Recognition of Traffic Signs based on Vision Models(基于视觉模型的交通信号辨别)
基于改进YOLOX的交通标志检测与识别
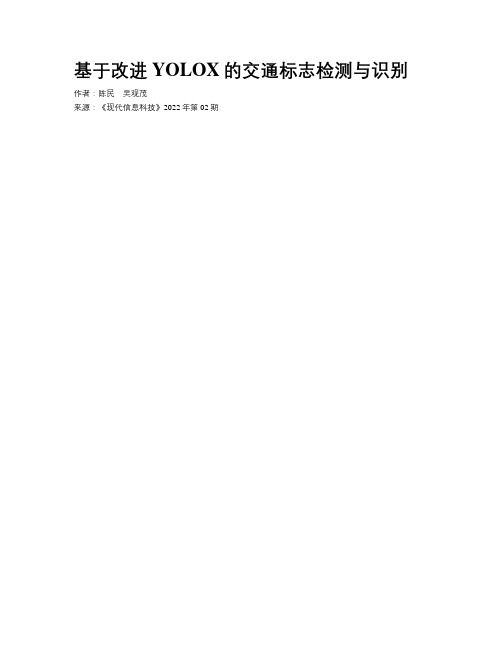
基于改进YOLOX的交通标志检测与识别作者:陈民吴观茂来源:《现代信息科技》2022年第02期摘要:现实中交通标志的检测和识别具有环境多变的特点,交通标志长时间暴露在外经常会出现损坏情况,对检测的精度和速度产生较大影响。
利用最新的YOLO系列算法——YOLOX,对网络结构的加强特征提取层进行改进,引入OPA-FPN网络,相较于原来的PANet 网络,后者精度提升2.2%。
在交通标志识别过程,对经典的卷积神经网络模型LeNet-5进行改进,在数据集TT100K中进行实验,相较于其他交通标志识别模型,使用改进的模型可以使识别正确率提升2.31%,识别时间减少了13.02 ms。
关键词:单步路径聚合网络;YOLO;卷积神经网络;FPN;LeNet-5中图分类号:TP273+.4 文献标识码:A文章编号:2096-4706(2022)02-0101-04Abstract: In reality, the detection and recognition of traffic signs have the characteristics of changeable environment. Traffic signs are often damaged after being exposed for a long time, which has a great impact on the accuracy and speed of detection. Using the latest YOLO series algorithm—YOLOX, the enhanced feature extraction layer of the network structure is improved, and the OPA-FPN network is introduced. Compared with the original PANet network, the accuracy of the latter is improved by 2.2%. In the process of traffic sign recognition, the classical convolutional neural network model LeNet-5 is improved, experiments are carried out in the data set TT100K. Compared with other traffic sign recognition models, using the improved model can improve the recognition accuracy by 2.31% and reduce the recognition time by 13.02 ms.Keywords: single-step path aggregation network; YOLO; convolutional neural network; FPN; LeNet-50 引言随着城市化的发展,人们的生活质量日益提高,道路上车辆的数量也远超过从前,无人驾驶作为极具发展前景的技术也逐步进入百姓生活中。
交通安全交通标志我知道作文

交通安全交通标志我知道作文(中英文版)Traffic Safety: The Importance of Traffic SignsIn our daily lives, traffic signs play a pivotal role in ensuring our safety on the road.These humble, colorful symbols are more than just decorations; they are essential tools that guide, warn, and regulate traffic flow.Understanding and respecting traffic signs is crucial for every individual, as they contribute significantly to the harmony and security of our transportation system.交通安全:交通标志的重要性在我们的日常生活中,交通标志在确保道路安全方面发挥着至关重要的作用。
这些不起眼的彩色标志不仅仅是装饰品,更是指导、警示和规范交通流的重要工具。
每个个体都应理解和尊重交通标志,因为它们对交通系统的和谐与安全贡献巨大。
Varying in shapes, sizes, and colors, traffic signs convey different messages to road users.For instance, a red octagon signals a mandatory stop, ensuring that drivers slow down and check for crossing pedestrians or oncoming traffic.Conversely, a green circle with a white arrow indicates the direction in which vehicles are allowed to proceed, thus preventing confusion and potential accidents.交通标志形状、大小和颜色各异,向道路使用者传递不同的信息。
交通标志论文:自然场景下交通标志图像识别方法研究

交通标志论文:自然场景下交通标志图像识别方法研究【中文摘要】随着社会进步和经济的发展,我国的公路交通行业得到了持续、快速地发展。
高度发达的现代交通为人类的生活带来了便利,但同时交通安全、交通拥挤等问题也变得越来越严重。
为了解决这些问题,智能交通系统ITS (Intelligent Traffic System) 这一研究领域应运而生。
道路交通标志识别系统TSR (Traffic Sig ns Recognition)作为智能交通系统研究方向,已成为国内外学者研究的热点之一,它通过安装在机动车辆上的摄像机摄取自然场景图像,并将图像送至系统的图像处理模块进行图像理解、交通标志检测与识别最后将识别结果告知驾驶员,以达到增强道路交通安全、降低交通拥挤的。
道路交通标志中,警告标志、禁令标志和指示标志是三种最重要、也是最常见的交通标志,它们均具有特定的颜色和形状用以区分其他物体,以达到提醒驾驶员或行人的。
十几年以来,道路交通标志识别研究有了很好的进展,并取得了一定的研究成果,但因背景复杂性以及光照等各种影响因素的存在,导致了它比非自然场景下的目标识别更具有挑战性,其影响因素主要表现在以下方面:光照条件时常变换且不可控、车辆震动导致摄取的图像模糊、交通标志被损坏、被污染或被遮挡、交通标志颜色褪色、雨雾等恶劣天气的存在,以及投影失真、尺度变换、倾斜、相同颜色背景等。
从交通标志的颜色信息和形状特征出发,研究一种交通标志的智能检测算法。
该算法主要包括基于HSV(Hue-Saturation-Values) 颜色空间的交通标志图像分割和基于颜色与形状的交通标志检测两部分。
首先将RGB(Red-Green-Blue) 图像转换至受光照影响较小的HSV颜色空间,通过提取不同颜色的阈值范围来定位目标区域;再根据目标区域的几何形状来划分警告、禁令和指示三种不同类别的交通标志,完成交通标志图像的检测。
针对自然场景下影响交通标志检测效果的不利因素,研究了基于多尺度Retinex 的交通标志图像增强和仿射变换的三角形交通标志校正算法,以及规范化圆形交通标志和矩形交通标志的算法。
复杂情况下交通标志的检测与识别
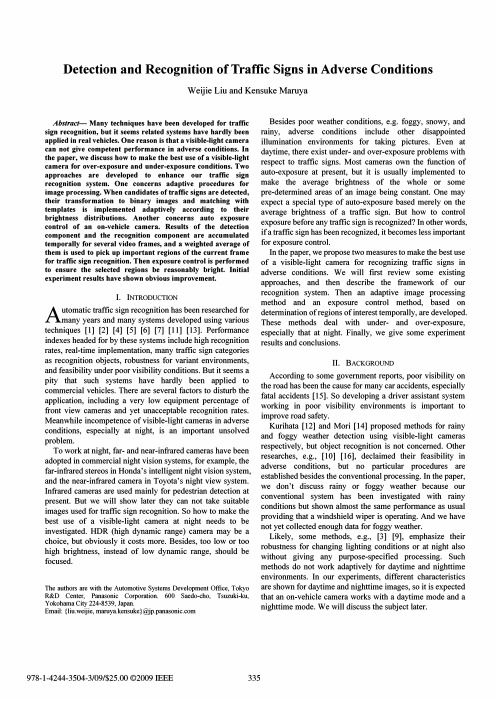
Abstract- Many techniques have been developed for traffic sign recognition, but it seems related systems have hardly been applied in real vehicles. One reason is that a visible-light camera can not give competent performance in adverse conditions. In the paper, we discuss how to make the best use of a visible-light camera for over-exposure and under-exposure conditions. Two approaches are developed to enhance our traffic sign recognition system. One concerns adaptive procedures for image processing. When candidates of traffic signs are detected, their transformation to binary images and matching with templates is implemented adaptively according to their brightness distributions. Another concerns auto exposure control of an on-vehicle camera. Results of the detection component and the recognition component are accumulated temporally for several video frames, and a weighted average of them is used to pick up important regions of the current frame for traffic sign recognition. Then exposure control is performed to ensure the selected regions be reasonably bright. Initial experiment results have shown obvious improvement.
复杂环境下交通标志牌的检测和识别
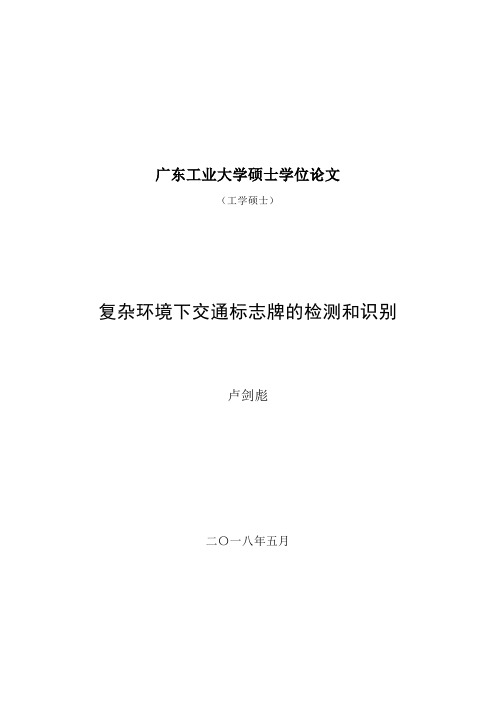
广东工业大学硕士学位论文(工学硕士)复杂环境下交通标志牌的检测和识别卢剑彪二〇一八年五月分类号:学校代号:11845 UDC:密级:学号:2111505003广东工业大学硕士学位论文(工学硕士)复杂环境下交通标志牌的检测和识别卢剑彪校内导师姓名、职称: 谭台哲副教授学科(专业)或领域名称: 计算机科学与技术学生所属学院: 计算机学院论文答辩日期: 二〇一八年五月A Dissertation Submitted toGuangdong University of Technologyfor the Master Degree of Engineering ScienceDetection and Recognition of Traffic Sign in ComplexEnvironmentCandidate: Jianbiao LuSupervisor: Prof. Taizhe TanMay 2018School of Computer Science and TechnologyGuangdong University of TechnologyGuangzhou, Guangdong, P. R. China, 510006摘要交通标志检测与识别作为智能交通系统重要的一个环节,对于发展无人驾驶具有十分重要的意义。
