The effect of EGM2008-based normal, normal-orthometric and Helmert orthometric height systems
Two algorithms for extracting building models from raw laser altimetry data
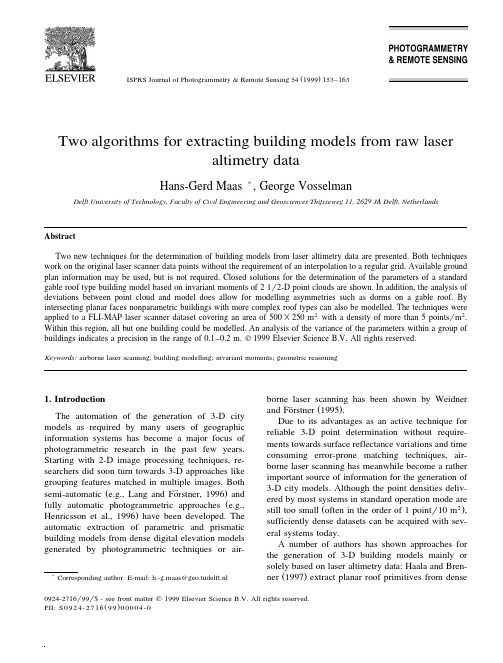
borne laser scanning has been shown by Weidner Ž1995.. and Forstner ¨ Due to its advantages as an active technique for reliable 3-D point determination without requirements towards surface reflectance variations and time consuming error-prone matching techniques, airborne laser scanning has meanwhile become a rather important source of information for the generation of 3-D city models. Although the point densities delivered by most systems in standard operation mode are still too small Žoften in the order of 1 pointr10 m2 ., sufficiently dense datasets can be acquired with several systems today. A number of authors has shown approaches for the generation of 3-D building models mainly or solely based on laser altimetry data: Haala and Brenner Ž1997. extract planar roof primitives from dense
EGM2008

EGM2008 - WGS 84 VersionIntroductionThe official Earth Gravitational Model EGM2008 has been publicly released by the U.S. National Geospatial-Intelligence Agency (NGA) EGM Development Team. This gravitational model is complete to spherical harmonic degree and order 2159, and contains additional coefficients extending to degree 2190 and order 2159. Full access to the model's coefficients and other descriptive files with additional details about EGM2008 are provided herein.Those wishing to use EGM2008 to compute geoid undulation values with respect to WGS 84,may do so using the self-contained suite of coefficient files, FORTRAN software, and pre-computed geoid grids provided on this web page. For other applications, the previous release of the full 'Geoscience' package for EGM2008 can be accessed through the link at the bottom of this web page.The WGS 84 constants used to define the reference ellipsoid, and the associated normal gravity field, to which the geoid undulations are referenced are:•a=6378137.00 m (semi-major axis of WGS 84 ellipsoid)•f=1/298.257223563 (flattening of WGS 84 ellipsoid)•GM=3.986004418 x 1014 m3s-2 (Product of the Earth's mass and the Gravitational Constant)•ω=7292115 x 10-11 radians/sec (Earth's angular velocity)All synthesis software, coefficients, and pre-computed geoid grids listed below assume a Tide Free system, as far as permanent tide is concerned.Note that the harmonic synthesis software provided below applies a constant,zero-degree term of -41 cm to all geoid undulations computed using EGM2008 with the height_anomaly-to-geoid_undulation correction model (also provided). Similarly, all pre-computed geoid undulations incorporate this constant zero-degree term. This term converts geoid undulations that are intrinsically referenced to an ideal mean-earth ellipsoid into undulations that are referenced to WGS 84. The value of -41 cm derives from a mean-earth ellipsoid for which the estimated parameters in the Tide Free system are: a=6378136.58 m and 1/f=298.257686.Description of Software and DataTo compute point geoid undulations from spherical harmonic synthesis of the EGM2008 Tide Free Spherical Harmonic Coefficients and its associated Correction Model, at any WGS 84 latitude/longitude coordinate pair listed in a coordinate input file (such as INPUT.DAT), use the FORTRAN harmonic synthesis program, hsynth_WGS84.f.At present, we are also providing two grids of pre-computed geoid undulations: one at 1 x 1-minute resolution and one at 2.5 x 2.5-minute resolution. To interpolate geoid undulations from the 1 x 1-Minute Geoid Undulation Grid file, for any WGS 84 latitude/longitude coordinate pair listed in a coordinate input file (such as INPUT.DAT), use the FORTRAN interpolation program interp_1min.f. Similarly, the FORTRAN interpolation program interp_2p5min.f, will interpolate geoid undulations from the 2.5 x 2.5-Minute Geoid Undulation Grid file.Filenames containing a ".gz" in the suffix have been compressed using the Unix "gzip" command.All files that are SMALL ENDIAN format are highlighted in green. All files that are BIG ENDIAN format are highlighted in purple.Please carefully review the README_WGS84 file for a complete description of coefficient and data files for computing EGM2008 geoid undulations with respect to WGS 84. Also, before using any of the data files on this web page, please read the disclaimer.For GIS data formats, please visit the EGM2008 GIS Data Page.Software and Coefficients for WGS 84 Geoid Undulation Computations by Harmonic Synthesis•EGM2008 Harmonic Synthesis Program(hsynth_WGS84.f -184 KB) - Use this FORTRAN program to generate WGS 84 geoid undulations by spherical harmonic synthesis of EGM2008 and its associated height_anomaly-to-geoid_undulation correction model. This program requires that the coefficients for both EGM2008 and the correction model, and an INPUT.DAT file, all be located in the same directory as the hsynth_WGS84.f program.•Harmonic Synthesis Executable for Windows XP(hsynth_WGS84.exe - 696 KB) - Windows executable version of EGM2008 Harmonic Synthesis Program. Use as described above.•Spherical Harmonic Coefficients for Earth's Gravitational Potential (Tide Free System)-(EGM2008_to2190_TideFree.gz - 72 MB) - EGM2008 coefficients required by the harmonic synthesis program.•Correction Coefficients(Zeta-to-N_to2160_egm2008.gz - 50 MB) -Height_anomaly-to-geoid_undulation coefficients required by the harmonic synthesis program.•INPUT.DAT(4 KB) - sample input file of WGS 84 latitude/longitude coordinate pairs for testing the harmonic synthesis program.•OUTPUT.DAT(4 KB)- sample output file of geoid undulation values, generated by reading INPUT.DAT into the harmonic synthesis program (for test verification).*Users - Please verify results by comparing to OUTPUT.DAT file immediately above. Software and Grids for WGS 84 Geoid Undulation Computation by Interpolation*Note: The 1 x 1 minute interpolation program below requires a large PC RAM capacity.•Interpolation Program for 1 x 1-Minute Geoid Grid(interp_1min.f - 28 KB) - Use this FORTRAN program to interpolate geoid undulations from the 1 x 1-Minute Geoid Undulation Grid file, for any WGS 84 latitude/longitude coordinate pair listed in a coordinate input file (such as INPUT.DAT). When applied to the 1 x 1-Minute Geoid Undulation Grid file, this interp_1min.f program will generate geoid undulation values that match the corresponding values generated by harmonic synthesis (hsynth_WGS84.f or hsynth_WGS84.exe above) to within 1 mm. The interp_1min.f program requires that the 1 x 1-Minute Geoid Undulation Grid file and the INPUT.DAT are located in the same directory as interp_1min.f.• 1 x 1-Minute Grid Interpolation Executable for Windows XP(interp_1min.exe - 434 KB) - Windows executable version of the Interpolation Program for 1 x 1-Minute Geoid Grid. Use as described above. To be used with the SMALL ENDIAN Geoid Undulation Grid below.• 1 x 1-Minute Geoid Undulation Grid in WGS 84 - SMALL ENDIAN (Und_min1x1_egm2008_isw=82_WGS84_TideFree_SE.gz - 825 MB) - 1 x 1-minute global grid of pre-computed geoid undulations. This file has a SMALL ENDIAN internal binary representation.• 1 x 1-Minute Geoid Undulation Grid in WGS 84 - BIG ENDIAN(Und_min1x1_egm2008_isw=82_WGS84_TideFree.gz - 828 MB) - 1 x 1-minute global grid of pre-computed geoid undulations. This file has a BIG ENDIAN internal binary representation.•INPUT.DAT(4 KB) - sample input file of WGS 84 latitude/longitude coordinate pairs for testing the Interpolation Program for 1 x 1-Minute Geoid Grid.