On Non-Perturbative Results in Supersymmetric Gauge Theories - A Lecture
Superoperator Representation of Nonlinear Response Unifying Quantum Field and Mode Coupling

Shaul Mukamel Department of Chemistry, University of California, Irvine, CA 92697-2025
(Dated: February 2, 2008)
Abstract
Computing response functions by following the time evolution of superoperators in Liouville space (whose vectors are ordinary Hilbert space operators) offers an attractive alternative to the diagrammatic perturbative expansion of many-body equilibrium and nonequilibrium Green functions. The bookkeeping of time ordering is naturally maintained in real (physical) time, allowing the formulation of Wick’s theorem for superoperators, giving a factorization of higher order response functions in terms of two fundamental Green’s functions. Backward propagations and the analytic continuations using artificial times (Keldysh loops and Matsubara contours) are avoided. A generating functional for nonlinear response functions unifies quantum field theory and the classical mode coupling formalism of nonlinear hydrodynamics and may be used for semiclassical expansions. Classical response functions are obtained without the explicit computation of stability matrices.
c, and

1Hale Waihona Puke 2)the truncation of the perturbation series at a xed order. They manifest themselves in the (unphysical) renormalization-scale dependence of theoretical predictions. This dependence is numerically strong at leading order, because the coupling is still relatively large, and runs relatively quickly. Next-to-leading order e ects compensate this sensitivity, and in practice reduce signi cantly the undesired renormalization-scale dependence. The second type of logarithms arise because of the presence of di erent scales, a hard scale characterizing the scattering, and softer scales characterizing the size of a jet. They modify the perturbative expansion from one solely in s to one in s ln2 yIR and s ln yIR as well, where yIR is the ratio of the di erent scales. Only at next-to-leading order can we justify quantitatively the harmless nature of these logarithms, and thereby the applicability of perturbation theory.
Nonperturbative heat kernel and nonlocal effective action

(1.2)
The first few orders of this expansion are graphically depicted below as Feynman graphs with the propagator – the Green’s function of the operator (1.1) – and relevant vertices calculated on the background of a generic ϕ Γ1−loop 1 1 = Tr ln F (∇) = 2 2
s
(1.3)
1 1 s + . (1.4) Γ2−loop = 8 12 s The one-loop part (1.3) is peculiar in that it does not explicitly contain the vertices of the classical action (unless it is expanded in powers of the mean field ϕ) and given by the functional trace of the logarithm of F (∇). In local field theories without loss of generality the operator (1.1) has the form F ( ∇) = − V (x), 3 (1.5)
1. Introduction: heat kernel and effective action 2. Approximation schemes and infrared behavior 2.1. Schwinger-DeWitt technique of local expansion . . . . . . . . . . . . . 2.2. Modified Schwinger-DeWitt expansion . . . . . . . . . . . . . . . . . . 2.3. Covariant perturbation theory . . . . . . . . . . . . . . . . . . . . . . . 3. Nonperturbative late-time asymptotics 3.1. Leading order . . . . . . . . . . . . . . . . . . . . . . . . . . . . . . . . 3.2. Subleading order . . . . . . . . . . . . . . . . . . . . . . . . . . . . . . 3.3. Resummation of covariant perturbation theory . . . . . . . . . . . . . . 4. New nonlocal effective action 5. Inclusion of gravity 5.1. Leading order . . . . . . . . . . . . . . . . . . . . . . . . . . . . . . . . 5.2. Conformal properties . . . . . . . . . . . . . . . . . . . . . . . . . . . . 5.3. Problems with the subleading order . . . . . . . . . . . . . . . . . . . . 6. Discussion and conclusions A. Late time asymptotics in perturbation theory B. Nonperturbative effective action B.1. Small potential . . . . . . . . . . . . . . . . . . . . . . . . . . . . . . . B.2. Big potential . . . . . . . . . . . . . . . . . . . . . . . . . . . . . . . . C. Nonlocal form of the Gibbons-Hawking surface integral D. Metric dependence in the subleading order 3 5 5 7 9 10 11 12 13 15 18 19 23 25 27 30 32 33 35 36 38
《神经网络与深度学习综述DeepLearning15May2014
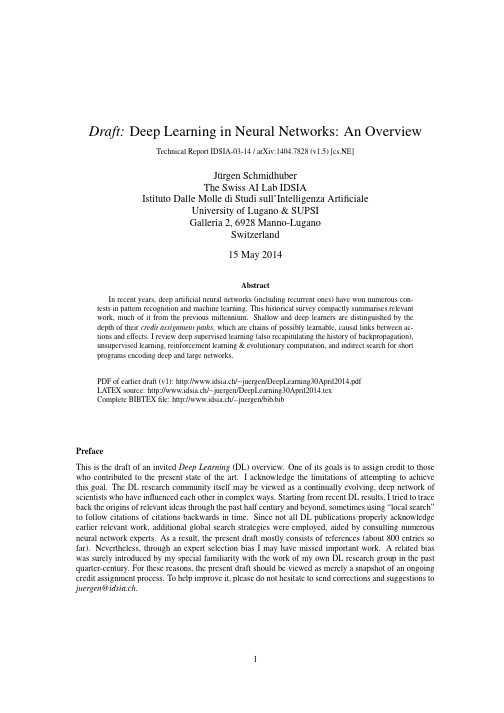
Draft:Deep Learning in Neural Networks:An OverviewTechnical Report IDSIA-03-14/arXiv:1404.7828(v1.5)[cs.NE]J¨u rgen SchmidhuberThe Swiss AI Lab IDSIAIstituto Dalle Molle di Studi sull’Intelligenza ArtificialeUniversity of Lugano&SUPSIGalleria2,6928Manno-LuganoSwitzerland15May2014AbstractIn recent years,deep artificial neural networks(including recurrent ones)have won numerous con-tests in pattern recognition and machine learning.This historical survey compactly summarises relevantwork,much of it from the previous millennium.Shallow and deep learners are distinguished by thedepth of their credit assignment paths,which are chains of possibly learnable,causal links between ac-tions and effects.I review deep supervised learning(also recapitulating the history of backpropagation),unsupervised learning,reinforcement learning&evolutionary computation,and indirect search for shortprograms encoding deep and large networks.PDF of earlier draft(v1):http://www.idsia.ch/∼juergen/DeepLearning30April2014.pdfLATEX source:http://www.idsia.ch/∼juergen/DeepLearning30April2014.texComplete BIBTEXfile:http://www.idsia.ch/∼juergen/bib.bibPrefaceThis is the draft of an invited Deep Learning(DL)overview.One of its goals is to assign credit to those who contributed to the present state of the art.I acknowledge the limitations of attempting to achieve this goal.The DL research community itself may be viewed as a continually evolving,deep network of scientists who have influenced each other in complex ways.Starting from recent DL results,I tried to trace back the origins of relevant ideas through the past half century and beyond,sometimes using“local search”to follow citations of citations backwards in time.Since not all DL publications properly acknowledge earlier relevant work,additional global search strategies were employed,aided by consulting numerous neural network experts.As a result,the present draft mostly consists of references(about800entries so far).Nevertheless,through an expert selection bias I may have missed important work.A related bias was surely introduced by my special familiarity with the work of my own DL research group in the past quarter-century.For these reasons,the present draft should be viewed as merely a snapshot of an ongoing credit assignment process.To help improve it,please do not hesitate to send corrections and suggestions to juergen@idsia.ch.Contents1Introduction to Deep Learning(DL)in Neural Networks(NNs)3 2Event-Oriented Notation for Activation Spreading in FNNs/RNNs3 3Depth of Credit Assignment Paths(CAPs)and of Problems4 4Recurring Themes of Deep Learning54.1Dynamic Programming(DP)for DL (5)4.2Unsupervised Learning(UL)Facilitating Supervised Learning(SL)and RL (6)4.3Occam’s Razor:Compression and Minimum Description Length(MDL) (6)4.4Learning Hierarchical Representations Through Deep SL,UL,RL (6)4.5Fast Graphics Processing Units(GPUs)for DL in NNs (6)5Supervised NNs,Some Helped by Unsupervised NNs75.11940s and Earlier (7)5.2Around1960:More Neurobiological Inspiration for DL (7)5.31965:Deep Networks Based on the Group Method of Data Handling(GMDH) (8)5.41979:Convolution+Weight Replication+Winner-Take-All(WTA) (8)5.51960-1981and Beyond:Development of Backpropagation(BP)for NNs (8)5.5.1BP for Weight-Sharing Feedforward NNs(FNNs)and Recurrent NNs(RNNs)..95.6Late1980s-2000:Numerous Improvements of NNs (9)5.6.1Ideas for Dealing with Long Time Lags and Deep CAPs (10)5.6.2Better BP Through Advanced Gradient Descent (10)5.6.3Discovering Low-Complexity,Problem-Solving NNs (11)5.6.4Potential Benefits of UL for SL (11)5.71987:UL Through Autoencoder(AE)Hierarchies (12)5.81989:BP for Convolutional NNs(CNNs) (13)5.91991:Fundamental Deep Learning Problem of Gradient Descent (13)5.101991:UL-Based History Compression Through a Deep Hierarchy of RNNs (14)5.111992:Max-Pooling(MP):Towards MPCNNs (14)5.121994:Contest-Winning Not So Deep NNs (15)5.131995:Supervised Recurrent Very Deep Learner(LSTM RNN) (15)5.142003:More Contest-Winning/Record-Setting,Often Not So Deep NNs (16)5.152006/7:Deep Belief Networks(DBNs)&AE Stacks Fine-Tuned by BP (17)5.162006/7:Improved CNNs/GPU-CNNs/BP-Trained MPCNNs (17)5.172009:First Official Competitions Won by RNNs,and with MPCNNs (18)5.182010:Plain Backprop(+Distortions)on GPU Yields Excellent Results (18)5.192011:MPCNNs on GPU Achieve Superhuman Vision Performance (18)5.202011:Hessian-Free Optimization for RNNs (19)5.212012:First Contests Won on ImageNet&Object Detection&Segmentation (19)5.222013-:More Contests and Benchmark Records (20)5.22.1Currently Successful Supervised Techniques:LSTM RNNs/GPU-MPCNNs (21)5.23Recent Tricks for Improving SL Deep NNs(Compare Sec.5.6.2,5.6.3) (21)5.24Consequences for Neuroscience (22)5.25DL with Spiking Neurons? (22)6DL in FNNs and RNNs for Reinforcement Learning(RL)236.1RL Through NN World Models Yields RNNs With Deep CAPs (23)6.2Deep FNNs for Traditional RL and Markov Decision Processes(MDPs) (24)6.3Deep RL RNNs for Partially Observable MDPs(POMDPs) (24)6.4RL Facilitated by Deep UL in FNNs and RNNs (25)6.5Deep Hierarchical RL(HRL)and Subgoal Learning with FNNs and RNNs (25)6.6Deep RL by Direct NN Search/Policy Gradients/Evolution (25)6.7Deep RL by Indirect Policy Search/Compressed NN Search (26)6.8Universal RL (27)7Conclusion271Introduction to Deep Learning(DL)in Neural Networks(NNs) Which modifiable components of a learning system are responsible for its success or failure?What changes to them improve performance?This has been called the fundamental credit assignment problem(Minsky, 1963).There are general credit assignment methods for universal problem solvers that are time-optimal in various theoretical senses(Sec.6.8).The present survey,however,will focus on the narrower,but now commercially important,subfield of Deep Learning(DL)in Artificial Neural Networks(NNs).We are interested in accurate credit assignment across possibly many,often nonlinear,computational stages of NNs.Shallow NN-like models have been around for many decades if not centuries(Sec.5.1).Models with several successive nonlinear layers of neurons date back at least to the1960s(Sec.5.3)and1970s(Sec.5.5). An efficient gradient descent method for teacher-based Supervised Learning(SL)in discrete,differentiable networks of arbitrary depth called backpropagation(BP)was developed in the1960s and1970s,and ap-plied to NNs in1981(Sec.5.5).BP-based training of deep NNs with many layers,however,had been found to be difficult in practice by the late1980s(Sec.5.6),and had become an explicit research subject by the early1990s(Sec.5.9).DL became practically feasible to some extent through the help of Unsupervised Learning(UL)(e.g.,Sec.5.10,5.15).The1990s and2000s also saw many improvements of purely super-vised DL(Sec.5).In the new millennium,deep NNs havefinally attracted wide-spread attention,mainly by outperforming alternative machine learning methods such as kernel machines(Vapnik,1995;Sch¨o lkopf et al.,1998)in numerous important applications.In fact,supervised deep NNs have won numerous of-ficial international pattern recognition competitions(e.g.,Sec.5.17,5.19,5.21,5.22),achieving thefirst superhuman visual pattern recognition results in limited domains(Sec.5.19).Deep NNs also have become relevant for the more generalfield of Reinforcement Learning(RL)where there is no supervising teacher (Sec.6).Both feedforward(acyclic)NNs(FNNs)and recurrent(cyclic)NNs(RNNs)have won contests(Sec.5.12,5.14,5.17,5.19,5.21,5.22).In a sense,RNNs are the deepest of all NNs(Sec.3)—they are general computers more powerful than FNNs,and can in principle create and process memories of ar-bitrary sequences of input patterns(e.g.,Siegelmann and Sontag,1991;Schmidhuber,1990a).Unlike traditional methods for automatic sequential program synthesis(e.g.,Waldinger and Lee,1969;Balzer, 1985;Soloway,1986;Deville and Lau,1994),RNNs can learn programs that mix sequential and parallel information processing in a natural and efficient way,exploiting the massive parallelism viewed as crucial for sustaining the rapid decline of computation cost observed over the past75years.The rest of this paper is structured as follows.Sec.2introduces a compact,event-oriented notation that is simple yet general enough to accommodate both FNNs and RNNs.Sec.3introduces the concept of Credit Assignment Paths(CAPs)to measure whether learning in a given NN application is of the deep or shallow type.Sec.4lists recurring themes of DL in SL,UL,and RL.Sec.5focuses on SL and UL,and on how UL can facilitate SL,although pure SL has become dominant in recent competitions(Sec.5.17-5.22). Sec.5is arranged in a historical timeline format with subsections on important inspirations and technical contributions.Sec.6on deep RL discusses traditional Dynamic Programming(DP)-based RL combined with gradient-based search techniques for SL or UL in deep NNs,as well as general methods for direct and indirect search in the weight space of deep FNNs and RNNs,including successful policy gradient and evolutionary methods.2Event-Oriented Notation for Activation Spreading in FNNs/RNNs Throughout this paper,let i,j,k,t,p,q,r denote positive integer variables assuming ranges implicit in the given contexts.Let n,m,T denote positive integer constants.An NN’s topology may change over time(e.g.,Fahlman,1991;Ring,1991;Weng et al.,1992;Fritzke, 1994).At any given moment,it can be described as afinite subset of units(or nodes or neurons)N= {u1,u2,...,}and afinite set H⊆N×N of directed edges or connections between nodes.FNNs are acyclic graphs,RNNs cyclic.Thefirst(input)layer is the set of input units,a subset of N.In FNNs,the k-th layer(k>1)is the set of all nodes u∈N such that there is an edge path of length k−1(but no longer path)between some input unit and u.There may be shortcut connections between distant layers.The NN’s behavior or program is determined by a set of real-valued,possibly modifiable,parameters or weights w i(i=1,...,n).We now focus on a singlefinite episode or epoch of information processing and activation spreading,without learning through weight changes.The following slightly unconventional notation is designed to compactly describe what is happening during the runtime of the system.During an episode,there is a partially causal sequence x t(t=1,...,T)of real values that I call events.Each x t is either an input set by the environment,or the activation of a unit that may directly depend on other x k(k<t)through a current NN topology-dependent set in t of indices k representing incoming causal connections or links.Let the function v encode topology information and map such event index pairs(k,t)to weight indices.For example,in the