.Maple’s implementation compares poorly with Mathematica’s and very poorly with Magma’s and the other special purpose systems mentioned above.One reason for this is that the general purpose data structure that Maple is using to represent expressions is not suitable for implementing Buch-berger’s algorithm.In contrast,all other systems mentioned are using dedicated polynomial data structures.Modular methods can also be applied to Gr¨o bner basis computation.The main theo-retical difficulty is that we do not yet have an efficient termination test.In[28]Arnold has provided some progress towards such a test.In de Kleine and Monagan[29]we have developed a modular implementation using the sugar selection strategy and an appropriate polynomial data structure.Our algorithm is probabilistic;we stop when we havefive26bit primes(40decimal digits)of agreement.The implementation computes modulo b primes si-multaneously,in order to amortize the cost of the monomial arithmetic across several primes and it uses IEEE double precisionfloating point arithmetic to speed up computation in Z p.Detailed ProposalDiscuss the scientific issues,research problems or technical complexities,and describe the research methodology and experimental design proposed to explain or resolve them.Provide a workplan and relate it to the milestone schedule.The detailed proposal is divided into four sub-projects.1:Polynomial data structure sub-project.Thefirst sub-project is to build a data structure for polynomials in one or more variables over the integers,finitefields,and algebraic numberfields,and secondly to implement a good modular algorithm for the polynomial GCD operation for all cases,including both sparse and dense polynomials.This sub-project will be undertaken jointly with Waterloo Maple personnel.Our goal is to complete it in thefirst year of the project.In[16]we experimented with a recursive data structure for implementing GCDs over algebraic numberfields andfinitefields which we plan to adopt.The recursive dense data structure makes the implementation efficient and facilitates operations onfield extensions. Our approach will be to implement polynomial arithmetic and support operations,such as polynomial evaluation and interpolation,in the C language for efficiency,but to write most cases of the GCD algorithm in Maple forflexibility.This implementation approach was effective for Z[x]where the facility developed by Monagan in[30]for Z n[x]enabled us to implement modular algorithms for polynomial GCD,resultant and factorization in Z[x] efficiently in Maple.In ongoing work,de Kleine and Monagan have just completed an implementation of Zippel’s sparse modular GCD algorithm for multivariate GCDs over numberfields andfinite fields for the monic case.We propose to investigate different approaches for handling the non-monic case,including developing a sparse rational function reconstruction technique. 2:Polynomial factorization sub-project.The second sub-project is to implement known algorithms for polynomial factorization over the integers,finitefields and algebraic numberfields using the new data structure.We will begin this sub-project in the second year,after the polynomial data structure is imple-mented,and work on it through the end of the project.Recent asymptotic improvements in polynomial factorization and absolute factorization give us many opportunities to make improvements on existing implementations.As such,this sub-project will provide excellent training opportunities for graduate students.For example,we mention Kaltofen and Shoup’s subquadratic time algorithm[32]for factorization in GF(q)[x]and the practical recommendations made therein.Another place for improvement is factorization in GF(q)[x,y].The Maple implementation of Bernardin in [33]uses Hensel lifting tofind the factors modulo m(y)k.This is not polynomial time in the worst case because of afinal combinatorial step.We propose to modify the solution of van Hoeij[19]for Z[x]to work in GF(q)[y][x].This should yield an asymptotically faster algorithm than that of Noro and Yokoyama in[22].3:Gr¨o bner basis sub-project.The third sub-project is to develop an efficient data structure and implementation for com-puting Gr¨o bner bases of ideals in F [x 1,...,x n ]using modular methods.In [29],we imple-mented a modular algorithm for F =Q which exploits fast floating point arithmetic.In the first year we will extend our implementation to include Faugere’s F4algorithm,the FGLM algorithm [27],and to integrate it into the Maple library.For the latter two years of the project we propose the following:(i)To allow for parameters a,b,c,...in F we will use evaluation and rational functionreconstruction.When there is more than one parameter,we will develop a sparse rational reconstruction technique to improve the performance in the sparse case.The technical difficulties here are dealing with the many possible failures that can occur so that we can guarantee termination of the algorithm.