A Multiarray Tomographic Approach for Through-Wall Imaging
array tomography技术在生命科学研究中的应用与发展
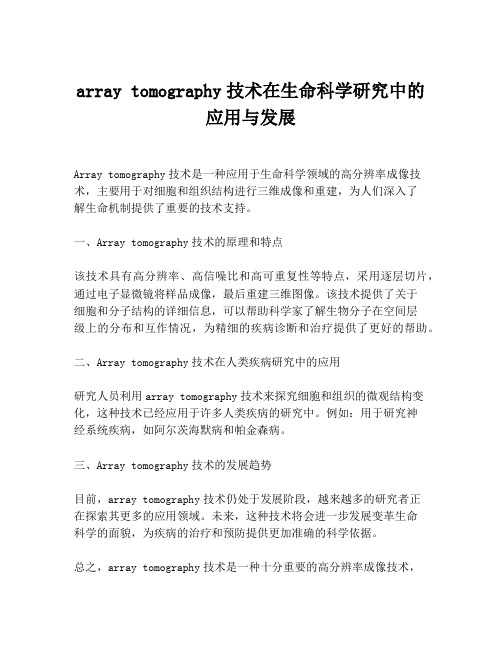
array tomography技术在生命科学研究中的
应用与发展
Array tomography技术是一种应用于生命科学领域的高分辨率成像技术,主要用于对细胞和组织结构进行三维成像和重建,为人们深入了
解生命机制提供了重要的技术支持。
一、Array tomography技术的原理和特点
该技术具有高分辨率、高信噪比和高可重复性等特点,采用逐层切片,通过电子显微镜将样品成像,最后重建三维图像。
该技术提供了关于
细胞和分子结构的详细信息,可以帮助科学家了解生物分子在空间层
级上的分布和互作情况,为精细的疾病诊断和治疗提供了更好的帮助。
二、Array tomography技术在人类疾病研究中的应用
研究人员利用array tomography技术来探究细胞和组织的微观结构变化,这种技术已经应用于许多人类疾病的研究中。
例如:用于研究神
经系统疾病,如阿尔茨海默病和帕金森病。
三、Array tomography技术的发展趋势
目前,array tomography技术仍处于发展阶段,越来越多的研究者正
在探索其更多的应用领域。
未来,这种技术将会进一步发展变革生命
科学的面貌,为疾病的治疗和预防提供更加准确的科学依据。
总之,array tomography技术是一种十分重要的高分辨率成像技术,
它为人们了解生物分子在空间层级上的分布和互作情况提供了更好的帮助。
未来,该技术的发展将会为人类疾病研究和治疗带来更多的帮助,这是该技术发展的一个新的趋势。
不需酶切位点的重叠延伸PCR在克隆表达中的应用
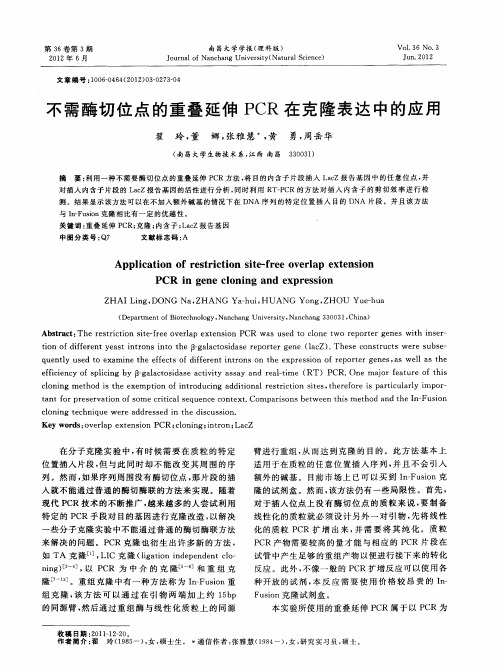
南 昌大 学 学 报 ( 科 版 ) 理
中介 的克 隆( C — dae lnn ) P R me itdc ig 中的一种 , o ] 这 种方 法基本 能够 在 基 因 的任 意 位置 插 入 目标 片 段 , 并且 不需 引入其 他 序 列 。相较 于 I- u in克 隆而 nF s o 言, 本方 法 不 需 要 使 用 昂 贵 的 I— u in重 组 酶 。 nF s o
特定 的 P R手段对 目的基 因进行 克隆 改造 , C 以解 决
一
线性 化 的质粒 就必 须 设计 另 外 一对 引物 , 将 线性 先 化 的质粒 P R 扩 增 出来 , 需 要 将 其 纯 化 。质 粒 C 并
P R产 物需要 较高 的量 才 能 与相 应 的 P R片 段在 C C
状 况 。为了不影 响 报 告基 因的 表 达 , 能确 保 内含 又
电转 化仪 : i—R dg n usr cl Bo a e ep lexel D NA 胶 回收试剂 盒 、 质粒 小提 试剂 盒 : 天根
Pr e TAR i S m H S DNA o y e a e Ta Ra P l m r s : Ka
文 章 编 号 :0 6 0 6 ( 0 2 0 — 2 30 1 0 — 4 4 2 1 ) 30 7 — 4
不 需 酶切 位 点 的重 叠延伸 P R 在 克 隆表 达 中的应 用 C
翟 玲, 董 娜 , 雅 慧 , 张 黄 勇 , 岳 华 周
( 昌大 学 生 物技 术 系, 西 南 昌 3 0 3 ) 南 江 3 0 1
( pa t e fBi t c no o De rm nto o e h l gy, nc ng U n v r iy, a ha g 30 1, i a) Na ha i e st N nc n 3 03 Ch n
简述同时表达两个基因的方法

简述同时表达两个基因的方法基因是一种遗传信息,它决定着我们有多少特定特性,即它们决定着我们何时表达或不表达这些特性。
因此,一个基因可以被理解为一段具有稳定特性的遗传信息,而通常情况下,表达一种特性的基因通常会失活,而表达另一种特性的基因则会被激活。
因此,如果有一种方法可以同时表达两个基因,那么,这种方法就非常有用,有助于更好地研究和利用遗传信息。
在本文中,我们将讨论如何同时表达两个基因的方法。
首先,我们可以利用细胞共同表达系统(CDS)来同时表达两个基因。
CDS是一种基因连接技术,它可以将两个基因连接在一起,使它们能够共同表达。
为了实现CDS,我们可以使用合成的DNA串联基因细胞中的基因,使它们能够共同表达。
另外,通过CRISPR技术,学者们可以在特定基因的位置切割一段特定DNA,然后与另一段特定的DNA结合,使两个基因能够同时表达。
此外,还可以在基因上添加一个特殊的标记,使其易于被认出,以便进行同时表达。
此外,还可以采用转录抑制和表达抑制的方式来同时表达两个基因。
在转录抑制方面,我们可以采用RNA干扰技术,这是一种利用小RNA分子来抑制特定基因的表达,从而实现同时表达两个基因的目的。
在表达抑制方面,我们可以利用特定的调控蛋白来阻断特定基因的表达,从而达到同时表达的目的。
最后,基因敲除技术也可以用来实现同时表达两个基因的目的。
基因敲除技术是通过改变特定基因的DNA来抑制或消除其表达,从而实现同时表达两个基因的目的。
此外,三级RNA干扰也可以作为同时表达两个基因的方法。
它是通过裂解特定的RNA,来抑制特定基因的表达,从而达到同时表达的目的。
综上所述,当前已有多种方法可以实现同时表达两个基因的目的,它们分别是细胞共同表达系统(CDS)、CRISPR技术,转录抑制和表达抑制,以及基因敲除技术和三级RNA干扰。
大部分方法利用了一些特殊的技术,从而实现同时表达两个基因的目的,但有些方法更为安全,也更为简单。
KEGG数据库在生物合成研究中的应用

#技术与方法#生物技术通报BI OTEC HNOLOG Y BULLETI N2011年第1期KEGG 数据库在生物合成研究中的应用韩增叶 田平芳(北京化工大学生命科学与技术学院,北京100029)摘 要: KEGG (K yoto Encyc l oped i a o f G enes and G eno m es)提供了一个操作平台,即以基因组信息(GEN ES)和化学物质信息(L I GAND )为构建模块,通过代谢网络(PATHW AY )将基因组和生物系统联系起来,然后根据功能等级进行归纳分类(BR ITE)。
KEGG 还为各种组学研究提供相关软件,用于代谢途径重建、遗传分析和化合物比对。
作为一个综合数据库,KEGG 不仅指导生物燃料、药物和新材料等生物基化学品的合成,而且致力于研究日趋严重的环境问题。
系统介绍了K EGG 数据库的结构、功能及其相关工具的最新进展,并展望在生物合成中的应用前景。
关键词: KEGG 基因 化合物 代谢途径 生物合成Applicati ons of KEGG Dat abase in Researc h of Biosynt hesisH an Zengye T i an P ingfang(Colle ge of L i fe Science and T ec hnology,B eiji ng Uni versit y of Che m ical T ec hnology,Beijing 100029)Abstrac:t KEGG set a stage for bio l og ists t o accom plish reprogra mm ing cell behav i o r or even crea ti ng a novel o rganis m.K EGGbasica ll y consi sts o f f our daughter da tabases :GENES ,L I GAND,PATHWAY,and BR ITE .GENES is a co llection of gene ca talogs for all comp l e te g eno m es and so m e partia l genomes .L I GAND cove rs both endogenous and ex og enous che m i ca l substances .PATHWAY pro -v i des m o l ecular i nteracti on net w orks such as path w ay s and m o l ecular comp l exes .BR I TE is an onto l ogy database representi ng functiona l h i erarch ies of va ri ous b i o l og ical objects .In additi on ,KEGG prov ides ana l y si s t oo ls for om ics research ,i nclud i ng m etabo lic path w ay re -constructi on ,gene ti c ana lysis ,and comparison o f chem ical co m pounds .A s a comprehensi ve da tabase ,K EGG not only gu i des t he bi o syn -t hesis o f bio -based chem icals ,such as bio -f ue l s ,drugs or nove l bio m ate rials ,but a l so ill um ina tes our perception o f pressi ng env iron m en -ta l issues .In t h is paper ,t he KEGG co m position ,functi on ,and re l evant soft w are w ere rev ie w ed and its potential u tilizations in biosynthe -si s w ere a l so tentati v ely env isi oned .K ey words : KEGG G eneCo m pound M etabo lic pa t hway B i osynthes i s 收稿日期:2010-05-25基金项目:国家自然科学基金项目(20876009)作者简介:韩增叶,男,硕士研究生,研究方向:分子生物学;E-m ai:l hanzengye @163.co m 通讯作者:田平芳,男,博士,副教授,研究方向:合成生物学;E-m ai:l ti anp @f m ai.l buct .edu .cn如何使细胞和有机体在计算机上完整地表达和演绎,是后基因组时代的重大挑战。
Scheduling flow shops using differential evolution algorithm
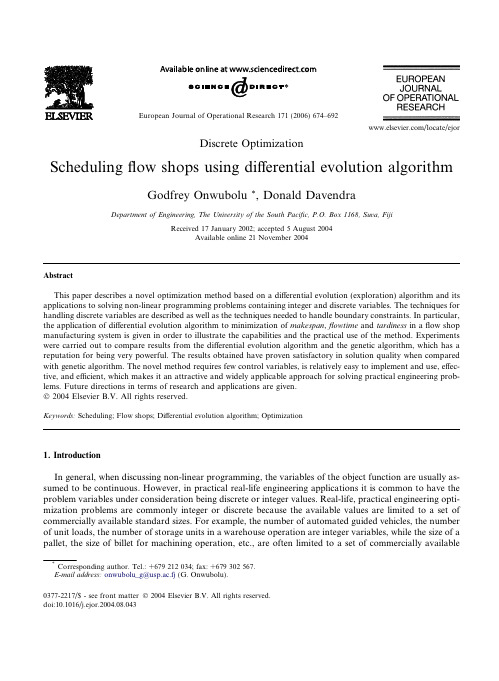
Discrete OptimizationScheduling flow shops using differential evolution algorithmGodfrey Onwubolu *,Donald DavendraDepartment of Engineering,The University of the South Pacific,P.O.Box 1168,Suva,FijiReceived 17January 2002;accepted 5August 2004Available online 21November 2004AbstractThis paper describes a novel optimization method based on a differential evolution (exploration)algorithm and its applications to solving non-linear programming problems containing integer and discrete variables.The techniques for handling discrete variables are described as well as the techniques needed to handle boundary constraints.In particular,the application of differential evolution algorithm to minimization of makespan ,flowtime and tardiness in a flow shop manufacturing system is given in order to illustrate the capabilities and the practical use of the method.Experiments were carried out to compare results from the differential evolution algorithm and the genetic algorithm,which has a reputation for being very powerful.The results obtained have proven satisfactory in solution quality when compared with genetic algorithm.The novel method requires few control variables,is relatively easy to implement and use,effec-tive,and efficient,which makes it an attractive and widely applicable approach for solving practical engineering prob-lems.Future directions in terms of research and applications are given.Ó2004Elsevier B.V.All rights reserved.Keywords:Scheduling;Flow shops;Differential evolution algorithm;Optimization1.IntroductionIn general,when discussing non-linear programming,the variables of the object function are usually as-sumed to be continuous.However,in practical real-life engineering applications it is common to have the problem variables under consideration being discrete or integer values.Real-life,practical engineering opti-mization problems are commonly integer or discrete because the available values are limited to a set of commercially available standard sizes.For example,the number of automated guided vehicles,the number of unit loads,the number of storage units in a warehouse operation are integer variables,while the size of a pallet,the size of billet for machining operation,etc.,are often limited to a set of commercially available 0377-2217/$-see front matter Ó2004Elsevier B.V.All rights reserved.doi:10.1016/j.ejor.2004.08.043*Corresponding author.Tel.:+679212034;fax:+679302567.E-mail address:onwubolu_g@usp.ac.fj (G.Onwubolu).European Journal of Operational Research 171(2006)674–692/locate/ejorG.Onwubolu,D.Davendra/European Journal of Operational Research171(2006)674–692675 standard sizes.Another class of interesting optimization problem isfinding the best order or sequence in which jobs have to be machined.None of these engineering problems has a continuous objective function; rather each of these engineering problems has either an integer objective function or discrete objective func-tion.In this paper we deal with the scheduling of jobs in aflow shop manufacturing system.Theflow shop scheduling-problem is a production planning-problem in which n jobs have to be pro-cessed in the same sequence on m machines.The assumptions are that there are no machine breakdowns and that all jobs are pre-emptive.This is commonly the case in many manufacturing systems where jobs are transferred from machine to machine by some kind of automated material handling systems.For large problem instances,typical of practical manufacturing settings,most researchers have focused on developing heuristic procedures that yield near optimal-solutions within a reasonable computation time. Most of these heuristic procedures focus on the development of permutation schedules and use makespan as a performance measure.Some of the well-known scheduling heuristics,which have been reported in the literature,include Palmer(1965),Campbell et al.(1970),Gupta(1971),Dannenbring(1977),Hundal and Rajagopal(1988)and Ho and Chang(1991).Cheng and Gupta(1989)and Baker and Scudder(1990)pre-sented a comprehensive survey of research work done inflow shop scheduling.In recent years,a growing body of literature suggests the use of heuristic search procedures for combi-natorial optimization problems.Several search procedures that have been identified as having great poten-tial to address practical optimization problems include simulated annealing(Kirkpatrick et al.,1983), genetic algorithms(Goldberg,1989),tabu search(Glover,1989,1990),and ant colony optimization(Dor-igo,1992).Consequently,over the past few years,several researchers have demonstrated the applicability of these methods,to combinatorial optimization problems such as theflow shop scheduling(see for example, Widmer and Hertz,1989;Ogbu and Smith,1990;Taillard,1990;Chen et al.,1995;Onwubolu,2000).More recently,a novel optimization method based on differential evolution(exploration)algorithm(Storn and Price,1995)has been developed,which originally focused on solving non-linear programming problems containing continuous variables.Since Storn and Price(1995)invented the differential evolution(explora-tion)algorithm,the challenge has been to employ the algorithm to different areas of problems other than those areas that the inventors originally focussed on.Although application of DE to combinatorial optimi-zation problems encountered in engineering is scarce,researchers have used DE to design complex digital filters(Storn,1999),and to design mechanical elements such as gear train,pressure vessels and springs (Lampinen and Zelinka,1999).This paper presents a new approach based on differential evolution algorithm for solving the problem of scheduling n jobs on m machines when all jobs are available for processing and the objective is to minimize the makespan.Other objective functions considered in the present work include meanflowtime and total tardiness.2.Problem formulationAflow shop scheduling is one in which all jobs must visit machines or work centers in the same sequence. Processing of a job must be completed on current machine before processing of the job is started on suc-ceeding machine.This means that initially all jobs are available and that each machine is restricted to pro-cessing only one job at any particular time.Since thefirst machine in the facility arrangement is thefirst to be visited by each job,the other machines are idle and other jobs are queued.Although queuing of jobs is prohibited in just-in-time(JIT)manufacturing environments,flow shop manufacturing continues tofind applications in electronics manufacturing,and space shuttle processing,and has attracted much research work(Onwubolu,2002).Theflow shop can be formatted generally by the sequencing of n jobs on m ma-chines under the precedence condition,with typical objective functions being the minimizing of average flowtime,minimizing the time required to complete all jobs or makespan,minimizing maximum tardiness,and minimizing the number of tardy jobs.If the number of jobs is relatively small,then the problem can be solved without using any generic optimizing algorithm.Every possibility can be checked to obtain results and then sequentially compared to capture the optimum value.But,more often,the number of jobs to be processed is large,which leads to big-O order of n !Consequently,some kind of algorithm is essential in this type of problem to avoid combinatorial explosion.The standard three-field notation (Lawler et al.,1995)used is that for representing a scheduling problem as a j b j F (C ),where a describes the machine environment,b describes the deviations from standard sched-uling assumptions,and F (C )describes the objective C being optimized.In the work reported in this paper,we are solving the n /m /F k F (C max )problem.Other problems solved include F ðC Þ¼F ðP C i Þand F ðC Þ¼F ðP T j Þ.Here a =n /m /F describes the multiple-machines flow shop problem,b =null,and F ðC Þ¼F ðC max ;P C i ;and P T j Þfor makespan,mean flowtime,and total tardiness,respectively.Stating these problem descriptions more elaborately,the minimization of completion time (makespan)for a flow shop schedule is equivalent to minimizing the objective function I :I ¼X n j ¼1C m ;j ;ð1Þs :t :C i ;j ¼max C i À1;j ;C i ;j À1ÀÁþP i ;j ;ð2Þwhere C m ,j =the completion time of job j ,C 1,1=k (any given value),C i ;j ¼P j k ¼1C 1;k ;C j ;i ¼P i k ¼1C k ;1,i )machine number,j )job in sequence,P i ,j )processing time of job j on machine i .For a given sequence,the mean flowtime,MFT =1P m i ¼1P n j ¼1c ij ,while the condition for tardiness is c m ,j >d j .The constraint of Eq.