Semantics Reinforcement and Fusion Learning for Multimedia Streams
纳米材料基本词汇
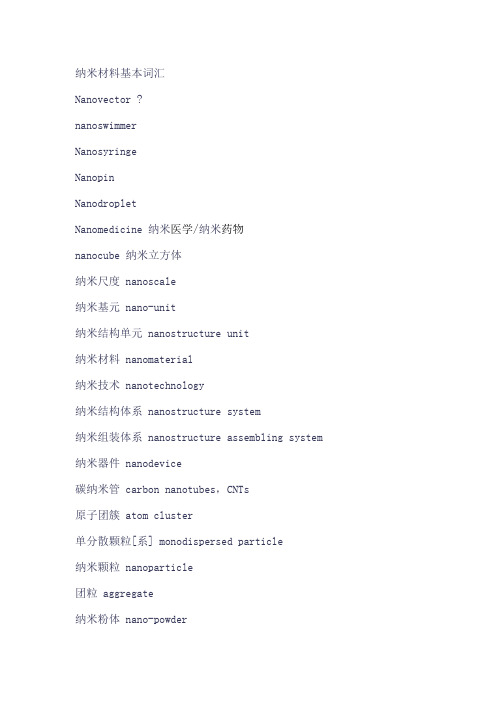
纳米材料基本词汇Nanovector ?nanoswimmerNanosyringeNanopinNanodropletNanomedicine 纳米医学/纳米药物nanocube 纳米立方体纳米尺度 nanoscale纳米基元 nano-unit纳米结构单元 nanostructure unit纳米材料 nanomaterial纳米技术 nanotechnology纳米结构体系 nanostructure system纳米组装体系 nanostructure assembling system 纳米器件 nanodevice碳纳米管 carbon nanotubes,CNTs原子团簇 atom cluster单分散颗粒[系] monodispersed particle纳米颗粒 nanoparticle团粒 aggregate纳米粉体 nano-powder纳米纤维 nano-fibre/Nanofiber纳米薄膜 nano-film纳米块体 nano-bulk纳米孔 nano-pore纳米晶体材料 nanocrystalline material纳米非晶材料 amorphous nanomaterial纳米准晶材料 quasi-crystal nanomaterial金属纳米材料 metallic nanomaterial无机非金属纳米材料 inorganic non-metallic nanomaterial高分子纳米材料 polymer nanomaterial纳米复合材料 nanocomposites结构纳米材料 structured nanomaterial功能纳米材料 functional nanomaterial生物医用纳米材料 biomedical nanomaterial小尺寸效应 small-size effect表面效应 surface effect量子尺寸效应 quantum size effect宏观量子隧道效应 macroscopic quantum tunneling effect,MQT 惰性气体沉积法 inert gas deposition物理粉碎法 physics grinding高能球磨法 high energy ball mill溅射法 sputtering物理粉碎法 physics grinding爆炸法 explosion喷雾法 spraying冷冻干燥法 freeze drying化学气相沉积法 chemical vapor deposition,CVD沉淀法 precipitation水热合成法 hydrothermal synthesis溶胶-凝胶法 sol-gel辐射化学合成法 radiation chemical synthesis快速凝固法 rapidly quenching强烈塑性变形法 severe(intense) plastic deformation 非晶晶化法 amorphous solid crystallization溅射法 sputtering非晶晶化法 crystallization of amorphous solid原位复合法 in-situ composite插层复合法 intercalation hybrids微乳液法 micro emulsion模板合成法 template synthesis自组装法 self-assembly石墨电弧放电法 graphite arc discharge快速凝固法 rapidly quenching表面处理 surface treatment表面修饰 surface decoration稳定化处理 passivating treatmentX射线衍射法 X-ray diffractometry ,XRD扫描探针显微镜 scanning probe microscopy, SPM扫描隧道显微镜 scanning tunneling microscopy, STM扫描近场光学显微镜 scanning near-field optical microscopy, SNOM 原子力显微镜 atomic force microscopy, AFM扫描电容显微镜 scanning capacitance microscopy, SCM磁力显微镜 magnetic force microscopy, MFM扫描热显微镜 scanning thermal microscopy, STHMX射线衍射法 X-ray diffractometry ,XRDX射线衍射线宽化法 X-ray diffractometry line broadening, XRD-LB X射线小角度散射法 small angle X-ray scattering, SAXS透射电子显微镜法 transmission electron microscopy ,TEM透射电镜法 TEM method扫描电子显微镜法 scanning electron microscopy , SEM扫描电镜法 SEM method拉曼光谱法 raman spectrometry红外吸收光谱法 infrared absorption spectroscopy穆斯堡尔谱法 mossbauer spectrometry光子相关谱法 photon correlation spectroscopyBET法 BET压汞仪法 mercury porosimetry纳米压痕仪 nano impress,NI4.6.16扫描探针显微法 scanning probe microscopy, SPM扫描隧道电子显微法 scanning tunneling electron microscopy,STM 扫描近场光学显微法 scanning near-field optical microscopy,SNOM 原子力显微法 atomic force microscopy,AFM扫描电容显微法 scanning capacitance microscopy, SCM扫描热显微法 scanning thermal microscopy, STHM场离子显微法 field ion microscopy, FIM磁力显微法 magnetic force microscopy, MFM激光干涉仪 laser interferometer激光衍射/散射法 laser diffraction and scattering离心沉降法 centrifugal sedimentation。
复合材料英语
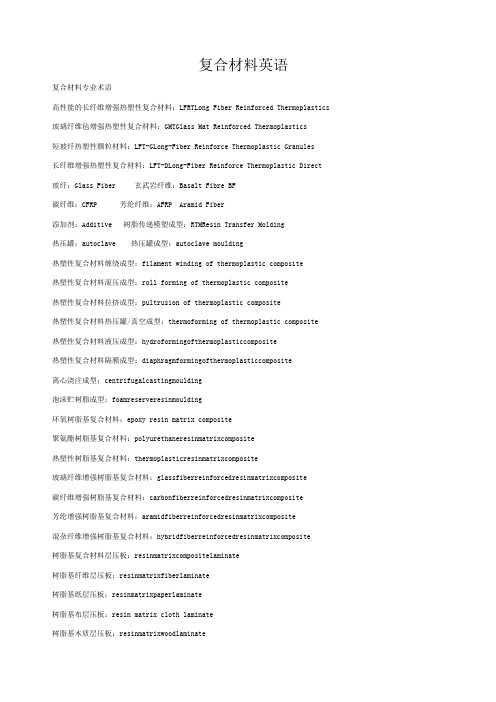
复合材料英语复合材料专业术语高性能的长纤维增强热塑性复合材料:LFRTLong Fiber Reinforced Thermoplastics 玻璃纤维毡增强热塑性复合材料:GMTGlass Mat Reinforced Thermoplastics短玻纤热塑性颗粒材料:LFT-GLong-Fiber Reinforce Thermoplastic Granules长纤维增强热塑性复合材料:LFT-DLong-Fiber Reinforce Thermoplastic Direct 玻纤:Glass Fiber 玄武岩纤维:Basalt Fibre BF碳纤维:CFRP 芳纶纤维:AFRP Aramid Fiber添加剂:Additive 树脂传递模塑成型:RTMResin Transfer Molding热压罐:autoclave 热压罐成型:autoclave moulding热塑性复合材料缠绕成型:filament winding of thermoplastic composite热塑性复合材料滚压成型:roll forming of thermoplastic composite热塑性复合材料拉挤成型:pultrusion of thermoplastic composite热塑性复合材料热压罐/真空成型:thermoforming of thermoplastic composite热塑性复合材料液压成型:hydroformingofthermoplasticcomposite热塑性复合材料隔膜成型:diaphragmformingofthermoplasticcomposite离心浇注成型:centrifugalcastingmoulding泡沫贮树脂成型:foamreserveresinmoulding环氧树脂基复合材料:epoxy resin matrix composite聚氨酯树脂基复合材料:polyurethaneresinmatrixcomposite热塑性树脂基复合材料:thermoplasticresinmatrixcomposite玻璃纤维增强树脂基复合材料:glassfiberreinforcedresinmatrixcomposite碳纤维增强树脂基复合材料:carbonfiberreinforcedresinmatrixcomposite芳纶增强树脂基复合材料:aramidfiberreinforcedresinmatrixcomposite混杂纤维增强树脂基复合材料:hybridfiberreinforcedresinmatrixcomposite树脂基复合材料层压板:resinmatrixcompositelaminate树脂基纤维层压板:resinmatrixfiberlaminate树脂基纸层压板:resinmatrixpaperlaminate树脂基布层压板:resin matrix cloth laminate树脂基木质层压板:resinmatrixwoodlaminate纤维增强金属层压板:fiberreinforcedmetallaminate吸胶材料:bleedingmaterials;bleeder 脱模布:releasecloth喷射成型:spray-upmoulding 纤维缠绕成型:filamentwinding压机模压成型:pressmoulding 拉挤成型:pultrusionprocess预压时间:dwellingtime 预吸胶:debulking 固化:curing加压时机:pressureapplyingopportunity 固化周期:curingcycle固化温度:curingtemperature 脱模剂:moldreleaseagent一、玻璃纤维:GFRP空心纤维:hollow fiber 非织造物:nonwovens, nonwoven fabric毡:mat 连续原丝毡:continuous strand mat, continuous filament mat短切原丝毡:chopped strand mat 干切原丝:dry chopped strands湿切原丝:wet chopped strands 复合毡:combination mat薄毡:veil,tissue 织物:fabric机织物:woven fabric 电子布:electronic fabric, PCB cloth无捻粗纱布/方格布:roving cloth, woven rovings 机织带:woven tape编织物:braided fabric 单向布:unidirectional fabric, UD网布:mesh fabric, scrim 非织造网布:nonwoven scrim, laid scrim陶瓷加工:ceramic processing 表格:tabulation 氧化铝陶瓷管:alumina tube有机物:organics 化学品安全说明书:material safety data sheets MSDS 天然橡胶:nature rubber 碳黑:carbon black 颗粒:particle中大颗粒增强复合材料:large-particle reinforced composites弥散强化复合材料:dispersion-strengthened composites原子或分子水平:atomic or molecular level增强机理:mechanism of reinforcement 直径:diameter晶须:whiskers 单晶:single crystals 硼:boron多晶或非晶体材料:polycrystalline or amorphous material片状结构:laminar composites 夹层结构:sandwich panels低密度:less-dense 硬度:stiffness 强度:strength 延展性:ductility 冲击强度:impact resistance 断裂韧性:fracture toughness拉伸:tension 压缩:compression 脆性材料:brittle material延性材料:ductile material 弹性材料:elastic material拉伸试验:tensile test 树脂:resin 增强体:reinforcement耐磨性:abrasion resistance陶瓷加工:ceramic processing 表格:tabulation 氧化铝陶瓷管:alumina tube有机物:organics 化学品安全说明书:material safety data sheets MSDS 天然橡胶:nature rubber 碳黑:carbon black 颗粒:particle中大颗粒增强复合材料:large-particle reinforced composites弥散强化复合材料:dispersion-strengthened composites原子或分子水平:atomic or molecular level增强机理:mechanism of reinforcement 直径:diameter晶须:whiskers 单晶:single crystals 硼:boron多晶或非晶体材料:polycrystalline or amorphous material片状结构:laminar composites 夹层结构:sandwich panels低密度:less-dense 硬度:stiffness 强度:strength 延展性:ductility 冲击强度:impact resistance 断裂韧性:fracture toughness拉伸:tension 压缩:compression 脆性材料:brittle material延性材料:ductile material 弹性材料:elastic material拉伸试验:tensile test 树脂:resin 增强体:reinforcement 耐磨性:abrasion resistanceAcetyl||乙酰Acid-proof paint||耐酸涂料, 耐酸油漆Acrylic fiber||丙烯酸纤维Acrylic resin||丙烯酸树脂Active filler||活性填料Adapter assembly||接头组件Addition polyimide||加成型聚酰亚胺Addition polymer||加聚物Adjusting valve||调整阀,调节阀Adhersion assembly||粘合装配Adhersion bond||胶结Adjustable-bed press||工作台可调式压力机Adjuster shim||调整垫片Adjusting accuracy||调整精度,调校精度Admissible error||容许误差Admissible load||容许载荷Adsorbed layer||吸附层Advanced composite material||先进复合材料,高级复合材料Advanced development vehicle||试制车,预研样车AEAutomobile Engineering||汽车工程技术Aeolotropic material||各向异性材料Aerated plastics||泡沫塑料, 多孔塑料Aerodynamic body||流线型车身Aft cross member||底盘/车架后横梁Air bleeder||排气孔Air clamp||气动夹具Air deflector||导流板;导风板,气流偏转板Air intake manifold||进气歧管Air servo||伺服气泵Air-tight joint||气密接头All-plastic molded||全塑模注的All polyster seat||全聚酯座椅Alligatoring||龟裂,涂膜皱皮,表面裂痕Amino resin||氨基树脂Angular test||挠曲试验Anti-chipping primer||抗破裂底漆底层涂料Apron||防护挡板Aramid fibre composites||芳胺纤维复合材料Assembly drawing||装配图Assembly jig||装配夹具Assembly part||装配件,组合件Autoclave forming||热压罐成型Autocorrection||自动校正Automatic compensation||自动补偿Automatic feed||自动进料Automobile instrument||汽车仪表板Automotive transmission||汽车传动装置,汽车变速器Auxiliary fasia console||副仪表板Axial strain||轴向应变Axle bushing||轴衬Axle fairing||底盘车桥整流罩A Stage||A 阶段某些热固性树脂聚合作用的初期阶段AACAuxiliary Air Control||辅助空气控制ABCActive Body Control||主动式车身控制装置Abherent||阻粘剂Ability meter||测力计,性能测试仪ABL Ablative||烧蚀剂Ablation||烧蚀Ablative composite material||烧蚀复合材料Ablative insulative material||烧蚀绝热材料Ablative polymer||烧蚀聚合物Ablative prepreg||烧蚀性预浸料Ablative resistance||耐烧蚀性ABRAcrylate Butadience Rubber||丙烯酸丁二烯橡胶Abradant material||研磨材料,磨料Abrade||研磨;用喷砂清理Abrasion||磨耗Abrasion coefficient||磨耗系数Abrasion loss||磨耗量,磨损量Abrasion performance||磨耗性Abrasion-proof material||耐磨材料Abrasion resistant paint||耐磨涂料Abrasion test||磨损试验Abrasive blast system||喷砂清理系统Abrasive cloth||砂布Abrasive disc||砂轮盘,砂轮片Abrasive finishing||抛光Abrasive paper||砂纸Abrasive resistance||耐磨性ABSAcrylonitrile Butadiene Styreneresin||ABS树脂,丙烯腈-丁二烯-苯乙烯热塑性树脂ABSMAmerican Bureau of Standard Materials||美国标准材料局Absolute dynamic modulus||绝对动态模量Absolute error||绝对误差Absorbent material||吸收性材料,吸收性物质,吸声材料,吸收剂Absorber||减振器,阻尼器,缓冲器ACAAutomotive Composite Alliance||汽车复合材料协会ACCAutomatic Clutch Control||自动离合器操纵控制Accelerant||促进剂,加速剂Accelerated aging test||加速老化试验,人工老化试验Accelerator pedal shaft||加速踏板轴Accelerator pump nozzle||加速泵喷嘴Acceptable life||有效使用寿命Acceptance test specification||验收测试规范Access panel||罩板,盖板Accessory||配件,附属品Accessory equipment||辅助设备Accessory kit||附件包,成套附件Accumulator can||储电池外壳Accumulator package||蓄压器组件,蓄压器单元Accuracy in calibration||校准精度Accuracy of finish||最终加工精度Accuracy of manufacture||制造精度Accuracy of positioning||定位精度Accuracy of repetition||重现精度,复制精度Acetal matrix composites||缩醛树脂基复合材料Acetal plastic||缩醛塑料,聚甲醛塑料Acetal resin||缩醛树脂Acetamide||乙酰胺Acetate fiber||醋酸纤维,乙酸纤维Acetone||丙酮Back corner panel||后围角板Back panel||后围板Back side panel||后侧板Back wall pillar||后围立柱Backer||衬料Baffler||挡板,阻尼器;导流叶片Bag Molding||气囊施压成型袋模法Baggage holder||行李架Barrier coat||阻挡层;防渗涂层Batch mixing||分批混合,批混Batching unit||分批加料装置Bearing assembly||轴承组合件Biaxial winding||双角缠绕, 双轴缠绕Binder fiber||粘合纤维Bipolymer||二元共聚物Bismaleimide composites||双马来酰亚胺复合材料Blank placement||坯料的放置Blanket||玻璃纤维毡;坯料Blanking press||冲压机, 冲割压力机Blending resin||掺合树脂BMCBulk Moulding Compound||团状膜塑料BMI Bismaleimide||双马来酰亚胺Body back panel||车身后板Body back wall||车身驾驶室后围Body bracket||车身支架Body control module||车身控制模块Body frame Body skeleton||车身骨架Body front panel||车身驾驶室前围板Body monocoque||单壳体车身,单壳式结构车身Body outer panel||驾驶室覆盖件;驾驶室覆盖件Body structural member||车身结构件Body trim||车身装饰件Bonded riveted structure||胶铆结构Bonnet||发动机罩Brake||制动器Brake arrangement||制动装置Brinell hardness test||布氏硬度试验Brittle coating||脆性涂层Bulk coat||整体涂层Bulk heat treatment||整体热处理Bulk moulding compound||增强塑料预制整体模塑料Bumper bracketholder||保险杠托架Bus brake system||客车制动系Butt flange||对接法兰Butt joint||对接接头;对接Butterfly valve||节流阀,节气门BWI Body In White||白车身Cab deflector shield||驾驶室导流板Cab fairing||驾驶室整流罩Cab floor||驾驶室地板Cab mounting||驾驶室悬置CADComputer Aided Design||计算机辅助设计CAE Computer Aided Engineering||计算机辅助工程设计Calibration tolerance||校准公差Calibrating instrument||校准仪表Camouflage paint||覆面漆, 盖面涂料, 伪假漆Cantilever beam impact test||悬臂梁冲击试验Carbon-felt reinforced carbon composites||碳毡增强碳复合材料Carbon fiber clutch||碳纤维离合器Carbon filament cloth||碳丝织物Case extension||外壳的伸出部分,延伸外壳Casing gasket||外壳密封垫Catalyst manifold||固化剂总成Catalyst pump||固化剂泵Catalyst ratio||固化剂比率Cavity||模槽,型腔;凹模Cavity block||阴模Cavity depth||模槽深度Cellular board||蜂窝状板,多孔板Cellular plastics||泡沫塑料,多孔塑料Centre boss||轮毂Centre pin||销轴,枢轴,主销Centrifugal casting moulding||离心浇铸成型Centrosymmetry||中心对称层板Ceramic matrix composites||陶瓷基复合材料Charge||填充气体,填充料Chasis||底盘;机壳,车架Chlorinated polyethlene||聚氯乙烯Chopped fiber||短切纤维Chopped random mat||短切无序毡Chopped strand||短切原丝CIRTMCo-Injection RTM||共注射RTMClamping fixture||夹具,夹紧装置Clamping force||夹持力,合模力Class A surface||A级表面Clear coat||透明涂层,透明罩漆,清漆层Clear coat finish||清漆涂层Clicker die||冲模Climb milling||同向铣削, 顺铣Clipping press||切边压力机Closure pressing speed||合模速度CMMClosed Mould Moulding||闭合模塑CMTCompression Molding||挤压成型工艺CNCComputerized Numerical Control||电脑数值控制Coarse grinding||粗磨,用砂轮初加工Coating defect||涂层缺陷Collision test||碰撞试验,撞车试验Combination property||综合性能Concept design||概念设计Convection modulus||对流模量Convergence test||收敛试验Cooling fixture||冷却夹具Cooling tower||冷却塔Crazing||龟裂,细裂纹Cresol resin||甲酚树脂Cutting felt||毡的剪切Cutting-off bushing||环形下料模; 下料环Damped structure||阻尼缓冲结构Damper bracket||件振器支架Dashboard illumination||仪表板照明Dash trimming||前围板衬板Deburring||去毛刺,倒角,除飞边Deepdrawing forming||深拉成型Deflection test||挠曲试验Dent resistance||耐冲击性Design freedom||设计自由度Detail drawing||祥图,零件图Die assembly||压模装置Die casting||压模铸件,压模铸法Dimethyl fomamide||二甲基甲酰胺Dimethyl ketone||二甲基甲酮; 丙酮Dip pretreatment||浸渍预处理Die prime coat||浸渍打底漆Dimensional stability||尺寸稳定性Dip coating||浸涂Dip forming||浸渍成型Durability testing||耐久性试验,寿命试验Dwell||保压,暂停加压;滞留时间Dynamometer||测力计Edge effect||边缘效应,边界效应Edge feed||边缘进料Edge gate||侧浇口Ejection force||脱模力Ejector||起模杆Ejector guide pillar||推板导套Ejector housing||支架Elasticizer||增塑剂Elastomeric composites||高弹体复合材料Elongation at break||断裂延伸率Energy absorbing foam||吸能泡沫塑料Epoxy resin||环氧树脂Ether ketone||酮醚Explosion proof||防爆Exterior body panelling||车身外板部蒙皮Exterior trim||外饰,外饰件Fabric composites||织物复合材料Fabric impregnation||织物浸渍Fabric preform||织物预成型Fabric prereg||织物预浸料Fabrication parameter||制造参数Fabrication procedure||制造工序Fabricating machinery||加工设备Face plate coupling||法兰式连接Factory primer||工厂底漆,工厂防锈漆Fairing||整流罩,整流装置Fairing panel||前裙板Fascia bracket||仪表板支架Fascia mask||仪表板罩板Fastening clamp||夹紧装置,紧固夹子Fatigue tension test||拉伸疲劳性试验FCMFibrous Composite material||纤维复合材料FEAFinite Element Anlysis||有限元分析Feed system||供料系统Feeding pump||供给泵Feeding