(ii)To investigate the application of a modular Gr¨o bner basis algorithm to the polynomialGCD problem.Let F be a field,possibly with parameters.Let f 1,f 2∈F [x 1,x 2,...,x n ]and let g =GCD(f 1,f 2).The following approach to computing g is a refinement of an idea given to us by Gianni and Trager.Let α1,...,αn ∈F satisfy lc x 1(f 1)(α2,...,αn )=0and lc x 1(f 2)(α2,...,αn )=0.Then a multiple of the the primitive part of g will appear in a Gr¨o bner basis for the ideal I = f 1,f 2,(x 2−α2)1+b 2,...,(x n −αn )1+b n provided b i ≥deg x i (g ).Now since we can bound deg x i (g )quickly and accurately with one univariate GCD in F [x i ]and,assuming we have a good modular Gr¨o bner basis implementation for F ,this approach to compute g may prove competitive.An advantage is that it is easy to extend it to treat algebraic numbers and algebraic functions.4:Algebraic function fields sub-project.The fourth sub-project is to develop a modular algorithm for computing a polynomial GCD over an algebraic function field,and to make a prototype implementation in Maple.This is a necessary first step for the development of an efficient algorithm for factorization over algebraic function fields.Let m t (z )∈Q (t )[z ]be the minimal polynomial for A ,an algebraic function.For example,m t (z )=z 2−t is the minimal polynomial for A =√t .Given f 1,f 2∈F [x,y ]where F =Q (t,A ),the problem is to compute the monic GCD of f 1and f 2.We also need to consider the case where the algebraic function depends on more than one parameter,for example A = Such problems arise naturally in many science and engineering applications.If there are many parameters,we will again need to develop a sparse reconstruction technique.We propose to develop and prototype an algorithm for one field extension with multiple parameters in the first year and to develop the polynomial data structure from the first sub-project to support also (multiple)function field extensions in the latter two years.Remark on rational reconstruction techniques:In current work we have improved on the rational number reconstruction algorithm of Wang,Guy and Davenport [6]so that when reconstructing a rational number n/d from it’s image u modulo m ,the size of the modulus need only be a few bits longer than the size of nd ,irrespective of whether the n is larger then dor d is larger then n.This means that we need fewer primes in general to reconstruct the n/d. The idea should be applicable to rational function reconstruction case.An asymptotically fast algorithm also seems possible.In[14]Pan described an asymptotically fast algorithm for rational number reconstruction but remarks that the algorithm may not be practical. However,Steel,in unpublished work,had already implemented a simpler asymptotically fast rational number reconstruction algorithm in Magma and believes our algorithm can similarly be accelerated.Remark about the data structure approach:The computer algebra systems Axiom and Magma,and our own experimental system Gauss[31]provide generic facilities for building polynomials and rational functions over anyfield whereas the data structure approach we are taking in this project restricts thefield to the cases that we decide to support.Surely the generic approach is the better?For example,in Magma,one can build the ring F[x,y] mentioned above as follows.>Q:=RationalField();>K<t>:=FunctionField(Q);//Q(t)>P[z]:=PolynomialRing(K);//Q(t)[z]>m:=z^2-t;>F<A>:=quo<P|m>;//F=Q(t,A)>S<y>:=PolynomialRing(F);//F[y]>R<x>:=PolynomialRing(S);//F[y][x]The advantage is that an efficient representation,at least for basic polynomial arithmetic,is immediately available for any coefficient ring,in this example for Q,F,K and also S.Having implemented modular algorithms using both approaches it is our experience that a special purpose data structure will provide us with enough opportunities for greater efficiency that it is worthwhile having such a data structure for the common coefficientfields.Team ExpertiseExplain how the knowledge and experience of each team member relates to the expertise needed to accomplish the project objectives,and how the contributions of the team members will be integrated.The research area of each team member below is computer algebra.Dr.Michael Mona-gan,the principal investigator,has expertise in polynomial GCD computation and the other areas of this proposal.He has also worked on the Axiom and Magma urent Bernardin is head of the Mathematics group at Waterloo Maple.His Ph.D.was on poly-nomial factorization overfinitefields and he will contribute to sub-projects1and2.Dr. Mark van Hoeij of Florida State University,whose expertise in polynomial factorization and algebraic functions,will be contributing to sub-projects2and4.Mrs.Jennifer de Kleine, who has recently graduated with a Master’s in computer algebra,will be paid