EXAMPLE-BASED CORRECTION OF WORD SEGMENTATION AND PART OF SPEECH LABELLING
语言学 考研真题
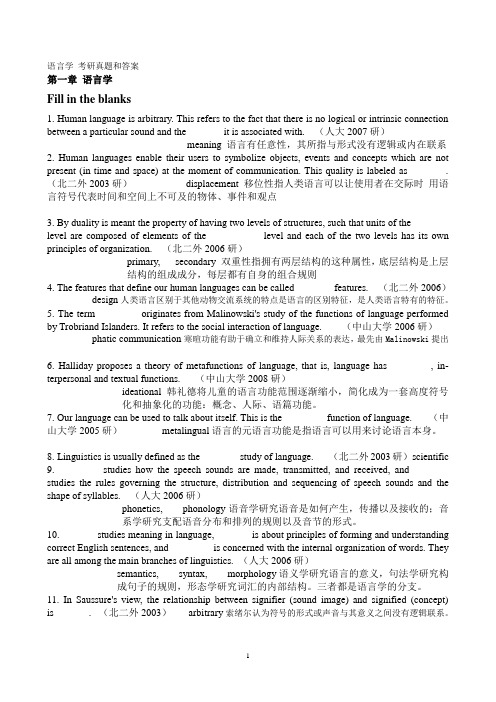
语言学考研真题和答案第一章语言学Fill in the blanks1. Human language is arbitrary. This refers to the fact that there is no logical or intrinsic connection between a particular sound and the _______it is associated with. (人大2007研)meaning 语言有任意性,其所指与形式没有逻辑或内在联系2. Human languages enable their users to symbolize objects, events and concepts which are not present (in time and space) at the moment of communication. This quality is labeled as _______. (北二外2003研)displacement 移位性指人类语言可以让使用者在交际时用语言符号代表时间和空间上不可及的物体、事件和观点3. By duality is meant the property of having two levels of structures, such that units of the _______ level are composed of elements of the __________ level and each of the two levels has its own principles of organization. (北二外2006研)primary, secondary 双重性指拥有两层结构的这种属性,底层结构是上层结构的组成成分,每层都有自身的组合规则4. The features that define our human languages can be called _______ features. (北二外2006)design人类语言区别于其他动物交流系统的特点是语言的区别特征,是人类语言特有的特征。
写作业小作文英语

Writing a short essay in English can be a straightforward task if you follow a few basic guidelines.Heres a stepbystep guide to help you compose a wellstructured essay:1.Choose a Topic:Start by selecting a topic that is interesting and relevant to the assignment.Make sure it is specific enough to be covered in the word limit you have.2.Understand the Requirements:Before you begin writing,ensure you understand the requirements of the essay.This includes the word count,format,and any specific points you need to address.3.Outline Your Essay:Create an outline to organize your thoughts.This should include: Introduction:Introduce the topic and provide some background information. Body:Break down the main points you want to discuss.Each point should have its own paragraph.Conclusion:Summarize the main points and provide a final thought or recommendation.4.Write the Introduction:Begin your essay with an engaging introduction that grabs the readers attention.This should include a thesis statement that outlines the main argument or point of your essay.5.Develop the Body:Each paragraph in the body of your essay should focus on one main idea.Start with a topic sentence,provide evidence or examples to support your point,and then explain how this evidence supports your thesis.e Transitions:Make sure your essay flows smoothly by using transition words and phrases to connect your ideas.7.Write the Conclusion:The conclusion should restate your thesis and summarize the main points of your essay.It should also provide a final thought or a call to action,if appropriate.8.Proofread and Edit:After writing your essay,take the time to proofread and edit it. Check for grammatical errors,spelling mistakes,and ensure that your essay is coherent and wellstructured.9.Follow Formatting Guidelines:Ensure that your essay follows any specific formatting guidelines provided,such as font size,line spacing,and citation style.10.Revise:Based on your proofreading,make any necessary revisions to improve theclarity,coherence,and overall quality of your essay.Heres a brief example of a short essay on the topic The Importance of Reading: Title:The Importance of ReadingIntroduction:Reading is a fundamental skill that not only enhances our knowledge but also shapes our perspectives on life.From early childhood,we are encouraged to read,but the true value of this practice extends far beyond academic achievement.Body:Firstly,reading improves our cognitive abilities.It stimulates the brain,enhancing memory and concentration.Example:Studies have shown that regular readers have better memory retention.Secondly,it broadens our horizons by exposing us to different cultures,ideas,and experiences.Example:Reading literature from various countries can provide insights into diverse ways of life.Thirdly,reading is a source of entertainment and relaxation.It allows us to escape from our daily routines and immerse ourselves in different worlds.Conclusion:In conclusion,reading is an essential activity that benefits our minds,broadens our understanding of the world,and provides a means of enjoyment.Encouraging a culture of reading can lead to a more informed and empathetic society.Remember,this is just a basic framework.Depending on the complexity of the topic and the length of the essay,you may need to delve deeper into each point or provide more examples and evidence.。
Academic Writing(学术英语写作)中国大学mooc课后章节答案期末考试题库2023年
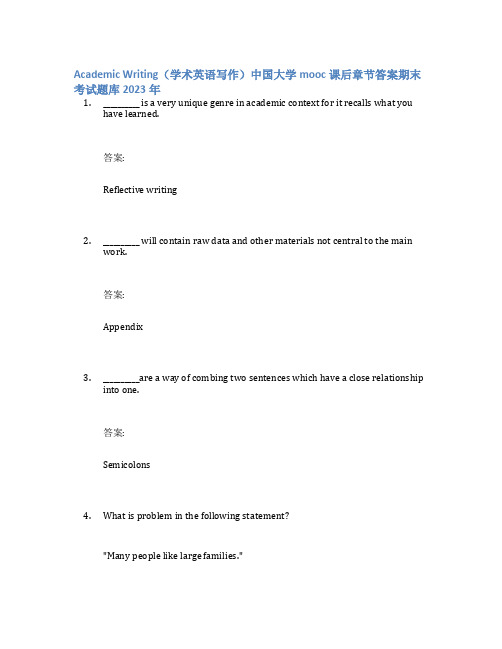
Academic Writing(学术英语写作)中国大学mooc课后章节答案期末考试题库2023年1.__________ is a very unique genre in academic context for it recalls what youhave learned.答案:Reflective writing2.__________ will contain raw data and other materials not central to the mainwork.答案:Appendix3.__________are a way of combing two sentences which have a close relationshipinto one.答案:Semicolons4.What is problem in the following statement?"Many people like large families."答案:Unspecific language5.What hedgin g language is used here to show the author’s degree ofconfidence?“One of the main functions of the pancreas is to produce hormones.”答案:Adjective6.The words “this”, “which” and “likewise” can be used as __________.答案:reference7.Read the following definition and identify the problem in it."By commercial advertisements, I mean these tricks and traps manufacturers use to advocate their products in all kinds of mass media."答案:Loaded definition with negative emotions8.Which of the following is NOT true about a paragraph developed byclassification?答案:Items from different classes can be discussed here.9.Read the following sentences and decide what kind of causal analysis thesesentences follow?"What has sparked the increasing interest in exercise? Firstly, people have gained a greater awareness of the need for physical fitness. Furthermore, the constantly improving standard of living enables Chinese people to patronize the increasing number of recreational venues. The main thing perhapscenters on the healthcare and psychological benefits exercise provides."