(2)applies to these two problem descriptions.3.Differential evolutionThe differential evolution (exploration)[DE]algorithm introduced by Storn and Price (1995)is a novel parallel direct search method,which utilizes NP parameter vectors as a population for each generation G .DE can be categorized into a class of floating-point encoded,evolutionary optimization algorithms .Currently,there are several variants of DE.The particular variant used throughout this investigation is the DE/rand/1/bin scheme.This scheme will be discussed here and more detailed descriptions are provided (Storn and Price,1995).Since the DE algorithm was originally designed to work with continuous variables,the opti-mization of continuous problems is discussed first.Handling discrete variables is explained later.Generally,the function to be optimized,I ,is of the form I ðX Þ:R D !R .The optimization target is to minimize the value of this objective function I ðX Þ,min ðI ðX ÞÞ;ð3Þby optimizing the values of its parameters X ={x 1,x 2,...,x D },X 2R D ,where X denotes a vector composed of D objective function ually,the parameters of the objective function are also subject to lower and upper boundary constraints,x (L )and x (U ),respectively,x ðL Þj P x j P x ðU Þj8j 2½1;D :ð4Þ3.1.InitializationAs with all evolutionary optimization algorithms,DE works with a population of solutions,not with a sin-gle solution for the optimization problem.Population P of generation G contains NP solution vectors called individuals of the population and each vector represents potential solution for the optimization problem 676G.Onwubolu,D.Davendra /European Journal of Operational Research 171(2006)674–692P ðG Þ¼X ðG Þi ¼x ðG Þj ;i ;i ¼1;...;NP ;j ¼1;...;D ;G ¼1;...;G max :ð5ÞIn order to establish a starting point for optimum seeking,the population must be initialized.Often there is no more knowledge available about the location of a global optimum than the boundaries of the problem variables.In this case,a natural way to initialize the population P (0)(initial population)is to seed it with random values within the given boundary constraints:P ð0Þ¼x ð0Þj ;i ¼x ðL Þj þrand j ½0;1 Âx ðU Þj Àx ðL Þj 8i 2½1;NP ;8j 2½1;D ;ð6Þwhere rand j [0,1]represents a uniformly distributed random value that ranges from zero to one.3.2.MutationThe self-referential population recombination scheme of DE is different from the other evolutionary algorithms.From the first generation onward,the population of the subsequent generation P (G +1)is obtained on the basis of the current population P (G ).First a temporary or trial population of candidate vectors for the subsequent generation,P 0ðG þ1Þ¼V ðG þ1Þ¼v ðG þ1Þj ;i ,is generated as follows:v ðG þ1Þj ;i ¼x ðG Þj ;r 3þF Âx ðG Þj ;r 1Àx ðG Þj ;r 2 ;if rand j ½0;1 <CR _j ¼k ;x ðG Þi ;j ;otherwise ;8<:ð7Þwhere i 2[1,NP];j 2[1,D ],r 1,r 2,r 32[1,NP],randomly selected,except:r 15r 25r 35i ,k =(int(rand i [0,1]·D )+1),and CR 2[0,1],F 2(0,1].Three randomly chosen indexes,r 1,r 2,and r 3refer to three randomly chosen vectors of population.They are mutually different from each other and also different from the running index i .New random values for r 1,r 2,and r 3are assigned for each value of index i (for each vector).A new value for the random num-ber rand[0,1]is assigned for each value of index j (for each vector parameter).3.3.CrossoverThe index k refers to a randomly chosen vector parameter and it is used to ensure that at least one vector parameter of each individual trial vector V (G +1)differs from its counterpart in the previous generation X (G ).A new random integer value is assigned to k for each value of the index i (prior to construction of each trial vector).F and CR are DE control parameters.Both values remain constant during the search process.Both values as well as the third control parameter,NP (population size),remain constant during the search pro-cess.F is a real-valued factor in range [0.0,1.0]that controls the amplification of differential variations.CR is a real-valued crossover factor in the range [0.0,1.0]that controls the probability that a trial vector will be selected form the randomly chosen,mutated vector,V ðG þ1Þj ;i instead of from the current vector,x ðG Þj ;i .Gener-ally,both F and CR affect the convergence rate and robustness of the search process.Their optimal values are dependent both on objective function characteristics and on the population size,ually,suitable values for F ,CR and NP can be found by experimentation after a few tests using different values.Practical advice on how to select control parameters NP,F and CR can be found in Storn and Price (1995,1997).3.4.SelectionThe selection scheme of DE also differs from the other evolutionary algorithms.On the basis of the cur-rent population P (G )and the temporary population P 0(G +1),the population of the next generation P (G +1)is created as follows:G.Onwubolu,D.Davendra /European Journal of Operational Research 171(2006)674–692677XðGþ1Þi ¼VðGþ1Þi;if I VðGþ1Þi6IðXðGÞiÞ;XðGÞi;otherwise:8<:ð8ÞThus,each individual of the temporary or trial population is compared with its counterpart in the current population.The one with the lower value of cost-function IðXÞto be minimized will propagate the pop-ulation of the next generation.As a result,all the individuals of the next generation are as good or better than their counterparts in the current generation.The interesting point concerning the DE selection scheme is that a trial vector is only compared to one individual vector,not to all the individual vectors in the cur-rent population.3.5.Boundary constraintsIt is important to notice that the recombination operation of DE is able to extend the search outside of the initialized range of the search space(Eqs.(6)and(7)).It is also worthwhile to notice that sometimes this is a beneficial property in problems with no boundary constraints because it is possible tofind the optimum that is located outside of the initialized range.However,in boundary-constrained problems,it is essential to ensure that parameter values lie inside their allowed ranges after recombination.A simple way to guarantee this is to replace parameter values that violate boundary constraints with random values generated within the feasible range:uðGþ1Þj;i ¼xðLÞjþrand j½0;1 ÂðxðUÞjÀxðLÞjÞ;if uðGþ1Þj;i<xðLÞj_uðGþ1Þj;i>xðUÞj;uðGþ1Þi;j;otherwise;(ð9Þwhere i2[1,NP];j2[1,D].This is the method that was used for this work.Another simple but less efficient method is to reproduce the boundary constraint violating values according to Eq.(7)as many times as is necessary to satisfy the boundary constraints.Yet another simple method that allows bounds to be approached asymptotically while minimizing the amount of disruption that results from resetting out of bound values(Price,1999) isuðGþ1Þj;i ¼ðxðGÞj;iþxðLÞjÞ=2;if uðGþ1Þj;i<xðLÞj;ðxðGÞj;iþxðUÞjÞ=2;if uðGþ1Þj;i>xðUÞj;uðGþ1Þj;i;otherwise:8>><>>:ð10Þ3.6.Conventional technique for integer and discrete optimization by DESeveral approaches have been used to deal with discrete variable optimization.Most of them round offthe variable to the nearest available value before evaluating each trial vector.To keep the population robust,successful trial vectors must enter the population with all of the precision with which they were generated(Storn and Price,1997).In its canonical form,the differential evolution algorithm is only capable of handling continuous vari-ables.Extending it for optimization of integer variables,however,is rather mpinen and Zelinka (1999)discuss how to modify DE for mixed variable optimization.They suggest that only a couple of sim-ple modifications are required.First,integer values should be used to evaluate the objective function,even though DE itself may still works internally with continuousfloating-point values.Thus, Iðy iÞ;i2½1;D ;ð11Þ678G.Onwubolu,D.Davendra/European Journal of Operational Research171(2006)674–692wherey i ¼x i for continuous variables;INTðx iÞfor integer variables;&wherey i ¼x i;INTðx iÞ: &x i2X:INT()is a function for converting a real-value to an integer value by truncation.Truncation is performed here only for purposes of cost-function value evaluation.Truncated values are not elsewhere assigned. Thus,DE works with a population of continuous variables regardless of the corresponding object variable type.This is essential for maintaining the diversity of the population and the robustness of the algorithm. Second,in case of integer variable,instead of Eq.(6),the population should be initialized as follows: Pð0Þ¼xð0Þj;i¼xðLÞjþrand j½0;1 ÂðxðUÞjÀxðLÞjþ1Þ8i2½1;NP ;8j2½1;D :ð12ÞAdditionally,instead of Eq.(9),the boundary constraint handling integer variables should be performed as follows:uðGþ1Þj;i ¼xðLÞjþrand j½0;1 ÂðxðUÞjÀxðLÞjþ1Þ;if INTðuðGþ1Þj;iÞ<xðLÞj_INTðuðGþ1Þj;iÞ>xðUÞj;uðGþ1Þi;ji;otherwise;(ð13Þwhere i2[1,NP];j2[1,D].They also discuss how discrete values can also be handled in a straightforward manner.Suppose that the subset of discrete variables,X(d),contains l elements that can be assigned to var-iable x:XðdÞ¼xðdÞi;i2½1;l ;ð14Þwhere xðdÞi<xðdÞiþ1.Instead of the discrete value x i itself,we may assign its index,i,to x.Now the discrete variable can be handled as an integer variable that is boundary constrained to range1,...,l.To evaluate the objective func-tion,the discrete value,x i,is used instead of its index i.In other words,instead of optimizing the value of the discrete variable directly,we optimize the value of its index i.Only during evaluation is the indicated discrete value used.Once the discrete problem has been converted into an integer one,the previously de-scribed methods for handling integer variables can be applied(Eqs.