under the project to work on sub-projects1and3.Training of highly qualified personnelDescribe the opportunities the project offers for advanced training,or other relevant experi-ence,for students,post doctoral fellows,or supporting organizations’R&D staff.There are many good and difficult research and development problems to work on within the scope of this proposal.For the graduate students,company personnel,and other research personnel,this project will provide excellent training in computational mathematics with a good balance between theoretical algorithmic development and practical implementation.It involves writing software for polynomial computations over different rings from scratch.Few people will ever have the opportunity to do this for a system that will be widely used. Intellectual propertyDiscuss the plans for protecting and disposing of intellectual property arising from the grant. Outline the broad terms of the agreement between the company and the university on the rights to exploit the technology being transferred.Waterloo Maple has established an intellectual property agreement with another research project between Waterloo Maple and Simon Fraser University that we intend to adopt. That agreement gives Waterloo Maple ownership rights to own and use any code(and doc-umentation)developed by the researchers.In the case of our project,this is in our interest as we do want our software to be made available to the Maple user community worldwide. The best way to facilitate that is to have it integrated into Maple rather than reside in an applications library.The agreement gives graduate students and research personnel the right to publish all scientific results without delay.Value of the resultsDescribe the anticipated value of the project results,highlighting the relevance of the scien-tific or technical advances,or the innovative techniques,processes or products that will be developed.Show how the outcome will address a current or future industrial or market need. The main expected results of this project:•New and improved modular algorithms for polynomial GCD computation.•New and improved rational reconstruction algorithms.•A polynomial data structure for Maple for multivariate polynomials over the integers,finitefields,and numberfields,and modular algorithms for polynomial GCDs and factorization will improve Maple’s performance across a range of applications.In par-ticular,the improved support forfinitefields will open up new applications of Maple in coding theory and cryptography.•A software module for Maple which supports Gr¨o bner basis computation over the rationals(with parameters)using modular methods will make Maple competitive at an important computation with many applications.This is an ambitious project and I am excited about it.The project will help make Maple the best general purpose system for algebraic computation and,important for the research community,give Maple a strong foundation for future development.References[1]K.Geddes,C.Czapor,and bahn,Algorithms for Computer Algebra,Kluwer Academic,1996.[2]R.Zippel,Effective Polynomial Computation,Kluwer Academic,1993.[3]J.von zur Gathen and J.Gerhard,Modern Computer Algebra.U.of Cambridge Press,1999.[4]T.Becker and V.Weisspfenning,Gr¨o bner bases–A Computational Approach to CommutativeAlgebra.Springer-Verlag,New York,1993.[5]W.S.Brown,On Euclid’s Algorithm and the Computation of Polynomial Greatest CommonDivisors,J.ACM18(1971),pp.476–504.[6]P.Wang,The EEZ-GCD Algorithm.SIGSAM Bulletin,14(2)pp.50–60,1980.[7]R.Zippel,Probabilistic algorithms for sparse polynomials,Proc.of EUROSAM’79,Springer-Verlag LNCS,2(1979),pp.216–226.[8] E.Kaltofen,B.Trager,Computing with polynomials given by black boxes for their evaluations:Greatest common divisors,factorization,separation of numerators and denominators.J.Symb.Comp.,9,pp.301–320,1990.[9] B.Char,K.Geddes,G.Gonnet,Gcdheu:Heuristic polynomial GCD algorithm based on aninteger GCD p.,7,pp.31–48,1989.[10] E.Kaltofen,M.B.Monagan,On the Genericity of the Modular Polynomial GCD Algorithm.Proc.of ISSAC’99,ACM Press,pp.59–66,1999.[11]ngemyr,S.McCallum,The Computation of Polynomial GCD’s over an Algebraic NumberField,J.Symbolic Computation8(1989),pp.429–448.[12]M.J.Encarnacion,Computing GCDs of Polynomials over Algebraic Number Fields,J.Sym-bolic Computation20(1995),pp.299–313.[13]P.Wang,M.J.T.Guy,J.H.Davenport,p-adic Reconstruction of Rational Numbers,inSIGSAM Bulletin,16,No2(1982).[14]V.Pan,Acceleration of Euclidean Algorithm and Extensions.Proc.of ISSAC’2002,ACMPress,pp.207–213,2002.[15]K.O.Geddes,G.H.Gonnet,T.J.Smedley,Heuristic Methods for Operations with AlgebraicNumbers.Proc.of ISSAC’88,Springer-Verlag LNCS358,pp.475–480,1988.[16]M.van Hoeij,M.B.Monagan,A Modular GCD Algorithm over Number Fields Presentedwith Multiple Field Extensions.Proc.of ISSAC’2002,ACM Press,pp.109–116,2002.