non-input case we may have x t=f t(net t)with real-valued net t= k∈in t x k w v(k,t)(additive case)or net t= k∈in t x k w v(k,t)(multiplicative case), where f t is a typically nonlinear real-valued activation function such as tanh.In many recent competition-winning NNs(Sec.5.19,5.21,5.22)there also are events of the type x t=max k∈int (x k);some networktypes may also use complex polynomial activation functions(Sec.5.3).x t may directly affect certain x k(k>t)through outgoing connections or links represented through a current set out t of indices k with t∈in k.Some non-input events are called output events.Note that many of the x t may refer to different,time-varying activations of the same unit in sequence-processing RNNs(e.g.,Williams,1989,“unfolding in time”),or also in FNNs sequentially exposed to time-varying input patterns of a large training set encoded as input events.During an episode,the same weight may get reused over and over again in topology-dependent ways,e.g.,in RNNs,or in convolutional NNs(Sec.5.4,5.8).I call this weight sharing across space and/or time.Weight sharing may greatly reduce the NN’s descriptive complexity,which is the number of bits of information required to describe the NN (Sec.4.3).In Supervised Learning(SL),certain NN output events x t may be associated with teacher-given,real-valued labels or targets d t yielding errors e t,e.g.,e t=1/2(x t−d t)2.A typical goal of supervised NN training is tofind weights that yield episodes with small total error E,the sum of all such e t.The hope is that the NN will generalize well in later episodes,causing only small errors on previously unseen sequences of input events.Many alternative error functions for SL and UL are possible.SL assumes that input events are independent of earlier output events(which may affect the environ-ment through actions causing subsequent perceptions).This assumption does not hold in the broaderfields of Sequential Decision Making and Reinforcement Learning(RL)(Kaelbling et al.,1996;Sutton and Barto, 1998;Hutter,2005)(Sec.6).In RL,some of the input events may encode real-valued reward signals given by the environment,and a typical goal is tofind weights that yield episodes with a high sum of reward signals,through sequences of appropriate output actions.Sec.5.5will use the notation above to compactly describe a central algorithm of DL,namely,back-propagation(BP)for supervised weight-sharing FNNs and RNNs.(FNNs may be viewed as RNNs with certainfixed zero weights.)Sec.6will address the more general RL case.3Depth of Credit Assignment Paths(CAPs)and of ProblemsTo measure whether credit assignment in a given NN application is of the deep or shallow type,I introduce the concept of Credit Assignment Paths or CAPs,which are chains of possibly causal links between events.Let usfirst focus on SL.Consider two events x p and x q(1≤p<q≤T).Depending on the appli-cation,they may have a Potential Direct Causal Connection(PDCC)expressed by the Boolean predicate pdcc(p,q),which is true if and only if p∈in q.Then the2-element list(p,q)is defined to be a CAP from p to q(a minimal one).A learning algorithm may be allowed to change w v(p,q)to improve performance in future episodes.More general,possibly indirect,Potential Causal Connections(PCC)are expressed by the recursively defined Boolean predicate pcc(p,q),which in the SL case is true only if pdcc(p,q),or if pcc(p,k)for some k and pdcc(k,q).In the latter case,appending q to any CAP from p to k yields a CAP from p to q(this is a recursive definition,too).The set of such CAPs may be large but isfinite.Note that the same weight may affect many different PDCCs between successive events listed by a given CAP,e.g.,in the case of RNNs, or weight-sharing FNNs.Suppose a CAP has the form(...,k,t,...,q),where k and t(possibly t=q)are thefirst successive elements with modifiable w v(k,t).Then the length of the suffix list(t,...,q)is called the CAP’s depth (which is0if there are no modifiable links at all).This depth limits how far backwards credit assignment can move down the causal chain tofind a modifiable weight.1Suppose an episode and its event sequence x1,...,x T satisfy a computable criterion used to decide whether a given problem has been solved(e.g.,total error E below some threshold).Then the set of used weights is called a solution to the problem,and the depth of the deepest CAP within the sequence is called the solution’s depth.There may be other solutions(yielding different event sequences)with different depths.Given somefixed NN topology,the smallest depth of any solution is called the problem’s depth.Sometimes we also speak of the depth of an architecture:SL FNNs withfixed topology imply a problem-independent maximal problem depth bounded by the number of non-input layers.Certain SL RNNs withfixed weights for all connections except those to output units(Jaeger,2001;Maass et al.,2002; Jaeger,2004;Schrauwen et al.,2007)have a maximal problem depth of1,because only thefinal links in the corresponding CAPs are modifiable.In general,however,RNNs may learn to solve problems of potentially unlimited depth.Note that the definitions above are solely based on the depths of causal chains,and agnostic of the temporal distance between events.For example,shallow FNNs perceiving large“time windows”of in-put events may correctly classify long input sequences through appropriate output events,and thus solve shallow problems involving long time lags between relevant events.At which problem depth does Shallow Learning end,and Deep Learning begin?Discussions with DL experts have not yet yielded a conclusive response to this question.Instead of committing myself to a precise answer,let me just define for the purposes of this overview:problems of depth>10require Very Deep Learning.The difficulty of a problem may have little to do with its depth.Some NNs can quickly learn to solve certain deep problems,e.g.,through random weight guessing(Sec.5.9)or other types of direct search (Sec.6.6)or indirect search(Sec.6.7)in weight space,or through training an NNfirst on shallow problems whose solutions may then generalize to deep problems,or through collapsing sequences of(non)linear operations into a single(non)linear operation—but see an analysis of non-trivial aspects of deep linear networks(Baldi and Hornik,1994,Section B).In general,however,finding an NN that precisely models a given training set is an NP-complete problem(Judd,1990;Blum and Rivest,1992),also in the case of deep NNs(S´ıma,1994;de Souto et al.,1999;Windisch,2005);compare a survey of negative results(S´ıma, 2002,Section1).Above we have focused on SL.In the more general case of RL in unknown environments,pcc(p,q) is also true if x p is an output event and x q any later input event—any action may affect the environment and thus any later perception.(In the real world,the environment may even influence non-input events computed on a physical hardware entangled with the entire universe,but this is ignored here.)It is possible to model and replace such unmodifiable environmental PCCs through a part of the NN that has already learned to predict(through some of its units)input events(including reward signals)from former input events and actions(Sec.6.1).Its weights are frozen,but can help to assign credit to other,still modifiable weights used to compute actions(Sec.6.1).This approach may lead to very deep CAPs though.Some DL research is about automatically rephrasing problems such that their depth is reduced(Sec.4). In particular,sometimes UL is used to make SL problems less deep,e.g.,Sec.5.10.Often Dynamic Programming(Sec.4.1)is used to facilitate certain traditional RL problems,e.g.,Sec.6.2.Sec.5focuses on CAPs for SL,Sec.6on the more complex case of RL.4Recurring Themes of Deep Learning4.1Dynamic Programming(DP)for DLOne recurring theme of DL is Dynamic Programming(DP)(Bellman,1957),which can help to facili-tate credit assignment under certain assumptions.For example,in SL NNs,backpropagation itself can 1An alternative would be to count only modifiable links when measuring depth.In many typical NN applications this would not make a difference,but in some it would,e.g.,Sec.6.1.be viewed as a DP-derived method(Sec.5.5).In traditional RL based on strong Markovian assumptions, DP-derived methods can help to greatly reduce problem depth(Sec.6.2).DP algorithms are also essen-tial for systems that combine concepts of NNs and graphical models,such as Hidden Markov Models (HMMs)(Stratonovich,1960;Baum and Petrie,1966)and Expectation Maximization(EM)(Dempster et al.,1977),e.g.,(Bottou,1991;Bengio,1991;Bourlard and Morgan,1994;Baldi and Chauvin,1996; Jordan and Sejnowski,2001;Bishop,2006;Poon and Domingos,2011;Dahl et al.,2012;Hinton et al., 2012a).4.2Unsupervised Learning(UL)Facilitating Supervised Learning(SL)and RL Another recurring theme is how UL can facilitate both SL(Sec.5)and RL(Sec.6).UL(Sec.5.6.4) is normally used to encode raw incoming data such as video or speech streams in a form that is more convenient for subsequent goal-directed learning.In particular,codes that describe the original data in a less redundant or more compact way can be fed into SL(Sec.5.10,5.15)or RL machines(Sec.6.4),whose search spaces may thus become smaller(and whose CAPs shallower)than those necessary for dealing with the raw data.UL is closely connected to the topics of regularization and compression(Sec.4.3,5.6.3). 4.3Occam’s Razor:Compression and Minimum Description Length(MDL) Occam’s razor favors simple solutions over complex ones.Given some programming language,the prin-ciple of Minimum Description Length(MDL)can be used to measure the complexity of a solution candi-date by the length of the shortest program that computes it(e.g.,Solomonoff,1964;Kolmogorov,1965b; Chaitin,1966;Wallace and Boulton,1968;Levin,1973a;Rissanen,1986;Blumer et al.,1987;Li and Vit´a nyi,1997;Gr¨u nwald et al.,2005).Some methods explicitly take into account program runtime(Al-lender,1992;Watanabe,1992;Schmidhuber,2002,1995);many consider only programs with constant runtime,written in non-universal programming languages(e.g.,Rissanen,1986;Hinton and van Camp, 1993).In the NN case,the MDL principle suggests that low NN weight complexity corresponds to high NN probability in the Bayesian view(e.g.,MacKay,1992;Buntine and Weigend,1991;De Freitas,2003), and to high generalization performance(e.g.,Baum and Haussler,1989),without overfitting the training data.Many methods have been proposed for regularizing NNs,that is,searching for solution-computing, low-complexity SL NNs(Sec.5.6.3)and RL NNs(Sec.6.7).This is closely related to certain UL methods (Sec.4.2,5.6.4).4.4Learning Hierarchical Representations Through Deep SL,UL,RLMany methods of Good Old-Fashioned Artificial Intelligence(GOFAI)(Nilsson,1980)as well as more recent approaches to AI(Russell et al.,1995)and Machine Learning(Mitchell,1997)learn hierarchies of more and more abstract data representations.For example,certain methods of syntactic pattern recog-nition(Fu,1977)such as grammar induction discover hierarchies of formal rules to model observations. The partially(un)supervised Automated Mathematician/EURISKO(Lenat,1983;Lenat and Brown,1984) continually learns concepts by combining previously learnt concepts.Such hierarchical representation learning(Ring,1994;Bengio et al.,2013;Deng and Yu,2014)is also a recurring theme of DL NNs for SL (Sec.5),UL-aided SL(Sec.5.7,5.10,5.15),and hierarchical RL(Sec.6.5).Often,abstract hierarchical representations are natural by-products of data compression(Sec.4.3),e.g.,Sec.5.10.4.5Fast Graphics Processing Units(GPUs)for DL in NNsWhile the previous millennium saw several attempts at creating fast NN-specific hardware(e.g.,Jackel et al.,1990;Faggin,1992;Ramacher et al.,1993;Widrow et al.,1994;Heemskerk,1995;Korkin et al., 1997;Urlbe,1999),and at exploiting standard hardware(e.g.,Anguita et al.,1994;Muller et al.,1995; Anguita and Gomes,1996),the new millennium brought a DL breakthrough in form of cheap,multi-processor graphics cards or GPUs.GPUs are widely used for video games,a huge and competitive market that has driven down hardware prices.GPUs excel at fast matrix and vector multiplications required not only for convincing virtual realities but also for NN training,where they can speed up learning by a factorof50and more.Some of the GPU-based FNN implementations(Sec.5.16-5.19)have greatly contributed to recent successes in contests for pattern recognition(Sec.5.19-5.22),image segmentation(Sec.5.21), and object detection(Sec.5.21-5.22).5Supervised NNs,Some Helped by Unsupervised NNsThe main focus of current practical applications is on Supervised Learning(SL),which has dominated re-cent pattern recognition contests(Sec.5.17-5.22).Several methods,however,use additional Unsupervised Learning(UL)to facilitate SL(Sec.5.7,5.10,5.15).It does make sense to treat SL and UL in the same section:often gradient-based methods,such as BP(Sec.5.5.1),are used to optimize objective functions of both UL and SL,and the boundary between SL and UL may blur,for example,when it comes to time series prediction and sequence classification,e.g.,Sec.5.10,5.12.A historical timeline format will help to arrange subsections on important inspirations and techni-cal contributions(although such a subsection may span a time interval of many years).Sec.5.1briefly mentions early,shallow NN models since the1940s,Sec.5.2additional early neurobiological inspiration relevant for modern Deep Learning(DL).Sec.5.3is about GMDH networks(since1965),perhaps thefirst (feedforward)DL systems.Sec.5.4is about the relatively deep Neocognitron NN(1979)which is similar to certain modern deep FNN architectures,as it combines convolutional NNs(CNNs),weight pattern repli-cation,and winner-take-all(WTA)mechanisms.Sec.5.5uses the notation of Sec.2to compactly describe a central algorithm of DL,namely,backpropagation(BP)for supervised weight-sharing FNNs and RNNs. It also summarizes the history of BP1960-1981and beyond.Sec.5.6describes problems encountered in the late1980s with BP for deep NNs,and mentions several ideas from the previous millennium to overcome them.Sec.5.7discusses afirst hierarchical stack of coupled UL-based Autoencoders(AEs)—this concept resurfaced in the new millennium(Sec.5.15).Sec.5.8is about applying BP to CNNs,which is important for today’s DL applications.Sec.5.9explains BP’s Fundamental DL Problem(of vanishing/exploding gradients)discovered in1991.Sec.5.10explains how a deep RNN stack of1991(the History Compressor) pre-trained by UL helped to solve previously unlearnable DL benchmarks requiring Credit Assignment Paths(CAPs,Sec.3)of depth1000and more.Sec.5.11discusses a particular WTA method called Max-Pooling(MP)important in today’s DL FNNs.Sec.5.12mentions afirst important contest won by SL NNs in1994.Sec.5.13describes a purely supervised DL RNN(Long Short-Term Memory,LSTM)for problems of depth1000and more.Sec.5.14mentions an early contest of2003won