(11)–(13)).3.7.Forward transformation and backward transformation techniqueThe problem formulation is already discussed in Section2.Solving theflow shop-scheduling problem and indeed most combinatorial optimization problems requires discrete variables and ordered sequence, rather than relative position indexing.To achieve this,we developed two strategies known as forward and backward transformation techniques respectively.In this paper,we present a forward transformation method for transforming integer variables into continuous variables for the internal representation of vec-tor values since in its canonical form,the DE algorithm is only capable of handling continuous variables.G.Onwubolu,D.Davendra/European Journal of Operational Research171(2006)674–692679We also present a backward transformation method for transforming a population of continuous variablesobtained after mutation back into integer variables for evaluating the objective function(Onwubolu,2001). Both forward and backward transformations are utilized in implementing the DE algorithm used in the present study for theflow shop-scheduling problem.Fig.1shows how to deal with this inherent represen-tational problem in DE.Level0deals with integer numbers(which are used in discrete problems).At this level,initialization andfinal solutions are catered for.In the problem domain areas of scheduling,TSP,etc., we are not only interested in computing the objective function cost,we are also interested in the proper order of jobs or cities respectively.Level1of Fig.1deals withfloating point numbers,which are suited for DE.At this level,the DE operators(mutation,crossover,and selection)take place.To transform the integer at level0intofloating point numbers at level1for DEÕs operators,requires some specific kind of coding.This type of coding is highly used in mathematics and computing science.For the basics of trans-forming an integer number into its real number equivalence,interested readers may refer to Michalewicz (1994),and Onwubolu and Kumalo(2001)for its application to optimizing machining operations using genetic algorithms.3.7.1.Forward transformation(from integer to real number)In integer variable optimization a set of integer number is normally generated randomly as an initial solution.Let this set of integer number be represented asz0i2z0:ð15ÞLet the real number(floating point)equivalence of this integer number be z i.The length of the real number depends on the required precision,which in our case,we have chosen two places after the decimal point. The domain of the variable z i has length equal to5;the precision requirement implies that the range be [0...4].Although0is considered since it is not a feasible solution,the range[0.1,1,2,3,4]is chosen,which gives a range of5.We assign each feasible solution two decimal places and this gives us5·100=500.Accordingly,the equivalent continuous variable for z0iis given as100¼102<5Â1026103¼1000:ð16ÞThe mapping from an integer number to a real number z i for the given range is now straightforward,given asz i¼À1þz0iÂ510À1:ð17Þ680G.Onwubolu,D.Davendra/European Journal of Operational Research171(2006)674–692Eq.(17)results in most conversion values being negative;this does not create any accuracy problem any way.After some studies by Onwubolu(2001),the scaling factor f=100was found to be adequate for con-verting virtually all integer numbers into their equivalent positive real numbers.Applying this scaling factor of f=100givesz i¼À1þz0iÂfÂ510À1¼À1þz0iÂ50010À1:ð18ÞEq.(18)is used to transform any integer variable into an equivalent continuous variable,which is then used for the DE internal representation of the population of vectors.Without this transformation,it is not pos-sible to make useful moves towards the global optimum in the solution space using the mutation mecha-nism of DE,which works better on continuous variables.For example in afive-job scheduling problem, suppose the sequence is given as{2,4,3,1,5}.This sequence is not directly used in DE internal representa-tion.Rather,applying Eq.(18),the sequence is transformed into a continuous form.Thefloating-pointequivalence of thefirst entry of the given sequence,z0i ¼2,is z i¼À1þ2Â500103À1¼0:001001.Other valuesare similarly obtained and the sequence is therefore represented internally in the DE scheme as {0.001001,1.002,0.501502,À0.499499,and1.5025}.3.7.2.Backward transformation(from real number to integer)Integer variables are used to evaluate the objective function.The DE self-referential population muta-tion scheme is quite unique.After the mutation of each vector,the trial vector is evaluated for its objective function in order to decide whether or not to retain it.This means that the objective function values of the current vectors in the population need to be also evaluated.These vector variables are continuous(from the forward transformation scheme)and have to be transformed into their integer number equivalence. The backward transformation technique is used for convertingfloating point numbers to their integer num-ber equivalence.The scheme is given as follows:z0 i ¼ð1þz iÞÂð103À1Þ500:ð19ÞIn this present form the backward transformation function is not able to properly discriminate between variables.To ensure that each number is discrete and unique,some modifications are required as follows: a¼intðz0iþ0:5Þ;ð20Þb¼aÀz0i;ð21ÞzÃi ¼ðaÀ1Þ;if b>0:5;a;if b<0:5:&ð22ÞEq.(22)gives zÃi ,which is the transformed value used for computing the objective function.It should bementioned that the conversion scheme of Eq.(19),which transforms real numbers after DE operations into integer numbers is not sufficient to avoid duplication;hence,the steps highlighted in Eqs.(20)–(22)are important.In our studies,these modifications ensure that after mutation,crossover and selection opera-tions,the convertedfloating numbers into their integer equivalence in the set of jobs for a new scheduling solution,or set of cities for a new TSP solution,etc.,are not duplicated.As an example,we consider a set of trial vector,z i={À0.33,0.67,À0.17,1.5,0.84}obtained after mutation.The integer values corresponding to the trial vector values are obtained using Eq.(22)as follows:G.Onwubolu,D.Davendra/European Journal of Operational Research171(2006)674–692681z0 1¼ð1À0:33ÞÂð103À1Þ=500¼1:33866;z02¼ð1þ0:67ÞÂð103À1Þ=500¼3:3367;z0 3¼ð1À0:17ÞÂð103À1Þ=500¼1:65834;z04¼ð1þ1:50ÞÂð103À1Þ=500¼4:9950;z05¼ð1þ0:84ÞÂð103À1Þ=500¼3:6763;a1¼intð1:333866þ0:5Þ¼2;b1¼2À1:33866¼0:66134>0:5;zÃ1¼2À1¼1;a2¼intð3:3367þ0:5Þ¼4;b2¼4À3:3367¼0:6633>0:5;zÃ2¼4À1¼3;a3¼intð1:65834þ0:5Þ¼2;b3¼2À1:65834¼0:34166<0:5;zÃ3¼2;a4¼intð4:995þ0:5Þ¼5;b4¼5À4:995¼0:005<0:5;zÃ4¼5;a5¼intð3:673þ0:5Þ¼4;b5¼4À3:673¼0:3237<0:5;zÃ5¼4:This can be represented schematically as shown in Fig.2.The set of integer values is given aszÃi ¼f1;3;2;5;4g.This set is used to obtain the objective function values.Like in GA,after mutation,crossover,and boundary checking operations,the trial vector obtained fromthe backward transformation is continuously checked until feasible solution is found.Hence,it is not nec-essary to bother about the ordered sequence,which is crucially important in the type of combinatorial opti-mization problems we are concerned with.Feasible solutions constitute about10–15%of the total trial vectors.3.8.DE strategiesPrice and Storn(2001)have suggested ten different working strategies of DE and some guidelines in applying these strategies for any given problem.Different strategies can be adopted in the DE algorithm depending upon the type of problem for which it is applied.Table1shows the ten different working strat-egies proposed by Price and Storn(2001).The general convention used in Table1is as follows:DE/x/y/z.DE stands for differential evolution algorithm,x represents a string denoting the vector to be perturbed,y is the number of difference vectors considered for perturbation of x,and z is the type of crossover being used(exp:exponential;bin:binomial). Thus,the working algorithm outline by Storn and Price(1997)is the seventh strategy of DE,that is,DE/ rand/1/bin.Hence the perturbation can be either in the best vector of the previous generation or in any ran-domly chosen vector.Similarly for perturbation,either single or two vector differences can be used.For perturbation with a single vector difference,out of the three distinct randomly chosen vectors,the weighted vector differential of any two vectors is added to the third one.Similarly for perturbation with two vector682G.Onwubolu,D.Davendra/European Journal of Operational Research171(2006)674–692。
临床检验中的分子诊断技术进展考核试卷
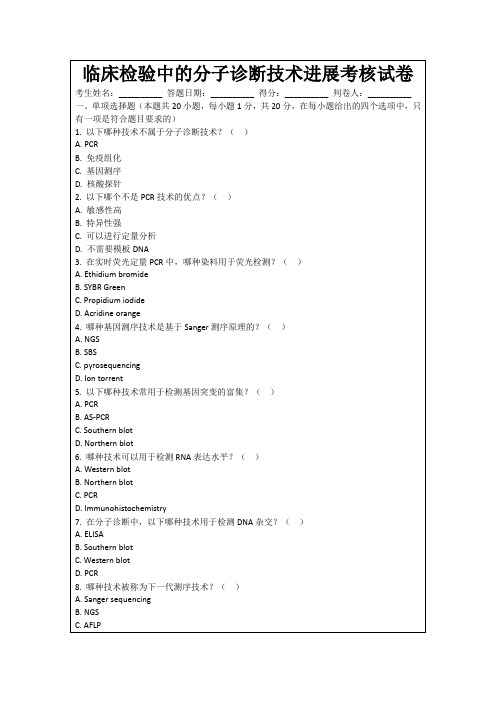
C. Western blot
D. Southern blot
11. 哪种技术可以用于检测单个细胞中的基因表达?( )
A. RNA-Seq
B. Single-cell PCR
C. qPCR
D. Northern blot
12. 以下哪种技术不是基于DNA芯片的检测方法?( )
A. Microarray
B. SNP array
C. CGH array
D. Western blot
13. 在分子诊断中,以下哪种技术用于检测基因重排?( )
A. PCR
B. FISH
C. Southern blot
D. Northern blot
14. 哪种技术可用于检测病原微生物的快速诊断?( )
D. PCR
8. 哪种技术被称为下一代测序技术?( )
A. Sanger sequencing
B. NGS
C. AFLP
D. RFLP
9. 以下哪个不是NGS技术的优点?( )
A. 高通量
B. 成本低
C. 准确性高
D. 速度快
10. 在分子诊断中,以下哪种技术用于检测DNA甲基化?( )
A. PCR
C. Western blot
D. Northern blot
19. 哪种技术可以用于检测miRNA的表达?( )
A. PCR
B. Northern blot
C. RNA-Seq
D. Western blot
20. 在分子诊断中,以下哪种技术用于检测病毒载量?( )
A. PCR
B. ELISA
C. Western blot
多基因遗传病基因研究的策略和方法
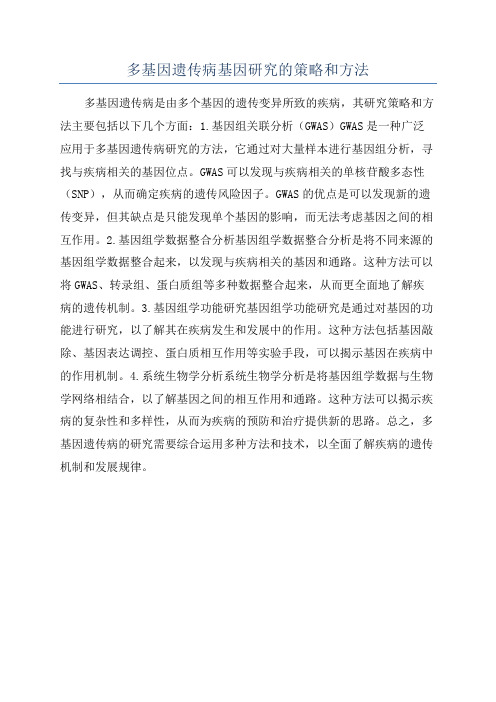
多基因遗传病基因研究的策略和方法多基因遗传病是由多个基因的遗传变异所致的疾病,其研究策略和方法主要包括以下几个方面:1.基因组关联分析(GWAS)GWAS是一种广泛应用于多基因遗传病研究的方法,它通过对大量样本进行基因组分析,寻找与疾病相关的基因位点。
GWAS可以发现与疾病相关的单核苷酸多态性(SNP),从而确定疾病的遗传风险因子。
GWAS的优点是可以发现新的遗传变异,但其缺点是只能发现单个基因的影响,而无法考虑基因之间的相互作用。
2.基因组学数据整合分析基因组学数据整合分析是将不同来源的基因组学数据整合起来,以发现与疾病相关的基因和通路。
这种方法可以将GWAS、转录组、蛋白质组等多种数据整合起来,从而更全面地了解疾病的遗传机制。
3.基因组学功能研究基因组学功能研究是通过对基因的功能进行研究,以了解其在疾病发生和发展中的作用。
这种方法包括基因敲除、基因表达调控、蛋白质相互作用等实验手段,可以揭示基因在疾病中的作用机制。
4.系统生物学分析系统生物学分析是将基因组学数据与生物学网络相结合,以了解基因之间的相互作用和通路。
这种方法可以揭示疾病的复杂性和多样性,从而为疾病的预防和治疗提供新的思路。
总之,多基因遗传病的研究需要综合运用多种方法和技术,以全面了解疾病的遗传机制和发展规律。
Numerical atomic orbitals for linear-scaling calculations
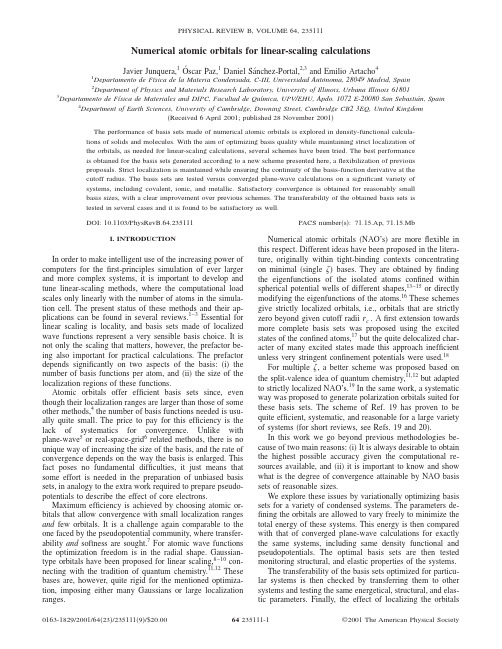
Numerical atomic orbitals for linear-scaling calculationsJavier Junquera,1O´scar Paz,1Daniel Sa´nchez-Portal,2,3and Emilio Artacho41Departamento de Fı´sica de la Materia Condensada,C-III,Universidad Auto´noma,28049Madrid,Spain2Department of Physics and Materials Research Laboratory,University of Illinois,Urbana Illinois618013Departamento de Fı´sica de Materiales and DIPC,Facultad de Quı´mica,UPV/EHU,Apdo.1072E-20080San Sebastia´n,Spain 4Department of Earth Sciences,University of Cambridge,Downing Street,Cambridge CB23EQ,United Kingdom͑Received6April2001;published28November2001͒The performance of basis sets made of numerical atomic orbitals is explored in density-functional calcula-tions of solids and molecules.With the aim of optimizing basis quality while maintaining strict localization ofthe orbitals,as needed for linear-scaling calculations,several schemes have been tried.The best performanceis obtained for the basis sets generated according to a new scheme presented here,aflexibilization of previousproposals.Strict localization is maintained while ensuring the continuity of the basis-function derivative at thecutoff radius.The basis sets are tested versus converged plane-wave calculations on a significant variety ofsystems,including covalent,ionic,and metallic.Satisfactory convergence is obtained for reasonably smallbasis sizes,with a clear improvement over previous schemes.The transferability of the obtained basis sets istested in several cases and it is found to be satisfactory as well.DOI:10.1103/PhysRevB.64.235111PACS number͑s͒:71.15.Ap,71.15.MbI.INTRODUCTIONIn order to make intelligent use of the increasing power of computers for thefirst-principles simulation of ever larger and more complex systems,it is important to develop and tune linear-scaling methods,where the computational load scales only linearly with the number of atoms in the simula-tion cell.The present status of these methods and their ap-plications can be found in several reviews.1–3Essential for linear scaling is locality,and basis sets made of localized wave functions represent a very sensible basis choice.It is not only the scaling that matters,however,the prefactor be-ing also