[17] E.Berlekamp,Factoring polynomials overfinitefields,Bell Systems Technical Journal,46,pp.1853–1859,1967.[18] A.Lenstra,H.Lenstra,L.Lovasz,Factoring Polynomials with Rational Coefficients,Mathe-matische Annalen,261pp.515–534,1982.[19]M.van Hoeij,Factoring Polynomials and the Knapsack Problem.J.Number Theory,95,pp.167–189,2002.[20] B.Trager,Algebraic Factoring and Rational Function Integration.Proc.of ISSAC’76,ACMPress,pp219–226,1976.[21]J.von zur Gathen,and E.Kaltofen,Factorization of Multivariate Polynomials over Finitep.45pp.251–261,1985.[22]M.Noro,K.Yokoyama,Yet another practical implementation of Polynomial Factorizationover Finite Fields.Proc.of ISSAC’02,ACM Press,pp.200–206,2002.[23] B.Buchberger,Groebner bases:an algorithmic method in polynomial ideal theory.Multidi-mensional Systems Theory,edited by N.K.Bose,D.Reidel Publishing Company,Dordrecht, pp.184-232,1985.[24] A.Giovini,T.Mora,G.Niesi,L.Robbiano,and C.Traverso,“One sugar cube,please”ORSelection strategies in the Buchberger algorithm.Proc.of ISSAC’91,ACM Press,pp.41–29, 1991.[25]J.Faugere,A New Efficient Algorithm for Computing Gr¨o bner Bases Without Reduction toZero(F5).Proc.of ISSAC’02,ACM Press,pp.75–83,2002.[26]J.Faugere,A New Efficient Algorithm for Computing Gr¨o bner Bases(F4).J.Pure and AppliedAlgebra,139,pp.61–83,1999.[27]J.Faugere,P.Gianni,zard,and T.Mora,Efficient computation of zero-dimensionalGr¨o bner bases by change of p.,16pp.329–244,1993.[28] E.Arnold,Modular Methods for Computing Groebner Bases.ISSAC’01Poster Session,2001.[29]J.de Kleine and M.B.Monagan,A Modular Design and Implementation of Buchberger’sAlgorithm.Proc.of RWCA’02,2002.[30]M.Monagan,In-place arithmetic for polynomials over Z n.Proc.of DISCO’92,Springer-VerlagLNCS,721,pp.22–34,1993.[31]M.Monagan,Gauss:a Parameterized Domain of Computation System with Support forSignature Functions.Proc.of DISCO’93,Springer-Verlag LNCS,722,pp.81–94,1993. [32] E.Kaltofen and V.Shoup,Subquadratic-Time Factoring of Polynomials over Finite Fields.p.67,pp.1179–1197,1998.[33]L.Bernardin,Factorization of Multivariate Polynomials over Finite Fields,Ph.D.Thesis,ETHZ¨u rich,1999.。
中国古代历史英语
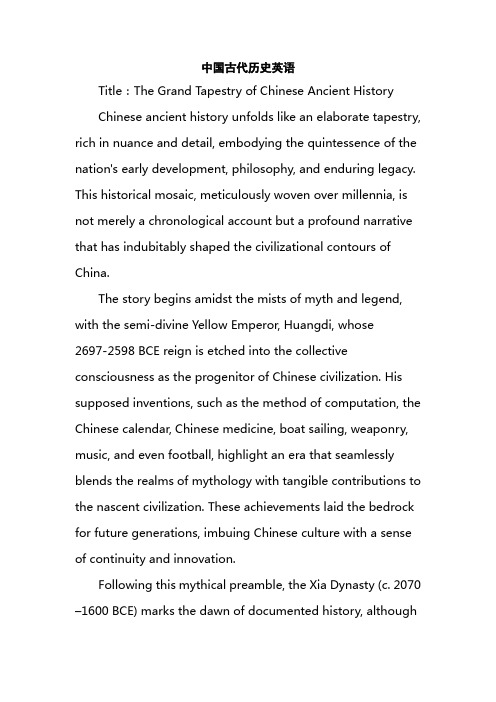
中国古代历史英语Title:The Grand Tapestry of Chinese Ancient HistoryChinese ancient history unfolds like an elaborate tapestry, rich in nuance and detail, embodying the quintessence of the nation's early development, philosophy, and enduring legacy. This historical mosaic, meticulously woven over millennia, is not merely a chronological account but a profound narrative that has indubitably shaped the civilizational contours of China.The story begins amidst the mists of myth and legend, with the semi-divine Yellow Emperor, Huangdi, whose2697-2598 BCE reign is etched into the collective consciousness as the progenitor of Chinese civilization. His supposed inventions, such as the method of computation, the Chinese calendar, Chinese medicine, boat sailing, weaponry, music, and even football, highlight an era that seamlessly blends the realms of mythology with tangible contributions to the nascent civilization. These achievements laid the bedrock for future generations, imbuing Chinese culture with a sense of continuity and innovation.Following this mythical preamble, the Xia Dynasty (c. 2070–1600 BCE) marks the dawn of documented history, althoughits existence remains intertwined with myth due to a dearth of archaeological evidence. Still, it represents a pivotal transition from society grounded in legend to one anchored in historical documentation, setting the stage for the Shang Dynasty (c. 1600–1046 BCE). The Shang are renowned for their advancements in writing, divination, and urban planning, notably through the intricacies of the oracle bones script and the majestic walls of Anyang. It is during this period that the blueprint for subsequent dynastic rule and cultural orthodoxy began to crystallize.The subsequent Zhou Dynasty (1046–256 BCE) introduced a paradigm shift with its feudal system, wherein power was decentralized amongst vassal states, each overseen by kings directly descended from the Zhou rulers. This era spawned the "Spring and Autumn" (770–476 BCE) and "Warring States" (475–221 BCE) periods, characterized by intense political fragmentation and intellectual ferment. It heralded the'Hundred Schools of Thought', including Confucianism, Daoism, and Legalism, which would leave an indelible mark on Chinese philosophical and ethical thought.The year 221 BCE marked the zenith of this turbulence with the Qin Dynasty's ascendancy, under whose brief yetimpactful rule, Emperor Qin Shi Huang orchestrated the unification of China. His legacy includes the iconic Great Wall, the standardization of currency, weights, and measures, and the establishment of a centralized imperial bureaucracy –pivotal elements that underscored the notion of a singular, cohesive empire.The succeeding Han Dynasty (206 BCE–220 CE) expanded upon this