比较线性模型和Probit模型Logit模型
probit模型与logit模型
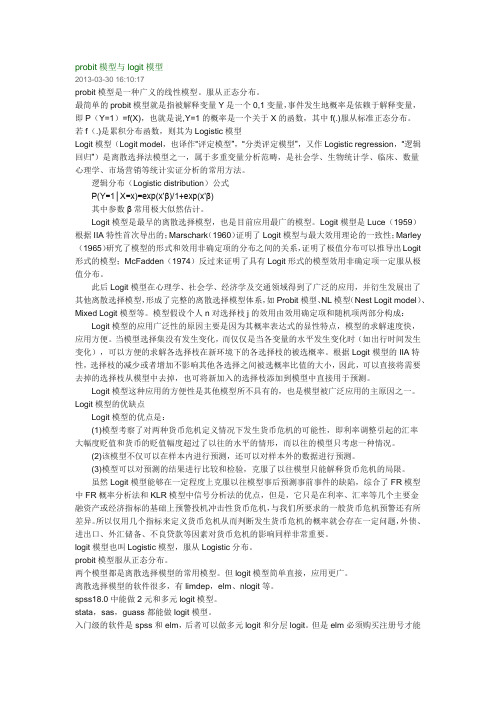
probit模型与logit模型2013-03-30 16:10:17probit模型是一种广义的线性模型。
服从正态分布。
最简单的probit模型就是指被解释变量Y是一个0,1变量,事件发生地概率是依赖于解释变量,即P(Y=1)=f(X),也就是说,Y=1的概率是一个关于X的函数,其中f(.)服从标准正态分布。
若f(.)是累积分布函数,则其为Logistic模型Logit模型(Logit model,也译作“评定模型”,“分类评定模型”,又作Logistic regression,“逻辑回归”)是离散选择法模型之一,属于多重变量分析范畴,是社会学、生物统计学、临床、数量心理学、市场营销等统计实证分析的常用方法。
逻辑分布(Logistic distribution)公式P(Y=1│X=x)=exp(x’β)/1+exp(x’β)其中参数β常用极大似然估计。
Logit模型是最早的离散选择模型,也是目前应用最广的模型。
Logit模型是Luce(1959)根据IIA特性首次导出的;Marschark(1960)证明了Logit模型与最大效用理论的一致性;Marley (1965)研究了模型的形式和效用非确定项的分布之间的关系,证明了极值分布可以推导出Logit 形式的模型;McFadden(1974)反过来证明了具有Logit形式的模型效用非确定项一定服从极值分布。
此后Logit模型在心理学、社会学、经济学及交通领域得到了广泛的应用,并衍生发展出了其他离散选择模型,形成了完整的离散选择模型体系,如Probit模型、NL模型(Nest Logit model)、Mixed Logit模型等。
模型假设个人n对选择枝j的效用由效用确定项和随机项两部分构成:Logit模型的应用广泛性的原因主要是因为其概率表达式的显性特点,模型的求解速度快,应用方便。
当模型选择集没有发生变化,而仅仅是当各变量的水平发生变化时(如出行时间发生变化),可以方便的求解各选择枝在新环境下的各选择枝的被选概率。
logit 和probit模型的系数解释 -回复
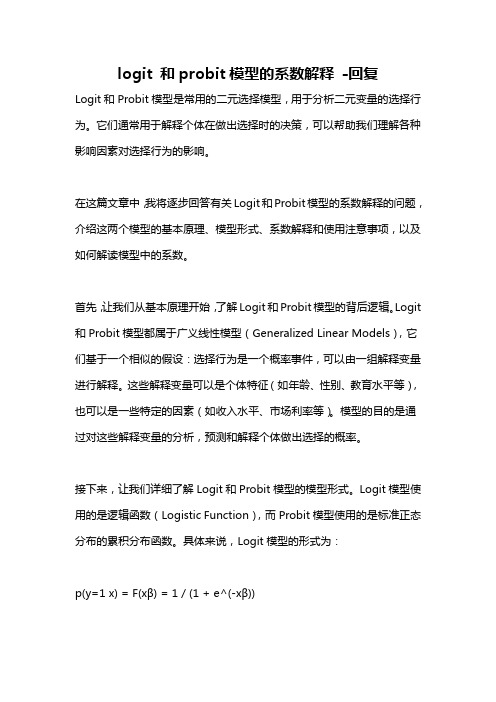
logit 和probit模型的系数解释-回复Logit和Probit模型是常用的二元选择模型,用于分析二元变量的选择行为。
它们通常用于解释个体在做出选择时的决策,可以帮助我们理解各种影响因素对选择行为的影响。
在这篇文章中,我将逐步回答有关Logit和Probit模型的系数解释的问题,介绍这两个模型的基本原理、模型形式、系数解释和使用注意事项,以及如何解读模型中的系数。
首先,让我们从基本原理开始,了解Logit和Probit模型的背后逻辑。
Logit 和Probit模型都属于广义线性模型(Generalized Linear Models),它们基于一个相似的假设:选择行为是一个概率事件,可以由一组解释变量进行解释。
这些解释变量可以是个体特征(如年龄、性别、教育水平等),也可以是一些特定的因素(如收入水平、市场利率等)。
模型的目的是通过对这些解释变量的分析,预测和解释个体做出选择的概率。
接下来,让我们详细了解Logit和Probit模型的模型形式。
Logit模型使用的是逻辑函数(Logistic Function),而Probit模型使用的是标准正态分布的累积分布函数。
具体来说,Logit模型的形式为:p(y=1 x) = F(xβ) = 1 / (1 + e^(-xβ))其中,p(y=1 x)表示个体在给定解释变量x的情况下选择y=1的概率,F(x β)表示Logistic函数,x是解释变量的值,β是模型的系数。
相比之下,Probit模型的形式稍有不同:p(y=1 x) = Φ(xβ)其中,Φ(xβ)表示标准正态分布的累积分布函数,其他符号的含义与Logit 模型相同。
两个模型的模型形式不同,但它们都具有类似的特点:在x 趋近于正无穷时,概率趋近于1,而在x 趋近于负无穷时,概率趋近于0。
这种形式可以帮助我们理解个体选择行为的变化趋势。
现在让我们转向系数解释的问题。
模型的系数代表着解释变量对选择行为的影响程度。
离散因变量模型(Logit模型,Probit模型).ppt
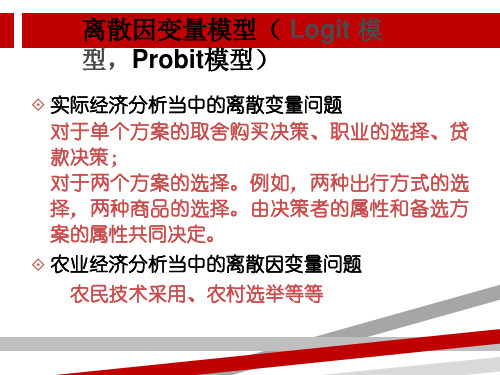
yi 0
yi 1
所以似然函数为:
n
L
( F (X i))yi (1 F (X i))1yi
i 1
n
ln L ( yi ln F ( X i ) (1 yi ) ln(1 F ( X i )))
i 1
ln L
n i 1
yi f i Fi
(1
yi
)
fi (1 Fi
)
X
P( yi*
0)
P(
* i
Xi)
1
P(
* i
Xi)
1 F (Xi) F (Xi)
F(t) 1 F(t)
E( yi Xi ) 1 P 0 (1 P) F (Xi)
Y E(Y X )
总体回归模型
样本回归模型
Y F ( XB) yi F ( Xi B) i (i 1, 2......n)
U
1 i
Xi 1
i1
第i个个体选择1的效用
U
0 i
Xi 0
i0
第i个个体不选择1(选择0)的效用
U
1 i
U
0 i
Xi (1
0 )
(i1
i0 )
yi* Xi
i
yi 1( yi 0) 选择1
yi
0( yi
0)
不选择1 (选择0)
(二) 二元选择的经济计量一般模型
P( yi
1
Xi)
模型 yi ( Xi B) i
f
(Z )
F'(Z)
eZ (1 eZ )2
(Z )(1 (Z ))
线性化 pi ( Xi B)
∵
(
Z
probit logit 解析表达式
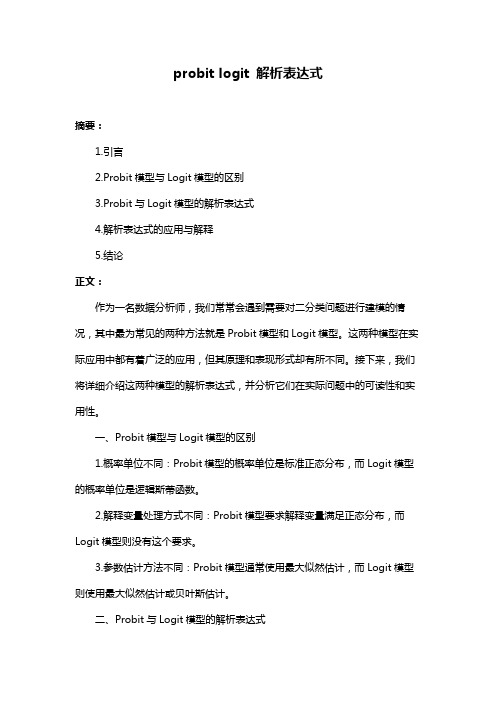
probit logit 解析表达式摘要:1.引言2.Probit模型与Logit模型的区别3.Probit与Logit模型的解析表达式4.解析表达式的应用与解释5.结论正文:作为一名数据分析师,我们常常会遇到需要对二分类问题进行建模的情况,其中最为常见的两种方法就是Probit模型和Logit模型。
这两种模型在实际应用中都有着广泛的应用,但其原理和表现形式却有所不同。
接下来,我们将详细介绍这两种模型的解析表达式,并分析它们在实际问题中的可读性和实用性。
