Synergy in a neural code
人工智能与人脑谁更厉害英语作文
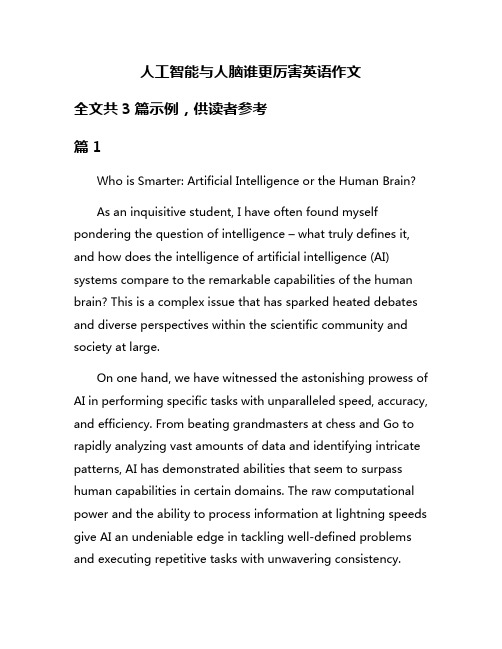
人工智能与人脑谁更厉害英语作文全文共3篇示例,供读者参考篇1Who is Smarter: Artificial Intelligence or the Human Brain?As an inquisitive student, I have often found myself pondering the question of intelligence – what truly defines it, and how does the intelligence of artificial intelligence (AI) systems compare to the remarkable capabilities of the human brain? This is a complex issue that has sparked heated debates and diverse perspectives within the scientific community and society at large.On one hand, we have witnessed the astonishing prowess of AI in performing specific tasks with unparalleled speed, accuracy, and efficiency. From beating grandmasters at chess and Go to rapidly analyzing vast amounts of data and identifying intricate patterns, AI has demonstrated abilities that seem to surpass human capabilities in certain domains. The raw computational power and the ability to process information at lightning speeds give AI an undeniable edge in tackling well-defined problems and executing repetitive tasks with unwavering consistency.However, it is important to recognize that the intelligence exhibited by AI is highly specialized and narrow. These systems excel at the tasks they are explicitly trained for, but they lack the general, flexible intelligence that humans possess. We are capable of adapting to novel situations, drawing insights from diverse experiences, and exercising creativity, emotional intelligence, and abstract reasoning – traits that are challenging for current AI systems to replicate.The human brain is an intricate and enigmatic organ, a product of millions of years of evolution. It is a marvel of complexity, with billions of interconnected neurons forming intricate networks that enable us to perceive, learn, reason, and experience the world in all its richness. Our intelligence ismulti-faceted, allowing us to navigate the nuances of social interactions, appreciate artistic expressions, and contemplate the profound mysteries of existence.Moreover, the human brain possesses a remarkable ability to learn and adapt continuously, seamlessly integrating new information and experiences into our existing knowledge frameworks. We can draw upon our vast repertoire of memories, emotions, and cultural contexts to inform our decisions and shape our understanding of the world around us. This flexibilityand capacity for lifelong learning are traits that AI systems have yet to fully emulate.Additionally, the human brain is not merely a computational machine; it is intimately intertwined with our consciousness, self-awareness, and sense of identity. Our intelligence is deeply rooted in our subjective experiences, values, and emotions –elements that are challenging to replicate in artificial systems, which operate based on predefined algorithms and data models.Yet, it would be naive to dismiss the incredible potential of AI or to underestimate its rapid advancement. As our understanding of neural networks, machine learning, and cognitive architectures deepens, we may witness AI systems that can more closely mimic or even surpass specific aspects of human intelligence. The development of artificial general intelligence (AGI), which aims to create AI systems with broad, flexible intelligence akin to human cognition, is an ambitious goal that could reshape our perceptions of intelligence altogether.Furthermore, the synergy between AI and human intelligence holds immense promise. AI can augment and amplify our capabilities, acting as powerful tools and assistants in fields ranging from scientific research to medical diagnosis tocreative endeavors. By offloading computational tasks and data analysis to AI systems, we can focus our cognitive resources on higher-order thinking, problem-solving, and decision-making –areas where human intelligence truly shines.Ultimately, the question of whether AI or the human brain is "smarter" may be misguided. Intelligence is multifaceted, and each form of intelligence excels in distinct domains. Rather than engaging in a zero-sum competition, we should strive for a symbiotic relationship where AI and human intelligence complement and enhance one another, leveraging their respective strengths to tackle complex challenges and unlock new frontiers of knowledge and understanding.As we navigate this rapidly evolving landscape, it is crucial to approach the development and application of AI with ethical considerations, transparency, and a deep respect for human values and well-being. While AI may outperform us in certain tasks, it is our moral compass, empathy, and ability to contemplate the existential and philosophical questions of life that truly define our humanity.In the end, the human brain remains an awe-inspiring marvel, a product of billions of years of evolution and a testament to the incredible complexity of the natural world. It is a wellspring ofcreativity, emotion, and consciousness – qualities that imbue our existence with meaning and richness. As we continue to push the boundaries of AI, let us not lose sight of the intrinsic value and uniqueness of human intelligence, for it is this very quality that allows us to ponder the nature of intelligence itself.篇2Which is Better: Artificial Intelligence or the Human Brain?As technology continues to advance at a breakneck pace, the debate over whether artificial intelligence (AI) or the human brain reigns supreme is becoming increasingly heated. On one side, proponents of AI tout its lightning-fast processing power, vast storage capacity, and unwavering consistency. On the other, defenders of the human mind point to our unmatched creativity, emotional intelligence, and ability to adapt to novel situations. As a student grappling with this complex issue, I find merits and flaws in both arguments.Let's start with AI's strengths. From a purely computational standpoint, even the most advanced AI systems today utterly dwarf the human brain's raw processing capabilities. While our gray matter operates at a sluggish 200 calculations per second, cutting-edge AI chips can perform a staggering 1 quintillion(1,000,000,000,000,000,000) calculations in the same timeframe. This blistering speed allows AI to crunch through vast datasets, identify intricate patterns, and arrive at insights that would take humans years, if not lifetimes, to uncover.Moreover, AI's storage capacity is virtually limitless. The human brain, a biological marvel though it may be, can only store around 2.5 petabytes of data – enough to store around 3 million hours of TV shows. In contrast, cloud-based AI systems can tap into essentially infinite storage, allowing them to maintain constantly updating databases on every conceivable topic. Need to know the latest COVID case rates across the globe? The nutritional value of an obscure Amazonian fruit? AI has that information on hand.Consistency is another AI strong suit. While human analysts, no matter how skilled, inevitably suffer from lapses in concentration, personal biases, and emotional vagaries, AI operates with robotic impartiality. Whether considering abillion-row spreadsheet or life-and-death medical diagnosis, AI will apply the same unwavering logic, free from the distortions of human whims and frailities. This consistency is a boon in fields where errors can prove catastrophic, such as aviation, nuclear power, and brain surgery.So with such a commanding lead in raw horsepower, storage, and consistency, does this mean AI will inevitably render the human mind obsolete? Not so fast. For while AI may outmuscle us in certain domains, the human brain retains critical advantages of its own – advantages rooted in our unique evolutionary heritage.Chief among these is creativity. While today's AI systems excel at optimizing within given parameters, they struggle to make the intuitive conceptual leaps that underlie true innovation. AI can incrementally improve an existing product or fine-tune an established process, but the human mind retains a scrambler-jet ability to blend disparate ideas into something entirely new. From the first stone tools to the dishwasher to the smartphone, all groundbreaking inventions have sprung from the primordial soup of human imagination – a faculty AI has yet to replicate.Emotional intelligence represents another bastion of human primacy. While AI can now mimic certain emotional responses, genuine self-awareness and the ability to empathize on a deep level remain strictly human provinces. A therapist, a kindergarten teacher, a war-time leader – these roles all require nuanced social understandings that even the most advanced AI struggles to match. Our lived experiences shape how we relate to others;we grasp context, subtext, and the vagaries of social interaction in ways that