在实时道路交通中,车载摄像机拍摄获取的场景图像,部分交通标志可能被周围的障碍物遮挡,受到的光照强度发生改变以及因运动而导致交通标志模糊等因素,这些都加大了智能交通系统中交通标志检测与识别的难度。
针对这些问题,提出了使用一种卷积神经网络的算法对交通道路环境下的交通标志进行检测与识别,以便提高交通标志的检测速率和识别率。
本文以我国道路交通标志为研究对象,主要包含三大类的交通标志:警告标志、禁止标志、指示标志。
在自然场景中,车载摄像机连续拍摄获取的高清分辨率的道路交通标志进行检测与识别分类展开研究。
辨认交通标志英语作文
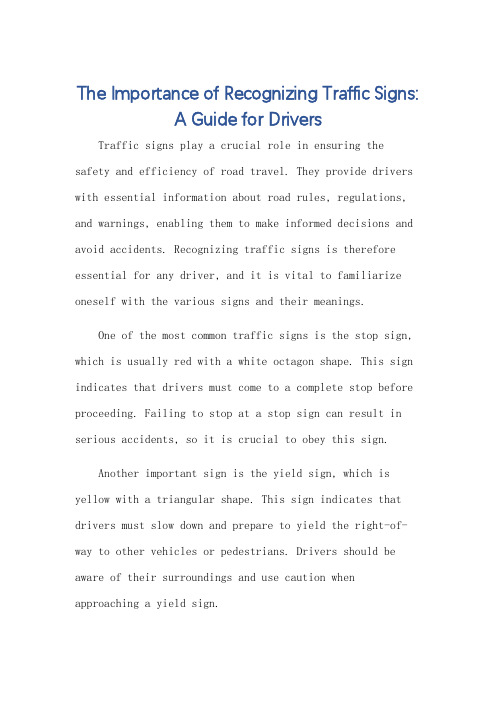
The Importance of Recognizing Traffic Signs:A Guide for DriversTraffic signs play a crucial role in ensuring the safety and efficiency of road travel. They provide drivers with essential information about road rules, regulations, and warnings, enabling them to make informed decisions and avoid accidents. Recognizing traffic signs is therefore essential for any driver, and it is vital to familiarize oneself with the various signs and their meanings.One of the most common traffic signs is the stop sign, which is usually red with a white octagon shape. This sign indicates that drivers must come to a complete stop before proceeding. Failing to stop at a stop sign can result in serious accidents, so it is crucial to obey this sign.Another important sign is the yield sign, which is yellow with a triangular shape. This sign indicates that drivers must slow down and prepare to yield the right-of-way to other vehicles or pedestrians. Drivers should be aware of their surroundings and use caution when approaching a yield sign.Speed limit signs are also crucial for drivers to recognize. These signs indicate the maximum speed allowed on a particular road or section of road. Drivers should always adhere to the speed limit to ensure their safety and the safety of other road users. Exceeding the speed limit can lead to serious consequences, including fines, license suspension, and even imprisonment.Warning signs are also important for drivers to pay attention to. These signs warn drivers of potential hazards or dangers ahead, such as sharp turns, narrow bridges, or slippery roads. Drivers should take extra care when approaching warning signs and be prepared to adjust their speed or take evasive action if necessary.In addition to these common signs, there are many other types of traffic signs that drivers need to be aware of. These include directional signs, which indicate the direction to various destinations; regulatory signs, which provide rules and regulations for road use; and information signs, which provide useful information about road conditions, services, and attractions.Recognizing traffic signs is not only essential for drivers' safety but also for the safety of other road users. By familiarizing oneself with the various signs and their meanings, drivers can make informed decisions, avoid accidents, and contribute to a safer and more efficientroad environment. Therefore, it is crucial for drivers to take the time to learn and understand the different typesof traffic signs and their meanings.**辨认交通标志的重要性:司机指南**交通标志在确保道路旅行的安全和效率方面发挥着至关重要的作用。
交通标识的英语作文
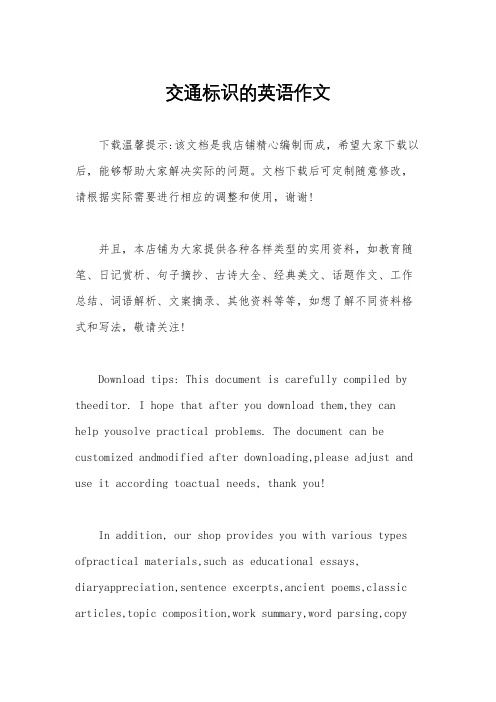
交通标识的英语作文下载温馨提示:该文档是我店铺精心编制而成,希望大家下载以后,能够帮助大家解决实际的问题。
文档下载后可定制随意修改,请根据实际需要进行相应的调整和使用,谢谢!并且,本店铺为大家提供各种各样类型的实用资料,如教育随笔、日记赏析、句子摘抄、古诗大全、经典美文、话题作文、工作总结、词语解析、文案摘录、其他资料等等,如想了解不同资料格式和写法,敬请关注!Download tips: This document is carefully compiled by theeditor. I hope that after you download them,they can help yousolve practical problems. The document can be customized andmodified after downloading,please adjust and use it according toactual needs, thank you!In addition, our shop provides you with various types ofpractical materials,such as educational essays, diaryappreciation,sentence excerpts,ancient poems,classic articles,topic composition,work summary,word parsing,copyexcerpts,other materials and so on,want to know different data formats andwriting methods,please pay attention!Sure, here's a snippet of an English essay abouttraffic signs, keeping in mind that it's a randomcollection of phrases without a clear structure or logical progression:1. At the heart of every bustling city, traffic signs hold the key to urban harmony. They're like silent conductors, guiding drivers through the maze of roads,their simple designs whispering rules to the unsuspecting traveler.2. The first one you'll encounter is the ubiquitous "yield," a friendly reminder to give way when you're about to enter a busy intersection. It's like a traffic version of "slow down, kids!"3. Then, there's the "stop" sign, a stern directivethat halts all progress, like a red traffic light in a busy intersection. It's a moment of pause, a chance to assessthe situation and respect the right of others.4. The "speed limit" sign, oh how it whispers the whispers of caution. It's a whisper, not a shout, reminding drivers to keep their pace within the allowed range, like a gentle breeze on a hot summer road.5. The "yield to pedestrians" sign is a gentle reminder to give way to those on foot, a nod to the human element in the urban jungle. It's a moment of shared space, a symbol of urban civility.6. And don't forget the "one-way" arrow, a clear directive that sets the direction of traffic, like atraffic arrow pointing the way forward, ensuring a smooth flow of vehicles.7. Each sign, a tiny puzzle piece in the grand urban puzzle, contributes to the efficient functioning of our roads. They're not just signs, they're silent teachers, teaching us to navigate life's daily commute with respect and awareness.Remember, this is a snapshot, and a complete essay would require more context and development, but this should give you a flavor of how you could structure an English essay about traffic signs in an informal, conversational tone.。