一、Probit模型与Logit模型的区别1.概率单位不同:Probit模型的概率单位是标准正态分布,而Logit模型的概率单位是逻辑斯蒂函数。
2.解释变量处理方式不同:Probit模型要求解释变量满足正态分布,而Logit模型则没有这个要求。
3.参数估计方法不同:Probit模型通常使用最大似然估计,而Logit模型则使用最大似然估计或贝叶斯估计。
二、Probit与Logit模型的解析表达式1.Probit模型:Probit模型的解析表达式为:P(Y=1|X)=Φ[β0+β1X1+...+βkXk]其中,Φ()表示标准正态分布的累积分布函数,β0、β1、...、βk为模型参数。
2.Logit模型:Logit模型的解析表达式为:P(Y=1|X)=exp(β0+β1X1+...+βkXk)/(1+exp(β0+β1X1+...+βkXk))其中,exp()表示自然对数的底数,β0、β1、...、βk为模型参数。
三、解析表达式的应用与解释1.模型评估:通过观察解析表达式,我们可以对模型的拟合效果进行评估。
一般来说,解析表达式中的参数β1、...、βk表示了解释变量对响应变量的影响程度,β1、...、βk的系数越大,说明解释变量对响应变量的影响越大。
2.预测分析:利用解析表达式,我们可以对未来的观测值进行预测。
例如,在Probit模型中,我们可以通过计算P(Y=1|X)来预测个体在某一特定条件下选择某一分类的概率;在Logit模型中,我们可以通过计算P(Y=1|X)来预测个体是否会选择某一分类。
170-演示文稿-线性概率模型、Logit模型与Probit模型的比较
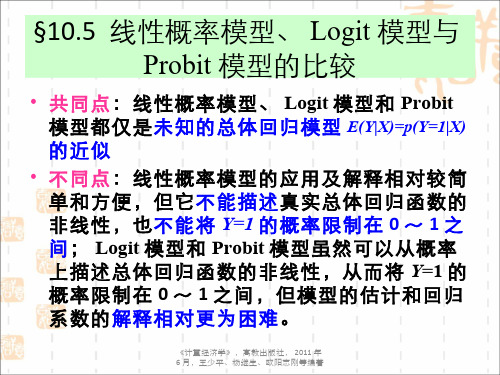
尽管线性概率模型有明显的不足,但当 解释变量的观察值中没有极端值时 ( 是 指那些能够使得 Y=1 的概率超出 0 ~ 1 区间的解释变量值,可回过去看看 10.2 中的例子 ) ,使用线性概率模型常常能 得到总体回归函数的较好近似。
《计量经济学》,高教出版社, 2011 年 6 月,王少平、杨继生、欧阳志刚等编著
§10.5 线性概率模型、 Logit 模型与 Probit 模型的比较
• 共同点:线性概率模型、 Logit 模型和 Probit 模型都仅是未知的总体回归模型 E(Y|X)=p(Y=1|X) 的近似
• 不同点:线性概率模型的应用及解释相对较简 单和方便,但它不能描述真实总体回归函数的 非线性,也不能将 Y=1 的概率限制在 0 ~ 1 之 间; Logit 模型和 Probit 模型虽然可以从概率 上描述总体回归函数的非线性,从而将 Y=1 的 概率限制在 0 ~ 1 之间,但模型的估计和回归 系数的解释相对更为困难。
《计量经济学》,高教出版社, 2011 年 6 月,王少平、杨继生、欧阳志刚等编著
• Logit 模型和 Probit 模型的比较: 逻辑分布有相对较平坦的尾部,也就是 说, Logit 的条件概率比 Probit 以更慢 的速度趋近于 0 和 1 。但由于 Logit 使用 相对简单的数学形式,因此,实践中常 常选用它。
比较logit 模型和probit 模型
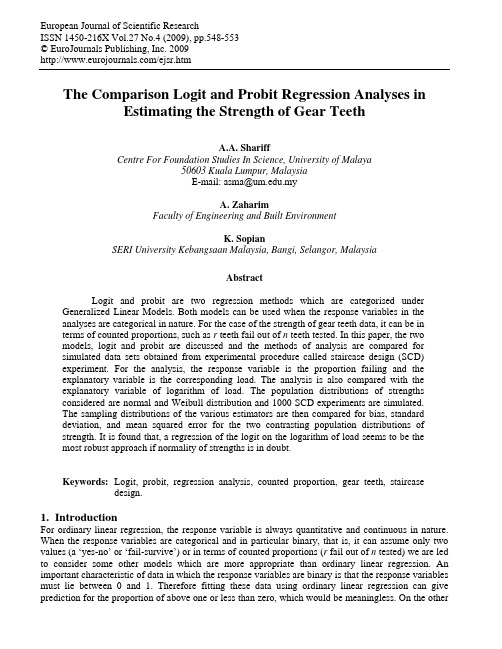
European Journal of Scientific ResearchISSN 1450-216X Vol.27 No.4 (2009), pp.548-553© EuroJournals Publishing, Inc. 2009/ejsr.htmThe Comparison Logit and Probit Regression Analyses inEstimating the Strength of Gear TeethA.A. ShariffCentre For Foundation Studies In Science, University of Malaya50603 Kuala Lumpur, MalaysiaE-mail: asma@.myA. ZaharimFaculty of Engineering and Built EnvironmentK. SopianSERI University Kebangsaan Malaysia, Bangi, Selangor, MalaysiaAbstractLogit and probit are two regression methods which are categorised under Generalized Linear Models. Both models can be used when the response variables in theanalyses are categorical in nature. For the case of the strength of gear teeth data, it can be interms of counted proportions, such as r teeth fail out of n teeth tested. In this paper, the twomodels, logit and probit are discussed and the methods of analysis are compared forsimulated data sets obtained from experimental procedure called staircase design (SCD)experiment. For the analysis, the response variable is the proportion failing and theexplanatory variable is the corresponding load. The analysis is also compared with theexplanatory variable of logarithm of load. The population distributions of strengthsconsidered are normal and Weibull distribution and 1000 SCD experiments are simulated.The sampling distributions of the various estimators are