speed||进给速度Female groove||凹模Female mouldtooling||阴模Fender||翼子板;护板Fender apron||挡泥板Fender inner panel||翼子板内衬护板Fiber composite laminate||纤维复合材料层板Fiber mat layer||纤维毡层FinisherFinishing component||装饰件Flange||法兰, 凸缘Flange fitting||法兰式管接头Flash||毛边Flash mold||毛边模具Front sheet metal||车前板制件Fuselage fairing||机身整流装置Gage kit||仪表组,仪表套件Gas cavity||气泡,砂眼Gauge panel||仪表板Gear assembly||齿轮传动装置, 减速器Gearbox cover||变速器壳盖Gear bracket support||齿轮托支架Gel coat||胶衣,凝胶涂层Gel coat drum||胶衣圆桶Gel coat flow monitor||胶衣流量监控器Gel time||凝胶时间Glass fiber winding machine||玻璃纤维缠绕机Glass wool||玻璃棉Glass yarn||玻璃丝Guiding device||导向装置Gunk||预混料Gusset||角撑件Gutter channel||流水槽Hand lay-up ||手工铺叠,手工铺贴Hardness testing machine||硬度测试仪Hauling truck||拖车Header board outside panel||前板外板Headrest||靠枕Heat barrier material||隔热材料Heat forming||热成型High molecular material||高分子材料High pressure bag molding||高压袋成型工艺High pressure injection moulding||高压注射成型,高压注射模塑High-strength structural adhesives||高强度结构粘合剂此资源来自:如需转载,请注明出处,谢谢合作~High temperature coating||高温涂层Hose support||软管支架Hub assembly||毂组件Hub bearing||车轮轮毂轴承Hydraulic device||液压装置Hydraulic engine||液压发动机Hydrostatic strength||流体静力强度IMCIn-Mold Coating||模具内部涂层Immersion paint||浸漆Immersion test||浸渍试验,浸泡试验Immovable support||固定刀架Impact analysis||碰撞试验撞击分析Impact bending||冲击挠曲Impact specimen||冲击试样Impegnate||浸渍Impelling strength||冲击韧性Injection head||注射头Injection-moulded composites||注射模塑复合材料Injection moulded part||注塑制件Injection nozzle||注射喷口,压注喷口Intermittent entry||间歇供给,不连续供给Intermittent failure||间接性故障Izod test||悬臂冲击试验Jack||千斤顶,起重器;传动装置Jack engine||辅助发动机Jackbit insert||切刀,刀具,刃口Jacket||护套,套管,保护罩,蒙皮Jar-proof||防震的Jaw||钳口;定位销Jell||胶凝,凝固,固结Jet milling||喷射研磨Jig||夹具,定位模具Jig-adjusted||粗调的Job program||工作程序Joining nipple||接合螺管Joining on butt||对头接合Joint face of a pattern||分模面Joint gate||分型面内浇口Joint packing||填充垫圈,接合填密Joint sealing material||填缝料Joint-shaped support||铰接支架Joint strenght||连接强度Jump welded tube||对缝焊管,焊接管Junction bolt||接合螺栓Junction point||接点Keeping life||保存期,产品有效期Kenel||型芯Ketene||乙烯酮, 烯酮Ketene dimethyl||二甲酮Ketimide||酰基酮亚胺Ketimine||酮亚胺Ketoamine||酮胺,氨基酮Ketol||乙酮醇Ketone||甲酮Keying strength||咬合强度Knife holder||刀具,刀架Knockout||脱模Knockout pin||脱模销Knockout plate||脱模板Knoop scale||努氏硬度标度Knuckle joint||铰链连接Koplon||高湿模量粘胶纤维Koroseal||氯乙烯树脂Lacquer||挥发性漆;涂漆Lacquer finish||喷漆,上漆,罩光Lacquer formation||漆膜形成,成漆Lacquer putty||腻子,整面用油灰Lacquering ||上清漆Laminate construction thickness||结构层厚度Laminated panel||薄层状板Laminated plastics||层压塑料制品, 塑料层板Laminated thermosetting plastics||层压热固塑料Latex paints ||清漆Lay-up||塑料,夹板的铺叠成型Light-alloy body part||轻合金车身零件Lining ||衬里,衬垫Loaded haul cycle||载货行程Location bearing||定位轴承Location guide||固定导杆,定位导杆Location hole||定位孔Location tolerance||位置公差, 安装公差Locatin pin||定位销Lock bolt||锁紧螺钉Low pressure injection moulding||低压模塑成型Low shrink resin||低收缩树脂Luggage rack||行李架Machining accuracy||加工精度Machining center||加工中心Main shaft gear bushing||主轴齿轮衬套Mandrel ||卷芯,模芯;芯轴Manifold hood||歧管外罩Manual Lay-Up||人工手糊Manual spray-up||手工喷射Manual truck||手推车Manufacturing drawing||制造图纸Matched molds||合模Matrix ||基体,基质Mechanical properties||机械性能Metal bonding||金属粘结Metal-working machine||金属加工机床Methanol||甲醇Mismachining tolerance||加工误差Modular||组装式的Mofulus of elasticity||弹性模量Mould operation||模具操作Moulded plastics||模压塑料Moulding||嵌条;成型;装饰件Mount support||装配支架Multi-axial stress||多轴向应力Multi-tool machining||多刀切削加工||Needled mat||针刺毡,针织毡Non-ductile fracture||无塑性破坏Nontwisting fiber||不加捻纤维Notched izod test||带缺口悬臂梁式冲击试验Nozzle||管嘴,喷嘴Numerically controlled engine lathe||数控普通车床Nylon resin||尼龙树脂OEM Original Equipment Manufacturer ||原始设备生产商Offset cab||侧置驾驶室On-site forming||现场发泡On-site winding||现场缠绕成型Open molding||敞开式模塑法Opening mould||开模Optimized design||优化设计Orifice||注孔Orthophenyl tolyl ketone||邻苯基甲苯基酮Orthophthalic resin ortho||邻苯二甲酸树脂Osmotic pressure||渗透压力Outboard wing||外翼Outer panel skin||蒙皮Oven heating||烘箱加热,加热固化Over-engineering||过份设计的Over flow||溢流Over-spray||过喷Overhead traveling crane||高空移动行车Overhead-valve engine||顶置气门发动机Overhung trailer||外伸式拖车Oxide paint||氧化物涂料Package power||动力装置总成Packed ||紧密的,密实的;有密封的,有填料的Packing||衬垫;填料,密封填料;包装PADPaint As Required||按需涂漆Paint base coat||上底漆Paint blemish||涂漆缺陷Paint blower||喷漆用压力机,喷漆枪Paint brush||涂漆刷Paint dilution||油漆稀释PEPolyethlene||聚乙烯Pedestal mounted||落地安装的Phenolic plastic||酚醛塑料Phenyl ketone||苯基甲酮Pit mounted||嵌入式安装Pivotal arm||枢轴Platic structural component||塑料结构零部件Plastic upholstery||座椅塑料蒙面Play compensation||间隙补偿PLCProgrammable Logical Controller ||可编程序逻辑控制器Polycarbonate plastics||聚碳酸脂塑料Polyester resin||聚脂树脂Polyimide||聚酰亚胺Polymer||聚合物,高分子,多聚体Polyurethane foam||聚氨酯泡沫塑料Polyvinyl||聚乙烯的, 聚乙烯Polyvinyl fluoride||聚氟乙烯Prefabricated parts||成品零部件,制造好的零部件Propylene resin||丙烯类树脂Protecting lacquer||防护漆PSFPolystyrene Foam||聚苯乙烯泡沫塑料PTFEPolytetrafluoroethylene||聚四氟乙烯Pultrusion||拉挤成型Putty knife||油灰腻子刮铲QCQuality Control||质量控制QCSQuality Control Standard||质量管理控制标准QRQuality Requirements||质量规格要求Quality certification||质量认证Quantity production||大量成批生产,大规模生产Quantity production||大量成批生产,大规模生产Quarter panel brace||后侧围板支撑件Quarter panel lower extension||后侧围板下延伸部Quarter trim cap||后侧围装饰板盖Quarte wheel house||后侧围轮滚罩,后侧围车轮室Quasi-isotropic laminate||准各向同性层板Quench||淬火Rack truck||架子车, 移动架Radial dispersion||径向位移Radial loading||径向力载荷Radial pump||径向离心泵Radiation protective paint||防辐射涂料Radiator||散热器Rag||毛刺RARTMRubber-assisted RTM||橡胶辅助RTM用橡胶取代芯材的热膨胀RTMReactive resin||活性树脂, 反应型树脂Rear skirt rail||后围裙边梁Reciprocating engine||活塞式发动机, 往复式发动机Reinforcement||车身加强件,增强材料;构架Repeat accuracy||重复精确度Repeatability||设备重复定位精度Resin formulation||树脂配方Retaining nest||定位槽Return trip||回程,返回行程Rib||筋,加强筋RIFTResin Infusion Under Flexible Tooling||挠性上模具树脂浸渍工艺RIMReaction Injection Molding||反应注射模塑Safety hood||安全罩Sample testing||样品试验Sand wet||车身/涂装湿砂打磨Sandwich body||夹层结构车身Sandwich construction||夹层结构Sandwich panel||多层板,复合板Shaft assembly||轴组件Skin coat||表层;罩面层Solvent reclaim||溶剂的回收Stiffener||加强件Storage modulus||储能模量Stress at definite elongation||定伸应力Stretched actylic plastic||拉伸丙烯酸塑料String milling||连续铣削Stroke||悬架减振器,冲程Structural instrument panel||结构仪表板Structural layer||结构层Styrene||苯乙烯Styrofoam||聚苯乙烯泡沫塑料Surface mat||表面薄毡Synthetic resin paint||合成树脂涂料Tack strength||粘着强度Tail gate||卡车等的后挡板Teflon||聚四氟乙烯塑料, 绝缘材料TERTMThermal-Expansion Resin Transfer Molding||热膨胀树脂传递模塑Thermoplastic plastics||热塑性塑料Thermoset resin||热固性树脂Thickening agent||增粘剂Trim waste||内饰废料Trimming orientation||修边定位Turbulent heating||湍流加热Turndown ratio||衰减比率Twisting stress||扭胁强, 扭应力U bolt||U形螺栓U bolt plate||U 形螺栓垫板Ultimate mechanical strength||极限机械强度Ultraviolent sensitive coating||紫外线感光涂层Undercoat paint||头道漆Uniaxial drawing||单轴拉伸Unsaturated polyester resin||非饱和聚酯树脂Unyielding support||不可压缩支架, 刚性支架Upper yield stress||上屈服应力Urethane coating||氨基甲酸乙酯涂层UVRTMUltra-violet RTM||紫外线固化RTM利用紫外线进行固化VA RTM Vacuum Assisted Resin Transfer Molding ||真空辅助RTMVacuum bag molding||真空袋模制法VARIVacuum Assisted Resin njection||真空辅助树脂注射Variable speed||无级变速Ventilation duct||通风管VentilatorVentilating equipment||通风装置Vibratory stress||振动应力VIMP Variable Infusion Molding Process||可变浸渍模塑Vinyl chloride resin||聚氯乙烯树脂VOCVolatile Organic Compound||挥发性有机化合物Volume modulus||体积模数Vortex generator||车身扰流器,导流板VRVVacuum Reducer Valve||真空减压阀Warping stress||翘曲应力Waste utilization||废物利用,废物处理Water shield||防水罩,挡泥板;密封条Water tolerance||耐水性Wedge gripping||楔形夹具Wheel fender||翼子板Wing trussgrid||翼子挡泥板加强件Winding||缠绕Wingtip assembly||翼尖整流罩Wire drawing||拉丝Wiring press||卷边压力机, 嵌线卷边机Workpiece grippe||工件夹子持器,机械手Woven roving fabric||玻璃纤维无捻粗纱布织物Xylenol Carboxylic Acid||二甲苯酚酸Xlylene||亚二甲苯基Xyster||刮刀X alloy||铜铝合金Xenidium||胶合板Xenidium||胶合板Xylene ||二甲苯Xylene resin||二甲苯树脂Yard-crane||移动吊车,场内移动起重机Yarn count||纱线支数,丝线支数Yarn strength||纱线强度,长丝强度Yield limit||屈服极限,屈服点Yield point under bending stress||弯曲应力下的屈服点Yield stress||屈服应力, 屈服点Yield stress controlled bonding||屈服应力粘结Zedeflon||四氟乙烯均聚物Zero checker||定零位装置, 零位校验Zero clearance||零间隙Zero compensation||零位补偿Zero initial condition||零初始条件Zero setting||仪表零位调整, 置零Zero shrinkage resin||零收缩树脂Zone control||区域控制。
语言学概论期末复习精要语言学概论期末复习精要

1.Students should be prepared to be able to define the following linguistics terms:1)Linguistics语言学Linguistics should be defined as the systematic (or scientific) study of language.2)Language语言it is a system of arbitrary ˈɑ:bitrəri主观的vocal symbols used for human communication. It is a means of verbal communication. It distinguishes us from animals because it is far more sophisticated.[səˈfistikeitid].精密的, 尖端的than animal communication system.3)Langue语言Langue refers to the abstract linguistic systems shared by all the members of a speech community.4)Parole言语refers to the actualized language5)linguistic competence语言能力Competence is the ideal language user's knowledge of the rules of his language or the knowledge that native speakers have of their language as system of abstract formal relation6)linguistic performance语言运用Performance is the actual realization of his knowledge in utterances. It refers to what we do when we speak or listen, that is, the indefinite varied individual acts of verbal behavior with their irregularities, inconsistencies, and errors.7)Phonetics语音学The study of human speech sound.8)Phonology音位学The study of the sound patterning(sounds functioning)9)minimal pairWhen two different forms are identical in every way except for one sound segment which occurs in the same place in the strings, the two sounds combinations are said to form a minimal pair.10)deep structureThe syntactic structure which is formed by the XP rule in accordance with the head’s subcategorization properties, is called deep structure11)surface structureThe syntactic structure ,which corresponding to the final syntactic form of the sentence which results from appropriate transformations is called surface structure.12)Semantics语义学the meaning of language13)Senseit is concerned with the inherent .[inˈhiərənt]固有的; 内在的meaning of the linguistic form. It is the collection of all the features of the linguistic form.14)Referenceit means what a linguistic form refers to in the real, physical world, it deals with the relationship between the linguistic element and the non-linguistic world of experience.15)Pragmatics语用学it is the study of how speakers of a language use sentences to effect successful communication.16)Register语域the type of language which is selected as appropriate to the type of situation is a register.17)Dialect方言A variety of a language used recognizably in a specific region or by a specific social class is called dialect.18)Acquisition获取First language acquisition refers to the child’s acquisition of his mother tongue. Second language acquisition refers to the systematic study of how one person acquires a second language subsequent to his native language.19)inter-lingual errorserrors result from language transfer, or caused by the learner’s mother tongue. i.e. caused by the learner’s NL e.g. “Elle regarde les”20)intra-lingual errorsThey mainly result form faulty or partial learning of the target language. It may be caused by the influence of one TL. Term upon another.21)applied linguisticsit refers to the application of linguistic theories, methods, and findings to the study of language learning and teaching22)the aptitude testit is designed to measure the learner’s aptitude or natural ability to learn languages.23)the achievement testit focus on assessing a testee’s mestery of the contents of a particular course.24)co-operative principleit refers to that the participants in a convasation normally communicate in a maximally efficient natual and cooperative way.2.Students should be able to answer the following questions:1)In what ways does modern linguistics differ from traditional grammar?The focus of research has been transformed from the language system to the use of language.The rise of the inter-displinery research and the borderline介于两者之间的subjects.2)For what reasons does modern linguistics give priority to speech rather than to writing? Speech is prior to writing;The writing system is invented when needed;Today there are languages which can only be spoken but not written;Speech plays a greater role than writing in daily communication;Each human being first acquires speech and then learns writing;Modern linguistics tends to pay more attention to authentic speech.3)What is a phone? How is it differ from a phoneme? How are allophones related to aphoneme?Phone is a phonetic unit or segment.These sounds we hear and produce during linguistic communication are all phones. A phoneme is a phonological unit . It is represented or realized by a certain phone in a certain phonetic context.in English the sounds (phones) that possess the distinctive value are called phonemes.Phonemes are said to be the distinctive sounds.4)What are the five sense relations between words? Please illustrate them. p1361. difference in origin answer-reply;2. difference in the shades不同程度的of meaning;3. difference in socio-expressive meaning;4. difference in stylistic meaning;5. difference in collocation and distribution.5)According to Austin, what are the three acts a person is possibly performing whilemaking an utterance? Give an example.locutionary act (言内行为) illocutionary act(言外行为) perlocutionary act(言后行为)“Good Morning!What did he do ?”He produced a sound, word, or sentence.The act performed in this sense is called locutionary act.言内行为He offered a greeting. illocutionary act言外行为. Locationary act (言内行为) is the act of saying something . It is an act of conveying literal meaning by means of syntax, lexicon and phonology.E.g. “John, it’s very cold outside! ”母亲说出这句话的本身就是言内行为;母亲说出这句话的意图是为了让JOHN穿上大衣再出去,这是言外行为;JOHN听了母亲的话后,穿上大衣在出门,这就是言后行为。