by an ensemble of shallow NNs, as well as good pattern recognition results with CNNs and LSTM RNNs(2003).Sec.5.15is mostly about Deep Belief Networks(DBNs,2006)and related stacks of Autoencoders(AEs,Sec.5.7)pre-trained by UL to facilitate BP-based SL.Sec.5.16mentions thefirst BP-trained MPCNNs(2007)and GPU-CNNs(2006). Sec.5.17-5.22focus on official competitions with secret test sets won by(mostly purely supervised)DL NNs since2009,in sequence recognition,image classification,image segmentation,and object detection. Many RNN results depended on LSTM(Sec.5.13);many FNN results depended on GPU-based FNN code developed since2004(Sec.5.16,5.17,5.18,5.19),in particular,GPU-MPCNNs(Sec.5.19).5.11940s and EarlierNN research started in the1940s(e.g.,McCulloch and Pitts,1943;Hebb,1949);compare also later work on learning NNs(Rosenblatt,1958,1962;Widrow and Hoff,1962;Grossberg,1969;Kohonen,1972; von der Malsburg,1973;Narendra and Thathatchar,1974;Willshaw and von der Malsburg,1976;Palm, 1980;Hopfield,1982).In a sense NNs have been around even longer,since early supervised NNs were essentially variants of linear regression methods going back at least to the early1800s(e.g.,Legendre, 1805;Gauss,1809,1821).Early NNs had a maximal CAP depth of1(Sec.3).5.2Around1960:More Neurobiological Inspiration for DLSimple cells and complex cells were found in the cat’s visual cortex(e.g.,Hubel and Wiesel,1962;Wiesel and Hubel,1959).These cellsfire in response to certain properties of visual sensory inputs,such as theorientation of plex cells exhibit more spatial invariance than simple cells.This inspired later deep NN architectures(Sec.5.4)used in certain modern award-winning Deep Learners(Sec.5.19-5.22).5.31965:Deep Networks Based on the Group Method of Data Handling(GMDH) Networks trained by the Group Method of Data Handling(GMDH)(Ivakhnenko and Lapa,1965; Ivakhnenko et al.,1967;Ivakhnenko,1968,1971)were perhaps thefirst DL systems of the Feedforward Multilayer Perceptron type.The units of GMDH nets may have polynomial activation functions imple-menting Kolmogorov-Gabor polynomials(more general than traditional NN activation functions).Given a training set,layers are incrementally grown and trained by regression analysis,then pruned with the help of a separate validation set(using today’s terminology),where Decision Regularisation is used to weed out superfluous units.The numbers of layers and units per layer can be learned in problem-dependent fashion. This is a good example of hierarchical representation learning(Sec.4.4).There have been numerous ap-plications of GMDH-style networks,e.g.(Ikeda et al.,1976;Farlow,1984;Madala and Ivakhnenko,1994; Ivakhnenko,1995;Kondo,1998;Kord´ık et al.,2003;Witczak et al.,2006;Kondo and Ueno,2008).5.41979:Convolution+Weight Replication+Winner-Take-All(WTA)Apart from deep GMDH networks(Sec.5.3),the Neocognitron(Fukushima,1979,1980,2013a)was per-haps thefirst artificial NN that deserved the attribute deep,and thefirst to incorporate the neurophysiolog-ical insights of Sec.5.2.It introduced convolutional NNs(today often called CNNs or convnets),where the(typically rectangular)receptivefield of a convolutional unit with given weight vector is shifted step by step across a2-dimensional array of input values,such as the pixels of an image.The resulting2D array of subsequent activation events of this unit can then provide inputs to higher-level units,and so on.Due to massive weight replication(Sec.2),relatively few parameters may be necessary to describe the behavior of such a convolutional layer.Competition layers have WTA subsets whose maximally active units are the only ones to adopt non-zero activation values.They essentially“down-sample”the competition layer’s input.This helps to create units whose responses are insensitive to small image shifts(compare Sec.5.2).The Neocognitron is very similar to the architecture of modern,contest-winning,purely super-vised,feedforward,gradient-based Deep Learners with alternating convolutional and competition lay-ers(e.g.,Sec.5.19-5.22).Fukushima,however,did not set the weights by supervised backpropagation (Sec.5.5,5.8),but by local un supervised learning rules(e.g.,Fukushima,2013b),or by pre-wiring.In that sense he did not care for the DL problem(Sec.5.9),although his architecture was comparatively deep indeed.He also used Spatial Averaging(Fukushima,1980,2011)instead of Max-Pooling(MP,Sec.5.11), currently a particularly convenient and popular WTA mechanism.Today’s CNN-based DL machines profita lot from later CNN work(e.g.,LeCun et al.,1989;Ranzato et al.,2007)(Sec.5.8,5.16,5.19).5.51960-1981and Beyond:Development of Backpropagation(BP)for NNsThe minimisation of errors through gradient descent(Hadamard,1908)in the parameter space of com-plex,nonlinear,differentiable,multi-stage,NN-related systems has been discussed at least since the early 1960s(e.g.,Kelley,1960;Bryson,1961;Bryson and Denham,1961;Pontryagin et al.,1961;Dreyfus,1962; Wilkinson,1965;Amari,1967;Bryson and Ho,1969;Director and Rohrer,1969;Griewank,2012),ini-tially within the framework of Euler-LaGrange equations in the Calculus of Variations(e.g.,Euler,1744). Steepest descent in such systems can be performed(Bryson,1961;Kelley,1960;Bryson and Ho,1969)by iterating the ancient chain rule(Leibniz,1676;L’Hˆo pital,1696)in Dynamic Programming(DP)style(Bell-man,1957).A simplified derivation of the method uses the chain rule only(Dreyfus,1962).The methods of the1960s were already efficient in the DP sense.However,they backpropagated derivative information through standard Jacobian matrix calculations from one“layer”to the previous one, explicitly addressing neither direct links across several layers nor potential additional efficiency gains due to network sparsity(but perhaps such enhancements seemed obvious to the authors).。
Principles of Plasma Discharges and Materials Processing9

CHAPTER8MOLECULAR COLLISIONS8.1INTRODUCTIONBasic concepts of gas-phase collisions were introduced in Chapter3,where we described only those processes needed to model the simplest noble gas discharges: electron–atom ionization,excitation,and elastic scattering;and ion–atom elastic scattering and resonant charge transfer.In this chapter we introduce other collisional processes that are central to the description of chemically reactive discharges.These include the dissociation of molecules,the generation and destruction of negative ions,and gas-phase chemical reactions.Whereas the cross sections have been measured reasonably well for the noble gases,with measurements in reasonable agreement with theory,this is not the case for collisions in molecular gases.Hundreds of potentially significant collisional reactions must be examined in simple diatomic gas discharges such as oxygen.For feedstocks such as CF4/O2,SiH4/O2,etc.,the complexity can be overwhelming.Furthermore,even when the significant processes have been identified,most of the cross sections have been neither measured nor calculated. Hence,one must often rely on estimates based on semiempirical or semiclassical methods,or on measurements made on molecules analogous to those of interest. As might be expected,data are most readily available for simple diatomic and polyatomic gases.Principles of Plasma Discharges and Materials Processing,by M.A.Lieberman and A.J.Lichtenberg. ISBN0-471-72001-1Copyright#2005John Wiley&Sons,Inc.235236MOLECULAR COLLISIONS8.2MOLECULAR STRUCTUREThe energy levels for the electronic states of a single atom were described in Chapter3.The energy levels of molecules are more complicated for two reasons. First,molecules have additional vibrational and rotational degrees of freedom due to the motions of their nuclei,with corresponding quantized energies E v and E J. Second,the energy E e of each electronic state depends on the instantaneous con-figuration of the nuclei.For a diatomic molecule,E e depends on a single coordinate R,the spacing between the two nuclei.Since the nuclear motions are slow compared to the electronic motions,the electronic state can be determined for anyfixed spacing.We can therefore represent each quantized electronic level for a frozen set of nuclear positions as a graph of E e versus R,as shown in Figure8.1.For a mole-cule to be stable,the ground(minimum energy)electronic state must have a minimum at some value R1corresponding to the mean intermolecular separation (curve1).In this case,energy must be supplied in order to separate the atoms (R!1).An excited electronic state can either have a minimum( R2for curve2) or not(curve3).Note that R2and R1do not generally coincide.As for atoms, excited states may be short lived(unstable to electric dipole radiation)or may be metastable.Various electronic levels may tend to the same energy in the unbound (R!1)limit. Array FIGURE8.1.Potential energy curves for the electronic states of a diatomic molecule.For diatomic molecules,the electronic states are specifiedfirst by the component (in units of hÀ)L of the total orbital angular momentum along the internuclear axis, with the symbols S,P,D,and F corresponding to L¼0,+1,+2,and+3,in analogy with atomic nomenclature.All but the S states are doubly degenerate in L.For S states,þandÀsuperscripts are often used to denote whether the wave function is symmetric or antisymmetric with respect to reflection at any plane through the internuclear axis.The total electron spin angular momentum S (in units of hÀ)is also specified,with the multiplicity2Sþ1written as a prefixed superscript,as for atomic states.Finally,for homonuclear molecules(H2,N2,O2, etc.)the subscripts g or u are written to denote whether the wave function is sym-metric or antisymmetric with respect to interchange of the nuclei.In this notation, the ground states of H2and N2are both singlets,1Sþg,and that of O2is a triplet,3SÀg .For polyatomic molecules,the electronic energy levels depend on more thanone nuclear coordinate,so Figure8.1must be generalized.Furthermore,since there is generally no axis of symmetry,the states cannot be characterized by the quantum number L,and other naming conventions are used.Such states are often specified empirically through characterization of measured optical emission spectra.Typical spacings of low-lying electronic energy levels range from a few to tens of volts,as for atoms.Vibrational and Rotational MotionsUnfreezing the nuclear vibrational and rotational motions leads to additional quan-tized structure on smaller energy scales,as illustrated in Figure8.2.The simplest (harmonic oscillator)model for the vibration of diatomic molecules leads to equally spaced quantized,nondegenerate energy levelse E v¼hÀv vib vþ1 2(8:2:1)where v¼0,1,2,...is the vibrational quantum number and v vib is the linearized vibration frequency.Fitting a quadratic functione E v¼12k vib(RÀ R)2(8:2:2)near the minimum of a stable energy level curve such as those shown in Figure8.1, we can estimatev vib%k vibm Rmol1=2(8:2:3)where k vib is the“spring constant”and m Rmol is the reduced mass of the AB molecule.The spacing hÀv vib between vibrational energy levels for a low-lying8.2MOLECULAR STRUCTURE237stable electronic state is typically a few tenths of a volt.Hence for molecules in equi-librium at room temperature (0.026V),only the v ¼0level is significantly popula-ted.However,collisional processes can excite strongly nonequilibrium vibrational energy levels.We indicate by the short horizontal line segments in Figure 8.1a few of the vibrational energy levels for the stable electronic states.The length of each segment gives the range of classically allowed vibrational motions.Note that even the ground state (v ¼0)has a finite width D R 1as shown,because from(8.2.1),the v ¼0state has a nonzero vibrational energy 1h Àv vib .The actual separ-ation D R about Rfor the ground state has a Gaussian distribution,and tends toward a distribution peaked at the classical turning points for the vibrational motion as v !1.The vibrational motion becomes anharmonic and the level spa-cings tend to zero as the unbound vibrational energy is approached (E v !D E 1).FIGURE 8.2.Vibrational and rotational levels of two electronic states A and B of a molecule;the three double arrows indicate examples of transitions in the pure rotation spectrum,the rotation–vibration spectrum,and the electronic spectrum (after Herzberg,1971).238MOLECULAR COLLISIONSFor E v.D E1,the vibrational states form a continuum,corresponding to unbound classical motion of the nuclei(breakup of the molecule).For a polyatomic molecule there are many degrees of freedom for vibrational motion,leading to a very compli-cated structure for the vibrational levels.The simplest(dumbbell)model for the rotation of diatomic molecules leads to the nonuniform quantized energy levelse E J¼hÀ22I molJ(Jþ1)(8:2:4)where I mol¼m Rmol R2is the moment of inertia and J¼0,1,2,...is the rotational quantum number.The levels are degenerate,with2Jþ1states for the J th level. The spacing between rotational levels increases with J(see Figure8.2).The spacing between the lowest(J¼0to J¼1)levels typically corresponds to an energy of0.001–0.01V;hence,many low-lying levels are populated in thermal equilibrium at room temperature.Optical EmissionAn excited molecular state can decay to a lower energy state by emission of a photon or by breakup of the molecule.As shown in Figure8.2,the radiation can be emitted by a transition between electronic levels,between vibrational levels of the same electronic state,or between rotational levels of the same electronic and vibrational state;the radiation typically lies within the optical,infrared,or microwave frequency range,respectively.Electric dipole radiation is the strongest mechanism for photon emission,having typical transition times of t rad 10À9s,as obtained in (3.4.13).The selection rules for electric dipole radiation areDL¼0,+1(8:2:5a)D S¼0(8:2:5b) In addition,for transitions between S states the only allowed transitions areSþÀ!Sþand SÀÀ!SÀ(8:2:6) and for homonuclear molecules,the only allowed transitions aregÀ!u and uÀ!g(8:2:7) Hence homonuclear diatomic molecules do not have a pure vibrational or rotational spectrum.Radiative transitions between electronic levels having many different vibrational and rotational initial andfinal states give rise to a structure of emission and absorption bands within which a set of closely spaced frequencies appear.These give rise to characteristic molecular emission and absorption bands when observed8.2MOLECULAR STRUCTURE239using low-resolution optical spectrometers.As for atoms,metastable molecular states having no electric dipole transitions to lower levels also exist.These have life-times much exceeding10À6s;they can give rise to weak optical band structures due to magnetic dipole or electric quadrupole radiation.Electric dipole radiation between vibrational levels of the same electronic state is permitted for molecules having permanent dipole moments.In the harmonic oscillator approximation,the selection