then compared for bias, standarddeviation, and mean squared error for the two contrasting population distributions ofstrength. It is found that, a regression of the logit on the logarithm of load seems to be themost robust approach if normality of strengths is in doubt.Keywords:Logit, probit, regression analysis, counted proportion, gear teeth, staircase design.1. IntroductionFor ordinary linear regression, the response variable is always quantitative and continuous in nature. When the response variables are categorical and in particular binary, that is, it can assume only two values (a ‘yes-no’ or ‘fail-survive’) or in terms of counted proportions (r fail out of n tested) we are led to consider some other models which are more appropriate than ordinary linear regression. An important characteristic of data in which the response variables are binary is that the response variables must lie between 0 and 1. Therefore fitting these data using ordinary linear regression can give prediction for the proportion of above one or less than zero, which would be meaningless. On the otherThe Comparison Logit and Probit Regression Analyses in Estimating the Strength of Gear Teeth 549hand what we actually need in this situation is a regression model which will predict the proportion ofoccurrences, p (let us call them p instead of y ) at certain levels of x .For this type of data, in particular, when the response variables are in terms of countedproportions, the relationship between response variables p and explanatory variables x is a non-linearcurved relationship (S-shaped) curve which is usually called sigmoid . The S-shaped behaviour is verycommon in modeling binomial responses as a function of predictors and also makes use of theassumption that the responses are from underlying binomial or binary distribution. The purpose oflogistic modelling (and also probit) is the same as other modelling techniques used in statistics, that is,to find a model that fits the data best and is the simplest, yet physically reasonable in describing therelationship between the response and the explanatory variables [1]. There is little to distinguishbetween logit and probit models. Both curves are so similar as to yield essentially identical results. Itwas found that probit and logit analysis applied to the same set of data produce coefficient estimateswhich differ approximately by a factor of proportionality, and that factor should be about 1.8 [2].2. Materials and Methods2.1. Logit Versus Probit Regression Techniques Logit model can be presented asp Z Z =+exp()exp()1 (1) where p is the proportion of occurrences, Z =βββ011+++x x k k ... and x x k 1... are the explanatory variables. The inverse relation of equation (1) isZ p p =−⎛⎝⎜⎞⎠⎟ln 1 (2) that