计量虚拟变量讲解
合集下载
计量经济学第5章 虚拟变量模型
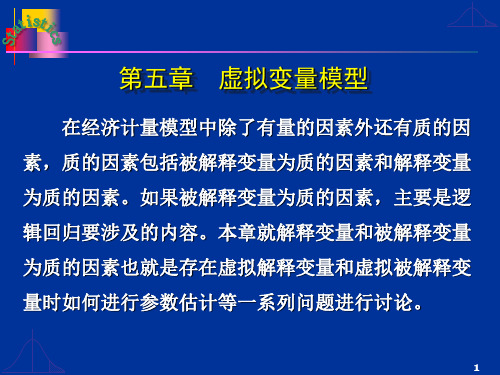
第五章 虚拟变量模型
在经济计量模型中除了有量的因素外还有质的因 素,质的因素包括被解释变量为质的因素和解释变量 为质的因素。如果被解释变量为质的因素,主要是逻 辑回归要涉及的内容。本章就解释变量和被解释变量 为质的因素也就是存在虚拟解释变量和虚拟被解释变 量时如何进行参数估计等一系列问题进行讨论。
1
为基础类型截距项。
12
三、虚拟变量的作用 ⑴ 可以描述和测量定性因素的影响。
⑵ 能够正确反映经济变量之间的相互关系,提 高模型的精度。
⑶ 便于处理异常数据。
即将异常数据作为一个特殊的定性因素
1 , 异常时期
D
0
,
正常时期
13
第二节 虚拟解释变量模型
一 、截距变动模型(加法模型)
虚拟变量与其它变量相加,以加法形式引入模
Y i 0 1 D 1 i 2 D 2 i 3 X i u i
Y i ------年支出医疗保健费用支出 X i ------居民年可支配收入
18
1 , 高中
D 1i
0
,
其他
1 , 大学
D 2i
0
,
其他
于是:小学教育程度:
E (Y i X i,D 1 i 0 ,D 2 i 0 )03 X i
7
二、虚拟变量的设置规则
虚拟解释变量模型的设定因为质的因素的多少 和这些因素特征的多少而引入的虚拟变量也会不同。
以一个最简单的虚拟变量模型为例,如果只包 含一个质的因素,而且这个因素仅有两个特征,则 回归模型中只需引入一个虚拟变量。如果是含有多 个质的因素, 自然要引入多个虚拟变量。
8
如果只有一个质的因素,且该质的因素具有 m 个 相互排斥的特征(或类型、属性),那么在含有截距 项的模型中,只能引入 m-1 个虚拟变量,否则会陷入 所谓“虚拟变量陷阱”(dummy variable trap),产 生 完全的多重共线性,会使最小二乘法无解;在不含有 截距项的模型中, 引入 m 个虚拟变量不会导致完全 的多重共线性,不过这时虚拟变量参数的估计结果, 实际上是 D = 1 时的样本均值。
在经济计量模型中除了有量的因素外还有质的因 素,质的因素包括被解释变量为质的因素和解释变量 为质的因素。如果被解释变量为质的因素,主要是逻 辑回归要涉及的内容。本章就解释变量和被解释变量 为质的因素也就是存在虚拟解释变量和虚拟被解释变 量时如何进行参数估计等一系列问题进行讨论。
1
为基础类型截距项。
12
三、虚拟变量的作用 ⑴ 可以描述和测量定性因素的影响。
⑵ 能够正确反映经济变量之间的相互关系,提 高模型的精度。
⑶ 便于处理异常数据。
即将异常数据作为一个特殊的定性因素
1 , 异常时期
D
0
,
正常时期
13
第二节 虚拟解释变量模型
一 、截距变动模型(加法模型)
虚拟变量与其它变量相加,以加法形式引入模
Y i 0 1 D 1 i 2 D 2 i 3 X i u i
Y i ------年支出医疗保健费用支出 X i ------居民年可支配收入
18
1 , 高中
D 1i
0
,
其他
1 , 大学
D 2i
0
,
其他
于是:小学教育程度:
E (Y i X i,D 1 i 0 ,D 2 i 0 )03 X i
7
二、虚拟变量的设置规则
虚拟解释变量模型的设定因为质的因素的多少 和这些因素特征的多少而引入的虚拟变量也会不同。
以一个最简单的虚拟变量模型为例,如果只包 含一个质的因素,而且这个因素仅有两个特征,则 回归模型中只需引入一个虚拟变量。如果是含有多 个质的因素, 自然要引入多个虚拟变量。
8
如果只有一个质的因素,且该质的因素具有 m 个 相互排斥的特征(或类型、属性),那么在含有截距 项的模型中,只能引入 m-1 个虚拟变量,否则会陷入 所谓“虚拟变量陷阱”(dummy variable trap),产 生 完全的多重共线性,会使最小二乘法无解;在不含有 截距项的模型中, 引入 m 个虚拟变量不会导致完全 的多重共线性,不过这时虚拟变量参数的估计结果, 实际上是 D = 1 时的样本均值。
金融计量经济第五讲虚拟变量模型和Probit、Logit模型
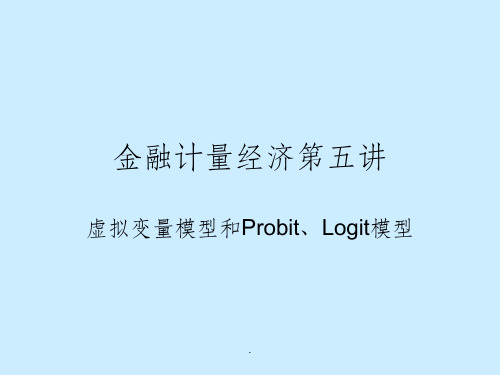
.