unification, ushering in a golden age characterized by the Silk Road's inauguration, which facilitated cultural and commercial exchanges with the Western world. The Han emperors' governance models, such as the imperial examination system for selecting bureaucrats, became blueprints for future administrations, reinforcing the ideals of meritocratic governance and scholarly excellence.As one delves deeper into the annals of China's ancient past, the intricate interplay of governance, culture, and innovation becomes evident. The Tang (618–907) and Song (960–1279) dynasties epitomize this interchange, showcasing artistic flourishes in poetry, porcelain, and painting, while also witnessing technological marvels like the invention of gunpowder and the compass. These eras underscore the synergy between cultural prowess and scientific ingenuity thathas come to define Chinese civilization.In retrospection, Chinese ancient history is far more than a chronological recitation; it is a testament to human ingenuity, philosophical depth, and unwavering perseverance. Each epoch, from the myth-laden origins to the zeniths of cultural and scientific achievements, weaves a continuous narrative that has indelibly shaped not only China but also the broader tapestry of global history. As one reflects upon this grand historical saga, it becomes evident that the echoes of the past continue to resonate within the heart of modern China, fostering a deep-seated pride and a collective memory that transcends the passage of time.。
线性代数(linearalgebra)
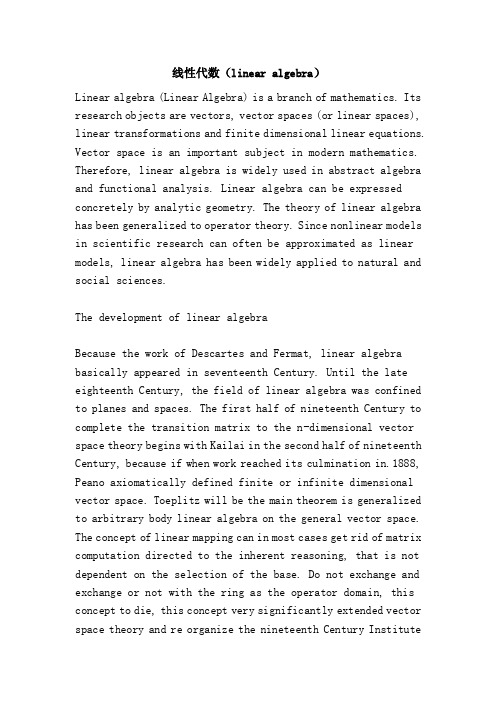
线性代数(linear algebra)Linear algebra (Linear Algebra) is a branch of mathematics. Its research objects are vectors, vector spaces (or linear spaces), linear transformations and finite dimensional linear equations. Vector space is an important subject in modern mathematics. Therefore, linear algebra is widely used in abstract algebra and functional analysis. Linear algebra can be expressed concretely by analytic geometry. The theory of linear algebra has been generalized to operator theory. Since nonlinear models in scientific research can often be approximated as linear models, linear algebra has been widely applied to natural and social sciences.The development of linear algebraBecause the work of Descartes and Fermat, linear algebra basically appeared in seventeenth Century. Until the late eighteenth Century, the field of linear algebra was confined to planes and spaces. The first half of nineteenth Century to complete the transition matrix to the n-dimensional vector space theory begins with Kailai in the second half of nineteenth Century, because if when work reached its culmination in.1888, Peano axiomatically defined finite or infinite dimensional vector space. Toeplitz will be the main theorem is generalized to arbitrary body linear algebra on the general vector space. The concept of linear mapping can in most cases get rid of matrix computation directed to the inherent reasoning, that is not dependent on the selection of the base. Do not exchange and exchange or not with the ring as the operator domain, this concept to die, this concept very significantly extended vector space theory and re organize the nineteenth Century Instituteof the.The word "algebra" appeared relatively late in China, in the Qing Dynasty when the incoming China, it was translated into "Alj Bala", until 1859, the Qing Dynasty famous mathematician, translator Li Shanlan translated it as "algebra", still in use.The status of linear algebraLinear algebra is a subject that discusses matrix theory and finite dimensional vector spaces combined with matrices and their linear transformation theory.The main theory is mature in nineteenth Century, and the first cornerstone (the solution of two or three Yuan linear equations) appeared as early as two thousand years ago (see in our ancient mathematical masterpiece "nine chapters arithmetic").The linear algebra has many important applications in mathematics, mechanics, physics and technology, so it has important place in various branches of algebra;In the computer today, computer graphics, computer aided design, cryptography, virtual reality and so on are all part of the theory and algorithm of linear algebra;.Between geometric and algebraic methods embodied in the concept of the subject of the connection from the axiomatic method on the abstract concept and rigorous logic reasoning, cleverly summed up, to strengthen people's training in mathematics, science and intelligent gain is very useful;And with the development of science, we should not only study the relationship between the individual variables, but also further study the relationship between multiple variables, all kinds of practical problems in most cases can be linearized, and because of the development of the computer, the linearized problem can be calculated, linear algebra is a powerful tool to solve