important for practical calculations.The prefactor depends significantly on two aspects of the basis:͑i͒the number of basis functions per atom,and͑ii͒the size of the localization regions of these functions.Atomic orbitals offer efficient basis sets since,even though their localization ranges are larger than those of some other methods,4the number of basis functions needed is usu-ally quite small.The price to pay for this efficiency is the lack of systematics for convergence.Unlike with plane-wave5or real-space-grid6related methods,there is no unique way of increasing the size of the basis,and the rate of convergence depends on the way the basis is enlarged.This fact poses no fundamental difficulties,it just means that some effort is needed in the preparation of unbiased basis sets,in analogy to the extra work required to prepare pseudo-potentials to describe the effect of core electrons.Maximum efficiency is achieved by choosing atomic or-bitals that allow convergence with small localization ranges and few orbitals.It is a challenge again comparable to the one faced by the pseudopotential community,where transfer-ability and softness are sought.7For atomic wave functions the optimization freedom is in the radial shape.Gaussian-type orbitals have been proposed for linear scaling,8–10con-necting with the tradition of quantum chemistry.11,12These bases are,however,quite rigid for the mentioned optimiza-tion,imposing either many Gaussians or large localization ranges.Numerical atomic orbitals͑NAO’s͒are moreflexible in this respect.Different ideas have been proposed in the litera-ture,originally within tight-binding contexts concentrating on minimal͑single)bases.They are obtained byfinding the eigenfunctions of the isolated atoms confined within spherical potential wells of different shapes,13–15or directly modifying the eigenfunctions of the atoms.16These schemes give strictly localized orbitals,i.e.,orbitals that are strictly zero beyond given cutoff radii r c.Afirst extension towards more complete basis sets was proposed using the excited states of the confined atoms,17but the quite delocalized char-acter of many excited states made this approach inefficient unless very stringent confinement potentials were used.18 For multiple,a better scheme was proposed based on the split-valence idea of quantum chemistry,11,12but adapted to strictly localized NAO’s.19In the same work,a systematic way was proposed to generate polarization orbitals suited for these basis sets.The scheme of Ref.19has proven to be quite efficient,systematic,and reasonable for a large variety of systems͑for short reviews,see Refs.19and20͒.In this work we go beyond previous methodologies be-cause of two main reasons:͑i͒It is always desirable to obtain the highest possible accuracy given the computational re-sources available,and͑ii͒it is important to know and show what is the degree of convergence attainable by NAO basis sets of reasonable sizes.We explore these issues by variationally optimizing basis sets for a variety of condensed systems.The parameters de-fining the orbitals are allowed to vary freely to minimize the total energy of these systems.This energy is then compared with that of converged plane-wave calculations for exactly the same systems,including same density functional and pseudopotentials.The optimal basis sets are then tested monitoring structural,and elastic properties of the systems.The transferability of the basis sets optimized for particu-lar systems is then checked by transferring them to other systems and testing the same energetical,structural,and elas-tic parameters.Finally,the effect of localizing the orbitalsPHYSICAL REVIEW B,VOLUME64,235111tighter than what they variationally choose is explored on a demanding system.II.METHODThe calculations presented below were all done using density-functional theory21,22͑DFT͒in its local-density23ap-proximation͑LDA͒.Core electrons were replaced by norm-conserving pseudopotentials7in their fully separable form.24 The nonlocal partial-core exchange-correlation correction25 was included for Cu to improve the description of the core-valence interactions.Periodic boundary conditions were used for all systems. Molecules were treated in a supercell scheme allowing enough empty space between molecules to make intermo-lecular interactions negligible.For solid systems,integra-tions over the Brillouin zone were replaced by converged sums over selected kជsets.26Thus far the approximations are exactly the same for the two different sets of calculations performed in this work: based on NAO’s and on plane-waves͑PW’s͒.The calcula-tions using NAO’s were performed with the SIESTA method, described elsewhere.18,27Besides the basis itself,the only additional approximation with respect to PW’s is the replace-ment of some integrals in real space by sums in afinite three-dimensional͑3D͒real-space grid,controlled by one single parameter,the energy cutoff for the grid.27This cutoff, which refers to thefineness of the grid,was converged for all systems studied here͑200Ry for all except for Si and H2,for which80and100Ry respectively,were used͒.Similarly,the PW calculations were done for converged PW cutoffs.28 Cohesive curves for the solids were obtained byfitting calculated energy values for different unit-cell volumes to cubic,quartic,and Murnaghan-like29curves,a procedure giving values to the lattice parameter,the bulk modulus and the cohesive energy of each system.The bulk moduli given by the Murnaghan and quarticfits deviate from each other by around3%,the Murnaghan values being the lowest and the ones shown in the tables.The deviations between Mur-naghan and cubicfits are of the order of7%.The other cohesive parameters do not change appreciably with thefits.The atomic-energy reference for the cohesive energy was taken from the atomic calculations within the same DFT and pseudopotentials,always converged in the basis set.They are hence the same reference for NAO’s and for PW’s,the dif-ference in cohesive energies between the two accounting for the difference in the total energy of the solid.The isolated-atom calculations included spin polarization.III.BASIS OF NUMERICAL ATOMIC ORBITALSThe starting point of the atomic orbitals that conform the basis sets used here is the solution of Kohn-Sham’s Hamil-tonian for the isolated pseudoatoms,solved in a radial grid, with the same approximations as for the solid or molecule ͑the same exchange-correlation functional and pseudopoten-tial͒.A strict localization of the basis functions is ensured either by imposing a boundary condition,by adding a con-fining͑divergent͒potential,or by multiplying the free-atom orbital by a cutting function.We describe in the following three main features of a basis set of atomic orbitals:size, range,and radial shape.A.Size:Number of orbitals per atomFollowing the nomenclature of quantum chemistry,we es-tablish a hierarchy of basis sets,from singleto multiplewith polarization and diffuse orbitals,covering from quick calculations of low quality to highly converged ones,as con-verged as thefinest calculations in quantum chemistry.A single͑also called minimal͒basis set͑SZ in the following͒has one single radial function per angular momentum chan-nel,and only for those angular momenta with substantial electronic population in the valence of the free atom.Radialflexibilization is obtained by adding a second func-tion per channel:double͑DZ͒.Several schemes have been proposed to generate this second function.In quantum chem-istry,the split valence11,30scheme is widely used:starting from the expansion in Gaussians of one atomic orbital,the most contracted Gaussians are used to define thefirst orbital of the doubleand the most extended ones for the second. Another proposal defines the secondas the derivative of thefirst one with respect to occupation.31For strictly local-ized functions there was afirst proposal17of using the ex-cited states of the confined atoms,but it would work only for tight confinement.An extension of the split valence idea of quantum chemistry to strictly localized NAO’s was proposed in Ref.19and has been used quite successfully in a variety of systems.It consists of suplementing each basis orbital with a new basis function that reproduces exactly the tail of the original orbital from a given matching radius r m out-wards.The inner part goes smoothly towards the origin as r l(aϪbr2),where a and b are chosen to ensure continuity of the function and its derivative at r m.We follow this scheme in this work,which generalizes to multipletrivially by adding more functions generated with the same procedure.Angularflexibility is obtained by adding shells of higher angular momentum.Ways to generate these so-called polar-ization orbitals have been described in the literature,both for Gaussians11,12and for NAO’s.19In this work,however,they will be obtained variationally,as the rest,within theflexibili-ties described below.B.Range:Cutoff radii of orbitalsStrictly localized orbitals͑zero beyond a cutoff radius͒are used in order to obtain sparse Hamiltonian and overlap ma-trices for linear scaling.The traditional alternative to this is based on neglecting interactions when they fall below a tol-erance or when the atoms are beyond some scope of neigh-bors.For long ranges or low tolerances both schemes are essentially equivalent.They differ in their behavior at shorter ranges,where the strict-localization approach has the advan-tage of remaining in the Hilbert space spanned by the basis, remaining variational,and avoiding numerical instabilities no matter how short the range becomes.For the bases made of strictly localized orbitals,the prob-lem isfinding a balanced and systematic way of defining all the different cutoff radii,since both the accuracy and theJUNQUERA,PAZ,SA´NCHEZ-PORTAL,AND ARTACHO PHYSICAL REVIEW B64235111computational efficiency in the calculations depend on them.A scheme was proposed19in which all radii were defined by one single parameter,the energy shift,i.e.,the energy raise suffered by the orbital when confined.In this work,however, we step back from that systematic approach and allow the cutoff radii to vary freely in the optimization procedure͑up to a maximum value of8a.u.