is, the natural logarithm of the odds ratio, known as the logit. It transforms p which is restricted tothe range [0, 1] to a range [,]−∞∞ .Probit regression analysis involves modeling the response function with the normal cumulativedistribution function. The probit of a proportion p is just the point on a normal curve with mean 0 andstandard deviation 1 which has this proportion to the left of it.The model can be presented asΦ−==+++1011()...p Z x x k k βββ (3) where p is the proportion and Φ−1 is the inverse of the cumulative distribution function of the standard normal distribution. That is,p Z u du Z ==−−∞∫Φ()exp(/)1222π (4) is the cumulative distribution function of the standard normal distribution.For logistic and probit regression, the binomial, rather than the normal distribution describesthe distribution of the errors and will be the statistic upon which the analysis is based. The principlesthat are used for ordinary linear regression analysis could be adapted to fit both regressions. However,instead of using least square method to fit the model, for logistic and probit regressions, it is moreappropriate to use maximum likelihood estimate. The likelihood function is given asL p p i r i mi n r i i i =−=−∏()11,where the p i are defined in terms of the parameters ββ0,...,k and the known values of the predictorvariables. This has to be maximized with respect to the parameters.550A. A. Shariff, A. Zaharim and K. Sopian2.2. Experimental Design Gear teeth are commonly tested by applying oscillatory loads, using a special machine called pulsator-test machine. In the experiment, the test specimen, in this case the gear tooth is subjected to vibrations of a resonant spring/mass system. When this happens, it experiences stresses and crack propagation takes place. Eventually, after certain number of cycles the tooth fails. The number of cycles to failure can then be recorded. If the tooth does not fail after a certain fixed number of cycles, it is considered to have survived in the experiment. The experimental procedure used is the well-known staircase design (SCD). SCD experiment is also known as sensitivity testing or ‘up-and-down’ method [3] where the testing of specimens is made close to the anticipated mean level. In the experiment the first test piece should be tested at a load level assumed to be near the mean value of the fatigue strength. If failure occurs before N cycles, the next test piece is tested at one step, a fixed change in load, below the first load level. Otherwise, the next test at the load one step above the first level. This procedure is continued until all the pieces have been tested. The increment between load levels should be equal for steps up and down and should be approximately one standard