基于SVM的城市快速路行程时间预测研究
城市快速路行程时间的统计分析与预测

城市快速路行程时间的统计分析与预测朱 彦1曹彦荣2杜道生11.武汉大学,测绘遥感信息工程国家重点实验室,武汉4300722.中国科学院,地理科学与资源研究所,北京100101摘 要:实时交通预测分析一直是ITS领域一个重要的研究课题,它的研究进展也直接影响着ITS子系统ATMS(Advanced Traffic Management System)的实施。
文章以北京二环快速路为研究对象,先使用浮动车数据计算出路段在不同时段的行程时间,再采用统计分析方法得出目标路段在相同时段下的行程时间的分布规律;在此基础上,对相同时段不同路段、相同路段不同时段的行程时间分布测度进行对比分析,并将其和道路服务水平进行对照,得出了若干有意义的结论和建议;最后,对行程时间计算结果进行了检验和评价,证明了计算结果的准确性。
关键词:智能交通;行程时间;统计分析;预测中图分类号:U412.37 文献标识码:A 文章编号:1672-4747(2009)01-0093-06Travel Time Statistical Analysisand Prediction for the Urban FreewayZHU Yan1 CAO Yan-rong2 DU Dao-sheng11.State Key Laboratory of Information Engineering in Surveying,Mapping and Remote Sensing,Wuhan University,Wuhan 430072,China2.Institute of Geographic Sciences and Natural Resources Research,Chinese Academy of Sciences,Beijing 100101, ChinaAbstract:Short-time traffic forecasting and analyzing are important issues in intelligent transport systems (ITS). It directly impacts on the implement of the sub-system—advanced traffic management system (ATMS) of ITS. Based on the travel time computed by floating car data and with statistical methods, this paper mainly studied the travel time distribution laws of 收稿日期:2008-03-19.作者简介:朱 彦(1983-),男,汉族,湖南望城人,武汉大学硕士研究生,研究方向:交通与GIS应用。
基于SV模型的行程时间预测
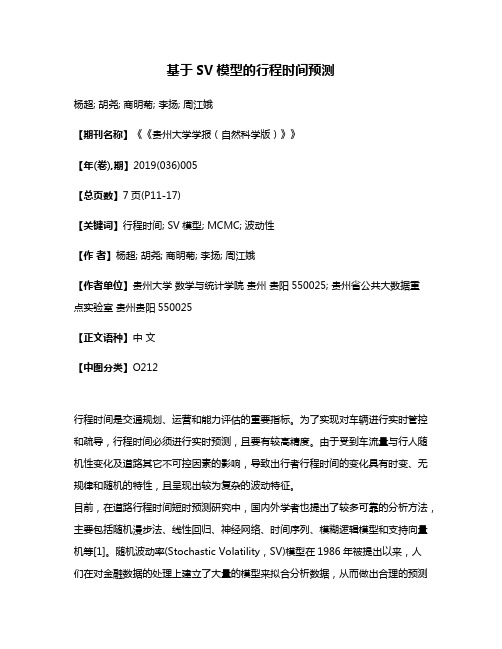
基于SV模型的行程时间预测杨超; 胡尧; 商明菊; 李扬; 周江娥【期刊名称】《《贵州大学学报(自然科学版)》》【年(卷),期】2019(036)005【总页数】7页(P11-17)【关键词】行程时间; SV模型; MCMC; 波动性【作者】杨超; 胡尧; 商明菊; 李扬; 周江娥【作者单位】贵州大学数学与统计学院贵州贵阳550025; 贵州省公共大数据重点实验室贵州贵阳550025【正文语种】中文【中图分类】O212行程时间是交通规划、运营和能力评估的重要指标。
为了实现对车辆进行实时管控和疏导,行程时间必须进行实时预测,且要有较高精度。
由于受到车流量与行人随机性变化及道路其它不可控因素的影响,导致出行者行程时间的变化具有时变、无规律和随机的特性,且呈现出较为复杂的波动特征。
目前,在道路行程时间短时预测研究中,国内外学者也提出了较多可靠的分析方法,主要包括随机漫步法、线性回归、神经网络、时间序列、模糊逻辑模型和支持向量机等[1]。
随机波动率(Stochastic Volatility,SV)模型在1986年被提出以来,人们在对金融数据的处理上建立了大量的模型来拟合分析数据,从而做出合理的预测和估计,其中SV模型就是大量被采用的一种金融模型,它具有数理金融学和金融计量经济学的双重根源[2]。
然而由SV模型含有潜在变量,致使其似然函数极为复杂,求解其估计较困难。