•OUTPUT.DAT(4 KB)- sample output file of geoid undulation values, generated by reading INPUT.DAT into the Interpolation Program for 1 x 1-Minute Geoid Grid above (for test verification).*Users - Please verify results by comparing to OUTPUT.DAT file immediately above.•Interpolation Program for 2.5 x 2.5 Minute Geoid Grid(interp_2p5min.f - 28 KB) - Use this FORTRAN program to interpolate geoid undulations from the 2.5 x2.5-Minute Geoid Undulation Grid file, for any WGS 84 latitude/longitude coordinatepair listed in a coordinate input file (such as INPUT.DAT). When applied to the 2.5 x2.5-Minute Geoid Undulation Grid file, this interp_2p5min.f program will generategeoid undulation values that match the corresponding values generated by harmonic synthesis (hsynth_WGS84.f or hsynth_WGS84.exe above) to within 1 cm. The interp_2p5min.f program requires that the 2.5 x 2.5-Minute Geoid Undulation Grid file and the INPUT.DAT are located in the same directory as interp_2p5min.f.• 2.5 x 2.5-Minute Interpolation Executable for Windows XP(interp_2p5min.exe - 434 KB) - Windows executable version of the Interpolation Program for 2.5 x 2.5 Minute Geoid Grid. Use as described above. To be used with the SMALL ENDIAN Geoid Undulation Grid below.• 2.5 x 2.5-Minute Geoid Undulation Grid in WGS 84 - SMALL ENDIAN (Und_min2.5x2.5_egm2008_isw=82_WGS84_TideFree_SE.gz - 135 MB) - 2.5 x2.5-minute global grid of pre-computed geoid undulations. This file has a SMALLENDIAN internal binary representation.• 2.5 x 2.5-Minute Geoid Undulation Grid in WGS 84 - BIG ENDIAN(Und_min2.5x2.5_egm2008_isw=82_WGS84_TideFree.gz - 135 MB) - 2.5 x2.5-minute global grid of pre-computed geoid undulations. This file has a BIG ENDIANinternal binary representation.•INPUT.DAT(4 KB) - sample input file of WGS 84 latitude/longitude coordinate pairs for testing the Interpolation Program for 2.5 x 2.5-Minute Geoid Grid.•OUTPUT.DAT(4 KB)- sample output file of geoid undulation values, generated by reading INPUT.DAT into the Interpolation Program for 2.5 x 2.5-Minute Geoid Grid above (for test verification).*Users - Please verify results by comparing to OUTPUT.DAT file immediately above. Software to Extract WGS 84 Geoid Undulations from Grid Files (No Interpolation)•Extract 1 x 1-Minute Grid Program(gridget_1min.f -20 KB) - Use this FORTRAN program to extract a user-defined sub-rectangle of geoid undulations from the 1 x 1-Minute Geoid Undulation Grid file. This program prompts the user for a rectangular area of interest and requires the 1 x 1-Minute Geoid Undulation Grid file to be located in the same directory as the gridget_1min.f program.•Extract 1 x 1-Minute Grid Executable for Windows XP(gridget_1min.exe - 401 KB) - Windows executable version of the Extract 1 x 1-Minute Grid Program. Use as described above. To be used with the SMALL ENDIAN Geoid Undulation Grid below.• 1 x 1-Minute Geoid Undulation Grid in WGS 84 - SMALL ENDIAN (Und_min1x1_egm2008_isw=82_WGS84_TideFree_SE.gz - 825 MB) - 1 x 1-minute global grid of pre-computed geoid undulations. This file has a SMALL ENDIAN internal binary representation.• 1 x 1-Minute Geoid Undulation Grid in WGS 84 - BIG ENDIAN(Und_min1x1_egm2008_isw=82_WGS84_TideFree.gz - 828 MB) - 1 x 1-minute global grid of pre-computed geoid undulations. This file has a BIG ENDIAN internal binary representation.•Extract 2.5 x 2.5-Minute Grid Program(gridget_2p5min.f - 20 KB) - Use thisFORTRAN program to extract a user-defined sub-rectangle of geoid undulations from the 2.5 x 2.5-Minute Geoid Undulation Grid file. This program prompts the user for a rectangular area of interest and requires the 2.5 x 2.5-Minute Geoid Undulation Grid file to be located in the same directory as the gridget_2p5min.f program.•Extract 2.5 x 2.5-Minute Grid Executable for Windows XP(gridget_2p5min.exe - 397 KB) - Windows executable version of the Extract 2.5 x 2.5-Minute Grid Program.Use as described above. To be used with the SMALL ENDIAN Geoid Undulation Grid below.• 2.5 x 2.5-Minute Geoid Undulation Grid in WGS 84 - SMALL ENDIAN (Und_min2.5x2.5_egm2008_isw=82_WGS84_TideFree_SE.gz - 135 MB) - 2.5 x2.5-minute global grid of pre-computed geoid undulations. This file has a SMALLENDIAN internal binary representation.• 2.5 x 2.5-Minute Geoid Undulation Grid in WGS 84 - BIG ENDIAN(Und_min2.5x2.5_egm2008_isw=82_WGS84_TideFree.gz - 135 MB) - 2.5 x2.5-minute global grid of pre-computed geoid undulations. This file has a BIG ENDIANinternal binary representation.Additional Information•Original Release of the EGM2008 Model Coefficients from EGU General Assembly, Vienna, Austria 2008: Geoscience Package•An Earth Gravitational Model to Degree 2160: EGM2008(NPavlis&al_EGU2008.ppt - 18.5 MB) - Presentation given at the 2008 European Geosciences Union General Assembly held in Vienna, Austria, April13-18, 2008.•Background Papers from Earlier Symposiums on the New EGM。
综合egm2008、剩余地势模型及地面重力数据的大地水准面精化
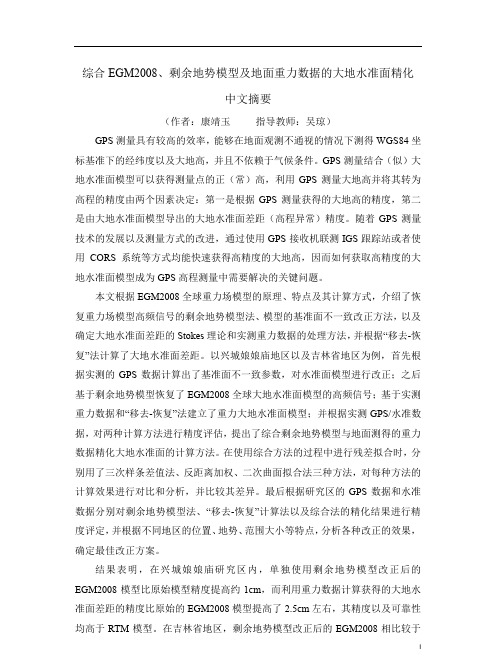
综合EGM2008、剩余地势模型及地面重力数据的大地水准面精化中文摘要(作者:康靖玉指导教师:吴琼)GPS测量具有较高的效率,能够在地面观测不通视的情况下测得WGS84坐标基准下的经纬度以及大地高,并且不依赖于气候条件。
GPS测量结合(似)大地水准面模型可以获得测量点的正(常)高,利用GPS测量大地高并将其转为高程的精度由两个因素决定:第一是根据GPS测量获得的大地高的精度,第二是由大地水准面模型导出的大地水准面差距(高程异常)精度。
随着GPS测量技术的发展以及测量方式的改进,通过使用GPS接收机联测IGS跟踪站或者使用CORS系统等方式均能快速获得高精度的大地高,因而如何获取高精度的大地水准面模型成为GPS高程测量中需要解决的关键问题。
本文根据EGM2008全球重力场模型的原理、特点及其计算方式,介绍了恢复重力场模型高频信号的剩余地势模型法、模型的基准面不一致改正方法,以及确定大地水准面差距的Stokes理论和实测重力数据的处理方法,并根据“移去-恢复”法计算了大地水准面差距。
以兴城娘娘庙地区以及吉林省地区为例,首先根据实测的GPS数据计算出了基准面不一致参数,对水准面模型进行改正;之后基于剩余地势模型恢复了EGM2008全球大地水准面模型的高频信号;基于实测重力数据和“移去-恢复”法建立了重力大地水准面模型;并根据实测GPS/水准数据,对两种计算方法进行精度评估,提出了综合剩余地势模型与地面测得的重力数据精化大地水准面的计算方法。
在使用综合方法的过程中进行残差拟合时,分别用了三次样条差值法、反距离加权、二次曲面拟合法三种方法,对每种方法的计算效果进行对比和分析,并比较其差异。
最后根据研究区的GPS数据和水准数据分别对剩余地势模型法、“移去-恢复”计算法以及综合法的精化结果进行精度评定,并根据不同地区的位置、地势、范围大小等特点,分析各种改正的效果,确定最佳改正方案。
结果表明,在兴城娘娘庙研究区内,单独使用剩余地势模型改正后的EGM2008模型比原始模型精度提高约1cm,而利用重力数据计算获得的大地水准面差距的精度比原始的EGM2008模型提高了2.5cm左右,其精度以及可靠性均高于RTM模型。
MODIS Land Surface Temperature as

MODIS Land Surface Temperature as an index of surface air temperature for operational snowpack estimationEylon Shamir a ,⁎,Konstantine P.Georgakakos a ,ba Hydrologic Research Center,12555High Bluff Drive,Suite 255,San Diego,CA 92130,USA bScripps Institution of Oceanography,UCSD,La Jolla,CA 92037,USAa b s t r a c ta r t i c l e i n f o Article history:Received 26November 2013Received in revised form 26April 2014Accepted 1June 2014Available online 24June 2014Keywords:MODIS LSTLand Surface Temperature Surface air temperature Snow pack Snow 17Regional operational modeling systems that support forecasters for the real-time warning of flash flood events often suffer from lack of adequate real-time surface air temperature data to force their accumulation and ablation snow model.The Land Surface Temperature (LST)product from MODIS,which provides four instantaneous readings per day,was tested for its feasibility to be used in real-time to derive spatially distributed surface air temperature (T a )forcing for the operational snow model.The study was conducted in the Southeast region of Turkey using an atypically dense network of hourly T a ,daily snow depth,snow water equivalent (SWE),and rainfall datasets for the period:October 2002–September 2010.A comparison between the T a and the corre-sponding LST grid-cell data indicated close associations that are different in nature for periods with and without snow on the ground.The LST-derived T a was compared with that obtained from on-site gauge-based interpola-tion procedures and climatological time series.The LST-derived T a was found inferior only to the T a derived from the interpolation of the dense gauge network (31-gauges).Snow-pack simulations using estimated T a time series were compared to simulations that were forced by the observed T a at each site of 18sites.The LST-derived T a performed well in simulating snow mass and maximum SWE magnitude,while it did not represent well the timing of the annual peak of SWE and the duration of spring melt.Our study concluded that the MODIS/LST product can be a valuable additional source of real time forcing data for regional operational snow models,especially in remote mountainous areas with sparse telemetric data.