A Simple Sample Size Formula for Estimating Means of Poisson Random Variables
Sample Size Determination
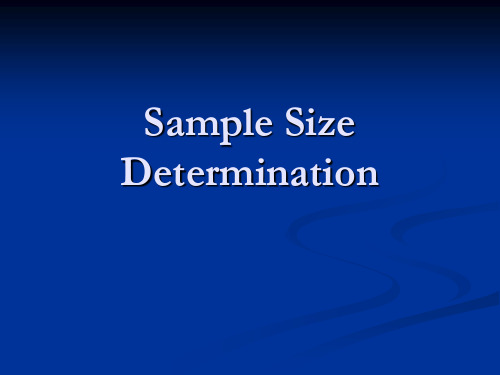
Iቤተ መጻሕፍቲ ባይዱtroduction
Freiman JA, NEJM, 1978;299:690-4
Reviewed the power of 71 published RCTs which had failed to detect a difference
Found that 67 could have missed a 25% therapeutic improvement
Factors That Influence Sample Size Calculations
Is the F/U long enough to be of any clinical relevance Desired level of significance Desired power One or two-tailed test Any explanation for the possible ranges or variations in outcome that is
Factors That Influence Sample Size Calculations
Research subjects
Target population Inclusion & exclusion criteria Baseline risk Pt. compliance rate Pt. drop-out rate
considerations) Adjustments (accounting for potential dropouts or
effect of covariates)
Introduction
Consequences of getting it wrong
Bayesian Forecasting for Financial Risk Management
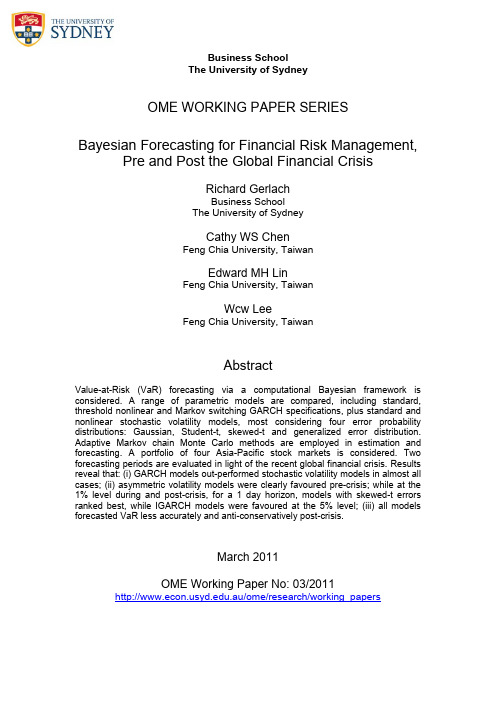
1 Feng 2 University
Chia University, Taiwan of Sydney Business School, Australia
ABSTRACT Value-at-Risk (VaR) forecasting via a computational Bayesian framework is considered. A range of parametric models are compared, including standard, threshold nonlinear and Markov switching GARCH specifications, plus standard and nonlinear stochastic volatility models, most considering four error probability distributions: Gaussian, Student-t, skewed-t and generalized error distribution. Adaptive Markov chain Monte Carlo methods are employed in estimation and forecasting. A portfolio of four Asia-Pacific stock markets is considered. Two forecasting periods are evaluated in light of the recent global financial crisis. Results reveal that: (i) GARCH models out-performed stochastic volatility models in almost all cases; (ii) asymmetric volatility models were clearly favoured pre-crisis; while at the 1% level during and post-crisis, for a 1 day horizon, models with skewed-t errors ranked best, while IGARCH models were favoured at the 5% level; (iii) all models forecasted VaR less accurately and anti-conservatively post-crisis.
英国诺丁汉大学讲义如何估计随机效应模型stata课件

英国诺丁汉大学讲义如何估计随机 效应模型stata
Estimation Methods for Multilevel
Models
Due to additional random effects no simple matrix formulae exist for finding estimates in multilevel models.