二、虚拟变量的设置原则
• 引入虚拟变量一般取0和1。
• 对定性因素一般取级别数减1个虚拟变量。例 子1:性别因素,二个级别(男、女)取一个 虚拟变量,D=1表示男(女),D=0表示女 (男)。
• 例子2:季度因素,四个季度取3个变量。
1, 一季度 D1 0, 其它季度
1, 二季度
D2
0,
其它季度
• 同样可以写成二个模型:
y ˆi ˆ0(ˆˆ1)x1iˆkxki D1
y ˆi ˆ0ˆ1x1iˆkxki
D0
• 可考虑同时在截距和斜率引入虚拟变量:
y i 0 0 D i (1 D i 1 ) x 1 i k x k iu i (5.
.
.
• 3、虚拟变量用于季节性因素分析。
•取
1, 当样本 i季为 度第 的数据 Di 0,其它季度的, i数 2,3据 ,4
• 工资模型为:
• Ii01 [S 1 (1 D 1 i D 2 i)S ( i S 1 )] 2 [D 2 i(S 2 S 1 ) D 1 i(S i S 1 ) ]3 D 2 i(S i S 2 ) u i (5.7
.
D2=1
S0
D1=1
S1
S2
.
• 作OLS得到参数估计值后,三个阶段的 报酬回归模型为: Iˆi ˆ0ˆ1Si, Si S1 Iˆi ˆ0ˆ1S1ˆ2(Si S1), S2Si S1 Iˆi ˆ0ˆ1S1ˆ2(S2S1)ˆ3(Si S2), Si S2
0.503543 0.500354 1.13E+03 1.99E+09 -13241.74 1.648066
Mean dependent var S.D. dependent var Akaike info criterion Schwarz criterion F-statistic Prob(F-statistic)
二、虚拟变量的设置原则
• 引入虚拟变量一般取0和1。
• 对定性因素一般取级别数减1个虚拟变量。例 子1:性别因素,二个级别(男、女)取一个 虚拟变量,D=1表示男(女),D=0表示女 (男)。
• 例子2:季度因素,四个季度取3个变量。
1, 一季度 D1 0, 其它季度
1, 二季度
D2
0,
其它季度
• 同样可以写成二个模型:
y ˆi ˆ0(ˆˆ1)x1iˆkxki D1
y ˆi ˆ0ˆ1x1iˆkxki
D0
• 可考虑同时在截距和斜率引入虚拟变量:
y i 0 0 D i (1 D i 1 ) x 1 i k x k iu i (5.
.
.
• 3、虚拟变量用于季节性因素分析。
•取
1, 当样本 i季为 度第 的数据 Di 0,其它季度的, i数 2,3据 ,4
• 工资模型为:
• Ii01 [S 1 (1 D 1 i D 2 i)S ( i S 1 )] 2 [D 2 i(S 2 S 1 ) D 1 i(S i S 1 ) ]3 D 2 i(S i S 2 ) u i (5.7
.
D2=1
S0
D1=1
S1
S2
.