code alone cannot (yet) emulate.Perhaps most critically, the human brain maintains an unparalleled ability to contextualize and adapt. AI operates based on predetermined rules and training data – helpful for tackling defined problems, but ill-suited for true open-ended reasoning or quickly adjusting to dynamic, ambiguous circumstances. Humans, by contrast, can seamlessly blend logic and intuition to navigate novel situations. We don't just optimize; we can fundamentally reframe the problem itself in creative ways. This cognitive flexibility has allowed our species to spread across every continent and master wildly divergent environments, from scorching deserts to Arctic tundra.Ultimately, while AI will likely continue outpacing human computing power, I believe the two possess complementary strengths that will drive mutual development. In the near future, AI's sheer brute force may be best suited for automating rote tasks and handling vast data flows, while humans providehigh-level strategic oversight. We'll team up on everything from scientific research to marketing analytics, with AI crunching the numbers while humans weave the insights into real-world stories and solutions.Over the longer term though, the road ahead remains hazy. Some foresee an "intelligence explosion" where AI bootstraps itself to superintelligence within the century, becoming a universal problem-solver that far outstrips human cognition. Others insist the brain's analogical reasoning and improvisational skills will be impossible for rigid code to ever authentically replicate. As with many revolutionary technologies, the reality will likely prove more complex and nuanced than either utopians or doomsayers predict.As a student, I'm excited to bear witness to this unfolding AI revolution, even as I grapple with its ethical ramifications around privacy, security, and the future of work. And who knows –perhaps one day I'll witness an AI that can finally match the human mind's infinite creative spark. Either way, our species has a strange new coevolutionary partner, one whose full implications remain tantalizing and deeply unclear. The game is on to determine which form of intelligence will remain primus inter pares – first among equals.篇3Who is Smarter: Artificial Intelligence or the Human Brain?As technology continues to advance at a blistering pace, the debate over whether artificial intelligence (AI) will eventually surpass human intelligence has become a hotly contested topic. On one side, AI enthusiasts believe that machines will inevitably outperform the human brain in nearly every cognitive domain. On the other hand, skeptics argue that the human mind's complexity and creativity are unmatched, rendering AI inferior. As a student fascinated by this subject, I find myself torn between these two viewpoints, recognizing the remarkable capabilities of AI while also appreciating the extraordinary depth of the human intellect.To begin, it's crucial to acknowledge the astonishing progress that AI has made in recent years. From chess engines that can outmaneuver grandmasters to language models that can generate coherent and contextually appropriate text, AI has proven its prowess in domains once thought to be exclusive to human intelligence. The ability of AI systems to process vast amounts of data, identify patterns, and make accurate predictions has revolutionized fields like healthcare, finance, and scientific research.Moreover, AI's computational power and lack of biological constraints give it a significant advantage in certain tasks. Forinstance, AI can perform complex calculations and simulations at speeds far exceeding human capabilities, making it invaluable in areas such as weather forecasting, climate modeling, and cryptography. Additionally, AI's tireless nature and immunity to cognitive biases make it well-suited for tasks that require sustained attention and objectivity, such as monitoring systems or analyzing large datasets.However, despite these impressive feats, the human brain remains unparalleled in its ability to navigate the complexities of the real world. Our capacity for contextual understanding, emotional intelligence, and creative problem-solving sets us apart from even the most advanced AI systems. While AI excels at narrowly defined tasks within constrained environments, humans possess a remarkable adaptability that allows us to navigate ambiguity, think abstractly, and find innovative solutions to novel challenges.Furthermore, the human brain's ability to learn and generalize from limited data is truly remarkable. Unlike AI, which requires vast amounts of training data to perform well, humans can quickly grasp new concepts and apply them in diverse contexts. This flexibility is a byproduct of our evolutionarilyhoned cognitive abilities, which have enabled us to thrive in a constantly changing world.Moreover, the study of human intelligence has revealed the intricate interplay between cognition, emotion, and consciousness – aspects that are still poorly understood and challenging to replicate in AI systems. Our emotions shape our decision-making processes, motivations, and interpersonal interactions in ways that are difficult to capture through pure computation. Similarly, the subjective experience of consciousness, with its rich inner world of thoughts, feelings, and sensations, remains a profound mystery that has eluded even the most sophisticated AI models.It's also important to consider the ethical implications of pursuing AI that surpasses human intelligence. While the potential benefits of such technology are vast, ranging from solving global challenges to advancing our understanding of the universe, the risks are equally significant. An AI system that exceeds human intelligence could potentially become uncontrollable, posing existential threats to humanity if its goals and values diverge from our own.Ultimately, the debate over whether AI or the human brain is "smarter" may be misguided. Instead, we should focus on thecomplementary strengths of each and explore ways to leverage their respective advantages for the betterment of humanity. AI's computational power and ability to process vast amounts of data could augment and enhance human decision-making processes, while human creativity, emotional intelligence, and ethical reasoning could guide the development and deployment of AI in responsible and beneficial ways.As we continue to push the boundaries of technology, it's imperative that we approach the pursuit of artificial intelligence with humility, caution, and a deep respect for the complexities of the human mind. By fostering a synergistic relationship between AI and human intelligence, we can unlock unprecedented opportunities for progress while safeguarding the unique qualities that make us human.In the end, the question of who is smarter – AI or the human brain – may be less important than how we can harness the strengths of both to create a future that benefits all of humanity. As a student passionate about this field, I am excited to witness and contribute to the ongoing dialogue and exploration of this fascinating intersection between technology and the human experience.。
关于人工智能思考的英语作文
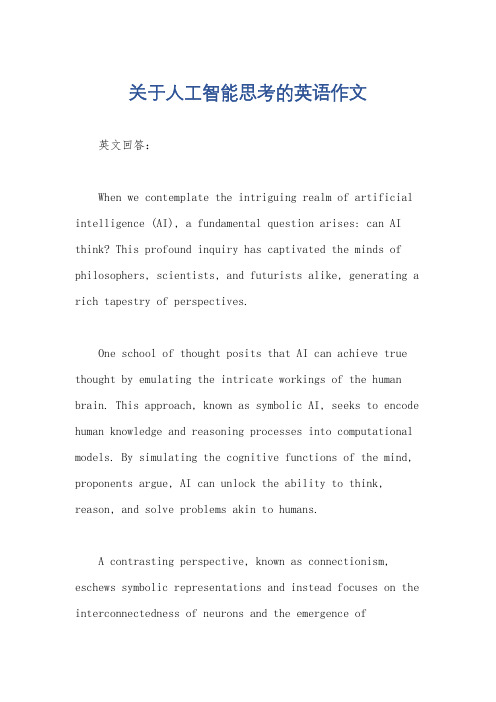
关于人工智能思考的英语作文英文回答:When we contemplate the intriguing realm of artificial intelligence (AI), a fundamental question arises: can AI think? This profound inquiry has captivated the minds of philosophers, scientists, and futurists alike, generating a rich tapestry of perspectives.One school of thought posits that AI can achieve true thought by emulating the intricate workings of the human brain. This approach, known as symbolic AI, seeks to encode human knowledge and reasoning processes into computational models. By simulating the cognitive functions of the mind, proponents argue, AI can unlock the ability to think, reason, and solve problems akin to humans.A contrasting perspective, known as connectionism, eschews symbolic representations and instead focuses on the interconnectedness of neurons and the emergence ofintelligent behavior from complex networks. This approach, inspired by biological neural systems, posits that thought and consciousness arise from the collective activity of vast numbers of nodes and connections within an artificial neural network.Yet another framework, termed embodied AI, emphasizes the role of physical interaction and embodiment in shaping thought. This perspective contends that intelligence is inextricably linked to the body and its experiences in the real world. By grounding AI systems in physical environments, proponents argue, we can foster a more naturalistic and intuitive form of thought.Beyond these overarching approaches, ongoing research in natural language processing (NLP) and machine learning (ML) is contributing to the development of AI systems that can engage in sophisticated dialogue, understand complex texts, and make predictions based on vast data sets. These advancements are gradually expanding the cognitive capabilities of AI, bringing us closer to the possibility of artificial thought.However, it is essential to recognize the limitations of current AI systems. While they may excel at performing specific tasks, they still lack the comprehensive understanding, self-awareness, and creativity that characterize human thought. The development of truly thinking machines remains a distant horizon, requiring significant breakthroughs in our understanding of consciousness, cognition, and embodiment.中文回答:人工智能是否能够思考?人工智能领域的核心问题之一就是人工智能是否能够思考。