辨认交通标志英语作文
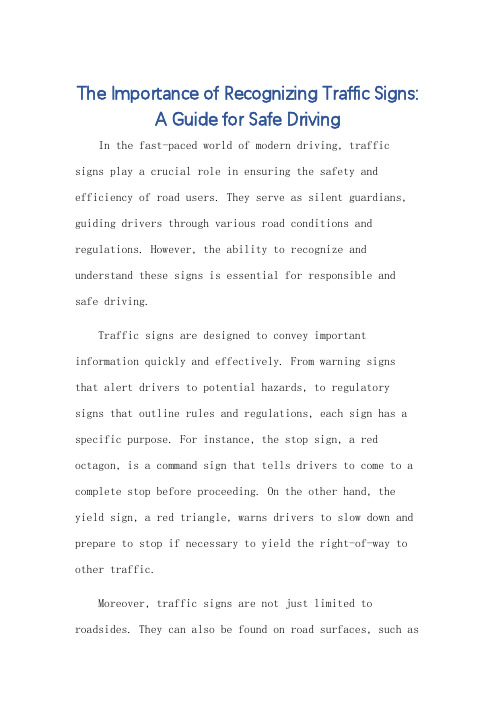
The Importance of Recognizing Traffic Signs:A Guide for Safe DrivingIn the fast-paced world of modern driving, traffic signs play a crucial role in ensuring the safety and efficiency of road users. They serve as silent guardians, guiding drivers through various road conditions and regulations. However, the ability to recognize and understand these signs is essential for responsible and safe driving.Traffic signs are designed to convey important information quickly and effectively. From warning signsthat alert drivers to potential hazards, to regulatory signs that outline rules and regulations, each sign has a specific purpose. For instance, the stop sign, a red octagon, is a command sign that tells drivers to come to a complete stop before proceeding. On the other hand, the yield sign, a red triangle, warns drivers to slow down and prepare to stop if necessary to yield the right-of-way to other traffic.Moreover, traffic signs are not just limited to roadsides. They can also be found on road surfaces, such aspavement markings, which indicate various drivingdirectives like parking spaces, stop lines, and lane markings. These markings are crucial for maintaining the flow of traffic and ensuring safety.Recognizing traffic signs is not just about memorizing their shapes and colors. It's about understanding their meaning and applying that knowledge in real-time driving situations. For instance, drivers need to be able to interpret the meaning of a yield sign and act accordingly, slowing down and preparing to stop if necessary. Similarly, drivers must be aware of the different speed limit signs and adjust their speed accordingly to ensure safe driving. However, with the ever-changing landscape of traffic regulations and the introduction of new signs, it's important for drivers to stay updated and informed. This can be achieved by taking driving courses, reading driving manuals, and staying attentive to road signs while driving. By doing so, drivers can ensure that they are well-equipped to handle various road conditions and situations.In conclusion, recognizing traffic signs is an integral part of safe and responsible driving. It's aboutunderstanding the language of the road and applying that knowledge to make informed decisions while on the road. By staying updated, attentive, and respectful of traffic signs, drivers can ensure a safer and smoother driving experience for themselves and other road users.**辨认交通标志的重要性:安全驾驶指南**在现代驾驶的快节奏世界中,交通标志在确保道路使用者安全和效率方面发挥着至关重要的作用。
- 1、下载文档前请自行甄别文档内容的完整性,平台不提供额外的编辑、内容补充、找答案等附加服务。
- 2、"仅部分预览"的文档,不可在线预览部分如存在完整性等问题,可反馈申请退款(可完整预览的文档不适用该条件!)。
- 3、如文档侵犯您的权益,请联系客服反馈,我们会尽快为您处理(人工客服工作时间:9:00-18:30)。
Recognition of Traffic Signs based on Vision ModelsXiaohong Gao, Kunbin Hong, Lubov Podladchikova*, Dmitry Shaposhnikov*, Natalia Shevtsova*,Peter PassmoreSchool of Computing Science, Middlesex University, Bounds Green, London N11 2NQ, UK,X.Gao@* Laboratory of Neuroinformatics of Sensory and Motor Systems, A.B. Kogan Research Institute for Neurocybernetics, Rostov State University, Rostov-on-Don, Russia, lnp@nisms.krinc.ruAbstractThis paper presents a new approach to segment and recognise traffic signs via two vision models: the colour appearance model CIECAM97s and Behaviour Model of Vision (BMV). This approach not only takes CIECAM97s into practical application for the first time since it was standardised in 1998, but also improves the accuracy of recognition of traffic signs dramatically, taking into account of changing weather conditions and varying transformations of signs. BMV model has been further developed to a foveal system for traffic signs (FOSTS). Comparison with the other colour spaces in terms of segmentation, including CIELUV and HSI, and RGB colour space is also carried out. The results show that CIECAM97s outperforms over the other three spaces with 94%, 90% and 85% accurate rate for sunny, cloudy and rainy viewing conditions respectively. It also confirms that CIECAM97s does predict the colour appearance similar to the average observer. For recognition stage, FOSTS system gives accuracy rates up to 95%.Key words: traffic sign segmentation, colour appearance model, CIECAM97s, BMV model, vision model, HSI space, CIELUV space, FOSTS system.1. IntroductionTo recognise traffic signs correctly at the right time and place is very important in ensuring a safe journey for car drivers and their passengers, as well as pedestrians crossing the road. Sometimes, due to the sudden change of viewing conditions, traffic signs are barely visible until it is too late, giving rise to a necessity for the development of an automatic system to assist car drivers in the recognition of traffic signs invariant of variety of transformations of signs and viewing environment. In the past, traffic signs were recognised with quick algorithms because of the limitation of computer power. With the advances of computer technology, especially