• 作OLS得到参数估计值后,三个阶段的 报酬回归模型为: Iˆi ˆ0ˆ1Si, Si S1 Iˆi ˆ0ˆ1S1ˆ2(Si S1), S2Si S1 Iˆi ˆ0ˆ1S1ˆ2(S2S1)ˆ3(Si S2), Si S2
0.503543 0.500354 1.13E+03 1.99E+09 -13241.74 1.648066
Mean dependent var S.D. dependent var Akaike info criterion Schwarz criterion F-statistic Prob(F-statistic)
计量经济学第07章 虚拟变量模型-第1节33

假设我们用 OLS对这两个方程分别进行估计,可 得到各自的误差平方和 RSS1 和 RSS2,由于对模型 的系数没有任何限制条件,可以将这两个误差平
方和相加求和表示无条件平方和,即
RS US R RS 1 SRS 2,S其自由度为
(N-K)+(M-K)= N + M-2K。
假设原假设为真,即 1 1,2 2,…, k k ,以及Var ( e 1 i ) = Var ( e 2 i ),则回归模型 (7.23)、(7.24)可以合并为用一个方程表示:
Y)与收入( X )的回归模型估计结果为:
Y ˆt 0.266 0.04Xt7
SE:(0.3053) (0.0266)
t:(-0.8719) (1.7669)
R 2 0 . 309 R 1 2 0 S . 1S 3d 9 2 6 f 6
改革开放后(1978—2000年)居民储蓄 (Y) 与收 入 ( X ) 的回归模型估计结果为:
D1i
1 0
城市 农村
1 男
D2i 0
女
C 为香烟消费;Y 为居民收入。
如果(7.7)式满足OLS的基本假设条件, 可估计出各类型居民香烟消费函数分别为:
农村女性居民: Cˆi ˆ0ˆ3Yi
(7.8)
城市女性居民: C ˆi (ˆ0ˆ1)ˆ3Yi (7.9)
农村男性居民: C ˆi (ˆ0ˆ2)ˆ3Yi (7.10)
回归(Parallel regression)( 见图7.6b)。 (3) D i 的系数等于零,Di X i 的系数不等于零,
即 2 , 1 2 1 ,说明两个回归模型之间的截距相 同,结构差异仅仅表现在斜率上,称之为汇合回
归(Concurrent regression)(见图7.6c)。
方和相加求和表示无条件平方和,即
RS US R RS 1 SRS 2,S其自由度为
(N-K)+(M-K)= N + M-2K。
假设原假设为真,即 1 1,2 2,…, k k ,以及Var ( e 1 i ) = Var ( e 2 i ),则回归模型 (7.23)、(7.24)可以合并为用一个方程表示:
Y)与收入( X )的回归模型估计结果为:
Y ˆt 0.266 0.04Xt7
SE:(0.3053) (0.0266)
t:(-0.8719) (1.7669)
R 2 0 . 309 R 1 2 0 S . 1S 3d 9 2 6 f 6
改革开放后(1978—2000年)居民储蓄 (Y) 与收 入 ( X ) 的回归模型估计结果为:
D1i
1 0
城市 农村
1 男
D2i 0
女
C 为香烟消费;Y 为居民收入。
如果(7.7)式满足OLS的基本假设条件, 可估计出各类型居民香烟消费函数分别为:
农村女性居民: Cˆi ˆ0ˆ3Yi
(7.8)
城市女性居民: C ˆi (ˆ0ˆ1)ˆ3Yi (7.9)
农村男性居民: C ˆi (ˆ0ˆ2)ˆ3Yi (7.10)
回归(Parallel regression)( 见图7.6b)。 (3) D i 的系数等于零,Di X i 的系数不等于零,
即 2 , 1 2 1 ,说明两个回归模型之间的截距相 同,结构差异仅仅表现在斜率上,称之为汇合回
归(Concurrent regression)(见图7.6c)。
金融计量经济第五讲虚拟变量模型和Probit、Logit模型

精品课件
原始模型:
YX (5.8)
• 其中Y为观测值取1和0的虚拟被解释变量,X为 解释变量。
• 模型的样本形式: yi Xii
(5.9)
• 因为E(i)0
,E所(y以i)Xi
• 令: p i P ( y i 1 ) 1 p i P ( y i 0 )
• 于是有: E ( y i) 1 P ( y i 1 ) 0 P ( y i 0 ) p i
其它季度
1, 三季度
D3
0,
其它季度
• 小心“虚拟变量陷阱”!