• Can easily be extended to more complex problems.
• Potential downside 1: Prior distributions required for all unknown parameters.
• Potential downside 2: MCMC estimation is much slower than the IGLS algorithm.
• Here there are 4 sets of unknown parameters:
,u,u2,e2
• We will add prior distributions
p(
),p(
),p( ) 2
2
u
英e 国诺丁汉大学讲义如何估计随机
sample size selection
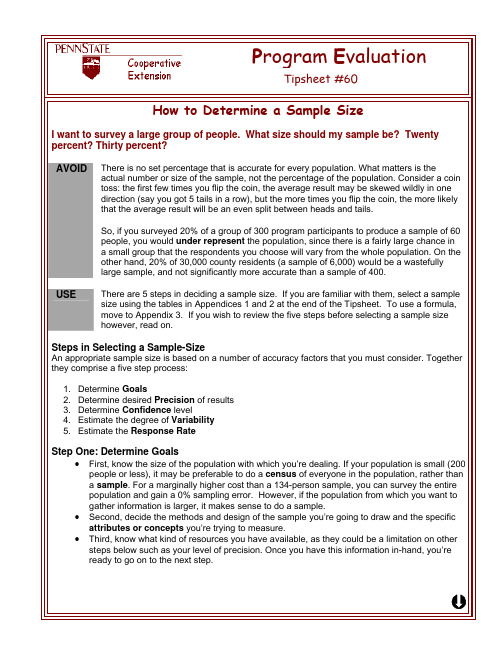
Step Two: Determine the Desired Precision of ResultsThe level of precision is the closeness with which the sample predicts where the true values in the population lie. The difference between the sample and the real population is called the sampling error. If the sampling error is ±3%, this means we add or subtract 3 percentage points from the value in the survey to find out the actual value in the population. For example, if the value in a survey says that 65% of farmers use a particular pesticide, and the sampling error is ±3%, we know that in the real-world population, between 62% and 68% are likely to use this pesticide. This range is also commonly referred to as the margin of error.The level of precision you accept depends on balancing accuracy and resources. High levels of precision require larger sample sizes and higher costs to achieve those samples, but high margins of error can leave you with results that aren’t a whole lot more meaningful than human estimation.The tables in Appendices 1 and 2 at the end of the Tipsheet provide sample sizes for precision levels of 5% and 3% respectively.Step Three: Determine the Confidence LevelThe confidence level involves the risk you’re willing to accept that your sample is within the average or “bell curve” of the population. A confidence level of 90% means that, were the population sampled 100 times in the same manner, 90 of these samples would have the true population value within the range of precision specified earlier, and 10 would be unrepresentative samples. Higher confidence levels require larger sample sizes.The tables at the end of this Tipsheet assume a 95% confidence level. This level is standard for most social-science applications, though higher levels can be used. If the confidence level that is chosen is too low, results will be “statistically insignificant”.Step Four: Estimate the Degree of VariabilityVariability is the degree to which the attributes or concepts being measured in the questions are distributed throughout the population. A heterogeneous population, divided more or less 50%-50% on an attribute or a concept, will be harder to measure precisely than a homogeneous population, divided say 80%-20%. Therefore, the higher the degree of variability you expect the distribution of a concept to be in your target audience, the larger the sample size must be to obtain the same level of precision.To come up with an estimate of variability, simply take a reasonable guess of the size of the smaller attribute or concept you’re trying to measure, rounding up if necessary. If you estimate that 25% of the population in your county farms organically and 75% does not, then your variability would be .25 (which rounds up to 30% on the table provided at the end of this Tipsheet). If variability is too difficult to estimate, it is best to use the conservative figure of 50%.Note: when the population is extremely heterogeneous (i.e., greater than 90-10), a larger sample may be needed for an accurate result, because the population with the minority attribute is so low.At this point, using the level of precision and estimate of variability you’ve selected, you