these problems.Basic introduction to linear algebraLinear algebra originated from the study of two-dimensional and three-dimensional Cartesian coordinate systems. Here, a vector is a line segment with a direction that is represented by both length and direction. Thus vectors can be used to represent physical quantities, such as force, or to add and multiply scalar quantities. This is the first example of a real vector space.Modern linear algebra has been extended to study arbitrary or infinite dimensional spaces. A vector space of dimension n is called n-dimensional space. In two-dimensional andthree-dimensional space, most useful conclusions can be extended to these high-dimensional spaces. Although many people do not easily imagine vectors in n-dimensional space, such vectors (i.e., n tuples) are very useful for representing data. Since n is a tuple, and the vector is an ordered list of n elements, most people can effectively generalize and manipulate data in this framework. For example, in economics, 8 dimensional vectors can be used to represent the gross national product (GNP) of 8 countries. When all the nationalorder (such as scheduled, China, the United States, Britain, France, Germany, Spain, India, Australia), you can use the vector (V1, V2, V3, V4, V5, V6, V7, V8) showed that these countries a year each GNP. Here, each country's GNP are in their respective positions.As a purely abstract concept used in proving theorems, vector spaces (linear spaces) are part of abstract algebra and have been well integrated into this field. Some notable examples are: irreversible linear maps or groups of matrices, rings of linear mappings in vector spaces. Linear algebra also plays an important role in mathematical analysis,Especially in vector analysis, higher order derivatives are described, and tensor product and commutative mapping are studied.A vector space is defined on a domain, such as a real or complex domain. Linear operators map the elements of a linear space into another linear space (or in the same linear space), and maintain the consistency of addition and scalar multiplication in the vector space. The set of all such transformations is itself a vector space. If a basis of linear space is determined, all linear transformations can be expressed as a table, called matrix. Further studies of matrix properties and matrix algorithms (including determinants and eigenvectors) are also considered part of linear algebra.We can simply say that the linear problems in Mathematics - those that exhibit linear problems - are most likely to be solved. For example, differential calculus studies the problemof linear approximation of functions. In practice, the difference between a nonlinear problem and a nonlinear one is very important.The linear algebra method refers to the problem of using a linear viewpoint to describe it and to describe it in the language of linear algebra and to solve it (when necessary) by using matrix operations. This is one of the most important applications in mathematics and engineering.Some useful theoremsEvery linear space has a base.The nonzero matrix n for a row of N rows A, if there is a matrix B that makes AB = BA = I (I is the unit matrix), then A is nonsingular matrix.A matrix is nonsingular if and only if its determinant is not zero.A matrix is nonsingular if and only if the linear transformation it represents is a automorphism.A matrix is semi positive if and only if each of its eigenvalues is greater than or equal to zero.A matrix is positive if and only if each of its eigenvalues is greater than zero.Generalizations and related topicsLinear algebra is a successful theory, and its method has been applied to other branches of mathematics.The theory of modulus is to study the substitution of scalar domains in linear algebra by ring substitution.Multilinear algebra transforms the "multivariable" problem of mapping into the problem of each variable, resulting in the concept of tensor.In the spectral theory of operators, by using mathematical analysis, infinite dimensional matrices can be controlled.All of these areas have very large technical difficulties.Basic contents of linear algebra in Chinese UniversitiesFirst, the nature and tasks of the courseThe course of linear algebra is an important basic theory course required by students of science and Engineering in universities and colleges. It is widely used in every field of science and technology. Especially today, with the development and popularization of computer, linear algebra has become the basic theory knowledge and important mathematical tool for engineering students. Linear algebra is to train thehigh-quality specialized personnel needed for the socialist modernization construction of our country. Through the study of this course, we should make students get:1 determinant2, matrix3. The correlation of vectors and the rank of matrices4 、 linear