精品课件
三、虚拟变量的应用
• 1、在常数项引入虚拟变量,改变截距。
y i0D 1 x 1 i kx k iu i (5.1)
• 对上式作OLS,得到参数估计值和回归模型:
y ˆiˆ0ˆD ˆ1 x 1 i ˆkx ki(5.2)
金融计量经济第五讲
虚拟变量模型和Probit、Logit模 型
精品课件
第一节 虚拟变量的一般应用
一、虚拟变量及其作用 1.定义:取值为0和1的人工变量,表示非量化
(定性)因素对模型的影响,一般用符号D表 示。例如:政策因素、地区因素、心理因素、 季节因素等。 2.作用: ⑴描述和测量定性因素的影响; ⑵正确反映经济变量之间的相互关系,提高模型 的精度; ⑶便于处理异常数据。
yˆt ˆ ˆxt yˆt ˆ ˆxt ˆ2 yˆt ˆ ˆxt ˆ3 yˆt ˆ ˆxt ˆ4
精品课件
一季度 二季度 三季度 四季度
例题:美国制造业的利润—销售额行为
• 模型:利 t 1 润 2 D 2 t 3 D 3 t 4 D 4 t ( 销 ) t u t售
0.503543 0.500354 1.13E+03 1.99E+09 -13241.74 1.648066
原始模型:
YX (5.8)
• 其中Y为观测值取1和0的虚拟被解释变量,X为 解释变量。
• 模型的样本形式: yi Xii
(5.9)
• 因为E(i)0
,E所(y以i)Xi
• 令: p i P ( y i 1 ) 1 p i P ( y i 0 )
• 于是有: E ( y i) 1 P ( y i 1 ) 0 P ( y i 0 ) p i
其它季度
1, 三季度
D3
0,
其它季度
• 小心“虚拟变量陷阱”!
精品课件
三、虚拟变量的应用
• 1、在常数项引入虚拟变量,改变截距。
y i0D 1 x 1 i kx k iu i (5.1)
• 对上式作OLS,得到参数估计值和回归模型:
y ˆiˆ0ˆD ˆ1 x 1 i ˆkx ki(5.2)
金融计量经济第五讲
虚拟变量模型和Probit、Logit模 型
精品课件
第一节 虚拟变量的一般应用
一、虚拟变量及其作用 1.定义:取值为0和1的人工变量,表示非量化
(定性)因素对模型的影响,一般用符号D表 示。例如:政策因素、地区因素、心理因素、 季节因素等。 2.作用: ⑴描述和测量定性因素的影响; ⑵正确反映经济变量之间的相互关系,提高模型 的精度; ⑶便于处理异常数据。
yˆt ˆ ˆxt yˆt ˆ ˆxt ˆ2 yˆt ˆ ˆxt ˆ3 yˆt ˆ ˆxt ˆ4
精品课件
一季度 二季度 三季度 四季度
例题:美国制造业的利润—销售额行为
• 模型:利 t 1 润 2 D 2 t 3 D 3 t 4 D 4 t ( 销 ) t u t售
0.503543 0.500354 1.13E+03 1.99E+09 -13241.74 1.648066
计量虚拟变量模型
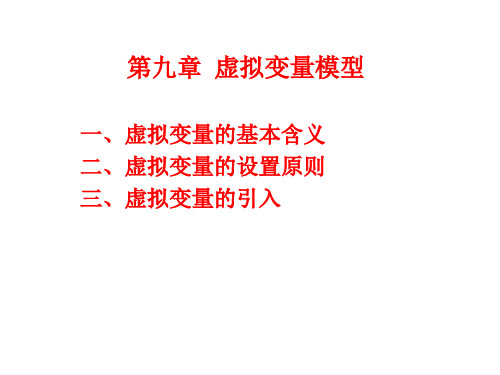
1 春季 D1t 0 其他
1 夏季 D2t 0 其他 1 D3t 0
秋季 其他
1 春季 D1t 0 其他
1 夏季 D2t 0 其他
1 D3t 0
秋季 其他
则冷饮销售量的模型为:
Yt 0 1 X 1t k X kt 1 D1t 2 D2t 3 D3t t
E(Yi | X i , D1 0, D2 0) 0 1 X i
•男职工本科以下学历的平均薪金:
E(Yi | X i , D1 1, D2 0) ( 0 2 ) 1 X i
•女职工本科以上学历的平均薪金:
E(Yi | X i , D1 0, D2 1) ( 0 3 ) 1 X i
如果只取六个观测值,其中春季与夏季取了 两次,秋、冬各取到一次观测值,则式中的:
1 1 1 ( X, D) 1 1 1 X 11 X k1 X 12 X k 2 X 13 X k 3 X 14 X k 4 X 15 X k 5 X 16 X k 6 1 0 0 0 0 1 0 0 0 0 1 0 0 0 0 1 0 1 0 0 1 0 0 0
在上述模型中,若再引入第四个虚拟变量 则冷饮销售模型变量为:
1 D4t 0
冬季 其他
Yt 0 1 X 1t k X kt 1 D1t 2 D2t 3 D3t 4 D4t t
其矩阵 形式为:
B Y ( X, D) A U
t ( 4.77) (11.73) ( 0.40) (1.13) R 0.99
2
表明:受教育水平对平 均保健支出没有影响。 收入
1 夏季 D2t 0 其他 1 D3t 0
秋季 其他
1 春季 D1t 0 其他
1 夏季 D2t 0 其他
1 D3t 0
秋季 其他
则冷饮销售量的模型为:
Yt 0 1 X 1t k X kt 1 D1t 2 D2t 3 D3t t
E(Yi | X i , D1 0, D2 0) 0 1 X i
•男职工本科以下学历的平均薪金:
E(Yi | X i , D1 1, D2 0) ( 0 2 ) 1 X i
•女职工本科以上学历的平均薪金:
E(Yi | X i , D1 0, D2 1) ( 0 3 ) 1 X i
如果只取六个观测值,其中春季与夏季取了 两次,秋、冬各取到一次观测值,则式中的:
1 1 1 ( X, D) 1 1 1 X 11 X k1 X 12 X k 2 X 13 X k 3 X 14 X k 4 X 15 X k 5 X 16 X k 6 1 0 0 0 0 1 0 0 0 0 1 0 0 0 0 1 0 1 0 0 1 0 0 0
在上述模型中,若再引入第四个虚拟变量 则冷饮销售模型变量为:
1 D4t 0
冬季 其他
Yt 0 1 X 1t k X kt 1 D1t 2 D2t 3 D3t 4 D4t t
其矩阵 形式为:
B Y ( X, D) A U
t ( 4.77) (11.73) ( 0.40) (1.13) R 0.99
2
表明:受教育水平对平 均保健支出没有影响。 收入
计量经济学虚拟变量
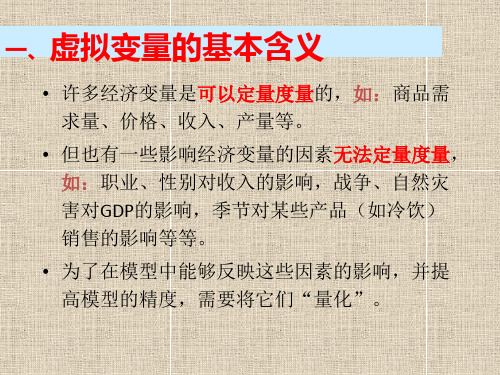
在实际分析当中,根据T检验的结 果,将不显著的季度虚拟变量从模型 中消除,用剩下的显著的虚拟变量对 模型进行估算就足够。
(2), 没有常数项的时候,可以设第4季 度的季度虚拟。
Yi 1D1 2D2 3D3 4D4 ui
(3),虚拟变量的陷阱
Yi a 1D1 2D2 3D3 4D4 ui
2,存在结果性变化。 3,需要对难以量化的数据进行处理。
• 计量经济中的虚拟变量,在明确其引入理 由基础上,被用于很多的多元回归模型。
二,虚拟变量的类型
1,临时虚拟
临时虚拟,也称为突发性虚拟。为了更好的对模型进行估算,经常需 要在回归模型中排除一些由突发性事件产生的异常值(outlier),及其对 模型的影响,例如战争,地震,内乱,罢工等。
• 第一季度到第四季度的常数项为:
第一季度:a 1
Yi (a 1) X i ui
第三季度:a 3
Yi (a 3 ) X i ui
第四季度: a
Yi a X i ui
• 现在第四季度是基准,分别表示第 四季度与各季度之差。
数虚拟变量和常数虚拟变量。
Yi a 1X i 2D ui
1 异常时期 D=
0 平时
Yi a 1Xi 2D1 3D2 ui
1
D1= 0
发生地震的年份 其他年份
1
D2= 0
发生水灾的年份 其他年份
2,定性数据的虚拟处理
学历,性别,人种等定性的差异
3,季度虚拟
(1),定义:季度虚拟是通过回归模型的常 数项的变化(斜率回归系数一定)来掌握 季度和月度等季节变化,因此,从技术角 度成为“常数项虚拟”。
这种“量化”通常是通过引入“虚拟变量” 来完成。根据这些因素的属性类型,构造只取 “0”或“1”的人工变量,通常称为虚拟变量 (dummy variables),记为D。