©2014Elsevier Inc.All rights reserved.1.IntroductionSnow accumulation and ablation models that track the snowpack seasonal evolution of the energy and mass balance have been applied routinely worldwide to evaluate the snowpack and melt characteristics.In operational setups which require real-time measurements to repre-sent watershed scale snowpack characteristics,surface air temperature is often a key observed variable that serves as an index for a range of energy fluxes in the atmosphere –snow pack interface and the internal snowpack (e.g.Anderson,1973and 2006).A major uncertainty source in the output of these snow models stems from the commonly insuf fi-cient density of the in-situ meteorological observation network that is required to derive reliable and accurate estimates of energy fluxes and their spatial variability.In mountainous regions with complex terrain and few gauges this uncertainty is expected to be larger (e.g.Bales et al.,2006).In addition,in complex terrain the use of a constant to describe the lapse rate and associate temperature in different elevations adds uncertainty,because in these regions the lapserate varies considerably,being dependent on the synoptic conditions (e.g.Lundquist &Cayan,2007).Snow model sensitivity to surface air temperature (T a )is well demonstrated in Fig.1.The upper panel of the figure compares simula-tions of snow water equivalent (SWE)for a selected meteorological station in Southeastern Turkey using observed and interpolated surface air temperatures from a relatively (and atypically)dense observation network (lower panel).The details about the snow model,air surface temperature dataset and the interpolation procedure will be further discussed in the following sections.Clearly,these two surface air temperature time series yield a substantial difference between the simulations of the snow water equivalent (SWE)during the winter accumulation and spring ablation time.The sensitivity of the snow model to the surface air temperature even during periods with small temperature differences is attributed to the nonlinear behavior of the snow model and the cumulative effect of the error with the progression of the snow season (e.g.Shamir &Georgakakos,2006).This uncertainty associated with the interpolation of surface air temperature is added to the uncertainty associated with the representa-tion of the energy fluxes as functions of surface air temperature to yield the model simulation uncertainty.Remote Sensing of Environment 152(2014)83–98⁎Corresponding author.E-mail address:Eshamir@ (E.Shamir)./10.1016/j.rse.2014.06.0010034-4257/©2014Elsevier Inc.All rightsreserved.Contents lists available at ScienceDirectRemote Sensing of Environmentj o u r n a l h om e p a g e :w w w.e l s e v i e r.c o m /l o c a t e /r s eIn this study we explore the applicability of the Moderate Resolution Imaging Spectroradiometer (MODIS)Land Surface Temperature (LST)product as a source of information to develop real-time surface air temperature forcing for the snow model.Our motivation to use the MODIS LST product as a proxy for surface air temperature is driven by its potential to resolve the finer scale features of variability which are commonly not inferable from in-situ gauge networks in complex terrain.In the following we present a feasibility study conducted in South-east Turkey.To our knowledge,this is one of the handful studies of LST MODIS in snow environment conducted in latitudes lower than 40°N.Following literature review,introduction of the study area,data,and procedures (Section 2)we discuss and evaluate the association between the LST and observed surface air temperature.In Section 3the availability of valid LST reports for the study region is evaluated,and a comparison between surface air temperature derived from the LST product and other commonly available time series is presented.Section 4compares the effect of the interpolated T a on the simulation of snow pack.Study conclusions are provided in Section 5.1.1.Literature reviewEnergy exchange fluxes at the land-surface are largely in fluenced by T a ,LST and their difference.Unlike T a that is commonly measured at a 2m height,LST has not been routinely measured in meteorological stations.In the U.S.for example,less than 2%of the snow measuring automatic stations measure radiant LST (Raleigh,Landry,Hayashi,Quinton,&Lundquist,2013).The complicated interlinks between LST and T a can be demonstrated by examining the energy exchange equation between the land surface and the immediate atmosphere:1−αðÞR s ↓−R L ↑þR L ↓−G ¼H þλEð1Þwhere R S ↓is the incoming shortwave radiation,R L ↓and R L ↑are the incoming and outgoing longwave radiation,respectively,αis the surface albedo,G is the ground heat flux,and H and λE are the sensible and latent heat fluxes,respectively.The units of the energy fluxes are in power per area (e.g.W m −2).All the energy flux terms in Eq.(1)are dependent on LST,T a ,or their difference.The longwave radiation flux association with temperature is explained by the Stephan –Boltzmann's equation,which ascribes R L ↓as a function of T a and the atmospheric emissivity,and R L ↑as a function of LST and the surface emissivity.The magnitude and direction of the turbulent latent and sensible heat fluxes,as seen in the equations below,are controlled by the difference between T a and LST.H ¼C H U T a −LST ðÞð2ÞλE ¼C E U e a −e s ðÞð3Þwhere C H and C E are the bulk transfer coef ficients for heat and moisture,respectively (kJ/m 3°C),U is the wind speed above the surface (m/s),and e s and e a are the vapor pressure (Pa)of the snow surface and the air surface,respectively.Notice that the vapor pressure is monotonically and positively associated with temperature.The ground flux (G )is often estimated as a function of the LST,and the surface albedo is often associated with LST (Jin &Dickinson,2010),which determines the absorbed shortwave radiation at the land surface.As a general rule the land surface warms up in the morning to yield a positive LST −T a difference,whereas during the night LST cools faster and the difference is negative.In dry soil,calm air,and clear sky condi-tions the LST follows the radiation cycle with a short time lag (~1h).In similar conditions for wet soil,the LST is considerably reduced because of latent heat releases from evaporation.Vegetation canopy cover050100150200250300350400450S n o w W a t e r E q u i v a l e n t (m m )-30-20-10010206-Hour IntervalT a i r (o C )Fig.1.SWE simulation using observed (solid black)and interpolated (red)air temperatures for Gauge #17920(2005–2006).The interpolation was based on a network of 31gauges.Lower panel shows the observed (solid black)and interpolated (red)surface air temperatures.84 E.Shamir,K.P.Georgakakos /Remote Sensing of Environment 152(2014)83–98depends on