高分子专业术语中英文对照表
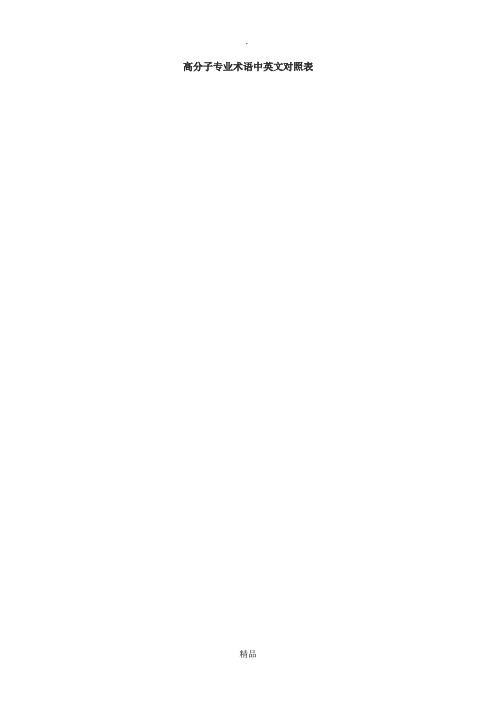
高分子专业术语中英文对照表加工processing反应性加工reactive processing等离子体加工plasma processing加工性processability熔体流动指数melt [flow] index门尼粘度Mooney index塑化plasticizing增塑作用plasticization内增塑作用internal plasticization外增塑作用external plasticization增塑溶胶plastisol增强reinforcing增容作用compatibilization相容性compatibility相溶性intermiscibility生物相容性biocompatibility血液相容性blood compatibility组织相容性tissue compatibility混炼milling, mixing素炼mastication塑炼plastication过炼dead milled橡胶配合rubber compounding共混blend捏和kneading冷轧cold rolling压延性calenderability压延calendering埋置embedding压片preforming模塑molding模压成型compression molding压缩成型compression forming冲压模塑impact moulding, shock moulding 叠模压塑stack moulding复合成型composite molding注射成型injection molding注塑压缩成型injection compression molding 射流注塑jet molding无流道冷料注塑runnerless injection molding 共注塑coinjection molding气辅注塑gas aided injection molding注塑焊接injection welding传递成型transfer molding树脂传递成型resin transfer molding铸塑cast熔铸fusion casting铸塑成型cast molding单体浇铸monomer casting挤出extrusion共挤出coextrusion多层挤塑multi-layer extrusion共挤吹塑coextrusion blow molding同轴挤塑coaxial extrusion吹胀挤塑blown extrusion挤出吹塑extrusion blow molding挤拉吹塑成型extrusion draw blow molding 反应性挤塑reactive extrusion固相挤出solid-phase extrusion发泡expanding foam后发泡post expansion物理发泡physical foam化学发泡chemical foam吹塑blow molding多层吹塑multi-layer blow molding拉伸吹塑成型stretch blow molding滚塑rotational moulding反应注射成型reaction injection molding, RIM 真空成型vacuum forming无压成型zero ressure molding真空烧结vacuum sintering真空袋成型vacuum bag molding热成型thermal forming拉伸热成型stretch thermoforming袋模塑bag molding糊塑paste molding镶铸imbedding冲压成型impact molding触压成型impression molding层压材料laminate泡沫塑料成型foam molding包模成型drape molding充气吹胀inflation橡胶胶乳rubber latex胶乳latex高分子胶体polymer colloid生橡胶raw rubber,crude rubber硬质胶ebonite再生胶reclaimed rubber充油橡胶oil-extended rubber母胶masterbatch交联crosslinking固化cure光固化photo-cure硫化vulcanization后硫化post cure,post vulcanization自硫[化] bin cure自交联self crosslinking , self curing过硫over cure返硫reversion欠硫under cure动态硫化dynamic vulcanization不均匀硫化heterogeneous vulcanization开始[硫化]效应set-up effect自动硫化self-curing, self-vulcanizing焦烧scorching无压硫化non-pressure cure模压硫化moulding curing常温硫化auto-vulcanization热硫化heat curing蒸汽硫化steam curing微波硫化micro wave curing辐射硫化radiation vulcanization辐射交联radiation crosslinking连续硫化continuous vulcanization无模硫化open vulcanization成纤fiber forming可纺性spinnability纺丝spinning干纺dry spinning湿纺wet spinning干湿法纺丝dry wet spinning干喷湿法纺丝dry jet wet spinning溶液纺丝solution spinning乳液纺丝emulsion spinning乳液闪蒸纺丝法emulsion flash spinning process 喷射纺丝jet spinning喷纺成形spray spinning液晶纺丝liquid crystal spinning熔纺melt spinning共混纺丝blended spinning凝胶纺[丝] gel spinning. 反应纺丝reaction spinning静电纺丝electrostatic spinning高压纺丝high-pressure spinning复合纺丝conjugate spinning无纺布non-woven fabrics单丝monofilament, monofil复丝multifilament全取向丝fully oriented yarn中空纤维hollow fiber皮芯纤维sheath core fiber共纺cospinning冷拉伸cold drawing, cold stretching单轴拉伸uniaxial drawing,uniaxial elongation双轴拉伸biaxial drawing多轴拉伸multiaxial drawing皮心效应skin and core effect皮层效应skin effect防缩non-shrink熟成ripening垂挂sag定型sizing起球现象pilling effect捻度twist旦denier特tex纱yarn股strand粘合adhesion反应粘合reaction bonding压敏粘合pressure sensitive adhesion底漆primer浸渍impregnation浸渍树脂solvent impregnated resin基体matrix聚合物表面活性剂polymeric surfactant高分子絮凝剂polymeric flocculant预发颗粒pre-expanded bead高分子膜polymeric membraneH-膜H-filmLB膜Langmuir Blodgett film (LB film)半透膜semipermeable membrane反渗透膜Reverse osmosis membrance多孔膜porous membrane各向异性膜anisotropic membrane正离子交换膜cation exchange membrane 负离子交换膜anionic exchange membrane 吸附树脂polymeric adsorbent添加剂additive固化剂curing agent潜固化剂latent curing agent硫化剂vulcanizing agent给硫剂sulfur donor agent, sulfur donor硫化促进剂vulcanization accelerator硫化活化剂vulcanization activator活化促进剂activating accelerator活化剂activator防焦剂scorch retarder抗硫化返原剂anti-reversion agent塑解剂peptizer偶联剂coupling agent硅烷偶联剂silane coupling agent钛酸酯偶联剂titanate coupling agent铝酸酯偶联剂aluminate coupling agent增强剂reinforcing agent增硬剂hardening agent惰性填料inert filler增塑剂plasticizer辅增塑剂coplasticizer增粘剂tackifier增容剂compatibilizer增塑增容剂plasticizer extender分散剂dispersant agent结构控制剂constitution controller色料colorant荧光增白剂optical bleaching agent抗降解剂antidegradant防老剂anti-aging agent防臭氧剂antiozonant抗龟裂剂anticracking agent抗疲劳剂anti-fatigue agent抗微生物剂biocide防蚀剂anti-corrosion agent光致抗蚀剂photoresist防霉剂antiseptic防腐剂rot resistor防潮剂moisture proof agent除臭剂re-odorant抗氧剂antioxidant热稳定剂heat stabilizer抗静电添加剂antistatic additive抗静电剂antistatic agent紫外线稳定剂ultraviolet stabilizer紫外光吸收剂ultraviolet absorber光稳定剂light stabilizer, photostabilizer光屏蔽剂light screener发泡剂foaming agent物理发泡剂physical foaming agent化学发泡剂chemical foaming agent脱模剂releasing agent内脱模剂internal releasing agent外脱模剂external releasing agent阻燃剂flame retardant防火剂fire retardant烧蚀剂ablator润滑剂lubricant湿润剂wetting agent隔离剂separant增韧剂toughening agent抗冲改性剂impact modifier消泡剂antifoaming agent减阻剂drag reducer破乳剂demulsifier粘度改进剂viscosity modifier增稠剂thickening agent, thickener阻黏剂abhesive洗脱剂eluant附聚剂agglomerating agent后处理剂after-treating agent催干剂drier防结皮剂anti-skinning agent纺织品整理剂textile finishing agent-----------------------高物高化类:结构单元constitutional unit重复结构单元constitutional repeating unit构型单元configurational unit立构重复单元stereorepeating unit立构规整度tacticity等规度, 全同立构[规整]度isotacticity间同度,间同立构[规整]度syndiotacticity无规度,无规立构度atacticity嵌段block规整嵌段regular block非规整嵌段irregular block立构嵌段stereoblock有规立构嵌段isotactic block无规立构嵌段atactic block单体单元monomeric unit二单元组diad三单元组triad四单元组tetrad五单元组pentad无规线团random coil自由连接链freely-jointed chain自由旋转链freely-rotating chain蠕虫状链worm-like chain柔性链flexible chain链柔性chain flexibility刚性链rigid chain棒状链rodlike chain链刚性chain rigidity聚集aggregation聚集体aggregate凝聚、聚集coalescence链缠结chain entanglement凝聚缠结cohesional entanglement物理缠结physical entanglement拓扑缠结topological entanglement凝聚相condensed phase凝聚态condensed state凝聚过程condensing process临界聚集浓度critical aggregation concentration 线团-球粒转换coil-globule transition受限链confined chain受限态confined state物理交联physical crosslinking统计线团statistical coil等效链equivalent chain统计链段statistical segment链段chain segment链构象chain conformation无规线团模型random coil model无规行走模型random walk model自避随机行走模型self avoiding walk model卷曲构象coiled conformation高斯链Gaussian chain无扰尺寸unperturbed dimension扰动尺寸perturbed dimension热力学等效球thermodynamically equivalent sphere近程分子内相互作用short-range intramolecular interaction 远程分子内相互作用long-range intramolecular interaction 链间相互作用interchain interaction链间距interchain spacing长程有序long range order近程有序short range order回转半径radius of gyration末端间矢量end-to-end vector链末端chain end末端距end-to-end distance无扰末端距unperturbed end-to-end distance均方根末端距root-mean-square end-to-end distance伸直长度contour length相关长度persistence length主链;链骨架chain backbone支链branch chain链支化chain branching短支链short-chain branch长支链long-chain branch支化系数branching index支化密度branching density支化度degree of branching交联度degree of crosslinking网络network网络密度network density溶胀swelling平衡溶胀equilibrium swelling分子组装,分子组合molecular assembly自组装self assembly微凝胶microgel凝胶点gel point可逆[性]凝胶reversible gel溶胶-凝胶转化sol-gel transformation临界胶束浓度critical micelle concentration,CMC组成非均一性constitutional heterogenity, compositional heterogenity摩尔质量平均molar mass average数均分子量number-average molecular weight, number-average molar mass 重均分子量weight-average molecular weight, weight-average molar massZ均分子量Z(Zaverage)-average molecular weight, Z-molar mass黏均分子量viscosity-average molecular weight,viscosity-average molar mass 表观摩尔质量apparent molar mass表观分子量apparent molecular weight聚合度degree of polymerization动力学链长kinetic chain length单分散性monodispersity临界分子量critical molecular weight分子量分布molecular weight distribution,MWD多分散性指数polydispersity index,PID平均聚合度average degree of polymerization质量分布函数mass distribution function数量分布函数number distribution function重量分布函数weight distribution function舒尔茨-齐姆分布Schulz-Zimm distribution最概然分布most probable distribution对数正态分布logarithmic normal distribution聚合物溶液polymer solution聚合物-溶剂相互作用polymer-solvent interaction溶剂热力学性质thermodynamic quality of solvent均方末端距mean square end to end distance均方旋转半径mean square radius of gyrationθ温度theta temperatureθ态theta stateθ溶剂theta solvent良溶剂good solvent不良溶剂poor solvent位力系数Virial coefficient排除体积excluded volume溶胀因子expansion factor溶胀度degree of swelling弗洛里-哈金斯理论Flory-Huggins theory哈金斯公式Huggins equation哈金斯系数Huggins coefficientχ(相互作用)参数χ-parameter溶度参数solubility parameter摩擦系数frictional coefficient流体力学等效球hydrodynamically equivalent sphere流体力学体积hydrodynamic volume珠-棒模型bead-rod model球-簧链模型ball-spring [chain] model流动双折射flow birefringence, streaming birefringence动态光散射dynamic light scattering小角激光光散射low angle laser light scattering沉降平衡sedimentation equilibrium沉降系数sedimentation coefficient沉降速度法sedimentation velocity method沉降平衡法sedimentation equilibrium method相对黏度relative viscosity相对黏度增量relative viscosity increment黏度比viscosity ratio黏数viscosity number[乌氏]稀释黏度计[Ubbelohde] dilution viscometer毛细管黏度计capillary viscometer落球黏度计ball viscometer落球黏度ball viscosity本体黏度bulk viscosity比浓黏度reduced viscosity比浓对数黏度inherent viscosity, logarithmic viscosity number 特性黏数intrinsic viscosity, limiting viscosity number黏度函数viscosity function零切变速率黏度zero shear viscosity端基分析analysis of end group蒸气压渗透法vapor pressure osmometry, VPO辐射的相干弹性散射coherent elastic scattering of radiation 折光指数增量refractive index increment瑞利比Rayleigh ratio超瑞利比excess Rayleigh ratio粒子散射函数particle scattering function粒子散射因子particle scattering factor齐姆图Zimm plot散射的非对称性dissymmetry of scattering解偏振作用depolarization分级fractionation沉淀分级precipitation fractionation萃取分级extraction fractionation色谱分级chromatographic fractionation柱分级column fractionation洗脱分级,淋洗分级elution fractionation热分级thermal fractionation凝胶色谱法gel chromatography摩尔质量排除极限molar mass exclusion limit溶剂梯度洗脱色谱法solvent gradient [elution] chromatography 分子量排除极限molecular weight exclusion limit洗脱体积elution volume普适标定universal calibration加宽函数spreading function链轴chain axis等同周期identity period链重复距离chain repeating distance晶体折叠周期crystalline fold period构象重复单元conformational repeating unit几何等效geometrical equivalence螺旋链helix chain构型无序configurational disorder链取向无序chain orientational disorder构象无序conformational disorder锯齿链zigzag chain双[股]螺旋double stranded helix[分子]链大尺度取向global chain orientation结晶聚合物crystalline polymer半结晶聚合物semi-crystalline polymer高分子晶体polymer crystal高分子微晶polymer crystallite结晶度degree of crystallinity, crystallinity高分子[异质]同晶现象macromolecular isomorphism聚合物形态学morphology of polymer片晶lamella, lamellar crystal轴晶axialite树枝[状]晶体dendrite纤维晶fibrous crystal串晶结构shish-kebab structure球晶spherulite折叠链folded chain链折叠chain folding折叠表面fold surface折叠面fold plane折叠微区fold domain相邻再入模型adjacent re-entry model接线板模型switchboard model缨状微束模型fringed-micelle model折叠链晶体folded-chain crystal平行链晶体parallel-chain crystal伸展链晶体extended-chain crystal球状链晶体globular-chain crystal长周期long period近程结构short-range structure远程结构long-range structure成核作用nucleation分子成核作用molecular nucleation阿夫拉米方程Avrami equation主结晶primary crystallization后期结晶secondary crystallization外延结晶,附生结晶epitaxial crystallization外延晶体生长,附生晶体生长epitaxial growth织构texture液晶态liquid crystal state溶致性液晶lyotopic liquid crystal热致性液晶thermotropic liquid crystal热致性介晶thermotropic mesomorphism近晶相液晶smectic liquid crystal近晶中介相smectic mesophase近晶相smectic phase条带织构banded texture环带球晶ringed spherulite向列相nematic phase盘状相discotic phase解取向disorientation分聚segregation非晶相amorphous phase非晶区amorphous region非晶态amorphous state非晶取向amorphous orientation链段运动segmental motion亚稳态metastable state相分离phase separation亚稳相分离spinodal decompositionbimodal decomposition微相microphase界面相boundary phase相容性compatibility混容性miscibility不相容性incompatibility不混容性immiscibility增容作用compatiibilization最低临界共溶(溶解)温度lower critical solution temperature, LCST 最高临界共溶(溶解)温度upper critical solution temperature , UCST 浓度猝灭concentration quenching激基缔合物荧光excimer fluorescence激基复合物荧光exciplex fluorescence激光共聚焦荧光显微镜laser confocal fluorescence microscopy 单轴取向uniaxial orientation双轴取向biaxial orientation, biorientation取向度degree of orientation橡胶态rubber state玻璃态glassy state高弹态elastomeric state黏流态viscous flow state伸长elongation高弹形变high elastic deformation回缩性,弹性复原nerviness拉伸比draw ratio, extension ratio泊松比Poisson's ratio杨氏模量Young's modulus本体模量bulk modulus剪切模量shear modulus法向应力normal stress剪切应力shear stress剪切应变shear strain屈服yielding颈缩现象necking屈服应力yield stress屈服应变yield strain脆性断裂brittle fracture脆性开裂brittle cracking脆-韧转变brittle ductile transition脆化温度brittleness(brittle) temperature延性破裂ductile fracture冲击强度impact strength拉伸强度tensile strength极限拉伸强度ultimate tensile strength抗撕强度tearing strength弯曲强度flexural strength, bending strength弯曲模量bending modulus弯曲应变bending strain弯曲应力bending stress收缩开裂shrinkage crack剪切强度shear strength剥离强度peeling strength疲劳强度fatigue strength, fatigue resistance挠曲deflection压缩强度compressive strength压缩永久变形compression set压缩变形compressive deformation. 