计量经济学课件虚拟变量
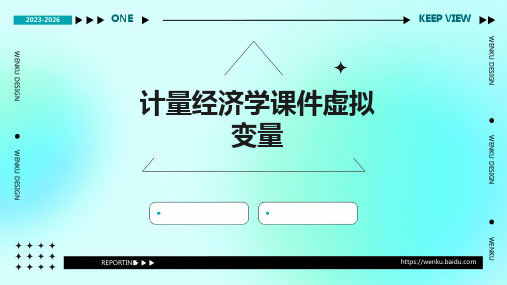
提高模型精度和预测能力
通过引入虚拟变量,可以更准确地刻画经济现象的非线性特征,从而提高计量经济学模型 的精度和预测能力。
拓展应用领域
虚拟变量的引入使得计量经济学模型能够应用于更多的领域,如金融、环境、社会等,进 一步拓展了计量经济学的应用范围。
未来研究方向和趋势
深入研究虚拟变量的理论 和方法
未来研究将进一步深入探讨虚 拟变量的理论和方法,包括虚 拟变量的选择、设定和估计方 法等,以更准确地刻画经济现 象。
https://
未来研究将积极推动虚拟变量 在交叉学科领域的应用,如环 境经济学、金融经济学等,以 促进不同学科之间的交流和合 作。
WENKU DESIGN
WENKU DESIGN
2023-2026
END
THANKS
感谢观看
KEEP VIEW
WENKU DESIGN
WENKU DESIGN
WENKU
REPORTING
要点二
虚拟变量的设置原则
在设置虚拟变量时,需要遵循完备性 和互斥性的原则。完备性要求虚拟变 量的取值能够覆盖所有可能的情况, 而互斥性则要求不同虚拟变量之间不 能存在重叠或交叉的情况。
要点三
虚拟变量的回归系数 解释
在线性回归模型中,虚拟变量的回归 系数表示该定性因素对因变量的影响 程度。当虚拟变量取值为1时,其对 应的回归系数表示该水平与参照水平 相比对因变量的影响;当虚拟变量取 值为0时,则表示该水平对因变量没 有影响。
参数估计与假设检验
参数估计
采用最小二乘法等估计方法,对引入虚拟变量后的模型进行参数估计,得到各 解释变量的系数估计值。
假设检验
根据研究问题和假设,构建相应的原假设和备择假设,通过t检验、F检验等方 法对参数进行假设检验,判断虚拟变量对模型的影响是否显著。
通过引入虚拟变量,可以更准确地刻画经济现象的非线性特征,从而提高计量经济学模型 的精度和预测能力。
拓展应用领域
虚拟变量的引入使得计量经济学模型能够应用于更多的领域,如金融、环境、社会等,进 一步拓展了计量经济学的应用范围。
未来研究方向和趋势
深入研究虚拟变量的理论 和方法
未来研究将进一步深入探讨虚 拟变量的理论和方法,包括虚 拟变量的选择、设定和估计方 法等,以更准确地刻画经济现 象。
https://
未来研究将积极推动虚拟变量 在交叉学科领域的应用,如环 境经济学、金融经济学等,以 促进不同学科之间的交流和合 作。
WENKU DESIGN
WENKU DESIGN
2023-2026
END
THANKS
感谢观看
KEEP VIEW
WENKU DESIGN
WENKU DESIGN
WENKU
REPORTING
要点二
虚拟变量的设置原则
在设置虚拟变量时,需要遵循完备性 和互斥性的原则。完备性要求虚拟变 量的取值能够覆盖所有可能的情况, 而互斥性则要求不同虚拟变量之间不 能存在重叠或交叉的情况。
要点三
虚拟变量的回归系数 解释
在线性回归模型中,虚拟变量的回归 系数表示该定性因素对因变量的影响 程度。当虚拟变量取值为1时,其对 应的回归系数表示该水平与参照水平 相比对因变量的影响;当虚拟变量取 值为0时,则表示该水平对因变量没 有影响。
参数估计与假设检验
参数估计
采用最小二乘法等估计方法,对引入虚拟变量后的模型进行参数估计,得到各 解释变量的系数估计值。
假设检验
根据研究问题和假设,构建相应的原假设和备择假设,通过t检验、F检验等方 法对参数进行假设检验,判断虚拟变量对模型的影响是否显著。
计量经济第七章虚拟变量模型课件

log
P2i P1i
21
21 X i ;
log
P3i P1i
31
31 X i ;
log
P3i P2i
32
32 X i .
其中 P1i、P2i、P3i 分别表示第 个决策者做出 第1、2、3个选择的概率。
23
Yi 0 1D1i ui ,
i 1,2, ,n.