医学英语构词法
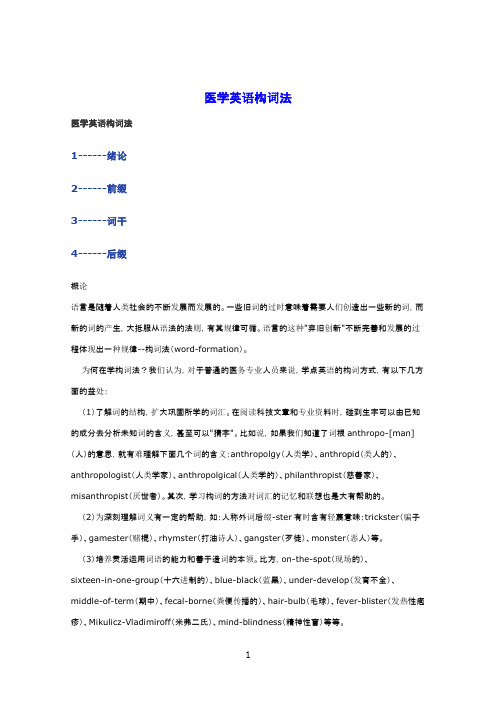
医学英语构词法医学英语构词法1------绪论2------前缀3------词干4------后缀概论语言是随着人类社会的不断发展而发展的。
一些旧词的过时意味着需要人们创造出一些新的词,而新的词的产生,大抵服从语法的法则,有其规律可循。
语言的这种"弃旧创新"不断完善和发展的过程体现出一种规律--构词法(word-formation)。
为何在学构词法?我们认为,对于普通的医务专业人员来说,学点英语的构词方式,有以下几方面的益处:(1)了解词的结构,扩大巩固所学的词汇。
在阅读科技文章和专业资料时,碰到生字可以由已知的成分去分析未知词的含义,甚至可以"猜字"。
比如说,如果我们知道了词根anthropo-[man](人)的意思,就有难理解下面几个词的含义:anthropolgy(人类学)、anthropid(类人的)、anthropologist(人类学家)、anthropolgical(人类学的)、philanthropist(慈善家)、misanthropist(厌世者)。
其次,学习构词的方法对词汇的记忆和联想也是大有帮助的。
(2)为深刻理解词义有一定的帮助,如:人称外词后缀-ster有时含有轻蔑意味:trickster(骗子手)、gamester(赌棍)、rhymster(打油诗人)、gangster(歹徒)、monster(恶人)等。
(3)培养灵活运用词语的能力和善于造词的本领。
比方,on-the-spot(现场的)、sixteen-in-one-group(十六进制的)、blue-black(蓝黑)、under-develop(发育不全)、middle-of-term(期中)、fecal-borne(粪便传播的)、hair-bulb(毛球)、fever-blister(发热性疱疹)、Mikulicz-Vladimiroff(米弗二氏)、mind-blindness(精神性盲)等等。
口诀

人体八种必须氨基酸(第一种较为顺口)1.“一两色素本来淡些”(异亮氨酸、亮氨酸、色氨酸、苏氨酸、苯丙氨酸、赖氨酸、蛋氨酸、缬氨酸)。
2.“写一本胆量色素来”(缬氨酸、异亮氨酸、苯丙氨酸、蛋氨酸、亮氨酸、色氨酸、苏氨酸、赖氨酸)。
3.鸡旦酥,晾(亮)一晾(异亮),本色赖。
借来一两本淡色书生糖、生酮、生糖兼生酮氨基酸:>生酮+生糖兼生酮=“一两色素本来老”(异亮氨酸、亮氨酸、色氨酸、苏氨酸、苯丙氨酸、赖氨酸、酪氨酸),其中生酮氨基酸为“亮赖”;除了这7个氨基酸外,其余均为生糖氨基酸。
酸性氨基酸:天谷酸——天上的谷子很酸,(天冬氨酸、谷氨酸)碱性氨基酸:赖精组芳香族氨基酸在280nm处有最大吸收峰色老笨---只可意会不可言传.一碳单位的来源肝胆阻塞死(甘氨酸、蛋氨酸、组氨酸、色氨酸、丝氨酸)。
<FONT>酶的竞争性抑制作用按事物发生的条件、发展、结果分层次记忆:1.“竞争”需要双方——底物与抑制剂之间;2.为什么能发生“竞争”——二者结构相似;3.“竞争的焦点”——酶的活性中心;4.“抑制剂占据酶活性中心”——酶活性受抑。
糖醛酸,合成维生素C的酶古龙唐僧(的)内子(爱)养画眉(古洛糖酸内酯氧化酶)双螺旋结构的特点:右双螺旋,反向平行碱基互补,氢键维系主链在外,碱基在内维生素A总结V.A视黄醇或醛,多种异构分顺反。
萝卜蔬菜多益善,因其含有V.A原。
主要影响暗视觉,缺乏夜盲看不见,还使上皮不健全,得上干眼易感染。
促进发育抗氧化,氧压低时更明显。
DNA双螺旋结构:DNA,双螺旋,正反向,互补链。
A对T,GC连,配对时,*氢键,,十碱基,转一圈,螺距34点中间。
碱基力和氢键,维持螺旋结构坚。
(AT2,GC3是指之间二个氢键GC间三个.螺距34点中间即3.4)RNA和DNA的对比如下:两种核酸有异同,腺鸟胞磷能共用。
RNA中为核糖,DNA中含有胸。
维生素B6B6兄弟三,吡哆醛、醇、胺。
他们的磷酸物,脱羧又转氨。
人工智能会使大脑退化吗专四英语作文
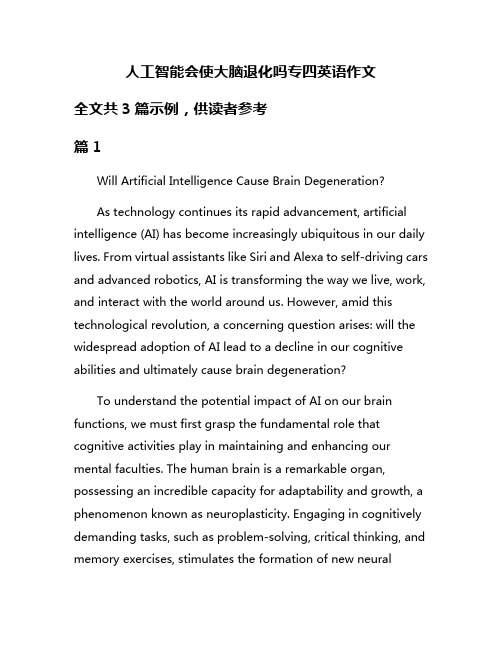
人工智能会使大脑退化吗专四英语作文全文共3篇示例,供读者参考篇1Will Artificial Intelligence Cause Brain Degeneration?As technology continues its rapid advancement, artificial intelligence (AI) has become increasingly ubiquitous in our daily lives. From virtual assistants like Siri and Alexa to self-driving cars and advanced robotics, AI is transforming the way we live, work, and interact with the world around us. However, amid this technological revolution, a concerning question arises: will the widespread adoption of AI lead to a decline in our cognitive abilities and ultimately cause brain degeneration?To understand the potential impact of AI on our brain functions, we must first grasp the fundamental role that cognitive activities play in maintaining and enhancing our mental faculties. The human brain is a remarkable organ, possessing an incredible capacity for adaptability and growth, a phenomenon known as neuroplasticity. Engaging in cognitively demanding tasks, such as problem-solving, critical thinking, and memory exercises, stimulates the formation of new neuralconnections and strengthens existing ones, thereby enhancing our cognitive abilities.With the advent of AI, there is a growing concern that we may become overly reliant on these advanced systems, leading to a reduction in the cognitive demands placed on our brains. As AI algorithms become increasingly adept at performing tasks that were once exclusively within the realm of human intelligence, we may find ourselves outsourcing more and more cognitive functions to these systems, potentially resulting in a lack of mental stimulation and ultimately, brain degeneration.One area of particular concern is memory. AI-powered virtual assistants and search engines have made it easier than ever to access and retrieve information, reducing the need for us to actively engage in memorization and recall. While this convenience is undeniably beneficial in many aspects of our lives, it may also lead to a decline in our ability to store and retrieve information from our own memory banks, potentially weakening the neural pathways associated with these processes.Additionally, AI-driven automation and decision-making systems could potentially diminish our problem-solving and critical thinking skills. As we increasingly rely on these systems to handle complex tasks and make decisions for us, we maybecome complacent and fail to exercise the cognitive processes that were once essential for navigating challenges and solving problems.However, it is important to note that the impact of AI on our brain functions is not necessarily a one-way street. Just as AI could potentially lead to cognitive decline, it could also serve as a powerful tool for enhancing our mental abilities. For instance, AI-powered educational technologies and brain-training applications could provide personalized and adaptive learning experiences, challenging our minds in novel ways and stimulating the growth of new neural connections.Moreover, the integration of AI into fields such as neuroscience and cognitive research could lead to groundbreaking discoveries and advancements in our understanding of the human brain, potentially enabling us to develop more effective strategies for maintaining and enhancing our cognitive abilities.Ultimately, the question of whether AI will cause brain degeneration is a complex one, with arguments on both sides. While the potential risks of cognitive decline due to over-reliance on AI cannot be ignored, it is essential to approach this issue with a balanced perspective. By actively engaging with AItechnologies in a mindful and responsible manner, we can harness their potential benefits while mitigating potential negative impacts on our cognitive abilities.One way to strike this balance is through education and awareness. Incorporating AI literacy into curricula at all levels of education can equip individuals with the knowledge and skills necessary to navigate the AI-driven world while maintaining a healthy balance between technology and cognitive engagement. This could involve teaching critical thinking and problem-solving strategies, encouraging active learning and memorization techniques, and promoting a deeper understanding of the principles underlying AI systems.Furthermore, fostering a culture of lifelong learning and cognitive enrichment can help counteract the potential negative effects of AI on our brain functions. Engaging in activities that challenge our minds, such as reading, puzzles, learning new skills, and participating in intellectually stimulating hobbies, can help maintain and strengthen our cognitive abilities, regardless of our reliance on AI technologies.In addition, it is imperative that we approach the development and implementation of AI systems with a strong emphasis on ethical considerations and human-centric designprinciples. By ensuring that AI technologies are developed and deployed in a manner that respects human agency and autonomy, we can mitigate the risk of excessive dependence and cognitive complacency.As we navigate this era of unprecedented technological advancement, it is crucial that we remain vigilant and proactive in safeguarding our cognitive abilities. While AI undoubtedly holds immense potential for enhancing various aspects of our lives, we must not lose sight of the fundamental importance of maintaining and nurturing our innate human intelligence.In conclusion, the relationship between AI and brain degeneration is a complex and multifaceted issue that requires careful consideration and a balanced approach. While the risks of cognitive decline due to over-reliance on AI should not be dismissed, the potential benefits of AI in enhancing our cognitive abilities and advancing our understanding of the human brain should also be recognized. By embracing a mindful and responsible integration of AI into our lives, fostering a culture of lifelong learning, and prioritizing ethical and human-centric design principles, we can harness the power of AI while safeguarding the integrity and vitality of our cognitive abilities.