rule is D v¼+1;weaker transitions D v¼+2,+3,...are permitted for anharmonic vibrational motion.The preceding description of molecular structure applies to molecules having arbi-trary electronic charge.This includes neutral molecules AB,positive molecular ions ABþ,AB2þ,etc.and negative molecular ions ABÀ.The potential energy curves for the various electronic states,regardless of molecular charge,are commonly plotted on the same diagram.Figures8.3and8.4give these for some important electronic statesof HÀ2,H2,and Hþ2,and of OÀ2,O2,and Oþ2,respectively.Examples of both attractive(having a potential energy minimum)and repulsive(having no minimum)states can be seen.The vibrational levels are labeled with the quantum number v for the attrac-tive levels.The ground states of both Hþ2and Oþ2are attractive;hence these molecular ions are stable against autodissociation(ABþ!AþBþor AþþB).Similarly,the ground states of H2and O2are attractive and lie below those of Hþ2and Oþ2;hence they are stable against autodissociation and autoionization(AB!ABþþe).For some molecules,for example,diatomic argon,the ABþion is stable but the AB neutral is not stable.For all molecules,the AB ground state lies below the ABþground state and is stable against autoionization.Excited states can be attractive or repulsive.A few of the attractive states may be metastable;some examples are the 3P u state of H2and the1D g,1Sþgand3D u states of O2.Negative IonsRecall from Section7.2that many neutral atoms have a positive electron affinity E aff;that is,the reactionAþeÀ!AÀis exothermic with energy E aff(in volts).If E aff is negative,then AÀis unstable to autodetachment,AÀ!Aþe.A similar phenomenon is found for negative molecular ions.A stable ABÀion exists if its ground(lowest energy)state has a potential minimum that lies below the ground state of AB.This is generally true only for strongly electronegative gases having large electron affinities,such as O2 (E aff%1:463V for O atoms)and the halogens(E aff.3V for the atoms).For example,Figure8.4shows that the2P g ground state of OÀ2is stable,with E aff% 0:43V for O2.For weakly electronegative or for electropositive gases,the minimum of the ground state of ABÀgenerally lies above the ground state of AB,and ABÀis unstable to autodetachment.An example is hydrogen,which is weakly electronegative(E aff%0:754V for H atoms).Figure8.3shows that the2Sþu ground state of HÀ2is unstable,although the HÀion itself is stable.In an elec-tropositive gas such as N2(E aff.0),both NÀ2and NÀare unstable. 240MOLECULAR COLLISIONS8.3ELECTRON COLLISIONS WITH MOLECULESThe interaction time for the collision of a typical (1–10V)electron with a molecule is short,t c 2a 0=v e 10À16–10À15s,compared to the typical time for a molecule to vibrate,t vib 10À14–10À13s.Hence for electron collisional excitation of a mole-cule to an excited electronic state,the new vibrational (and rotational)state canbeFIGURE 8.3.Potential energy curves for H À2,H 2,and H þ2.(From Jeffery I.Steinfeld,Molecules and Radiation:An Introduction to Modern Molecular Spectroscopy ,2d ed.#MIT Press,1985.)8.3ELECTRON COLLISIONS WITH MOLECULES 241FIGURE 8.4.Potential energy curves for O À2,O 2,and O þ2.(From Jeffery I.Steinfeld,Molecules and Radiation:An Introduction to Modern Molecular Spectroscopy ,2d ed.#MIT Press,1985.)242MOLECULAR COLLISIONS8.3ELECTRON COLLISIONS WITH MOLECULES243 determined by freezing the nuclear motions during the collision.This is known as the Franck–Condon principle and is illustrated in Figure8.1by the vertical line a,showing the collisional excitation atfixed R to a high quantum number bound vibrational state and by the vertical line b,showing excitation atfixed R to a vibra-tionally unbound state,in which breakup of the molecule is energetically permitted. Since the typical transition time for electric dipole radiation(t rad 10À9–10À8s)is long compared to the dissociation( vibrational)time t diss,excitation to an excited state will generally lead to dissociation when it is energetically permitted.Finally, we note that the time between collisions t c)t rad in typical low-pressure processing discharges.Summarizing the ordering of timescales for electron–molecule collisions,we havet at t c(t vib t diss(t rad(t cDissociationElectron impact dissociation,eþABÀ!AþBþeof feedstock gases plays a central role in the chemistry of low-pressure reactive discharges.The variety of possible dissociation processes is illustrated in Figure8.5.In collisions a or a0,the v¼0ground state of AB is excited to a repulsive state of AB.The required threshold energy E thr is E a for collision a and E a0for Array FIGURE8.5.Illustrating the variety of dissociation processes for electron collisions with molecules.collision a0,and it leads to an energy after dissociation lying between E aÀE diss and E a0ÀE diss that is shared among the dissociation products(here,A and B). Typically,E aÀE diss few volts;consequently,hot neutral fragments are typically generated by dissociation processes.If these hot fragments hit the substrate surface, they can profoundly affect the process chemistry.In collision b,the ground state AB is excited to an attractive state of AB at an energy E b that exceeds the binding energy E diss of the AB molecule,resulting in dissociation of AB with frag-ment energy E bÀE diss.In collision b0,the excitation energy E b0¼E diss,and the fragments have low energies;hence this process creates fragments having energies ranging from essentially thermal energies up to E bÀE diss few volts.In collision c,the AB atom is excited to the bound excited state ABÃ(labeled5),which sub-sequently radiates to the unbound AB state(labeled3),which then dissociates.The threshold energy required is large,and the fragments are hot.Collision c can also lead to dissociation of an excited state by a radiationless transfer from state5to state4near the point where the two states cross:ABÃðboundÞÀ!ABÃðunboundÞÀ!AþBÃThe fragments can be both hot and in excited states.We discuss such radiationless electronic transitions in the next section.This phenomenon is known as predisso-ciation.Finally,a collision(not labeled in thefigure)to state4can lead to dis-sociation of ABÃ,again resulting in hot excited fragments.The process of electron impact excitation of a molecule is similar to that of an atom,and,consequently,the cross sections have a similar form.A simple classical estimate of the dissociation cross section for a level having excitation energy U1can be found by requiring that an incident electron having energy W transfer an energy W L lying between U1and U2to a valence electron.Here,U2is the energy of the next higher level.Then integrating the differential cross section d s[given in(3.4.20)and repeated here],d s¼pe24021Wd W LW2L(3:4:20)over W L,we obtains diss¼0W,U1pe24pe021W1U1À1WU1,W,U2pe24021W1U1À1U2W.U28>>>>>><>>>>>>:(8:3:1)244MOLECULAR COLLISIONSLetting U2ÀU1(U1and introducing voltage units W¼e E,U1¼e E1and U2¼e E2,we haves diss¼0E,E1s0EÀE11E1,E,E2s0E2ÀE1EE.E28>>>><>>>>:(8:3:2)wheres0¼pe4pe0E12(8:3:3)We see that the dissociation cross section rises linearly from the threshold energy E thr%E1to a maximum value s0(E2ÀE1)=E thr at E2and then falls off as1=E. Actually,E1and E2can depend on the nuclear separation R.In this case,(8.3.2) should be averaged over the range of R s corresponding to the ground-state vibrational energy,leading to a broadened dependence of the average cross section on energy E.The maximum cross section is typically of order10À15cm2. Typical rate constants for a single dissociation process with E thr&T e have an Arrhenius formK diss/K diss0expÀE thr T e(8:3:4)where K diss0 10À7cm3=s.However,in some cases E thr.T e.For excitation to an attractive state,an appropriate average over the fraction of the ground-state vibration that leads to dissociation must be taken.Dissociative IonizationIn addition to normal ionization,eþABÀ!ABþþ2eelectron–molecule collisions can lead to dissociative ionizationeþABÀ!AþBþþ2eThese processes,common for polyatomic molecules,are illustrated in Figure8.6.In collision a having threshold energy E iz,the molecular ion ABþis formed.Collisionsb andc occur at higher threshold energies E diz and result in dissociative ionization,8.3ELECTRON COLLISIONS WITH MOLECULES245leading to the formation of fast,positively charged ions and neutrals.These cross sections have a similar form to the Thompson ionization cross section for atoms.Dissociative RecombinationThe electron collision,e þAB þÀ!A þB Ãillustrated as d and d 0in Figure 8.6,destroys an electron–ion pair and leads to the production of fast excited neutral fragments.Since the electron is captured,it is not available to carry away a part of the reaction energy.Consequently,the collision cross section has a resonant character,falling to very low values for E ,E d and E .E d 0.However,a large number of excited states A Ãand B Ãhaving increasing principal quantum numbers n and energies can be among the reaction products.Consequently,the rate constants can be large,of order 10À7–10À6cm 3=s.Dissocia-tive recombination to the ground states of A and B cannot occur because the potential energy curve for AB þis always greater than the potential energycurveFIGURE 8.6.Illustration of dissociative ionization and dissociative recombination for electron collisions with molecules.246MOLECULAR COLLISIONSfor the repulsive state of AB.Two-body recombination for atomic ions or for mol-ecular ions that do not subsequently dissociate can only occur with emission of a photon:eþAþÀ!Aþh n:As shown in Section9.2,the rate constants are typically three tofive orders of magnitude lower than for dissociative recombination.Example of HydrogenThe example of H2illustrates some of the inelastic electron collision phenomena we have discussed.In order of increasing electron impact energy,at a threshold energy of 8:8V,there is excitation to the repulsive3Sþu state followed by dissociation into two fast H fragments carrying 2:2V/atom.At11.5V,the1Sþu bound state is excited,with subsequent electric dipole radiation in the ultraviolet region to the1Sþg ground state.At11.8V,there is excitation to the3Sþg bound state,followedby electric dipole radiation to the3Sþu repulsive state,followed by dissociation with 2:2V/atom.At12.6V,the1P u bound state is excited,with UV emission tothe ground state.At15.4V,the2Sþg ground state of Hþ2is excited,leading to the pro-duction of Hþ2ions.At28V,excitation of the repulsive2Sþu state of Hþ2leads to thedissociative ionization of H2,with 5V each for the H and Hþfragments.Dissociative Electron AttachmentThe processes,eþABÀ!AþBÀproduce negative ion fragments as well as neutrals.They are important in discharges containing atoms having positive electron affinities,not only because of the pro-duction of negative ions,but because the threshold energy for production of negative ion fragments is usually lower than for pure dissociation processes.A variety of pro-cesses are possible,as shown in Figure8.7.Since the impacting electron is captured and is not available to carry excess collision energy away,dissociative attachment is a resonant process that is important only within a narrow energy range.The maximum cross sections are generally much smaller than the hard-sphere cross section of the molecule.Attachment generally proceeds by collisional excitation from the ground AB state to a repulsive ABÀstate,which subsequently either auto-detaches or dissociates.The attachment cross section is determined by the balance between these processes.For most molecules,the dissociation energy E diss of AB is greater than the electron affinity E affB of B,leading to the potential energy curves shown in Figure8.7a.In this case,the cross section is large only for impact energies lying between a minimum value E thr,for collision a,and a maximum value E0thr for8.3ELECTRON COLLISIONS WITH MOLECULES247FIGURE 8.7.Illustration of a variety of electron attachment processes for electron collisions with molecules:(a )capture into a repulsive state;(b )capture into an attractive state;(c )capture of slow electrons into a repulsive state;(d )polar dissociation.248MOLECULAR COLLISIONScollision a 0.The fragments are hot,having energies lying between minimum and maximum values E min ¼E thr þE affB ÀE diss and E max ¼E 0thr þE af fB ÀE diss .Since the AB Àstate lies above the AB state for R ,R x ,autodetachment can occur as the mol-ecules begin to separate:AB À!AB þe.Hence the cross section for production of negative ions can be much smaller than that for excitation of the AB Àrepulsive state.As a crude estimate,for the same energy,the autodetachment rate is ffiffiffiffiffiffiffiffiffiffiffiffiffiM R =m p 100times the dissociation rate of the repulsive AB Àmolecule,where M R is the reduced mass.Hence only one out of 100excitations lead to dissociative attachment.Excitation to the AB Àbound state can also lead to dissociative attachment,as shown in Figure 8.7b .Here the cross section is significant only for E thr ,E ,E 0thr ,but the fragments can have low energies,with a minimum energy of zero and a maximum energy of E 0thr þE affB ÀE diss .Collision b,e þAB À!AB ÀÃdoes not lead to production of AB Àions because energy and momentum are not gen-erally conserved when two bodies collide elastically to form one body (see Problem3.12).Hence the excited AB ÀÃion separates,AB ÀÃÀ!e þABunless vibrational radiation or collision with a third body carries off the excess energy.These processes are both slow in low-pressure discharges (see Section 9.2).At high pressures (say,atmospheric),three-body attachment to form AB Àcan be very important.For a few molecules,such as some halogens,the electron affinity of the atom exceeds the dissociation energy of the neutral molecule,leading to the potential energy curves shown in Figure 8.7c .In this case the range of electron impact ener-gies E for excitation of the AB Àrepulsive state includes E ¼0.Consequently,there is no threshold energy,and very slow electrons can produce dissociative attachment,resulting in hot neutral and negative ion fragments.The range of R s over which auto-detachment can occur is small;hence the maximum cross sections for dissociative attachment can be as high as 10À16cm 2.A simple classical estimate of electron capture can be made using the differential scattering cross section for energy loss (3.4.20),in a manner similar to that done for dissociation.For electron capture to an energy level E 1that is unstable to autode-tachment,and with the additional constraint for capture that the incident electron energy lie within E 1and E 2¼E 1þD E ,where D E is a small energy difference characteristic of the dissociative attachment timescale,we obtain,in place of (8.3.2),s att¼0E ,E 1s 0E ÀE 1E 1E 1,E ,E 20E .E 28>><>>:(8:3:5)8.3ELECTRON COLLISIONS WITH MOLECULES 249wheres 0%p m M R 1=2e 4pe 0E 1 2(8:3:6)The factor of (m =M R )1=2roughly gives the fraction of excited states that do not auto-detach.We see that the dissociative attachment cross section rises linearly at E 1to a maximum value s 0D E =E 1and then falls abruptly to zero.As for dissociation,E 1can depend strongly on the nuclear separation R ,and (8.3.5)must be averaged over the range of E 1s corresponding to the ground state vibrational motion;e.g.,from E thr to E 0thr in Figure 8.7a .Because generally D E (E 0thr ÀE thr ,we can write (8.3.5)in the forms att %p m M R 1=2e 4pe 0 2(D E )22E 1d (E ÀE 1)(8:3:7)where d is the Dirac delta ing (8.3.7),the average over the vibrational motion can be performed,leading to a cross section that is strongly peaked lying between E thr and E 0thr .We leave the details of the calculation to a problem.Polar DissociationThe process,e þAB À!A þþB Àþeproduces negative ions without electron capture.As shown in Figure 8.7d ,the process proceeds by excitation of a polar state A þand B Àof AB Ãthat has a separ-ated atom limit of A þand B À.Hence at large R ,this state lies above the A þB ground state by the difference between the ionization potential of A and the electron affinity of B.The polar state is weakly bound at large R by the Coulomb attraction force,but is repulsive at small R .The maximum cross section and the dependence of the cross section on electron impact energy are similar to that of pure dissociation.The threshold energy E thr for polar dissociation is generally large.The measured cross section for negative ion production by electron impact in O 2is shown in Figure 8.8.The sharp peak at 6.5V is due to dissociative attachment.The variation of the cross section with energy is typical of a resonant capture process.The maximum cross section of 10À18cm 2is quite low because autode-tachment from the repulsive O À2state is strong,inhibiting dissociative attachment.The second gradual maximum near 35V is due to polar dissociation;the variation of the cross section with energy is typical of a nonresonant process.250MOLECULAR COLLISIONS。