为解决这一问题:2002年,JACQULER等[3]将贝叶斯理论与SV模型相结合,首次使用马尔科夫链蒙特卡洛(Markov Chain Monte Carlo,MCMC)方法估计,发现其估计效果比似然方法更有效;同年,TSE等[4]实证分析了MCMC估计SV模型的效果,得出在SV模型参数估计方法中MCMC要优于伪似然估计等方法;陈杨林等[2]用MCMC方法求解SV模型对黄金价格指数数据的未来趋势与变化进行了研究,且验证了该模型能够较准确地拟合黄金价格的未来变化趋势和较好地进行预测。
《基于最小二乘支持向量机的短时交通流预测方法研究》范文
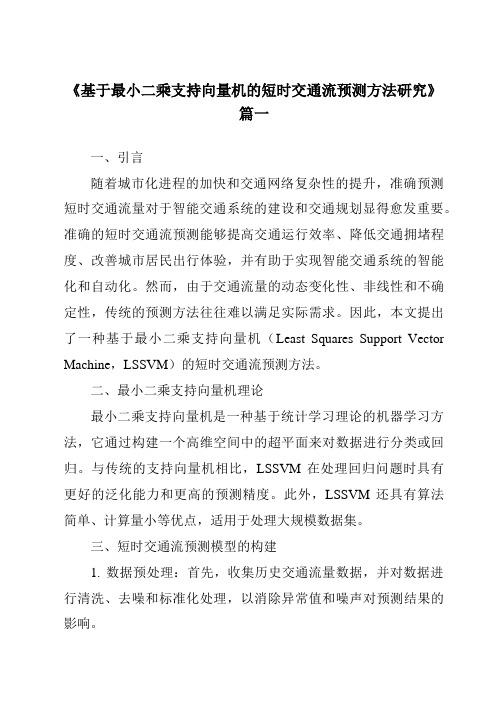
《基于最小二乘支持向量机的短时交通流预测方法研究》篇一一、引言随着城市化进程的加快和交通网络复杂性的提升,准确预测短时交通流量对于智能交通系统的建设和交通规划显得愈发重要。
准确的短时交通流预测能够提高交通运行效率、降低交通拥堵程度、改善城市居民出行体验,并有助于实现智能交通系统的智能化和自动化。
然而,由于交通流量的动态变化性、非线性和不确定性,传统的预测方法往往难以满足实际需求。
因此,本文提出了一种基于最小二乘支持向量机(Least Squares Support Vector Machine,LSSVM)的短时交通流预测方法。
二、最小二乘支持向量机理论最小二乘支持向量机是一种基于统计学习理论的机器学习方法,它通过构建一个高维空间中的超平面来对数据进行分类或回归。
与传统的支持向量机相比,LSSVM在处理回归问题时具有更好的泛化能力和更高的预测精度。
此外,LSSVM还具有算法简单、计算量小等优点,适用于处理大规模数据集。
三、短时交通流预测模型的构建1. 数据预处理:首先,收集历史交通流量数据,并对数据进行清洗、去噪和标准化处理,以消除异常值和噪声对预测结果的影响。
2. 特征提取:从历史交通流量数据中提取出与短时交通流预测相关的特征,如时间、天气、节假日等。
3. 模型构建:利用LSSVM构建短时交通流预测模型。
具体地,将历史交通流量数据作为输入,将预测的目标值(如未来某一时刻的交通流量)作为输出,通过优化算法求解得到模型参数。
4. 模型训练与优化:利用训练数据集对模型进行训练,通过交叉验证等方法对模型进行优化,以提高模型的预测精度。
四、实验与分析1. 数据集与实验环境:本文采用某城市实际交通流量数据作为实验数据集,实验环境为高性能计算机。
2. 实验方法与步骤:将实验数据集分为训练集和测试集,利用训练集对模型进行训练和优化,利用测试集对模型进行测试和评估。
3. 结果与分析:通过对比LSSVM与其他传统预测方法的预测结果,发现LSSVM在短时交通流预测方面具有更高的预测精度和更强的泛化能力。
lssvm在时间序列预测中的理论与应用研究
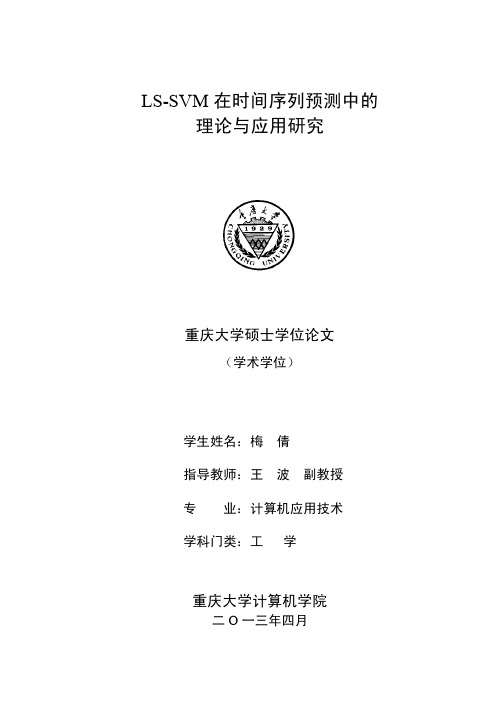
LS-SVM在时间序列预测中的理论与应用研究重庆大学硕士学位论文(学术学位)学生姓名:梅*指导教师:王波副教授专业:计算机应用技术学科门类:工学重庆大学计算机学院二O一三年四月Theory and Application Research on LS-SVM in Time Series PredictionA Thesis Submitted to Chongqing Universityin Partial Fulfillment of the Requirement for theMaster’s Degree of EngineeringByMei QianSupervised by Ass. Prof. Wang Bo Specialty: Computer Application TechnologyCollege of Computer Science ofChongqing University, Chongqing, ChinaApril 2013摘要时间序列预测方法已经应用到几乎所有预报与决策的领域,广泛地应用在实际中。
对这种方法的研究不仅具有理论研究的重要意义,而且一直是国内外学者研究的热点和难点。
在支持向量机模型中,成功地应用了结构风险最小化、核函数映射和凸二次规划等技术,有效地解决了在传统机器学习中出现的维数灾难和局部极小等问题。
而最小二乘支持向量机(LS-SVM)作为支持向量机(SVM)的一个改进简化模型,在保证预测精度不减弱的情况下,具有比支持向量机运算更加简化的优点。
本文针对LS-SVM中的一些问题进行了研究,主要工作如下:①提出了经验模态分解与LS-SVM组合预测的方法,结合建筑能耗预测的实际应用,该方法的主要思路是把能耗数据形成的时间序列用EMD方法分解成多个本征模式分量,然后对每个本征模式分别建立LS-SVM模型进行分开预测,最后将所有本征模式分量对应的LS-SVM模型预测结果进行求和。