篇2Will AI Cause Brain Degeneration?The rapid development of artificial intelligence (AI) has sparked concerns about its potential impact on human cognition. Some argue that our reliance on AI technologies could lead to a decline in mental capacities, ultimately causing our brains to degenerate. As a student facing the challenges of the modern world, I find this topic particularly relevant and worth exploring.On one hand, the convenience and efficiency offered by AI technologies are undeniable. From voice assistants that can answer our queries to recommendation algorithms that curate personalized content, AI has seamlessly integrated into our daily lives. The ability to outsource tasks to these intelligent systems could potentially reduce the cognitive load on our brains, allowing us to conserve mental energy for more demanding endeavors.However, there is a valid concern that this reliance on AI could lead to a gradual atrophy of our cognitive abilities. Just as muscles can weaken from lack of use, our brains may lose their sharpness if we become too dependent on AI for tasks that traditionally challenged our problem-solving and critical thinking skills.One area where this potential degeneration could manifest is in our ability to navigate and orient ourselves in physical spaces. With the advent of GPS and navigation apps, many of us have become accustomed to relying on these technologies to guide us through unfamiliar territories. While convenient, this dependence could potentially weaken our innate sense of direction and spatial awareness, skills that our ancestors had to hone for survival.Similarly, the proliferation of search engines and information retrieval systems has made it easier than ever to access vast amounts of knowledge at our fingertips. While this accessibility is undoubtedly valuable, it could also diminish our motivation to commit information to memory. The ability to instantly look up facts and figures may lead to a decline in our capacity for memorization and recall, cognitive functions that were once essential for academic and professional success.Moreover, the algorithms behind many AI systems are designed to cater to our preferences and biases, creating personalized echo chambers that reinforce our existing beliefs and perspectives. This could potentially stunt the development of critical thinking skills, as we become less exposed to diverse viewpoints and challenging ideas that would traditionallyprompt us to question our assumptions and broaden our perspectives.On the other hand, proponents of AI argue that these technologies can actually enhance our cognitive abilities if utilized correctly. For instance, AI-powered educational tools can provide personalized learning experiences tailored to individual strengths and weaknesses, potentially improving our ability to acquire and retain knowledge more effectively.Additionally, the automation of routine and repetitive tasks could free up mental resources for more complex and creative endeavors, allowing us to focus our cognitive capacities on higher-order thinking and problem-solving. AI systems could also augment our decision-making processes by providing data-driven insights and analyses, potentially mitigating the effects of cognitive biases and emotional influences.Ultimately, the impact of AI on our cognitive abilities will likely depend on how we choose to integrate these technologies into our lives. If we become overly reliant on AI as a crutch, outsourcing tasks that would traditionally challenge and exercise our brains, then there is a risk of cognitive degeneration. However, if we approach AI as a tool to augment and enhance our existing abilities, leveraging its strengths while activelyengaging our own cognitive faculties, then it could potentially amplify our mental capacities.As a student navigating the complexities of the modern world, I believe it is essential to strike a balance between embracing the conveniences of AI and maintaining a commitment to exercising our cognitive abilities. We should remain vigilant about the potential risks of over-reliance on these technologies and actively seek opportunities to challenge our minds through activities that promote problem-solving, critical thinking, and lifelong learning.Furthermore, educational institutions and policymakers should prioritize the development of curricula and programs that foster cognitive resilience in the face of technological advancements. This could involve incorporating activities that strengthen skills such as memorization, spatial awareness, and analytical reasoning, while also promoting digital literacy and responsible use of AI technologies.In conclusion, the question of whether AI will cause brain degeneration is a complex one with valid arguments on both sides. While the convenience and efficiency of AI technologies are undeniable, we must remain mindful of the potential risks of over-reliance and strive to maintain a balance betweenleveraging these tools and actively exercising our cognitive abilities. By adopting a thoughtful and measured approach to AI integration, we can harness its potential while preserving and enhancing our mental capacities for generations to come.篇3Will AI Cause Brain Degeneration?The rapid advancement of artificial intelligence (AI) technology has ignited a heated debate about its potential impact on human cognitive abilities. As AI systems become increasingly sophisticated, capable of performing tasks that were once solely within the human domain, concerns have arisen that our reliance on these technologies may lead to a decline in our brain's capabilities. In this essay, I will explore the arguments on both sides of this contentious issue and ultimately conclude that while AI does present some risks, it is unlikely to cause widespread brain degeneration if used judiciously.On one hand, proponents of the brain degeneration hypothesis argue that our growing dependence on AI could result in a phenomenon akin to the atrophy of unused muscles. Just as our physical muscles weaken when we become sedentary, the cognitive muscles of our brains may deteriorate if we offloadtoo many mental tasks onto AI systems. They contend that by outsourcing cognitive functions like memory, problem-solving, and decision-making to AI, we may lose the ability to exercise and maintain these crucial mental faculties.This line of reasoning is bolstered by numerous examples of how technology has already impacted our cognitive abilities. The advent of calculators and spell-checkers, for instance, has arguably diminished our ability to perform mental arithmetic and spelling tasks. Similarly, the ubiquity of GPS navigation systems has reduced our reliance on mental mapping and spatial reasoning skills. Proponents argue that AI poses an even greater threat, as it has the potential to automate increasingly complex cognitive tasks, leaving our brains underutilized and susceptible to degeneration.Moreover, there are concerns that the convenience and efficiency offered by AI could foster a culture of intellectual laziness and complacency. If we become accustomed to relying on AI for mental tasks, we may lose the motivation to challenge ourselves and develop our cognitive abilities, leading to a gradual erosion of our mental capabilities.On the other hand, opponents of the brain degeneration hypothesis argue that AI is a tool, and like any tool, its impact onour cognitive abilities depends on how we choose to use it. They contend that AI has the potential to augment and enhance our mental capabilities rather than diminish them. By offloading routine and tedious tasks to AI systems, we can free up cognitive resources to focus on higher-order thinking, creativity, and problem-solving.Furthermore, AI can serve as a powerful educational tool, providing personalized learning experiences and adaptive curricula tailored to individual needs and learning styles. This could potentially enhance our cognitive development and foster a deeper understanding of complex concepts, rather than promoting intellectual atrophy.Opponents also argue that the human brain is remarkably plastic and adaptable, capable of reorganizing and rewiring itself in response to new challenges and experiences. As we interact with AI systems, our brains may develop new cognitive pathways and strategies to integrate and leverage these technologies effectively. This process of cognitive adaptation could ultimately strengthen and diversify our mental capabilities, rather than causing degeneration.Additionally, the development of AI is not a one-way street; it is a collaborative process that requires human intelligence andoversight. By actively engaging with AI systems, we can continually challenge ourselves to understand and improve these technologies, fostering a symbiotic relationship that stimulates cognitive growth and innovation.In my opinion, the truth likely lies somewhere between these two extremes. While the potential for brain degeneration due to excessive reliance