hardware, the trade-off between processing speed and accuracy should be improved. In this study, two vision models are applied to segment and recognise traffic signs, including a colour appearance model, CIECAM97s for image segmentation of potential traffic signs, and a Behaviour Model of Vision (BMV) to recognise segmented regions.Based on the appearance of traffics signs, two segmentation approaches become popular, which are colour-based and shape-based. Usually, each approach only works well for a particular group of signs with constrained viewing conditions. For example, most colour-based techniques run into problems if the illumination source varies not only in intensity but also in colour, i.e., colour spectral distribution, as well. This is because the spectral composition, and therefore the colour, of daylight changes depending on weather conditions: e.g., sky with/without clouds, time of the day, e.g., night when all sorts of artificial lights are present [1].1.1Traffic Sign Segmentation Based on ColourColour is a dominant visual feature and is undoubtedly a key piece of information for drivers to handle. Colour information is widely used in traffic sign recognition systems [2], especially forsegmentation of traffic sign images from the rest of a scene. Colour is regulated not only for the traffic sign category (red = stop, yellow = danger, etc.) but also for the tint of the paint that covers the sign, which should correspond, with a tolerance, to a specific wavelength in the visible spectrum. Eight colours: red, orange, yellow, green, blue, violet, brown and achromatic [3], are the most discriminating colours for traffic signs.Many researchers have developed various techniques in order to make use of the colour information from traffic signs, which includes clustering method in a colour space [4] and a recursive region splitting method to achieve colour segmentation [5]. The colour spaces they have applied are HSI (Hue, Saturation,Intensity), and CIE L*a*b* space. These colour spaces normally are limited to only one lighting condition, which is D65, the average overcast daylight. Hence, the range of each colour attribute, such as hue, will be narrowed down due to the fact that weather conditions only change with colour temperatures ranging from 5000K to 7000K.Many other researchers focus on a few colours contained in the signs, including ‘stop’, ‘warning’, and ‘danger’ signs [6]. Their system is able to detect 55% of the ‘warning’ signs within the 55 images. Classification of traffic signs into different colour groups is also attempted [7]. RGB space is the most used space in this application. Additional procedures are also developed to improve the RGB performance based on estimation of shape, size and location of primarily segmented areas [8]. Combined approaches are proposed as well, including combination of colour and intensity [9] to determine candidates of traffic signs with white circular and blue rectangular regions. Although this approach does not miss any candidate, it detects many false candidate regions. Applying both RGB and HSI colour spaces in order to detect blue and red regions is another combined method [10], which is able to detect traffic sign of four pre-defined shapes with accuracy up to 90%.Due to the change of weather conditions and times of the day (for example, in the evening all sorts of artificial lights are present), the colour of the traffic signs, as well as illumination sources, appear different. Therefore, most colour-based techniques for traffic sign segmentation and recognition may not work properly, no existing method being widely accepted [11].In this study, traffic signs are segmented based on colour contents using a colour appearance model CIECAM97s that is recommended by the CIE (International Committee on Illumination) [12]. After segmentation, the recognition stage is carried out applying a modified Behaviour Model of Vision (BMV) [13] to retrieve the correct sign that matches the sign in the segment from a database of standard traffic signs.1.2 CIECAM97s colour Appearance ModelCIECAM97s is based on a simplified theory of colour vision for chromatic adaptation together with a uniform colour space [12]. It can predict colour appearance as accurate as an average observer. This colour appearance model is expected to extend traditional colorimetry (e.g., CIEXYZ, and CIELAB) to the prediction of the observed appearance of coloured stimuli under a wide variety of viewing conditions, which is accomplished by taking into account the tristimulus values (X, Y, and Z) of the stimulus, its background, its surround, the adapting stimulus, the luminance level, and other factors such as cognitive discounting the illuminant. The output of colour appearance models includes mathematical correlates for perceptual attributes that are brightness, lightness, colourfulness, chroma, saturation, and hue.Since it is standardised, the CIECAM97s has not been applied to any practical application. In the present study, this model is investigated on the segmentation of traffic signs. Comparisons withCIELUV, HSI, and RGB colour spaces are also conducted on the performance of traffic sign segmentation.1.3 Behaviour Model of Vision (BMV)BMV