͒.C.ShapeWithin the pseudopotential framework it is important to keep the consistency between the pseudopotential and the form of the pseudoatomic orbitals in the core region.This is done by using as basis orbitals the solutions of the same pseudopotential in the free atom.The shape of the orbitals at larger radii depends on the cutoff radius͑see above͒and on the way the localization is enforced.Thefirst proposal13used an infinite square-well potential͑see Fig.1͒.It has been widely and successfully used for minimal bases within the ab initio tight-binding scheme of Sankey and collaborators13us-ing the FIREBALL program,but also for moreflexible bases using the methodology of SIESTA.This scheme has the disadvantage,however,of generating orbitals with a discontinuous derivative at r c as seen in Fig.1.This discontinuity is more pronounced for smaller r c’s and tends to disappear for long enough values of this cutoff.It does remain,however,appreciable for sensible values of r c for those orbitals that would be very wide in the free atom.It is surprising how small an effect such a kink produces in the total energy of condensed systems͑see below͒.It is,never-theless,a problem for forces and stresses,especially if they are calculated using a͑coarse͒finite three-dimensional grid.Another problem of this scheme is related to its defining the basis considering the free atoms.Free atoms can present extremely extended orbitals,their extension being,besides problematic,of no practical use for the calculation in con-densed systems:the electrons far away from the atom can be described by the basis functions of other atoms.Both problems can be addressed simultaneously by add-ing a soft confinement potential to the atomic Hamiltonian used to generate the basis orbitals:it smooths the kink and contracts the orbital as variationally suited.Two soft confine-ment potentials have been proposed in the literature͑Fig.1͒, both of the form V(r)ϭV o r n,one for nϭ2͑Ref.14͒and the other for nϭ6.15They present their own inconveniences,however.First,there is no radius at which the orbitals be-come strictly zero,they have to be neglected at some point. Second,these confinement potentials affect the core region spoiling its adaptation to the pseudopotential.This last problem affects a more traditional scheme as well,namely,the one based on the radial scaling of the or-bitals by suitable scale factors.In addition to very basic bonding arguments,32it is soundly based on restoring virial’s theorem forfinite bases,in the case of Coulombic potentials ͑all-electron calculations͒.33The pseudopotentials limit its applicability,allowing only for extremely small deviationsfrom unity(ϳ1%)in the scale factors obtained variationally ͑with the exception of hydrogen that can contract up to 25%͒.34An alternative scheme to avoid the kink has also beenproposed:16Instead of modifying the potential,it directlymodifies the orbitals of the atom.Following ideas of previ-ous mixed-basis schemes37the atomic orbital is multiplied by1Ϫexp͓Ϫ␣(rϪr c)2͔for rϽr c and zero otherwise.16In Ref.16it is the hard confined wave function which is thenmodified,while in Ref.37it is the free atom wave function.We follow Ref.37.This method is tested in the next section. This scheme does provide strict localization beyond r c,but introduces a different problem:for large␣and small r c a bump appears in the orbital close to r c,which becomes a discontinuity in the wave function in the limit of infinite␣͑Ref.37͒͑this is not the case in Ref.16͒.FIG.1.Shape of the3s orbital of Mg in MgO for the different confinement schemes͑a͒and corresponding potentials͑b͒.NUMERICAL ATOMIC ORBITALS FOR LINEAR-...PHYSICAL REVIEW B64235111In this work we propose a new soft confinement potential avoiding the mentioned deficiencies.It is shown in Fig.1.It isflat͑zero͒in the core region,starts off at some internal radius r i with all derivatives continuous,and diverges at r c ensuring the strict localization there.It isV͑r͒ϭV o eϪ(r cϪr i)/(rϪr i)r cϪr.͑1͒In the following the different schemes are compared,theirdefining parameters being allowed to change variationally.Finally,the shape of an orbital is also changed by theionic character of the atom.Orbitals in cations tend to shrink, and they swell in anions.Introducing a␦Q in the basis-generating free-atom calculations gives orbitals betteradapted to ionic situations in the condensed systems.IV.OPTIMIZATION PROCEDUREGiven a system and a basis size,the range and shape ofthe orbitals are defined by a set of parameters as describedabove.The parameters are described in the following.Per atomic species there is a global␦Q,an extra charge͑positive or negative͒added to the atom at the time of solving theatomic DFT problem for obtaining the basis orbitals͑see below͒.Confinement is imposed separately for each angular mo-mentum shell,with its corresponding parameters that depend on the scheme used.Hard confinement implies one param-eter per shell(r c),and our soft confinement implies three (r c,r i,and V o).One parameter(V o)is needed only in the r n-confinement schemes,14,15and two parameters in the scheme of Elsaesser et al.37(r c and the width of the cutting function͒.Finally,for eachbeyond thefirst,there is a matching radius as mentioned above.19The values of these parameters are defined variationally, according to the following procedure:͑i͒Given a set of parameters,the Kohn-Sham Hamil-tonian͑including the pseudopotential͒is solved for the iso-lated atom,in the presence of the confining potential and the extra charge␦Q.͑In the case of the scheme of Elsaesser et al.,37there is no confining potential,but an a posteriori modification of the solution wave functions.͒This is done for all the relevant l shells of all the different atomic species. The multiple zetas are built from the former using the match-ing procedure described above,19according to the r m’s within the set of parameters.This procedure gives a basis set for each set of parameter values.͑ii͒Given the basis set,a full DFT calculation is per-formed of the system for which the basis is to be optimized, normally a condensed system,solid or molecule.The Kohn-Sham total energy of this system becomes then a function of that set of parameters.Note that neither the extra charge nor the confinement potentials are added to the Kohn-Sham Hamiltonian of the system,they were just used to define the basis.The total-energy calculations are performed for given structural parameters of the studied system.We have chosen to work with experimental structures.This choice is,how-ever,of no great importance since the basis sets are supposed to be transferable enough to render any bias negligible.This is certainly the case at the DZP level,not so much for mini-mal bases.See the section on transferability below.͑iii͒The previous two steps are built in as a function into a minimization algorithm.As a robust and simple minimiza-tion method not requiring the evaluation of derivatives,we have chosen the downhill simplex method.38We have not dedicated special efforts to maximizing the efficiency of the minimization procedure since the systems used for basis op-timization typically involve a small number of atoms and the total-energy calculations are quick.The possible improve-ment in the minimization efficiency is therefore of no rel-evance to the present study.We have no argument to discard the existence of several local minima in the energy function.For the systems studied here there may be sets of parameters giving better bases than the ones we obtain.We systematically tested their robustness by restarting new simplex optimizations from the already optimized sets.More systematic searches for absolute minima,however,would require much more expensive tech-niques,which would not be justified at this point.We have thus satisfied ourselves with the ones obtained,that show good and consistent convergence characteristics.The values obtained for the parameters in the optimizations described below can be obtained from the authors.39V.RESULTSparison of different confinement schemesTable I shows the performance for MgO of the different schemes described above for constructing localized atomic orbitals.The basis sets of both magnesium and oxygen were variationally optimized for all the schemes.Mg was chosen because the3s orbital is very extended in the atom and both the kink and the confinement effects due to other orbitals are very pronounced.Results are shown for a SZ͑single s and p TABLE parison of different confinement schemes on the cohesive properties of MgO,for SZ and DZP basis sets.The gen-eralization of the different schemes to DZP is done as explained in the text.Unconfined refers to using the unconfined pseudoatomic orbitals as basis.a,B,and E c stand for lattice parameter,bulk modulus,and cohesive energy,respectively.The PW calculations were performed with identical approximations as the NAO ones except for the basis.Experimental values were taken from Ref.40.SZ DZPBasis a B E c a B E c scheme͑Å͒͑GPa͒͑eV͒͑Å͒͑GPa͒͑eV͒Unconfined 4.25119 6.49Sankey 4.1722210.89 4.1216511.82 Elsaesser 4.1622811.12 4.1216311.84 Porezag 4.1819611.17 4.0918311.83 Horsfield 4.1522111.26 4.1116811.86 This work 4.1522611.32 4.1016711.87PW 4.1016811.90 Expt. 4.2115210.3JUNQUERA,PAZ,SA´NCHEZ-PORTAL,AND ARTACHO PHYSICAL REVIEW B64235111channels for both species͒and a DZP basis͑double s and p channels plus a single d channel͒.Figure1shows the shape of the optimal3s orbital for the different schemes,and the shape of the confining potentials.The following conclusions can be drawn from the results:͑i͒Within the variational freedom offered here,the3s orbital of Mg wants to be confined to a radius of around6.5bohr, irrespective of scheme,which is extremely short for the free atom.This confinement produces a pronounced kink in the hard scheme.͑ii͒The total energy is relatively insensitive to the scheme used to generate the basis orbitals,as long as there is effective confinement.͑iii͒The basis made of uncon-fined atomic orbitals is substantially worse than any of the others.͑iv͒The pronounced kink obtained in Sankey’s hard confinement scheme is not substantially affecting the total energy as compared with the other schemes.It does perturb, however,by introducing inconvenient noise in the energy variation with volume and other external parameters,and especially in the derivatives of the energy.͑v͒The scheme proposed in this work is variationally slightly better than the other ones,but not significantly.Its main advantage is the avoidance of known problems.In the remainder of the paper, the confinement proposed in this work will be used unless otherwise specified.B.Basis convergenceTable II shows how NAO bases converge for bulk silicon.This is done by comparing different basis sizes,each of them optimized.The results are compared to converged͑50Ry͒PW results͑converged basis limit͒keeping the rest of the calculation identical.Figure2͑a͒shows the cohesion curves for this system.Even though the main point of this work is testing the convergence of NAO basis sets independently of other is-sues,we consider it interesting to gauge the relevance of the errors introduced by the basis by comparing them with other typical errors that appear in these calculations.The NAO and PW results are thus compared to all-electron LDA results49to compare basis errors with the ones produced by the pseudo-potentials.Experiment gives then reference to the error co-mitted by the underlying LDA.The comparisons above are made with respect to the converged-basis limit,for which we used PW’s up to very high cutoffs.It is important to distinguish this limit from the PW calculations at lower cutoffs,as used in many computa-tions.To illustrate this point,Fig.2͑b͒compares the energy convergence for PW’s and for NAO’s.Even though the con-vergence of NAO results is a priori not systematic with the way the basis is enlarged,the sequence of bases presented in thefigure shows a nice convergence of total energy with respect to basis size͑the number of basis functions per atom are shown in parentheses in thefigure͒:the convergence rate is similar to the one of PW’s͑DZP has three times more orbitals than SZ,and a similar factor is found for their equivalents in PW’s͒.For the particular case of Si,Fig.2 shows that the polarization orbitals(3d shell͒are very im-portant for convergence,more than the doubling of the basis. This fact is observed from the stabilization of SZP with re-spect to SZ,which is much larger than for DZ.Figure2shows that an atomic basis at the DZP level requires ten times less functions than its͑energetically͒equivalent PW basis,being Si the easiest system forPW’s.FIG.2.Convergence of NAO basis sets for bulk Si.͑a͒Cohe-sive curve for different basis sets.The lowest curve shows the PW results,filled symbols the NAO bases of this work(opt),and open symbols the NAO bases following Ref.19.Basis labels are like in Table II.