on AI cannot be dismissed entirely, it is unlikely to occur on a widespread scale if we adopt a balanced and judicious approach to integrating AI into our lives.The key lies in striking a careful balance between leveraging the benefits of AI while still engaging in activities that exercise and stimulate our cognitive faculties. Rather than outsourcing all mental tasks to AI, we should selectively offload routine and repetitive tasks, freeing up mental resources to focus on more complex and creative endeavors. This approach could potentially enhance our cognitive abilities by allowing us to concentrate on higher-order thinking and problem-solving, while still maintaining and exercising our fundamental cognitive skills.Moreover, it is crucial to foster a culture of lifelong learning and intellectual curiosity, where we continually challenge ourselves to acquire new knowledge and skills, both with and without the aid of AI. By embracing a growth mindset andactively seeking opportunities for cognitive enrichment, we can counteract the potential risks of complacency and intellectual laziness.Education and awareness also play a vital role in mitigating the risks of brain degeneration. By understanding the potential pitfalls of overreliance on AI, we can develop strategies and best practices for integrating these technologies in a responsible and cognitively-enriching manner. This includes promoting media literacy, critical thinking skills, and a healthy skepticism towards the outputs of AI systems, ensuring that we remain actively engaged and discerning users.In conclusion, while the potential for AI to cause brain degeneration should not be dismissed entirely, it is a risk that can be effectively mitigated through a balanced and thoughtful approach to integrating these technologies into our lives. By selectively leveraging the benefits of AI while actively engaging in cognitively-stimulating activities, fostering a culture of lifelong learning, and promoting responsible AI usage through education and awareness, we can harness the power of AI to enhance and augment our cognitive abilities, rather than diminish them.。
超级智能——大脑芯片(英文)
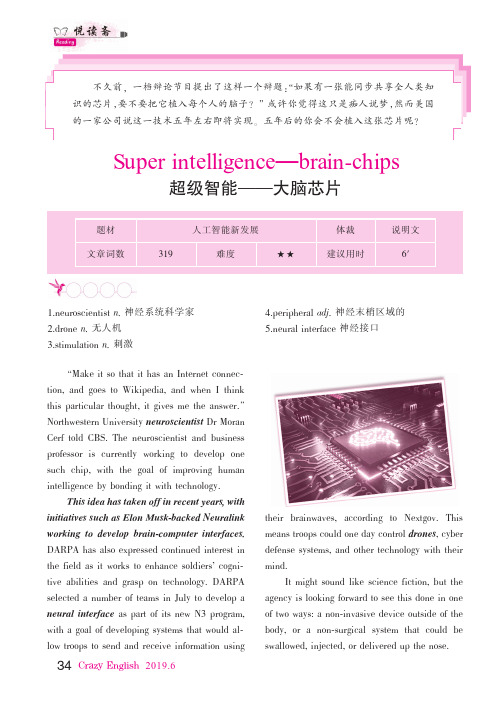
34 Crazy English 2019.6
their brainwaves, according to Nextgov. This means troops could one day control drones, cyber defense systems, and other technology with their mind.
不久前, 一档辩论节目提出了这样一个辩题:“如果有一张能同步共享全人类知 识的芯片,要不要把它植入每个人的脑子? ” 或许你觉得这只是痴人说梦,然而美国 的一家公司说这一技术五年左右即将实现。 五年后的你会不会植入这张芯片呢?
Super intelligence—brain-chips
我的奇思妙想纳米战甲作文400字

English:Title: My Ingenious Imagination: The Nanosuit ArmorIn the realm of my boundless imagination, I have conjured up a futuristic marvel: the Nanosuit Armor. A fusion of advanced nanotechnology and cutting-edge combat engineering, this suit is not merely a protective garment, but a transformative tool that amplifies its wearer's abilities to unprecedented levels.The Nanosuit Armor is composed of millions of intelligent, self-replicating nanobots, each smaller than a speck of dust. These microscopic machines work in perfect synchrony, forming a seamless exoskeleton that conforms perfectly to the user's body. Its sleek, adaptive design allows for unparalleled mobility and flexibility, ensuring that the wearer retains full range of motion despite the armor's formidable strength.At the heart of the Nanosuit lies its adaptive capabilities. The nanobots can instantaneously alter the armor's density, rendering it impervious to bullets, energy beams, or even extreme temperatures. This dynamic defense mechanism ensures that the wearer remains protected in any combat scenario. Additionally, the armor possesses regenerative properties, repairing damage sustained in battle almost instantly, further enhancing its durability.The Nanosuit's true prowess lies in its augmentation of the wearer's physical and cognitive abilities. Enhanced strength and agility modules grant superhuman feats of strength and speed, enabling the wearer to leap over tall structures, lift heavy objects with ease, or move with uncanny stealth. A built-in cloaking device, utilizing advanced nanophotonics, renders the wearer virtually invisible, providing strategic advantage in covert operations.Moreover, the Nanosuit integrates a sophisticated neural interface, allowing the wearer to control various functions with mere thoughts. This mind-machine synergy also grants access to real-time battlefield data, enhances situational awareness, and even facilitates rapid learning of new skills and languages. The suit's AI assistant, a virtual embodiment of strategic genius, offers tactical advice and supports decision-making in the heat of battle.Beyond combat applications, the Nanosuit Armor has immense potential for humanitarian and exploratory missions. Its environmental adaptability enables wearers to withstand the harshest conditions, whether traversing scorching deserts, diving into the ocean depths, or venturing into the vacuum of space. Its medical module can monitor vital signs, administer emergency treatments, and even slow the effects of aging, ensuring the wearer's health and longevity.In my imaginative universe, the Nanosuit Armor transcends the boundaries of conventional warfare and human capability. It is a symbol oftechnological prowess, a testament to the potential of merging human intellect with advanced nanotechnology. Although currently confined to the realm of fantasy, the Nanosuit embodies the relentless pursuit of innovation and the boundless possibilities that lie ahead in the realm of scientific exploration.汉语:标题:我的奇思妙想:纳米战甲在我的无垠想象空间中,我构想出一款未来科技奇迹:纳米战甲。
生物专业英语词汇-常用前缀

生物专业英语词汇 - 常用前缀第一节表示无,抗,非的前缀一.a-,an-无,非。
无可以进一步理解为离开,除去,脱去等1. 无abacteria 无菌的,atony 无力,anemia 贫血(无血之意)。
abiosis 无生命,abraehia 无臂畸形,adaerya 无泪,asaetylia 无指(趾)畸形,asendrie无树突的,adermotrophia 皮肤萎缩(即无皮肤营养之意),adiaphoresis 无汗症。
2. 否定asymmetercal 不对称的,asynergy 不协调,astnthests 不连接,aststole 心搏停止(心脏不收缩)。
atactic 共济失调的(不协调),astnchronous 不同步的,askyllabia 拼音不能。
3. 离开aspiration 吸引(即吸除之意),aberrent 迷走的(即离开正常途径的)。
4. an-在元音前用an-anaerove 厌氧菌,anesthesia 无感觉,麻醉,analgesia 无痛法,痛觉消失,anamniotic 无羊膜的,anangioplasia 血管发育不全,anapepsia 胃蛋白酶缺乏,anaplasia 发育不全,anascitia 无腹水的,anastigmatic 无散光的,anacholia 胆汁缺乏。
二,ab-去,离开,除abnormal 不正常的(即背离正常的),abapical 离尖的,离心尖的(尖以外的,心尖外的)。
abarticular 关节外的,abaxial 轴外的,离轴的,abduct 外展神经,ablactation 断奶(离开奶)。
abneural 神经外的。
三.ant-,anti-,对抗,取消,抑制,解除antagonistic 对抗的,antioxin 搞毒素,antibody 抗体,antigen 抗原,antacid 制酸剂,antipyretie 解热剂,antibiotic 抗菌素,antispasmin 解痉剂,→antiamylase 抗淀粉酶,antieoagulant 抗凝的。
- 1、下载文档前请自行甄别文档内容的完整性,平台不提供额外的编辑、内容补充、找答案等附加服务。
- 2、"仅部分预览"的文档,不可在线预览部分如存在完整性等问题,可反馈申请退款(可完整预览的文档不适用该条件!)。
- 3、如文档侵犯您的权益,请联系客服反馈,我们会尽快为您处理(人工客服工作时间:9:00-18:30)。
a rX iv:physics /99267v1[physics.bio-ph]23Feb1999Symbols and synergy in a neural code Naama Brenner,1Steven P.Strong,1,2Roland Koberle,1,3William Bialek,1and Rob R.de Ruyter van Steveninck 11NEC Research Institute,4Independence Way,Princeton,New Jersey 085402Institute for Advanced Study,Olden Lane,Princeton,New Jersey 085443Instituto di F ´isica de S˜a o Carlos,Caixa Postal 369Universidade de S˜a o Paulo,13560–970S˜a o Carlos,SP Brasil1IntroductionThroughout the nervous system,information is encoded in sequences of iden-tical action potentials or spikes.The representation of sense data by these spike trains has been studied for seventy years[1],but there remain many open questions about the structure of this code.A full understanding of the code requires that we identify its elementary symbols and that we charac-terize the messages which these symbols represent.Many different possible elementary symbols have been considered,implicitly or explicitly,in previ-ous work on neural coding.These might be the numbers of spikes in time windows offixed size,or alternatively the individual spikes themselves might be the building blocks of the code.In cells that produce bursts of action potentials,these bursts might be special symbols that convey information in addition to that carried by single spikes.Yet another possibility is that patterns of spikes—across time in one cell or across a population of cells—can have a special significance;this last possibility has received renewed attention as techniques emerge for recording the activity of many neurons simultaneously.In many methods of analysis,questions about the symbolic structure of the code are mixed with questions about what the symbols represent.Thus, in trying to characterize the feature selectivity of neurons,one often makes the a priori choice to measure the neural response as the spike count or rate in afixed window.Conversely,in trying to assess the significance of synchronous spikes from pairs of neurons,or bursts of spikes from a single neuron,one might search for a correlation between these events and some particular stimulus features.In each case conclusions about one aspect of the code are limited by assumptions about another aspect.Here we show that questions about the symbolic structure of the neural code can be separated out and answered in an information theoretic framework,using data from suitably designed experiments.This framework allows us to address directly the significance of spike patterns or other compound spiking events:How much information is carried by a compound event?Is there redundancy or synergy among the individual spikes?Are particular patterns of spikes especially informative?2Methods to assess the significance of spike patterns in the neural code share a common intuitive basis:•Patterns of spikes can play a role in representing stimuli if and only if the occurrence of patterns is linked to stimulus variations.