压痕硬度indentation hardness洛氏硬度Rockwell hardness布氏硬度Brinell hardness抗刮性scrath resistance断裂力学fracture mechanics力学破坏mechanical failure应力强度因子stress intensity factor断裂伸长elongation at break屈服强度yield strength断裂韧性fracture toughness弹性形变elastic deformation弹性滞后elastic hysteresis弹性elasticity弹性模量modulus of elasticity弹性回复elastic recovery不可回复形变irrecoverable deformation裂缝crack银纹craze形变;变形deformation永久变形deformation set剩余变形residual deformation剩余伸长residual stretch回弹,回弹性resilience延迟形变retarded deformation延迟弹性retarded elasticity可逆形变reversible deformation应力开裂stress cracking应力-应变曲线stress strain curve拉伸应变stretching strain拉伸应力弛豫tensile stress relaxation热历史thermal history热收缩thermoshrinking扭辫分析torsional braid analysis,TBA应力致白stress whitening应变能strain energy应变张量strain tensor剩余应力residual stress应变硬化strain hardening应变软化strain softening电流变液electrorheological fluid假塑性pseudoplastic拉胀性auxiticity牛顿流体Newtonian fluid非牛顿流体non-Newtonian fluid宾汉姆流体Bingham fluid冷流cold flow牛顿剪切黏度Newtonian shear viscosity剪切黏度shear viscosity表观剪切黏度apparent shear viscosity剪切变稀shear thinning触变性thixotropy塑性形变plastic deformation塑性流动plastic flow体积弛豫volume relaxation拉伸黏度extensional viscosity黏弹性viscoelasticity线性黏弹性linear viscoelasticity非线性黏弹性non-linear viscoelasticity蠕变creep弛豫[作用] relaxation弛豫模量relaxation modulus蠕变柔量creep compliance热畸变温度heat distortion temperature弛豫谱relaxation spectrum推迟[时间]谱retardation [time] spectrum弛豫时间relaxation time推迟时间retardation time动态力学行为dynamic mechanical behavior动态黏弹性dynamic viscoelasticity热-机械曲线thermo-mechanical curve动态转变dynamic transition储能模量storage modulus损耗模量loss modulus复数模量complex modulus复数柔量complex compliance动态黏度dynamic viscosity复数黏度complex viscosity复数介电常数complex dielectric permittivity 介电损耗因子dielectric dissipation factor介电损耗常数dielectric loss constant介电弛豫时间dielectric relaxation time玻璃化转变glass transition玻璃化转变温度glass-transition temperature次级弛豫secondary relaxation次级转变secondary transition次级弛豫温度secondary relaxation temperature 开尔文模型Kelvin model麦克斯韦模型Maxwell model时-温叠加原理time-temperature superposition principle玻耳兹曼叠加原理Boltzmann superposition principle平移因子shift factorWLF公式WLF[Williams-Lendel-Ferry] equation软化温度softening temperature平衡熔点equilibrium melting point物理老化physical ageing光老化photoageing热老化thermal ageing热氧老化thermo-oxidative ageing人工老化artificial ageing加速老化accelerated ageing计算机模拟computer simulation分子动力学模拟molecular dynamics simulation蒙特卡洛模拟Monte Carlo simulation---------------------------------聚合反应类:单体monomer官能度functionality平均官能度average functionality双官能[基]单体bifunctional monomer三官能[基]单体trifunctional monomer乙烯基单体vinyl monomer1,1-亚乙烯基单体,偏[二]取代乙烯单体vinylidene monomer1,2-亚乙烯基单体,1,2-二取代乙烯单体vinylene monomer双烯单体,二烯单体diene monomer极性单体polar monomer非极性单体non polar monomer共轭单体conjugated monomer非共轭单体non conjugated monomer活化单体activated monomer官能单体functional monomer大分子单体macromer, macromonomer环状单体cyclic monomer共聚单体comonomer聚合[反应]polymerization均聚反应homopolymerization低聚反应,齐聚反应(曾用名) oligomerization调聚反应telomerization自发聚合spontaneous polymerization预聚合prepolymerization后聚合post polymerization再聚合repolymerization铸塑聚合, 浇铸聚合cast polymerization链[式]聚合chain polymerization烯类聚合,乙烯基聚合vinyl polymerization双烯[类]聚合diene polymerization加[成]聚[合]addition polymerization自由基聚合,游离基聚合(曾用名) free radical polymerization,radical polymerization控制自由基聚合,可控自由基聚合controlled radical polymerization,CRP活性自由基聚合living radical polymerization原子转移自由基聚合atom transfer radical polymerization,ATRP反向原子转移自由基聚合reverse atom transfer radical polymerization,RATRP 可逆加成断裂链转移reversible addition fragmentation chaintransfer,RAFT氮氧[自由基]调控聚合nitroxide mediated polymerization稳定自由基聚合stable free radical polymerization,FRP自由基异构化聚合free radical isomerization polymerization自由基开环聚合radical ring opening polymerization氧化还原聚合redox polymerization无活性端聚合,死端聚合(曾用名) dead end polymerization光[致]聚合photo polymerization光引发聚合light initiated polymerization光敏聚合photosensitized polymerization四中心聚合four center polymerization电荷转移聚合charge transfer polymerization辐射引发聚合radiation initiated polymerization热聚合thermal polymerization电解聚合electrolytic polymerization等离子体聚合plasma polymerization易位聚合metathesis polymerization开环易位聚合ring opening metathesis polymerization,ROMP精密聚合precision polymerization环化聚合cyclopolymerization拓扑化学聚合topochemical polymerization平衡聚合equilibrium polymerization离子[型]聚合ionic polymerization辐射离子聚合radiation ion polymerization离子对聚合ion pair polymerization正离子聚合,阳离子聚合cationic polymerization碳正离子聚合carbenium ion polymerization,carbocationic polymerization 假正离子聚合pseudo cationic polymerization假正离子活[性]聚合pseudo cationic living polymerization活性正离子聚合living cationic polymerization负离子聚合,阴离子聚合anionic polymerization碳负离子聚合carbanionic polymerization活性负离子聚合living anionic polymerization负离子环化聚合anionic cyclopolymerization负离子电化学聚合anionic electrochemical polymerization负离子异构化聚合anionic isomerization polymerization烯丙基聚合allylic polymerization活[性]聚合living polymerization两性离子聚合zwitterion polymerization齐格勒-纳塔聚合Ziegler Natta polymerization配位聚合coordination polymerization配位离子聚合coordinated ionic polymerization配位负离子聚合coordinated anionic polymerization配位正离子聚合coordinated cationic polymerization插入聚合insertion polymerization定向聚合,立构规整聚合stereoregular polymerization, stereospecific polymerization 有规立构聚合tactic polymerization全同立构聚合isospecific polymerization不对称诱导聚合asymmetric induction polymerization不对称选择性聚合asymmetric selective polymerization不对称立体选择性聚合asymmetric stereoselective polymerization对映[体]不对称聚合enantioasymmetric polymerization对映[体]对称聚合enantiosymmetric polymerization异构化聚合isomerization polymerization氢转移聚合hydrogen transfer polymerization基团转移聚合group transfer polymerization,GTP消除聚合elimination polymerization模板聚合matrix polymerization,template polymerization插层聚合intercalation polymerization无催化聚合uncatalyzed polymerization开环聚合ring opening polymerization活性开环聚合living ring opening polymerization不死的聚合immortal polymerization酶聚合作用enzymatic polymerization聚加成反应,逐步加成聚合(曾用名) polyaddition偶联聚合coupling polymerization序列聚合sequential polymerization闪发聚合,俗称暴聚flash polymerization氧化聚合oxidative polymerization氧化偶联聚合oxidative coupling polymerization逐步[增长]聚合step growth polymerization缩聚反应condensation polymerization,polycondensation酯交换型聚合transesterification type polymerization,ester exchange polycondensation自催化缩聚autocatalytic polycondensation均相聚合homogeneous polymerization非均相聚合heterogeneous polymerization相转化聚合phase inversion polymerization本体聚合bulk polymerization, mass polymerization固相聚合solid phase polymerization气相聚合gaseous polymerization,gas phase polymerization吸附聚合adsorption polymerization溶液聚合solution polymerization沉淀聚合precipitation polymerization淤浆聚合slurry polymerization悬浮聚合suspension polymerization反相悬浮聚合reversed phase suspension polymerization 珠状聚合bead polymerization, pearl polymerization分散聚合dispersion polymerization反相分散聚合inverse dispersion polymerization种子聚合seeding polymerization乳液聚合emulsion polymerization无乳化剂乳液聚合emulsifier free emulsion polymerization 反相乳液聚合inverse emulsion polymerization微乳液聚合micro emulsion polymerization连续聚合continuous polymerization半连续聚合semicontinuous polymerization分批聚合,间歇聚合batch polymerization原位聚合in situ polymerization均相缩聚homopolycondensation活化缩聚activated polycondensation熔融缩聚melt phase polycondensation固相缩聚solid phase polycondensation体型缩聚three dimensional polycondensation界面聚合interfacial polymerization界面缩聚interfacial polycondensation环加成聚合cycloaddition polymerization环烯聚合cycloalkene polymerization环硅氧烷聚合cyclosiloxane polymerization引发剂initiator引发剂活性activity of initiator聚合催化剂polymerization catalyst自由基引发剂radical initiator偶氮[类]引发剂azo type initiator2,2′偶氮二异丁腈2,2'- azobisisobutyronitrile, AIBN过氧化苯甲酰benzoyl peroxide, BPO过硫酸盐引发剂persulphate initiator复合引发体系complex initiation system氧化还原引发剂redox initiator电荷转移复合物,电荷转移络合物charge transfer complex, CTC聚合加速剂,聚合促进剂polymerization accelerator光敏引发剂photoinitiator双官能引发剂bifunctional initiator,difunctional initiator 三官能引发剂trifunctional initiator大分子引发剂macroinitiator引发-转移剂initiator transfer agent, inifer引发-转移-终止剂initiator transfer agent terminator, iniferter光引发转移终止剂photoiniferter热引发转移终止剂thermoiniferter正离子催化剂cationic catalyst正离子引发剂cationic initiator负离子引发剂ionioic initiator共引发剂coinitiator烷基锂引发剂alkyllithium initiator负离子自由基引发剂anion radical initiator烯醇钠引发剂alfin initiator齐格勒-纳塔催化剂Ziegler Natta catalyst过渡金属催化剂transition metal catalyst双组分催化剂bicomponent catalyst后过渡金属催化剂late transition metal catalyst金属络合物催化剂metal complex catalyst[二]茂金属催化剂metallocene catalyst甲基铝氧烷methylaluminoxane, MAOμ氧桥双金属烷氧化物催化剂bimetallic μ-oxo alkoxides catalyst双金属催化剂bimetallic catalyst桥基茂金属bridged metallocene限定几何构型茂金属催化剂constrained geometry metallocene catalyst 均相茂金属催化剂homogeneous metallocene catalyst链引发chain initiation热引发thermal initiation染料敏化光引发dye sensitized phtoinitiation电荷转移引发charge transfer initiation诱导期induction period引发剂效率initiator efficiency诱导分解induced decomposition再引发reinitiation链增长chain growth, chain propagation增长链端propagating chain end活性种reactive species活性中心active center持续自由基persistent radical聚合最高温度ceilling temperature of polymerization链终止chain termination双分子终止bimolecular termination初级自由基终止primary radical termination扩散控制终止diffusion controlled termination歧化终止disproportionation termination偶合终止coupling termination单分子终止unimolecular termination自发终止spontaneous termination终止剂terminator链终止剂chain terminating agent假终止pseudotermination自发终止self termination自由基捕获剂radical scavenger旋转光闸法rotating sector method自由基寿命free radical lifetime凝胶效应gel effect自动加速效应autoacceleration effect链转移chain transfer链转移剂chain transfer agent尾咬转移backbitting transfer退化链转移degradation (degradative) chain transfer加成断裂链转移[反应]addition fragmentation chain transfer 链转移常数chain transfer constant①缓聚作用②延迟作用retardation阻聚作用inhibition缓聚剂retarder缓聚剂,阻滞剂retarding agent阻聚剂inhibitor封端[反应]end capping端基terminal group聚合动力学polymerization kinetics聚合热力学polymerization thermodynamics聚合热heat of polymerization共聚合[反应]copolymerization二元共聚合binary copolymerization三元共聚合ternary copolymerization竞聚率reactivity ratio自由基共聚合radical copolymerization离子共聚合ionic copolymerization无规共聚合random copolymerization理想共聚合ideal copolymerization交替共聚合alternating copolymerization恒[组]分共聚合azeotropic copolymerization 接枝共聚合graft copolymerization嵌段共聚合block copolymerization开环共聚合ring opening copolymerization共聚合方程copolymerization equation共缩聚copolycondensation逐步共聚合step copolymerization同种增长homopropagation自增长self propagation交叉增长cross propagation前末端基效应penultimate effect交叉终止cross terminationQ值Q valuee值e valueQ,e概念Q, e scheme序列长度分布sequence length distribution侧基反应reaction of pendant group扩链剂,链增长剂chain extender交联crosslinking化学交联chemical crosslinking自交联self crosslinking光交联photocrosslinking交联度degree of crosslinking硫化vulcanization固化curing硫[黄]硫化sulfur vulcanization促进硫化accelerated sulfur vulcanization 过氧化物交联peroxide crosslinking无规交联random crosslinking交联密度crosslinking density交联指数crosslinking index解聚depolymerization①降解②退化degradation链断裂chain breaking解聚酶depolymerase细菌降解bacterial degradation生物降解biodegradation化学降解chemical degradation辐射降解radiation degradation断链降解chain scission degradation自由基链降解free radical chain degradation无规降解random degradation水解降解hydrolytic degradation热降解thermal degradation热氧化降解thermal oxidative degradation光降解photodegradation光氧化降解photo oxidative degradation力化学降解mechanochemical degradation接枝聚合graft polymerization活化接枝activation grafting接枝点grafting site链支化chain branching支化度degree of branching接枝效率efficiency of grafting接枝度grafting degree辐射诱导接枝radiation induced grafting嵌段聚合block polymerization---------------------------通用类:高分子macromolecule, polymer超高分子supra polymer天然高分子natural polymer无机高分子inorganic polymer有机高分子organic polymer无机-有机高分子inorganic organic polymer金属有机聚合物organometallic polymer元素高分子element polymer高聚物high polymer聚合物polymer低聚物oligomer二聚体dimer三聚体trimer调聚物telomer预聚物prepolymer均聚物homopolymer无规聚合物random polymer无规卷曲聚合物random coiling polymer头-头聚合物head-to-head polymer头-尾聚合物head-to-tail polymer尾-尾聚合物tail-to-tail polymer反式有规聚合物transtactic polymer顺式有规聚合物cistactic polymer规整聚合物regular polymer非规整聚合物irregular polymer无规立构聚合物atactic polymer全同立构聚合物isotactic polymer间同立构聚合物syndiotactic polymer杂同立构聚合物heterotactic polymer有规立构聚合物stereoregular polymer, tactic polymer 苏型双全同立构聚合物threo-diisotactic polymer苏型双间同立构聚合物threo-disyndiotactic polymer 赤型双全同立构聚合物erythro-diisotactic polymer赤型双间同立构聚合物erythro-disyndiotactic polymer 全同间同等量聚合物equitactic polymer共聚物copolymer二元共聚物binary copolymer三元共聚物terpolymer多元聚合物multipolymer序列共聚物sequential copolymer多层共聚物multilayer copolymer多相聚合物multiphase polymer统计[结构]共聚物statistical copolymer无规共聚物random copolymer。
从_爱丽斯漫游奇遇记_和_穿过镜子_看英语的歧义
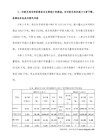
Amdguity)两大类.下面我们结合例句来剖析一下这
两类歧义.
(一)词汇性歧义(LexiealAmbiguity)
在英语中.凡引起句子歧义的词汇通称为歧义
8
性词汇.当句中含有一个或数个歧义性词汇,从而造
成句子的语义不清,我们称这种歧义为词汇性歧义.
self.Andanextremelysmallvoiee.elosetoherear,
said.臼Youmightmakeajokeonit一some-
thingabout’hoarse,youknow·’
—《镜》在这段话中,“hoarse”和”horse”即属同性同音
异义词汇,正是它们造成的句子的歧义.这种歧义只
Queen.
—《镜》这段话的歧义缘于两组歧义性词汇.即:”Flow-
er即<花>和”Flour”<面粉>,以及两个”ground”.
前者属同性同音异义词汇;而后者则为同音异义词
汇,因为Alice所说的”ground”是动词‘grind”<研
磨、磨面>过去分词,而thew卜iteQueen所指的”
ground“则是‘earth,<土地>,为名词.
伙在英语中)读音和词义不存在单一的对应关
系,所以一个人不能总是仅从读音上来确定(词句)
的准确含义”①由此可见,英语中的同音异义是造成
歧义的一个重要原因.