Tight Bounds on the Capacity of Binary Input random CDMA Systems
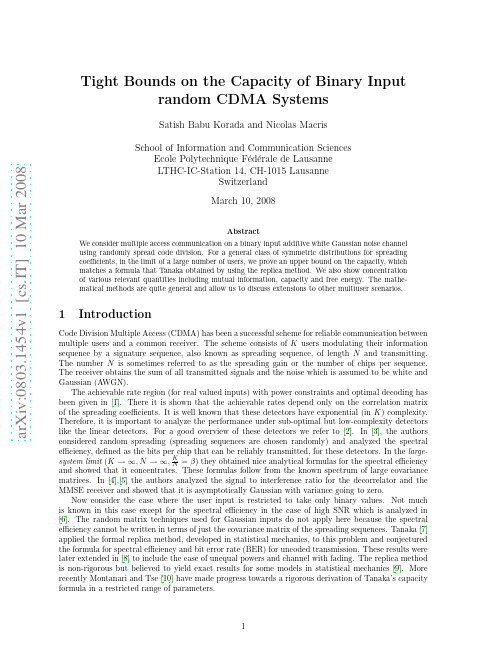
a rXiv:083.1454v1[cs.IT]1Mar28Tight Bounds on the Capacity of Binary Input random CDMA Systems Satish Babu Korada and Nicolas Macris School of Information and Communication Sciences Ecole Polytechnique F´e d´e rale de Lausanne LTHC-IC-Station 14,CH-1015Lausanne Switzerland March 10,2008Abstract We consider multiple access communication on a binary input additive white Gaussian noise channel using randomly spread code division.For a general class of symmetric distributions for spreading coefficients,in the limit of a large number of users,we prove an upper bound on the capacity,which matches a formula that Tanaka obtained by using the replica method.We also show concentration of various relevant quantities including mutual information,capacity and free energy.The mathe-matical methods are quite general and allow us to discuss extensions to other multiuser scenarios.1Introduction Code Division Multiple Access (CDMA)has been a successful scheme for reliable communication between multiple users and a common receiver.The scheme consists of K users modulating their information sequence by a signature sequence,also known as spreading sequence,of length N and transmitting.The number N is sometimes referred to as the spreading gain or the number of chips per sequence.The receiver obtains the sum of all transmitted signals and the noise which is assumed to be white and Gaussian (AWGN).The achievable rate region (for real valued inputs)with power constraints and optimal decoding has been given in [1].There it is shown that the achievable rates depend only on the correlation matrix of the spreading coefficients.It is well known that these detectors have exponential (in K )complexity.Therefore,it is important to analyze the performance under sub-optimal but low-complexity detectors like the linear detectors.For a good overview of these detectors we refer to [2].In [3],the authorsconsidered random spreading (spreading sequences are chosen randomly)and analyzed the spectral efficiency,defined as the bits per chip that can be reliably transmitted,for these detectors.In the large-system limit (K →∞,N →∞,KOur main contributions in this paper are twofold.First we prove that Tanaka’s formula is an upper bound to the capacity for all values of the parameters and second we prove various useful concentration theorems in the large-system limit.1.1Statistical Mechanics ApproachThere is a natural connection between various communication systems and statistical mechanics of random spin systems,stemming from the fact that often in both systems there is a large number of degrees of freedom(bits or spins),interacting locally,in a random environment.So far,there have been applications of two important but somewhat complementary approaches of statistical mechanics of random systems.Thefirst one is the very important but mathematically uncontrolled replica method.The merit of this approach is to obtain conjectural but rather explicit formulas for quantities of interest such as, free energy,conditional entropy or error probability.In some cases the naturalfixed point structure embodied in the meanfield formulas allows to guess good iterative algorithms.This program has been carried out for linear error correcting codes,source coding,multiuser settings like broadcast channel(see for example[11],[12],[13])and the case of interest here[7]:randomly spread CDMA with binary inputs.The second type of approach aims at a rigorous understanding of the replica formulas and has its origins in methods stemming from mathematical physics(see[14,15],[9]).For systems whose underlying degrees of freedom have Gaussian distribution(Gaussian input symbols or Gaussian spins in continuous spin systems)random matrix methods can successfully be employed.However when the degrees of freedom are binary(binary information symbols or Ising spins)these seem to fail,but the recently developed interpolation method[14],[15]has had some success1.The basic idea of the interpolation method is to study a measure which interpolates between the posterior measure of the ideal decoder and a meanfield measure.The later can be guessed from the replica formulas and from this perspective the replica method is a valuable tool.So far this program has been developed only for linear error correcting codes on sparse graphs and binary input symmetric channels[16],[17].In this paper we develop the interpolation method for the random CDMA system with binary inputs (in the large-system limit).The situation is qualitatively different than the ones mentioned above in that the“underlying graph”is complete.Superficially one might think that it is similar to the Sherrington-Kirkpatrick model which was thefirst one treated by the interpolation method.However as we will see the analysis of the randomly spread CDMA system is substantially different due to the structure of the interaction between degrees of freedom.1.2Communication SetupWe consider a scenario where K users send binary information symbols xk =(s1k,...,s Nk)t where the components are independently identically distributed.For each timedivision(or chip)interval i=1,...,N the received signal y√=(n1,...,n N)t are independent identically distributed Gaussian variables N(0,1)so that the noise power isσ2.The variance of s ik is set to1and the scaling factor1/√1Let us point out that,as will be shown later in this paper,the interpolation method can also serve as an alternative to random matrix theory for Gaussian inputs.In particular,our favorite Gaussian and binary cases are included in this class,and also any compactly supported distribution.An inspection of our proofs suggests that the results could be extended to a larger class satisfying:Assumption B.The distribution p(s ik)is symmetric withfinite second and fourth moments. However to keep the proofs as simple as possible only one of the theorems is proven with such generality.In the sequel we use the notations s for the N×K matrix(s ik),S for the corresponding random matrix,and X for the input and output random vectors.Our main interest is in proving a“tight”upper bound onC K=1E S[I(X)](1)in the large-system limit K→+∞with K(x;Yand thus so is its average.Moreover the later is invariant under the transformations p X(ǫ1x1,ǫ2x2,...,ǫK x K)whereǫi=±bining these two facts we deduce that the maximum in(1)is attained for the convex combination1(ǫ1x1,...,ǫK x K)=1KmaxQ Kk=1p k(x k)I(X)(2)where the maximum is over p i(x)=p iδ(x−1)+(1−p i)δ(x+1)and p i∈[0,1],i=1,...,k.In the large-system limit we are able to prove a concentration theorem for the mutual information I(X) which implies that if(p1,...,p K)belongs to afinite discrete set D with cardinality increasing at most polynomially in K,then(2)concentrates on1;Y2as long as1;YThen,by the analysis in[18],formula(1)gives the capacity.If users do not cooperate p X| Y|s[H(X)]is the average over Y|y(xZ(y2σ2 y2s x,s)= x(x2σ2 y2s xis carried out with the distribution induced by the channel transition probabilityp(y0p X(x2σ2y2s x(√(√,s)(5)where in the sum x.In view of this it is not surprising that the free energyf(yKln Z(yK I(X)=−1|s[f(y2β−min p X,S[f(yis attained for p X)=12(1+m)−1λz+λ))(10)withλ=12 2πdz,has to be maximized over a parameter2m.It iseasy3to see that the maximizer must satisfy thefixed point conditionm= Dz tanh(√2this parameter can be interpreted as the expected value of the MMSE estimate for the information bits 3using integration by parts formula for Gaussian random variablesThe formal calculations involved in the replica method make clear that the formula(9)should not depend on the distribution of the spreading sequence(see[7]).In the present problem one expects a priori that replica symmetry is not broken because of a gauge symmetry induced by channel symmetry.For this reason Tanaka’s formula is conjectured to be exact. Our upper bound(Theorem6)on the capacity precisely coincides with the above formulas and strongly supports this conjecture.Recent work announced by Montanari and Tse[10]also provides strong support to the conjecture at least in a regime ofβwithout phase transitions(more precisely,forβ≤βs(σ)whereβs(σ)is the maximal value ofβsuch that the solution of(12)remains unique).The authorsfirst solve the case of sparse signature sequence(using the area theorem and the data processing inequality)in the limit K→∞.Then the dense signature sequence(which is of interest here)is recovered by exchanging the K→∞and sparse→dense limits.1.4Gaussian inputsIn the case of continuous inputs x k∈R,in formulas(4),(5) x.The capacity is maximized by a Gaussian prior,p X)=e−||x22log(1+σ−2−12βlog(1+σ−2β−18βσ−2(14)whereQ(x,z)= z)2+1− z)2+1 2On the other hand Tanaka applied the formal replica method to this case and found(9)withc RS(m)=12βlogλσ2−λ1+λ(16)Solving(16)we obtain m=σ2N fixed(Theorems1,3in section2.1).As we will see the mathematical underpinning of this is the concentration of a more fundamental object,namely,the“free energy”of the associated spin system(Theorem2).Infact this turns out to be important in the proof of the bound on capacity.When the spreading coefficients are Gaussian the main tool used is a powerful theorem[9]of the concentration of Lipschitz functions of many independent Gaussian variables,and this leads to subexponential concentration bounds.For more general spreading coefficient distributions such tools do not suffice and we have to combine them with martingale arguments which lead to weaker algebraic bounds.Since the concentration proofs are mainly technical they are presented in appendices B,C.Sections3and4form the core of the paper.They detail the proof of the main Theorem6announced in section2.4,namely the tight upper bound on capacity.We use ideas from the interpolation method combined with a non-trivial concentration theorem for the empirical average of soft bit estimates.Section5shows that the average capacity is independent of the spreading sequence distribution at least for the case where it is symmetric and decays fast enough(Theorem4in section2.2).This enables us to restrict ourselves to the case of Gaussian spreading sequences which is more amenable to analysis. The existence of the limit K→∞for the capacity is shown in section6.Section7discusses various extensions of this work.We sketch the treatment for unequal powers for each user as well as colored noise.As alluded to before the bound on capacity for the case of Gaussian inputs can also be obtained by the present method and we give some indications to this effect.The appendices contain the proofs of various technical calculations.Preliminary versions of the results obtained in this paper have been summarized in references[20]and[21].2Main Results2.1ConcentrationIn the case of a Gaussian input signal,the concentration can be deduced from general theorems on the concentration of the spectral density for random matrices,but this approach breaks down for binary inputs.Here we prove,Theorem1(concentration of capacity,Gaussian spreading sequence,binary inputs).Assume the distribution p(s ik)are standard Gaussians.Givenǫ>0,there exists an integer K1=O(|lnǫ|) independent of p X;Y;Yσ4(64β+32+σ2)−1.16The mathematical underpinning of this result is in fact a more general concentration result for the free energy(6),that will be of some use latter on.Theorem2(concentration of free energy,Gaussian spreading sequence,binary inputs.). Assume the distribution p(s ik)are standard Gaussians.Givenǫ>0,there exists an integer K2= O(|lnǫ|)independent of p X√,s)−E Y,s)]|≥ǫ]≤3e−α2ǫ2σ4β3β+σ)−2.32We prove these theorems thanks to powerful probabilistic tools developed by Ledoux and Talagrand for Lipschitz functions of many Gaussian random variables.These tools are briefly reviewed in Appendix B for the convenience of the reader and the proofs of the theorems are presented in Appendix C. Unfortunately the same tools do not apply directly to the case of other spreading sequences.However in this case the following weaker result can at least be obtained.Theorem3(concentration,general spreading sequence).Assume the spreading sequence satisfies assumption B.There exists an integer K1independent of p X;Y;YKǫ2P[f(y,S[f(yKǫ2for some constantα>0and independent of K.To prove such estimates it is enough(by Chebycheff)to control second moments.For the mutual information we simply have to adapt martingale arguments of Pastur,Scherbina and Tirrozzi,[22,23] whereas the case of free energy is more complicated because of the additional Gaussian noisefluctuations. We deal with these by combining martingale arguments and Lipschitz function techniques.The concentration of capacity,namelyP[|maxp X ;Y E S[I(X)]|≥ǫK]≤α)P[maxp X ;Y;YKǫ2(18)To see this it suffices to note that for two positive functions f and g we have|max f−max g|≤max|f−g|. But unfortunately it is not clear how to extend our proofs to obtain(18).However as announced in the introduction we can deduce(18)from our theorems,by using the union