͑b͒Comparison of NAO convergence with PW conver-gence.In parentheses is the number of basis functions per atom.TABLE II.Basis comparisons for bulk Si.a,B,and E c stand forlattice parameter͑inÅ͒,bulk modulus͑in GPa͒,and cohesive en-ergy͑in eV͒,respectively.SZ,DZ,and TZ stand for single,double,and triple.P stands for polarized,DP for doubly polar-PW results were taken from Ref.41,and the experimentalvalues from Ref.42.SZ DZ TZ SZP DZP TZP TZDP PW LAPW Expt.a 5.52 5.49 5.48 5.43 5.40 5.39 5.39 5.38 5.41 5.43B85878597979797969698.8E c 4.70 4.83 4.85 5.21 5.31 5.32 5.34 5.37 5.28 4.63NUMERICAL ATOMIC ORBITALS FOR LINEAR-...PHYSICAL REVIEW B64235111For other systems the ratio is much larger,as shown in Table III.It is important to stress that deviations smaller than the ones due to the pseudopotential or the DFT used are obtained with a relatively modest basis size as DZP.This fact is clearin Table II for Si,and in Table IV for other systems.Table IV summarizes the cohesion results for a variety of solids of different chemical kind.They are obtained with optimal DZP basis sets.It can be observed that DZP offers results in good agreement with converged-basis numbers,showing the con-vergence of properties other than the total energy.The devia-tions are similar or smaller than those introduced by LDA or by the pseudopotential.47VI.TRANSFERABILITYTo what extent do optimal bases keep their performance when transferred to different systems than the ones they were optimized for?This is an important question,since if the performance does not suffer significantly,one can hope to tabulate basis sets per species,to be used for whatever sys-tem.If the transferability is not satisfactory,a new basis set should then be obtained variationally for each system to be studied.Of course the transferability increases with basis size,since the basis has more flexibility to adapt to different environments.In this work we limit ourselves to try it on DZP bases for a few representative systems.Satisfactory transferability has been obtained when check-ing in MgO the basis set optimized for Mg bulk and O in a water molecule.Similarly,the basis for O has been tested in H 2O and O 2,and the basis for C in graphite and diamond.TABLE III.Equivalent PW cutoff (E cut )to optimal DZP basesfor different parison of number of basis functions per atom for both bases.For the molecules,a cubic unit cell of 10Åof side was used.System No.funct.DZPNo.funct.PWE cut ͑Ry ͒H 251129634O 2134544286Si1322722Diamond 1328459␣-quartz1392376TABLE IV .Basis comparisons for different solids.a ,B ,and E c stand for lattice parameter ͑in Å͒,bulk modulus ͑in GPa ͒,and co-hesive energy ͑in eV ͒,respectively.ExpLAPW Other PW PW DZP Aua 4.08a 4.05b 4.07c 4.05 4.07B 173a 198b 190c 191188E c 3.81a -- 4.19 4.03MgOa 4.21d 4.26e - 4.10 4.11B 152d 147e -168167E c 10.30d 10.40e -11.9011.87Ca 3.57a3.54f 3.54g 3.53 3.54B 442a 470f 436g 466453E c 7.37a 10.13f 8.96g 8.908.81Sia 5.43a 5.41h 5.38g 5.38 5.40B 99a 96h 94g 9697E c 4.63a 5.28h 5.34g 5.37 5.31Naa 4.23a 4.05i 3.98g 3.95 3.98B 6.9a 9.2i 8.7g 8.89.2E c 1.11a 1.44j 1.28g 1.22 1.22Cua 3.60a 3.52b 3.56g - 3.57B 138a 192b 172g -165Ec 3.50a 4.29k 4.24g - 4.37Pba 4.95a - 4.88- 4.88B 43a -54-64E c2.04a- 3.77- 3.51aC.Kittel,Ref.42.bA.Khein,D.J.Singh,and C.J.Umrigar,Ref.43.cB.D.Yu and M.Scheffler,Ref.44.dF.Finocchi,J.Goniakowski,and C.Noguera,Ref.40.eJ.Goniakowski and C.Noguera,Ref.45.fN.A.W.Holzwarth et al.,Ref.46.gM.Fuchs,M.Bockstedte,E.Pehlke,and M.Scheffler,Ref.47.hC.Filippi,D.J.Singh,and C.J.Umrigar,Ref.41.iJ.P.Perdew et al.,Ref.48.jM.Sigalas et al.,Ref.49.kP.H.T.Philipsen and E.J.Baerends,Ref.50.TABLE V .Transferability of basis sets.‘‘Transf.’’stands for the DZP basis transferred from other systems,while ‘‘Opt.’’refers to the DZP basis optimized for the particular system.For MgO the basis was transferred from bulk Mg and an H 2O molecule,for graphite the basis was transferred from diamond,and for H 2O it was taken from H 2and O 2.a ,B ,and E c stand for lattice parameter,bulk modulus,and cohesive energy,respectively.⌬E stands for the energy difference per atom between graphite and a graphene plane.E b is the binding energy of the molecule.System BasisProperties MgOa ͑Å͒B ͑GPa ͒E c ͑eV ͒Transf. 4.1315711.81Opt. 4.1016711.87PW 4.1016811.90Expt.4.2115210.30Graphitea ͑Å͒c ͑Å͒⌬E ͑meV ͒Transf. 2.456 6.5038PW a 2.457 6.7224Expt.b2.456 6.67423c H 2Od O-H ͑Å͒H-O-H ͑deg ͒E b ͑eV ͒Transf.0.975105.012.73Opt.0.972104.512.94PW 0.967105.113.10LAPW d 0.968103.911.05Expt.e0.958104.510.08a M.C.Schabel and J.L.Martins,Ref.51.bY .Baskin and L.Mayer,Ref.52.cL.A.Girifalco and dd,Ref.53.dP.Serena,A.Baratoff,and J.M.Soler,Ref.54.eG.Herzberg,Ref.55.JUNQUERA,PAZ,SA´NCHEZ-PORTAL,AND ARTACHO PHYSICAL REVIEW B 64235111。
- 1、下载文档前请自行甄别文档内容的完整性,平台不提供额外的编辑、内容补充、找答案等附加服务。
- 2、"仅部分预览"的文档,不可在线预览部分如存在完整性等问题,可反馈申请退款(可完整预览的文档不适用该条件!)。
- 3、如文档侵犯您的权益,请联系客服反馈,我们会尽快为您处理(人工客服工作时间:9:00-18:30)。
A Multiarray Tomographic Approachfor Through-Wall ImagingFrancesco Soldovieri,Raffaele Solimene,and Giancarlo PriscoAbstract—A through-wall imaging problem for a2-D scalar geometry is addressed.It is cast as an inverse scattering prob-lem and tackled under the linear Born model by means of the truncated singular value decomposition inversion scheme.A mul-tiarray-based inversion strategy is considered.In particular,first the data collected by each single array are processed to obtain different tomographic images of the same scene under test.Then, the different images are suitably combined to obtain the overall image.The inversion scheme is tested for the challenging case of objects located within a complex environment resembling a room in a building.Index Terms—Microwave tomography,singular value decom-position,through-wall imaging(TWI).I.I NTRODUCTIONT HROUGH-W ALL imaging(TWI)consists of imaging ob-jects hidden behind an obstacle by using electromagnetic (EM)waves at the microwave frequencies[1],[2].This problem is of great interest,as the aim of detecting and localizing hidden objects is shared in many applicative contexts(i.e.,both military and civilian)such as in search and rescue,security, peacekeeping,and law enforcement operations.In this framework,the research and technological develop-ment activities concern innovative observation instrumentations and processing techniques complying the necessities related to surveillance and imaging in complex environments.The overall aim is to achieve reliable and high-resolution“images”of the investigated scene in almost real time.To this end,the modeling of the EM scattering in complex environments is needed.In particular,the crucial point to be addressed is the presence of the obstacle representing in some cases a wall structure.Indeed,the presence of a wall can hinder a successful imaging procedure due to the absorption and refraction losses that the illuminating radiation undergoes when passing through the wall.This restricts the exploitable frequency range from a few hundreds of megahertz to2–3GHz for which most of the building materials are relatively transparent[3],[4]. Furthermore,if the presence of the wall is not accounted for,blurred images,where the objects appear distorted and delocalized with respect to their actual positions,arise fromManuscript received May4,2007;revised September12,2007.F.Soldovieri is with the Institute for Electromagnetic Sensing of the Environ-ment(IREA),National Research Council(CNR),80124Naples,Italy(e-mail: soldovieri.f@r.it).R.Solimene and G.Prisco are with the Dipartimento di Ingegneria dell’Informazione,Seconda Universitàdi Napoli,81031Aversa,Italy.Color versions of one or more of thefigures in this paper are available online at .Digital Object Identifier10.1109/TGRS.2008.915754the refraction phenomenon.To avoid this circumstance,the propagation path through the wall has to be properly accounted for while processing the scatteredfield data to obtain the image. This has been done,for example,in[5]where a beamforming imaging procedure accommodating the refraction introduced by a single wall structure has been presented.The effect of the wall is implicitly accounted for,and hence, blurring is avoided,if the imaging is cast as an inverse scattering problem.Indeed,in this case,the problem amounts to solving a pair of coupled integral equations where the presence of the wall is embodied within the so-called background Green’s func-tion[6].However,it is known that inverse scattering problems are nonlinear.This leads to iterative inversion schemes[7], which are time consuming and generally require a great deal of computational resources.Moreover,these inversion strategies might prove unreliable due to the occurrence of the false solutions corresponding to the local minima of the nonconvex cost functional that they attempt to minimize[8].The problem is drastically simplified if the scattering equa-tions are linearized,for example,by means of the Born or the Kirchhoff approximations[6].It is known that for realistic cases,linear inversion schemes do not permit to obtain quanti-tative reconstructions of the scattering object features.Instead, they generally allow one to determine the scatterers’locations and their geometrical features[9].However,linear inversion schemes do not suffer from the local minima problem and are computationally effective.This allows one to deal with large (in terms of wavelength)investigation domain in a reasonable amount of time.Furthermore,well-assessed numerical and theoretical tools to achieve stability against noise can be ex-ploited[10].Therefore,if the main aim is to detect and localize scatterers by inspecting a large area with some constraints on the time required to achieve the image(as in TWI applications), it is worth investigating the performance achievable by a linear inversion scheme[11].The computational advantages of the linear model-based inversion schemes are also shared by the so-called qualitative method[12]–[14].Such methods directly aim at only retrieving the scatterer supports and do not require simplified models of the scattering phenomenon.In particular,they identify the spatial support of the scatterers by adopting certain indicator functions that assume very different values in norm depending whether they are evaluated within or outside the scatterer supports.To this class of inversion algorithms belongs the linear sampling[12]and the factorization[13]methods.They require a multistatic/multiview/single-frequency configuration, i.e.,a configuration different with respect to the one of concern herein(see subsequent sections for details about the adopted0196-2892/$25.00©2008IEEEFig.1.Geometry of the problem.configuration),and their performances can be reduced when an aspect-limited configuration(such as the one considered here)is adopted[15].The time-reversal multiple signal clas-sification(MUSIC)imaging algorithm[14]also works in the multifrequency case but still requires a multistatic/multiview configuration.In this paper,we are concerned with a TWI problem where the unknown scatterers are located within an environment resembling a building’s room(see Fig.1)for a2-D and scalar case.In particular,we consider a multiarray configu-ration where data processing is performed by a microwave tomographic approach divided in two steps.First,the scattered field collected by each single array is processed to obtain a microwave imaging of the scene under test by exploiting a Born linear inverse scattering algorithm as described in[11]. Then,the various reconstructions are“combined”to achieve the overall image.As to thefirst step of the procedure,i.e.,the single array TWI reconstruction,we outline that the problem is cast as an inverse scattering one where the“background”scenario is identified by a three-layered1-D medium(the second layer being representative of the wall)and the object to be detected resides within the third layer.This allows one to simplify the formulation curtailing the computational burden and the time