答案:One single effect is caused by multiple causes.10.When we are planning to write an essay developed by comparison orcontrast, what should we consider as the first step?答案:Points of comparison11.Which of the following is NOT a function of the Conclusion?答案:It adds some new evidence or ideas to the essay.12.Read the following short paragraph and decide which arguing approach is used here."Autonomous vehicles (AVs) are imperfect, but they are likely to eventually become safer drivers than humans. According to data of automotive fatalities from the World Health Organization in 2018, 1.35 million humans died in car accidents, with tens of millions more injuries and disabilities (World Health Organization, 2018). Few of those deaths were the results of part failure or bad luck; the majority results from intoxication, texting while driving, and other distractions."答案:deductive13.Look at the copyright page of a book. What is the publishing house?答案: Routledge14.Look at the copyright page of a book, who is the author of the book?答案:Stephen Bailey15.In general, titles of science papers have around __________ words.答案:10-1516.When writing the Results section, we should consider the following steps__________:①summarize your findings with the relevant data②determine which results to present③present the data in an appropriate way④organize the data in order答案:②④③①17.From specific to general, a conclusion should contain: ____________,_______________, __________, _______________.①restatement of research purpose②limitation and recommendation③implications and significance④synthesis of main results答案:①④③②18.Which of the following is correct when the same author is cited for twopapers published within the same year?答案:Ellis, 2019a; Ellis, 2019b19._________ can include a general topic in the first part and a specific topic abouta distinctive feature of the study in the second part, which are oftenconnected by a colon.答案:Two-part titles20.__________ is a series of words that will often appear together.答案:Collocation21.“Replacing some of the author’s words in your text with synonyms, butmaintaining the overall structures and the vocabulary of the original” is not considered as plagiarism.答案:错误22.Academic writing is complicated.答案:错误23.In your academic writing, you should try to maximize the number of contentwords. One way to do this is to increase the number of nouns or nounphrases.答案:正确24.Many collocations in English contain function words, such as nouns, verbs,adjectives and adverbs.答案:错误25.Only transitional words, such as “because” and “thus”, can be regarded asgood transition to achieve coherence.答案:错误26.In an essay developed by comparison and contrast, the block pattern ispreferable in long writing in which there are numerous points underdiscussion.答案:错误27.When we write a conclusion for a process essay, we only need to repeat theinformation mentioned in the essay without providing any specific details.答案:正确28.An argumentative essay is an essay in which you agree with an issue andsupport it with evidence.答案:错误29. A thesis statement may state not only the author’s own point of view but a lsothe view from the other side.答案:正确30.An annotated bibliography include the list of all your sources and thesummary of the content of each source.答案:正确31.The following is an acceptable direct quotation:Gould and Brown (1991) explained that Darwin used the metaphor of thetree of life "to express the other form of interconnectedness–genealogicalrather than ecological” (p. 14).Reference:Gould, J. & Brown, A. (1991). The mating mind. Behavior Ecology,22(3),10-19.答案:正确32.Titles need to keep short and concise, and attracts the reader’s attention.答案:正确33.The abstract is the first thing you write.答案:错误34.An abstract should be fully understandable on its own to someone whohasn’t read your full paper or related sources.答案:正确35. A formal review of literature should be in the Introduction section of aresearch paper.答案:错误。
SESUG 2016 Paper BB-186 True is not False说明书

SESUG2016Paper BB-186True is not False:Evaluating Logical ExpressionsRonald J.Fehd,Stakana AnalyticsAbstract Description:The SAS R software language provides methods to evaulate logical ex-pressions which then allow conditional execution of parts of programs.In cases where logical expressions contain combinations of intersection(and),negation(not),and union(or),later readers doing maintenance mayquestion whether the expression is correct.Purpose:The purpose of this paper is to provide a truth table of Boole’s rules,De Mor-gan’s laws,and sql joins for anyone writing complex conditional statementsin data steps,macros,or procedures with a where clause.Audience:programmers,intermediate to advanced usersKeywords:Boolean algegra,Boolean logic,De Morgan’s law,evaluation,logical oper-ators,sql joinsIn this paper Introduction1Venn Diagrams of Logical Expressions4Truth Tables of Logical Expressions5Programs6T ruth T able (6)T rue is not False (7)Summary8References9 IntroductionOverviewThis article combines the ideas of three logicians,Boole,De Morgan andVenn with the language of set theory and sql in order to assemble a table oflogical expressions which describe each of the four permutations of pairsof true and false values.The intent of this exercise is to provide a thesaurus for programmers whohave specifications written by non-programmers.The introduction contains these topics.