type and the fractional cover changes the albedo and modulates the fraction of shortwave radiation absorbed at the surface. The dominant impact of vegetation however,is associated with its evaporative cooling.Plant canopy actively exchanges absorbed solar radiation through evaporation and thus maintains daytime canopy temperature that is close to the ambient T a(e.g.Nemani,Pierce, Running,&Band,1993).Remotely sensed LST measurements often represent a mixture of soil and vegetation canopy temperatures.The observed portion of soil and vegetation varies with the viewing angle and the fraction and type of vegetation.LST measurements in these mixed areas are also influenced by the lower atmosphere and the temperature difference between the vegetation canopy and the soil background(Weng,Lu,& Schubring,2004).In snow,because of its low thermal conductivity and insulating characteristics,the surface layer quickly reaches temperature equilibri-um with the atmosphere even for large differences between LST and T a(e.g.,Liston,1995).Snow pack LST cannot exceed melting temperature(0.0°C)except for cases of vegetation litter(e.g.,Hardy,Davis,Jordan, Ni,&Woodcock,1998)or dust(e.g.,Painter et al.,2007).During calm nights radiative cooling can reduce the LST below the T a(5–10°C) and cold surface temperature often causes near surface inverse stable conditions that reduces turbulent mixing.Warmer snow surface temperature,as might seem counter intuitive,in general,decreases the snowpack's melting rate.This is because sensible and latent heat fluxes decrease as a consequence of smaller temperature and vapor pressure gradients(see Eqs.2and3),and increase of outgoing longwave radiation(e.g.Sade et al.,2011).During periods of complete areal snow cover it is reasonable to assume that horizontalfluxes between the grid cells are less dominant than the verticalfluxes.However,in patchy snow pack during melt season the intergrid cell differences in vertical energyfluxes between the snow covered and uncovered areas create considerable horizontal fluxes that introduce large uncertainty to the energy balance equation (Liston,1995).The relationship between LST and surface air temperature was stud-ied by many,both from theoretical and empirical perspectives.A few large scale global studies concluded that this relationship is dependent on local variables such as land use/cover,soil moisture,snow cover, frozen ground,regional microclimate conditions,terrain and local landscape features(drexler,Zhao,&Running,2011;Jin& Dickinson,2010;Prihodko&Goward,1997;Sade,Rimmer,Litaor, Shamir,&Furman,2011).1.2.MODIS LSTDetails of the MODIS LST algorithm,its day–night split-window regression,and its theoretical basis can be found in Wan and Dozier (1996),Wan and Li(1997),Wan(2003)and Wan(2008).The MODIS LST product can be made operationally available in near real-time, within hours of the satellite overpass,from the MODIS Rapid Response System(Pinheiro et al.,2007).Land Surface Temperature(LST)is an instantaneous measurement of the skin temperature that is mapped from the radiometric(kinetic) temperature and derived from the thermal IR(TIR)radiation emitted from the land surface.Because TIR measures radiance at the top of the atmosphere,during cloudy conditions the measured signal is from the cloud-top and therefore the MODIS LST is retrieved only in clear-sky conditions.Sensible heatfluxes emitted from the earth surfaces are detected by the MODIS satellite and are used to estimate instantaneous LST.The LST generalized split-window algorithm depends on the MODIS brightness temperature bands(11and12μm channels31 and32)and surface emissivity estimates for these two channels.The emissivity in the thermal infrared is estimated using regression coefficients that depend on land cover classification(MOD12Q1)and daily binary snow cover(MOD10_L2).During snow and ice conditions the emissivity is set to0.993and0.99for bands31and32,respectively (Wan,2008).The algorithm also requires dynamic estimates of the atmospheric transmission,which is derived directly from the MOD07_L2product of vertical atmospheric temperature and vapor profiles and the MODIS cloud masking product(MOD35_L2).In addition to night and day LST estimates,the product also includes information on the emissivity,view-angle,cloud-cover,time of acquisi-tion,and quality-control assessment for each grid cell.Under clear sky conditions the accuracy of the MODIS/LST product was reported to be within1°C for a temperature range of−10to50°C.It was also report-ed to perform better in desert regions(Wan et al.,2004).The performance of MODIS LST in snow and ice covered areas and under clear sky conditions was reported to be accurate within +/−1°C(Wan,Zhang,Zhang,&Li,2002)for a temperature range of −15–0°C(Hall et al.,2008).The accuracy of MODIS LST in snow and ice conditions and temperature below−15°C is still unknown.For these conditions it is reasonable to assume+/−1°C accuracy as report-ed by Wan et al.(2002)(Hall et al.,2008).Although the accuracy is assumed reasonable,Westermann,Langer,and Boike(2012)claim that during clear sky conditions the snow surface temperature is usually colder than during cloudy conditions,and therefore the MODIS LST in snow and ice has a systematic cold bias.MODIS LST was used to detect the thermal regime of permafrost. Several studies conducted in the Arctic found MODIS LST to correspond well with permafrost and the detection of thawing(e.g.,Langer, Westermannb,Heikenfelda,Dorna,&Boikea,2013for the Lena River Deltain in Siberia;Hachem et al.,2012for Northern Quebec,Canada). Hall,Nghiem,Schaaf,DiGirolamo,and Neumann(2009)used the MODIS LST product to detect melting areas in Greenland's ice sheet. Compared with shallow ground based measurements,the LST was found to have better association with surface air temperature and reported to perform better during periods with snow cover(Hachem, Duguay,&Allard,2012).Another study from the Arctic that compared among various LST products derived from polar orbiter satellites con-cluded that the MODIS LST product had the best association with surface air temperature(Urban,Eberle,Hüttich,Schmullius,&Herold,2013). Tight association between monthly MODIS LST and T a was also reported at the Lambert Glacier basin in East Antarctica(Wang,Wang,&Zhao, 2013).All the above described validation studies for LST in snow,ice and permafrost environment were conducted in high latitude relatively homogeneous snow and ice surfaces.However,only a handful of studies evaluated the performance of MODIS LST in mid-latitude mountainous terrain with seasonal snow pack.One example is afield study from the Italian Alps that reported correlation between the skin temperatures of snow and observed surface air temperature to be greater than0.85 and0.89for day and night,respectively(Colombi,De Michele,Pep,& Rampini,2007).This strong association enables derivation of detailed maps for surface air temperature in snow dominated mountainous terrain that can potentially improve the estimation of the spatial variability of melt.2.Data2.1.Study regionThe study was conducted in the Southeast region of Turkey (black thick rectangular outline in Fig.2).The study domain (500km×330km)contains the headwaters of the Euphrates and Tigris Rivers and other east-flowing rivers draining into the Caspian Sea.The domain expands across two continental climate zones:south-eastern Anatolia and Eastern Anatolia.In the western part of the domain is the Anti-Taurus mountain range with average peak elevation at 3000m;while the eastern part near the border with Armenia is the Armenian Highlands mountain range that peaks at Mount Ararat85E.Shamir,K.P.Georgakakos/Remote Sensing of Environment152(2014)83–98(5137m).Turkey's largest lake,Lake Van,is situated in the mountains at an elevation of 1546m.The Southern slopes of the Anti-Taurus Mountains constitute a region of rolling hills and a broad plateau surface that extends into Syria.The region experiences severe winters with frequent heavy snow-fall events and warm summers.The dominant land cover is grasslandwith patches of closed and open shrubland and extended areas of cultivated agricultural land.The MODIS LST product was extensively validated for these dominant land cover of grassland and cultivated agriculture by Wan et al.