equations5, similar matrix and two typeAnd other basic concepts, basic theories and basic operational skills, and lay the necessary mathematical foundation for further courses and further knowledge of mathematics.While imparting knowledge through various teaching links gradually cultivate students with abstract thinking ability, logical reasoning ability, spatial imagination ability and self-learning ability, but also pay special attention to cultivate students with good operation ability and comprehensive use of the knowledge to the ability to analyze and solve problems.Two, the content of the course teaching, basic requirements and class allocation(1) teaching content1 determinant(1) definition of order n determinant(2) the nature of determinant(3) the calculation of the determinant is carried out in rows (columns)(4) the Clem rule for solving linear equations2, matrix(1) the concept of matrix, unit matrix, diagonal matrix, symmetric matrix(2) linear operations, multiplication operations, transpose operations and laws of matrices(3) inverse matrix concept and its properties, and inverse matrix with adjoint matrix(4) the operation of partitioned matrices3 vector(1) the concept of n-dimensional vectors(2) the linear correlation, linear independence definition and related theorems of vector groups, and the judgement of linear correlation(3) the maximal independent group of vectors and the rank of vectors(4) the concept of rank of matrix(5) elementary transformation of matrix, rank and inverse matrix of matrix by elementary transformation(6) n-dimensional vector spaces and subspaces, bases, dimensions, coordinates of vectors4 、 linear equations(1) the necessary and sufficient conditions for the existence of nonzero solutions of homogeneous linear equations and the necessary and sufficient conditions for the existence of solutions of nonhomogeneous linear equations(2) the fundamental solution, the general solution and the solution structure of the system of linear equations(3) the condition and judgement of the solution of nonhomogeneous linear equations and the solution of the system of equations(4) finding the general solution of linear equations by elementary row transformation5, similar matrix and two type(1) eigenvalues and eigenvectors of matrices and their solutions(2) similarity matrix and its properties(3) the necessary and sufficient conditions and methods of diagonalization of matrices(4) similar diagonal matrices of real symmetric matrices(5) two type and its matrix representation(6) the method of linearly independent vector group orthogonal normalization(7) the concept and property of orthogonal transformation and orthogonal matrix(8) orthogonal transformation is used as the standard shape of the two type(9) the canonical form of quadratic form and two form of two type are formulated by formula(10) the inertia theorem, the rank of the two type, the positive definite of the two type and their discrimination(two) basic requirements1, understand the definition of order n determinant, will use the definition of simple determinant calculation2, master the basic calculation methods and properties of determinant3, master Clem's law4. Understand the definition of a matrix5, master the matrix operation method and inverse matrix method6. Understanding the concept of vector dependency defines the relevance of the vector by definition7, grasp the method of finding the rank of the matrix, and understand the relation between the rank of the matrix and the correlation of the vector group8, understand the concept of vector space, will seek vector coordinates9. Master the matrix rank and inverse matrix with elementary transformation, and solve the system of linear equations10, master the method of solving linear equations, and know the simple application of linear equations11. Master the method of matrix eigenvalue and eigenvector12. Grasp the concept of similar matrices and the concept of diagonalization of matrices13, master the orthogonal transformation of two times for standard type method14, understand the inertia theorem of the two type, and use thematching method to find the sum of squares of the two type15. Grasp the concept and application of the positive definiteness of the two typeMATLABIt is a programming language and can be used as a teaching software for engineering linear algebra. It has been introduced into many university textbooks at home and abroad.。
- 1、下载文档前请自行甄别文档内容的完整性,平台不提供额外的编辑、内容补充、找答案等附加服务。
- 2、"仅部分预览"的文档,不可在线预览部分如存在完整性等问题,可反馈申请退款(可完整预览的文档不适用该条件!)。
- 3、如文档侵犯您的权益,请联系客服反馈,我们会尽快为您处理(人工客服工作时间:9:00-18:30)。
2
T. GANTUMUR AND R.P. STEVENSON