can use either the table or the equation provided at the bottom of this Tipsheet to determine the base sample size for your project.Step Five: Estimate the Response RateThe base sample size is the number of responses you must get back when you conduct your survey. However, since not everyone will respond, you will need to increase your sample size, and perhaps the number of contacts you attempt to account for these non-responses. To estimate response rate that you are likely to get, you should take into consideration the method of your survey and the populationÄinvolved. Direct contact and multiple contacts increase response, as does a population which is interested in the issues, involved, or connected to the institution doing the surveying, or, limited or specialized in character. You can also look at the rates of response that may have occurred in similar, previous surveys.When you’ve come up with an estimate of the percentage you expect to respond, then divide the base sample size by the percentage of response. For example, if you estimated a response rate of 70% and had a base sample size of 220, then your final sample size would be 315 (220/0.7).Once you have this, you’re ready to begin your sampling!One final note about response rates: the past thirty years of research have demonstrated that the characteristics of non-respondents may differ significantly from those of respondents. Follow-up samples may need to be taken of the non-respondent population to determine what differences, if any, may exist.Appendix 1 Example: 5% Error and Qualification.Appendix 2 Example: 3% Error and Qualification.Appendix 3 Example: An Equation for Determining Final Sample Size.References:Blalock, Hubert M. (1972). Social Statistics. New York: McGraw-Hill Book Company.Israel, Glen D. 1992. “Determining Sample Size.” Program Evaluation and Organizational Development, IFAS, University of Florida. PEOD-6.National Science Foundation, Research and Development in Industry: 1992, NSF 95-324. Arlington, VA.Smith, M.F. 1983. “Sampling Considerations in evaluating Cooperative Extension Programs.” Cooperative Extension Service, IFAS, University of Florida. DRAFT.Taylor-Powell, Ellen. May 1998. “Sampling.” Program Development and Evaluation, University of Wisconsin Extension. G3658-3.Sudman, Seymour (1976). Applied Sampling. New York: Academic Press.Warmbrod, J. Robert (1965). “The Sampling Problem in Research Design.” Agriculture Education Magazine. pp 106-107, 114-115.Yamane, Taro (1973). “Statistics: an introductory analysis.” New York: Harper & Row.Jeff Watson, Research Assistant, Cooperative Extension & OutreachThe reference citation for this Tipsheet is: Watson, Jeff (2001). How to Determine a Sample Size: Tipsheet #60, University Park, PA: Penn State Cooperative Extension.Available at: /evaluation/pdf/TS60.pdfThis Web site is copyrighted by The Pennsylvania State University. The information may be used for educational purposes but not sold for profit.ÄAppendix 1: Tables a for Finding a Base Sample Size b+/- 5% Margin of Error cSample SizeVariabilitydPopulation 50% 40% 30% 20% 10%100 e 81 79 63 50 37125 96 93 72 56 40150 110 107 80 60 42175 122 119 87 64 44200 134 130 93 67 45225 144 140 98 70 46250 154 149 102 72 47275 163 158 106 74 48300 172 165 109 76 49325 180 173 113 77 50350 187 180 115 79 50375 194 186 118 80 51400 201 192 120 81 51425 207 197 122 82 51450 212 203 124 83 52500 222 212 128 84 52600 240 228 134 87 53700 255 242 138 88 54800 267 252 142 90 54900 277 262 144 91 551,000 286 269 147 92 552,000 333 311 158 96 