其中 Yi
为个人月支出,
D1i
=
1,已婚 0,未婚
6
• 未婚者的月期望支出为:
E Yi | D1i 0 E 0 1 0 ui 0
• 已婚者的月期望支出为:
E Yi | D1i 1 E 0 1 1 ui 0 1
0 :未婚者的月平均支出 1 :未婚者与已婚者的月平均支出差距 0 1 :已婚者的月平均支出
Zi
f
1
Pi
ln
1
Pi Pi
ln
Pi 1 Pi
0
1
X1i
+
+k X ki
17
二、二元Logit模型估计
• 1.可重复观测数据的二元Logit模型 参数估计
• P144 【相关链接】
• 2.不可重复观测数据的二元Logit模 型参数估计
• P145 【相关链接】
18
三、模型检验与拟合优度
定义:以虚拟变量为因变量的线性回 归模型称为线性概率模型。
(linear probability model,LPM) 模型的基本形式为:
Yi 0 1X1i +2 X2i k Xki ui ,
E Yi | X 0 1X1i +2 X2i k Xki ,
i 1,2, ,n.
- 1、下载文档前请自行甄别文档内容的完整性,平台不提供额外的编辑、内容补充、找答案等附加服务。
- 2、"仅部分预览"的文档,不可在线预览部分如存在完整性等问题,可反馈申请退款(可完整预览的文档不适用该条件!)。
- 3、如文档侵犯您的权益,请联系客服反馈,我们会尽快为您处理(人工客服工作时间:9:00-18:30)。
中级计量经济学
INTERMEDIATE ECONOMETRICS
多元回归虚拟变量之二
Chapter Outline
Describing Qualitative Information A Single Dummy Independent Variable Using Dummy Variable for Multiple Categories Interactions Involving Dummy Variables A Binary Dependent Variable: The Linear Probability Model
female female educ fedu
9 October 2010
educ 1 0.0832
fedu
1 -0.085 0.9636
1
11
Example: Log hourly wage equation
What to do? Notice the wage differential between women and men is estimated when educ=0. Not interesting. More interesting would be to estimate the gender differential at, say, the average education level in the sample (about 12.5). To do this, we would replace female educ with female(educ -12.5) and rerun the regression; this only changes the coefficient on female and its standard error.
9 October 2010 5
Interacting Dummies with Other Explanatory Variables
To be more specific, we wish to model to be log(wage)=β0+ β1 educ+u for male, log(wage)=β’0+ β’1 educ+u for female, where β0 ≠ β’0 as we have already found evidence that there exists wage differential between male and female. Let β’0= β0+δ0 , β’1= β1+δ1 , then we can combine the above two equations using a dummy female: log(wage)= β0+δ0 female+(β1+δ1 female) educ+u, rearranging, log(wage)= β0+δ0 female+β1educ+δ1 female educ+u, where we observe the dummy, and the interaction of the dummy with other explanatory variables.
9 October 2010 12
Example: Effects of race on baseball player salaries
The following equation is estimated for the 330 major league baseball players for which city racial composition statistics are available. black and hispan : binary indicators for the individual players. (The base group is white players.) percblck :percentage of the team’s city that is black, perchisp is the percentage of Hispanics. Other variables measure aspects of player productivity and longevity. The interactions blackpercblck and hispanperchisp are added in to observe race effects.
9 October 2010
2
Interactions Among Dummy Variables
Interactions of dummy variables with other dummy variables and/or other explanatory variables makes the regression analysis much more powerful. We start with the interaction of a dummy with another dummy. Interactions of dummies allows another way to observe and test group difference among different groups.
9 October 2010 4
Interacting Dummies with Other Explanatory Variables
For what? To allow differences in slopes. Now suppose that we wish to test whether the return to education is the same for men and women, allowing for a constant wage differential between men and women. We need a model that allows for a constant wage differential as well as different returns to education.
9 October 2010 13
9 October 2010
8
Hypothesis testing
H0: the return to education is the same for women and men. This amounts to test H0: δ1 =0. That is, the wage differential should be the same for all levels of education level. H0:Average wages are identical for men and women who have the same levels of education. This is to test H0: δ 0=0, δ1 =0. F test can be used in this case.
9 October 2010
10
Example: Log hourly wage equation
Caution: interacting dummy with other explanatory variables can cause multicollinearity problem. Evidence 1: The standard error the dummy female is 0.036 without interaction term, it is 0.168 with it. 5 times larger. Evidence 2: the correlation matrix directly measures the degree of correlation:
9 October 2010 6
Interacting Dummies with Other Explanatory Variables
The equation log(wage)= β0+δ0 female+β1educ+δ1 female educ+u, shows that δ0 measures the difference in intercepts between women and men, and δ1 measures the difference in the return to education between women and men. After we estimate the above equation using OLS, we can perform hypothesis about return to education and wage differential. Holding other factors unchanged, we can use figures to show the impacts of different combinations of δ0 and δ1 on log(wage).