(2002)and Wan (2008),respectively.Because of therelativelyFig.2.A map of the study area.123456789101112MonthsV a l i d L S T R e p o r t s (F r a c t i o n )31-gauges Oct 2002-Sept 2010Fig.3.Aqua and Terra monthly summary of the valid day and night LST reports (Oct 2002–Sep 2010)for grid-cells that are associated with the 31gauges of surface air temperature.MONTHP E R C E N TAvg. Reports Binary Snow Product: Sept 2002 - Oct 2010Fig.4.Classi fication of the Snow Cover Area (SCA)reports from Aqua into valid reports (red),clouds (green)and no data (black).In addition,the valid reports that indicated snow cover are in blue.86 E.Shamir,K.P.Georgakakos /Remote Sensing of Environment 152(2014)83–98low vegetation canopy,the seasonal snow pack in such landscape is relatively homogeneous.Therefore,it is expected that during periods of snow cover the LST signal will represent well the snow surface temperature as reported in the validation studies mentioned in Section 1.2.2.In situ observationsMeteorological time series of observations for 1October 2002–30September 2010were received from the Turkish State Meteorological Service (TSMS).For the study domain we identi fied 31stations with hourly surface air temperature and daily snow survey information,such as snow water equivalent and snow depth (white triangles in Fig.2).Eighteen of those locations also have daily precipitation records.The range of station elevations is 370–2300m,with a mean average el-evation of 1300m.Most of the stations are located in areas that were identi fied as either cultivated crop or grassland.This dataset represents a fairly dense observation network with an average distance between nearest neighbors equal to 37km (range 11to 80km).Such network density is considered highly dense by operational network standards,especially for high mountains and rugged terrain regions,as in the study domain.2.3.MODIS LST data for the study areaThe LST product from the Aqua and Terra spacecraft MODIS sensors was retrieved from NASA's Earth Observing System Data and Informa-tion System for 1October 2002–30September 2010.We used version 5of the daily Aqua and Terra Land Surface Temperature/Emissivity L3which is available globally at 1km in a sinusoidal projection (MOD11A1and MYD11A1)and use the generalized split-window algorithm (Weng et al.,2004).The study area is covered by a single tile that includes 1200rows and columns (h21v05).Most overpasses for Terra [Aqua]occurred around 11:00and 23:00[13:00and 01:00]local time.2.3.1.LST product availabilityFor the study region and the duration of analysis the LST dataset has 505missing daily products (out of 6574),most having occurred prior to 2005.The availability of valid LST reports,which are cases with actual temperature reports,for the study duration and for the grid-cells that match the 31surface air temperature gauges was summarized by month and is presented in Fig.3.It is seen that valid LST products are available less than 40%of the time during November –April.The availability of valid LST products rises to about 60–80%during June–parison of MODIS LST and 31surface air temperature gauges.Black dots indicate gauges of snow measurement and gray are gauges with measurement of no snow.Red lines are regression estimates and con fidence bounds (5%and 95%)for the snow and no snow separately.87E.Shamir,K.P.Georgakakos /Remote Sensing of Environment 152(2014)83–98September for Aqua and Terra,respectively.We note that only 2%and 0.5%of the missing LST products from both Terra and Aqua lasted longer than 15and 26consecutive days,respectively.Fig.4shows the status of the MODIS snow cover product for the same period and domain available from the Aqua 500m daily snow cover product (MYD10A1)(Hall,Riggs,&Salomonson,1995).As mentioned above,the snow cover product is being used as input to the MODIS LST algorithm.In Fig.4we consider the entire dataset as valid and invalid reports.Valid reports are considered as grid-cells that report snow cover,bare ground,or water;and invalid reports are grid-cells that considered as cloud masking and missing data that are flagged by the quality assessment index.In addition,as a point of reference,we present the percent of snow cover reports (out of the total),which is also part of the valid report category.During November –April,the period with reported snow cover (blue line),the cloud cover was reported in 50–70%of the time (red line).On the other hand,cloud cover decreased considerably during the summer months,and valid daily reports of the product (green line)became abundant.Notice however,that for this period (summer)the number of snow cover reports is marginal (blue line).The black line,which ranges between 8and 12%,summarizes the periods in which the SCA product quality assessment index indicated a problem with-15-10-50510152025Days: 1 Aug 2005 - 31 July 2006T e m p e r a t u r e D i f f e r e n c e (L S T -T a )o CFig.6.An example of annual plot (August 2005–July 2006)of LST,T a ,and snow water equivalent from a gauge that is located in the southeast part of the study domain.The upper panels show the day and night Terra (left)and Aqua (right)LSTs (red and black circles,respectively),day and night gauge air surface temperatures (red and black stars,respectively)and snow water equivalent (cm)(black line).The differences between the LST and the T a are shown for Terra and Aqua (left and right,respectively)and for day and night red and black markers,respectively.88 E.Shamir,K.P.Georgakakos /Remote Sensing of Environment 152(2014)83–98the SCA product.The prevalence of cloud cover in the study region,which obscures the MODIS/SCA product during the snow accumulation season,was previously documented by Tekeli,Akyürek,Şorman,Şensoy,and Şorman (2005and 2006)and Tekeli,Şensoy,Şorman,Akyürek,and Şorman (2006).The analyses presented in Figs.3and 4indicate that the LST product is highly infrequent during the winter,when snow is likely to accumulate,and becomes available with higher frequency during spring –summer (melting period).2.3.2.LST correspondence with surface air temperatureThe correspondence between the LST and gauge surface air temper-ature for the study region is shown in Fig.5.The black [gray]dots in this figure indicate cases in which snow [no snow]was reported at the gauge locations.The red lines show the estimated linear regressions and the 5and 95percentile prediction con fidence intervals,calculated separately for gauges with and without snow.The data in the figure was screened through the quality control procedure that is described in Section 3.3.Although there is an overall monotonic association between the gauges and the LST values,there is signi ficant scatter in the