deviation of the fatigue strength distribution. Since the data obtained are categorical in nature, particularly in terms of counted proportions, fatigue strength of a gear is then determined by analysing the data obtained using appropriate statistical techniques, in this case logit and probit.2.3. Analysis For SCDThe results obtained in the experiment are then analysed using logit and compared with probit. Thelogit transformation of p is defined by ln(p p1−, and the lines ln()p px 101−=+ββ are fitted, using maximum likelihood. A comparison is made with fitting the line,ln()ln p px 101−=+ββ which is equivalent to assuming a log-normal distribution of strengths.For probit, results of SCD experiment are analysed by fitting the lineΦ−=+101()p x ββwhere p is the proportion failing and x is the corresponding load. This is equivalent to assuming a normal distribution of strengths. Then a comparison is made withΦ−=+101()ln p x ββThe estimated mean fatigue strength, μ, and the lower 1% point of the distribution of fatiguestrength, .x 099, are the values of x corresponding to p =05. and p =001. respectively. The standarddeviation can be estimated from ( )/..σμ=−x 099233 .The methods of analysis have been compared for simulated data sets. The population distribution of strengths is specified and 1000 SCD experiments are simulated. The sampling distributions of the various estimators can thus be compared for bias, standard deviation, and mean squared error. Two contrasting population distributions of strength are considered:(i). normal distribution with mean of 20.0 and standard deviation of 2.0;(ii).W eibull distribution [4 – 6], which has a cumulative distribution function F(x) defined by Pr()()exp[(/)]X x F x x b c <==−−1),with shape parameter, c = 2, and scale parameter, b = 22.56. These parameter values correspond to a mean of 20 and standard deviation of 10.45. The probability density functions of both distributions are plotted in Figure 1. The Weibull distribution has a substantial area near zero. This might be realistic forThe Comparison Logit and Probit Regression Analyses in Estimating the Strength of Gear Teeth 551the strength of a component being tested under extreme conditions, as in an accelerated testing programme. It could also be interpreted as strength above some minimum value.Figure 2: Probability density function of normal (,.)