•natural language•set theory•sql•comparison operators•combinations,permutations•four setsnatural languageEach natural language has a set of grammar rules about conjunctions thatare used to describe pairs of ideas.This is a list of common phrases;logical operators are in text font.phrase operator logic join unionboth...and and disjunction inner intersectioneither...or xor,exclusive left,right exceptbut not botheither...or or,inclusive conjunction full unionneither...nor norNote:The words also and only are used in oral and written descriptions.set theorySet theory has four descriptions:union,intersection,set difference andsymmetric difference.phrase Boolean written spokenintersection and A∩B A cap Bset difference xor(T,F)A\B A and not BA minus Bsymmetric difference xor A∆B A xor Bunion or A∪B A cup BsqlStructured Query Language(sql)has two groups of operators,joins and unions.Note:Some dialects of sql insert the word outer between the keywords left,right,full and join;e.g.:full outer join is equivalent to full join.type operator Boolean descriptionjoins inner and only in both tablesleft xor(T,F)only in leftright xor(F,T)only in rightfull or all from both tablesunions except xor(T,F)compare to left joinintersect and compare to inner joinunion or compare to full joinA logical expression is evaluated according to the rules of Boolean algebra. comparison operatorsExpressions with comparisons,or relations,are reduced to the Boolean setof values(0,1)with this set of operators.parentheses:evaluate expression insideequality:equal:eq,=not equal:ne,^=(caret=),~=(tilde=)quantity:less than:lt,<;less than or equal:le,<=greater than:gt,>;greater than or equal:ge,>= Note:Other programming languages refer to this concept as relational operators. combinations,The difference between a combination and a permutation is a very impor-permutations tant idea in deconstructing logical expressions.The permutations of twovalues T and F are two sets:(T,F)and(F,T);but these two sets are dif-ferent examples of the single combination(T,F).four setsThis table list the four permutations of two expressions,Left L,and RightR,each with two values,true T,and false F.L RT TT FF TF FThe task is to use Boole’s operators and De Morgan’s Laws to uniquelyidentify each permutation.OverlappingThis set diagram shows which permutations return true for each operator. Operators Notice that the operators and and nor define only one element as T rue,whereas or,xor and nand have two or more elements defined as T rue.or norxor(T,T)(T,F)(F,T)(F,F)and nandThis list provides the operators with common natural language constructsand explanations.or:inclusive or:one,or more,Truenor:not or:neither True;both values Falsexor:exclusive or:only one True,but not both(and)and:both True;neither Falsenand:not and:one,or more,FalseVenn Diagrams of Logical ExpressionsJohn Venn was an English logician known for the visual representations of Overviewset theory known as Venn diagrams.The diagrams shown below illustratethe three operators and,xor and or with these three permutations of trueand false values.(T,T) and (T,F)(F,T)xororname:and phrase:both...and expression:L and R logic:disjunction join:inner union:intersect:xor-left:only one:but not the other :L and not R:leftjoin:xor-right:only one:but not the other:not L and R:rightjoin name:exclusive orphrase:either...or:but not bothexpression:bxor(L,R)union:(L union R):except:(L intersectR)name:inclusive orphrase:either...orexpression:L or Rlogic:conjunctionjoin:fullTruth Tables of Logical ExpressionsThis section contains the following topics.Overview•overlapping sets •expressions •De Morgan’s LawsThis table shows the four permutations of sets of pairs of values,overlapping setsand(T ,T),xor-left(T ,F),xor-right(F ,T),nor(F ,F),and the logical operators xor ,or ,and nand which include two or more of the basic four.name values and T ,T xor-left T ,F xor-right F ,Txoror nandnor F ,F }norThis table shows the logical expressions that are used to describe each ofexpressionsthe four permutations of pairs of (T,F)values.nandvalues and or and L R xor or nor,orL and R T T L or R not(L and R)not L or not R L and not R T F bxor(L,R)L or R not(L and R)not L or not R not L and R F T bxor(L,R)L or Rnot(L and R)not L or not Rnot L and not R F Fnot(L or R)nor,andAugustus De Morgan was a contemporary of Boole.These rules are stated De Morgan’s Lawsin formal logic.Conjunction means and ;disjunction means or .nand :The negation of a conjunction is the disjunction of the negations.nor :The negation of a disjunction is the conjunction of the expression parentheses nand:not and not L or not R nonot (L and R)required nor:not ornot L and not R nonot (L or R)requiredProgramsTruth Table This program shows a truth table of the logical expressions defined aboveand their resolution.16Title3"Truth Table with L and R";17%let sysparm=1,0;*boolean;1819PROC format;value TF0=’F’201=’T’;2122DATA truth_table;23label nand_and=’nand-and;not(L and R)’24nand_or=’nand-or;not L or not R’25L=’L’26R=’R’27and_L_R=’and(T,T)’28and_L_not_R=’xor-left;and(T,F)’29and_not_L_R=’xor-right;and(F,T)’30and_not_L_not_R=’nor-and;and(not(F),not(F))’31xor=’xor(T,F);xor(F,T)’32or=’or(L,R)’33nor_and=’nor-and;not L and not R’34nor_or=’nor-or;not(L or R)’;35format_numeric_TF.;36do L=&sysparm;37do R=&sysparm;38nand_and=not(L and R);39nand_or=not L or not R;40and_L_R=L and R;41and_L_not_R=L and not R;42and_not_L_R=not L and R;43and_not_L_not_R=not L and not R;***duplicate;44xor=bxor(L,R);45or=L or R;46nor_and=not L and not R;***duplicate;47nor_or=not(L or R);48output;49end;50end;51stop;52run;53PROC print data=&syslast label noobs54split=’;’;output1nand.and nand.or xor-left xor-right nor.and xor(T,F)nor.and nor.or2not(L and R)not L or not R L R and(T,T)and(T,F)and(F,T)and(F,F)xor(F,T)or(L,R)and(F,F)not(L or R) 3--------------------------------------------------------------------------------------------4F F T T T F F F F T F F5T T T F F T F F T T F F6T T F T F F T F T T F F7T T F F F F F T F F T T8duplicate duplicateTrue is not FalseThis section contains programs for the following topics.Overview•data step function ifc•implicit evaluation in macro expressions•refactoring macro values with%evalThe ifc function has four parameters: data step function ifc1.a logical expression2.character value returned when true3.value returned when false4.value returned when missing,which is optionalThis example shows the function in a data step.1%let false=false<---<<<;2DATA test_ifc_integers;3attrib integer length=44text_if length=$%length(false) 5text_ifc length=$%length(&false); 6do integer=.,-1to2;7if not integer then text_if=’false’;8else text_if=’true’;9text_ifc=ifc(integer,’true’10,"&false"11,’missing’);12output;13end;14stop;15run;1617PROC print