573,000 353 328 163 98 574,000 364 338 165 99 585,000 370 343 166 99 586,000 375 347 167 100 587,000 378 350 168 100 588,000 381 353 168 100 589,000 383 354 169 100 5810,000 385 356 169 100 5815,000 390 360 170 101 5820,000 392 362 171 101 5825,000 394 363 171 101 5850,000 397 366 172 101 58100,000 398 367 172 101 58Qualificationsa) This table assumes a 95% confidence level, identifying a risk of 1 in 20 that actual erroris larger than the margin of error (greater than 5%).b) Base sample size should be increased to take into consideration potential non-response.c) A five percent margin of error indicates willingness to accept an estimate within +/- 5 ofthe given value.d) When the estimated population with the smaller attribute or concept is less than 10percent, the sample may need to be increased.e) The assumption of normal population is poor for 5% precision levels when the population is 100or less. The entire population should be sampled, or a lesser precision accepted.Ä。
Using_Minitab_to_Determine_SampleSize

Power 0.9900
Difference 0.9705
Electronics & Safety
Quality Technical Excellence
1 Proportion
Electronics & Safety
Quality Technical Excellence
And the Answer is…
Power and Sample Size
Quality Technical Excellence
And the Answer is…
Power and Sample Size
1-Sample t Test Testing mean = null (versus not = null) Calculating power for mean = null + difference Alpha = 0.05 Sigma = 1 Sample Size 54 Target Power 0.9900 Actual Power 0.9911
Click “Stat”, then Click “Power and Sample Size”, then click “1-Sample Z”
Electronics & Safety
Quality Technical Excellence
Power and Sample Size Dialog Box
Demonstrating Systematic Sampling
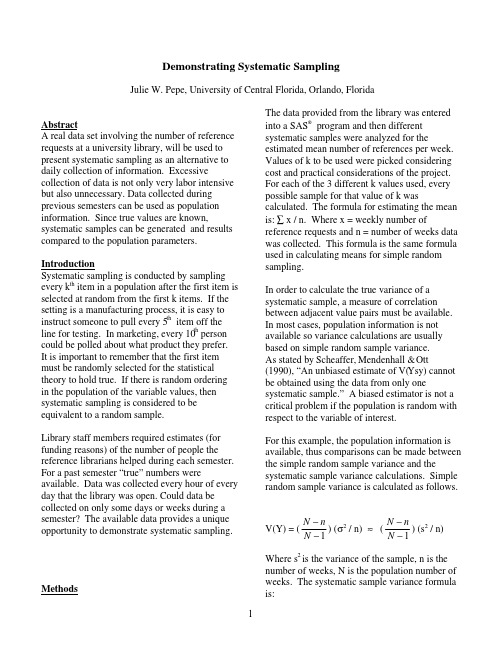
Demonstrating Systematic SamplingJulie W. Pepe, University of Central Florida, Orlando, FloridaAbstractA real data set involving the number of reference requests at a university library, will be used to present systematic sampling as an alternative to daily collection of information. Excessive collection of data is not only very labor intensive but also unnecessary. Data collected during previous semesters can be used as population information. Since true values are known, systematic samples can be generated and results compared to the population parameters. IntroductionSystematic sampling is conducted by sampling every k th item in a population after the first item is selected at random from the first k items. If the setting is a manufacturing process, it is easy to instruct someone to pull every 5th item off the line for testing. In marketing, every 10th person could be polled about what product they prefer. It is important to remember that the first item must be randomly selected for the statistical theory to hold true. If there is random orderingin the population of the variable values, then systematic sampling is considered to be equivalent to a random sample.Library staff members required estimates (for funding reasons) of the number of people the reference librarians helped during each semester. For a past semester “true” numbers were available. Data was collected every hour of every day that the library was open. Could data be collected on only some days or weeks during a semester? The available data provides a unique opportunity to demonstrate systematic sampling. Methods The data provided from the library was entered into a SAS® program and then different systematic samples were analyzed for the estimated mean number of references per week. Values of k to be used were picked considering cost and practical considerations of the