bound,as long as the maximum is carried out over afinite set(sufficiently small with respect to K)of distributions.We wish to argue here that Theorem2suggests a method for proving the concentration of the bit error rate(BER)for uncoded communication1KKk=1x0,kˆx k)(19)where the MAP bit estimate for uncoded communication is defined through the marginal of(3),namelyˆx k=argmax xk ={±1}p(x k|yx k p(x,s)(a soft bit estimate or“magnetization”)can be obtained from the free energy by addingfirst an in-finitesimal perturbation(“small external magneticfield”)to the exponent in(3),namely h K k=1x0k x k, and then differentiating the perturbed free energy4,1dh 1,s)However one really needs to relate sign x k to the derivative of the free energy and this does not appear to be obvious.One way out is to introduce product measures of n copies(also called“real replicas”)of the posterior measurep(x,s)p(x,s)...p(x,s)and then relateKk=1(x0k x k )n=K k=1 x0k x1k...x0k x n k nto a suitable derivative of the replicated free energy.Then from the set of all moments one can in principle reconstruct sign x k .Thus one could try to deduce the concentration of the BER from the one for the free energy.However the completion of this program requires a uniform,with respect the system size,control of the derivative of the free energy precisely at h=0,which at the moment is still lacking5.2.2Independence with respect to the distribution of the spreading sequence The replica method leads to the same Tanaka formula for general class of symmetric distributions p(s ik)=p(−s ik).We are able to prove this:in particular binary and Gaussian spreading sequences lead to the same capacity.Theorem4.Consider CDMA with binary inputs and assume A for the spreading sequence.Let C g be the capacity for Gaussian spreading sequences(symmetric i.i.d with unit variance).Thenlim K→+∞(C K−C g)=0This theorem turns out to be very useful in order to obtain the bound on capacity because it allows us to make use of convenient integration by parts identities that have no clear counterpart in the non-Gaussian case.The proof of the theorem is given in section5.2.3Existence of the limit K→+∞The interpolation method can be used to show the existence of the limit K→+∞for C K.Theorem5.Consider CDMA with binary inputs and assume A for the spreading sequences with uniform input distribution.ThenlimK→∞C K exists(20)The proof of this theorem is given in section6for Gaussian spreading sequences.The general case then follows because of Theorem4.2.4Tight upper bound on the capacityThe main result of this paper is that Tanaka’s formula(10)is an upper bound to the capacity for all values ofβ.Theorem6.Consider CDMA with binary inputs and assume A for the spreading sequence.We havelim K→∞C K≤minm∈[0,1]c RS(m)(21)where c RS(m)is given by(10).If we combine this result with an inequality in Montanari and Tse[10],and exchanging as they do the limits of K→+∞and sparse→dense,one can deduce that the equality holds for some regime of noise smaller than a critical value.This value corresponds to the threshold for belief propagation decoding.Note that this equality is valid even ifβis such that there is a phase transition(thefixed point equation(12)has many solutions),whereas in[10]the equality holds for values ofβfor which the phase transition does not occur.Since the proof is rather complicated wefind it useful to give the main ideas in an informal way.The integral term in(10)suggests that we can replace the original system with a simpler system where the user bits are sent through K independent Gaussian channels given by˜y k=x k+1λw k(22)where w k∼N(0,1)andλis an effective SNR.Of course this argument is a bit naive because this effective system does not account for the extra terms in(10),but it has the merit of identifying the correct interpolation.We introduce an interpolating parameter t∈[0,1]such that the independent Gaussian channels correspond to t=0and the original CDMA system corresponds to t=1(see Figure2.4)It is convenient to denote the SNR of the original Gaussian channel as B(that is B=σ−2).Then(11)becomesλ=Bsx t ))yλ(t ))N (0,λ(t ))˜y ˜y ˜y Figure 1:The information bits x k are transmitted through the normal CDMA channel with variance 1λ(t )We introduce two interpolating SNR functions λ(t )and B (t )such thatλ(0)=λ,B (0)=0and λ(1)=0,B (1)=B (23)andB (t )1+βB (1−m )(24)The meaning of (24)is the following.In the interpolating t -system the effective SNR seen by each user has an effective t -CDMA part and an independent channel part λ(t )chosen such that the total SNR is fixed to the effective SNR of the CDMA system.There is a whole class of interpolating functions satisfying the above conditions but it turns out that we do not need to specify them more precisely except for the fact that B (t )is increasing,λ(t )is decreasing and with continuous first derivatives.Subsequent calculations are independent of the particular choices of functions.The parameter m is to be considered as fixed to any arbitrary value in [0,1].All the subsequent calculations are independent of its value,which is to be optimized to tighten the final bound.We now have two sets of channel outputs y(from the independent channels with noise variance λ(t )−1)and the interpolating communication system has a posterior distributionp t (x ,˜y 2K Z (y ,s )exp −B (t )−N −1 2−λ(t )−x (X2K .By analyzing the mutual information E S [I t (X ,˜Y ;Y ;˜Y,˜Y ,˜y 2K x ( 2πλ(t )−1)K e −B (t )−N −10 2−λ(t )−xIn order to carry out this program successfully it turns out that we need a concentration result on empirical average of the“magnetization”,m1=1,˜y0is transmitted.The distribution of the received vectors with this assumption isp t(y|s)=12πB(t)−1)N( 2 y2s x2 ˜y0 2(27)For technical reasons that will become clear only in the next section we consider a slightly more general interpolation system where the perturbation termh u(x uKk=1h k x k+u K k=1x0k x k−√=B(t)−1/2n0and˜y+x|n,h Zt,uexp −1+N−12s(x) 2(29)−1+λ(t)10−x)with the obvious normalization factor Z t,u.We define a free energyf t,u(n,hKln Z t,u(30) For t=1we recover the original free energy,E[f(y2+limu→0E[f1,u(n,hwhile for t=0the statistical sums decouple and we have the explicit result61,w,s)]=−1λz+λ))(31)where E denotes the appropriate collective expectation over random objects.In view of formula(7)in order to obtain the average capacity it is sufficient to computelimK→+∞limu→0E[f1,u(n,h2(32)There is no loss in generality in settingx0k=1(33) for the input symbols.From now on in sections3,4,and6we stick to(33).We also use the shorthand notationsz k=x0k−x k=1−x k,f t,u(n,h)|≤2√u E[|h k|]+u(34) therefore we can permute the two limits in(32)and computelimu→0limK→+∞E[f1,u]+1dtE[f t,u](35) Our task is now reduced to estimatinglimu→0limK→+∞1dtdKKk=1x k(36) A closely related quantity is the“overlap parameter”q12=1(1)|n,h(2)|n,h,w,s).u),uniformly in KLemma1.The distributions of m1and q12defined asP m1(x)=E δ(x−m1) t,u,P q12(x)=E δ(x−q12) t,uare equal,namelyP m1(x)=P q12(x)In particular the following identity holdsE[ m1 t,u]=E[ q12 t,u](38) Such identities are known as Nishimori identities in the statistical physics literature and are a consequence of a gauge symmetry satisfied by the measure E − t,u.They have also been used in the context of communications(see[11],[16]).For completeness a sketch of the proof is given in Appendix F.The next two identities also follow from similar considerations.Lemma2.LetZ+Ns z(α),α=1,2corresponding to z(α)k =1−x(α)k.We have then12 t,u]=1(39) andE[ (n(2))(z(2)) t,u]= k E[ (n)z k t,u](40)3.3Concentration of MagnetizationA crucial feature of the calculation in the next paragraph is that m1(and q12)concentrate,namely Theorem7.Fix anyǫ>0.For Lebesgue almost every u>ǫ,lim N→∞ 1dt E |m1−E m1 t,u| t=0The proof of this theorem,which is the point where the careful tuning of the perturbation is needed, has an interest of its own and is presented section4.Similar statements in the spin glass literature have been obtained by Talagrand[9].The usual signature of replica symmetry breaking is the absence of concentration for the overlap parameter q12.This theorem combined with the Nishimori identity “explains”why the replica symmetry is not broken.We will also need the following corollaryCorollary1.The following holds1·s z N3/2E n t,u(1−E m1 t,u)+o N(1)with lim N→+∞o N(1)=0for almost every u>0.Proof.By the Cauchy-Schwartz inequality1·s z N3/2(E (n)2 t,u)1/2×(E (E m1 t,u−m1)2 t,u)1/2Because of the concentration of the magnetization m1(theorem7)it suffices to prove thatE N−33.4Computation ofddtE [f t,u ]=T 1+T 2(42)whereT 1=−λ′(t )λ(t )KE wt,u −λ′(t )·zK√2·s z2+)·z 2(1)·(wλ(t )z2KE zt,u=−λ′(t )2E 1−m 1 t,uTo obtain the second equality we remark that the w2(1+β(1−m )B (t ))2E 1−m 1 t,u(45)3.4.2Transforming T 2The term T 2can be rewritten asT 2=−B ′(t ) 2t,u +B ′(t )2+B ′(t )B (t )K√·s z2NE nt,u (46)Now we use integration by parts with respect to s ik ,T 2=−B ′(t )·Z·z2KNE (n(2))(z(2)) t,uand the Nishimori identity (40)T 2=−B ′(t )·Z212 z k t,u ]−B ′(t ) 2KN 3/2kE (n)(1−x k ) t,uSince12=12βE 1−m 1 t,u +o N (1)−βB ′(t ) 2KN 1/2E (n )(1−m 1) t,uApplying Corollary 1to the last expression for T 2together with (46)we obtain a closed affine equation for the later,whose solution isT 2=−B ′(t )E 1−m 1 t2βln(1+βB (1−m ))from (35)and use the integral representation12β1dtβB ′(t )(1−m )2βln(1+βB (1−m ))+1dtd2(1+βB (t )(1−m ))If one uses (42)and expressions (45),(47)some remarkable algebra occurs in the last integral.The integrand becomesR (t )+B ′(t )(1−m )2(1+βB (t )(1−m ))2(1+βB (t )E 1−m 1 t,u )So the integral has a positive contribution 10dtR (t )≥0plus a computable contribution equal to B (1−m )2(1−m ).Finally thanks to (31)we find1λz +λ))−12βln(1+βB (1−m ))−λu )(48)where for a.e u >ǫ,lim N →∞o N (1)=0.We take first the limit N →∞,then u →ǫ(along some appropriate sequence)and then ǫ→0to obtain a formula for the free energy where the only non-explicit contribution is 10dtR (t ).Since this is positive for all m ,we obtain a lower bound on the free energy which is equivalent to the announced upper bound on the capacity.4Concentration of MagnetizationThe goal of this section is to prove Theorem 7.The proof is organized in a succession of lemmas.By the same methods used for Theorem 2we can proveLemma 3.There exists a strictly positive constant α(which remains positive for all t and u )such thatP [|f t,u −E [f t,u ]|≥ǫ]=O (e−αǫ2√Lemma 4.When considered as a function of u ,f t,u is convex in u .Proof.We simply evaluate the second derivative and show it is positive.d f t,u) t,u −1uk|h k |where we have definedL (xK1ukh k x k +1du 2=14u 3/2kh k x kt,u+1)2 t,u − L (x)turns out to be very useful and satisfies two concentration properties.Lemma 5.For any a >ǫ>0fixed,a ǫdu EL (x) t,u t,u=O1KProof.From equation (49),wehavea ǫdu EL (x) t,u2 t,u≤aǫdu1du 2E [f t,u ]≤1duE [f t,a ]−dKIn the very last equality we use that the first derivative of E [f t,u ]is bounded for u ≥ǫ.Using Cauchy-Schwartz inequality for E − t,u we obtain the lemma.Lemma 6.For any a >ǫ>0fixed,a ǫdu EL (x) t,u=O116Proof.From convexity of f t,u with respect to u (lemma 4)we have for any δ>0,dduE [f t,u ]≤f t,u +δ−f t,udu E [f t,u ]≤f t,u +δ−E [f t,u +δ]δ+dduE [f t,u ]A similar lower bound holds with δreplaced by −δ.Now from Lemma 3we know that the first twoterms are O (K1KKk =1|h k |are O (1K )we get EL (x) t,u ≤1√δO14+dduE [f t,u ]We will choose δ=18.Note that we cannot assume that the difference of the two derivatives is smallbecause the first derivative of the free energy is not uniformly continuous in K (as K →∞it may develop jumps at the phase transition points).The freeenergy itself is uniformly continuous.For this reason if we integrate with respect to u,using (34)we geta ǫdu EL (x) t,u ≤O 116Using the two last lemmas we can prove Theorem 7.Proof of Theorem 7:Combining the concentration lemmas we geta ǫdu E |L (x) t,u | t,u ≤O 116For any function g (x)|≤1,we haveaǫdu |E L (x) t,u −E L (x) t,u | t,u ≤aǫdu E |L (x) t,u | t,uMore generally the same thing holds if one takes a function depending on many replicas such asg (x(2))=q ing integration by parts formula with respect to h k ,E L (x2K √2E (1+q 12)q 12 t,u −12E (1+q 12)q 12 t,u =1) t,u E q 12 t,u =12(E m 1 t +(E m 1 t )2)(51)From equations (50)and (51),we geta ǫdu |E m 21 t,u −(E m 1 t,u )2|≤O116Now integrating with respect to t and exchanging the integrals (by Fubini’s theorem),we geta ǫdu 10dt |E m 21 t,u −(E m 1 t,u )2|≤O116The limit of the left hand side as K →∞therefore vanishes.By Lebesgue’s theorem this limit can beexchanged with the u integral and we get the desired result.(Note that one can further exchange the limit with the t -integral and obtain that the fluctuations of m 1vanish for almost every (t,u )).5Proof of independence from spreading sequence distribution:Theorem 4We consider a communication system with spreading values r ik generated from a symmetric distribution with unit variance and satisfying assumption A.We compare the capacity of this system to the Gaussian N (0,1)case whose spreading sequence values are denoted by s ik .The comparison is done through an interpolating system with respect to the two spreading sequencesv ik (t )=√1−ts ik ,0≤t ≤1。
The decay constant of the first excited pion from lattice QCD
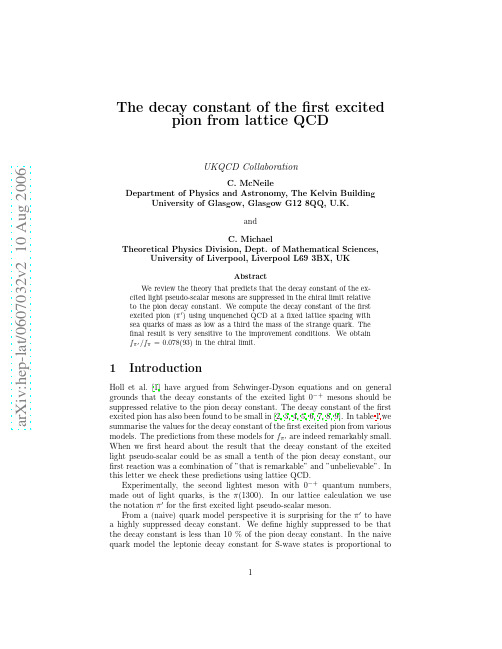
is
∂µAµ = mqπ
(2)
where π is the interpolating operator for pion states (pseudo-scalar density) and mq is the quark mass. Equation 2 is an operator relation, hence is true between any states. This allows us to write:
decay width of between 200 to 600 MeV. The predominant decay mode is to πππ (this includes ρπ). There is readable discussion about the experimental
2
Group Volkov and Weiss [4]
∂µAµ =
m2πn fπn πn
(5)
n
where πn is the interpolating operator for the n-th excited light 0−+ meson. The PDG [13] quotes the mass of the π(1300) as 1300 ± 100 MeV with a
Elias et al. [5] Maltman and Kambor [7]
Andrianov et al. [6] Kataev et al. [19, 20]
fπ′ MeV 0.68
4.2 ± 2.4 3.11 ± 0.65 0.52 − 2.26
4.3
Table 1: Summary of the values of the π′ decay constant determined from models and sum rules. Our normalisation convention is fπ = 131 MeV.
super string
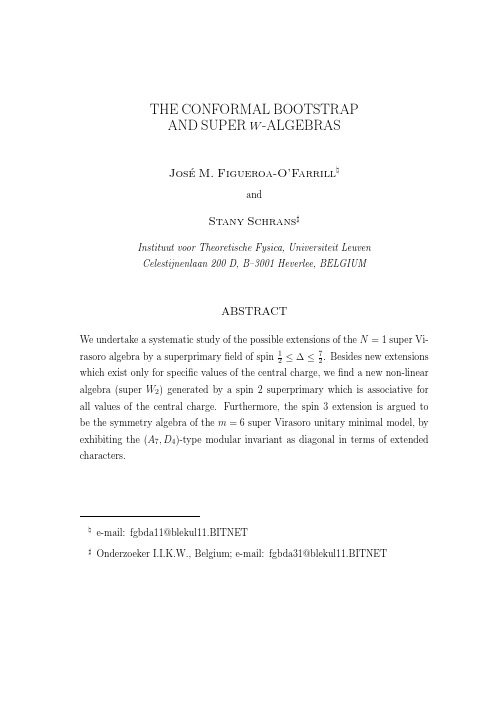
´ M. Figueroa-O’Farrill Jose
and
Stany Schrans
Instituut voor Theoretische Fysica, Universiteit Leuven Celestijnenlaan 200 D, B–3001 Heverlee, BELGIUM
e-mail: fgbda11@blekul11.BITNET Onderzoeker I.I.K.W., Belgium; e-mail: fgbda31@blekul11.BITNET
§1
Introduction Extended conformal and superconformal algebras have received a great deal
which exist only for specific values of the central charge, we find a new non-linear algebra (super W2 ) generated by a spin 2 superprimary which is associative for all values of the central charge. Furthermore, the spin 3 extension is argued to be the symmetry algebra of the m = 6 super Virasoro unitary minimal model, by exhibiting the (A7 , D4 )-type modular invariant as diagonal in terms of extended characters.