基于支持向量机的快速路小时交通量预测
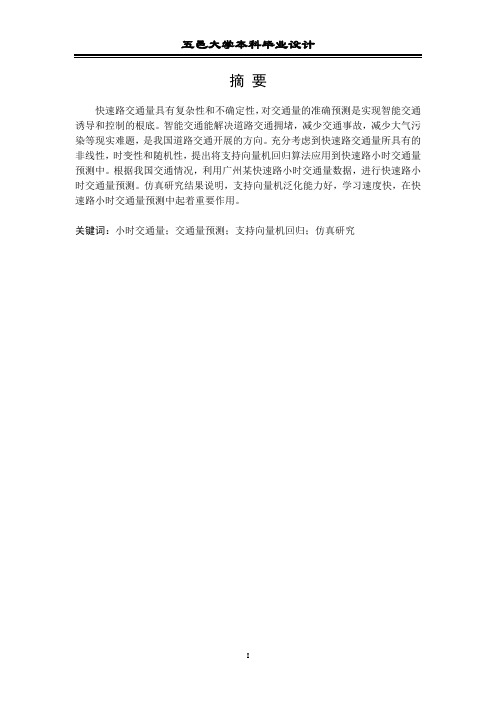
摘要快速路交通量具有复杂性和不确定性,对交通量的准确预测是实现智能交通诱导和控制的根底。
智能交通能解决道路交通拥堵,减少交通事故,减少大气污染等现实难题,是我国道路交通开展的方向。
充分考虑到快速路交通量所具有的非线性,时变性和随机性,提出将支持向量机回归算法应用到快速路小时交通量预测中。
根据我国交通情况,利用广州某快速路小时交通量数据,进行快速路小时交通量预测。
仿真研究结果说明,支持向量机泛化能力好,学习速度快,在快速路小时交通量预测中起着重要作用。
关键词:小时交通量;交通量预测;支持向量机回归;仿真研究AbstractExpressway traffic flow has the complexity and uncertainty, the accurate traffic flow forecasting is the foundation to realize intelligent traffic guidance to control. Intelligent transportation can solve traffic congestion, reduce traffic accidents, reduce the realistic problems such as air pollution, is the direction of our country road traffic development. The expressway traffic flow is nonlinear, time-varying and randomness, the support vector machine (SVM) regression algorithm was applied to expressway hours in traffic flow prediction. According to the traffic situation in our country, use the one of Guangzhou’s expressways traffic flow data to forecast an hour expressway traffic flow. Simulation results show that the generalization and learning ability of support vector machine (SVM) is well, hours in expressway traffic flow forecasting plays an important role.Keywords: hourly traffic volume; traffic flow forecasting; support vector machine regression; simulation research目录摘要 (I)Abstract........................................................... I I 第1章绪论 (1)1.1 课题背景 (1)研究目的和意义 (2)1.3 国内外文献综述 (2)1.4 本论文主要内容 (3)第2章支持向量机理论 (5)2.1 支持向量机概述 (5)2.2 支持向量机回归及其类型 (9)2.3 本章小结 (9)第3章交通流参数及预测方法 (10)3.1 交通流根本参数 (10)3.2 交通流量数据的采集和特性 (10)3.3 交通流预测的方法 (11)3.3.1 交通状态预测概述 (11)3.3.2 交通量短时预测方法 (12)3.4 本章小结 (13)第4章基于支持向量机的小时交通流预测 (14)4.1 概述 (14)4.2 支持向量机回归的交通信息预测 (14)4.3 支持向量机的交通信息预测的具体步骤 (15)4.4 本章小结 (16)第5章仿真研究 (17)5.1 交通流量预测 (17)5.2 本章小结 (30)结论 (31)参考文献 (32)致谢 (33)第1章绪论课题背景城市交通系统是城市经济社会活动的根底设施,城市化的开展和汽车数量的增加导致交通道路通行压力的增加。
城市道路路段行程时间计算及预测模型研究

摘要城市道路交通流基本信息为智能交通系统的运行提供了根本支持,是实施智能交通系统的基础。