project. For each of the 3 different k values used, every possible sample for that value of k was calculated. The formula for estimating the mean is: ∑ x / n. Where x = weekly number of reference requests and n = number of weeks data was collected. This formula is the same formula used in calculating means for simple random sampling.In order to calculate the true variance of a systematic sample, a measure of correlation between adjacent value pairs must be available. In most cases, population information is not available so variance calculations are usually based on simple random sample variance.As stated by Scheaffer, Mendenhall & O tt (1990), “An unbiased estimate of V(Ysy) cannot be obtained using the data from only one systematic sample.” A biased estimator is not a critical problem if the population is random with respect to the variable of interest.For this example, the population information is available, thus comparisons can be made between the simple random sample variance and the systematic sample variance calculations. Simple random sample variance is calculated as follows. V(Y) = (N nN−−1) (σ2 / n) ≈ (N nN−−1) (s2 / n)Where s2 is the variance of the sample, n is the number of weeks, N is the population number of weeks. The systematic sample variance formula is:V(Ysy) = (σ2 / n)[1+(n-1)ρ]where ρ = ()()k nMST SSTn SST−−−11ρ = intracluster correlationMST = mean square totalSST = sums of squares totalk= value of k pickedn= sample sizeThe values necessary are available from PROC ANOVA or PROC GLM output.ResultsPROC MEANS was used to calculate the means and variances of each systematic sample.Table 1 shows the results of k=4, k=3 and k=2 for samples from the 110 weeks of data available. Simple random sample confidence intervals for the mean were calculated in a data step and plotted in Figure 1. This graph gives the client information on what future sample information would look like. Because complete information was available, the plot shows that all the possible samples captured the true mean value (µ=1493). The true mean value is shown as the horizontal line. The vertical lines are formed by the upper and lower limits with the mean marked as a box. Samples 1 to 4 are for k=4, samples 5 to 7 fork=3 and samples 8 to 9 for k=2. The intervals decrease as n increases (k decreases).PROC GLM is used to produce values for calculating the systematic variance. Table 2 shows the PROC GLM results. Calculation of ρ= (3*28)67161-30127481/30127481(27). The resulting value of ρ is -0.030. The intracluster correlation is close to zero therefore, the interpretation is the population is random. The resulting variance calculation for systematic samples would then be 1798.95 (references squared). Bound on the error is ± 83.13 references per week. Figure 2 shows the confidence intervals for samples based on population information (specifically intracluster correlation). As in Figure 1, samples 1 to 4 are for k=4, 5 to 7 for k=3 and 8 to 9 for k=2. SummaryHaving the population information available, reduced the error, yielding smaller confidence intervals. These confidence intervals would not be available when only sample information is collected. These intervals are presented here for demonstration purposes only. Instead of just assuming population values are random, historical data is used to test the assumption. After calculating the intracluster correlation it was determined weeks had random values for the number of reference requests. Thus, systematic sampling is the perfect tool to use in this situation. It cuts down on