model was developed based on biologically plausible algorithms of space-variant image representation and image viewing. It has the ability to recognise complex grey-level images invariantly with respect to shift, plain rotation, and in a certain extent to scale, and has been extensively applied for face recognition. The basic version of the BMV model has been further extended for the task of traffic sign recognition, leading to FOSTS model (Foveal System for Traffic Signs) [14]. FOSTS model provides a compressed and invariant representation of each fragment of signs along the trajectory of viewing using representative space-variant features that are extracted from the fragment by Attention Window (AW). These descriptions have been stored with the images and form a model-specific database of traffic sign images, which needs to be built only once.2. Methodology2.1 Camera CalibrationTo collect traffic sign images, a high quality Olympus Digital Camera C-3030 Zoom is utilised to capture pictures in the real viewing conditions. Before taking the photos, camera calibration is carried out to ensure the consistency of the camera. Figure 1 illustrates the steps of calibrations.It starts with the setting the white balance of the camera to D65. The 24 colour patches from the Gretag Macbech Colour Checker are then captured under Verivide Viewing Cabinet with D65 illuminant. After the images are transferred to the computer, which has been calibrated into D65illuminant by a monitor calibration software (OptiCAL [15]), the images are displayed using MATLAB image processing software which shows the original image format (TIFF in this case). The X, Y, Z tristimulus values of 24 colours from the colour chart are measured by a colour meter CS-100A. After the X, Y, Z values are obtained, the relationships between XZY and RGB values in the image are worked out using linear regression, which is shown in Eq. (1). The measurements for the other viewing illuminant, such as D50, also give similar matrix as in Eq.(1). Therefore Eq. (1) is applied as the camera calibration model to convert the RGB values from an image into XYZ values for all the weather conditions.⎥⎥⎥⎦⎤⎢⎢⎢⎣⎡⋅⎥⎥⎥⎦⎤⎢⎢⎢⎣⎡−=⎥⎥⎥⎦⎤⎢⎢⎢⎣⎡B G R Z Y X 3209.00249.01319.00183.02068.01671.0048.01068.02169.0 (1)2.2 Image data collectionThe collection of sign images reflects the variety of viewing conditions and the variation of traffic sign sizes caused by differences in distance between traffic signs and driver (the position while taking pictures). The viewing conditions contain two elements: the weather conditions including sunny, cloudy, and rainy conditions, with each group sharing similar level of luminances; and the viewing angles, with complex traffic sign positions as well as multiple signs at a junction.The distance between the driver (and therefore the car) and the sign determines the size of traffic sign inside an image and is related to the recognition speed. According to The Highway Code [16] from UK, the stopping distance should be more than 10 meters under 30MPH (miles per hour), giving around 5 seconds to brake the car in case of emergency. Therefore, the photos are taken under the distance of 10, 20, 30, 40, and 50 meters respectively for each sign. In total, there are two sets ofdata are collected, each with 145 and 128 pictures respectively. They are taken under different weather conditions for some representative signs with various colour combinations. The first set of data is utilised to develop the segmentation and recognition systems, whilst the second set of data is applied to evaluate the systems. The first set of data consists of 145 pictures: 52 taken on a sunny day, 60 on a rainy day, and 33 on a cloudy day. All the photos were taken using similar camera settings.2.3 Image classification according to weather conditionsTo apply the colour appearance model, CIECAM97s, a particular set of viewing parameters should be utilised for each of different viewing conditions such as weather conditions or luminance levels. Therefore, image classification should be performed first in order to apply the right sets of parameters.Most sign photographs are taken in a similar position, leading to an image consisting of three parts from top to the bottom, containing sky, scenes, and the road respectively. If, however, some images miss one or two parts (for example, an image may miss the road when taken uphill), then these images are classified into sunny day condition, which can be verified at the later recognition stage. From this information, image classification can be carried out based on the saturation of sky or the texture of the road. The degree of saturation of the sky (the colour blue in this case) will decide the sunny, cloudy and rainy status, which is determined by using an optimal thresholding method. A sunny sky is clearly distinguishable from cloudy and rainy sky; it is easy to classify a sunny day based on the colour of sky. For cloudy or rainy conditions, another measure has to be enclosed to further the confirmation. This is done by the introduction of texture feature of the road that appears in the third part of the bottom of an image. The texture of the road is measured using fast Fourier Transform (FFT) with the average magnitude (AM) as threshold, which is shown in Eq.