车辆在道路上的行程时间作为交通流信息中最直观、最重要的参数之一,它的计算和预测一直是研究的重点内容。
车辆行程时间的精确计算及预测,为出行者选择合理出行方式、路径等选择提供参考,也是道路交通管理者进行交通控制和管理的前提。
本文首先介绍了获取道路交通调查基本数据的各种方法与技术,结合本文所需的数据特点,科学合理的设计制定了道路交通调查方案,并采取科学方法对道路交通调查数据进行筛选处理,进而得到本文所需的道路交通数据,为后续车辆行程时间计算及预测模型的标定和验证计算提供数据基础。
其次,针对城市道路路段行程时间计算和预测问题,本文以城市道路网络中典型的道路路段和信号控制道路交叉口为研究对象,采用道路交通信息视频采集技术获得基本的道路交通信息数据。
通过对道路路段及其下游信号控制道路交叉口交通流特性分析,建立和标定了城市道路路段行程时间计算模型和下游信号道路交叉口交通延误计算模型,其二者计算结果之和正是由道路路段及其下游信控交叉口构造而成的典型的城市道路路段车辆行程时间。
通过实例计算与分析,结果表明计算模型具有较好的适用性,计算结果具有较高的准确性。
最后,在运用城市道路路段行程时间计算模型得到现状道路路段行程时间后,为了精确的预测未来道路路段行程时间,本文研究分析了国外经典的行程时间计算模型,并通过实例计算,验证了模型准确性。
同时结合我国城市道路路段交通流相对复杂的特点,根据数据融合理论,建立了城市道路路段行程时间综合预测模型,利用改进的定长最小二乘法计算各模型权重,进而实现了道路路段行程时间的预测。
最后加以实例验证,证明了模型具有良好的适用性,计算结果具有较高的准确性。
关键词:行程时间;计算模型;预测模型;城市道路;交通流AbstractThe basic information of Traffic flow provide the basic support for the realization of the intelligent transportation system, it is the foundation of the intelligent transportation system. As one of the most direct and important parameters in traffic flow information, the calculation and prediction of travel time are always the key point of the research content. The precise calculation and prediction of travel time make it reasonable for travelers to choose the travel mode and path and it is also a prerequisite for traffic management to do traffic control and traffic guidance.First, this paper introduces the different methods and technology to get the basic data in traffic survey and designs the traffic survey scheme in a scientific and rational way combining with its own characteristics. The data that is filtrated in scientific way provides the foundation of subsequent model calibration and verification.Second, according to the calculation and prediction for vehicular link travel time on urban road segments as well as its downstream intersection, this paper chooses the typical road segments and signalized intersections in the urban road network and uses the scientific method to get basic traffic information such