•The patterns have a special role only if this correlation between sensory signals and patterns is not decomposable into separate correlations be-tween the signals and the pieces of the pattern,e.g.the individual spikes.We believe that these statements are not controversial.Difficulties arise when we try to quantify this intuitive picture:What is the correct measure of correlation?How much correlation is significant?Can we make statements independent of models and elaborate null hypotheses?The central claim of this paper is that many of these difficulties can be resolved by careful use of ideas from information theory.Shannon[2] proved that entropy and information provide the only measures of variability and correlation that are consistent with simple and plausible requirements. Further,while it may be unclear how to interpret,for example,a20%increase in correlation between spike trains,an extra bit of information carried by patterns of spikes means precisely that these patterns provide a factor of two increase in the ability of the system to distinguish among different sensory inputs.In this work we show see that there is a direct method of measuring the information(in bits)carried by particular patterns of spikes,independent of models for the stimulus features that these patterns might represent.In particular,we can compare the information conveyed by spikes patterns with the information conveyed by the individual spikes that make up the pattern, and determine quantitatively whether the whole is more or less than the sum of its parts.While this method allows us to compute unambiguously how much in-formation is conveyed by particular patterns,it does not tell us what this information is.Making the distinction between two questions,the structure of the code and the algorithm for translation,we only answer thefirst of these two.We believe thatfinding the structural properties of a neural code3independent of the translation algorithm is an essentialfirst step towards un-derstanding anything beyond the single spike approximation.The need for identifying the elementary symbols is especially clear when complex multi neuron codes are considered.Moreover,a quantitative measure of the in-formation carried by compound symbols will be useful in the next stage of modeling the encoding algorithm,as a control for the validity of models.2FormalismIn the framework of information theory[2]signals are generated by a source with afixed probability distribution,and encoded into messages by a channel. The coding is probabilistic,and the joint distribution of signals and coded messages determines all quantities of interest;in particular the information transmitted by the channel about the source is an average over this joint distribution.In studying a sensory system,the signals generated by the source are the stimuli presented to the animal,and the messages in the communication channel are sequences of spikes in a neuron or in a population of neurons.Both the stimuli and the spike trains are random variables,and they convey information mutually because they are correlated.The problem of quantifying this information has been discussed from several points of view [3,4,5,6].Here we address the question of how much information is carried by particular“events”or combinations of action potentials.2.1Defining information from one eventA discrete event E in the spike train is defined as a specific combination of spikes.Examples are a single spike,a pair of spikes separated by a given time, spikes from two neurons that occur in synchrony,and so rmation is carried by the occurrence of events at particular times and not others, implying that they are correlated with some stimulus features and not with others.Our task is to express this information in terms of quantities that are easily measured experimentally.In experiments,as in nature,the animal is exposed to stimuli at each instant of time.We can describe this sensory input by a function s(t′),4which may have many components to parameterize the time dependence of multiple stimulus features.In general,the information gained about s(t′)by observing a set of neural responses isI= responses Ds(t′)P[s(t′)&response]log2 P[s(t′)&response]The range of possible responses is then the range of times0<t E<T in our observation window.Alternatively,when we observe the response in a particular small time bin of size∆t,information is carried by the fact that the event E either occurs or does not.The range of possible responses then includes just two possibilities.Both of these points of view have an arbitrary element:the choice of bin size∆t and the window size T.Characterizing the properties of the system,as opposed to our observation power,requires taking the limit of high time resolution(∆t→0)and long observation times (T→∞).As will be shown in the next section,in this limit the two points of view give the same answer for the information carried by an event.2.2Information and event ratesA crucial role is played by the event rate r E(t),the probability per unit time that an event of type E occurs at time t,given the stimulus history s(t′).Empirical construction of the event rate r E(t)requires repetition of same stimulus history many times,so that a histogram can be formed(see Figure1).For the case where events are single spikes,this is the familiar time dependentfiring rate or post-stimulus time histogram(Figure1c);the generalization to other types of events is illustrated by Figs.1c and1d. Intuitively,a uniform event rate implies that no information is transmitted, whereas the presence of sharply defined features in the event rate implies that much information is transmitted by these events;see,for example,the discussion by Vaadia et al.[9].We now formalize this intuition,and show how the average information carried by a single event is related quantitatively to the time dependent event rate.Let us take thefirst point of view about the neural response variable, in which the range of responses is described by the possible arrival times t of the event.What is the probability offinding the event at a particular time t?Before we know the stimulus history s(t′),all we can say is that the event can occur anywhere in our experimental window of size T,so that the probability is uniform P(response)=P(t)=1/T,with an entropy of S[P(t)]= log2T.Once we know the stimulus,we also know the event rate r E(t), and so our uncertainty about the occurrence time of the event is reduced.6Events will occur preferentially at times where the event rate is large,so the probability distribution should be proportional to r E (t );with proper normalization P (response |s )=P (t |s )=r E (t )/(T ¯r E ).Then the conditional entropy isS [P (t |s )]=−T 0dt P (t |s )log 2P (t |s )=−1¯r E log 2 r E (t )T T 0dt r E (t )¯r E .(5)This formula expresses the information conveyed by an event of type E as an integral over time,of a quantity which depends only on the responses.There is no explicit dependence on the joint distribution of stimuli and responses;it is implicit that by integrating over time we are in fact sampling the distri-bution of stimuli,whereas by estimating the function r E (t )as a histogram we are sampling the distribution of the responses given a stimulus.We may write the information equivalently as an average over the stimulus instead of over time,I (E ;s )= r E (t )¯r E s,(6)where here the average is over all possible value of s weighted by their prob-abilities P (s ).In the second view,the neural response is a binary random variable,σE ∈{0,1},marking the occurrence or non occurrence of an event of type E in a small time bin of size ∆t .Suppose,for simplicity,that the stimulus takes on a finite set of values s with probabilities P (s ).These in turn induce7the event E with probability p E(s)=P(σE=1|s)=r E(s)∆t,with an average probability for the occurrence of the event¯p E= s P(s)r E(s)∆t=¯r E∆t. The information is the difference between the prior entropy and the condi-tional entropy:I(E;s)=S(s)− S(s|σE) ,where the conditional entropy is an average over the two possible values ofσE.The conditional probabilities are found from Bayes’rule,P(s|σE=1)=P(s)p E(s)(1−¯p E),(7)and with these onefinds the information,I(E;s)=− s P(s)log2P(s)+ σE=0,1P(s|σE)log s P(s|σE)= s P(s) p E(s)log2 p E(s)1−¯p E .