- 1、下载文档前请自行甄别文档内容的完整性,平台不提供额外的编辑、内容补充、找答案等附加服务。
- 2、"仅部分预览"的文档,不可在线预览部分如存在完整性等问题,可反馈申请退款(可完整预览的文档不适用该条件!)。
- 3、如文档侵犯您的权益,请联系客服反馈,我们会尽快为您处理(人工客服工作时间:9:00-18:30)。
a rXiv:h ep-th/9611152v12N ov1996CERN-TH/96-268hep-th/9611152ON NON-PERTURBATIVE RESULTS IN SUPERSYMMETRIC GAUGE THEORIES –A LECTURE 1Amit Giveon 2Theory Division,CERN,CH-1211,Geneva 23,Switzerland ABSTRACT Some notions in non-perturbative dynamics of supersymmetric gauge theories are being reviewed.This is done by touring through a few examples.CERN-TH/96-268September 19961IntroductionIn this lecture,we present some notions in supersymmetric Yang-Mills(YM) theories.We do it by touring through a few examples where we face a variety of non-perturbative physics effects–infra-red(IR)dynamics of gauge theories.We shall start with a general review;some of the points we consider follow the beautiful lecture notes in[1].Phases of Gauge TheoriesThere are three known phases of gauge theories:•Coulomb Phase:there are massless vector bosons(massless photonsγ;no confinement of both electric and magnetic charges).The behavior of the potential V(R)between electric test charges,separated by a large distance R,is V(R)∼1/R;the electric charge at large distance behaves like a constant:e2(R)∼constant.The potential of magnetic test charges separated by a large distance behaves like V(R)∼1/R,and the magnetic charge behaves like m2(R)∼constant,e(R)m(R)∼1 (the Dirac condition).•Higgs Phase:there are massive vector bosons(W bosons and Z bosons), electric charges are condensed(screened)and magnetic charges are confined(the Meissner effect).The potential between magnetic test charges separated by a large distance is V(R)∼ρR(the magnetic flux is confined into a thin tube,leading to this linear potential witha string tensionρ).The potential between electric test charges isthe Yukawa potential;at large distances R it behaves like a constant: V(R)∼constant.•Confining Phase:magnetic charges are condensed(screened)and elec-tric charges are confined.The potential between electric test charges separated by a large distance is V(R)∼σR(the electricflux is confined1into a thin tube,leading to the linear potential with a string tensionσ).The potential between magnetic test charges behaves like a constant at large distance R.Remarks1.In addition to the familiar Abelian Coulomb phase,there are theorieswhich have a non-Abelian Coulomb phase[2],namely,a theory with massless interacting quarks and gluons exhibiting the Coulomb poten-tial.This phase occurs when there is a non-trivial IRfixed point of the renormalization group.Such theories are part of other possible cases of non-trivial,interacting4d superconformalfield theories(SCFTs)[3,4]. 2.When there are matterfields in the fundamental representation of thegauge group,virtual pairs can be created from the vacuum and screen the sources.In this situation,there is no invariant distinction between the Higgs and the confining phases[5].In particular,there is no phase with a potential behaving as V(R)∼R at large distance,because the flux tube can break.For large VEVs of thefields,a Higgs description is most natural,while for small VEVs it is more natural to interpret the theory as“confining.”It is possible to smoothly interpolate from one interpretation to the other.3.Electric-Magnetic Duality:Maxwell theory is invariant underE→B,B→−E,(1.1) if we introduce magnetic charge m=2π/e and also interchangee→m,m→−e.(1.2) Similarly,Mandelstam and‘t Hooft suggested that under electric-magnetic duality the Higgs phase is interchanged with a confining phase.Con-finement can then be understood as the dual Meissner effect associated with a condensate of monopoles.2Dualizing a theory in the Coulomb phase,one remains in the same phase.For an Abelian Coulomb phase with massless photons,this electric-magnetic duality follows from a standard duality transforma-tion,and is extended to SL(2,Z)S-duality,acting on the complex gauge coupling byτ→aτ+b2π+i4πBy effective,we mean in Wilson sense:[modes p>µ]e−S=e−S ef f(µ,light modes),(2.3) so,in principle,L eff depends on a scaleµ.But due to supersymmetry,the dependence on the scaleµdisappear(except for the gauge couplingτwhich has a logµdependence).When there are no interacting massless particles,the Wilsonian effec-tive action=the1PI effective action;this is often the case in the Higgs or confining phases.2.1The Effective SuperpotentialWe will focus on a particular contribution to L eff–the effective superpo-tential term:L int∼ d2θW eff(X r,g I,Λ)+c.c,(2.4) where X r=light chiral superfields,g I=various coupling constants,and Λ=dynamically generated scale(associated with the gauge dynamics): log(Λ/µ)∼−8π2/g2(µ).Integrating overθ,the superpotential gives a scalar potential and Yukawa-type interaction of scalars with fermions.The quantum,effective superpotential W eff(X r,g I,Λ)is constrained by holomorphy,global symmetries and various limits[9,1]:1.Holomorphy:supersymmetry requires that W eff is holomorphic in thechiral superfields X r(i.e.,independent of the X†r).Moreover,we will think of all the coupling constants g I in the tree-level superpotential W tree and the scaleΛas background chiral superfield sources.This implies that W eff is holomorphic in g I,Λ(i.e.,independent of g∗I,Λ∗).2.Symmetries and Selection Rules:by assigning transformation laws bothto thefields and to the coupling constants(which are regarded as back-ground chiral superfields),the theory has a large global symmetry.This implies that W eff should be invariant under such global symmetries.43.Various Limits:W eff can be analyzed approximately at weak coupling,and some other limits(like large masses).Sometimes,holomorphy,symmetries and various limits are strong enough to determine W eff!The results can be highly non-trivial,revealing interesting non-perturbative dynamics.2.2The Gauge“Kinetic Term”in a Coulomb Phase When there is a Coulomb phase,there is a term in L eff of the formL gauge∼ d2θIm τeff(X r,g I,Λ)W2α ,(2.5) where Wα=gauge supermultiplet(supersymmetricfield strength);schemat-ically,Wα∼λα+θβσµνβαFµν+....Integrating overθ,W2αgives the term F2+iF˜F and its supersymmetric extension.Therefore,τeff=θeffg2eff(2.6)is the effective,complex gauge coupling.τeff(X r,g I,Λ)is also holomorphic in X r,g I,Λand,sometimes,it can be exactly determined by using holomorphy, symmetries and various limits.2.3The“Kinetic Term”The kinetic term is determined by the K¨a hler potential K:L kin∼ d2θd2¯θK(X r,X†r).(2.7) If there is an N=2supersymmetry,τeff and K are related;for an N=2 supersymmetric YM theory with a gauge group G and in a Coulomb phase, L eff is given in terms of a single holomorphic function F(A i):L eff∼Im d4θ∂F2 d2θ∂2FA manifestly gauge invariant N =2supersymmetric action which reduces to the above at low energies is[10]Imd 4θ∂F2 d 2θ∂2F3Some of these results also appear in the proceedings [13]of the 29th International Symposium on the Theory of Elementary Particles in Buckow,Germany,August 29-September 2,1995,and of the workshop on STU-Dualities and Non-Perturbative Phe-nomena in Superstrings and Supergravity ,CERN,Geneva,November 27-December 1,1995.6N A supermultiplets in the adjoint representation,Φab α,α=1,...,N A ,and N 3/2supermultiplets in the spin 3/2representation,Ψ.Here a,b are fundamental representation indices,and Φab =Φba (we present Ψin a schematic form as we shall not use it much).The numbers N f ,N A and N 3/2are limited by the condition:b 1=6−N f−2N A −5N 3/2≥0,(3.1)where −b 1is the one-loop coefficient of the gauge coupling beta-function.The main result of this section is the following:the effective superpoten-tial of an (asymptotically free or conformal)N =1supersymmetric SU (2)gauge theory,with 2N f doublets and N A triplets (and N 3/2quartets)isW N f ,N A (M,X,Z,N 3/2)=−δN 3/2,0(4−b 1) Λ−b 1Pf 2N f X det N A (Γαβ)2 1/(4−b 1)+Tr N A ˜mM +1√4Integrating in the “glueball”field S =−W 2α,whose source is log Λb 1,gives the non-perturbative superpotential:W (S,M,X,Z )=S log Λb 1S 4−b 1Here,the a,b indices are raised and lowered with anǫab tensor.The gauge-invariant superfields X ij may be considered as a mixture of SU(2)“mesons”and“baryons,”while the gauge-invariant superfields Zαij may be considered as a mixture of SU(2)“meson-like”and“baryon-like”operators.Equation(3.2)is a universal representation of the superpotential for all infra-red non-trivial theories;all the physics we shall discuss(and beyond) is in(3.2).In particular,all the symmetries and quantum numbers of thevarious parameters are already embodied in W Nf,N A .The non-perturbativesuperpotential is derived in refs.[11,12]by an“integrating in”procedure, following refs.[14,15].The details can be found in ref.[12]and will not be presented here5.Instead,in the next sections,we list the main results concerning each of the theories,N f,N A,N3/2,case by case.Moreover,a few generalizations to other gauge groups will be discussed.4b1=6:N f=N A=N3/2=0This is a pure N=1supersymmetric SU(2)gauge theory.The non-perturbative effective superpotential is6W0,0=±2Λ3.(4.1) The superpotential in eq.(4.1)is non-zero due to gaugino(gluino)conden-sation7.Let us consider gaugino condensation for general simple groups[1].Pure N=1Supersymmetric Yang-Mills TheoriesPure N=1supersymmetric gauge theories are theories with pure superglue with no matter.We consider a theory based on a simple group G.The theorycontains vector bosons Aµand gauginosλαin the adjoint representation of G.There is a classical U(1)R symmetry,gaugino number,which is broken tosubgroup by instantons,a discrete Z2C2(λλ)C2 =const.Λ3C2,(4.2) where C2=the Casimir in the adjoint representation normalized such that, for example,C2=N c for G=SU(N c).This theory confines,gets a mass gap,and there are C2vacua associ-symmetry to Z2by gaugino ated with the spontaneous breaking of the Z2C2condensation:λλ =const.e2πin/C2Λ3,n=1,...,C2.(4.3) Each of these C2vacua contributes(−)F=1and thus the Witten index is Tr(−)F=C2.This physics is encoded in the generalization of eq.(4.1)to any G,givingW eff=e2πin/C2C2Λ3,n=1,...,C2.(4.4) For G=SU(2)we have C2=2.Indeed,the“±”in(4.1),which comes from the square-root appearing on the braces in(3.2)when b1=6,corresponds, physically,to the two quantum vacua of a pure N=1supersymmetric SU(2) gauge theory.The superpotentials(4.1),(4.3)can be derived byfirst adding fundamen-tal matter to pure N=1supersymmetric YM theory(as we will do in the next section),and then integrating it out.5b1=5:N f=1,N A=N3/2=0There is one case with b1=5,namely,SU(2)with oneflavor.The superpo-tential isΛ5W1,0=vacuum degeneracy of the classical low-energy effective theory is lifted quan-tum mechanically;from eq.(5.1)we see that,in the massless case,there is no vacuum at all.SU(N c)with N f<N cEquation(5.1)is a particular case of SU(N c)with N f<N c(N f quarks Q i and N f anti-quarks¯Q¯i,i,¯i=1,...,N f)[1].In these theories,by using holomorphy and global symmetries,U(1)Q×U(1)¯Q×U(1)RQ:100¯Q:010(5.2)Λ3N c−N f:N f N f2N c−2N fW:002onefinds thatW eff=(N c−N f) Λ3N c−N f N c−N f,(5.3) whereX i¯i≡Q i¯Q¯i,i,¯i=1,...,N f.(5.4) Classically,SU(N c)with N f<N c is broken down to SU(N c−N f).The ef-fective superpotential in(5.3)is dynamically generated by gaugino condensa-tion in SU(N c−N f)(for N f≤N c−2)8,and by instantons(for N f=N c−1).The SU(2)with N f=1ExampleFor example,let us elaborate on the derivation and physics of eq.(5.1).An SU(2)effective theory with two doublets Q a i has one light degree of freedom: four Q a i(i=1,2is aflavor index,a=1,2is a color index;2×2=4)threeout of which are eaten by SU(2),leaving4−3=1.This single light degree of freedom can be described by the gauge singletX=Q1Q2.(5.5) When X =0,SU(2)is completely broken and,classically,W eff,class=0 (when X =0there are extra masslessfields due to an unbroken SU(2)). Therefore,the classical scalar potential is identically zero.However,the one-instanton action is expected to generate a non-perturbative superpotential.The symmetries of the theory(at the classical level and with their cor-responding charges)are:U(1)Q=number of Q1fields(quarks or squarks),1=number of Q2fields(quarks or squarks),U(1)R={number of U(1)Q2gluinos}−{number of squarks}.At the quantum level these symmetries are anomalous–∂µjµ∼F˜F–and by integrating both sides of this equation one gets a charge violation when there is an instanton background I.The instanton background behaves likeI∼e−8π2/g2(µ)= Λ9For SU(N c)with N fflavors,the instanton background I has2C2=2N c gluino zero-modesλand2N f squark zero-modes q and,therefore,its R-charge is R(I)=number(λ)−number(q)=2N c−2N f.Since I∼Λb1and b1=3N c−N f,we learn thatΛ3N c−N f has an R-charge=2N c−2N f,as it appears in eq.(5.2).11and,therefore,W eff has charges:U(1)Q(5.9)1×U(1)Q2×U(1)RW eff:002Finally,because W eff is holomorphic in X,Λ,and is invariant under symme-tries,we must haveΛ5W eff(X,Λ)=c10This is reflected in eq.(3.2)by the vanishing of the coefficient(4−b1)in front of the braces,leading to W=0,and the singular power1/(4−b1)on the braces,when b1=4, which signals the existence of a constraint.12At the classical limit,Λ→0,the quantum constraint collapses into the clas-sical constraint,Pf X=0.SU(N c)with N f=N cEquations(6.1),(6.2)are a particular case of SU(N c)with N f=N c.[1]In these theories one obtains W eff=0,and the classical constraint det X−B¯B=0is modified quantum mechanically todet X−B¯B=Λ2N c,(6.3) whereX i¯i=Q i¯Q¯i(mesons),B=ǫi1...i N c Q i1···Q i N c(baryon),¯B=ǫ¯i1...