as video camera. Based on the analysis of traffic flow characteristics of the road and signalized intersections, this paper reestablishes and calibrates the travel time calculation model on road segments and the delay model at its downstream signalized intersections. Through the calculation and analysis of an example, the results show that the model has good applicability and accuracy.Finally, the paper gets the current travel time on Urban Road Segments in the use of road travel time calculation model. In order to predict the future travel time on urban road accurately, this paper analyzes the several foreign classic travel time calculation model, combined with the characteristics of urban road traffic flow which is complicated in our country and then establish a new comprehensive travel time prediction according to different road congestion. The new comprehensive travel time prediction model combines the advantages and applicability of the classic models and then it realized dynamic precision of the link travel time prediction in different traffic conditions with the addition of dynamic weighting function. At last, the paper proves that the model has good practicability and accuracy with the instance validation.Key Words: Travel time; Calculation model; Prediction model; Urban road; Traffic flow目录学位论文原创性声明和学位论文版权使用授权书 (I)摘要 (II)Abstract ................................................................................................................. I II 插图索引 . (VII)附表索引 (VIII)第1章绪论 (1)1.1研究背景及意义 (1)1.2国内外研究现状 (2)1.2.1 国外研究现状 (2)1.2.2 国内研究现状 (3)1.3研究内容及技术路线 (5)1.3.1 主要研究内容 (5)1.3.2 研究思路及技术路线 (6)第2章道路交通调查与数据处理 (7)2.1道路交通调查 (7)2.1.1 道路交通调查的分类及方法 (7)2.1.2 车辆行程时间调查 (7)2.1.3 道路交通量调查 (9)2.1.4道路交通调查方案设计 (10)2.2道路交通调查数据统计与处理 (11)2.2.1 道路交通调查数据统计 (11)2.2.2 异常数据判定与处理 (12)2.3本章小结 (15)第3章城市道路路段行程时间计算模型研究 (16)3.1概述 (16)3.2.