(2) [17].Although a Gabor filter is an alternative approach to represent texture with more comprehensive features, experimental results show that FFT is good enough in this application providing faster processing time. )/(),(,∑=k j N k j F AM(2) where ),(k j F is the amplitudes of the spectrum calculated by Eq. (3) and N is the number of frequency components.∑−=∑−=+−=⎥⎦⎤⎢⎣⎡1010)(2exp ),(1),(M m N n N nv M mu n m f MN v u F π (3) where f(n,m) is the value of the pixel with coordinates n, m. N , M is the image size, and u , v are frequency components.2.4 Traffic sign segmentationAfter classification, the reference white is obtained by repeatedly measuring a piece of white paper using a colour meter, CS-100A, under each viewing condition. The average of these values are given in Table 1 and applied in the subsequent calculations.The images taken under real viewing conditions are transformed from RGB space to CIE XYZ values using Eq.(1) and then to LCH (Lightness, Chroma, Hue), the space generated by CIECAM97s. The range of Hue, Chroma, Lightness for each weather condition is therefore obtained, which is shown in Table 2. These values are the mean values ± standard deviations. Only Hue and Chroma are utilised in the segmentation procedure due to the fact that lightness barely change with thechange of viewing conditions, which is consistent with the findings from the other researchers [18]. These ranges are applied as thresholds to segment potential traffic sign.2.5 Recognition of Signs using FOSTS modelEach sign in the FOSTS model is represented by specific description of sign inner content. The basic structure and operations in the FOSTS is illustrated in Figure 2 and consist of:(i)an image in each sensor fixation point is described by oriented segments extracted in thevicinity of each of 49 sensor nodes;(ii)the sensor nodes are located at the intersections of sixteen radiating lines and three concentric circles, each with a different radius;(iii)orientation of segments in the vicinity of each sensor node is determined by means of calculation of the difference between two oriented Gaussian functions with spatiallyshifted centres having the step of 22.5o;(iv)space-variant representation of image features is emulated by Gaussian convolution with different kernels increasing with the distance from the sensor centre.Contrary to [13], the input window (IW) size increases to 36 pixels (instead of 16 pixels in the basic BMV) and kernel sizes are changed to process a sign by one fixation of the AW, i.e., they are equal to 5x5 for the central part of the IW, 7x7 for the immediate, and 9x9 for the peripheral part. On the other hand, estimation of oriented elements in the context area of 48 IW nodes (minus the central node) is used to receive a detailed feature description of a sign. The size of context area is varied for different parts of the IW, being equal to 3x3 for 16 nodes located on the central ring of the IW, 5x5 for the immediate one, and 7x7 for the peripheral ring. Each IW node is described by two values, i.e., orientation dominating in its context area (if it is detected in morethan 50% of context area points) and by density of oriented elements detected in the context area (Fig. 2, b). Such structure of IW and its location in the sign centre provides maximal representation of oriented elements in informative parts of sign at the first and second resolution levels (up to 90%). An example of oriented elements detected in context area of indicated node of a sign is shown in Fig. 2, b.3. Experimental Results3.1 SegmentationFigure 3 gives an interface for traffic sign segmentation, showing three potential signs segmented from the image. The bottom right of segmented results contain a rear part of a car rather than a sign, which however, will be discarded by the recognition stage (to be discussed later).To evaluate the results of segmentation, two measures are used. One is the probability of correct detection, denoted by P c , and the other is the probability of false detection , denoted by P f , as calculated in Eqs. (4) and (5) respectively.P c = signs total of number signs with regions segmented of number (4)P f =regionssegmented of number total signs no with regions segmented of number (5) To evaluate CIECAM97s model, the second set of data with 128 pictures is applied to avoid duplication when applied performing segmentation, including 48, 53, and 27 pictures taken under sunny, rainy, and cloudy days respectively. Within these images, 142 traffic signs are visible. Amongthem 53 signs are from sunny days, 32 signs from cloudy days , and 57 signs from rainy days. The results of segmentation are listed in Table 3.Table 3 illustrates that for the sunny day, 94% signs have been correctly segmented using CIECAM97s model. However, it also gives 23% false segments, i.e., the regions without any signs at all, like the segment at the bottom right in Fig. 3 showing the rear light of a car. Table 3 also demonstrates that the model works better on sunny day than cloudy or rainy days, the last two conditions receive P c value of 90% and 85% respectively. Although the segmentation process gives some false segments, these segments can be discarded during recognition stages.3.2 RecognitionThe 49-dimension vector for a traffic-sign-to-be image is compared with descriptions of database images of the corresponding subgroup by the formula Eq.