the amount of data collection yet is an easy method to utilize in the library setting.ReferencesSAS Institute Inc. (1990), SAS Language: Reference, Version 6, First Edition., CaryNC:SAS Institute Inc.SAS Institute Inc. (1990), SAS/STAT Users Guide Vol. 1 and 2, Version 6, Fourth Edition., Cary NC:SAS Institute Inc.Scheaffer, Richard L., Mendenhall, William and Ott, Lyman. (1996), Elementary Survey Sampling, Fifth Edition. Wadsworth Publishing, Belmont, California.The author may be contacted at:University of Central FloridaDepartment of StatisticsPost Office Box 162370Orlando, Florida 32816-2370or pepe@Table 1: Mean and Standard deviations for systematic samples Population Information N= 110 (number of weeks) Mean=1493.44 requestsAnalysis Variable : VALUE-------------------------------------- K = 4 sample 1 -------------------------------------N Mean Std Dev Minimum Maximum28 1466.25 543.1177913 440.0000000 2282.00-------------------------------------- K = 4 sample 2 ------------------------------------- 28 1452.75 609.8147032 336.0000000 2305.00-------------------------------------- K = 4 sample 3 ------------------------------------- 27 1564.00 481.1602963 280.0000000 2155.00-------------------------------------- K = 4 sample 4 ------------------------------------- 27 1493.26 476.4300411 500.0000000 2135.00-------------------------------------- K = 3 sample 1 ------------------------------------- N Mean Std Dev Minimum Maximum37 1484.86 538.2227524 336.0000000 2207.00-------------------------------------- K = 3 sample 2 ------------------------------------- 37 1475.84 535.8665108 280.0000000 2305.00-------------------------------------- K = 3 sample 3 ------------------------------------- 36 1520.33 516.0603508 344.0000000 2282.00-------------------------------------- K = 2 sample 1 ------------------------------------- N Mean Std Dev Minimum Maximum55 1514.24 511.2640821 280.0000000 2282.00-------------------------------------- K = 2 sample 2 ------------------------------------- 55 1472.64 543.7315979 336.0000000 2305.00Table 2: PROC GLM resultsGeneral Linear Models ProcedureDependent Variable: VALUESum of MeanSource DF Squares Square F Value Pr > F Model 3 201485.36936 67161.78979 0.24 0.8698 Error 106 29925995.68519 282320.71401Corrected Total 109 30127481.05455R-Square C.V. Root MSE VALUE Mean0.006688 35.57826 531.33861 1493.4364 Source DF Type I SS Mean Square F Value Pr > FI 3 201485.36936 67161.78979 0.24 0.8698 Source DF Type III SS Mean Square F Value Pr > FI 3 201485.36936 67161.78979 0.24 0.8698Table 3: Confidence Interval CalculationsSimple Random Sample Formula Systematic FormulaOBS LOWER MEAN UPPER N LSYST USYST1 1292.56 1466.25 1639.94 28 1383.12 1549.382 1257.73 1452.75 1647.77 28 1369.62 1535.883 1406.35 1564.00 1721.65 27 1480.87 1647.134 1337.15 1493.26 1649.36 27 1410.13 1576.395 1343.58 1484.86 1626.15 37 1401.73 1567.996 1335.18 1475.84 1616.50 37 1392.71 1558.977 1382.06 1520.33 1658.60 36 1437.20 1603.468 1418.69 1514.24 1609.78 55 1431.11 1597.379 1371.02 1472.64 1574.25 55 1389.51 1555.77。
israel(1992)提出的计算样本大小的公式
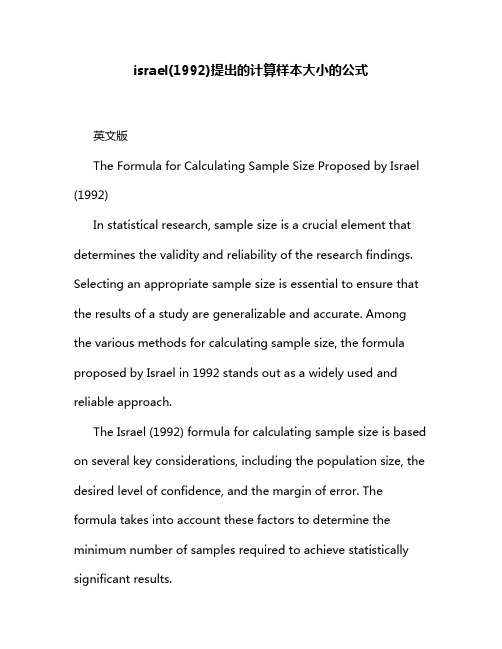