The main reason why a stiffness matrix entry is small is that the kernel of the involved integral operator is increasingly smooth away from its diagonal, and that the wavelets have vanishing moments, i.e., wavelets are L2 -orthogonal to polynomials up to a certain degree. Another advantage of the Galerkin-wavelet discretization is that the diagonally scaled stiffness matrices are well-conditioned uniformly in their sizes, guaranteeing a uniform convergence rate of iterative methods for the linear systems. Moreover, recent developments suggest a natural use of wavelets in adaptive discretization methods that approximate the solution using, up to a constant factor, as few degrees of freedom as possible. In the following, we will consider the adaptive wavelet method from [CDD02]. Let H t (Γ) be the usual Sobolev space defined on a sufficiently smooth n-dimensional manifold Γ ⊂ I Rn+1 , and let H −t (Γ) be its dual space. Then we consider the problem of finding the solution u ∈ H t (Γ) of Lu = g, where L : H (Γ) → H (Γ) is a boundedly invertible linear operator, and g ∈ H −t (Γ). We will think of this problem as being the result of a variational formulation of a strongly elliptic boundary integral equation of order 2t. With Ψ = {ψ λ : λ ∈ Λ} being a Riesz basis for H t (Γ), an equivalent infinite matrix-vector problem reads as (1.1) Mu = g, where, with , denoting the duality product on H t (Γ) × H −t (Γ), M := Ψ, LΨ : T 2 (Λ) → 2 (Λ) is boundedly invertible, g := Ψ, g ∈ 2 (Λ), and u = u Ψ. Considering Ψ to be a wavelet basis, in [CDD02] an iterative adaptive method has been developed for approximating the solution of this infinite dimensional problem by a finitely supported vector within any given tolerance. Roughly speaking, the method consists of the application of a simple iterative scheme to the infinite matrixvector problem, where each application of the infinite stiffness matrix M is replaced by an inexact version. To assess the quality of the method, the 2 (Λ)-error of the obtained approximation after spending O(N ) operations is compared with that of a best N -term approximation for u, i.e., a vector uN with at most N non-zero coefficients that has 2 (Λ)-distance to u less or equal to that of any vector with a support of that size. In any case for wavelets that are sufficiently smooth, the theory of non-linear approximation ([DeV98]) shows that if both 0<s<
COMPUTATION OF SINGULAR INTEGRAL OPERATORS IN WAVELET COORDINATES
TSOGTGEREL GANTUMUR AND ROB STEVENSON Abstract. With respect to a wavelet basis, singular integral operators can be well approximated by sparse matrices, and in [Found. Comput. Math., 2 (2002), pp. 203–245] and [SIAM J. Math. Anal., 35 (2004), pp. 1110–1132], this property was used to prove certain optimal complexity results in the context of adaptive wavelet methods. These results, however, were based upon the assumption that, on average, each entry of the approximating sparse matrices can be computed at unit cost. In this paper, we confirm this assumption by carefully distributing computational costs over the matrix entries in combination with choosing efficient quadrature schemes.
Date : January 20, 2005. 2000 Mathematics Subject Classification. 41A25, 47A20, 65D30, 65F50, 65N38. Key words and phrases. Wavelets, matrix compression, boundary integral operators, adaptivity, numerical integration, singular integrals, nearly singular integrals. This work was supported by the Netherlands Organization for Scientific Research and by the EC-IHP project “Breaking Complexity”.
1. Introduction Boundary integral methods reduce elliptic boundary value problems in domains to integral equations formulated on the boundary of the domain. Although the dimension of the underlying manifold decreases by one, the finite element discretization of the resulting boundary integral equations gives densely populated stiffness matrices, causing serious obstructions to accurate numerical solution processes. n order to overcome this difficulty, various successful approaches for approximating the stiffness matrix by sparse ones have been developed, such as multipole expansions, panel clustering, and wavelet compression, see e.g. [Atk97, Hac95]. We will restrict ourselves here to the latter approach. In [BCR91], Beylkin, Coifman and Rokhlin first observed that wavelet bases give rise to almost sparse stiffness matrices for the Galerkin discretization of singular integral operators, meaning that the stiffness matrix has many small entries that can be discarded without reducing the order of convergence of the resulting solution. This result ignited the development of efficient compression techniques for boundary integral equations based upon wavelets. In [vPS97, Sch98, DHS02] it was shown that for a wide class of boundary integral operators a wavelet basis can be chosen so that the full accuracy of the Galerkin discretization can be retained at a computational work of the order N (possibly with a logarithmic factor in some studies), where N is the number of degrees of freedom used in the discretization. First nontrivial implementations of these algorithms and their performance tests are reported in [LS99, Har01].