C、隐喻(Metaphor)和成语(Ldiom)也是导致词
汇性歧义的重要因素.如隐喻.其词组或词汇字面上
我们只知其字面意,却不了解们的真正含义.那么,
SLA
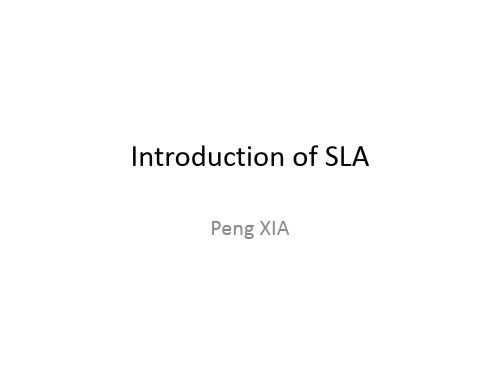
What learners know they are doing and can describe
Lead Ss to reflect their behaviors
Help Ss the generalize
Teacher interference and correction
Final stage
Language learning is a process of imitation, accumulation and recreation
Contrastive analysis
• Describing L1 and L2 • Selecting a linguistic feature • Making L1-L2 comparison(not only differences but also similarities) on this feature • Using the result to predict or explain errors in the learners’ L2 performance • Strong form—predict
Reformulates the hypothesis according to the results of such testing
Inter-language hypothesis
• Transfer errors (developmental errors): an indication of learners’ efforts on the L2
Example:
• Do you need a hand? It looks pretty heavy. • No, it doesn’t matter. (I can manage it myself) Question: why Chinese students tend to make such a mistake?
Reviewing materials12.22
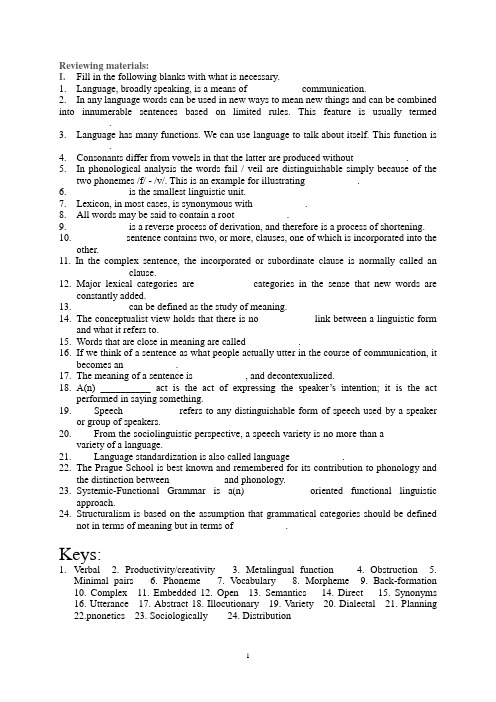
Reviewing materials:I.Fill in the following blanks with what is necessary.1. Language, broadly speaking, is a means of __________ communication.2. In any language words can be used in new ways to mean new things and can be combined into innumerable sentences based on limited rules. This feature is usually termed __________.3. Language has many functions. We can use language to talk about itself. This function is __________.4. Consonants differ from vowels in that the latter are produced without __________.5. In phonological analysis the words fail / veil are distinguishable simply because of thetwo phonemes /f/ - /v/. This is an example for illustrating __________.6. __________ is the smallest linguistic unit.7. Lexicon, in most cases, is synonymous with __________.8. All words may be said to contain a root __________.9. __________ is a reverse process of derivation, and therefore is a process of shortening.10. __________ sentence contains two, or more, clauses, one of which is incorporated into theother.11. In the complex sentence, the incorporated or subordinate clause is normally called an__________ clause.12. Major lexical categories are __________ categories in the sense that new words areconstantly added.13. __________ can be defined as the study of meaning.14. The conceptualist view holds that there is no __________ link between a linguistic formand what it refers to.15. Words that are close in meaning are called __________.16. If we think of a sentence as what people actually utter in the course of communication, itbecomes an __________.17. The meaning of a sentence is __________, and decontexualized.18. A(n) __________ act is the act of expressing the speaker’s intention; it is the actperformed in saying something.19. Speech __________ refers to any distinguishable form of speech used by a speakeror group of speakers.20. From the sociolinguistic perspective, a speech variety is no more than a __________variety of a language.21. Language standardization is also called language __________.22. The Prague School is best known and remembered for its contribution to phonology andthe distinction between __________ and phonology.23. Systemic-Functional Grammar is a(n) __________ oriented functional linguisticapproach.24. Structuralism is based on the assumption that grammatical categories should be definednot in terms of meaning but in terms of __________.Keys:1.Verbal2. Productivity/creativity3. Metalingual function4. Obstruction5.Minimal pairs 6. Phoneme 7. V ocabulary 8. Morpheme 9. Back-formation10. Complex 11. Embedded 12. Open 13. Semantics 14. Direct 15. Synonyms16. Utterance 17. Abstract 18. Illocutionary 19. Variety 20. Dialectal 21. Planning22.pnonetics 23. Sociologically 24. DistributionII.Decide whether the following statements are True (T) or False (F).1. A study of the features of the English used in Shakespeare’s time is an example of the diachronic study of language.2. Speech and writing came into being at much the same time in human history.3. All the languages in the world today have both spoken and written forms.4. Received Pronunciation is the pronunciation accepted by most people.5. The maximal onset principle states that when there is a choice as to where to place aconsonant, it is put into the coda rather than the onset.6. In most cases, the number of syllables of a word corresponds to the number ofmorphemes.7. Back-formation is a productive way of word-formations.8. Minor lexical categories are open because these categories are not fixed and newmembers are allowed for.9. In English syntactic analysis, four phrasal categories are commonly recognized anddiscussed, namely, noun phrase, verb phrase, infinitive phrase, and auxiliary phrase. 10. In English the subject usually precedes the verb and the direct object usually follows theverb.11. The meaning of a sentence is the sum total of the meanings of all its components.12. Most languages have sets of lexical items similar in meaning but ranked differentlyaccording to their degree of formality.13. What essentially distinguishes semantics and pragmatics is whether in the study ofmeaning the context of use is considered.14. The major difference between a sentence and an utterance is that a sentence is not utteredwhile an utterance is.15. The meaning of a sentence is abstract, but context-dependent.16. The most distinguishable linguistic feature of a regional dialect is its grammar and uses ofvocabulary.17. A person’s social backgroun ds do not exert a shaping influence on his choice of linguisticfeatures.18. Every speaker of a language is, in a stricter sense, a speaker of a distinct idiolect.19. American Structuralism is a branch of diachronic linguistics that emerged independentlyin the United States at the beginning of the twentieth century.20. The Standard Theory focuses discussion on language universals and universal grammar. Keys:1—5 FFFFF 6---10 FFFFT 11---15 FTTFF 16---20 FFTFFIII.Match the following linguists with their books and theories.Understand and know what books or theory the following linguists (in your book) has written or put forward.1. A. N. Chomsky2. L. Bloomfield3. G. Leech4. John Austin5. HerbertGrice 6. Gass & Selinker 7. J. R. Firth 8. Sapir 9. Malinowski 10. M. K.Halliday 11. F. Boas 12. D. H. Hymes 13. Sperber and Wilson 14. Saussure IV. Define the following terms.1. the cohort theory2. garden path sentences3. ostensive communication4. interlanguage5. deep structure6. behaviourism7.design features 8. langue9. phoneme 10. I-narrator11. morpheme 12. synonymy13. componential analysis 14. the Sapir-Worf Hypothesis15. cross-cultural communication 16. communicative competence17. cooperative principle 18. function19. metaphorKeys:1.As soon as people hear speech, they start narrowing down the possible words that theymay be hearing. If the first sound they hear is /s/, that eliminates all words beginning with other sounds; if the next sound is /p/, many other possibilities are eliminated. A word is identified as soon as there is only one possibility left. This account is referred to as the COHORT THEORY and hypothesizes that auditory word recognition begins with the formation of a group of words at the perception of the initial sound and proceeds sound by sound with the cohort of words decreasing as more sounds are perceived.2.GARDEN PATH sentences are sentences that are initially interpreted with a differentstructure than they actually have. It typically takes quite a long time to figure out what the other structure is if the first choice turns out to be incorrect. They have been “led up the garden path,” fooled into thinking the sentence has a different structure than it has.Examples are The horse raced past the barn fell. The boat floated downstream sank.While Mary was mending the sock fell off her lap.3. It is one of the two key notions of Sperber and Wilson’s Relevance Theory.Communication is not simply a matter of encoding and decoding, it also involves inference. They maintain that inference has only to do with the hearer. From the speaker’s side, communicat ion should be seen as an act of making clear one’s intention to express something. This is called ostensive act.4. Interlanguage is the type of language constructed by second or foreign languagelearners who are still in the process of learning a language / a language system between the target language and the learner’s native language. It has features of both the t a r g e t l a n g u a g e a n d t h e l e a r n e r’s n a t i v e l a n g u a g e b u t i s n e i t h e r.5.In transformational generative grammar, the deep structure may be defined as the abstractrepresentation of the syntactic properties of a construction, i.e. the underlying level of structural relations between its different constituents, such as the relation between the underlying subject and its verb, or a verb and its object.6. Behaviourism is a principle of scientific method, based on the belief that human beings cannot know anything they have not experienced. Behaviourism in linguistics holds that children learn language through a chain of “stimulus-response reinforcement”, and the adult’s use of language is also a process of stimulus-response.7.The features that define our human languages can be called design feartures.8. Langue refers to the linguistic competence of the speaker.9. A phoneme is the smallest linguistic unit of sound that can signal a difference in meaning.10. The person who tells the story may also be a character in the fictional world of the story, relating the story after the event. In this case the critics call the narrator a first-person narrator or I-narrator.11.Morpheme is defined as the smallest unit in terms of relationship between expression and content. .12. Synonymy is the technical name for the sameness relation.13. Componential analysis defines the meaning of a lexical element in terms of semantic components.The meaning of a word is not an unanalysable whole. It is a complex of different semantic features. There are semantic units smaller than the meaning of a word.14. Our language helps mould our way of thinking and, consequently, different languages may probably express our unique ways of understanding the world. Following this argument, two important points could be captured in the theory. On the one hand, language may determine our thinking patterns; on the other hand, similarity between language is relative, the greater their structural differentiation is, the more diverse their conceptualization of the world will be.15. Cross-cultural communication refers to an exchange of ideas, information, etc. between persons from different cultural backgrounds.16. Communicative competence refers to what a learners knows about how a language us used in particularsituations for effective and appropriate communication. It includes knowledge of the grammar and vocabular, knowledge of rules of speaking, knowledge of how to use and respond to different types of speech acts land social conventions, and knowledge of how to use language appropriately.17. This is the principle suggested by Grice about the regularity in conversation, which reads“Make your conversational contribution such as is required, at the stage at which it occurs, by the accepted purpose or direction of the talk exchange in which you are engaged”.There are four categories of maxims under it, namely, quantity maxim, quality maxim, relation maxim, and manner maxim.18. The role language plays in communication (e.g. to express ideas, attitudes) or in particularsocial situations (e.g. religious, legal).19. Metaphor is a cognitive tool. It involves the comparison of two concepts in that one is construed interms of the other. It’s often described in terms of a target domain and a source domain.V. Do the following according to the requirements.1.Make a componential analysis for the semantic components of father.e contrastive or non-contrastive strategies to analyze the underlined errors andmistakes committed by Chinese learners of English:1)A: Is he recovering from his bad cold?B: Recovering.2)This shows that how capable he is.3.The following is an extract from actual teaching materials. Point out the type of syllabusthat it best fits into.Text Dialogue Grammar Pronunciation/IntonationA Day at My Home 1) Introduction of tenses Intonations of generalCollege 2) Simple present tense questions and their answers3) General questions4) The possessive caseA Letter to Weather 1) Special questions Intonation of speciala Friend 2) Form questions on questionsdifferent parts of asentence3) The cardinal number4) Non-personal “it”4.Mark out the theme and rheme of each of the following sentences according to FunctionalSentence Perspective.1)John was reading the newspaper.2)On the table stands Sally.5. A is reading the newspaper. When B asks “What’s on television tonight?”he answers “Nothing.”What does A mean in normal situations? Think of two situations in which this interpretation of “Nothing” will be cancelled.6. Identify the type of trope employed in the following examples.1) The boy was as cunning as a fox.2) --- the innocent sleep, --- the death of each day’s life, ---3) Buckingham Palace has already been told the train may be axed when the rail network has been privatised.4) Ted Dexter confessed last night that England are in a right old spin as to how they can beat India this winter.7. Analyze the following conversation according to the CP.A: Let’s get the kids something.B: Okey, but I veto I-C-E-C-R-E-A-M-S.8. Analyze the following sentence in a tree diagram according to the phrase structure rules. The man hit the ball.9. Find the deep structure for the following 3 sentences and analyze the types of transformation according to Chomsky’s TG Grammar.1) The man didn’t open the door.2) Did the man open the door?3) The door was opened by the man.10. Consider the following dialogue between a man and his daughter. Try to explain the illocutionary force in each of the utterances.[The daughter walks into the kitchen and takes some popcorn.]Father: I thought you were practicing your violin.Daughter: I need to get the [violin] stand.Father: Is it under the popcorn?Keys:1. father = PARENT ( x, y ) & MALE (x)2. 1) Contrastive. Influenced by the speaker’s knowledge about the habitual expression of his/her mother tongue.2) Non-contrastive. Overgeneralization3. Structural syllabus. The structural syllabus is a grammar oriented syllabus based on a selection of language items and structures. From the teaching materials, we can see there are a lot of rules about the simple tense, general questions, the possesive case, special questions, the cardinal number, and intonations.4. 1) theme: John rheme: was reading the newspaper2) theme: On the table stands rheme: Sally5. Normally “Nothing” here means “Nothing interesting”. If A adds after “Nothing”“The workers are on strike today” or “There’s going to be a blackout tonight”, then the interpretation of “Nothing interesting”will be cancelled.6. (1) The explicit comparison of the boy to a fox is, doubtlessly, a simile.(2) The implicit comparison of “sleep” to “death” is a metaphor.(3) The use of “axed” is a metaphor, since the train will not literally be chopped up like wood.(4) “England”and “India”are metonymic references to the cricket teams which represent England and India respectively.7. This is a case in which B is being deliberately obscure, so that the children will not be able to understand what they are talking about. Children love icecreams, but B doesn’t want thekids to eat icecreams. Therefore, B violates the maxim of manner by saying icecreams in this8.9. The 3 sentences have the same deep structure: The man opened the door. The first sentence has undergone the transformation of negation, the second sentence has undergone the transformation interrogation, and the third sentence has undergone the passive transformation.10. The illocutionary force of “I thought you were practicing your violin” is a criticism of the daughter for her not practicing the violin. That of the daughter’s answer is a defence for her self---I’m going to do that. And that of the father’s retort is a denial of the daughter’s excuse.VI. Answer the following questions.1.What are some of the social factors that are believed to influence our language behavior in a social context?2.How do you understand Krashen’s concept of “i + 1” principle?3.What are the three important points of the Prague School?4.How do you understand Chomsky’s LANGUAGE ACQUISITION DEVICE?5. Does the traffic light system have duality, why?6. What are the special features of American Structuralism?7. What are the problems in IC analysis?8.How is behaviourist psychology related to linguistics?9. If you ask somebody “Can you open the door?” and he answers “Yes” but does not actually do it, what would be your reaction? Why?10. How many stages of development has Chomsky’s TG Grammar undergone?11. Describe the three categories of conceptual metaphors.Keys:1. 1) class 2) gender 3) age 4) ethnic identity 5) education background6) occupation 7) religious belief2. Any input must be comprehensible if it is to have any effect on learning. According to Krashen’s INPUT HYPOTHESIS, learners acquire language as a result of comprehending input addressed to them. Krashen brought forward the concept of “i+ 1” principle, i.e. t he language that learners are exposed to should be just far enough beyond their current competence that they can understand most of it but still be challenged to make progress.3. First, it was stressed that the synchronic study of language is fully justified as it can draw on complete and controllable material for investigation but no rigid theoretical barrier is erected to separate diachronic study. Second, there was an emphasis on the systemic character of language. No element of any language can be satisfactorily analyzed or evaluated if viewed in isolation. Third, language was looked on as functional in another sense, that is, as a tool performing a number of essential functions or tasks for the community using it.4. Chomsky believes that language is somewhat innate, and that children are born with a Language Acquisition Device, which is a unique kind of knowledge that fits them for language learning. He argues the child comes into the world with specific innate endowment, not only with general tendencies or potentialities, but also with knowledge of the nature of the world, and specifically with the knowledge of the nature of language. According to this view, children are born with knowledge of the basic grammatical relations and categories, and this knowledge is universal.5. No. No discrete units on the first level that can be combined freely in the second level to form meaning. There is only simple one-to one relationship between signs and meaning, namely, red— stop, green— go and yellow— get ready to go or stop.6. American Structuralism is a branch of synchronic linguistics that developed in a very different style from of Europe. Structuralism is based on the assumption that grammatical categories should be defined not in terms of meaning but in terms of distribution, and that the structure of each language should be described without reference to the alleged universality of such categories as tense, mood and parts of speech. Firstly, structural grammar describes everything that is found in a language instead of laying down rules. Secondly, structural grammar is empirical, aiming at objectivity in the sense that all definitions and statements should be verifiable or refutable. Thirdly, structural grammar examines all languages recognizing and doing justice to the uniqueness of each language. Lastly, structural grammar describes even the smallest contrasts that underlie any construction or use of a language, not only those discoverable in some particular use.7. There are some technical problems caused by the binary division and discontinuous constituents. But the main problem is that there are structures whose ambiguities cannot be revealed by IC analysis, e.g. the love of God. In terms of both the tree diagram and the labels, there is only one structure, but the word God is in two different relations with love, i.e. either as a subject or object.8. For Bloomfield, linguistics is a branch of psychology, and specifically of the positivistic brand of psychology known as “behaviourism”. Behaviourism is a principle of scientific method, based on the belief that human beings cannot know anything they have not experienced. Behaviourism in linguistics holds that children learn language through a chain of “stimulus-response reinforcement”, and the adult’s use of language is also a process of “stimulus-response”. When the behaviourist methodology entered linguistics via Bloomfield’s writings, the popular practice in linguistic studies was to accept what a native speaker says in his language and to discard what he says about it. This is because of the belief that a linguistic description was reliable when based on observation of unstudied utterances by speakers; itwas unreliable if the analyst had resorted to asking speakers questions such as “Can you say --- in your language?”9. I would be angry with him. “Can you open the door?” is normally a request of the hearer to do it rather than a question about his ability. The fact that he answers “Yes” but does not actually do it shows that he declined my request.10. Chomsky’s TG Grammar has seen five stages of development. The Classical Theory aims to make linguistics a science. The Standard Theory deals with how semantics should be studied in a linguistics theory. The Extended Standard Theory focuses discussion on language universals and universal grammar. The Revised Extended Standard Theory (or GB) focuses discussion on government and binding. The latest is the Minimalist Program, a further revision of the previous theory.11. Lakoff and Johonson classify conceptual meaphors into three categories: ontological metaphors, structural metaphors and orientational metaphors. Ontological metaphors mean that human experiences with physical objects provide the basis for ways of viewing events, activities, emotions, ideas, etc., as entities and substances. Structural metaphors play the most important role because they allow us to go beyond orientation and referring and give us the possibility to structure one concept according to another. This means that structural metaphors are grounded in our experience. Orientational metaphors give a concept a spatial orientation. They are characterized not so much by structuring one concept in terms of another, but by a co-occurrence in our experience. The orientational metaphors are grounded in an experiential basis, which link together the two parts of the metaphor.。