association.It is also seen that the relationships during snow and no snow periods are different as seen by the slopes of the regressions.In general during periods of snow on the ground small changes in LST correspond with a larger range of surface air temperature values.During these periodsthe LST values are below or equal to 0°C,while the surface air temper-ature can possibly rise above freezing temperature.The intra-annual correspondence between LST from Terra and Aqua,gauge surface air temperature,and SWE is further demonstrated in Fig.6,for a high elevation meteorological station (~2300m)in a grass-land dominated land cover at the southeast corner of the study domain.During periods of no snow the LST day –night amplitude brackets the surface air temperature with LST being warmer [colder]than surface air temperature during the day [night](upper panels).During periods with snow measured at the gauge (black solid line),the day –night amplitudes of the LST and surface air temperature were comparable.The LST temperature remained consistently below zero while the surface air temperature occasionally increased above zero.Although snow was measured at the gauge as late as mid-March,the LST increased above zero about a month earlier.The lower panels,which depict the temperature differences between the LST and T a ,show that the daily LST was warmer than T a during no snow periods.This difference ranges 0–20°C with highest differences observed in the summer (June –September).During periods with snow on the ground the daily LST was occasionally colder than the T a .The night LST was consistently colder than T a throughout the year and about an additional 5°C colder than the T a during periods with snow cover.The daily LST values from both sensors were comparable for this location,and although one might expect a warmer Aqua LST because its overpass is closer to the daily maximum short wave irradiance,no-40-200204000 Local time-40-200204006 Local Time200400600-200204060 October 2002 -September 2004 (Days)12 Local Time200400600-40-200204018 Local Time-40-200204000 Local time-200204006 Local Time200400600-20204060October 2002 -September 2004 (Days)200400600-200204018 Local Time-40-200204000 Local time-40-200204006 Local Time-200204060October 2002 -September 2004 (Days) -200204018 Local Time -40-2002000 Local time-40-20204006 Local Time-20204060October 2002 -September 2004 (Days)-40-200204018 Local TimeT e m p e r a t u r e (o C )T e m p e r a t u r e (o C )T e m p e r a t u r e (o C )T e m p e r a t u r e (o C )Fig.7.Two years (1October 2002–30September 2004)of LST and surface air temperature at 00,06,12,and,18hour local time for four selected stations that represent different elevations.89E.Shamir,K.P.Georgakakos /Remote Sensing of Environment 152(2014)83–98distinct differences are apparent.The night time LST however,shows a persistent colder temperature (about 2°C)for the Aqua sensor.Spring LST values that exceeded 0°C although snow was measured at the gauge indicate considerable uncertainty in the association between LST and T a .This uncertainty is likely attributed to the patchi-ness of snow cover that is prevalent during spring times and the classi fication of the LST product as snow-free grid-cells while patches of snow existed in high elevations.During spring it is expected that in-situ snow reports will often misrepresent the patchiness of snow cover at their corresponding grid-cells.In addition,it is expected that during spring time when daily surface air temperature can rise to relatively warm temperatures the snow surface temperature can't rise above freezing.The generalized split-window algorithm for version 5ingests binary snow cover estimates and therefore grid cells that are fractionally covered with snow are assigned to either completely covered or not covered.This in turn increases the uncertainty in the LST estimates and likely to under (over)estimate in cases of low (high)snow fraction-al cover (Rittger,Painter,&Dozier,2012).The recent addition of the fractional snow cover to the MODIS operational products can potentially be incorporated to improve LST estimates in fractionally snow covered grid cells (Dozier,Painter,Rittger,&Frew,2008).Another depiction of the relationship between LST and surface air temperature is provided in Fig.7.Two years of LST and T a daily time series for 0,6,12,and 18hour local time are plotted from four gauges in a grassland environment that were selected in order to represent different elevations.The interpolation of the MODIS LST from the obser-vation times to these hours and for the periods for which LST valid reports are unavailable is described in Section 3.1.The general patterns among these selected gauges are similar except that in the higher-elevation gauges (lower panels)during the winters there are more missing LST values,and the reported LST values for these periods are based on climatological values for their estimates and thus appear as straight lines.The plot further demonstrates theStart t ing g tim m e Oc c tob b er 1 (6-h h our r tim m e int t erv v als)Fig.8.Sensitivity of simulated SWE to biased air temperature (+/−3°C)on each of the three processes:temperature threshold to distinguish between rain and snow (left),snow melt(middle)and snowpack energy balance during non-melt periods (right).-40-2002040MOD 11am-40-2002040MOD 22pm-40-2002040Surface Air Temperature (o C)MYD 13pm-40-2002040MYD 02amM O D I S L S T (o C )Fig.9.Scatter plots of observed hourly surface air temperature from 31gauges and the corresponding LST values for the day and night overpasses from Terra and Aqua satellites.The red dots indicate cases in which the difference between T a and LST exceeds 15°C.90 E.Shamir,K.P.Georgakakos /Remote Sensing of Environment 152(2014)83–98。
基于EGM2008模型确定的昆明地区似大地水准面精化

基于EGM2008模型确定的昆明地区似大地水准面精化作者:胡翀来源:《价值工程》2014年第02期摘要:本文主要介绍了EGM2008模型与利用GPS水准点与EGM2008模型相结合进行大地水准面精化的方法。
结合昆明市呈贡区部分GPS水准点数据在EGM2008模型的基础上利用GPS水准点与EGM2008模型结合计算出高程异常然后采用多项式拟合的方法拟合,最后对拟合后的结果进行了一个检验和精度评估。
Abstract: This paper mainly introduces the model EGM2008 and how to use the GPS leveling point and the model EGM2008 to refine the geoid. With the partial leveing point data of Chenggong District of Kunming and the model EGM2008, we can calculate the height anomaly which is to be used for polynomial fitting, and finally conduct text and accuracy evaluation for the result.关键词:大地水准面精化方法;EGM2008模型;GPS/水准;多项式拟合Key words: refining of geoid;the model EGM2008;GPS/level;the polynomial fitting function中图分类号:P223 文献标识码:A 文章编号:1006-4311(2014)02-0182-020 引言EGM2008模型是由美国国家地理空间情报局(NGA:US National Geospatial-IntelligenceAgency)在奥地利维也纳科学联盟大会上推出的最新一代全球重力场模型[1]。
Temperature dependent Electron Land'e g-Factor and Interband Matrix Element in GaAs
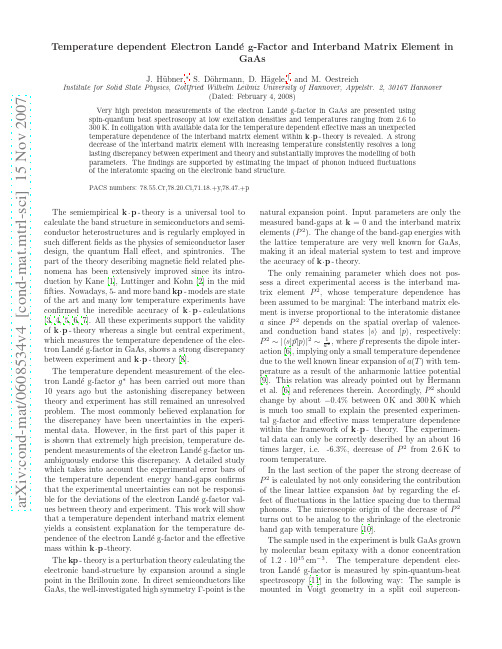