路段上游车辆行程时间计算模型 (16)3.2.1 模型建立 (16)3.2.2 模型参数标定 (18)3.2.3 模型确定 (19)3.3路段下游信号交叉口交通延误计算模型 (19)3.3.1 HCM2010交通延误计算模型 (20)3.3.1 模型参数标定 (21)3.4城市道路路段总行程时间计算模型 (24)3.5模型计算与验证 (24)3.6本章小结 (26)第4章城市道路路段行程时间预测模型研究 (28)4.1经典行程时间预测方法研究 (28)4.1.1 历史趋势法 (28)4.1.2 非参数回归法 (28)4.1.3 卡尔曼滤波预测法 (29)4.1.4 时间序列法 (30)4.1.5 人工神经网络法 (31)4.1.6 行程时间预测模型方法总结 (33)4.2城市道路路段行程时间综合预测模型 (33)4.2.1 模型建立 (34)4.2.2 权重的确定 (35)4.3实例计算与验证 (38)4.3.1 ARMA时间序列预测模型验证 (38)4.3.2 BP神经网络预测模型验证 (39)4.3.3 综合预测模型验证 (40)4.3.4 预测结果误差分析 (41)4.4本章小结 (42)结论 (43)参考文献 (45)致谢 (49)附录A(攻读学位期间发表的学术论文目录) (50)插图索引图1.1 本文技术路线 (6)图2.1桃子湖路-牌楼路路段(由北往南)道路交通调查示意图 (10)图2.2 同一车辆经过两个不同拍摄点的视频图像 (11)图2.3 数据样本正态性检验 (13)图2.4异常值处理前观测道路路段交通量曲线 (15)图2.5异常值处理后观测道路路段交通量曲线 (15)图3.1 城市道路路段的构造及其车辆行程时间划分 (16)图3.2 关于β值的线性拟合曲线 (18)图3.3道路路段行程时间计算模型与BPR模型计算结果对比 (19)图3.4 不同A值计算结果误差曲线 (23)图3.5 天马路-牌楼路(由南往北)行程时间及误差对比曲线 (25)图3.6 牌楼路-桃子湖路(由南往北)行程时间及误差对比曲线 (25)图3.7 桃子湖路-新民路(由南往北)行程时间及误差对比曲线 (26)图4.1 时间序列模型预测流程图 (31)图4.2BP神经网络结构图 (31)图4.3BP神经网络算法流程图 (32)图4.4 综合预测模型预测流程图 (35)图4.5 时段长∆t划分示意图 (35)图4.6ARMA模型预测结果曲线 (39)图4.7ARMA模型预测误差曲线 (39)图4.8BP神经网络模型预测结果曲线 (39)图4.9BP神经网络模型预测误差曲线 (40)图4.10 预测模型动态误差曲线 (40)图4.11 预测模型权重曲线 (40)图4.12 综合模型预测结果曲线 (41)图4.13 综合模型预测误差曲线 (41)附表索引表3.1HCM2010模型道路交通延误计算表 (21)表3.2 不同A值下信控道路交叉口交通延误计算值 (22)表3.3不同A值计算结果误差比较 (23)表3.4 城市道路路段行程时间误差比较 (26)表4.1 各预测模型方法总结 (33)表4.2 模型预测评价指标 (42)第1章绪论1.1 研究背景及意义近年来,随着社会经济持续发展、城市化进程的加快以及机动车保有量的急速增长,使得人们对交通运输的需求迅速增加,城市道路交通系统承受着巨大的压力,道路交通问题成为了国家亟待解决的基础问题之一。
基于支持向量回归的行程时间预测算法

基于支持向量回归的行程时间预测算法作者:邱淳风王珊王超群来源:《计算机时代》2014年第04期摘要:作为交通规划、运营和通行能力评估的重要指标,行程时间的预测对出行者的路线和时间点的选择,以及交通规划部门的信号控制策略有着重要的实际意义。
对于高级交通诱导系统而言,行程时间预测是一项关键的研究内容。
现有行程时间预测方法较少,且预测误差较大。
为此,运用浮动车和微波雷达测速数据,提出了基于支持向量机解决行程时间预测的方法,并且与历史平均法进行了比较。
在杭州市高架路线上的实验结果表明,所提方法的预测精度大幅度超过了历史平均法。
关键词:支持向量机;行程时间;智能交通;历史平均中图分类号:TP391 文献标志码:A 文章编号:1006-8228(2014)04-40-03Abstract: As an important indicator of transportation planning, operations and capacity assessment, the forecasted travel time has important practical meaning for the choice of route and timing, as well as for traffic signal control strategy of transportation planning department. For advanced