(8) This expression is again an average over all stimulus values,of a property which only depends on the responses.Taking the limit∆t→0,consistent with the requirement that the event can occur at most once,onefinds the average information conveyed in a small time bin;dividing by the average probability of an event one obtains Eq.(6)as the information per event.Equation(6),and its time averaged form(5),is an exact formula which can be used in any situation where a rich stimulus history can be presented repeatedly.It enables the evaluation of the information for arbitrarily com-plex events,independent of assumptions about the encoding algorithm.Let us consider in more detail the simple case where events are single spikes.Then the average information conveyed by a single spike becomes an integral over the time dependent spike rate r(t),I(1spike;s)=1¯r log2r(t)the single particle density describes the one body statistics of a gas or liquid. Several previous works have noted this relation,and the formula(9)has an interesting history.If the spike train is a modulated Poisson process,then Eq.(9)provides an upper bound on information transmission(per spike)by the spike train as a whole[10].In studying the coding of location by cells in the rat hippocampus,Skaggs et al.[11]assumed that successive spikes carried independent information,and that the spike rate was determined by the instantaneous location,and obtained Eq.(9)with the time average re-placed by an average over locations.DeWeese[12]showed that the rate of information transmission by a spike train could be expanded in a series of integrals over correlation functions,where successive terms would be small if the number of spikes per correlation time were small;the leading term, which would be exact if spikes were uncorrelated,is Eq.(9).Panzeri et al.[13]show that Eq.(9),multiplied by the mean spike rate to give an informa-tion rate(bits/s),is the correct information rate if we count spikes in very brief segments of the neural response,which is equivalent to asking for the information carried by single spikes.For further discussion of the relation to previous work,see Appendix A.A crucial point here is the generalization to Eq.(5),and this result applies to the information content of any point events in the neural response—pairs of spikes with a certain separation,coincident spikes from two cells,...—not just single spikes.Moreover,in the analysis of experiments we will emphasize the use of this formula as an exact result for the information content of single events,rather than an approximate result for the spike train as a whole,and this approach will enable us to address questions concerning the structure of the code and the role played by various point events.3Experiments in thefly visual systemIn this section,we use our formalism to analyze experiments on the movement sensitive cell H1in the visual system of the blowfly Calliphora vicina.We address the issue of the information conveyed by pairs of spikes in this neuron, as compared to the information conveyed independently by single spikes.The quantitative results of this section—numbers for the information,effects of9synergy and redundancy among spikes—are specific to this system and to the stimulus conditions used.The theoretical approach,however,is valid generally and can be applied similarly to other experimental systems,tofind out the significance of various patterns in single cells or in a population. 3.1Synergy between spikesThe experimental setup described in Appendix B gives us control over the input and output of the H1neuron in thefly.The horizontal motion across the visualfield is the input sensory stimulus s(t),which we draw from a probability distribution P(s),and the spike train recorded from H1is the neural response.Figure1a shows a segment of the stimulus presented to the fly,and1b illustrates the response to many repeated presentations of this segment.The histogram of spike times across the ensemble of repetitions provides an estimate of the spike rate r(t)(Fig.1c),and Eq.(5)gives the information carried by a single spike,I(1spike;s)=1.53±0.05bits.Figure 2illustrates the details of how the formula was used,with an emphasis on the effects offiniteness of the data.In this experiment,a stimulus of length T=10sec was repeated350times.As seen from Figure2,a stable result could be obtained from a smaller number of repetitions.If each spike were to convey information independently,then with the mean spike rate¯r=37spikes/s,the total information rate would be R info= 56bits/s.We used the variability and reproducibility of continuous segments in the neural response[6],in order to estimate the total information rate in the spike train in this experiment,and found that R info=75bits/s.Thus, the information conveyed by the spike train as a whole is larger than the sum of contributions from individual spikes,indicating cooperative informa-tion transmission by patterns of spikes in time.This synergy among spikes motivates the search for especially informative patterns in the spike train.We consider compound events that consist of two spikes separated by a timeτ,with no constraints on what happens between them.Figure1 shows segments of the event rate rτ(t)forτ=3(±1)ms(Fig.1d),and for τ=17(±1)ms(Fig.1e).The information carried by spike pairs as a function of the interspike timeτ,computed from Eq.(5),is shown in Fig.3.For large10τspikes contribute independent information,as expected.This independence is established within∼30−40ms,comparable to the behavioral response times of thefly[14].There is a mild redundancy(∼10−20%)at intermediate separations,and a very large synergy(up to∼130%)at smallτ.Related results were obtained using the correlation of spike patterns with stimulus features[7].There the information carried by spike patterns was es-timated from the distribution of stimuli given each pattern,thus constructing a statistical model of what the patterns“stand for”(see details in Appendix A).Since the time dependent stimulus is in general of high dimensionality,its distribution cannot be sampled directly and some approximations must be made.de Ruyter van Steveninck and Bialek[7]made the approximation that patterns of a few spikes encode projections of the stimulus onto low dimen-sional subspaces,and the information carried by such patterns was evaluated only in this subspace.The informations obtained in this approximation are bounded from above by the true information carried by the patterns,as estimated directly with the methods presented here.3.2Origins of synergySynergy means,quite literally,that two spikes together tell us more than two spikes separately.Synergistic coding is often discussed for populations of cells,where extra information is conveyed by patterns of coincident spikes from several neurons[15,16,17,18],while here we see direct evidence for extra information in pairs of spikes across time.The mathematical framework for describing these effects is the same,and a natural question is:what are the conditions for synergistic coding?The average synergy Syn[E1,E2;s]between two events E1and E2is the difference between the information about the stimulus s conveyed by the pair,and the information conveyed by the two events independently,Syn[E1,E2;s]=I[E1,E2;s]−(I[E1;s]+I[E2;s]).(10) We can rewrite the synergy as:Syn[E1,E2;s]=I[E1;E2|s]−I[E1;E2].(11)11The first term is the mutual information between the events computed across an ensemble of repeated presentations of the same stimulus history.It de-scribes the gain in information due to the locking of compound event (E 1,E 2)to particular stimulus features.If events E 1and E 2are correlated individ-ually with the stimulus but not with one another,this term will be zero,and these events cannot be synergistic on average.The second term is the mutual information between events when the stimulus is not constrained,or equivalently the predictability of event E 2from E 1.This predictability lim-its the capacity of E 2to carry information beyond that already conveyed by E 1.Synergistic coding (Syn >0)thus requires that the mutual information among the spikes is increased by specifying the stimulus,which makes precise the intuitive idea of ‘stimulus dependent correlations’.Returning to our experimental example,we identify the events E 1and E 2as the arrivals of two spikes,and consider the synergy between them as a function of the time τbetween them.In terms of event rates,we compute the information carried by a pair of spikes separated by a time τ,Eq.