required to achieve the images.In particular,the illuminating source is located in thefirst layer and the scattered field is collected only at the same position of the source while the latter moves.In addition,the inversion scheme exploits multifrequency data.Hence,a multimonostatic/multifrequency configuration is addressed.This paper is organized as follows.In Section II,the inverse problem is formulated under the Born approximation and the inversion algorithm based on the singular value decomposition is briefly recalled.Furthermore,the procedure to combine single array images is described.In Section III,we present the numerical results that show the validation of the multiarray configuration in the challenging cases of objects located in a closed environment resembling a parison between the reconstructions obtained by the two-step-based combining procedure and the ones obtained by simultaneously inverting the data collected over the two arrays is provided as well. Conclusions follow.II.F ORMULATION OF THE P ROBLEM AND THEM ULTIARRAY I NVERSION P ROCEDUREThe actual geometry of the problem is depicted in Fig.1.Tar-gets are hidden within a known structure resembling the walls of a room.The walls have thickness d,dielectric permittivity b,and electric conductivityσb.The medium inside and outside the wall structure is assumed as free space,and 0is the cor-responding dielectric permittivity.The magnetic permeability µ0is everywhere equal to that of the free space.We consider a2-D geometry where both the obstacle and the targets are assumed to be infinitely long and invariant along the y-axis. Furthermore,we assume that the targets are located within an investigation domain D=[−x M,x M]×[z min,z max].The source of the incidentfield E inc(·)is afilamentary current located at the air/obstacle interface(see Fig.1)and is radiating within a frequency bandwidth f∈[f min,f max].The scattered field is collected at the same position as the source while the latter moves along a rectilinear domain.In particular,two differ-ent measurement arrays are synthesized on two different sides of the room,i.e.,Σ1=[−x O,x O]along the x-axis andΣ2= [z1,z2]along an axis parallel to the z-axis.Thus,a multiarray multimonostatic/multifrequency configuration is exploited.In the following formulation,time dependence exp(j2πft) is assumed and understood.The problem of detecting and localizing scatterers located within the room is cast as a linear inverse scattering one,and the Born approximation is used to achieve the linearization of the scattering equations.Under this hypothesis,the problem amounts to inverting the following mathematical relationship linking the scatteredfield E S(·)(the data of the problem)and the unknown object[6]:E S(r O,ω)=k20DG(r O,x,z,ω)E inc(r O,x,z,ω)×χ(x,z)dx dz,r O∈Σ1Σ2(1)whereω=2πf,r O is the source/observation position,G(·) is the background Green’s function,andχ(·)is the so-called contrast function,which is the actual unknown of the problem. In particular,it is defined asχ(x,z)=eq(x,z)− 0(2) witheq= D(x,z) 0−jσD(x,z)ω(3)and accounts for the relative difference between the background EM parameters and D andσD of the scatterers.To solve the inverse problem(1),a two-step-based procedure is adopted.First,we separately process the data collected by each single array to obtain two different images of the sameFig.2.Geometry considered while achieving the reconstruction correspond-ing to arrayΣ1.scene from two different points of view.Then,the two images are combined to achieve the overall image of the investigation domain.Step one means that the inverse problem outlined in(1) is split in two similar problems,one for each array,i.e.,E S(r Om ,ω)=k20DG(r Om,x,z,ω)E inc(r Om,x,z,ω)×χ(x,z)dx dz,r Om∈Σm(4)with m∈{1,2}.Note that the aforementioned choice entails that not all the data has to be processed simultaneously.This allows one to deal with lower computational resources and to also speed up the reconstruction procedure.We further simplify the problem by considering for each single array the background geometry in Fig.2.For such a geometry,a three-layered background medium is considered. In particular,as far as the inversion of(4)with m=1is concerned,we consider the background medium to be three-layered along the z-axis(and invariant along x and y)with the second layer being representative of the wall and the scatterers embedded in the third layer.Thefirst and third layers are free space(see Fig.2).Analogously,the inversion of(4)with m=2is achieved by considering a three-layered background medium along the x-axis(and invariant along y and z).In other words,in the inversion procedure,only the walls over which the antennas move are considered and assumed infi-nitely long along x(for m=1)and along z(for m=2). Under this assumption,the Green’s function relevant to the layered background medium and the corresponding incident field have the plane-wave expansion as reported,for example, in[11].According to the previous arguments,the imaging problem amounts to solving(4)(separately for m=1and m=2)for χ(·)when approximated expressions of the Green’s function and of the incidentfield are considered.We emphasize that the same three-layered background Green’s functions are exploited to compute the so-called unperturbedfield,i.e.,thefield scat-tered by the scenario under test when the scattering objects areTABLE IP ARAMETERS OF THE CONFIGURATIONabsent.As the scatteredfield is the difference between thefield scattered by the obstacle plus the objects and the unperturbed one,this also means that the scatteredfield is approximated and different from that of the actual.The linear inverse problem is solved by resorting to the trun-cated singular value decomposition(TSVD)inversion scheme after the integral operators appearing in(4)have been converted in matrix form by means of the method of moments as done in[11].Thus,regularized solutions of integral(4)can be writ-ten asR1χ=Nn=0E S1,v n1σn1u n1,for m=1(5) R2χ=Nn=0E S2,v n2σn2u n2,for m=2(6)where E Smis the scatteredfield collected over arrayΣm, and R mχ’s are the corresponding regularized reconstructions. The sets{σnm}∞n=0denote the singular values ordered in a nonincreasing sequence,whereas{u nm}∞n=0and{v nm}∞n=0 form orthonormal bases in the unknown and data spaces,and the truncation index N is chosen according to the available signal-to-noise ratio(SNR)[10].At this point,we have two different reconstructions of the same scene.According to the previous discussion,this is the first step of the multiarray inversion procedure.The second step,which leads to the overall image,con-sists of combining these reconstructions.This is achieved by comparing the two reconstructions pixel by pixel within the investigation domain and creating a new image by retaining for“each pixel”the larger value attained by the modulus of the retrieved contrast function between the two reconstructions achieved under the single array configurations.III.R ECONSTRUCTION R ESULTSIn this section,some numerical examples are given to assess the reconstruction capabilities of the inversion algorithm.In particular,the following examples refer to the configuration depicted in Fig.1whose parameters,which are maintained throughout this section,are listed in Table I.Moreover,for all the following reconstruction examples,the TSVD procedure is implemented by retaining the singular functions corresponding to the singular values larger than0.1times that of maximum. The exact“total”scatteredfield,i.e.,thefield scattered by the room’s wall plus the scatters,is computedfirst in theFig. 3.Tomographic reconstruction of a square object using the single arrayΣ1.time domain by means of afinite-difference time-domain-based code[16],and then,it is Fourier transformed to obtain data in the frequency domain within which the inversion scheme works.Afilamentary source,which radiates a Ricker wavelet with central frequency equal to800MHz,is used.Then,the scatteredfield data are obtained as the difference between the total scatteredfield for the actual situation under investigation and the unperturbedfield,i.e.,thefield collected when only the obstacle is present in the scene.As aforementioned,the latter is the one predicted by the approximate scenarios considered in (4),i.e.,by considering as background medium a three-layered medium(see Fig.2for the case of m=1).It is important to outline that,as the synthetic data have been generated independently of the model adopted to develop the inversion algorithm,the so-called inverse crime is avoided.As aforementioned,by exploiting a linear inversion scheme, only a qualitative reconstruction of the geometrical features of the targets can be obtained.Thus,for all the following cases,we consider as relevant quantity the modulus of the reconstructed contrast function normalized to its maximum.To better“interpret”the outcome of the multiarray recon-struction procedure,wefirst look into how the reconstructions obtained by exploiting each single array,i.e.,R1χor R2χ, appear.As an example,in Fig.3,the reconstruction of a lossless homogeneous square dielectric object of side0.4m,dielectric permittivity9 0,and located at the center of the investigation domain is shown when arrayΣ1is exploited(the configuration reported in Fig.2has been considered).As can be seen,the reconstruction allows one to identify the upper and lower sides of the object,the latter being delocalized in depth(along z)due to the difference between the actual electromagnetic velocity in the object(0.1m/ns)and the one assumed by the model (0.3m/ns).In addition,the second side is at a lower level in the reconstruction due to the refraction occurring within the object.This result is consistent with the theoretical expecta-tion concerning the spatialfiltering introduced by the TSVD inversion scheme for a configuration such as the one considered in Fig.3.Indeed,the TSVD inversion scheme acts as a low-pass spatialfilter along the direction where the measurement array is synthesized(hence,in the specific case along x)and as a bandpassfilter along the direction orthogonal to the array line(hence,along z)[11].Analogously,the reconstruction R2χthat can be obtained by exploiting arrayΣ2would allow one to identify the object’s edge,which is orthogonal to the x-direction.Hence,by adopting the multiarray strategy,we mainly expect to be able to reconstruct the two sides of the scatterers,which directly face the two arrays.Now,we turn to consider the case depicted in Fig.1,which is our main concern in this contribution.In particular,in all the following TSVD reconstruction examples,the data are corrupted in the frequency domain by adding to the real and imaginary parts of the scatteredfield two independent zero-mean additive white Gaussian noise processes with a standard deviationη0equal toαtimes each of the root mean square (rms)of the scatteredfield,so that an SNR(evaluated as 10log10[rms2/(2η20)])of20dB is obtained.As previously explained,in this case,the model that the single array reconstructions rely on does not account for all the room’s walls as it considers only the wall over which the array is synthesized.Moreover,thefiniteness of this is also neglected.To mitigate the effect due to thisfiniteness,we consider arrays whose extents are smaller than the side of the square investigation domain and,hence,than the room wall length.Thefirst multiarray TWI reconstruction example is shown in Fig.4and refers to the same square object as considered in Fig.3,which is now enclosed in a room(see Fig.1) whose external perimeter is represented by the square hav-ing sides of3.8m;the parameters of the configuration are listed in Table I.For comparison,besides the reconstruction obtained by the combining procedure[Fig.4(c)]described in the previous section,the two individual reconstructions(i.e., the ones obtained by exploiting separately the arraysΣ1and Σ2)and the reconstruction obtained by inverting(1),i.e.,by simultaneously exploiting the data collected overΣ1andΣ2, are also reported.First,it can be noted that,although the data are noisy and despite of having adopted an approximate Green’s function,the single array reconstructions[Fig.4(a)and(b)] allow one to localize the edges of the scatterer with a quality comparable to the one obtained in Fig.3.Furthermore,as expected,in the combined reconstruction[Fig.4(c)]the directly illuminated sides of the objects are correctly localized with a quality superior to that obtainable by simultaneously inverting the data collected over the two arrays.This can be roughly jus-tified by the following reasons.While the combining procedure picks up,for each pixel,the“best”of the amplitude of the two single array reconstructions,simultaneously inverting the two sets of data approximately corresponds tofirst adding the two reconstructions and then taking the overall amplitude. Therefore,the reconstruction will be emphasized where the reconstructions add“constructively”(at the left top corner of the object for the example at hand)and will be lowered where the summation is not in“phase.”Finally,also in this case,the spots at lower intensity account for the sides of the object not directly“visible”by the two arrays.As a further example,in Fig.5,we report the multiarray to-mographic reconstruction for the case of two dielectric objects with different shapes:an object of square cross section havingobtained by exploitingΣ2.