¯i N c¯Q¯i1···¯Q¯iN c(anti−baryon).(6.4)6.2N f=0,N A=1,N3/2=0The massless N A=1case is a pure SU(2),N=2supersymmetric Yang-Mills theory.This model was considered in detail in ref.[17].The non-perturbative superpotential vanishesW non−per.0,1=0,(6.5) and by the integrating in procedure we also get the quantum constraint:M=±Λ2.(6.6) This result can be understood because the starting point of the integrating in procedure is a pure N=1supersymmetric Yang-Mills theory.Therefore,it leads us to the points at the verge of confinement in the moduli space.These are the two singular points in the M moduli space of the theory;they are due to massless monopoles or dyons.Such excitations are not constructed out of the elementary degrees of freedom and,therefore,there is no trace for them in W.(This situation is different if N f=0,N A=1;in this case,monopoles are different manifestations of the elementary degrees of freedom.)137b1=3There are two cases with b1=3:either N f=3,or N A=N f=1.In both cases,for vanishing bare parameters in(3.2),the semi-classical limit,Λ→0, imposes the classical constraints,given by the equations of motion:∂W=0; however,quantum corrections remove the constraints.7.1N f=3,N A=N3/2=0The superpotential isW3,0=−Pf X2Tr mX.(7.1)In the massless case,the equations∂X W=0give the classical constraints; in particular,the superpotential is proportional to a classical constraint: Pf X=0.The negative power ofΛ,in eq.(7.1)with m=0,indicates that small values ofΛimply a semi-classical limit for which the classical constraints are imposed.SU(N c)with N f=N c+1Equation(7.1)is a particular case of SU(N c)with N f=N c+1[1].In these theories one obtainsW eff=−det X−X i¯iB i¯B¯iThis is consistent with the negative power ofΛin W eff which implies that in the semi-classical limit,Λ→0,the classical constraints are imposed. 7.2N f=1,N A=1,N3/2=0In this case,the superpotential in(3.2)readsW1,1=−Pf X2Tr mX+12TrλZ.(7.5)Here m,X are antisymmetric2×2matrices,λ,Z are symmetric2×2 matrices andΓ=M+Tr(ZX−1)2.(7.6) This superpotential was foundfirst in ref.[18].Tofind the quantum vacua, we solve the equations:∂M W=∂X W=∂Z W=0.Let us discuss some properties of this theory:•The equations∂W=0can be re-organized into the singularity condi-tions of an elliptic curve:y2=x3+ax2+bx+c(7.7)(and some other equations),where the coefficients a,b,c are functions of only thefield M,the scaleΛ,the bare quark masses,m,and Yukawa couplings,λ.Explicitly,a=−M,b=Λ316,(7.8)whereα=Λ62Γ.(7.10)15•W1,1has2+N f=3vacua,namely,the three singularities of the elliptic curve in(7.7),(7.8).These are the three solutions,M(x),of the equations:y2=∂y2/∂x=0;the solutions for X,Z are given by the other equations of motion.•The3quantum vacua are the vacua of the theory in the Higgs-confinement phase.•Phase transition points to the Coulomb branch are at X=0⇔˜m= 0.Two of these singularities correspond to a massless monopole or dyon,and are the quantum splitting of the classically enhanced SU(2) point.A third singularity is due to a massless quark;it is a classical singularity:M∼m2/λ2for large m,and thus M→∞when m→∞, leaving the two quantum singularities of the N A=1,N f=0theory.•The elliptic curve defines the effective Abelian coupling,τ(M,Λ,m,λ), in the Coulomb branch:Elliptic Curves and Effective Abelian CouplingsA torus can be described by the one complex dimensional curve in C2 y2=x3+ax2+bx+c,where(x,y)∈C2and a,b,c are complex parameters.The modular parameter of the torus isτ(a,b,c)= βdx αdxIn this form,the modular parameterτis determined(modulo SL(2,Z)) by the ratio f3/g2through the relation4(24f)3j(τ)=8b1=2There are three cases with b1=2:N f=4,or N A=1,N f=2,or N A=2.In all three cases,for vanishing bare parameters in(3.2),there are extra massless degrees of freedom not included in the procedure;those are expected due toa non-Abelian conformal theory.8.1N f=4,N A=N3/2=0The superpotential isW4,0=−2(Pf X)1Λ+12N c<N f<3N c the theory is in an interacting,non-AbelianCoulomb phase(in the IR and for m=0).In this range of N f the theory is asymptotically ly,at short distance the coupling18constant g is small,and it becomes larger at larger distance.However, it is argued that for32N c<N f<3N c,the IR theory is a non-trivial4d SCFT.The elementary quarks and gluons are not confined but appear as in-teracting massless particles.The potential between external massless electric sources behaves as V∼1/R,and thus one refers to this phase of the theory as the non-Abelian Coulomb phase.•The Seiberg Duality:it is claimed[2]that in the IR an SU(N c)theory with N fflavors is dual to SU(N f−N c)with N fflavors but,in addition to dual quarks,one should also include interacting,massless scalars. This is the origin to the branch cut in W eff at X =0,because W eff does not include these light modes which must appear at X =0. The quantum numbers of the quarks and anti-quarks of the SU(N c) theory with N fflavors(=theory A)are11A.SU(N c),N f:The Electric TheorySU(N f)L×SU(N f)R×U(1)B×U(1)RQ:N f111−N cN f(8.2)The quantum numbers of the dual quarks q i and anti-quarks¯q¯i of the SU(N f−N c)theory with N fflavors theory(=theory B)and its mass-less scalars X i¯iareB.SU(N f−N c),N f:The Magnetic TheorySU(N f)L×SU(N f)R×U(1)B×U(1)R q:¯N f1N cN f ¯q:1N f−N c N fX:N f¯N f02 1−N ctheory A and theory B have the same anomalies:U(1)3B:0U(1)B U(1)2R:0U(1)2B U(1)R:−2N2cSU(N f)3:N c d(3)(N f)SU(N f)2U(1)R:−N2cN2f(8.5)Here d(3)(N f)=Tr T3f of the global SU(N f)symmetries,where T fare generators in the fundamental representation,and d(2)(N f)=Tr T2f2.Deformations:theory A and theory B have the same quantummoduli space of deformations.Remarks•Electric-magnetic duality exchanges strong coupling with weak cou-pling(this can be read offfrom the beta-functions),and it interchanges a theory in the Higgs phase with a theory in the confining phase.•Strong-weak coupling duality also relates an SU(N c)theory with N f≥3N c to an SU(N f−N c)theory.SU(N c)with N f≥3N c is in a non-Abelian free electric phase:in this range the theory is not asymptoti-cally ly,because of screening,the coupling constant becomes smaller at large distance.Therefore,the spectrum of the theory at large distance can be read offfrom the Lagrangian–it consists of the elemen-tary quarks and gluons.The long distance behavior of the potential between external electric test charges isV(R)∼1R,e(R→∞)→0.(8.6)For N f≥3N c,the theory is thus in a non-Abelian free electric phase; the massless electrically chargedfields renormalize the charge to zero21at long distance as e −2(R )∼log(R Λ).The potential of magnetic test charges behave at large distance R asV (R )∼log(R Λ)R ,⇒e (R )m (R )∼1.(8.7)SU (N c )with N f ≥3N c is dual to SU (˜N c )with ˜N c +2≤N f ≤3R ∼e 2(R )2˜N c ,the massless magnetic monopoles renormal-ize the electric coupling constant to infinity at large distance,with a conjectured behavior e 2(R )∼log(R Λ).The potential of magnetic test charges behaves at large distance R asV (R )∼1R ⇒e (R )m (R )∼1.(8.9)•The Seiberg duality can be generalized in many other cases,includ-ing a variety of matter supermultiplets (like superfields in the adjoint representation [20])and other gauge groups [21].8.2N f =2,N A =1,N 3/2=0In this case,the superpotential in (3.2)readsW 2,1=−2(Pf X )1ΛΓ+˜mM +1√•The equations∂W=0can be re-organized into the singularity condi-tions of an elliptic curve(7.7)(and some other equations),where the coefficients a,b,c are functions of only thefield M,the scaleΛ,the bare quark masses,m,and Yukawa couplings,λ.Explicitly[11,12],a=−M,b=−α4Pf m,c=α16detλ,µ=λ−1m.(8.12)•As in section7.2,the parameter x,in the elliptic curve(7.7),is given in terms of the compositefield:x≡1•As in section7.2,the negative power ofΛ,in eq.(8.10)with˜m= m=λ=0,indicates that small values ofΛimply a semi-classical limit for which the classical constraints are imposed.Indeed,for vanishing bare parameters,the equations∂W=0are equivalent to the classical constraints,and their solutions span the Higgs moduli space[22].•For special values of the bare masses and Yukawa couplings,some of the 4vacua degenerate.In some cases,it may lead to points where mutually non-local degrees of freedom are massless,similar to the situation in pure N=2supersymmetric gauge theories,considered in[3].For example,when the masses and Yukawa couplings approach zero,all the 4singularities collapse to the origin.Such points might be interpreted as in a non-Abelian Coulomb phase[1]or new non-trivial,interacting, N=1SCFTs.•The singularity at X=0(inΓ)and the branch cut at Pf X=0 (due to the1/2power in eq.(8.10))signal the appearance of extra massless degrees of freedom at these points;those are expected similar to references[2,20].Therefore,we make use of the superpotential only in the presence of bare parameters,whichfix the vacua away from such points.8.3N f=0,N A=2,N3/2=0In this case,the superpotential in eq.(3.2)readsdet MW0,2=±212The fractional power1/(4−b1)on the braces in(3.2),for any theory with b1≤2, may indicate a similar phenomenon,namely,the existence of confinement and oblique24theory has two quantum vacua;these become the phase transition points to the Coulomb branch when det˜m=0.The moduli space may also contain a non-Abelian Coulomb phase when the two singularities degenerate at the point M=0[18];this happens when˜m=0.At this point,the theory has extra massless degrees of freedom and,therefore,W0,2fails to describe the physics at˜m=0.Moreover,at˜m=0,the theory has other descriptions via an electric-magnetic triality[1].9b1=1There are four cases with b1=1:N f=5,or N A=1,N f=3,or N A=2, N f=1,or N3/2=1.9.1N f=5,N A=N3/2=0The superpotential isW5,0=−3(Pf X)1Λ12Tr mX.(9.1)This theory is a particular case of SU(N c)with N f>N c+1.The discussion in section8.1is relevant in this case too.9.2N f=3,N A=1,N3/2=0In this case,the superpotential in(3.2)readsW3,1=−3(Pf X)1Λ13+˜mM+1√confinement branches of the theory,corresponding to the4−b1phases due to the fractional power.It is plausible that,for SU(2),such branches are related by a discrete symmetry.25•The equations∂W=0can be re-organized into the singularity condi-tions of an elliptic curve(7.7)(and some other equations),where the coefficients a,b,c are[11,12]a=−M−α,b=2αM+α4Pf m,c=α64detλ,µ=λ−1m.(9.4) In eq.(9.3)we have shifted the quantumfield M toM→M−α/4.(9.5)•The parameter x,in the elliptic curve(7.7),is given in terms of thecompositefield:x≡12.(9.6)Therefore,as before,we have identified a physical meaning of the pa-rameter x.•W3,1has2+N f=5quantum vacua,corresponding to the5singularities of the elliptic curve(7.7),(9.3);these are the vacua of the theory in the Higgs-confinement phase.•From the phase transition points to the Coulomb branch,we conclude that the elliptic curve defines the effective Abelian coupling,τ(M,Λ,m,λ), for arbitrary bare masses and Yukawa couplings.As before,on the sub-space of bare parameters,where the theory has N=2supersymmetry, the result in eq.(9.3)coincides with the result in[7]for N f=3.•For special values of the bare masses and Yukawa couplings,some of the 5vacua degenerate.In some cases,it may lead to points where mutually non-local degrees of freedom are massless,and might be interpreted as in a non-Abelian Coulomb phase or another new superconformal theory in four dimensions(see the discussion in sections7.2and8.2).26•The singularity and branch cuts in W3,1signal the appearance of extra massless degrees of freedom at these points.•The discussion in the end of sections7.2and8.2is relevant here too.9.3N f=1,N A=2,N3/2=0In this case,the superpotential in(3.2)reads[12]W1,2=−3(Pf X)1/32Tr mX+12TrλαZα.(9.7)Here m and X are antisymmetric2×2matrices,λαand Zαare symmetric 2×2matrices,α=1,2,˜m,M are2×2symmetric matrices andΓαβis given in eq.(3.3).This theory has3quantum vacua in the Higgs-confinement branch.At the phase transition points to the Coulomb branch,namely, when det˜m=0⇔det M=0,the equations of motion can be re-organized into the singularity conditions of an elliptic curve(7.7).Explicitly,when ˜m22=˜m12=0,the coefficients a,b,c in(7.7)are[12]a=−M22,b=Λ˜m21132 2detλ2.(9.8)However,unlike the N A=1cases,the equations∂W=0cannot be re-organized into the singularity condition of an elliptic curve,in general.This result makes sense,physically,since an elliptic curve is expected to“show up”only at the phase transition points to the Coulomb branch.For special values of the bare parameters,there are points in the moduli space where (some of)the singularities degenerate;such points might be interpreted as in a non-Abelian Coulomb phase,or new superconformal theories.For more details,see ref.[12].9.4N f=N A=0,N3/2=1This chiral theory was shown to have W non−per.0,0(N3/2=1)=0;[24]perturb-ing it by a tree-level superpotential,W tree=gU,where U is given in(3.4), may lead to dynamical supersymmetry breaking[24].2710b1=0There arefive cases with b1=0:N f=6,or N A=1,N f=4,or N A= N f=2,or N A=3,or N3/2=N f=1.These theories have vanishing one-loop beta-functions in either conformal or infra-red free beta-functions and, therefore,will possess extra structure.10.1N f=6,N A=N3/2=0This theory is a particular case of SU(N c)with N f=3N c;the electric theory is free in the infra-red[1].1310.2N f=4,N A=1,N3/2=0In this case,the superpotential in(3.2)readsW4,1=−4(Pf X)1Λb12+˜mM+1√β2 2α+1β2α13A related fact is that(unlike the N A=1,N f=4case,considered next)in the(would be)superpotential,W6,0=−4Λ−b1/4(Pf X)1/4+1。