data=&syslast noobs;integer text_if text_ifc---------------------------.false missing-1true true0false false<---<<<1true true2true trueNote zero and missing are false,negative and positive values are true!This program shows that the macro language performs an evaluation of an Implicit Evaluation inMacro Expressions integer,similar to the data step function ifc.1%macro test_tf;2%do value=-1%to2;3%if&value%then4%put&=value is true; 5%else6%put&=value is false; 7%end;8%mend;9%test_tf;VALUE=-1is true VALUE=0is false VALUE=1is true VALUE=2is trueMany programmers provide a macro variable to use while debugging or Refactoring MacroValues With%eval testing.This macro variable may be initialized to any number of valuesrepresenting false,such as(no,off,),etc.The problem of checking for thecorrectly spelled value such as YES,Y es,yes,Y,y,ON,On,on,can beeliminated by recoding the value to boolean with this comparison expres-sion,%eval(0ne&testing)!→which acknowledges any value other than zero as true.1%macro testing2(testing=0/*default:false,off*/ 3);4%*recode:any value turns testing on;5%let testing=%eval(0ne&testing);6%if&testing%then%do;7%put&=testing is true;8%end;9%else%do;10%put&=testing is false;11%end;12%mend;110%testing()2TESTING=0is false 311%testing(testing=1)4TESTING=1is true 512%testing(testing=.)6TESTING=1is true 713%testing(testing=?)8TESTING=1is true 914%testing(testing=T)10TESTING=1is true 1115%testing(testing=True)12TESTING=1is true 1316%testing(testing=yes)14TESTING=1is true 1517%testing(testing=no)16TESTING=1is trueSummarySuggested Reading people:[3],George Boole[2],Augustus De Morgan[4],John Venn predecessors:[6]shows three logical and expressions to choose output data sets[7]provides examples of checking command-line options during test-ing to add additional code to programs[8],using%sysfunc with ifc[9],macro design ideassets:[5]set theorysql:[10],using sql except operator for a report similar to compare proce-dure;[13],review of sql set operators outer union,union,intersect,and except with Venn diagrams;[12]Lassen to SAS-L about sql xor;[11]Lafler,sql,beyond basics,2e;[1]J.Celko on sql relational divisionEvaluating logical expressions has two aspects,conversion of comparisons Conclusionto boolean values and logical algebra using the operators not,and andor.This paper provides the following benefits.The names of each ofthe permutations are and,xor-left,xor-right,and nor.Each permutationis identified using not with and.The names of sets of permutations are xor,or,and nand.Venn diagrams are provided for each of the permutations;these visual representations are helpful in understanding the sql conceptsof addition and subtraction of the permutations.With this vocabulary andconceptual representations a programmer may be confident of understand-ing requirements and specifications no matter what language,discipline orscientific dialect they are written in.Contact Information Ronald J.Fehd****************************** About the author:sco.wiki /wiki/Ronald_J._FehdLinkedIn /Ronald.Fehdaffiliation Stakana Analytics,Senior Maverickalso known as macro maven on SAS-L,Theoretical ProgrammerPrograms:/wiki/Evaluating Logical ExpressionsT ruth T ableWriting T esting Aware Programs AcknowledgementsKirk Lafler and Søren Lassen reviewed a draft of this paper;each provided clarifi-cation on sql ssen noted his SAS-L post with reference to Celko’s sqlexplanation.TrademarksSAS and all other SAS Institute Inc.product or service names are registered trademarks ortrademarks of SAS Institute Inc.In the USA and other countries R indicates USA registration.Other brand and product names are trademarks of their respective companies. References[1]Joe Celko.Divided we stand:The sql of relational division.In Simple T alk,2009.URL https:///sql/t-sql-programming/divided-we-stand-the-sql-of-relational-division/.[2]Wikipedia Editors et al.Augustus De Morgan.In Wikipedia,The Free Encyclopedia,2016.URL https:///wiki/Augustus_De_Morgan.[3]Wikipedia Editors et al.George Boole.In Wikipedia,The Free Encyclopedia,2016.URL https:///wiki/George_Boole.[4]Wikipedia Editors et al.John Venn.In Wikipedia,The Free Encyclopedia,2016.URL https:///wiki/John_Venn.[5]Wikipedia Editors et al.Set theory.In Wikipedia,The Free Encyclopedia,2016.URL https:///wiki/Set_theory.[6]Editor R.J.Fehd.Macro Extract.In ,2008.URL /wiki/Macro_Extract.given two snapshots,extract database adds,changes,deletes.[7]Ronald J.Fehd.Writing testing-aware programs that self-report when testing options are true.In NorthEast SAS Users Group ConferenceProceedings,2007.URL /Proceedings/nesug07/cc/cc12.pdf.Coders’Corner,20pp.;topics:options used while testing: echoauto,mprint,source2,verbose;variable testing in data step or macros;call execute;references.[8]Ronald ing functions Sysfunc and Ifc to conditionally execute statements in open code.In SAS Global Forum Annual ConferenceProceedings,2009.URL /resources/papers/proceedings09/054-2009.pdf.Coders Corner,10pp.;topics:combining functions ifc,nrstr,sysfunc;assertions for testing:existence of catalog,data,file,orfileref;references.[9]Ronald J.Fehd.Macro design ideas:Theory,template,practice.In SAS Global Forum Annual Conference Proceedings,2014.URL/resources/papers/proceedings14/1899-2014.pdf.21pp.;topics:logic,quality assurance,testing,style guide,doc-umentation,bibliography.[10]Stanley Fogleman.Teaching a new dog old tricks—using the except operator in proc sql and generation data sets to produce a comparison report.In MidWest SAS Users Group Conference Proceedings,2006.URL /nesug/nesug06/cc/cc10.pdf.Beyond Basics,3pp.;using sql except to produce report similar to compare procedure.[11]Kirk Paul Lafler.PROC SQL:Beyond the Basics Using SAS(R),Second Edition.SAS Institute,2013.URL /store/prodBK_62432_en.html.[12]Søren Lassen.Re:Excellent short tutorial on sql.In SAS-L archives,2016.URL https:///cgi-bin/wa?A2=SAS-L;aa4234fb.1604b.[13]Howard Schreier.SQL set operators:So handy Venn you need them.In SAS Users Group International Annual Conference Proceedings,2006.URL /proceedings/sugi31/242-31.pdf.T utorials,18pp.;outer union,union,intersect,and except.。