(c)Reconstruction obtained by the combining procedure.(d)Reconstruction obtained by simultaneously exploitingΣ1andΣ2.Fig.5.TSVD tomographic reconstruction of square and circular dielectric objects located within a room.(a)Reconstruction obtained by exploitingΣ1.(b)Reconstruction obtained by exploitingΣ2.(c)Reconstruction obtained by the combining procedure.(d)Reconstruction obtained by simultaneously exploitingΣ1andΣ2.Fig.6.Normalized radargram obtained by collecting the data on the horizon-tal arrayΣ1and corresponding to the case shown in Fig.5.sides equal to0.4m and another one of circular cross sectionof radius0.3m.Both the objects have a dielectric permittivityequal to30 0.As for the previous example,the reconstructionallows one to determine the positions of the two objects andto deduce the information about their dimensions.In addition,in this case,the combined reconstruction[Fig.5(c)]appearsbetter than the one reported in Fig.5(d).In particular,previousdiscussion applies well to the case of the square object forwhich the two single reconstructions overlap once again at theleft top corner.For the circular object,the single reconstructionsdo not overlap and the outcome of the combining procedure isalmost equal to the reconstruction in Fig.5(d).We furthermore note that the square object is reconstructedat a lower level than the circular one.This is because it is fartherfrom the arrays than the circular object and outside the apertureof the vertical arrayΣ2.According to the analysis reportedin[17],this result is to be expected.Indeed,in the paper bySolimene and Pierri,it is shown that,for a configuration such asthe one at hand,the TSVD inversion scheme introduces a spa-tially varyingfiltering,which leads to reconstructing at lowerlevel objects that are more deeply located with respect to themeasurement array.For the sake of comparison,we also reportthe radargram corresponding to the previous case(see Fig.6),i.e.,the noise-free scatteredfield in the time domain,which isobtained by exploiting the horizontal measurement arrayΣ1.Itis evident that the radargram is very difficult to interpret.The next example reported in Fig.7concerns the case ofan object elongated along x resembling an internal wall.Inparticular,it consists of a rectangular dielectric object of2.7×0.1m2in size with dielectric permittivity equal to3 0.Thereconstruction obtained by the combining procedure(the oneobtainable by simultaneously inverting all the sets of data isalmost equal to it)permits to obtain information about thelocation of the longer side of the object due to the contributionof the horizontal arrayΣ1.The spot reconstructed at about −1.35m along the x-axis,instead,results from the contribution due to the vertical arrayΣ2and permits to have informationabout the location of the object’s side parallel and closer toarrayΣ2.As a concluding example,we present the reconstruction(see Fig.8)corresponding to the case in which theobjects Fig.7.Multiarray tomographic reconstruction by exploiting the combiningprocedure of an object resembling an internalwall.Fig.8.Multiarray tomographic reconstruction by exploiting the combining procedure of two dielectric objects located in two different internalrooms. Fig.9.Normalized radargram obtained by collecting the data on the horizon-tal arrayΣ1and corresponding to the case shown in Fig.8.considered in Figs.5and7are both present.This situation is meant as a representative of the objects located in two different internal rooms.The multiarray tomographic reconstruction allows one to retrieve both the objects,and the internal wall can be discerned as well.Furthermore,the aforementioned spatially varying behavior of the reconstruction can be still observed. Finally,it is instructive to present the time-domain scattered field(radargrams)concerning this configuration(no noise are considered in the radargrams).The radargrams are shown in Figs.9and10.From thesefigures,the presence and theFig.10.Normalized radargram obtained by collecting the data on the vertical arrayΣ2and corresponding to the case shown in Fig.8.locations of the two scatterers can be roughly deduced,mainly from the radargram obtained by using the vertical arrayΣ2for which the effect of the internal wall is negligible.However, the reconstruction reported in Fig.8proves to be more detailed and objective.IV.C ONCLUSIONWe have tackled the TWI problem of imaging scattering objects that are hidden by a known obstacle resembling a building’s room.In particular,we have presented and checked against synthetic data a tomographic approach based on the Born approximation for the scattering phenomenon and based on a multiarray configuration.The latter consists of suitably combining the reconstruction obtained by each single array. The reconstruction results we have shown allow one to state that the proposed approach also works well in detecting and localizing the objects’“edges”in the complex scenario we have considered and even under the simplifications adopted to de-velop the inversion procedure.Indeed,the inversion procedure has proven to be able to achieve more objective and detailed reconstructions of the investigated zone as compared to the raw data in the time domain(radargram).Many future developments can be identified.In the inversion scheme to obtain each single tomographic image,we have adopted a Green’s function of an ideal situation represented by a three-layered background medium(nonethe-less,the inversion scheme has worked well in the same sense stated previously).This,on the one hand,is of great importance in view of practical situations where the background is un-known or known with some degree of uncertainty[18].Indeed, the previous results suggest that it is sufficient to estimate only the parameters of the wall[19]over which the antennas move. On the other hand,it is worth investigating if better results can be achieved when a more accurate expression of the Green’s function,accounting for all the walls,is exploited.While showing the reconstructions,we have remarked that objects located in different positions are reconstructed differ-ently(in terms of resolution and level).As aforementioned, this is a consequence of the spatially varyingfiltering that the TSVD inversion procedure introduces for configurations such as the one considered herein.This leads the more“weakly”reconstructed objects to be more sensitive to the effect of noise in the reconstruction and,in general,to be less detectable. To mitigate such an effect,a spatially varying thresholding procedure,as the one employed in[17],can also be devised for the case considered in this paper.Finally,the analysis has been confined to canonical scatter-ers;further efforts are needed to check the inversion scheme against more involved scatterers(in geometry and composition) of concern for human body imaging[20].The analysis of these cases,both from synthetic and experimental situations,is left for future developments.R EFERENCES[1]D.D.Ferris and N.C.Currie,“A survey of current technologies forthrough-wall-surveillance(TWS),”Proc.SPIE,vol.3577,pp.62–72, 1998.[2]F.Soldovieri,R.Solimene,A.Brancaccio,and R.Pierri,“Localization ofthe interfaces of a slab hidden behind a wall,”IEEE Trans.Geosci.Remote Sens.,vol.45,no.8,pp.2471–2482,Aug.2007.[3]L.Frazier,“MDR for law enforcement,”IEEE Potentials,vol.16,no.5,pp.23–26,Dec.1997–Jan.1998.[4]A.R.Hunt,“Image formation through walls using a distributed radarsensor array,”in Proc.IEEE32nd AIPR Workshop,2003,pp.232–237.[5]F.Ahmad,M.G.Amin,and S.A.Kassam,“Synthetic aperture beam-former for imaging through a dielectric wall,”IEEE Trans.Aerosp.Elec-tron.Syst.,vol.41,no.1,pp.271–283,Jan.2005.[6]W.C.Chew,Waves and Fields in Inhomogeneous Media.Piscataway,NJ:IEEE Press,1995.[7]L.P.Song,C.Yu,and Q.H.Liu,“Through-wall imaging(TWI)by radar:2-D tomographic results and analyses,”IEEE Trans.Geosci.Remote Sens.,vol.43,no.12,pp.2793–2798,Dec.2005.[8]T.Isernia,V.Pascazio,and R.Pierri,“On the local minima in a tomo-graphic imaging technique,”IEEE Trans.Geosci.Remote Sens.,vol.39, no.7,pp.1596–1607,Jul.2001.[9]R.Persico,R.Bernini,and F.Soldovieri,“The role of the measurementconfiguration in inverse scattering from buried objects under the Born approximation,”IEEE Trans.Antennas Propag.,vol.53,no.6,pp.1875–1887,Jun.2005.[10]M.Bertero and P.Boccacci,Introduction to Inverse Problems in Imaging.Bristol,U.K.:Inst.Phys.,1998.[11]F.Soldovieri and R.Solimene,“Through-wall imaging via a linear inversescattering algorithm,”IEEE Geosci.Remote Sens.Lett.,vol.4,no.4, pp.513–517,Oct.2007.[12]D.Colton and A.Kirsch,“A simple method for solving inverse scatteringproblems in the resonance region,”Inv.Prob.,vol.12,no.4,pp.383–393, Aug.1996.[13]A.Kirsch,“Factorization of the far-field operator for the inhomogeneousmedium case and an application in inverse scattering theory,”Inv.Prob., vol.15,no.2,pp.413–429,1999.[14]E.A.Marengo,F.K.Grouber,and F.Simonetti,“Time-reversal MUSICimaging of extended targets,”IEEE Trans.Image Process.,vol.16,no.8, pp.1967–1984,Aug.2007.[15]A.Liseno and R.Pierri,“Shape reconstruction by the spectral data ofthe far-field operator:Analysis and performances,”IEEE Trans.Antennas Propag.,vol.52,no.3,pp.899–903,Mar.2004.[16]A.Giannopoulos,GprMax2D/3D,User’s Guide,2002.[Online].Available:[17]R.Solimene and R.Pierri,“Resolution and threshold for imaging thinPEC objects,”in Proc.Mediterranean Microw.Symp.,Genova,Italy, Sep.2006.[18]D.Liu,J.Krolik,and L.Carin,“Electromagnetic target detection inuncertain media:Time-reversal and minimum-variance algorithms,”IEEE Trans.Geosci.Remote Sens.,vol.45,no.4,pp.934–944,Apr.2007. [19]C.Kao,J.Li,Y.Wang,H.Xing,and C.R.Liu,“Measurement oflayer thickness and permittivity using a new multilayer model from GPR data,”IEEE Trans.Geosci.Remote Sens.,vol.45,no.8,pp.2463–2470, Aug.2007.[20]R.Solimene,F.Soldovieri,G.Prisco,and R.Pierri,“Three-dimensionalmicrowave tomography by a2-D slice-based reconstruction algorithm,”IEEE Geosci.Remote Sens.Lett.,vol.4,no.4,pp.556–560,Oct.2007.。