混合函数相关

4Mixed Functions4.1SemanticsMixed aggregation functions are those whose behavior depends on the inputs. These functions exhibit conjunctive,disjunctive or averaging behavior on dif-ferent parts of their domain.We have the following general definition.Definition4.1(Mixed aggregation).An aggregation function is mixed if it is neither conjunctive,nor disjunctive or averaging,i.e.,it exhibits different types of behavior on different parts of the domain.Note4.2.An immediate consequence of the above definition is that mixed aggrega-tion functions are not comparable with min and/or are not comparable with max.The main use of mixed aggregation functions is in those situations where some inputs positively reinforce each other,while other inputs have negative or no reinforcement.This is strongly related to bipolar aggregation,in which some inputs are considered as“positive”and others as“negative”evidence,see Section1.5.For example,in expert systems such as MYCIN and PROSPEC-TOR[38,88],certain pieces of evidence confirm a hypothesis,whereas others disconfirm it(these are called certainty factors).This is modeled by positive and negative inputs on the scale[−1,1],with0being a“neutral”value.We know,however,that any bounded scale can be transformed into[0,1](seep.31),therefore we shall use the inputs from the unit interval,with12beingthe“neutral”value,and interpret the inputs smaller than12as“negative”evidence and those larger than12as“positive”evidence.Example4.3.Consider the following rule systemSymptom A confirms diagnosis D(with certaintyα);Symptom B disconfirms diagnosis D(with certaintyβ);Symptom C confirms diagnosis D(with certaintyγ);etc.The inputs are:A(with certainty a),B(with certainty b),C(with cer-tainty c).1984Mixed FunctionsThe certainty of the diagnosis D on[−1,1]scale is calculated using f(g(a,α),−g(b,β),g(c,γ)),where f is conjunctive for negative inputs,dis-junctive for positive inputs and averaging elsewhere,and g is a different con-junctive aggregation function(e.g.,g=min).A different situation in which the aggregation function needs to be of mixed type is when modeling heterogeneous rules likeIf t1is A1AND(t2is A2OR t3is A3)THEN...and x1,x2,...denote the degrees of satisfaction of the rule predicates t1is A1, t2is A2,etc.Here we want to aggregate the inputs x1,x2,ing a single function f(x),but the rule is a mixture of conjunction and disjunction.Consider two specific examples of mixed type aggregation functions. Example4.4.MYCIN[38]is the name of a famous expert system which was one of thefirst systems capable of reasoning under uncertainty.Certainty factors,represented by numbers on the bipolar scale[−1,1],were combined by means of the functionf(x,y)=⎧⎨⎩x+y−xy,if min(x,y)≥0,x+y1−min(|x|,|y|),if min(x,y)<0<max(x,y),x+y+xy,if max(x,y)≤0.(4.1)This function is symmetric and associative(recall that the latter implies that it is defined uniquely for any number of arguments),but does not define outputs at(−1,1)and(1,−1).It is understood though that the output is-1 in these cases.On[0,1]scale it is given asf(x,y)=⎧⎨⎩2(x+y−xy)−1,if min(x,y)≥12,x+y−11−min(|2x−1|,|2y−1|)+12,if min(x,y)<12<max(x,y),2xy,if max(x,y)≤12.(4.2)Example4.5.PROSPECTOR was another pioneering expert system for min-eral exploration[88].PROSPECTOR’s aggregation function on the scale[−1,1]is defined asf(x,y)=x+y1+xy.(4.3)It is symmetric and associative.It is understood that f(−1,1)=−1.On[0,1]scale it is given asf(x,y)=x+yxy+(1−x)(1−y).(4.4)4.1Semantics199 In both mentioned examples,the aggregation functions exhibited conjunc-tive behavior on[0,12]n and disjunctive behavior on[12,1]n.On the rest of thedomain the behavior was averaging.This is not the only way to partition the domain into disjunctive,conjunctive and averaging parts,as we shall see later in this Chapter.The class of mixed aggregation functions includes many different families. Some of them,such as uninorms,nullnorms,compensatory T-S functions and ST-OWAs,are related–in some sense which will be detailed later–to triangular norms and conorms.Other important families of mixed functions are the symmetric sums and some kinds of generated functions.Uninorms and nullnorms are two popular families of associative aggre-gation functions with clearly defined behavior(see Figure4.1for the two-dimensional case):Fig.4.1.Behavior of uninorms(left)and nullnorms(right).•Uninorms are associative aggregation functions that present conjunctive behavior when dealing with low input values(those below a given valuee which is,in addition,the neutral element),have disjunctive behaviorfor high values(those above e)and are averaging otherwise(i.e.,when receiving a mixture of low and high inputs).•On the other hand,nullnorms are associative aggregation functions that are disjunctive for low values(those below a given value a which is,in addition,the absorbing element),conjunctive for high values(those abovea)and are otherwise averaging(actually,in these cases they provide asthe output the absorbing element a).The aggregation functions in Examples4.4and4.5turn out to be uni-norms,as we shall see in the next section.Of course,not only uninorms and nullnorms exhibit the behavior illustrated on Fig.4.1:some generated func-tions discussed in Section4.4and some symmetric sums behave as uninorms2004Mixed Functions(but they are not associative).However,uninorms and nullnorms are the only types of associative functions with the behavior on Fig.4.1,so we start with them.4.2UninormsUninorms1were introduced as a generalization of t-norms and t-conorms based on the observation that these two classes of aggregation functions(see Chapter3)are defined by means of the same three axioms–associativity, symmetry and possession of a neutral element–just differing in the value of the latter,which is1for t-norms and0for t-conorms.This observation leads to studies of associative and symmetric aggregation functions that have a neutral element which may take any value between the two end points of the unit interval.Since their introduction,uninorms have proved to be useful for practical purposes in different situations,and have been applied in differentfields2. 4.2.1DefinitionUninorms are defined in the bivariate case as follows:Definition4.6(Uninorm).A uninorm is a bivariate aggregation function U:[0,1]2→[0,1]which is associative,symmetric and has a neutral element e belonging to the open interval]0,1[.Note4.7.An alternative definition allows the neutral element e to range over the whole interval[0,1],and thus includes t–norms and t–conorms as special limiting cases.Note4.8.Since uninorms are associative,they are extended in a unique way to func-tions with any number of arguments.Thus uninorms constitute a class of extended aggregation functions(see Definition1.6on p.4).4.2.2Main propertiesThe general behavior of uninorms is depicted in Figure4.1,which shows that a uninorm with neutral element e is conjunctive in the square[0,e]2and disjunctive in the square[e,1]2.More precisely,uninorms act as t-norms in [0,e]2and as t-conorms in[e,1]2,that is,any uninorm with neutral element e is associated with a t-norm T U and a t-conorm S U such that:1Uninorms appeared under this name in1996[278],but a special class of them –nowadays known as the class of representable uninorms–wasfirst studied in 1982[78].2For example,expert systems[65,246]or fuzzy systems modeling[269,277].4.2Uninorms 201∀(x,y )∈[0,e ]2,U (x,y )=e ·T U x e ,y e ,∀(x,y )∈[e,1]2,U (x,y )=e +(1−e )·S U x −e 1−e ,y −e 1−e.The functions T U and S U are usually referred to as the underlying t-norm and t-conorm related to the uninorm U .On the remaining parts of the unit square,uninorms have averaging be-havior,i.e:∀(x,y )∈[0,e ]×[e,1]∪[e,1]×[0,e ],min(x,y )≤U (x,y )≤max(x,y ).Note that contrary to what happens in the squares [0,e ]2and [e,1]2,the behavior of uninorms in the rest of the unit square is not tied to any specific class of (averaging)functions.The structure of uninorms that has just been described is summarized inFigure 4.2,using the following notation:T ∗U (x,y )=e ·T U x e ,y e ,S ∗U (x,y )=e +(1−e )·S U x −e 1−e ,y −e 1−e.Fig.4.2.Structure of a uninorm with neutral element e ,underlying t-norm T U and underlying t-conorm S U .Other interesting properties of uninorms are the following:Absorbing element For any uninorm U (0,1)∈{0,1}.This allows one toclassify uninorms into two different categories:•Conjunctive uninorms are uninorms which verify U (0,1)=0.These uninorms have absorbing element a =0(due to monotonicity).2024Mixed Functions•Disjunctive uninorms are uninorms which verify U(0,1)=1.These uninorms have absorbing element a=1(due to monotonicity). Duality The class of uninorms is closed under duality,that is,the dual of any uninorm U with respect to an arbitrary strong negation N,defined asU d(x,y)=N(U(N(x),N(y))),is also a uninorm,and it has the following properties:•If U has neutral element e,U d has neutral element N(e).•If U is a conjunctive(respectively disjunctive)uninorm,then U d is a disjunctive(resp.conjunctive)uninorm.Clearly,no uninorm can be self-dual,since at least the values U(0,1)and U d(0,1)=N(U(1,0))=N(U(0,1))will be different.Continuity Uninorms are never continuous on the whole unit square.Nev-ertheless,it is possible tofind uninorms that are continuous on the open square]0,1[2.Moreover,there are uninorms which are almost continu-ous,i.e.,which are continuous everywhere except at the corners(0,1)and (1,0).These are called representable uninorms,see Section4.2.3. Idempotency Recall from Chapter3,that the only idempotent t-norms and t-conorms are minimum and maximum respectively.In the case of uni-norms,there are different kinds of idempotent functions3that have been found and characterized[64,170].Strict monotonicity The existence of an absorbing element prevents uni-norms from being strictly monotone in the whole unit square.Notwith-standing,some of them(such as the already mentioned representable uni-norms)are strictly monotone on the open square]0,1[2.4.2.3Main classes of uninormsThere are several classes of uninorms that have been identified and character-ized.Two of the most important and useful ones are described below.The families U min and U maxIn Section4.2.2we saw that in the region[0,e]×[e,1]∪[e,1]×[0,e]uninorms have averaging behavior.This raises the question of whether it is possible to have uninorms acting on this region exactly as the limiting averaging functions min and max.The answer is affirmative;this idea provides two important families of conjunctive and disjunctive uninorms,known,respectively,as U min and U max(seefigure4.3).Proposition4.9(Uninorms U min and U max).3Of course,because of monotonicity(see p.9)such idempotent uninorms are, actually,averaging functions instead of mixed ones.4.2Uninorms203 Fig. 4.3.Structure of the families of uninorms U min(left)and U max(right)asdefined on p.202.•Let T be an arbitrary t-norm,S be an arbitrary t-conorm and e∈]0,1[.The functionU min(T,S,e)(x,y)=⎧⎪⎨⎪⎩e·Txe,ye,if(x,y)∈[0,e]2,e+(1−e)·Sx−e1−e,y−e1−e,if(x,y)∈[e,1]2,min(x,y)otherwise is a conjunctive uninorm with the neutral element e,and the family of all such uninorms is denoted by U min.•Let T be an arbitrary t-norm,S be an arbitrary t-conorm and e∈]0,1[.The functionU max(T,S,e)(x,y)=⎧⎪⎨⎪⎩e·Txe,ye,if(x,y)∈[0,e]2,e+(1−e)·Sx−e1−e,y−e1−e,if(x,y)∈[e,1]2,max(x,y)otherwise is a disjunctive uninorm with the neutral element e,and the family of all such uninorms is denoted by U max.Observe that uninorms in the two mentioned families satisfy the following properties:•If U∈U min then the section U1,given by t→U(1,t),is continuous on [0,e[.•If U∈U max then the section U0,given by t→U(0,t),is continuous on ]e,1].Note4.10.The associativity allows one to determine uniquely the n-ary extensions of the families U min and U max,which are the following:U min(T,S,e)(x1,...,x n)=⎧⎪⎨⎪⎩e·Tx1e,...,x ne,if(x1,...,x n)∈[0,e]n,e+(1−e)·Sx1−e1−e,...,x n−e1−e,if(x1,...,x n)∈[e,1]n,min(x1,...,x n)otherwise,2044Mixed FunctionsU max(T,S,e)(x1,...,x n)=⎧⎪⎨⎪⎩e·Tx1e,...,x ne,if(x1,...,x n)∈[0,e]n, e+(1−e)·Sx1−e1−e,...,x n−e1−e,if(x1,...,x n)∈[e,1]n, max(x1,...,x n)otherwise.Representable uninormsWe saw in Chapter3,that there are t-norms and t-conorms—in particular, the continuous Archimedean ones,—that may be represented in terms of some specific single-variable functions known as their additive(or multiplicative) generators.A similar property exists for uninorms,i.e.,there is a class of uninorms(including both conjunctive and disjunctive ones),usually known as representable uninorms4,that can be built by means of univariate generators: Definition4.11(Representable uninorms).Let u:[0,1]→[−∞,+∞] be a strictly increasing bijection5such that u(e)=0for some e∈]0,1[.•The function given byU(x,y)=u−1(u(x)+u(y)),if(x,y)∈[0,1]2\{(0,1),(1,0)}, 0otherwiseis a conjunctive uninorm with the neutral element e,known as a conjunc-tive representable uninorm.•The function given byU(x,y)=u−1(u(x)+u(y)),if(x,y)∈[0,1]2\{(0,1),(1,0)}, 1otherwiseis a disjunctive uninorm with the neutral element e,known as a disjunctive representable uninorm.The function u is called an additive generator of the uninorm U and it is determined up to a positive multiplicative constant6.Observe that each u provides two different uninorms,a conjunctive one and a disjunctive one,that differ only in the corners(0,1)and(1,0).Note4.12.Similarly to the case of t-norms and t-conorms,representable uninorms may also be described by means of multiplicative generators7.4Also known as generated uninorms,compare with Section4.4.5Note that such a function will verify u(0)=−∞and u(1)=+∞.6That is,if u as an additive generator of U,then so is v(t)=cu(t),c>0.7See,for example,[141],where representable uninorms are called“associative com-pensatory operators”.4.2Uninorms 205Note 4.13.In the case of n arguments,representable uninorms can be built from an additive generator u as follows:•For inputs not containing simultaneously the values 0and 1,that is,for tuples(x 1,...,x n )belonging to [0,1]n \{(x 1,...,x n ):{0,1}⊆{x 1,...,x n }}:U (x 1,...,x n )=u −1n i =1u (x i ) •Otherwise —that is,for tuples containing simultaneously the values 0and 1:–U (x 1,...,x n )=0for a conjunctive uninorm;–U (x 1,...,x n )=1for a disjunctive uninorm.Proposition 4.14(Properties of representable uninorms).1.Representable uninorms are almost continuous (i.e.,continuous on[0,1]2\{(0,1),(1,0)}).Moreover,they are the only uninorms verifying this property (a uninorm is almost continuous if and only if it is representable).2.The function N u :[0,1]→[0,1]given by N u (t )=u −1(−u (t ))is a strong negation with fixed point e ,and U is self-dual ,excluding the points (0,1)and (1,0),with respect to N u ,that is:U (x,y )=N u (U (N u (x ),N u (y )))∀(x,y )∈[0,1]2\{(0,1),(1,0)}.3.Representable uninorms verify thefollowing equalities and inequalities(note that the latter may be understood as a generalization of the Archimedean property of continuous t-norms and t-conorms):a)∀t ∈[0,1[:U (t,0)=0;b)∀t ∈]0,1]:U (t,1)=1;c)∀t ∈]0,1[:U (t,N u (t ))=e ;d)∀t ∈]0,e [:U (t,t )<t ;e)∀t ∈]e,1[:U (t,t )>t .4.Since their generators u are strictly increasing,representable uninorms are strictly increasing on ]0,1[2.5.Strict monotonicity,along with almost continuity,ensure that the underly-ing t-norm and t-conorm of representable uninorms are necessarily strict (see section 3.4.3).Moreover:•If U is a representable uninorm with additive generator u and neutral element e ,then the functions g,h :[0,1]→[0,+∞],given by g (t )=−u (e ·t )and h (t )=u (e +(1−e )·t ),are additive generators,respectively,of the underlying strict t-norm T U and strict t-conorm S U .•Conversely,given a value e ∈]0,1[,a strict t-norm T with an additive generator g and a strict t-conorm S with an additive generator h ,themapping u :[0,1]→[−∞,+∞]defined by2064Mixed Functionsu (t )=−g t e ,if t ∈[0,e ],h t −e 1−e ,if t ∈]e,1],is an additive generator of a representable uninorm with the neutral element e and underlying functions T and S .Note 4.15.With regards to the last statement,it is interesting to point out 8that given a value e ∈]0,1[,a strict t-norm T and a strict t-conorm S ,the triplet (e,T,S )does not determine a unique (conjunctive or disjunctive)representable uninorm with neutral element e ,but rather a family of them.This is due to the fact that additive generators of t-norms and t-conorms (see Proposition 3.43)are unique only up to a multiplicative positive constant,and then one can choose among the different additive generators of T and S .The choice of these generators does not affect the behavior of the corresponding uninorms on the squares [0,e ]2and [e,1]2(T and S always remain the underlying t-norm and t-conorm),but it does influence the behavior obtained on the remaining parts of the domain.For example,let g and h be additive generators of a strict t-norm T and a strict t-conorm S respectively,and take e ∈]0,1[.Then,for each k >0,the function u k (t )= −k ·g t e ,if t ∈[0,e ],h t −e 1−e ,if t ∈]e,1],provides a representable uninorm U k .All the members of the family {U k }k>0have neutral element e ,underlying t-norm T and underlying t-conorm S ,but differ on the region [0,e ]×[e,1]∪[e,1]×[0,e ].4.2.4ExamplesExample 4.16(The weakest and t he strongest uninorms).Given e ∈]0,1[,the weakest and the strongest uninorms with neutral element e are given below (the structure and a 3D plot of these uninorms can be viewed,respectively,in Figures 4.4and 4.5):•The weakest uninorm with neutral element e is the conjunctive uninorm belonging to U min built by means of the weakest t-norm (the drastic prod-uct T D )and the weakest t-conorm,max:U min(T D ,max ,e )(x,y )=⎧⎨⎩0,if (x,y )∈[0,e [2,max(x,y ),if (x,y )∈[e,1]2,min(x,y )otherwise .