2 Example-based segmentation

An Example-Based Chinese Word Segmentation System for CWSB-2Chunyu Kit Xiaoyue LiuDepartment of Chinese,Translation and LinguisticsCity University of Hong KongTat Chee Ave.,Kowloon,Hong Kong{ctckit,xyliu0}@.hkAbstractThis paper reports the example-basedsegmentation system for our participa-tion in the second Chinese Word Seg-mentation Bakeoff(CWSB-2),present-ing its basic ideas,technical details andevaluation.It is a preliminary imple-mentation.CWSB-2valuation showsthat it performs very well in identify-ing known words.Its unknown worddetection module also illustrates greatpotential.However,proper facilities foridentifying time expressions,numbersand other types of unknown words areneeded for improvement.1IntroductionWord segmentation is to identify lexical items, especially individual word forms,in a text.It involves two fundamental tasks,both aiming at minimizing segmentation errors:one is to in-fer out-of-vocabulary(OOV)words,also known as unknown(or unseen)word detection,and the other to identify in-vocabulary(IV)words,with an emphasis on disambiguation.OOV words and ambiguities are the two major causes to segmen-tation errors.Accordingly,word segmentation approaches can be divided into the categories summarized in Table1in terms of the resources in use to tackle these two causes.The closed and open tracks in CWSB correspond,respectively,to the last two categories,both involving inferring OOV wordsCategory Resource in use Major TaskLexicon Tr.Corpus OOV Disamb. WD a-(-)b+WS/CL c+--+WS/IL d+-++WS/TC e(+)f+++WS/TC+L g++++a Word discovery,or unsupervised lexical acquisitionb Input data is used for unsupervised trainingc Word segmentation with a complete lexicond Word segmentation with an incomplete lexicone Word segmentation with a pre-segmented training corpusf It can be extracted from the given training corpus.g Word segmentation with a pre-segmented training corpus and an extra lexiconTable1:Categories of segmentation approachbeyond disambiguating IV words.Word discov-ery and OOV word detection pursue a similar tar-get,i.e.,inferring new words.The continuum connecting them is the size of the lexicon in use: the former assumes few words known and the lat-ter an existing lexicon to some scale.Inferring new words is an essential task in word segmen-tation,for a complete lexicon is rarely a realistic assumption in practice.This paper presents our segmentation system for participation in CWSB-2.It takes an example-based approach to recognize IV words and fol-lows description length gain(DLG)to infer OOV words in terms of their text compression effect. Sections2and3below introduce the example-based and DLG-based segmentation respectively. Section4presents a strategy to combine their strength and Section5reports our system’s per-formance in CWSB-2.Following error analysis in Section6,Section7concludes the paper.2Example-based segmentationHow to utilize as much information as possi-ble from the training corpus to adapt a segmen-tation system towards a segmentation standard has been a critical issue.Kit et al.(2002)and Kit et al.(2003)attempt to integrate case-based learning with statistical models(e.g.,n-gram)by extracting transformation rules from the train-ing corpus for disambiguation via error correc-tion;Gao et al.(2004)adopt a similar strategy for adaptive segmentation,with transformation templates(instead of case-based rules)to modify word boundaries(instead of individual words). The basic idea of example-based segmentation is very simple:existing pre-segmented strings in training corpus provide reliable examples for seg-menting similar strings in input texts.In contrast to dictionary checking for locating possible words in an input sentence to facilitate later segmenta-tion operations,pre-segmented examples give ex-act segmentation to copy.The example-based segmentation can be im-plemented in the following steps.1.Find all exemplar pre-segmented fragments,with regards to a training corpus,and allpossible words,with regards to a lexicon,from each character in an input sentence; 2.Identify the optimal sequence,among allpossibilities,of the above items over the sen-tence following some optimization criterion. If adopting the minimal number of fragments or words in a sequence as optimization criterion,we have a maximal matching approach to word seg-mentation.However,it differs remarkably from the previous maximal matching approaches:it matches pre-segmented fragments,instead of dic-tionary words,against an input sentence.It can be carried out by a best-first strategy:repeatedly se-lect the next longest example or word until the en-tire sentence is properly covered.Unfortunately, the best-first approach does not guarantee to give the best answer.For CWSB-2,we implemented a program following the Viterbi algorithm to per-form a complete search in terms of the number of fragments,and then words,in a sequence. However,a serious problem with this example-based approach is the sparse data problem.Long exemplar fragments are more reliable but small in number,whereas short ones are large in num-ber but less reliable.In the case of no exemplar fragment available for an input sentence,this ap-proach draws back to the maximal match segmen-tation with a dictionary.How to incorporate sta-tistical inference into example-based segmenta-tion to infer more reliable optimal segmentation beyond string matching remains a critical issue for us to tackle.3DLG-based segmentationDLG is formulated in Kit and Wilks(1999)and Kit(2000)as an empirical measure for the com-pression effect of extracting a substring from a given corpus as a lexical item.DLG optimization is applied to detect OOV words for our participa-tion in CWSB-2.It works as follows in two steps.1.Calculate the DLG for all known wordsand all new word candidate(i.e.,substringswith frequency≥2,preferably,in the testcorpus),based on frequency information inthe training and the test corpora;2.Find the optimal sequence of such items overan