transportation guidance systems, it is a key issue to predict travel times between pairs of points of interest. There are few travel time prediction methods with high probability of prediction error. In this paper, the speed data returned from probe vehicles and microwave radars is used to predict travel times based on support vector regression(SVR), and the new algorithm is compared to the historical mean algorithm. The experimental results over elevatedroads in Hangzhou show that the SVR based algorithm significantly outperforms the historical mean algorithm.Key words: support vector machine; travel time; intelligent transportation; historical average0 引言行程时间是交通规划、运营和通行能力评估的重要指标。
基于SVM和Kalman滤波的BRT行程时间预测模型研究
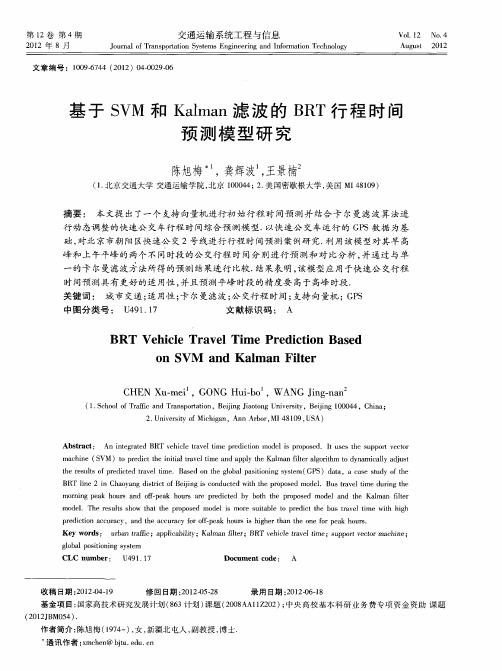
摘 要 : 本 文提 出 了一 个 支持 向量机进 行初 始行 程 时 间预 测 并 结合 卡 尔曼滤 波 算 法进
行 动 态调整 的 快速公 交车行 程 时 间综合预 测模 型 . 以快 速公 交车 运行 的 G S数 据 为基 P
时 间预 测 具有 更好 的适 用性 , 并且 预 测平峰 时段 的精度要 高 于高峰 时段 .
关 键词 : 城 市交通 ; 用性 ; 尔曼滤波 ; 交行程 时间 ; 适 卡 公 支持 向量机 ; P GS
中图分 类号 : U 9 . 7 4 1 1 文 献标 识码 : A
B T l e2 i C a y n i r t f e igi c n u t i epo oe d 1 u a e t ed r gte R n n h o a gds i in o d c dw t t rp sdmo e.B st v l i ui i tc o B j s e hh r m n h
( . col f rfca dTasot i , eigJ o n nvri , e ig10 4 ,C i ; 1 Sho o Ta i n rnpr t n B in i t gU i sy B in 0 04 hn f ao j ao e t j a 2 U i r t o c i n A nAb rMI 80 , S . n esy f hg , n ro, 19 U A) v i Mi a 4
BRT h c e Tr v lTi e Pr d c i n Ba e Ve i l a e m e i t s d o
- 1、下载文档前请自行甄别文档内容的完整性,平台不提供额外的编辑、内容补充、找答案等附加服务。
- 2、"仅部分预览"的文档,不可在线预览部分如存在完整性等问题,可反馈申请退款(可完整预览的文档不适用该条件!)。
- 3、如文档侵犯您的权益,请联系客服反馈,我们会尽快为您处理(人工客服工作时间:9:00-18:30)。
Pr d c i n o b n Ex e s y Tr v lTi e Ba e n S e i to f Ur a pr s wa a e m s d o VM
ZHANG u n,S Ja UN i n Ja
A b t a t W ih t e de eo sr c : t h v lpme to h b n Ex e s y T afc I fr to le to y t m , i a t・ n ft e Ur a pr swa r f no ma in Colc in S se i n p ri c a ,t e de eo ulr h v lpme to i e ie e p ae c pur y t m ,i i s i l o a c s e ltme y a c e — n fv d o lc ns l t a t e s se t spo sbe t c e s r a・i d n mi x prs wa r v ltme,a d a h a i e s y ta e i n tt e s me tme,t e de a d o h o ei a e e r h a d p a t la p iainso h m n fte r tc lr s a c n r ci p lc to f ca