•The strongest uninorm with the neutral element e is the disjunctive uni-norm belonging to U max built by means of the strongest t-norm,min,and the strongest t-conorm,S D :U max(min ,S D ,e )(x,y )=⎧⎨⎩min(x,y ),if (x,y )∈[0,e ]2,1,if (x,y )∈]e,1]2,max(x,y )otherwise .8For details on this,see [141,184].4.2Uninorms207Fig.4.4.Structure of the weakest(left)and the strongest(right)bivariate uninorms with neutral element e∈]0,1[(example4.16,p.206).Fig.4.5.3D plots of the weakest(left)and the strongest(right)bivariate uninorms with neutral element e=0.5(example4.16,p.206).Example4.17(Idempotent uninorms in U min and U max).Other commonly cited examples of uninorms are the ones obtained from the families U min and U max by choosing T=min and S=max(see Figures4.6and4.7):U min(min,max,e)(x,y)=max(x,y),if(x,y)∈[e,1]2,min(x,y)otherwise.U max(min,max,e)(x,y)=min(x,y),if(x,y)∈[0,e]2,max(x,y)otherwise.Note that the above examples are idempotent,and are,as a consequence, averaging functions.2084MixedFunctionsFig.4.6.Structure of the idempotent uninorms(conjunctive on the left,disjunctive on the right)in the families U min and U max (example4.17,p.207).Fig.4.7.3D plot of the idempotent uninorms in the families U min and U max with the neutral element e=0.5(example4.17,p.207).Example4.18.An important family of parameterized representable uninorms is given by[99,141],see also[78],Uλ(x,y)=λxyλxy+(1−x)(1−y)(x,y)∈[0,1]2\{(0,1),(1,0)},whereλ∈]0,+∞[and either Uλ(0,1)=Uλ(1,0)=0(in which case Uλis conjunctive)or Uλ(0,1)=Uλ(1,0)=1(and then Uλis disjunctive).Uλhas neutral element eλ=11+λand it can be obtained by means of the additive generatoruλ(t)=logλt1−tThe corresponding underlying t–norm and t–conorm are:4.2Uninorms 209T U λ(x,y )=λxy λ+1−(x +y −xy )and S U λ(x,y )=x +y +(λ−1)xy1+λxy which belong to the Hamacher family (see Chapter 3):indeed,a simple calculation shows that T U λis the Hamacher t-norm T H λ+1λ,whereas S U λis theHamacher t-conorm S H λ+1.Example 4.19(The 3−Πfunction).Taking λ=1in the above example provides a well-known representable uninorm,usually referred to as the 3−Πfunction ,which,in the general n -ary case is written as:U (x 1,x 2,...,x n )=ni =1x i ni =1x i +n i =1(1−x i ),with the convention 00=0if one wants to obtain a conjunctive uninorm,andchoosing 00=1in order to obtain a disjunctive one.Its additive generator isu (t )=log t 1−t.The 3−Πfunction is a special case of Dombi’s aggregative operator [78].Note that PROSPECTOR’s aggregation function in Example 4.5is pre-cisely the 3−Πfunction when using the [0,1]scale.Example 4.20(MYCIN’s aggregation function).MYCIN’s aggregation func-tion on [0,1],(cf.Example 4.4)is a conjunctive representable uninorm with an additive generator [65,246]u (t )= log(2t ),if t ≤12,−log(2−2t )otherwise .(4.5)It has neutral element e =12,and the inverse of u is given asu −1(t )= e t2,if t ≤0,1−e −t 2otherwise .(4.6)Thus evaluation of MYCIN’s function for any number of inputs can be done by using U (x )=u −1n i =1u (x i ) ,with u and u −1given in (4.5)and (4.6).2104Mixed FunctionsFig.4.8.3D plots of the 3−Π(left)and MYCIN’s (right)uninorms.The two intersecting straight lines illustrate the presence of the neutral element.Example 4.21.An interesting example arises in the case of u (t )=−log(log λ(t )),where λ∈(0,1).u is a strictly increasing bijection,and u (λ)=0.The inverse is u −1(t )=λexp(−t ).ThenU (x )=λ n i =1log λx i =exp (log λ)1−nn i =1log x i is a representable uninorm with the neutral element λ.9In the special case n =2we obtain an alternative compact expressionU (x,y )=x log λy =y log λx ,and,of course,we can have either conjunctive (U (0,1)=U (1,0)=0)or disjunctive (U (0,1)=U (1,0)=1)cases (compare to the mean in the Example2.26).The limiting cases of λ=1and λ=0correspond to drastic t–norm and t–conorm respectively.The corresponding underlying (strict)t–norm and t–conorm are given,respectively,by the additive generators g (t )=−u (λt )=log(log λ(λt ))and h (t )=u (λ+(1−λ)t )=−log(log λ(λ+(1−λ)t )).Interestingly,the underlying t–norm is related to U by the equationT U (x,y )=xyx log λy =xyU (x,y ).The t–norm T U is a Gumbel-Barnett copula (see Example 3.119,part 4)forλ∈[0,1e ],with parameter a =−1log λ.9To avoid confusion with the Euler number e ,we denote the neutral element by λin this example.4.2Uninorms211 4.2.5CalculationCalculation of numerical values of uninorms can be performed recursively, or,for representable uninorms,by using their additive generators.Similarly to the case of t–norms and t–conorms,care should be taken with respect to evaluation of functions with asymptotic behavior,as well as numerical underflow and overflow.However,evaluation of uninorms presents an additional challenge:these functions are discontinuous,at the very least at the points(0,1)and(1,0). In the surrounding regions,these outputs exhibit instability with respect to input inaccuracies.For example,for a conjunctive uninorm(like MYCIN’s or PROSPECTORS’s functions),the value f(0,1)=0,but f(ε,1)=1for any ε>0,like0.00001.Evaluation using additive generators also has its specifics.For t–norms and t–conorms,an additive generator could be multiplied by any positive constant without changing the value of the t–norm.This is also true for the generators of the representable uninorms.However,when an additive generator is defined piecewise,like in Example4.20,one may be tempted to multiply just one part of the expression aiming at numerical stability.While this does not affect the values of the underlying t–norm or t–conorm in the regions[0,e]n and[e,1]n, it certainly affects the values in the rest of the domain(see Note4.15).4.2.6Fitting to the dataWe examine the problem of choosing the most suitable uninorm based on empirical data,following the general approach outlined in Section1.6.We have a set of empirical data,pairs(x k,y k),k=1,...,K,which we want to fit as best as possible by using a uninorm.Our goal is to determine the best function from that class that minimizes the norm of the differences between the predicted(f(x k))and observed(y k)values.We will use the least squares or least absolute deviation criterion,as discussed on p.33.For uninorms,this problem has two aspects:fitting the actual uninorm and choosing the value of the neutral element.First we consider thefirst part, assuming e isfixed or given.Then we discussfitting the value of e.If the uninorm is given algebraically,e.g.,through parametric families of the underlying t–norms and t–conorms,then we have a generic nonlinear optimization problem offitting the parameter(s)to the data.In doing so, one has to be aware that even if the underlying t–norm and t–conorm are specified,there is a degree of freedom for the values outside[0,e]n and[e,1]n. Therefore a rule for how the values in this part of the domain are determined has to be specified.For representable uninorms,there is an alternative method based on the additive generators.It can be applied in parametric and non-parametric form. In parametric form,when the algebraic form of the additive generators of the underlying t–norm and t–conorm are specified,we aim atfitting three2124Mixed Functionsparameters,λT,λS andα,thefirst two are the parameters identifying a specific member of the families of t–norms/t–conorms,andαis the parameter which determines the values outside[0,e]n and[e,1]n.Specifically,let the underlying t–norm and t–conorm have additive gener-ators gλT and hλS.The additive generator of the uninorm with the neutralelement e has the formu(t;λT,λS,α)=−gλT(t/e),if t≤e,αhλS(t−e1−e)otherwise.Note that for anyα>0u generates a uninorm with exactly the same underly-ing t–norm and t–conorm,but the values outside[0,e]n and[e,1]n depend on α.Therefore,the least squares or LAD criterion has to be minimized with re-spect to variablesλT,λS andα>0.This is a nonlinear optimization problem with possibly multiple locally optimal solutions.We recommend using global optimization methods discussed in the Appendix A.5.4and A.5.5.The nonparametric approach is similar to the one used tofit additive generators of t–norms in Section3.4.15.We represent the additive generator with a monotone increasing regression splineS(t)=Jj=1c j B j(t),(4.7)with the basis functions B j chosen in such a way that the monotonicity con-dition is expressed as a set of linear inequalities(see[13,15]).Our goal is to determine from the data the unknown coefficients c j>0(for monotone increasing S),subject to conditionsS(e)=Jj=1c j B j(e)=0,S(a)=Jj=1c j B j(a)=1.Since the underlying t–norm and t–conorms are necessarily strict,we use the well-founded generators[136,137],defined asu(t)=⎧⎪⎨⎪⎩−(1t+S(ε1)−1ε1),if t≤ε1,11−t+S(1−ε2)−1ε2,if1−t≤ε2,S(t)otherwise.(4.8)The values ofε1,ε2are chosen in such a way thatε1is smaller than the smallest strictly positive number out of x ik,y k,i=1,...,n k,k=1...,K,andε2is smaller than the smallest strictly positive number out of1−x ik,1−y k,i= 1,...,n k,k=1...,K.The value a can be chosen as a=min{ε1,1−ε2}.Fitting the coefficients is performed by solving a quadratic programming problem(in the case of LS criterion)。
- 1、下载文档前请自行甄别文档内容的完整性,平台不提供额外的编辑、内容补充、找答案等附加服务。
- 2、"仅部分预览"的文档,不可在线预览部分如存在完整性等问题,可反馈申请退款(可完整预览的文档不适用该条件!)。
- 3、如文档侵犯您的权益,请联系客服反馈,我们会尽快为您处理(人工客服工作时间:9:00-18:30)。
Semantics Reinforcement and Fusion Learning forMultimedia StreamsDhiraj Joshi Department of Computer Science and Engineering The Pennsylvania StateUniversity University Park,P A16802 djoshi@Milind NaphadeIBM Thomas J.WatsonResearch CenterHawthorne,NY10532naphade@Apostol NatsevIBM Thomas J.WatsonResearch CenterHawthorne,NY10532natsev@ABSTRACTFusion of multimedia streams for enhanced performance is a critical problem for retrieval.However,fusion performance tends to easily overfit the hillclimb set used to learn fusion rules.In this paper,we perform fusion learning for multimedia streams using a greedy performance driven algorithm.In our fusion learning paradigm,fused output is a linear combination of multiple classifiers or ranked streams.The algorithm is inspired from Ensemble Learning [2]but takes that idea further for improving generalization capability.A key application of our fusion learning algorithm,described in this work,is semantics reinforcement using an ensemble of classifiers built using the same training dataset but groundtruth corresponding to different concepts.We expect that classifiers built for semantically close concepts should reinforce each other’s performance and fusion learning is an excellent post-classification way to reinforce semantics and performance.Fusion learning experiments have been performed on TRECVID2005test set.Experiments using the well established retrieval effectiveness measure of mean average precision reveal that our proposed algorithm improves over the best classifier (oracle)by 3.8%.We also present and discuss some interesting and intuitive semantic reinforcement trends observed during fusion learning.Categories and Subject DescriptorsH.3.3[Information Search and Retrieval]:[retrieval models;search process;selection process]General TermsAlgorithms,Design,Experimentation,Performance Keywordsmultimedia streams,mean average precision,late fusion, bagging,semantics reinforcementCIVR’07,July9–11,2007,Amsterdam,The Netherlands.1.INTRODUCTIONThe last decade has witnessed an explosion in the production of digital data from all over the world.In comparison,the rate of meaningful information extraction from digital data has been rather slow.One of the key reasons is that digital data usually comes in multiple modalities or media forms (i.e.text,images,video,audio),thus making it difficult for generic information extraction methods to be used across multiple media.Moreover,extraction of semantics from multimedia largely remains an open problem.This results from an inherent semantic gap between high level human understanding of the world and the low level visual features used by most techniques to model semantics.One of the main challenges faced in multimedia analysis and retrieval is integration,combination or fusion of multiple modalities.It has been argued and reported that multimodal fusion almost always enhances retrieval performance[15].Moreover, machine learning for multimedia usually involves building classifiers for numerous different concepts with possible semantic coherence.Another challenge is to leverage multiple learners to boost or enhance performance for individual target concepts for retrieval purposes.If the combination is performed post-classification,it usually boils down to fusion of multiple classifier streams.Modeling inter-concept correlation is useful for large-scale semantic image and video analysis[28].In our work,we treat the challenge of semantics reinforcement using a fusion learning framework.Semantic patterns are discovered and reinforcements applied post classification.We also study the effect of negative semantic reinforcement.2.RELATED WORKMultimedia information retrieval has been one of the most happening research areas in the last few years.There are extensive surveys on modeling and retrieval approaches proposed in the last two decades[22,19,20].Here, we discuss some work on classifier combination and some important applications of multimedia fusion and boosting which have motivated our current research.2.1Multimedia FusionTwo of the key components of most multimedia retrieval systems are the feature extractor and feature modeler. Both feature extraction and modeling can be practically performed in a number of distinct ways to possibly tapdifferent aspects of the data.As a consequence,in most multimedia analysis scenarios,one is faced with the possibility of using multiple algorithms,or systems and somehow combining their outputs(whether they are ranked lists or confidences).There is ample evidence that fusion or combination of multiple decision streams performs better than even the best individual algorithm[8,15].Such late stage combination of decisions is often termed late fusion or decision fusion and generally gives robust improvements in several domains including the video classification and retrieval[17].Fusing such outputs with little or no knowledge of the individual classifiers or search engines is a very productive approach for leveraging an ensemble of such systems or algorithms.In the past,many fundamental multimedia(video,text,and audio)based applications have employed different fusion schemes with success.In[27]multimodal fusion from multiple media sources is treated as a two step problem. Thefirst step involvesfinding statistically independent modalities followed by super-kernel fusion to determine optimal combination.The fusion step involves retraining of SVM classifiers multiple times which could be a very expensive process.Fusion from two modalities,audio and video have been shown to be beneficial for authentication of people[4].In the cited work,a Bayesian approach to determine biases of experts has been used to guide the fusion of audio and video streams.Fusion of audio and video modalities has been found to be useful for detection of documentary scene changes[26].In[29],fusion of text and video has been proposed for story segmentation in news video.Fusion can also be performed at the level of feature descriptors or models built from them.In[10],color and edge descriptors have been adaptively fused for detection of people.When the individual systems whose output decisions are being fused have nearly comparable performances,naive strategies such as simply averaging their decisions perform adequately and beat several supposedly smarter fusion approaches[8,15].Here we assume that given a set of entities that need to be classified or ranked,all individual systems and/or algorithms are able to process these entities and provide to the late fusion algorithm a ranking or confidence where higher values indicate that the document is being found relevant to the query and ranked towards the top and lower values indicate that the document is not considered relevant and is being pushed to the bottom of the ranked list.The goal is to then learn a strategy which leverages all available decision inputs optimally.Since fusion learning is usually performed using a hill-climb set,the learned fusion strategy could easily overfit the data used. Hence,avoiding overfitting poses a major challenge. 2.2Classifier CombinationCombination of classifiers is a well studied problem and has outreach in several research areas including multimedia analysis.A classic treatise on combination of classifiers can be found in[12].The cited work presents a statistical framework which encompasses many existing methods of compound classifier combinations.A theoretical study of certain basic classifier combination strategies(such as average,minimum,maximum,median,majority vote,and oracle)has been performed in[13].The focus of the cited work is to establish formulas for the classification error at a single point in feature space in a two class scenario. Each classifier outputs a posterior probability of belonging to different classes and classifier responses are assumed to be independent and identically distributed.The authors of the cited work themselves agree that the independence assumption is not very realistic.Employing neighborhood information for determining relative authority of classifiers for combination has been studied in[25,6].In[12],Kitler et. al.have described two fundamentally different scenarios of combining classifiers.(1)Classifiers which have been built using the same data representation or measurement vectors but different data modeling strategies.(2)Classifiers which have been built using different data representations and possibly different data modeling strategies.We argue and study a third scenario of combination when classifiers have been built using different or similar data representations, modeling strategies,but different ground truth.Fusion in this context corresponds to reinforcement of semantically similar learners.Consider two visual classifiers being built for the concepts outdoor and sky.We expect such systems to benefit from combination or fusion.Similarly,classifiers built from semantically opposing concepts(e.g.indoor and sky)are expected to negatively reinforce each other.In[2], Caruana et.al.proposed an ensemble learning algorithm, NACHOS,which performs a greedy forward selection on a hillclimb set and learns weights across multiple classifiers. Applicability of the algorithm to certain problems was studied and was found to perform better than many other fusion learning algorithms.2.3Boosting for MultimediaBoosting is a principled feature selection procedure used for supervised classification.Boosting methods which produce complex composite hypothesis using multiple weak classifiers are very popular in literature.