(6):()(())[]∑<=−−⋅−=4901sgn i i rw i b i rw i b i babs Or Or K ρρ(6) ()⎩⎨⎧==verwise; o x if x ,0;0,1sgn where Or i b is dominating orientation extracted from the context area of a given IW node (orientations are determined by the step 22.5° and indicated as 1, 2, 3…., 16), whereas superscript b stands for images from a database and rw the real world image segmented using colour-based approach. ρ is the density of the dominating oriented segment in the vicinity of the given IW node. Preliminary results have shown that minimal value of resulting b K is 25; otherwise the region of interest will be considered a false sign to be rejected. Figure 4 illustrates the rejection of falsely segmented regions after segmentation and recognition procedures.For the data set of 142 signs from 128 pictures, 99% of false positive regions are discarded. Figure 5 demonstrates the interface of the system combining both segmentation and recognition. In the left bottom picture, two regions are segmented (with square boundary). One is the sign with 40 inside a circle, the other is the rear light of a car at the right-hand corner of bottom left picture. After recognition, only the 40mph speed limit sign is framed (top left picture), with a matching sign retrieved from the database (right bottom picture).4. Comparison with HSI and CIELUV methodsIn the literature, nearly all the segmentation approaches apply one of RGB, HIS, and CIELUV spaces to represent a pixel. Comparisons with these three methods are therefore carried out. Because RGB space is device dependent, the comparison will be discussed in the next section.The HSI (Hue, Saturation, and Intensity) method has been widely used in colour segmentation as it is much closer to human perception [19] than RGB, the space that images are originally formatted. Eq.(7) gives the conversion from RGB to HSI.()()()()()⎪⎭⎪⎬⎫⎪⎩⎪⎨⎧−−+−−+−=−B G B R G R B R G R H 212cos G R ≠or B R ≠ ()(B G R Min B G R Max S ,,,,−=)(7) ()3B G R I ++=Another popular space used on colour segmentation is CIELUV, which is recommended by CIE for specifying colour differences and is uniformed as equal scale intervals representing approximatelyequal perceived differences in the attributes considered. The attributes generated by the space are Hue (H), Chroma (C), and lightness (L), which are calculated by Eq.(8) [20].16)(116*3/10−=Y Y L , if Y/Y 0 >0.008856 )(3.903*0Y Y L ⋅= if Y/Y 0 <=0.008856)''(*13*0u u L u −=(8) )''(*13*0v v L v −=*)/*arctan(u v H =22*)(*)(v u C +=where Y 0, u 0, v 0, are the Y, u, v values for the reference white.The segmentation procedure using these two spaces is similar to the application of CIECAM97s. Firstly, the colour ranges for each attribute are obtained for each weather condition. Then images are segmented using optimal thresholding method based on these colour ranges. Table 4 lists the comparison results between three colour spaces.These data show that for each weather condition, CIECAM97s performs best with correct segmentation rate of 94%, 90% and 85% respectively for sunny, cloudy, and rainy conditions. CIELUV performs better than HSI for the cloudy and rainy conditions. Also HSI gives largest percentage of false segmentation with 29%, 37% and 39% respectively for each weather condition. The results also demonstrate that all colour spaces do not perform as well for rainy days as for the other two weather conditions (sunny and cloudy), which is in line with everyday experience, i.e., the visibility is worse on a rainy day than on a sunny or cloudy day for drivers. Figure 6 demonstrates the process of segmentation in a real example carried out by the three colour spaces, which depictsthat CIECAM97s gives two correct segments with signs inside. Whereas CIELUV colour space method segments two signs plus a false segment. For the HSI colour space, two signs are segmented correctly in addition to two false segments, which again illustrates that HSI performs worst in traffic sign segmentation task based on colour.5. Traffic Sign Segmentation Based on RGBTraffic sign segmentation by RGB space is also performed in comparison with the above discussed methods on a calibrated monitor. The calibration setting is the average daylight of D65. Based on the preliminary evaluation of RGB composition for traffic signs, the ranges of [35, 255], [-20, 20], [15, 230], [5, 85] are determined for signs R-B, G-R, G-R, and B-G respectively. On the other hand, while determining each segmented region as a potential traffic sign, two additional conditions should be taken into account. One is the size of clustered colour blobs that should be no less than 10x10 pixels. The other is the relation of width/height of the segmented region that should be in a range 0.5-1.5.The same set of testing road sign pictures (n=128) are applied with the implementation of RGB as segmentation method, which is shown in Table 5.Tables 4 and 5 indicate that probability of correct traffic sign segmentation by RGB is lower than by CIECAM97 for sunny and cloudy weather conditions. Further