a r X i v :c o n d -m a t /0608534v 4 [c o n d -m a t .m t r l -s c i ] 15 N o v 2007Temperature dependent Electron Land´e g-Factor and Interband Matrix Element inGaAsJ.H¨u bner,∗S.D¨o hrmann,D.H¨a gele,†and M.OestreichInstitute for Solid State Physics,Gottfried Wilhelm Leibniz University of Hannover,Appelstr.2,30167Hannover(Dated:February 4,2008)Very high precision measurements of the electron Land´e g-factor in GaAs are presented using spin-quantum beat spectroscopy at low excitation densities and temperatures ranging from 2.6to 300K.In colligation with available data for the temperature dependent effective mass an unexpected temperature dependence of the interband matrix element within k ·p -theory is revealed.A strong decrease of the interband matrix element with increasing temperature consistently resolves a long lasting discrepancy between experiment and theory and substantially improves the modelling of both parameters.The findings are supported by estimating the impact of phonon induced fluctuations of the interatomic spacing on the electronic band structure.PACS numbers:78.55.Cr,78.20.Ci,71.18.+y,78.47.+pThe semiempirical k ·p -theory is a universal tool to calculate the band structure in semiconductors and semi-conductor heterostructures and is regularly employed in such different fields as the physics of semiconductor laser design,the quantum Hall effect,and spintronics.The part of the theory describing magnetic field related phe-nomena has been extensively improved since its intro-duction by Kane [1],Luttinger and Kohn [2]in the mid fifties.Nowadays,5-and more band k ·p -models are state of the art and many low temperature experiments have confirmed the incredible accuracy of k ·p -calculations [3,4,5,6,7].All these experiments support the validity of k ·p -theory whereas a single but central experiment,which measures the temperature dependence of the elec-tron Land´e g-factor in GaAs,shows a strong discrepancy between experiment and k ·p -theory [8].The temperature dependent measurement of the elec-tron Land´e g-factor g ∗has been carried out more than 10years ago but the astonishing discrepancy between theory and experiment has still remained an unresolved problem.The most commonly believed explanation for the discrepancy have been uncertainties in the experi-mental data.However,in the first part of this paper it is shown that extremely high precision,temperature de-pendent measurements of the electron Land´e g-factor un-ambiguously endorse this discrepancy.A detailed study which takes into account the experimental error bars of the temperature dependent energy band-gaps confirms that the experimental uncertainties can not be responsi-ble for the deviations of the electron Land´e g-factor val-ues between theory and experiment.This work will show that a temperature dependent interband matrix element yields a consistent explanation for the temperature de-pendence of the electron Land´e g-factor and the effective mass within k ·p -theory.The k ·p -theory is a perturbation theory calculating the electronic band-structure by expansion around a single point in the Brillouin zone.In direct semiconductors like GaAs,the well-investigated high symmetry Γ-point is thenatural expansion point.Input parameters are only the measured band-gaps at k =0and the interband matrix elements (P 2).The change of the band-gap energies with the lattice temperature are very well known for GaAs,making it an ideal material system to test and improve the accuracy of k ·p -theory.The only remaining parameter which does not pos-sess a direct experimental access is the interband ma-trix element P 2,whose temperature dependence has been assumed to be marginal:The interband matrix ele-ment is inverse proportional to the interatomic distance a since P 2depends on the spatial overlap of valence-and conduction band states |s and |p ,respectively:P 2∼| s | p |p |2∼12 ducting magnet and excited with circular polarized lightpulses from an80MHz picosecond laser.The sampletemperature is varied from2.6K to room temperature,whereas the excess-energy of the exciting light is about6meV above the direct band gap for temperatures upto80K.At higher temperatures,the contribution of theexcess energy is negligible compared to the thermal en-ergies present in the sample lattice[12].The excitedcarrier densities amount to about6·1015cm−3.The pho-toluminescence from the sample is collected in backwarddirection.Energy-and time-resolution is performed bya spectrometer followed by a synchroscan streak cam-era.The electron Land´e g-factor is deduced from theoscillating time evolution of the cross circular polarizedcomponent of the photoluminescence via the relationg∗=ωL /(µB B),withωL being the Larmor precessionfrequency of the conduction electron spins,µB Bohr’smagneton,and B the magneticfield.Great care has been taken in the time calibration ofthe detection system as well as in the correct determina-tion of the magneticfield present in the superconductingmagnet.Superconducting magnets can often show unap-parent remanencefields and incorrectfield calibrationswhich easily influence the experimental data.There-fore the magneticfield is calibrated with a precise Hallsensor for all appliedfields.The often cited value ofg∗=−0.44±5%[13]in GaAs applies for donor boundelectrons,whereas for the free conduction band electronshigher g-factor values are reported[14,15].From thedata presented here a very high accuracy extrapolatedelectron Land´e g-factor of g∗=−0.484±0.7%at T=0K,B=0T,and P exc.=0mW is determined.Figure1shows the electron Land´e g-factor versus sam-ple temperature.Each value is extrapolated to zero mag-neticfield and zero excitation power from measurementsat differentfields and excitation powers at constant tem-perature to eliminate any residual effects of those entitieson the g-factor.The measurements from2.6to62K arecarried out with alternating excitation ofσ+-andσ−-light and a small tilt of the sample against the magneticfield.This technique enables us to monitor and subtractthe influence of the effective nuclearfield on the electronLand´e g-factor measurement(see Ref.[16]for details).The experimental results are compared with estab-lished k·p-theory.The central5-level k·p-result forg∗and m∗was presented by Hermann et al.[6]:g∗3…1E(Γc6−Γv8)+∆0«−P′2E(Γc7−Γc6)−1m∗=1+P2E(Γc6−Γv8)+1 3…1E(Γc8−Γc6)«+C(2)Here,g0=2.0023is the free electron Land´e g-factor,m0electrong-factortemperature [K]FIG.1:High precision measurement of the temperature de-pendence of the electron Land´e g-factor in bulk GaAs(filled circles).The red solid line is afit of the experimental data by Eq.1with a strong temperature dependent interband ma-trix element.The green dashed line is the calculated g∗with P2(T)depending only on the anharmonic lattice expansion.the free electron mass,and E(Γv8,Γc6,Γc7,Γc8)and∆0are the energies of the band extrema at the center of the Brillouin zone and the split-offenergy of theΓv7valence band,respectively.The contributions from higher bands are summarized in the constants C′=−0.02and C=−2 [6].The temperature dependence of the band-gap ener-gies E(T)are well known by experiment and described by the semi-phenomenological model introduced by Vi˜n a et al.[17]E(T)=E B−αB1+2k B T ek B T e.(4)The integration starts at the minimum of the conduc-tion band,D3D(E)=1/2π2 2m∗/ 2 3/2√TABLE I:Parameters usedE BαBΘeV meV K Ref.of P2.Now,P2(T)is estimated by taking into account the statistics of phonon induced lattice elongations.The effective average lattice distance is stretched by the vari-ance of the mean squared strain(MSS)due to the adia-batic following of the electrons:a eff=a(T)(1+σMSS). Here the MSS is given byσMSS= ∂x u and u′2 =1Mω2u20.Hence,the MSS can be written as2u′2 =1v2s Z(ω) 2 dω(5)where n(ω)is the Bose-Einstein distribution n(ω)= (eβω−1)−1,β= /k B T,and M=M1+M2is the mass of the two atomic basis in the fcc lattice.The 3D Debye density of states in the unit volume V in the continuum approximation for one phonon polarization is: Z(ω)=V14π2v5s Ming length.Acc.to Eq.3P2changes starting from≈40K.[25]Landolt-B¨o rnstein,Group III Condensed Matter,vol.41,(Springer,2001).[26]−ζ4+ζ2P∞l=1(−lζ+3)e lζl3−e lζ15。
基于EGM2008模型的小区域似大地水准面模型构建与优化