[21].One of the most popular and successful boosting algorithms is known as Adaboost[5]which uses an adaptive approach to reduce misclassification of data points by adjusting weights assigned to them during learning.Boosting has been successfully used for several applications in multimedia analysis and retrieval.Selection of features for learning and retrieval is not straightforward especially for multimedia applications.Boosting opens up the possibility for automatic selection of features based on performance from a potentially very large number of available features. One of the early works uses boosting for feature selection from a pool of45000highly selective features,for the purpose of image retrieval[23].In[30],a boosting based method has been used for automatically detecting orientation of images with the purpose of classifying them as outdoors versus indoors.The application of AdaBoost algorithm to video retrieval has been found to be useful[18]. Another interesting work uses boosting for enhancing retrieval of images with cluttered scenes[1].The cited work uses multi-dimensional histogram based image features to represent images and boosting for feature selection with a goal to characterize different object parts and their mutual spatial relationships.In[7],AdaBoost algorithm has been employed for fast face recognition.An analysis of boosting applications for image and video retrieval is presented in[9].Performance has been found to be sensitive to the way boosting is performed and several guidelines for appropriate boosting have been presented.Need for a new Late Fusion AlgorithmIn a previous and related work,we studied post-classification multimodal fusion for the TRECVID2005search task. The ensemble learning algorithm for late fusion NACHOS proposed in[2]seemed a suitable choice for fusion to begin with.We applied NACHOS to the TRECVID2005 search task[24]with the aim of fusing three independently designed search sub-systems,one based on text retrieval, another on visual similarity based retrieval and a third based on detecting relevant semantic concepts in the query videos and the target data set[11].Our experiments revealed that NACHOS[2]performed worse than naive fusion that combines these three sub-systems with equal weights.Fusion with equal weights is in general shown to perform reasonably well when all individual decision streams exhibit performance in the same ball park[15].Overfitting to the hillclimb set seemed to be the main problem.In our quest,we designed a novel algorithm for ensemble fusion that was still in the same spirit as the NACHOS algorithm but was robust and had the ability to generalize better. In[11],we evaluate fusion experiments using the mean average precision measure.The proposed fusion technique improves over the baseline(fusion with equal weights)by 36%and over NACHOS by46%on the TRECVID2005 query retrieval task.In this paper,we describe the application of fusion learning to reinforce semantics post classification.The target dataset is the TRECVID2005test set.The dataset is split into hillclimb and test sets of approximately equal sizes. Classifiers constructed using the SVM light algorithm and four different types of visual features[15]are used for classification.For each concept,the baseline is fusion with equal weights of the four different forms of classification outputs.The goal is to employ these individual classification streams of all the39LSCOM-LITE concepts[14]to infer best fusion rules taking one target concept at a time.Fusion learning is thus equivalent to discovering semantic patterns and applying semantic reinforcements post classification.In addition to positive reinforcement, we describe an experiment which involves both positive and negative reinforcements of semantics.We also discuss interesting patterns observed as an application of fusion task.Figure1shows the top15retrieval results for two LSCOM-LITE concepts using the IBM MAR VEL system1. Notice the visual similarity between retrieval results for the two concepts shown and the potential for fusing responses from the two classifiers.At a later discussion,in the paper,we point out the reinforcement patterns,we discover between them.The rest of the paper is organized as follows.In Section3, we motivate the proposed approach and give a stepwise description and formalism of our fusion learning algorithm. We also discuss the computational advantage over brute force search.Experiments are describe in Section4.In Section5,we conclude and list future directions.Figure1:Screenshots from IBM MAR VEL Multimedia Analysis and Retrieval System for two LSCOM-LITE concepts,top:people-marching,and bottom:walking-running.Notice the visual similarity and the potential for fusion.Figure 2:A graphical representation of the component processes in the fusion learning algorithm.streams we shall use the notationP =˘w D 1,w D 2,...,w D N¯(1)where w D i refers to the weight of stream D i in the pool.If the pool does not contain a certain stream D j then w D j =0.The notation P +D i will denote a temporary addition of stream D i into the pool for the purpose of evaluation.The error of decision stream,with respect to E will be denoted by E (D i )and the error of the entire pool,or the ensemble error,will be denoted by E (P ).1.The pool is initialized with the top K decision streams each assigned a pre-determined weight.If the decision streams ordered by their performance with respect to the error function E are denoted as D t 1,D t 2,...,D t N such thatE (D t i )<E (D t j )if i <j.(2)we initialize the pool P with D t 1,D t 2,...,D t K .At this step,we also allow that the best decision stream be triggered with a higher weight.This could be useful in the presence of some a-priori confidence in the best decision stream.If all streams are believed to be equally authoritative for the problem,it is a good strategy to begin with equal weights.The parameter K can be varied to control performance and avoid overfitting.2.For the inclusion step,we consider the decision stream which decreases the error of the pool by the maximum amount.We consider the stream D i ∗such thatD i ∗=arg max D i˘E (P )−E (P +D i )¯.(3)However,we require that in order to be formally added to the decision pool,the candidate decision streamD i ∗must decrease the error of the pool by a certain percentage (usually set between 4%and 7%).This is a step introduced to avoid overfitting.3.If addition of no decision stream helps decrease the error of the pool,the algorithm allows for two strategies to perturb the solution and allow for more hillclimbing.•We identify the decision stream having the highest weight in the pool.We perform the following operation:w D i ∗←w D i ∗+δ,(4)s.t.D i ∗=arg max D i{w D i }.(5)This scheme is believed to be beneficial when one decision stream is apriori believed to be more authoritative than the rest.•A random decision stream can be included to add diversity to the pool.This scheme is found to be a good way to get out of local optimas.4.Steps 2and 3are repeated for a fixed number of iterations or till convergence.Additionally,we maintain history about the best set of decision stream weights obtained during the hillclimb.The algorithm rolls back to these weights in the end.In order to increase generalization of decision weights to different and potentially larger sets of data,we allow for the following kind bagging based resampling to be used with fusion learning.Bagging :Only a proportion of data points in the hillclimb set are used to perform decision fusion learning.This is repeated for a fixed number of iterations and the final weights are obtained as the average of weights in each run.Figure3:Pool error with iterations for four different bagged data samples.Note the roll-back points for each trend.Figure3represents the decay in error of the pool during fusion learning for an LSCOM-LITE primary concept Walking Running.Plots for four different bagged-runs have been presented for the purpose of illustration.Notice the roll-back points identified by the algorithm for each run. The details of the experiment will be presented in Section4. The complexity of the fusion learning algorithm is determined by the error computation step which is performed for each decision stream at each iteration. The error calculation involves performing a sort on the dataset.Suppose the size of the hillclimb set is denoted as V and the number of decision streams is N,the computational complexity of each iteration of the algorithm is O(NV log V).If the number of iterations is T,the complexity becomes O(T NV log V).An alternative to the proposed approach could be to perform an exhaustive search for fusion weights.Assuming that there are N decision streams,as earlier,and one allows each stream to take integer weights between0and M−1,this approach would involve searching M N weight combinations for a hillclimb set of size V.The complexity for this would come out to be O(M N V log V).Moreover,this approach is not scalable when the number of decision streams N is very large. Hence the proposed approach is a polynomial time greedy search leading to a locally optimal solution as opposed to exponential time global grid search.4.EXPERIMENTSApplication of fusion to semantic-content reinforcement is motivated by a practical problem in the TRECVID retrieval task.TRECVID[24]is an annual semantic video retrieval benchmark run by the National Institute of Standards and Technology(NIST)in which a common corpus and a common set of concepts are made available to participants every summer.Participants then analyze the corpus and use their own techniques for ranking video shots in the test corpus based on their relevance to the concepts.Such ranking systems leverage modelsor Figure4:A graphical representation of three interesting semantic reinforcement patterns we obtained using decision fusion learning.The thickness of the arrows correspond to the amount of reinforcement.classifiers constructed for the concepts in question.Visual similarity based retrieval with respect to a concept then becomes equivalent to ranking video shots by the respective confidences provided by the corresponding classifier.We have pioneered[16,17]the approach of detecting a large number of semantic concepts such as objects,locations and events in videos and then using those to retrieve video shots that are relevant to the query text and the concepts in the accompanying query images and videos(For details see[15]). Our experiments were performed with the39LSCOM-LITE concepts of the TRECVID2005retrieval task.For more details please see[15].The dataset of80hours of broadcast news video comprising45765shots was split into a hillclimb set of21175shots and test set of24590shots.Care was taken to ensure that all shots from a particular video end up in only one of those two sets without being split across the training and testing set.The fusion algorithm was trained on the hillclimb set and tested on the test set.For each concept,we fused four different visual based classification systems with equal weights to form the baseline.We will refer to these as the parent streams for the corresponding concepts.For details of the visual features used please refer to[15].Training for each target concept involved learning from all the39parent streams.To measure retrieval effectiveness,we used the standard NIST measure of non-interpolated average precision for each query and the mean average precision for an overall evaluation of the system.Let R be the number of true relevant documents in a set of size S;L the ranked list of documents returned.At any given index j let R j be the number of relevant documents in the top j documents.Let I j=1if the j th document is relevant and0otherwise.Assuming R<S,the non-interpolated average precision(AP)is then defined as1j∗I j We performed two sets of experiments as shown in Table1. In thefirst experiment,fusion learning and combination was performed using a39dimensional model vector(one for each of the39LSCOM-LITE primary concepts parentConcept F usion−bag1Walking Running0.34830.11270.1120Maps0.50680.21060.2200Building0.50400.46100.4727Mountain0.44830.00150.0014Sports0.38550.33710.33840.32440.3366Table1:Semantics reinforcement using classifier fusion.Performance is shown for10concepts from TRECVID2005test set for which NIST provided ground truth.The baseline is the best classifier for each concept.F usion−bag1denotes fusion using a39dimensional model vector.F usion−bag2denotes fusion using a78dimensional model vector.(a negative decision stream for each concept corresponding to the negation of the response of its parent stream).streams).In a second experiment,for each of the39 concepts,we included a negative stream corresponding to the negation of the response of the parent classifier stream.Our fusion learning algorithm does not allow removal of decision streams from the pool once they are inside.Formation of a78dimensional model vector for fusion training and testing gave our algorithm theflexibility to decrease weight of a certain stream(by giving weight to its corresponding negative stream).Additionally,we expect that some concepts can benefit from reinforcement by negative decision streams alone(e.g.outdoor can be reinforced by not indoor).An apriori confidence in the model built for each concept resulted in using thefirst scheme in step3of the algorithm(increasing weight of highest-weight classifier to get out of local optimas).The percentage used as inclusion criteria in step2was set as 7%.Bagging was performed across15runs.In Figure4,we show some of the interesting reinforcement patterns which we observed.The target concepts are shown in green.We have tried to make the thickness of the arrows correspond to the respective reinforcements.•The concept car with a gain in AP of0.4%over baseline has been reinforced by truck and road.The corresponding weights obtained hence fractional reinforcement is0.914,0.046,and0.040by concepts car,road,and truck.•The concept mountain with a gain in AP of 4.6% over baseline has been reinforced by snow and waterscape-waterfront.The corresponding fractional reinforcement is0.75,0.125,and0.125by concepts mountain,snow,and waterscape-waterfront.•The concept walking-running with a gain in AP of13.1%over baseline has been reinforced by people-marching and sports.The corresponding fractional reinforcement is0.696,0.152,and0.152by concepts walking-running,sports,and people-marching.As expected,the parent stream for each concept exercises the maximum reinforcement on itself.Besides the patterns shown,we also observed1)building was reinforced by road and urban,2)waterscape-waterfront was reinforced by boat-ship and mountain,3)sports was reinforced by crowd inv,snow,vegetation and walking-running.The overall gain in performance for10concepts evaluated using the mean-average-precision measure at a depth of1000was 3.1%and3.8%when using reinforcement using39and78 dimensional model vectors respectively(Table1).In certain cases,reinforcement could be caused by a high degree of co-occurrence of concepts rather than visual or semantic similarity from a human viewpoint e.g.car being reinforced by road.Besides,patterns such as snow reinforcing sports could be due to a significant number of snow sports scenes. Neverthless,we have shown that fusion learning is capable of reinforcing semantics post classification.Fusion learning,in our case,is an offline process and does not involve classifier retraining.Moreover,application of fusion at real time is an extremely inexpensive process.5.CONCLUSIONS AND FUTURE WORK In this paper,we described application of a greedy performance driven algorithm for fusion learning and semantic reinforcement using multiple classifiers.We assume that each individual classification system provides a ranking or confidence in answering a query orfinding a concept and many of such systems need to be consulted and fused to generate thefinal ranking.The algorithm is inspired from the Ensemble Learning idea proposed by Caruana et.al.[2]but has added features in order to make this learning procedure robust with improved generalization capability. In addition to a greedy forward selection procedure,the algorithm also incorporates techniques for decision pool perturbation and utilization of history during a hillclimb search.Fusion learning was performed using the2005 TRECVID Test Set and performance was demonstrated on the10LSCOM-LITE concepts for which NIST has provided ground truth.The baseline consisted of equal-weight fused response of single best classifiers for each concept built using four different forms of low-level features[15].We described interesting and intuitive reinforcement patterns observed asa result of fusion learning and showed an improvement of3.8%over baseline.In the future,it would be interesting to experiment with stochastic stream inclusion criteria,as suggested by one of our reviewers.Another important futurework is integration of the fusion learner with IBM MAR VEL Multimedia Analysis and Retrieval System.Application of fusion learning to future TRECVID search and retrieval tasks is also anticipated.The fusion learning algorithm is generic and can be used for other multimedia tasks as well.6.REFERENCES[1]J.Amores,N.Sebe,P.Radeva,T.Gevers,and A.Smeulders,“Boosting Contextual Information inContent-based Image Retrieval,”Proc.Int.Workshop on Multimedia Information Retrieval,2004.[2]R.Caruana,A.Niculescu-Mizil,G.Crew,and A.Ksikes,“Ensemble Selection from Library of Models,”Proc.Int.Conference on Machine Learning,2004. [3]K.Deb,A.Anand,and D.Joshi,“AComputationally Efficient Evolutionary Algorithmfor Real-Parameter Optimization,”EvolutionaryComputation Journal(MIT Press),vol.10,no.4,pp.371-395,2002.[4]B.Duc,E.S.Bigun,J.Bigun,G.Maitre,and S.Fischer,“Fusion of Audio and Video Information forMultimodal Person Authentication,”PatternRecognition Letters,vol.18,no.9,pp.835–843,1997.[5]Y.Freund,and R.E.Schapire,“Experiments with aNew Boosting Algorithm,”Proc.Int.Conference onMachine Learning,1996.[6]G.Gianto,F.Roli,“Dynamic Classifier Selectionbased on Multiple Classifier Behavior,”PatternRecognition,vol.34,no.9,pp.1879-1881,2001 [7]G.D.Guo,H.J.Zhang,“Boosting for Fast FaceRecognition,”Proc.Int.Workshop for Recognition,Analysis,and Tracking of Faces and Gestures inReal-Time Systems,2001.[8]A.G.Hauptmann,and M.G.Christel,“SuccessfulApproaches in the TREC Video RetrievalEvaluations,”Proc.ACM Int.Conference onMultimedia,2004.[9]N.Howe,“A Closer Look at Boosted ImageRetrieval,”Proc.Int.Conference on Image andVideo Retrieval,2003.[10]S.Jabri,Z.Duric,H.Wechsler,and A.Rosenfeld,“Detection and Location of People in Video Imagesusing Adaptive Fusion of Color and EdgeInformation,”Proc.Int.Conference on PatternRecognition,2000.[11]D.Joshi,M.Naphade,and A.Natsev,“A GreedyPerformance Driven Algorithm for Decision FusionLearning,”submitted to IEEE Int.Conference onImage Processing,2007.[12]J.Kitler,M.Hatef,R.P.W.Duin,and J.Matas,“On Combining Classifiers,”IEEE Trans.PatternAnalysis and Machine Intelligence,vol.20,no.3,pp.226–239,1998.[13]L.I.Kuncheva,“A Theoretical Study on SixClassifier Fusion Strategies,”IEEE Trans.PatternAnalysis and Machine Intelligence,vol.40,no.2,pp.281–286,2002.[14]M.Naphade,L.Kennedy,J.R.Kender,S.-F.Chang,P.Over,and A.Hauptmann,“A Light ScaleConcept Ontology for Multimedia Understanding forTRECVID2005(LSCOM-Lite),”IBM ResearchTechnical Report,2005.[15]A.Amir,J.Argillander,A.Haubold,F.Kang,M.Naphade,A.Natsev,J.Smith,and J.Tesic,“IBMResearch TRECVID-2005Video Retrieval System,”Gaithersburg,2005.[16]M.R.Naphade,I.Kozintsev,and T.S.Huang,“AFactor Graph Framework for Semantic VideoIndexing”,IEEE Trans.on Circuits and Systems forVideo Technology,vol.12,no.1,pp.40–52,2002. [17]M.Naphade,J.Smith,and F.Souvannavong,“Onthe Semantic Detection of Concepts at TRECVID,”Proc.ACM Multimedia,2004.[18]M.J.Pickering,S.M.Ruger,and D.Sinclair,“VideoRetrieval by Feature Learning in Key Frames,”Proc.Int.Conference on Image and Video Retrieval,2002.[19]Y.Rui,T.S.Huang,S.F.Chang,“Image Retrieval:Current Techniques,Promising Directions and Open Issues,”Journal of Visual Communication and Image Representation,vol.10,pp.39–62,1999.[20]N.Sebe,M.S.Lew,X.Zhou,T.S.Huang,E.Bakker,“The State of the Art in Image and VideoRetrieval,”Proc.ACM Int.Conference onMultimedia,2003.[21]R.E.Schapire,“The Boosting Approach to MachineLearning:An Overview,”MSRI Workshop onNonlinear Estimation and Classification,2002. [22]A.W.M.Smeulders,M.Worring,S.Santini,A.Gupta,R.Jain,“Content-based image retrieval atthe end of the early years,”IEEE Transaction onPattern Analysis and Machine Intelligence,vol.22,no.12,pp.1349–1380,2000.[23]K.Tieu,and P.Viola,“Boosting Image Retrieval,”Int.Journal of Computer Vision,vol.56,no.1-2,pp.17–36.[24]TREC Video Retrieval,“National Institute ofStandards and Technology,”/projects/t01v,2005.[25]K.Woods,W.P.Kegelmeyer,and K.Bower,“Combination of Multiple Classifiers Using LocalAccuracy Estimates,”IEEE Trans.Pattern Analysis and Machine Intelligence,vol.19,no.4,pp.405–410, 1997.[26]A.Velivelli,C.-W.Ngo,and T.S.Huang,“Detection of Documentary Scene Changes byAudio-Visual Fusion,”Proc.Int.Conference onImage and Video Retrieval,2004.[27]Y.Wu,E.Y.Chang,K.C.C.Chang,and J.R.Smith,“Optimal Multimodal Fusion for MultimediaData Analysis,”Proc.ACM Int.Conference onMultimedia,2004.[28]R.Yan,M.-Y.Chen,and A.G.Hauptmann,“Mining Relationship between Video Concepts using Probabilistic Graphical Model”,Proc.Int.Conference on Multimedia and Expo,2006.[29]Y.Zhai,A.Yilmaz,and M.Shah,“StorySegmentation in News Videos using Visual andTextual Cues,”Proc.ACM Int.Conference onMultimedia,2005.[30]L.Zhang,M.Li,and H.-J.Zhang,“Boosting ImageOrientation Detection with Indoor versus OutdoorClassification,”Proc.Int.Workshop on Applications of Computer Vision,2002.。