input sentence with the greatest sum ofDLG.Step2above in our system re-implements only thefirst round of DLG-based lexical learning in Kit(2000).It is implemented by the same algo-rithm as the one for example-based segmentation, with DLG as optimization criterion.Evaluation results show that this learning-via-compression approach discovers many OOV words success-fully,in particular,person names.4IntegrationThe example-based segmentation is good at iden-tifying IV words but incapable of recognizing any new words.In contrast,the DLG-based segmen-tation performs slightly worse but has potential to detect new words.It is expected that the strength of the two could be combined together for perfor-mance enhancement.However,because of inadequate time we had to take a shortcut in order to catch the CWSB-2deadline:DLG segmentation is only applied to recognize new words among the sequences of mono-character items in the example-based seg-mentation output.Track P R F OOV ROOV RIVAS c.944.902.923.043.234.976 PKU c.929.904.916.058.252.971 MSR c.965.935.950.026.189.986Table2:System performance in CWSB-25PerformanceOur group took part in three closed tracks in CWSB-2,namely,AS c,PKU c and MSR c,with a preliminary implementation of the example-based word segmentation presented above.Our sys-tem’s performance in terms of CWSB-2’s offi-cial scores is presented in Table2.Its ROOV scores look undesirable,showing that applyingthefirst round of DLG-based segmentation to se-quences of mono-character items is inadequate for the OOV word discovery task.Nevertheless, its RIVscores are,in general,quite close to the top systems in CWSB-2,although it does not have a disambiguation module to polish its maximal matching output.However,this is not to say that the DLG-based segmentation deserves no credit in unknown word detection.It does recognize many OOV words, as shown in Table3.The low ROOVrate has to do with our system’s incapability in handling time expressions,numbers,and foreign words.6Error analysisMost errors made by our system are due to the following causes:(1)no knowledge,overt or implicit,in use for recognizing time expres-sions,numbers and foreign words,as restricted by CWSB-2rules,(2)a premature module for OOV word detection,(3)no further disambiguation be-sides example application,and(4)significant in-consistency in the training and test data.The inconsistency exists not only between the training and test corpora for each track but,more surprisingly,also within individual training cor-pora.Some suspected cases are illustrated in Ta-bles4,5and6.They are observed to be in a large number in the CWSB-2corpora.Scoring with a golden standard involving so many of them ap-pears to be problematic,for it penalizes the sys-tems for handling such cases right and rewards the others for producing“correct”answers.What AS c:小森(106)左詩雅(45)馮禮(31)霍金(29)瑪雅(21)丹紐(20)張庭(18)英特爾(17)黑盤鮑(16)沈時華(15)證期會(13)厲莉(12)黃銘坤(11)卡門(11)佛指舍利(11)陳慶浩(10)璩女(9)范曉萱(8)郭女(8)邱太三(7)網站(7)狄亞尼(7)張惠妹(6)米田真澄(6)鄭優(5)丁克華(5)經發會(5)蘇盈貴(5)陳秀玲(5)八美圖(5)房貸(5)蔡信弘(5)郭玉鈴(4)地型(4)御膳(4)白藜蘆醇(4)羅麗泰(3)張玨(3)米仓涼子(3)陳兆伸(2)羅勒(2)幼發拉底河(2)溥傑(2)······PKU c:罢免(38)世清(23)任免(21)拉姆斯菲尔德(20)哈苏(19)海合会(17)军级(16)加纳(15)水心村(12)网友(11)小阜村(10)宋双(10)吐逊江(9)申奥(9)库福尔(8)金税(8)亚布力(8)金门(7)三阳镇(7)教主(6)罢免书(6)法轮功(6)香客(6)普京(6)福清市(5)柯尔克孜(5)华能(5)罗林斯(5)松诺斯(5)洋务(5)马祖(5)甲午(5)哈斯达(4)奥申委(4)高唐县(4)文津(4)门市部(4)妈祖(4)刁民(4)闭会(4)商机(4)临武县(4)湄洲岛(4)圈套(4)法塔赫(3)流星雨(3)堆龙德庆县(3)阿族(3)哥德堡(3)信众(3)钱柜(3)衡水(3)内坦亚(3)双丰村(3)······MSR c:博古(26)玲英(19)游景玉(19)任尧森(17)秦机(15)亚仿(14)进占(14)刘积仁(13)东宝(13)internet(12)猴王(12)双保(11)张肇群(10)抚州(10)南丁格尔(10)彭珮云(10)海塘(10)王常力(9)穆守家(9)大关村(8)赞皇(8)张钧(8)嶂石岩(7)三老四严(7)局域网(7)透支(6)秦家山(6)东软(6)后金(6)八旗(6)弄堂(5)黎秀芳(5)提灌(5)大关(5)王丙乾(5)棉铃虫(5)百亿次(4)西文(4)米夫(4)陆冰(4)郑守仁(4)秦邦宪(4)关小瑛(3)二进制(3)顺延(3)李朋朋(3)瞿秋白(3)黄华平(3)汪赛进(3)汪延(3)何元亮(3)陆佑楣(3)阜平县(3)中信所(3)导流(3)徐殿龙(3)重男轻女(2)······Table3:Illustration of new words successfully detected,with frequency in parenthesesis even more worth noting is that(1)an inconsis-tent case involves more than one word,and(2) the difference between a correct and an erroneous judgment of a word is1,in a sense,but the differ-ence between one system that loses it for doing right and another that earns it by doing wrong is surely greater.7ConclusionsIn the above sections we have reported the example-based word segmentation system for our participation in CWSB-2,including its ba-sic ideas,technical details and evaluation results. It has illustrated an excellent performance in IV word identification and nice potential in OOV word discovery.However,its weakness in han-dling time expressions,numbers and other types of unknown words has hindered it from perform-ing better.We are expecting to implement a full-fledged version of the system for improvement. AcknowledgementsThe work described in this paper was supported by the RGC of HKSAR,China,through the CERG grant9040861.We wish to thank Alex Fang and Robert Neather for their help.Training&Answer fT /fAGolden Standard fT/fA繁殖場4/8繁殖場0/0老歌28/7老歌0/0準備率5/7準備率0/0小火車11/6小火車0/0本文186/5本文0/0第三者41/4第三者0/0新一代29/4新一代0/0稱之為129/4稱之為0/0夠不夠23/3夠不夠0/0短時間47/3短時間0/0中正機場33/2中正機場0/0台北市長32/2台北市長0/0民意代表85/2民意代表0/0清醒過來10/2清醒過來0/0統治者62/2統治者0/0新生命23/2新生命0/0也就是說192/1也就是說0/0不景氣149/1不景氣0/0中央政府66/1中央政府0/0挑戰性31/1挑戰性0/0看電影80/1看電影0/0研究者68/1研究者0/0面無表情13/1面無表情0/0曾文水庫13/1曾文水庫0/0無意識20/1無意識0/0無話不談6/1無話不談0/0另一面29/1另一面0/0紅透半邊天4/1紅透半邊天0/0民間團體24/7民間團體25/0女性主義17/3女性主義53/0是不是1201/2是不是2/0Table4:Some inconsistent cases in AS corpusTraining&Answer fT /fAGolden Standard fT/fA乡政府14/26乡政府0/0区政府6/1区政府0/0镇政府5/21镇政府0/0世纪之交24/19世纪之交0/0改为23/18改为0/0生产总值66/15生产总值0/0个人所得税10/9个人所得税0/0无党派人士10/5无党派人士0/0新年伊始45/5新年伊始0/0组成部分42/5组成部分0/0固定资产27/4固定资产0/0世界各地21/4世界各地0/0乡镇企业126/4乡镇企业0/0至关重要20/4至关重要0/0综合国力15/4综合国力0/0总参谋长25/4总参谋长0/0经济社会25/3经济社会0/0劳动生产率13/3劳动生产率0/0去年底32/3去年底0/0世界纪录30/3世界纪录0/0无党派11/3无党派0/0议事日程15/3议事日程0/0中小企业22/3中小企业0/0不同于11/2不同于0/0此事25/2此事0/0火树银花不夜天3/1火树银花不夜天0/0雨夹雪13/1雨夹雪0/0县政府24/5县政府1/0意味着49/4意味着1/0很多112/3很多14/0发达国家48/1发达国家1/0Table5:Some inconsistent cases in PKU corpusTraining&Answer fT/fAGolden Standard fT/fA 