more, the probability of false positive detection is much higher for the RGB method, illustrating strongly dependence on weather conditions.6. Conclusions and DiscussionsThis paper introduces a new approach for recognition of traffic signs based on vision moodels, which utilises the application of the CIECAM97s and FOSTS, developed based on human perception and BMV respectively. The experimental results show that this CIECAM97s model performs very well and can give very accurate segmentation results, with up to 94% accuracy rate for sunny days. When compared with HSI, CIELUV and RGB, the three most popular colour spaces used in colour segmentation research, CIECAM97s over-performs the others. The result not only confirms that the model’s prediction is closer to the average observer’s visual perception, but also opens up a new approach for colour segmentation while doing image processing. However, when it comes to the calculation, CIECAM97s is more complex than the other colour spaces and needs larger amount of calculations, which will be a problem when processing video images in real time. At the moment, the processing time for segmentation can be reduced to 1.8 seconds and the recognition time is 0.19 second, leading to 2 seconds for processing one frame of image. When processing video images, there are normally eight frames in 1 second, which means the total time (= segmentation time + recognition time) should be 0.25 seconds for one frame of image. Therefore, more work needs to be done to further optimise algorithms for segmentation and recognition in order to meet the demand for real time traffic sign recognition. Although the correct segmentation rate is less than 100% when applying CIECAM97s, the main reason is the size of signs in an image being too small in some scenes. When processing video images, the signs of interest will gradually become larger when the car is approaching to the signs. Hence the correct segmentation rate can be improved dynamically. As for recognition, FOSTS can retrieve the correct signs with 95% accuracy. Some signs, e.g., a speed limit sign covered with leaves, are difficult to recognise its contents.AcknowledgementThis work is partly support by The Royal Society, UK, under the International Scientific Exchange Scheme and partly sponsored by Russian Foundation for Basic Research, Russia, grant № 05-01-00689. Their support is gratefully acknowledged.References:1.Judd D., MacAdam D., and Wyszecki G. Spectral Distribution of Typical Daylight as aFunction of Correlated Color Temperature. J. Opt. Soc. Am., 54(8):1031-1040, 1964.londe M. and Li Y. Road Sign Recognition -- Survey of the State of Art, technique reportfor Sub-Project 2.4, CRIM-IIT-95/09-35,http://nannetta.ce.unipr.it/argo/theysay/rs2/#publications.3.CIE, Recommendations for Surface Colours for Visual Signalling. CIE No. 39-2 (TC-1.6) ed.1983.4.Tominaga S. Color Image Segmentation Using Three Perceptual Attributes. Proc. CVPR-86,pp. 628-630, 1986.5.Ohlander R., Price K., Reddy D., Picture Segmentation Using a Recursive Region SplittingMethod, Computer Graphics and Image Processing, 13:224-241, 1978.6.Kehtarnavaz N., Griswold N.C., and Kang D.S. Stop-sign Recognition Based on Colour-shape Processing. Machine Vision and Applications, 6: 206-208. 1993.7.Paclík P., Novovicová J., Pudil P., and Somol P. Road Sign Classification using the LaplaceKernel Classifier. Pattern Recognition Letters, 21 (13-14) :1165-1173, 2000.8.Yang H.-M., Liu C.-L., Liu K.-H., and Huang S.-M., Traffic Sign Recognition in DisturbingEnvironments, Lecture Notes in Computer Science 2871: 252-261, 2003.9.Miura, J., Kanda, T., Nakatani, S., Shirai, Y. An Active Vision System for on-line TrafficSign Recognition, IEICE Transaction on Information System, E85-D(11):.1784-1792, 2002.10.Madeira S. R., Bastos L. C., Sousa A., Sobral J.L., Santos L.P. Automatic Traffic SignsInventory Using a Mobile Mapping System (GIS PLANET 2005), International Conference and Exhibition on Geographic Information, Portugal, 2005.11.de la Escalera A., Armingol J. M., Pastor J. M., and Rodriguez F. J. Visual Sign InformationExtraction and Identification by Deformable Models for Intelligent Vehicles, IEEE transactions on intelligent transportation systems, 5(2): 57-68, 2004.12.Fairchild M.D., A revision of CIECAM97s for practical applications, Color Research andApplication, 26:418-427, 2001.13.Rybak I.A., Gusakova V.I., Golovan A.V., Podladchikova L.N., Shevtsova N.A., A Modelof Attention-guided Visual Perception and Recognition, Vision Research, 38:2387-2400, 1998.14.Shaposhnikov D.G., Podladchikova L.N., Gao, X.W., Classification of Images on the Basisof the Properties of Informative Regions, Pattern Recognition and Image Analysis, 13(2): 349-352, 2003.15./.16.Driving Standards Agency. The Highway Code, London, England: The Stationery Office,1999.17.Clements M.F., L.E., and Shaw K.A, Performance Evaluation of Texture Measures forGround Cover Identification in Satellite Images by Means of a Neural Network Classifier.IEEE trans. Geoscience and Remote Sensing, 33 (3): 616-626, 1995.18.Romero J., Hernandez-Andres J., Nieves J. L., Garcia J. A., Color Coordinates of Objectswith Daylight Changes, COLOR research and application, 28(1): 25-25, 2003.。