μσ==2020 and Weibull (,.45)μσ==2010distributionIn the simulation, each SCD used 50 test specimens. There are 1000 independent SCD experiments within a simulation. Each specimen put on test is randomly selected from the 50 specimens. For the normal distribution the load increment is chosen to be 2, while for the Weibull distribution it is chosen to be 6, since the standard deviation for this distribution is larger.Results obtained from the above experiments are analysed using logit and then compared with probit analyses for each distribution. The means and standard deviations of the estimated mean, standard deviation and lower 1% point of the strength distribution are computed from 1000 SCD experiments for each distribution.These results are tabulated in Table 1; the standard deviations of these statistics are shown in brackets, and the root mean square error (RMSE) is also calculated using the formula552A. A. Shariff, A. Zaharim and K. Sopian Table 1:Results of Staircase Experiments Analysed by Probit and Logit Regression Techniques with the load on a linear scale for Each Distribution.RMSE (standard deviation)(bias)22=+where, bias = (actual value - mean of estimated value).These RMSE values are presented in square brackets. Table 2 shows results for the logit and probit analysis using a logarithmic scale for load.Table 2: Results of Staircase Experiments Analysed by Probit and Logit Regression Techniques with the loadon a logarithmic scale for Each DistributionThe Comparison Logit and Probit Regression Analyses in Estimating the Strength of Gear Teeth 553 3. Results and DiscussionTable 1 indicates that for load on linear scale, results obtained by probit analysis are more realistic with less error as compared to logit for normal distribution of strength. Logit analysis appears to overestimate the standard deviation and hence underestimate the lower one percent point of the distribution. However, for the Weibull distribution, which has a large standard deviation, both methods predict negative lower 1% points which are physically impossible.Regressing the sample probit against the logarithm of load (refer to Table 2) gives estimates with a smaller standard deviation and, somewhat surprisingly, a slightly smaller mean squared error. Regressing logit of the sample proportion against the logarithm of load is a slight improvement on the probit analysis and aconsiderable improvement on a regression of logit against load.When sampling from both normal and Weibull distributions the regression of the logit against the logarithm of load gives an estimate of the lower 1% point with the smallest mean squared error. Overall, a regression of the logit on the logarithm of load seems to be the most robust approach if normality of strengths is in doubt.References[1]Hosmer, D.W., & Lemeshow, S. (1989). Applied Logistic Regression. Wiley Series inProbability and Mathematical Science. Wiley-Interscience Publication.[2]Aldrich, J.H. & Nelson, F.D. (1984). Linear Probability, Logit and Probit Models. SageUniversity Paper series on Quantitative Applications in the Social Sciences, 07-045. Beverly Hills and London: Sage Pubns.