营业部12/7营业部0/0高性能16/6高性能0/0“八五29/5“八五0/0大型企业集团6/3大型企业集团0/0径流量3/3径流量0/0海信电器1/2海信电器0/0投资银行?4/2投资银行0/0一版10/2一版0/0浙东3/2浙东0/02000多人7/12000多人0/0采访团2/1采访团0/0产油国家1/1产油国家0/0第一、二、三?4/1第一、二、三0/0葛洲坝大江1/1葛洲坝大江0/0国际互联网络1/1国际互联网络0/0计算机应用软件1/1计算机应用软件0/0家门口?10/1家门口0/0两点?16/1两点0/0吕梁?4/1吕梁0/0一口气?16/1一口气0/0中国人民122/1中国人民0/0中外合资经营3/1中外合资经营0/0 Table6:Some inconsistent cases in MSR corpusReferencesE.Brill.1993.A Corpus-Based Approach to Lan-guage Learning.PhD thesis,University of Pennsyl-vania,Philadelphia.J.Gao,A.Wu,M.Li,C.Huang,H.Li,X.Xia and H.Qin.2004.Adaptive Chinese word segmentation.In ACL-04.Barcelona,July21-26.C.Kit and Y.Wilks.1999.Unsupervised learning ofword boundary with description length gain.In M.Osborne and E.T.K.Sang(eds.),CoNLL-99,pp.1-6.Bergen,Norway,June12.C.Kit2000.Unsupervised Lexical Learning asInductive Inference.PhD thesis,University of Sheffield.C.Kit,H.Pan and H.Chen.2002.Learning case-based knowledge for disambiguating Chinese word segmentation:A preliminary study.SIGHAN-1, pp.33―39.Taipei,Sept.1,2002.C.Kit,Z.Xu and J.J.Webster.2003.Integratingn-gram model and case-based learning for Chinese word segmentation.In Q.Ma and F.Xia(eds.), SIGHAN-2,pp.160-163.Sapporo,11July,2003. D.Palmer.A trainable rule-based algorithm for wordsegmentation.In ACL-97,pp.321-328.Madrid.。
Automated essay evaluation The Criterion online writing service

Grammar, Usage and Mechanics
The writing analysis tools identify five main types of errors—agreement errors, verb formation errors, wrong word use, missing punctuation, and typographical/proofreading errors. Some examples are shown in table 1. The approach to detecting violations of general English grammar is corpus-based and statistical. The system is trained on a large corpus of edited text, from which it extracts and counts sequences of adjacent word and part-of-speech pairs called bigrams. The system then searches student essays for bigrams that occur much less often than is expected based on the corpus frequencies. The expected frequencies come from a model of English that is based on 30-million words of newspaper text. Every word in the corpus is
scoring and evaluation. Work in automated essay scoring began in the early 1960s and has been extremely productive (Page 1966; Burstein et al. 1998; Foltz, Kintsch, and Landauer 1998; Larkey 1998; Rudner 2002; Elliott 2003). Detailed descriptions of most of these systems appear in Shermis and Burstein (2003). Pioneering work in the related area of automated feedback was initiated in the 1980s with the Writer’s Workbench (MacDonald et al. 1982). The Criterion Online Essay Evaluation Service combines automated essay scoring and diagnostic feedback. The feedback is specific to the student’s essay and is based on the kinds of evaluations that teachers typically provide when grading a student’s writing. Criterion is intended to be an aid, not a replacement, for classroom instruction. Its purpose is to ease the instructor’s load, thereby enabling the instructor to give students more practice writing essays. Criterion contains two complementary applications that are based on natural language processing (NLP) methods. Critique is an application that is comprised of a suite of programs that evaluate and provide feedback for errors in grammar, usage, and mechanics, that identify the essay’s discourse structure, and that recognize potentially undesirable stylistic features. The companion scoring application, e-rater version 2.0, extracts linguistically-based features from an essay and uses a statistical model of how these features are related to overall writing quality to assign a holistic score to the essay. Figure 1 shows Criterion’s interface for submit-
英语作文考后反思
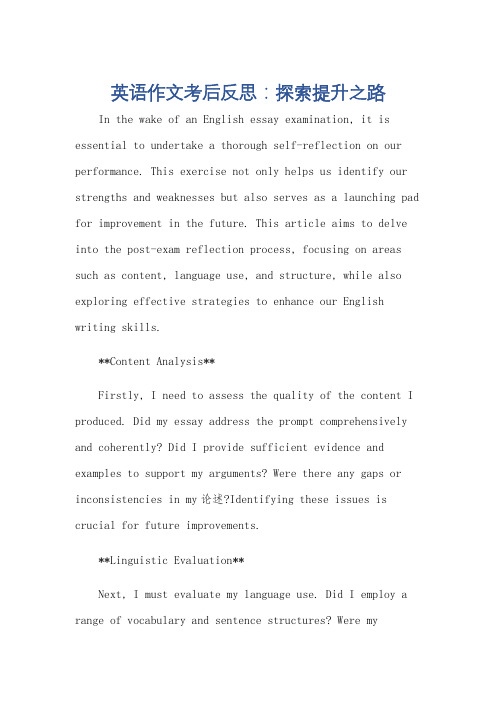
英语作文考后反思:探索提升之路In the wake of an English essay examination, it is essential to undertake a thorough self-reflection on our performance. This exercise not only helps us identify our strengths and weaknesses but also serves as a launching pad for improvement in the future. This article aims to delve into the post-exam reflection process, focusing on areas such as content, language use, and structure, while also exploring effective strategies to enhance our English writing skills.**Content Analysis**Firstly, I need to assess the quality of the content I produced. Did my essay address the prompt comprehensively and coherently? Did I provide sufficient evidence and examples to support my arguments? Were there any gaps or inconsistencies in my论述?Identifying these issues is crucial for future improvements.**Linguistic Evaluation**Next, I must evaluate my language use. Did I employ a range of vocabulary and sentence structures? Were myparagraphs well-constructed and varied in sentence length and complexity? Did I avoid common grammatical errors such as subject-verb agreement or tense inconsistencies? Linguistic proficiency is a key component of effective writing, and regular practice is essential to improve in this area.**Structural Analysis**Moreover, the structure of my essay is crucial. Did I introduce my topic clearly and concisely? Did I develop my ideas logically, with well-placed transitional phrases? Did I conclude my essay strongly, summing up my main points and leaving a lasting impression on the reader? Analyzing these aspects helps me identify areas where I can tighten up my writing and make it more impactful.**Strategies for Improvement**To enhance my English writing skills, I plan to adopt several strategies. Firstly, I will focus on increasing my vocabulary by reading widely and actively incorporating new words into my writing. Secondly, I will work on varying my sentence structures to avoid monotony and improve readability. Thirdly, I will practice writing regularly,seeking feedback from teachers or peers to identify areas for improvement. Additionally, I will focus on refining my essay structure, ensuring a logical flow from introduction to conclusion.In conclusion, post-exam reflection is a vital part of the learning process. By carefully analyzing our performance in the English essay examination, we can identify areas for improvement and devise effective strategies to enhance our writing skills. By embracing a growth mindset and committing to regular practice, we can turn our weaknesses into strengths and achieve excellence in English writing.**英语作文考后反思:探索提升之路**在英语作文考试之后,进行深入的自我反思是至关重要的。