第 1 卷 第2 1 期
21 0 1年 4 月
交 n p r t n S se n i e r g a d I f r t n T c n lg o r a f a s ot i y tms E gn e i n n o mai e h o o y T ao n o
选择预 测 时段 的前 4个 时段 的 数据作 为输 入 特征 值 , 以遗传 算 法建 立模 型 参数 优 化 算
法, 得到 训练模 型及 其参数 , 而 实现 车辆 行程 时 间的动 态预测 .最后 以上海 市快 速路 从 系统 中的 三个 典型路段 的 实测数 据进行 实例 分析 .结果表 明 : 传 统的 指数 平 滑法 、 与 多
hih a c r c r v ltm e p e ito sr ie g c u a y ta e i r dc in i a s d. Ba e n ma s f hso ia i e s re d t e e t d y i s d o s o itrc ltm ei s aa d tc e b l- c n e plt e o nto y tm n e p e s y,f u e dso a a b fr o e a tp ro r ee td a h e s ae r c g iin s se o x r s wa o rp do fd t eo e fr c s e id a e s lc e s te i u e t e . I r e o a h e e d na i r v ltm e p e cin, s p r e tr r ge so a hie i — np tfaurs n o d rt c i v y m c ta e i r dito up o v co e r s in m c n s a t d pe o t d,a d mo e rm ee so tmiain ag rt n d lpaa t r p i z to l oihm s ce td,t n tane d li ot n. F n ly,t e i r ae he r i d mo e sg te i al hre
元 回 归 法 、 R MA 法 预 测 结 果 对 比 , 于 S M 的 预 测 路 段 平 均 绝 对 百 分 误 差 在 5 以 A I 基 V %
内, 尔不 等 系数 非 常接 近 0 S M模 型显 示 了更 高的预 测精度 . 希 ,V 关 键词 : 交通工程 ; 行程 时间预测 ; 支持 向量机 ; 市快速路 城
摘要 : 随 着城 市快速路 交通信 息采 集 系统 的发展 , 别是视 频 车牌采 集 系统 的应 用 , 特
使 实 时 动 态 获 得 快 速 路 行 程 时 间 成 为 可 能 , 时 也 促 进 了 高 精 度 行 程 时 间 预 测 的 理 论 同
研 究和 实际应 用需求 .本 文基 于快 速路 车牌 识 别数 据检 测 的 海 量历 史 时 间序 列数 据 ,
V0 . 1 1l
Ap i rl
No 2 .
2 1 01
文 章 编 号 :1 0 .7 4( 0 1 20 7 - 0 9 6 4 2 1 )0 - 140 6
基 于 S M 的 城 市 快 速 路 行 程 时 间 预 测 研 究 V
张 娟, 剑 孙
( 同济 大 学 道 路 与 交 通 工 程 教 育 部 重 点 实 验 室 , 海 2 1 0 ) 上 0 84
t p c ls ci n r m a g a x e s y s se r h s n a d t e VM de s u e . Co p rd wi y ia e to sfo Sh n h ie prs wa y t ms a e c o e n h S mo li s d m ae t h
( yL b rtr fRo da d T af n iern Ke a oaoy o a n rf cE gn eig,Miit fE u ain,T n j Unvri i ns o d ct y r o o gi iesy,S a g a 010 t h nhi 2 8 4,C ia) hn