israel(1992)提出的计算样本大小的公式英文版The Formula for Calculating Sample Size Proposed by Israel (1992)In statistical research, sample size is a crucial element that determines the validity and reliability of the research findings. Selecting an appropriate sample size is essential to ensure that the results of a study are generalizable and accurate. Among the various methods for calculating sample size, the formula proposed by Israel in 1992 stands out as a widely used and reliable approach.The Israel (1992) formula for calculating sample size is based on several key considerations, including the population size, the desired level of confidence, and the margin of error. The formula takes into account these factors to determine the minimum number of samples required to achieve statistically significant results.The formula can be expressed as follows:n = N / (1 + N * e^2)where:n represents the sample sizeN is the population sizee is the margin of error, expressed as a decimal (e.g., 0.05 for a 5% margin of error)This formula allows researchers to calculate the sample size based on their specific research requirements and constraints. By plugging in the values for the population size and the desired margin of error, the formula provides a scientifically sound estimate of the minimum number of samples needed for the study.It is important to note that while the Israel (1992) formula provides a useful starting point for sample size calculation, it may not be applicable in all scenarios. The formula assumes a simple random sampling without replacement, and it may needto be adjusted for more complex sampling designs or specific research contexts.Nevertheless, the Israel (1992) formula remains a valuable tool for researchers seeking to determine an appropriate sample size for their studies. By carefully considering the relevant factors and using this formula, researchers can ensure that their sample size is adequate to support statistically valid and reliable research findings.中文版以色列(1992)提出的计算样本大小的公式在统计研究中,样本大小是决定研究结果的有效性和可靠性的关键因素。
sampling and estimation(chp5)经济学专业课 统计学双语资料
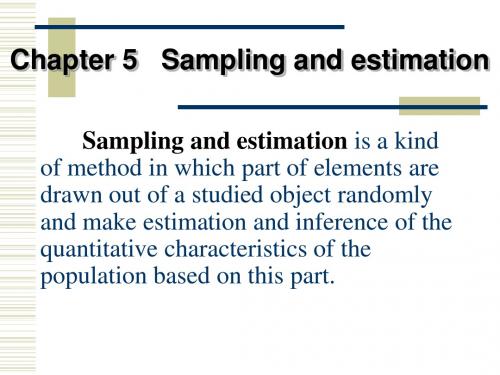
250 400 300 50 Total 1,000
Cluster sampling
Cluster sampling is to draw out a simple random sample of groups or clusters of elements out of a population. This procedure is useful when
5.1 Procedures of sampling and estimation
Sampling design Drawing sample units out of the population Collecting the sample data Organizing the sample data Making the estimation of the quantitative characteristics of the population
A sample of size 1,000 is to be drawn
Stratum Income Population proportion Stratum size
1 2 3 4
under $15,000 15,000-29,999 30.000-50,000 over $50,000
25% 40% 30% 5%
Simple random sampling In simple random sampling, a sample formulated so that each item or person in the population has the same chance / is equally likely to be chosen.
- 1、下载文档前请自行甄别文档内容的完整性,平台不提供额外的编辑、内容补充、找答案等附加服务。
- 2、"仅部分预览"的文档,不可在线预览部分如存在完整性等问题,可反馈申请退款(可完整预览的文档不适用该条件!)。
- 3、如文档侵犯您的权益,请联系客服反馈,我们会尽快为您处理(人工客服工作时间:9:00-18:30)。
a r X i v :0804.3033v 1 [m a t h .S T ] 18 A p r 2008
A Simple Sample Size Formula for Estimating Means
of Poisson Random Variables ∗
Xinjia Chen Submitted in April,2008
Abstract
In this paper,we derive an explicit sample size formula based a mixed criterion of absolute and relative errors for estimating means of Poisson random variables.
1Sample Size Formula
It is a frequent problem to estimate the mean value of a Poisson random variable based on sampling.Specifically,let X be a Poisson random variable with mean E [X ]=λ>0,one wishes to estimate λas λ
= n
i =1X i εa ×ln 2(1+εr )ln(1+εr )−εr
.(1)
It should be noted that conventional methods for determining sample sizes are based on normal approximation,see [3]and the references therein.In contrast,Theorem 1offers a rigorous method for determining sample sizes.To reduce conservatism,a numerical approach has been developed by Chen [1]which permits exact computation of the minimum sample size.