[3]Lloyd, D. K., & Lipow, M. (1989). Reliability: managements, methods, and mathematics(Second ed.). American Society for Quality Control.[4]ISO/CD 12107. (1997). Draft for Public Comment, Metallic Materials - Fatigue Testing -Statistical Planning and Analysis of Data, British Standard Institution.[5]Weibull, W. (1961). Fatigue Testing and the Analysis of Results. Pergamon Press. Oxford.[6]Crowder, M.J., Kimber, A.C., Smith, R.L. & Sweeting, T.J. (1991). Statistical Analysis ofReliability Data. Chapman and Hall.。
Probit回归模型
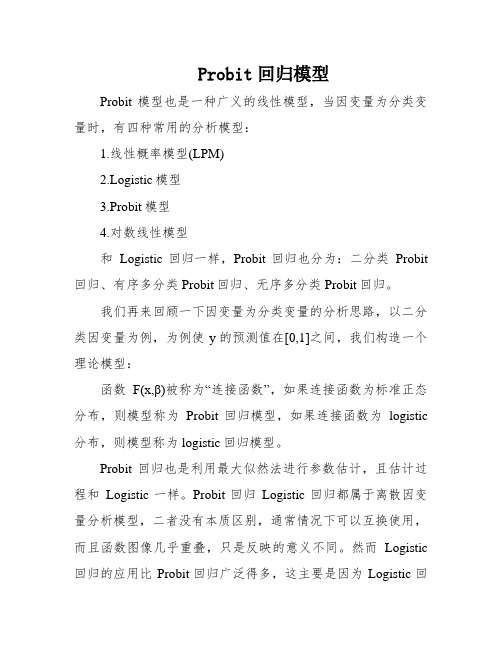
Probit回归模型
Probit模型也是一种广义的线性模型,当因变量为分类变量时,有四种常用的分析模型:
1.线性概率模型(LPM)
2.Logistic模型
3.Probit模型
4.对数线性模型
和Logistic回归一样,Probit回归也分为:二分类Probit 回归、有序多分类Probit回归、无序多分类Probit回归。
我们再来回顾一下因变量为分类变量的分析思路,以二分类因变量为例,为例使y的预测值在[0,1]之间,我们构造一个理论模型:
函数F(x,β)被称为“连接函数”,如果连接函数为标准正态分布,则模型称为Probit回归模型,如果连接函数为logistic 分布,则模型称为logistic回归模型。
Probit回归也是利用最大似然法进行参数估计,且估计过程和Logistic一样。
Probit回归Logistic回归都属于离散因变量分析模型,二者没有本质区别,通常情况下可以互换使用,而且函数图像几乎重叠,只是反映的意义不同。
然而Logistic 回归的应用比Probit回归广泛得多,这主要是因为Logistic回
归的偏回归系数解释起来更加直观和易于理解——Probit回归的偏回归系数含义为其他自变量保持不变的时该自变量每增加一个单位,出现某个结果的概率密度函数的改变值,这很难以理解。
那么什么情况下能够使用Probit回归替代Logistic回归呢?可从以下两个方面考虑
1.自变量中连续型变量较多
2.残差符合正态分布。
probit logit 解析表达式 -回复
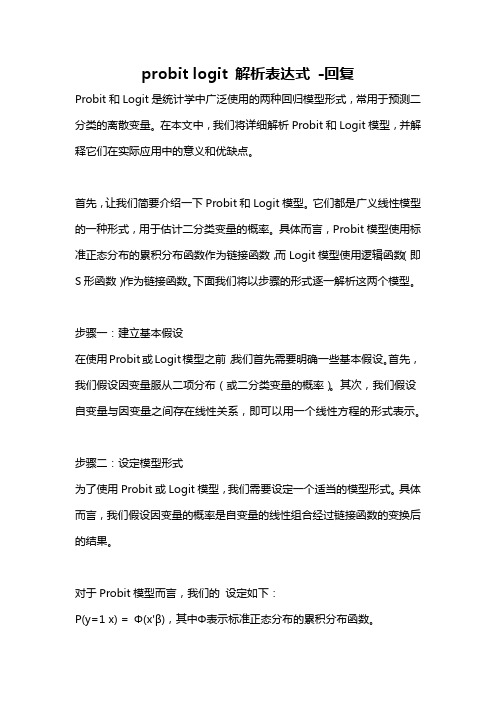
probit logit 解析表达式-回复Probit和Logit是统计学中广泛使用的两种回归模型形式,常用于预测二分类的离散变量。
在本文中,我们将详细解析Probit和Logit模型,并解释它们在实际应用中的意义和优缺点。
首先,让我们简要介绍一下Probit和Logit模型。
它们都是广义线性模型的一种形式,用于估计二分类变量的概率。
具体而言,Probit模型使用标准正态分布的累积分布函数作为链接函数,而Logit模型使用逻辑函数(即S形函数)作为链接函数。
下面我们将以步骤的形式逐一解析这两个模型。
步骤一:建立基本假设在使用Probit或Logit模型之前,我们首先需要明确一些基本假设。
首先,我们假设因变量服从二项分布(或二分类变量的概率)。
其次,我们假设自变量与因变量之间存在线性关系,即可以用一个线性方程的形式表示。
步骤二:设定模型形式为了使用Probit或Logit模型,我们需要设定一个适当的模型形式。
具体而言,我们假设因变量的概率是自变量的线性组合经过链接函数的变换后的结果。
对于Probit模型而言,我们的设定如下:P(y=1 x) = Φ(x'β),其中Φ表示标准正态分布的累积分布函数。
对于Logit模型而言,我们的设定如下:P(y=1 x) = 1 / (1 + exp(-x'β)),其中exp表示指数函数。
步骤三:估计参数为了估计Probit或Logit模型的参数,我们使用最大似然估计方法。
简言之,我们希望找到一组参数,使得给定自变量后,模型预测的因变量概率最大。
在实际应用中,我们通过最大似然估计方法,求解出模型中的参数。
这些参数可以在一定程度上解释自变量对因变量的影响。
步骤四:解释结果最后,我们可以通过估计得到的参数来解释模型的结果。
在Probit和Logit 模型中,参数的正负符号以及显著性水平可以告诉我们自变量与因变量之间的关系。
需要注意的是,Probit和Logit模型都是非线性回归模型,因此参数的解释并不像传统的线性回归模型那样直观。
- 1、下载文档前请自行甄别文档内容的完整性,平台不提供额外的编辑、内容补充、找答案等附加服务。
- 2、"仅部分预览"的文档,不可在线预览部分如存在完整性等问题,可反馈申请退款(可完整预览的文档不适用该条件!)。
- 3、如文档侵犯您的权益,请联系客服反馈,我们会尽快为您处理(人工客服工作时间:9:00-18:30)。
比较线性模型和P r o b i t 模型L o g i t模型
Document serial number【LGGKGB-LGG98YT-LGGT8CB-LGUT-
研究生考试录取相关因素的实验报告
一,研究目的
通过对南开大学国际经济研究所1999级研究生考试分数及录取情况的研究,引入录取与未录取这一虚拟变量,比较线性概率模型与Probit模型,Logit模型,预测正确率。
二,模型设定
表1,南开大学国际经济研究所1999级研究生考试分数及录取情况见数据表
定义变量SCORE:考生考试分数;Y:考生录取为1,未录取为0。
上图为样本观测值。
1.线性概率模型
根据上面资料建立模型
用Eviews得到回归结果如图:
Dependent Variable: Y
Method: Least Squares
Date: 12/10/10 Time: 20:38 Sample: 1 97
Included observations: 97
Variable Coefficient Std. Error
t-Statistic
Prob.