GENERAL PURPOSE CORRECTION OF GRAMMATICAL AND WORD
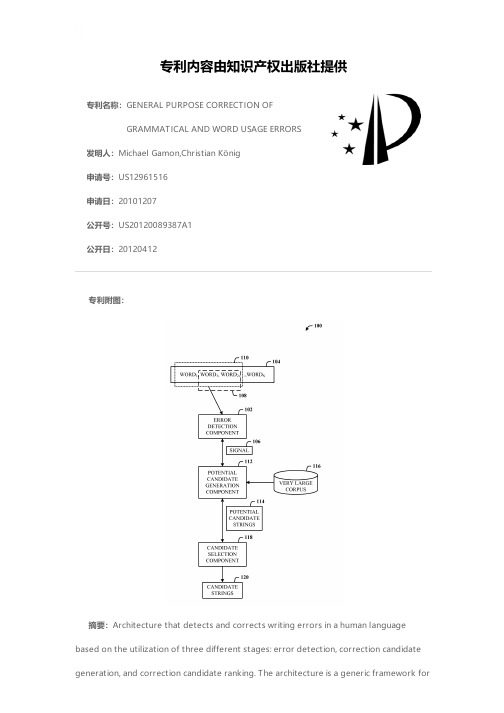
专利名称:GENERAL PURPOSE CORRECTION OF GRAMMATICAL AND WORD USAGE ERRORS
发明人:Michael Gamon,Christian König 申请号:US12961516 申请日:20101207 公开号:US20120089387A1 公开日:201204 12 专利附图:
申请人:Michael Gamon,Christian König
地址:Seattle WA US,Kirkland WA US
国籍:US,US
更多信息请下载全文后查看
Hale Waihona Puke 摘要:Architecture that detects and corrects writing errors in a human language based on the utilization of three different stages: error detection, correction candidate generation, and correction candidate ranking. The architecture is a generic framework for
generating fluent alternatives to non-grammatical word sequences in a written sample. Error detection is addressed by a suite of language model related scores and other scores such as parse scores that can identify a particularly unlikely sequence of words. Correction candidate generation is addressed by a lookup in a very large corpus of “correct” English that looks for alternative arrangements of the same or similar words or subsequences of these words in the same context. Correction candidate ranking is addressed by a language model ranker.
- 1、下载文档前请自行甄别文档内容的完整性,平台不提供额外的编辑、内容补充、找答案等附加服务。
- 2、"仅部分预览"的文档,不可在线预览部分如存在完整性等问题,可反馈申请退款(可完整预览的文档不适用该条件!)。
- 3、如文档侵犯您的权益,请联系客服反馈,我们会尽快为您处理(人工客服工作时间:9:00-18:30)。
227
2. ARCHITECTURE
The architecture in Figure 1 was chosen to minimize labor and to maximize use of existing software. It employs JUMAN first to provide initial word segmentation of the text, an annotation-based algorithm second to correct both segmentation errors and part of speech errors in JUMAN output, and POST third both to select among ambiguous alternative segmentations/partof-speech assignments and also to predict the part of speech of unkcribes an example-based correction component for Japanese word segmentation and part of speech labelling (AMED), and a way of combining it with a pre-existing rule-based Japanese morphological analyzer and a probabilistic part of speech tagger.
E X A M P L E - B A S E D C O R R E C T I O N OF W O R D S E G M E N T A T I O N A N D P A R T OF S P E E C H L A B E L L I N G
Tomoyoshi Matsukawa , Scott Miller, and Ralph Weischedel
1. INTRODUCTION
Probabilistic part of speech taggers have proven to be successful in English part of speech labelling [Church 1988; DeRose, 1988; de Marcken, 1990; Meteer, et. al. 1991, etc.]. Such stochastic models perform very well given adequate amounts of training data representative of operational data. Instead of merely stating what is possible, as a non-stochastic rule-based model does, probabilistic models predict the likelihood of an event. In determining the part of speech of a highly ambiguous word in context or in determining the part of speech of an unknown word, they have proven quite effective for English. By contrast, rule-based morphological analyzers employing a hand-crafted lexicon and a hand-crafted connectivity matrix are the traditional approach to Japanese word segmentation and part of speech labelling [Aizawa and Ebara 1973]. Such algorithms have already achieved 90-95% accuracy in word segmentation and 9095% accuracy in part-of-speech labelling (given correct word segmentation). The potential advantage of a rulebased approach is the ability of a human coding rules that cover events that are rare, and therefore may be inadequately represented in most training sets. Furthermore, it is commonly assumed that large training sets are not required.
- A probabilistic part-of-speech tagger (POST) [Meteer, et al., 1991] which assumed a single sequence of words as input.
-
Limited human resources for creating training data.
BBN Systems and Technologies 70 Fawcett St. Cambridge, M A 02138
A
B
S
T
R
A
C
T
A third approach combines a rule-based part of speech tagger with a set of correction templates automatically derived from a training corpus [Brill 1992]. We faced the challenge of processing Japanese text, where neither spaces nor any other delimiters mark the beginning and end of words. We had at our disposal the following: A rule-based Japanese morphological processor (JUMAN) from Kyoto University. A context-free grammar of Japanese based on part of speech labels distinct from those produced by JUMAN.
This presented us with four issues: 1) how to reduce the cost of modifying the rule-based morphological analyzer to produce the parts of speech needed by the grammar, 2) how to apply probabilistic modeling to Japanese, e.g., to improve accuracy to -97%, which is typical of results in English, 3) how to deal with unknown words, where JUMAN typically makes no prediction regarding part of speech, and 4) how to estimate probabilities for low frequency phenomena. Here we report on an example-based technique for correcting systemmatic errors in word segmentation and part of speech labelling in Japanese text. Rather than using handcrafted rules, the algorithm employs example data, drawing generalizations during training. In motivation, it is similar to one of the goals of Brill (1992).
I JUMAN [
Segment Correction Model
Part-of-speecl~ Model
i
Word segments with Part of Speech
Figure 1: Architecture Let us briefly review each component. JUMAN, available from Kyoto University makes segmentation decisions and part of speech assignments to Japanese text. To do this, it employs a lexicon of roughly 40,000 words, including their parts of speech. Where alternative segmentations are possible, the connectivity matrix eliminates some possibilities, since it states what parts of speech may follow a given part of speech. Where the connectivity matrix does not dictate a single segmentation and part of speech, generally longer words are preferred over shorter segmentations. An example JUMAN output is provided in Figure 2. The Japanese segment is given first, followed by a slash