基于EGM2008模型的小区域似大地水准面模型构建与优化摘要:本文阐述利用EGM2008模型构建优化区域似大地水准面,利用EGM2008的2190高阶模型,结合区域已知水准成果,进行模型的拟合优化,针对模型误差较大的区域,进行实地几何水准测量,改善误差较大区域的水准成果。
利用EGM2008模型构建的正常高成果精度为±13.7cm,利用水准改进的2次模型优化,正常高成果精度为±4.8cm,满足一般工程测量的应用。
关键字:EGM2008;似大地水准面;模型优化;高程异常Abstract: this paper using EGM2008 model building optimization area like the geoid, the use of EGM2008 2190 high order model, in combination with regional known level results, the fitting model optimization, in view of the model error larger area, field geometry level measurement, improve the level of the large area error results. EGM2008 model is built using the normal GaoChengGuo accuracy of + /-13.7 cm, use level improvement 2 times model optimization, normal GaoChengGuo accuracy of + /-4.8 cm, meet general engineering measurement applications.Keyword: EGM2008; Like the geoid; Model optimization; Abnormal height 引言似大地水准面模型在全国多地已经开展,国内应用较多的是利用武汉大学李建成院士的移去恢复法,利用WDM94等重力模型构建的省域似大地水准面模型,利用该法可有效建立高精度区域似大地水准面。
基于EGM2008和SRTM模型确定的新疆地区似大地水准面及其精度评估
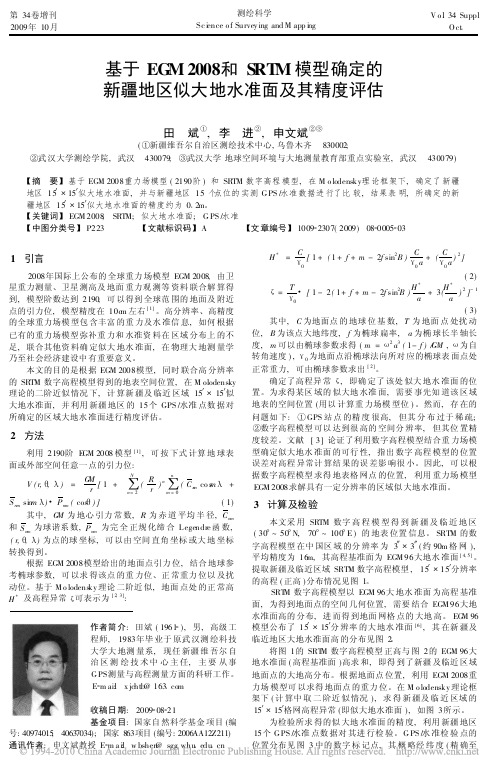
&
N
&
n
( 2) * * T H H = ∋ [ 1 - 2 ( 1 + f + m - 2f sin2B ) + 3( ) 2 ]- 1 ! a a 0 ( 3) 其中 , C 为地面点 的 地球 位 基 数 , T 为 地面 点 处扰 动 位 , B 为该点大地纬度 , f 为椭球 扁率 , a 为椭 球长半 轴长 度 , m 可以由椭球参数求得 ( m = ∀ 2 a3 ( 1 - f ) /GM , ∀ 为自 转角速度 ) , !0 为地面点沿椭球法向所对 应的椭球表 面点处 正常重力 , 可由椭球参数求出 [ 2] 。 确定了高程异 常 , 即确 定了 该处 似大 地水 准面 的位 置。为求得某区 域的 似大地 水准 面 , 需要 事先知 道该 区域 地表的空间位置 ( 用以 计算重 力场模 型位 ) 。然而 , 存 在的 问题如 下 : GPS 站 点 的精 度 很高 , 但其 分 布 过于 稀 疏 ; 数字高程模型 可以 达到很 高的 空间分 辨率 , 但其位 置精 度较差。文献 [ 3] 论证了利用数字高程模型结合重 力场模 型确定似大地水 准面 的可行 性 , 指出 数字 高程 模型的 位置 误差对高程 异常计 算结 果的误 差影 响很 小。因此 , 可 以根 据数字高程模型 求得 地表格 网点 的位置 , 利用 重力场 模型 EGM 2008 求解具有一定分辨率的区域似 大地水准面。
[ 2] [ 3]
[ 4] 分 ) 见表 1 。根据 15∃ % 15∃ 似大地 水准面 的高程 异常网 格点 分布值 , 利用三 次样 条插 值得 到 15 个 GPS /水准 检验 点处 的高程异常 , 此 为计 算值。检 验点的 大地 高与正 常高 的差 值作为实测值 , 计算值 与实 测值的 比较 见表 1 。 15 个 检验 点处高程异常值的残 差统计分析见表 2 。 由表 2 可知 , 基于 EGM 2008 重力 场模型和 SRTM 高程 模型求得新疆 地区 似大 地水 准面 , 与 15 个 GPS /水准 检验 点的平均差异为 0 11m, 精度为 0 23m。 [ 5]
- 1、下载文档前请自行甄别文档内容的完整性,平台不提供额外的编辑、内容补充、找答案等附加服务。
- 2、"仅部分预览"的文档,不可在线预览部分如存在完整性等问题,可反馈申请退款(可完整预览的文档不适用该条件!)。
- 3、如文档侵犯您的权益,请联系客服反馈,我们会尽快为您处理(人工客服工作时间:9:00-18:30)。
Received: 16 November 2009 / Accepted: 18 May 2010 / Published online: 11 June 2010 © Springer-Verlag 2010
Abstract This paper investigates the normal-orthometric correction used in the definition of the Australian Height Datum, and also computes and evaluates normal and Helmert orthometric corrections for the Australian National Levelling Network (ANLN). Testing these corrections in Australia is important to establish which height system is most appropriate for any new Australian vertical datum. An approximate approach to assigning gravity values to ANLN benchmarks (BMs) is used, where the EGM2008-modelled gravity field is used to ‘re-construct’ observed gravity at the BMs. Network loop closures (for first- and second-order levelling) indicate reduced misclosures for all height corrections considered, particularly in the mountainous regions of south eastern Australia. Differences between Helmert orthometric and normal-orthometric heights reach 44 cm in the Australian Alps, and differences between Helmert orthometric and normal heights are about 26 cm in the same region. Normalorthometric heights differ from normal heights by up to 18 cm in mountainous regions >2,000 m. This indicates that the quasigeoid is not compatible with normal-orthometric heights in Australia. Keywords AHD Height systems · EGM2008 · Vertical datums ·
123
502 Fig. 1 The Australian National Levelling Network (ANLN). First-order sections are in yellow, second-order sections in light green, third-order in thin grey, fourth-order in dark green, one-way (third-order) in reபைடு நூலகம் and two-way (order undefined; Steed 2006, pers. comm.) in blue. Lambert projection. ANLN data courtesy of Geoscience Australia
J Geod (2010) 84:501–513 DOI 10.1007/s00190-010-0388-0
ORIGINAL ARTICLE
The effect of EGM2008-based normal, normal-orthometric and Helmert orthometric height systems on the Australian levelling network
M. S. Filmer (B) · W. E. Featherstone · M. Kuhn Western Australian Centre for Geodesy, The Institute for Geoscience Research, Curtin University of Technology, GPO Box U1987, Perth, WA 6845, Australia e-mail: M.Filmer@.au W. E. Featherstone e-mail: W.Featherstone@.au M. Kuhn e-mail: M.Kuhn@.au
1 Introduction The Australian Height Datum (AHD) is Australia’s first and only official height datum. On the mainland, it was established in 1971 from the least-squares adjustment [fixed to mean sea level (MSL) = zero at 30 tide gauges] of the (then named) Australian Levelling Survey (ALS) comprising ∼97,000 km of ‘primary’ levelling (Roelse et al. 1971). Due to the lack of computing power at that time, the primary network was adjusted on a State-by-State basis before the full national adjustment was conducted. Approximately 80,000 km of ‘supplementary’ levelling were subsequently least-squares adjusted onto the primary junction point (JP) heights (held fixed), which were defined in the full national adjustment (Roelse et al. 1971). The Australian National Levelling Network (ANLN) in Tasmania was adjusted in 1983 (NMC 1986), with MSL at two tide gauges held fixed at zero in the adjustment. An offset between AHD(mainland) and AHD(Tas) thus exists which is estimated to be 10–20 cm (Rizos et al. 1991; Featherstone 2000). A number of issues have arisen with regard to the AHD, with numerous studies investigating different deficiencies in the datum (e.g., Featherstone 2001). The major areas of concern in the AHD include the fixing of the levelling network (now referred to as ANLN; see Fig. 1) to 30 tide gauges using MSL as the zero reference (e.g., Hamon and Greig 1972; Mitchell 1973b; Coleman et al. 1979), the quality of the levelling (e.g., Morgan 1992; Kearsley et al. 1993; Filmer and Featherstone 2009) and the omission of gravity-based height corrections (e.g., Mitchell 1973a; Allister and Featherstone 2001). The levelling errors in the ANLN (data provided by Geoscience Australia; GA; G. Johnston pers. comm. 2007) are particularly problematic. The cause of the largest regional distortions in the AHD is gross levelling errors, chiefly in
M. S. Filmer et al.
Kilometers
0 250 500 1,000 1,500 2,000
the interior of the continent (Filmer and Featherstone 2009). The magnitude of the above-tolerance loop misclosures often exceeds 0.5 m in central Australia, with the maximum reaching 0.93 m. Note that the perimeter length of some of these loops is >2,000 km (Fig. 1). When the AHD was defined, insufficient gravity observations were available to apply gravimetric height corrections. Instead, a truncated version of the so-called normal-orthometric correction of Rapp (1961) was applied; there is no requirement for observed gravity whatsoever in this correction. As such, the AHD should be considered a normalorthometric height system (Holloway 1988; Featherstone and Kuhn 2006). However, sufficient gravity data now exist in Australia to retrospectively investigate the effects of gravimetric height corrections to the ANLN (cf. Mitchell 1973a). The problems with the AHD have become considerably more apparent with the emergence of GNSS heighting and regional gravimetric quasigeoid models (e.g., Featherstone 2008). Various methods of overcoming the incompatibility of GNSS-derived ellipsoid heights and AUSGeoid98 (Featherstone et al. 2001) with the AHD have been investigated (e.g., Featherstone 1998; Featherstone and Sproule 2006; Soltanpour et al. 2006). However, the long-term solution to this