C
SCORE
R-squared
Mean dependent var
Adjusted R-squared . dependent var . of regression
Akaike info criterion Sum squared resid Schwarz criterion Log likelihood
F-statistic
Durbin-Watson stat
Prob(F-statistic)
参数估计结果为: i
Y ˆ+ i SCORE Se=( t=
p=
预测正确率:
Forecast: YF Actual: Y
Forecast sample: 1 97 Included observations: 97
Root Mean Squared Error
Mean Absolute Error
Mean Absolute Percentage Error Theil Inequality Coefficient Bias Proportion
Variance Proportion Covariance Proportion
模型
Dependent Variable: Y
Method: ML - Binary Logit (Quadratic hill climbing)
Date: 12/10/10 Time: 21:38
Sample: 1 97
Included observations: 97
Convergence achieved after 11 iterations
Covariance matrix computed using second derivatives Variable
Coefficient Std. Error
z-Statistic
Prob.
C SCORE
Mean dependent var
. dependent var
. of regression
Akaike info criterion Sum squared resid Schwarz criterion Log likelihood
Hannan-Quinn criter. Restr. log likelihood Avg. log likelihood LR statistic (1 df) McFadden R-squared
Probability(LR stat)
Obs with Dep=0
83 Total obs
97
Obs with Dep=1
14
得Logit 模型估计结果如下 p i = F (y i ) =
)
6794.07362.243(11
i x e
+--+ 拐点坐标 ,
其中Y=+
预测正确率
Forecast: YF Actual: Y
Forecast sample: 1 97 Included observations: 97
Root Mean Squared Error
Mean Absolute Error
Mean Absolute Percentage Error Theil Inequality Coefficient Bias Proportion
Variance Proportion Covariance Proportion
模型
Dependent Variable: Y
Method: ML - Binary Probit (Quadratic hill climbing)
Date: 12/10/10 Time: 21:40
Sample: 1 97
Included observations: 97
Convergence achieved after 11 iterations
Covariance matrix computed using second derivatives
Variable Coefficient Std. Error
z-Statistic
Prob.
C
SCORE
Mean dependent var . dependent var
. of regression Akaike info criterion
Sum squared resid Schwarz criterion
Log likelihood Hannan-Quinn criter.
Restr. log likelihood Avg. log likelihood
LR statistic (1 df) McFadden R-squared
Probability(LR stat)
Obs with Dep=0 83 Total obs 97
Obs with Dep=1 14
Probit模型最终估计结果是
p i = F(y i) = F + x i) 拐点坐标 ,
预测正确率
Forecast: YF
Actual: Y
Forecast sample: 1 97
Included observations: 97
Root Mean Squared Error
Mean Absolute Error
Mean Absolute Percentage Error
Theil Inequality Coefficient
Bias Proportion
Variance Proportion
Covariance Proportion
预测正确率结论:线性概率模型RMSE= MAE= MAPE=
Logit模型 RMSE= MAE= MAPE=
Probit模型 RMSE= MAE= MAPE=
由上面结果可知线性概率模型的RMSE、MAE、MAPE 均远远大于Logit模型和Probit模型,说明其误差率比Logit模型和Probit模型大很多,所以正确率远远小于Logit模型和Probit模型。
而Logit模型和Probit模型的RMSE、MAE、MAPE相差很小,所以正确率相差不大。
综上所诉,此数据可以用Logit模型和Probit模型代替线性概率模型进行分析。