9 October 2010 9
Example: Log hourly wage equation
The wage equatiБайду номын сангаасn is estimated with tenure:
Interpretation: The estimated return to education for men in this equation is .082, or 8.2%. For women, it is .082 -.0056=.0764, or about 7.6%. The difference, .56%, or just over one-half a percentage point less for women, is not economically large nor statistically significant. No evidence against the hypothesis that the return to education is the same for men and women.
INTERMEDIATE ECONOMETRICS
多元回归虚拟变量之二
Chapter Outline
Describing Qualitative Information A Single Dummy Independent Variable Using Dummy Variable for Multiple Categories Interactions Involving Dummy Variables A Binary Dependent Variable: The Linear Probability Model
female female educ fedu
9 October 2010
educ 1 0.0832
fedu
1 -0.085 0.9636
1
11
Example: Log hourly wage equation
What to do? Notice the wage differential between women and men is estimated when educ=0. Not interesting. More interesting would be to estimate the gender differential at, say, the average education level in the sample (about 12.5). To do this, we would replace female educ with female(educ -12.5) and rerun the regression; this only changes the coefficient on female and its standard error.
9 October 2010 5
Interacting Dummies with Other Explanatory Variables
To be more specific, we wish to model to be log(wage)=β0+ β1 educ+u for male, log(wage)=β’0+ β’1 educ+u for female, where β0 ≠ β’0 as we have already found evidence that there exists wage differential between male and female. Let β’0= β0+δ0 , β’1= β1+δ1 , then we can combine the above two equations using a dummy female: log(wage)= β0+δ0 female+(β1+δ1 female) educ+u, rearranging, log(wage)= β0+δ0 female+β1educ+δ1 female educ+u, where we observe the dummy, and the interaction of the dummy with other explanatory variables.
9 October 2010 12
Example: Effects of race on baseball player salaries
The following equation is estimated for the 330 major league baseball players for which city racial composition statistics are available. black and hispan : binary indicators for the individual players. (The base group is white players.) percblck :percentage of the team’s city that is black, perchisp is the percentage of Hispanics. Other variables measure aspects of player productivity and longevity. The interactions blackpercblck and hispanperchisp are added in to observe race effects.
9 October 2010
2
Interactions Among Dummy Variables
Interactions of dummy variables with other dummy variables and/or other explanatory variables makes the regression analysis much more powerful. We start with the interaction of a dummy with another dummy. Interactions of dummies allows another way to observe and test group difference among different groups.
9 October 2010 4
Interacting Dummies with Other Explanatory Variables
For what? To allow differences in slopes. Now suppose that we wish to test whether the return to education is the same for men and women, allowing for a constant wage differential between men and women. We need a model that allows for a constant wage differential as well as different returns to education.
9 October 2010 13
9 October 2010
8
Hypothesis testing
H0: the return to education is the same for women and men. This amounts to test H0: δ1 =0. That is, the wage differential should be the same for all levels of education level. H0:Average wages are identical for men and women who have the same levels of education. This is to test H0: δ 0=0, δ1 =0. F test can be used in this case.
9 October 2010
10
Example: Log hourly wage equation
Caution: interacting dummy with other explanatory variables can cause multicollinearity problem. Evidence 1: The standard error the dummy female is 0.036 without interaction term, it is 0.168 with it. 5 times larger. Evidence 2: the correlation matrix directly measures the degree of correlation:
9 October 2010 6
Interacting Dummies with Other Explanatory Variables
The equation log(wage)= β0+δ0 female+β1educ+δ1 female educ+u, shows that δ0 measures the difference in intercepts between women and men, and δ1 measures the difference in the return to education between women and men. After we estimate the above equation using OLS, we can perform hypothesis about return to education and wage differential. Holding other factors unchanged, we can use figures to show the impacts of different combinations of δ0 and δ1 on log(wage).
9 October 2010 9
Example: Log hourly wage equation
The wage equatiБайду номын сангаасn is estimated with tenure:
Interpretation: The estimated return to education for men in this equation is .082, or 8.2%. For women, it is .082 -.0056=.0764, or about 7.6%. The difference, .56%, or just over one-half a percentage point less for women, is not economically large nor statistically significant. No evidence against the hypothesis that the return to education is the same for men and women.