2Proof of Theorem1
We need some preliminary results.
Lemma1Let K be a Poisson random variable with meanθ>0.Then,Pr{K≥r}≤e−θ θe
r r for any positive real number r<θ. Proof.For any real number r>θ,using the Chernoffbound[2],we have
Pr{K≥r}≤inf
t>0
E e t(K−r) =inf t>0∞ i=0e t(i−r)θi
i!e−θe t=inf
t>0
e−θeθe t−r t,
where the infimum is achieved at t=ln r r r. It follows that Pr{K≥r}≤e−θ θe r r.
2 In the sequel,we shall introduce the following function
g(ε,λ)=ε+(λ+ε)ln
λ
r r
=exp(n g(−ε,λ)),
where g(−ε,λ)is monotonically increasing with respect toλ∈(ε,∞)because
∂g(−ε,λ)
λ −ε
have
Pr λ≥λ+ε =Pr{K≥r}≤e−θ θe
=−ln 1+ελ>0.
∂λ
2 Lemma4g(ε,λ)>g(−ε,λ)forλ>ε>0.
Proof.Since g(ε,λ)−g(−ε,λ)=0forε=0and
∂[g(ε,λ)−g(−ε,λ)]
>0
λ2−ε2
forλ>ε>0,we have
g(ε,λ)−g(−ε,λ)>0
for anyε∈(0,λ).Since such arguments hold for arbitraryλ>0,we can conclude that
g(ε,λ)>g(−ε,λ)
forλ>ε>0.2
Lemma5Let0<ε<1.Then,Pr λ≤λ(1−ε) ≤exp(n g(−ελ,λ))and g(−ελ,λ)is mono-tonically decreasing with respect toλ>0.
Proof.Letting K= n i=1X i,θ=nλand r=nλ(1−ε)and making use of Lemma1,for 0<ε<1,we have
Pr λ≤λ(1−ε) =Pr{K≤r}≤e−θ θe
we have
Pr λ≥λ(1+ε) ≤exp(n g(ελ,λ))
where
g(ελ,λ)=[ε−(1+ε)ln(1+ε)]λ,
which is monotonically decreasing with respect toλ>0,sinceε−(1+ε)ln(1+ε)<0forε>0.
2 We are now in a position to prove the theorem.It suffices to show
Pr λ−λ ≥εa& λ−λ ≥εrλ <δ
for n satisfying(1).It can shown that(1)is equivalent to
δ
exp(n g(εa,εa))<
;
εr
Case(iv):λ>εa
.
2
Hence,
Pr λ−λ ≥εa& λ−λ ≥εrλ <δ
Noting that ln2<1,we can show that−εa<g(εa,εa)and hence
Pr{ λ=0}=Pr{X i=0,i=1,···,n}
=[Pr{X=0}]n
=e−nλ
=e−nεa
<exp(n g(εa,εa))
<exp n g εa,εa2 where the second inequality follows from Lemma(3).Hence,
Pr λ−λ ≥εa& λ−λ ≥εrλ <δ
εr +exp n g εa,εa
εr <δ.
In Case(iv),by Lemma(5),Lemma(6)and Lemma(4),we have
Pr λ−λ ≥εa& λ−λ ≥εrλ =Pr λ−λ ≥εrλ
=Pr{ λ≤(1−εr)λ}+Pr{ λ≥(1+εr)λ}
≤exp(n g(−εrλ,λ))+exp(n g(εrλ,λ))
<exp n g −εa,εaεr
<2exp n g εa,εa
[2]Chernoff,H.(1952).A measure of asymptotic efficiency for tests of a hypothesis based on
the sum of observations.Ann.Math.Statist.23493–507.
[3]M.M.Desu and D.Raghavarao,Sample Size Methodology,Academic Press,1990.
6。