(5),as well as the information carried by two individual spikes.The difference between these two quantities is the synergy between two spikes,which can be written as Syn(τ)=−log 2 ¯r τT T 0dt r τ(t )r (t )r (t −τ) +1¯r τ+r τ(t +τ)¯r log 2[r (t )].(12)The first term in this equation is the logarithm of the normalized correlation function,and hence measures the rarity of spike pairs with separation τ;the average of this term over τis the mutual information between events in Eq.(11).The second term is related to the local correlation function and measures the extent to which the stimulus modulates the likelihood of spike pairs.The average of this term over τgives the mutual information conditional on knowledge of the stimulus [the first term in Eq.(11)].The average of the third term over τis zero,and numerical evaluation of this term from the data shows that it is negligible at most values of τ.We thus find that the synergy between spikes is approximately a sum of two terms,whose averages over τare the terms in Eq.(11).A spike pair with12a separationτthen has two types of contributions to the extra information it carries:the two spikes can be correlated conditional on the stimulus,or the pair could be a rare and thus surprising event.The rarity of brief pairs is related to neural refractoriness,but this effect alone is insufficient to enhance information transmission;the rare events must also be related reliably to the stimulus.In fact,conditional on the stimulus,the spikes in rare pairs are strongly correlated with each other,and this is visible in Fig.1a:from trial to trial,adjacent spikes jitter together as if connected by a stiffspring. To quantify this effect,wefind for each spike in one trial the closest spike in successive trials,and measure the variance of the arrival times of these spikes.Similarly,we measure the variance of the interspike times.Figure4a shows the ratio of the interspike time variance to the sum of the arrival time variances of the spikes that make up the pair.For large separations this ratio is unity,as expected if spikes are locked independently to the stimulus,but as the two spikes come closer it falls below one quarter.Both the conditional correlation among the members of the pair(Fig. 4a)and the relative synergy(Fig.4b)depend strongly on the interspike separation.This dependence is nearly invariant to changes in image contrast, although the spike rate and other statistical properties are strongly affected by such changes.Brief spike pairs seem to retain their identity as specially informative symbols over a range of input ensembles.If particular temporal patterns are especially informative,then we would lose information if we failed to distinguish among different patterns.Thus there are two notions of time resolution for spike pairs:the time resolution with which the interspike time is defined,and the absolute time resolution with which the event is marked.Figure5shows that,for small interspike times,the information is much more sensitive to changes in the interspike time resolution(open symbols)than to the absolute time resolution(filled symbols).This is related to the slope in Figure2:in regions where the slope is large,events should be finely distinguished in order to retain the information.133.3Implications of synergyThe importance of spike timing in the neural code has been under debate for some time now.We believe that some issues in this debate can be clarified using a direct information theoretic approach.Following MacKay and Mc-Culloch[3],we know that marking spike arrival times with higher resolution provides an increased capacity for information transmission.The work of Strong et al.[6]shows that for thefly’s H1neuron,the increased capacity associated with spike timing is indeed used with nearly constant efficiency down to millisecond resolution.This efficiency can be the result of a tight locking of individual spikes to a rapidly varying stimulus,and it could also be the result of temporal patterns providing information beyond rapid rate modulations.The analysis given here shows that for H1,pairs of spikes can provide much more information than two individual spikes,information transmission is much more sensitive to the relative timing of spikes than to their absolute timing,and these synergistic effects survive averaging over all similar patterns in an experiment.On the time scales of relevance tofly behavior,the amount of synergy among spikes in H1allows this single cell to provide an extra factor of two in resolving power for distinguishing different trajectories of motion across the visualfield.4SummaryIn summary,information theory allows us to quantify the symbolic structure of a neural code independent of the rules for translating between spikes and stimuli.In particular,this approach tests directly the idea that patterns of spikes are special events in the code,carrying more information than expected by adding the contributions from individual spikes.These quantities can be measured directly from data.It is of practical importance that the formulas rely on low order statistical measures of the neural response,and hence do not require enormous data sets to reach meaningful conclusions.The method is of general validity and is applicable to patterns of spikes across a population of neurons,as well as across time.In our experiments on thefly visual system,we found that an event com-14posed of a pair of spikes can carry far more than the information carried independently by its parts.Two spikes that occur in rapid succession appear to be special symbols that have an integrity beyond the locking of individual spikes to the stimulus.This is analogous to the encoding of sounds in writ-ten English:the symbols‘th,’‘sh,’and‘ch’are each elementary and stand for sounds that are not decomposable into sounds represented by each of the constituent letters.For such pairs to act effectively as special symbols, mechanisms for‘reading’them must exist at subsequent levels of processing. Synaptic transmission is sensitive to interspike times in the2–20ms range [19],and it is natural to suggest that synaptic mechanisms on this time scale play a role in such reading.Recent work on the mammalian visual system [20]provides direct evidence that pairs of spikes close together in time can be especially efficient in driving postsynaptic neurons.AcknowledgementsWe thank G.Lewen and A.Schweitzer for their help with the experiments and N.Tishby for many helpful discussions.Work at the IAS was supported in part by DOE grant DE–FG02–90ER40542,and work at the IFSC was supported by the Brazilian agencies FAPESP and CNPq.Appendix A:Relation to previous workPatterns of spikes and their relation to sensory stimuli have been quantified in the past through the use of correlation functions.The event rates that we have defined here,which are directly connected to the information carried by patterns of spikes by Eq.(5),are in fact just properly normalized correla-tion functions.The event rate for pairs of spikes from two separate neurons is related to the joint post-stimulus time histogram defined by Aertsen and coworkers[21,9]Making this connection explicit is also an opportunity to see how the present formalism applies to events defined across two cells. Consider two cells,A and B,generating spikes at times{t A i}and{t B i}, respectively.It will be useful to think of the spike trains as sums of unit15impulses at the spike times,ρA(t)= iδ(t−t A i)(A.1)ρB(t)= iδ(t−t B i).(A.2) Then the time dependent spike rates for the two cells arer A(t)= ρA(t) trials,(A.3)r B(t)= ρB(t) trials,(A.4) where ··· trials denotes an average over multiple trials in which the same time dependent stimulus s(t′)is presented.These spike rates are the probabilities per unit time for the occurrence of a single spike in either cell A or cell B,also called the post-stimulus time histogram(PSTH).We can define the probability per unit time for a spike in cell A to occur at time t and a spike in cell B to occur at time t′,and this will be the joint post-stimulus time histogram,JPSTH AB(t,t′)= ρA(t)ρB(t′) trials.(A.5) Alternatively,we can consider an event E defined by a spike in cell A at time t and a spike in cell B at time t−τ,with the relative timeτmeasured to a precision of∆τ.Then the rate of these events isr E(t)= ∆τ/2−∆τ/2dt′JPSTH AB(t,t−τ+t′)(A.6)≈∆τJPSTH AB(t,t−τ),(A.7) where the last approximation is valid if our time resolution is sufficiently high.Applying our general formula for the information carried by single events,Eq.(5),the information carried by pairs of spikes from two cells can be written as an integral over diagonal“strips”of the JPSTH matrix,I(E;s)=1JPSTH AB(t,t−τ) t log2JPSTH AB(t,t−τ)。